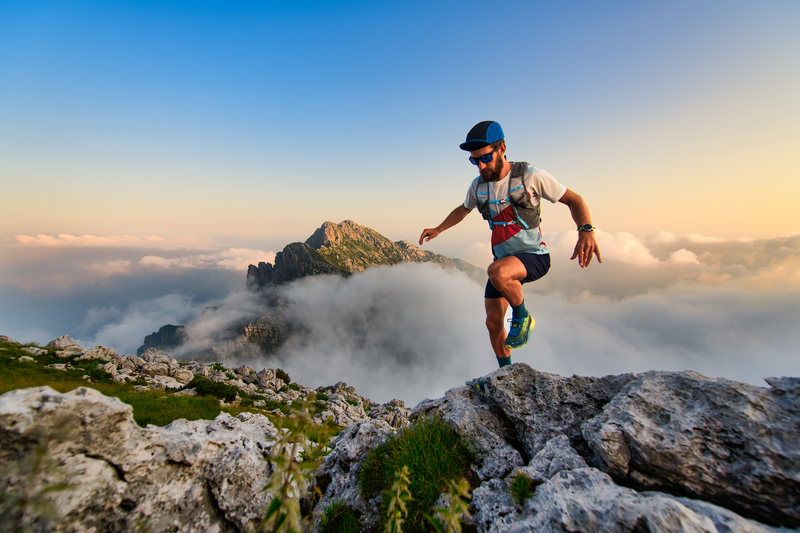
95% of researchers rate our articles as excellent or good
Learn more about the work of our research integrity team to safeguard the quality of each article we publish.
Find out more
ORIGINAL RESEARCH article
Front. Audiol. Otol. , 19 December 2024
Sec. Technology and Innovation in Auditory Implants and Hearing Aids
Volume 2 - 2024 | https://doi.org/10.3389/fauot.2024.1430992
This article is part of the Research Topic Advancing Audiology Practice and Knowledge at the World Congress of Audiology 2024: A Comprehensive Collection View all 12 articles
Introduction: Current hearing aids have an abundance of feature options and technologies. It is important to understand the clinical impact of hearing aid technology selection and how to individualize fittings to optimize hearing aid performance according to listening environment. To probe the naturalistic listening experiences researchers can use in-situ outcome measures. Survey-based real-world assessments can increase knowledge of hearing aid users' everyday scenarios, beyond the limits of lab-based scenarios. This study aimed to assess the relationship between subjective preference ratings of adult listeners and hearing aid technology level using Ecological Momentary Assessment (EMA). A secondary research question explored survey completion as a function of real-world participation in socially involved situations.
Methods: This study aimed to capture and assess in-the-moment listening situations and participant preference for hearing aid technology levels, using EMA through an app-based survey. Surveying was completed indoors (at home), indoors (away from home); and outdoors, and while in a listening situation with at least one communication partner. Fourteen older adults, aged 61–82 years, who were experienced bilateral hearing aids users were included in this study. Participants completed a 2-week acclimatization period wearing study-provided hearing aids, and a 2-week data collection period. In-situ surveying was used to evaluate technology-level preference in real-world listening situations with at least one communication partner. Survey data captured in-the-moment details surrounding environment, activity, and listening preference. Mixed methods were used to analyze the data, including Bayesian analyses for preference data and content analysis for text-based survey responses, including the use of the International Classification of Functioning, Disability and Health to guide activity categorization.
Results: Across a wide variety of categorized activities, participants demonstrated a preference for mid- to high-level hearing aid technologies when compared to the lowest level. Technology preference also varied according to reported activity location.
Discussion: The use of in-situ surveying provided a broader understanding of hearing aid users' listening environments when conversing with one or more communication partners and related technology preferences. EMA was found to be a feasible method of data collection for this cohort and may help guide clinical, person-centered selection of technology level.
Hearing aid technologies are traditionally evaluated using in-lab measures involving pre-recorded stimuli and sound treated rooms. These methods are effective in establishing performance benchmarks using standardized materials; however, resulting outcomes may not generalize to performance in real-world listening situations. When looking at the correlates of hearing aid use, research suggests that adult hearing aid users are likely to be involved in more social activities than nonusers (Sawyer et al., 2019). Furthermore, participation in socially involved listening situations (defined as a listening environment where the hearing aid user is involved in conversing with one or more people) is one of the most important use cases for hearing impaired listeners wearing hearing aids (Sawyer et al., 2019; Holman et al., 2021). Hearing aid users often report difficulties in challenging socially involved listening situations, including effects on social isolation and emotional wellbeing (Yadav et al., 2023). Social interactions generally involve one or more communication partners, with increased communication effort in the presence of background noise (Pasta et al., 2022). Recognizing situation-specific challenges can help address technology needs.
To better probe the naturalistic listening experiences from the user's perspective, researchers often incorporate outcome measurement options, such as real-world assessments involving the use of surveys, questionnaires, or interview-style follow-up. This allows for the exploration of everyday scenarios encountered by users of hearing aids in real life. With the abundance of feature options and technology levels available in current hearing aids, it is important to learn how to best individualize fittings, to optimize hearing aid performance for each listening environment, and to utilize hearing aid technology to the fullest.
Experience Sampling Methods (ESM) can be used to collect data surrounding unique real-world listening environments. One type of ESM that captures repeated sampling of participants' behaviors and experiences in real-world natural environments is known as Ecological Momentary Assessment (EMA; Schinkel-Bielefeld et al., 2020; Shiffman et al., 2008). EMA data can be collected in-the-moment via questionnaires delivered through paper-and-pencil survey formats or through electronic surveys, during or shortly after an activity of interest (often referred to as real-time sampling, or near-real-time sampling). Studies that use traditional survey methods are often subject to systematic errors in self-reporting resulting from memory decay, also known as recall-bias (Khare and Vedel, 2019). This memory decay occurs when participants are required to retroactively complete surveys that are not simultaneously occurring with the activity of interest. This bias is reduced by using EMA, which allows for the assessment of individual experiences in day-to-day life and in real-time, when using mobile devices to administer (Shiffman et al., 2008). In a recent study, in-situ self-reports collected through EMA methods were found to be more sensitive to measures of outcomes between different hearing aid listening conditions than retrospectively collected data (Wu et al., 2020). EMA is described as a feasible and valid research methodology to assess hearing aid users' individualized hearing experiences and can inform more responsive, personalized, and family-centered hearing care (Galvez et al., 2012; Glista et al., 2021; Timmer et al., 2017; Wu et al., 2015; Xu et al., 2020; Christensen et al., 2024a; Vercammen et al., 2023). While EMA can be used to collect information at certain times or upon detection of specific environmental parameters (e.g., through hearing-aid integrated applications), it is also commonly self-initiated, allowing listeners to decide when an event of interest takes place as the trigger to report on their experiences by manually accessing a survey (Holube et al., 2020). Additionally, EMA can provide the opportunity to describe experiences or environments using an open-text field.
Mobile device-based EMA methods have been used more recently to capture daily life listening experiences from individuals wearing hearing aids (Wu et al., 2020, 2015, 2023). One common type of evaluation completed using EMA is a preference assessment, which can be used to help assess an individual's understanding and provide clarity of their personal values, health care situations, treatment options, and likely outcomes through an iterative, cognitive process (Brennan and Strombom, 1998). Preference ratings are highly valued by clinicians as one of the most important factors in decision-making processes, especially when considering patient-centered care (Boisvert et al., 2017; Bridges et al., 2012). Additionally, being able to infer preference in real-world settings using EMA, may make clinical decision-making about hearing aid technology levels more relevant and contextualized for the hearing aid users. Outside of EMA methods and over the past decade, studies have begun to focus on end-user preferences for different technology levels, including a comparison of basic and premium hearing aids, evaluations with new hearing aid users, evaluations of audiological parameters such as noise reduction, brightness, and soft gain, and in field trial or lab-based environments (Pasta et al., 2022; Christensen et al., 2024a; Cox et al., 2016; Hausladen et al., 2022; Plyler et al., 2021; Saleh et al., 2022; Houmøller et al., 2023; von Gablenz et al., 2023). Several of these field trial studies used recall-based assessments, identifying that participants indicated varying levels of preference for premium hearing aids, from just over half, to a strong majority (Hausladen et al., 2022; Plyler et al., 2021; Saleh et al., 2022; Christensen et al., 2024b). In contrast, Cox et al. (2016) found no significant differences between hearing aid technology level preferences across four pairs of hearing aids from various brands. While there are studies evaluating technology levels and user preference, there remains a gap in the literature surrounding knowledge of users' in-the-moment preference for real-world scenarios, rather than retrospective evaluation or simulated environments, and evaluations of hearing aid technology levels beyond basic and premium. Additionally, no studies were found to capture the complexity of a listening situation through the use of pre-established categorization frameworks.
Hearing aid manufacturers spend considerable research and development resources creating new and updated iterations of their technology (referred to as platforms). These platforms are offered at different price points (often associated with technology level) that are differentiated by varying degrees of device performance. Typically, the latest product at the highest price point will include the newest features and offer the best performance. Products at a lower price point may not offer the newest features or will offer features with reduced performance capability. For example, the highest price point may include an automatic program that can characterize the listening situation based on acoustic classification and adjust the performance according to the demands of the situation. Such a product may have a fine resolution in acoustic classification (e.g., number of classes) and included the latest signal processing features. At lower price points the device may still include an automatic program, but with fewer situations that can be detected and may not include the most advanced signal processing features or reduced performance of the features that are available. Portfolios of hearing aid technology levels are often marketed to the consumer as successive “sophistication levels”, ranging from entry-level devices to premium, each of which provide a prescribed set of features or feature capabilities. More channel numbers or levels (i.e., feature potential) have been found to relate to more technologically advanced devices, with the potential to be fitted with a higher level of sophistication (Lansbergen and Dreschler, 2020). Innovations such as environmental adaptation and binaural data streaming may be embedded in higher-end devices, whereas more simplified technologies would be incorporated into basic-level devices, such as limited environmental classifications, fixed microphone directionality, and fewer compression channels (Hausladen et al., 2022; Plyler et al., 2021; Johnson et al., 2016). Although cost often plays a large contributing role in device-level selection, there may be perceptible differences in sound quality, listening difficulty/effort, and program options that may influence end-user decisions (Johnson et al., 2016). It is important to note that perceptual differences may be viewed as a benefit to some hearing aid users and as a barrier to use for others (Searchfield et al., 2024; Windle et al., 2023). As part of a holistic aural rehabilitation and fitting process, these functional differences may have a greater contribution than device cost in influencing the listener's overall experience with their hearing aids, including how they perceive daily life activities.
To better understand the activities that hearing aid users engage in as a function of real-world hearing aid use, we can use classification frameworks, such as the International Classification of Functioning, Disability, and Health (ICF) (World Health Organization, 2004) or the common sound scenarios (CoSS) (Wolters et al., 2016). These frameworks offer further information a common language for describing health-related states. For example, the ICF domain activities and participation offers classification information including (1) learning and applying knowledge; (2) general tasks and demands; (3) communication; (4) mobility; (5) self-care; (6) domestic life; (7) interpersonal interactions and relationships; (8) major life areas; and (9) community, social, and civic life. The CoSS can be used to categorize a listening environment into (1) main intention categories (speech communication, focused listening, and non-specific), (2) task categories (number of people, live or media device sounds, or monitoring surroundings and passive listening), and (3) the sound scenario (conversation at home, conversation on metro, meeting in office, car ride with family, phone call at home, mobile call in the street, lecture, at a concert, watching tv, listening to car radio, vacuum cleaning, city walk, relaxing with a book, relaxing on train). The ICF framework was selected to categorize the execution of a task of action, related to participation in conversation with listening partner(s), without overlapping with additional survey questions or evaluating listeners' intent. The use of the ICF classification framework for the purpose of collecting and distilling important details linking hearing aid users' technology preference with associated daily activities is a novel addition to field trial literature. The current study is part of a larger project investigating objective and subjective metrics of hearing aid technology level in socially involved situations. The primary objective of this study was to assess the correlation between subjective preference ratings of adult listeners and hearing aid technology level using in-the-moment EMA methods. Specifically, this study explored technology level (or maximum reported device performance by product price point) separate from automatic configuration depending on device classification of listening situation. Device-level preferences were evaluated in the context of participant location at the time of EMA surveys, as well as participant-reported background noise exposure. The secondary objective of this study was to investigate the open-text responses of the EMA survey using content analyses to guide systematic categorization of activities reported.
This study was approved by the Western University Research Ethics Board. All participants received details of the study in the form of an electronic letter of information and provided electronic written consent prior to participation. Fourteen participants, with an equal split of males and females between the ages of 61 and 82 years (M = 71.9; SD = 6.3), were recruited to participate in this study. One additional participant withdrew during the trial period of the study, prior to initiating data collection, due to health-related reasons, two participants were deemed ineligible to participate due to their hearing loss falling outside the fitting range of the devices used for the study. This study took place following the onset of the COVID-19 pandemic, with participant enrollment beginning in December 2021 through to September 2022. Study delays and participant recruitment challenges related primarily to public health restrictions and/or participant illnesses. Participants were recruited from a database held at the National Center for Audiology and using snowball sampling methods.
Participants were included in the study if they fulfilled the following criteria: (a) adult listeners (minimum 18 years of age); (b) those with self-reported frequent social interactions with one or more friends, family members, or acquaintances; (c) those who presented with symmetrical mild to severe hearing loss thresholds [with a four frequency pure-tone average (4PTA), calculated using 500, 1,000, 2,000, and 4,000 Hz values, not exceeding 70 dB HL, bilaterally; symmetry not exceeding 15 dB HL using the 4PTA], and (d) with at least 12 months' experience of hearing aid use (American Speech-Language-Hearing Association, 2020; Dawes and Munro, 2017; Yamada et al., 2017). Participant self-reported frequency of social interactions suggested that participants would be able to fulfill the required social interaction component of the study, reducing barriers to participate for those who may be more prone to social isolations. Air conduction audiometric assessment included pure-tone threshold measurement at octave and inter-octave frequencies between 250 and 8,000 Hz, in addition to otoscopy and middle ear analyses. Participants were also required to be able to use the technologies involved in the study on their own with a pre-trial demonstration, the hearing aids (including the program and volume toggle and the charging unit), and the Bluetooth functionality of the tablet. These capabilities were assessed by a member of the research team at the initial appointment.
Participants attended 2–3 appointments during this study, depending on whether the participant required additional hearing aid adjustments. During the first study appointment, participants underwent audiometric threshold measurements and real ear to coupler difference (RECD) measures. In the case that a recent clinical audiogram was available (i.e., within 6 months), the recent audiogram was then used as the basis for the hearing aid fitting. Participants were loaned Unitron Discover Next (DX) Moxi Move rechargeable hearing aids, fitted with medium power (M) receivers. Participants were fit with vented domes (n = 10), power domes (n = 2), or open domes (n = 1), and one participant was fit with a vented dome in one ear and an open dome in the other; dome selection (herein referred to as acoustic coupling) depended on (1) evidence-based best fit for their hearing loss, (2) previous experience, or (3) participant preference. On-ear hearing aid fitting included the application of the DSL v5.0 adult prescriptive method (Scollie et al., 2005), incorporating foam-tip RECD values and real-ear probe tube measurements using the Audioscan Verifit 2 (Version 4.24.4). Fine-tuning was completed using the Verifit 2 standard speech signal at input levels of 55, 65, and 75 dB SPL, and for tone bursts at 90 dB SPL to assess the maximum power output. Further adjustments were made to the hearing aids to address participant subjective reports. Fit-to-target deviations were within 5 dB root mean square error using 500, 1,000, 2,000, 4,000, and 6,000 Hz, for speech input levels at 65 dB SPL, aligning with recommendations for hearing aid fittings (Baker and Jenstad, 2017; Brennan et al., 2017; Dao et al., 2021). In addition, feedback optimization was enabled for three participants and frequency compression for seven participants. Frequency compression was activated to maximize audibility of calibrated/s/stimuli and verified using on-ear measurements (Scollie et al., 2016).
In studies evaluating hearing aid technology levels, it is often necessary to have the different technology levels represented in different pairs of hearing aids. For the purposes of this study, it was not feasible to provide the participants with three different pairs of hearing aids to fully represent the technology levels, where greater burden would have been placed on the participants to bring along additional hearing aids and swap out the devices during each listening environment evaluation. Instead, we created manual programs which emulated the best capability that could be delivered at each product price point. This was achieved through creating three user activated (manual) programs, removing features and reducing the performance capability of the remaining adaptive features in each consecutive manual program. It is acknowledged that the use of manual programs removes the benefits of an automatic program that can adjust the HI performance based on classification of the situation. Instead, this investigation focused on the subjective benefit of the emulated technology levels when each manual program was user activated and delivered the best performance that would be possible in that device (when running in a fully automatic mode).
The study worn hearing aids included the highest technology level of the Unitron Discover Next hearing instruments, with access to the automatic program and the specifically configured manual programs. The automatic program (termed SoundNav) automatically adjusts the strength of the signal processing features based on an environmental classification (i.e., conversations in quiet, small group, crowd, and noise; no conversation in quiet and noise; and music). For this study, hearing aid configuration included four programs (Table 1): Program 1 (P1) was set to SoundNav; Programs 2 through 4 (P2, P3, and P4) included manual programs that did not include the use of SoundNav. P1 functioned as the base fitting program in which individualized frequency gain adjustments were made; this was held constant across all manual programs. For the automatic program (P1), each signal processing feature's maximum strength varied based on environmental classification.
Table 1. Comparison of signal processing features and settings across programs representing varying technology levels.
For all programs, the amount of signal processing adjusted adaptively between a setting of zero and the maximum fitted strength. For noise reduction and speech enhancement, the amount of signal modification was adaptively adjusted based on the signal-to-noise-ratio (SNR) and the fitting strength of the feature. The microphone mode included a level dependent characteristic such that at average and lower input levels the microphone mode replicated a head related transfer function, while at higher levels the fitted strength determined the microphone mode (such as an adaptive directional beamformer).
The three manual programs under investigation were configured to offer the maximum signal processing of each feature representative of three different technology levels (highest, mid-level, and lowest). The manual program which emulated the highest technology level included the ability to binaurally and asymmetrically adjust several adaptive features (the beamformer, noise canceller, and speech enhancement) based on the location of a speech target in one of four quadrants around the listener (for SpeechPro, the device performance was asymmetrical for speech targets to the side). The other two manual programs which emulated lower price points did not include the ability to modify performance based on a speech target location and did not include any asymmetrical adjustments. The manual program which emulated the lowest technology level included a restricted beamformer setting which replicated an average head related transfer function.
To reduce the likelihood of bias, the automatic program was configured to perform similarly to a mid-level device. Specifically, in the automatic program, SpeechPro was disabled (the microphones were configured as a fixed wide directional beamform) and the adaptive signal processing features (speech enhancement and noise cancellation) were set to a mid-level strength (not the maximum that would be achieved in the highest-level technology product). For all manual programs, the following features were kept at default settings: Wind control (off), AntiShock2 (Moderate), Phase Canceller (Moderate). Feature modification included functionality for speech understanding, localization, and noise reduction.
Programs were preloaded into the hearing aids to limit the number of times the participants had to return for fitting modifications during COVID-19. Participants were instructed to stay in P1 during the acclimatization phase of the study, which also remained the default program throughout the study. P2, P3, and P4 were manually accessible using the multi-function button with program switching functionality (on the top of the hearing aid). Participant instructions for accessing programs P2 through P4 during the trial period included randomization (refer to Phase 2). The use of manual programs made it possible to provide the equivalence of three different hearing aids in the one device by replicating the feature settings that are commercially offered in the chosen technology level. Participants were single blinded to all aspects of hearing aid program feature settings. Participants were instructed to identify the different programs through the number of beeps presented when toggling between the programs (e.g., P2 beeped twice, P4 beeped four times).
Following the hearing aid fitting portion of the study, each participant began a two-phase real-world hearing aid trial. Phase 1 consisted of a 2-week acclimatization period allowing participants to become familiar with the new study-worn hearing aid fitting. If participants required additional fine-tuning or adjustments to their study hearing aids, they were instructed to contact the research team prior to beginning the next phase. Phase 2 included a data collection period, spanning a minimum of 2 weeks, and included the use of a proprietary EMA survey application, MobEval3, to survey contextual and perceptual information. This app was installed on loaner tablets (Asus ZenPad 8.0 or Samsung Galaxy Tab A7 Lite- 7″ or A8- 8″) that were provided to participants for the duration of the trial, along with a travel case. The tablets did not require internet access; all data was stored locally on each device. During the hearing aid fitting appointment, participants were given a personalized tutorial of the physical hearing aid features (volume/program switches/charging), tablet features (power and applications), and survey execution within the app. Participants practiced utilizing all features in the laboratory, with a member of the research team until they were comfortable with the technology, prior to starting the trial. Participants also received paper instructions outlining general hearing aid use guidelines and all study procedures; these instructions contained both written and visual representations of the steps involved. Paper instructions were presented to participants to refresh participants on study steps following the acclimatization period.
Following the 2 week acclimatization phase, participants were asked to complete EMA surveys using event-based monitoring (Shiffman et al., 2008), in alignment with three pre-identified listening situations over a 14-day period. In this study, participants were instructed to complete surveys when in a social listening situation with at least one communication partner. In addition, they were instructed to complete a minimum of three surveys in each of the following situations, for a total of nine surveys: (1) indoors (at home), (2) indoors (away from home), and (3) outdoors. If a participant chose to collect extra survey data, these data were included in the analyses. Each survey contained a series of short questions and ratings of their listening activity with paired comparisons of hearing aid program preference. Participants were instructed to stay in a listening situation for a minimum of 2 minutes prior to initiating an EMA survey. EMA responses were collected using a variety of question types, including multiple choice, text-based responses, and Likert response scales. A study checklist was provided to each participant to allow them to keep a record of the listening situations they had completed. EMA studies often include prompted survey entries using random or scheduled notifications; however, the research team felt that notifications would not align well to the pre-determined location types, therefore, this study allowed the participants to select the days and times in which they completed the surveys in situations of interest and in the pre-determined location types. Reporting of communication partner type was included as part of the EMA (refer to Section 3.3).
The EMA portion of this study first surveyed participant eligibility according to their in-the-moment involvement in a social situation (with one or more communication partners). In the case where a participant indicated they were not with at least one communication partner, the survey was terminated, and the participant was directed to repeat the survey in an appropriate situation. In the case where the participant indicated they were in an eligible social situation they were prompted to continue the survey.
The EMA survey next collected information to better describe the listening situation the participant was in. Participants were asked to categorize their listening location as either: indoors (at home), indoors (away from home), or outdoors. This was followed by a prompt to include a text-based description of the activity completed in the specified location. Participants were also asked to indicate how many people they were with, according to the following categories: a partner (1), a small group (2–3), or a large group (4 or more); this was followed by a prompt to enter a text-based description of the people/person they were with (provided examples included “my spouse”, “my two children”). In addition, participants were asked to indicate where their communication partner(s) was in relation to themselves (e.g., located to the front, to the side, or behind). To subjectively assess the presence and type of background noise, participants were asked if they could hear background sounds during the EMA survey; if they answered “yes,” they were then asked to describe the noise as either speech, music, or non-speech.
The next step of the EMA survey was to assess program preference. Depending on the testing condition, participants were asked to toggle to their first evaluation program (P2, P3, or P4), and were then prompted to toggle between the remaining programs to make their evaluations. They were asked to be in the chosen listening situation for at least 2 min and to indicate a preferred program or no preference according to blocks of program pairs (e.g., P4 vs. P2, P2 vs. P3, and P3 vs. P4); these were presented as multiple-choice questions; evaluation order varied between the five testing conditions. All surveys included the same questions; however, the order of preference testing differed; individual program ratings were set to occur in different orders (testing order could have included P3–P4–P2, as one example). Using block randomization, participants were assigned to one of five pre-determined EMA testing orders.
Data from the MobEval3 application were stored locally on each tablet and exported upon each participant's completion of Phase 2. Survey data were analyzed using mixed methods with the application of descriptive and statistical analysis to draw quantitative inference and content analyses to describe qualitative data.
An content analysis approach was used to guide a systematic categorization scheme for the open text-based responses reported as part of the EMA (Huxley, 2020). Activity-based responses were categorized in alignment with the World Health Organization's International Classification of Functioning, Disability and Health (ICF) using the Activities and Participation categories (World Health Organization, 2004); this provided a description of the types of listening activities across location types, in which the preference data was collected. The ICF categories are separated according to broad coding, first level, and narrowed down to second- and third-level coding. A member of the research team coded each text-based response, verified by a second member of the team. Coding applied the ICF in a systematic manner, including categorizing activities into the nine first level codes. For example, the activity “having supper with my spouse” was categorized as Self-Care (ICF D5), Domestic Life (ICF D6), and as Communication (ICF D3). A second-level item coding method was used, as applicable, for each written entry in the EMA based on participant involvement in a life situation (World Health Organization, 2004). For our example, Self-Care was categorized into Eating (ICF D550) and Domestic Life was categorized into Other Specified Domestic Life such as discussing day's appointments with spouse. A common component of the ICF is the evaluation of capacity and performance; this evaluation was beyond the scope of the current study. Communication-partner-based responses were categorized according to perceived familiarity (immediate family, extended family or friends, acquaintances or groups, and strangers or tradespeople); one text-based entry could have multiple categories of communication partners.
Quantitative data analyses were conducted with a generalized linear mixed effects regression model under a Bayesian framework. This Bayesian framework estimates the joint probability distribution of generative model parameters (Leijon et al., 2023). Our model implemented an extension of the Bradley-Terry model to allow for ties in preference ratings (i.e., no preference) to model paired-comparison preference (Critchlow and Flinger, 1991). We included contrast-coded programs (e.g., P3 and P4), location, background noise type, and the interaction between location and background noise type as population-level effects with varying effects for participants. The model was constructed using the Stan programming language (Carpenter et al., 2017) through the cmdstanr package (Gabry and Cešnovar, 2021) in R statistical computing software (R Core Team, 2022).
The model estimated a posterior distribution of the probability of preference rating for each combination of participant, location, and background noise type for each Markov chain Monte Carlo (MCMC) iteration (Gallagher et al., 2009). To derive a single estimate of preference ratings for each participant, location, and background noise type, a sum-score was calculated for each MCMC iteration as follows:
where p(k) is the probability of each preference rating. A sum-score represents the means of the probability distributions for each response choice, i.e., the central tendency of the response distributions.
A total of 14 individuals participated in this study. Participants presented with mild to moderately severe symmetrical hearing losses according to better ear 4PTA values (M = 47, SD = 7.3). The range in group-level pure-tone audiometric thresholds, as well as group-level mean thresholds per ear, are displayed in Figure 1. Between-ear symmetry ranged from 0 to 15 dB HL, across all participants. Audiometric thresholds were measured using a GSI AudioStar Pro audiometer with ER-3A insert earphones coupled to foam tips; middle ear analyses were completed using a Titan middle ear system as a screen for normal middle ear function.
Participants reported daily social interactions (71%) or frequent interactions (29%), as defined by their report of interaction within the last 3 days. Many participants reported living with one person (50%), living alone (14%), or living with two or more people (7%); the remaining participants did not report their living situation (29%).
Thirteen participants reported owning receiver-in-the-canal (RIC) hearing aids of various makes/models outside of this study (one participant was unable to report this information). All participants were experienced hearing aids users, with at least 1 year of hearing aid experience. Twelve participants reported wearing hearing aids for at least 8 h per day, in their daily life, with the remaining two reporting 6 h or less of hearing aid use.
When asked to report on mobile device ownership and usage, most participants reported owning a tablet (n = 12), smartphone (n = 12), and/or laptop/desktop (n = 13), with variable ratings of smartphone or tablet comfort level [novice (n = 1), average (n = 7), above average (n = 5), and expert (n = 1)]. Ten participants reported using these devices every day, in situations both inside and away from their home. When asked how they felt about using new mobile applications (apps), eight reported that they would be comfortable using apps on their own and two indicated that they would need help.
Participants were asked to complete nine surveys each over their 2-week period, for a total of 126 surveys. In fact, participants completed 128 surveys (102% compliance; Table 2). On one occasion a participant initiated a survey and indicated they were in a listening situation without a communication partner, hence the survey self-terminated and is not included in the analysis, Individual participant compliance rates varied from 66 to 188% across all situations. Six participants completed the minimum required location-specific surveys for each location; one participant only completed EMA surveys indoors (at home). Participants had the highest compliance in completing surveys indoors (at home), with only one participant not completing the minimum three surveys; compliance was lowest for indoors (away from home), with six participants not completing the minimum surveys. The group average compliance rate was 121% compliance indoors (at home), 86% compliance indoors (away from home), and 98% compliance in outdoor situations. Participants took an average of 17 days to complete Phase 2 (SD = 9.16). Hearing aid data logging was not used to measure compliance; the Log-it-All feature (i.e., data logging) in the hearing aids is only capable of capturing the cumulative average percentage of time in each environment between fitting appointments and could not have been used to capture details around hearing aid usage per program, per day, or down to the exact time stamps required to evaluate compliance. According to the data collected as part of the EMA survey, the average completion time of the survey was 7:47 min (SD = 4:07); this first included an average of 3:20 min of general questions used to describe the listening situation (i.e., location, communication partner, and type of background noise), completed as part of the block-randomization. Participants first assigned to P2 completed the general questions in an average time of 3:08 min, 3:16 min (P3), and 3:52 min (P4). Time stamps that related to the program switching notification in the survey were used to estimate how long each participant was in each program for. Participants were in P2 for an average of 1:20 min (SD = 0:38), P3 for 1:15 min (SD = 0:38), and P4 for 0:55 min (SD = 0:39).
As all open-text responses contained communication aspects and at least one communication partner, all data points were coded as a communication activity (ICF: d3). The following Activity and Participation domains applied to the dataset: learning and applying knowledge (ICF: d1); mobility (ICF: d4); self-care (ICF: d5); domestic life (ICF: d6); interpersonal interactions and relationships (ICF: d7); major life areas (ICF: d8); and community, social, and civic life (ICF: d9). Table 3 outlines the frequency of reported activities according to ICF second-level coding and according to participant-reported listening situation; sample statements and communication partners are listed for each category. Communication partners were described using open-ended responses and categorized according to immediate family (68%), extended family/friends (23%), acquaintances/groups (9%), strangers/tradespeople (5%), and pets (2%).
Table 3. Listening activities by location and sample communication partner, according to ICF categorization.
Data from the 128 completed EMA surveys were analyzed to better describe the listening situations experienced during the project by this group of participants, as outlined in Table 4. EMA surveys were completed in all three locations with 40% of all surveys completed indoors (at home), 28% indoors (away from home), and 32% outdoors. Participants were in a variety of listening situations, with most participants in situations with one listening partner (71%), followed by a large group (four or more people; 16%), and least often in situations with a small group (2–3 people; 13%).
Analyses according to type of background noise, including whether the participant classified the noise as non-speech, speech, music, or quiet, and the frequency of the noise (constant vs. intermittent noise), yielded the following EMA results: background noise as constant non-speech (31%), constant speech (22%), or quiet/no noise (21%), with fewer instances of intermittent non-speech (17%), intermittent speech (5%), and fewest situations in constant music (4%). Table 4 displays results according to background noise type, collapsing noise frequency categorization.
Participants' subjective listening preference across the three technology levels were calculated using sum-scores for each combination of program comparison, location, and background noise type (Figure 2). Points represent the median preference rating, error bars depict the 89% highest density credible interval (CI), and asterisks indicate statistically significant preferences (Kruschke, 2014). In most cases, the two higher technology levels (P2 and P3) were preferred over the lowest technology level (P4). In outdoor situations, the highest technology level, P2, was preferred compared to P4 only in speech background noise. P2 was reported as the preferred program over both lower technology levels (P3 and P4) in the indoors when away from home, when the background noise type was speech, which is likely the most challenging situation for listeners to participate in communication. No significant differences were found for preference when in the presence of music. Results from linear mixed effect regression analyses are outlined in Supplementary material.
Figure 2. Paired comparisons for preference according to location and background noise type. *Indicates significance.
This study presents findings specific to EMA data collected during real-world, real-time situations and in socially involved listening situations (i.e., including at least one communication partner). Findings suggest that real-world data collection by adults across various socially involved listening situations is a viable way to collect preference ratings, and that some activities may yield higher data collection patterns. Participants were asked to complete surveys while in a situation that involved conversation in one of three pre-defined listening situations: indoors (at home), indoors (away from home), and outdoors.
In alignment with the current literature, the participants' subjective preference ratings were evaluated using repeated measurement using real-time in-the-moment EMA surveying in complex listening situations, allowing for the evaluation of technology using identical measurement processes (Christensen et al., 2024a,b). We also asked our listeners to report on the listening conditions under which the self-reports were made according to classification of real-time (a) location (indoors at home, indoors away from home, and outdoors), (b) background (quiet, non-speech noise, speech noise), and (c) communication partner(s) during data collection. We also asked participants to contribute open-text responses to describe situation-specific activities that they were engaged in while completing the EMA surveys; these were analyzed using content analysis. Previous studies evaluating listener preference for basic vs. premium hearing aid technology level have included the use of back-to-back hearing aid trial periods to evaluate fitting differences within and across hearing aid manufacturers (Cox et al., 2016; Hausladen et al., 2022; Plyler et al., 2021). In comparison, our study incorporated a shortened trial period when evaluating signal processing features differences within one device, using hearing aid programs that exemplified the technology difference of interest.
More recent studies have incorporated smartphone-based EMA systems to inform research designs. For example, EMA methods have been evaluated as part of auditory lifestyle research (Xu et al., 2020; Wu et al., 2018), to compare hearing aid technologies (e.g., traditional vs. advanced noise reduction systems), describing listening experiences as part of daily-life situations (Christensen et al., 2024a,b), and to compare outcomes of different hearing aid technologies using in-situ self-report applications (Wu et al., 2020). In addition, EMA methods used to evaluate real-world effectiveness of advanced digital noise reduction have evaluated different dimensions of listener experience, including satisfaction, as part of larger lab and real-world test batteries (Wu et al., 2019). The methods reported in this study incorporated a selection of previously reported EMA methods including the assessment of auditory lifestyle through mobile-based, real-time surveying, to facilitate in-situ measurement of listener preference for technology levels. Multiple paired comparisons were used to evaluate three levels of hearing aid signal processing features to gain a rich understanding of listener preference in the context of clinically relevant technology level options. The inclusion of real-world, in-the-moment preference ratings via EMA methods provide insight into hearing aid user technology preference, assessed as part of socially involved listening situations. Data collection methods used in this study therefore aimed to reduce participants' recall-bias and potential data collection burden, with the collection of multiple preference ratings built into one real-world hearing aid trial.
A total of 128 EMA surveys with data aligning with each of the pre-defined listening situations were captured. The requirement for participants to have completed EMA surveys with at least one listening partner present may have contributed to the lower frequency of data collected for quiet listening situations, paired with the high occurrence of non-speech categorized background noise. Overall, data collection was lowest for outdoor situations, when participants were experiencing background noise reported as quiet (3%) or speech (7%). Further investigation is needed to explore technology level preferences in outdoor situations and without social distancing restrictions.
High EMA compliance rates were recorded as part of this study, indicating effective completion of in-the-moment EMA surveys accessed through a tablet as part of the participants' daily lives. The highest number of EMA surveys were reported to be completed during participation in activities related to communication within their community, social, and civic life that took place indoors (away from home), or in outdoor situations. The second highest reported ICF category included activities related to domestic life; EMA responses collected during these activities were commonly reported indoors at home when learning or applying knowledge, or when outdoors (often associated with activities within the home's property). The prevalence of home-based findings may be attributed to the data collection timeline occurring during the COVID-19 pandemic, with several provincial lockdowns occurring during data collection and the participants' comfort level and/or ability to participate in social activities away from the home. The least often reported listening situations related to those experienced as part of major life areas, including education, work, and employment. This likely related to the participants' ages, as most were retired, or may have related to participant hesitance to complete the EMA survey during work-based activities. The findings from this study can therefore be generalized to a variety of listening situations, inside and outside of the home, and as experienced by a group of adult Canadian listeners that were socially engaged during COVID-19.
Participants reported a preference for both premium and mid-level technology levels, when compared to the basic technology level, for indoor listening environments (at home and away from home), and in environments that included background noise categorized as quiet, non-speech, and speech; this trend in preference was also reported when in outdoor listening environments with background speech noise. Our application of EMA used multiple response formats, including open-text options to allow the listener to describe listening situation-specific activities in greater details. This EMA application has been reported to provide real-time insights into common themes linked to hearing aid use and/or challenges (Vercammen et al., 2023). In our study, commonly reported indoor activities included conversations during meal consumption, when watching TV, and when visiting with family members, whereas when outdoors, commonly reported activities included conversations while relaxing outside or while completing seasonal chores. A preference for the premium-level technology, over the mid- and basic-level technology, was indicated when listeners were located indoors-outside home, when background noise was specific to speech. When participants were located indoors-outside of the home they commonly reported dining in restaurants and participating in other group-based activities, such as sporting events, and educational classes.
Findings from this study suggest that subjective preference ratings for hearing aid technology level were influenced by both the location of the listener, as well as the type of background noise present, with a preference for mid-level and premium technologies, compared to basic technology, across most socially involved listening situations experienced as part of the study. These findings likely relate to differences in the settings and features activated across technology levels (refer to Table 1) that aim to optimize listening in conversation, especially when in the presence of noise. For example, the strength of the sound optimization setting increased from the basic to the premium technology level within this study, which may have contributed variations in reported preference ratings associated with speech understanding, comfort, and/or sound quality listening dimensions. In addition, the premium level technology included an adaptive speech-finding beamformer feature called SpeechPro (dynamic location of target speech signal, including noise cancellation and speech enhancement), which may have contributed to higher perceived listening abilities when in conversations in noise and/or crowds. Plyler et al. (2021), also examined post-trial preference for similar premium vs. basic hearing aid features using recall-based investigator interviews, and reported at the group level these results were not found to be significant. The authors concluded that examination of the laboratory and field trial results revealed that listeners in highly-demanding listening environments performed comparably or better with the premium devices (Plyler et al., 2021). Participants in the current study were engaged in complex and varied environments when completing preference ratings, which included conversation with one or more communication partners and in the presence of speech (27%) or non-speech (48%) background noise as part of the activity. In these contexts, Participants reported preference for the two higher technology levels (P2 and P3) over the lowest technology level (P4); with a greater preference, across locations, for P2 over P4. This study allowed participants to directly compare technologies within the same environment, as part of their daily life.
One limitation of survey-based studies is the inability to confirm whether participants completed data collection in the self-reported activity at the logged times (Shiffman et al., 2008). Time stamps of the EMA data suggest that surveys were not completed at scheduled times or at consistent intervals. Participants completed 27% of the surveys in the morning (between 6:00 and 11:59 a.m.), 30% in the afternoon (12:00–4:59 p.m.), and 56% in the evening (4:59 p.m. or later). In addition, compliance rates were recorded to be highest when EMA data was collected in the home and dropped considerably when compared to EMA data collection completed indoors-outside of the home. This may be an indication of the participant's ability to complete, or their comfort around completing, survey-based tasks outside of a more contrived situation, such as their home. Five surveys were initiated with no responses selected throughout; this small number of incomplete surveys may indicate that participants did not feel the survey was too long. It is not possible to know the number of situations that may have been of interest where the participant did not feel comfortable taking the time to complete a survey. To improve participant convenience, future studies could include the use of smaller mobile devices to collect survey data, such as a smartphone, the use of shorter surveys to collect information around situation suitability may also provide more accurate information (e.g., one or two questions that require a quick answer). Future research should consider how we can better capture perceptions in rapidly-changing listening environments as well as provide clear instructions on how to manage in-situ surveying in challenging social situations.
Outside of the devices used in this study, one manufacturer's interpretation of what a premium product is comprised of may not be the same as the next. In this study, the listeners reported a preference for high and medium technology levels, when compared to the basic level. This may not be the case across all manufacturers depending on feature composition per technology level. Future research is needed to validate whether these findings generalize across other manufacturers' product lines.
This study is part of a larger study that aims to pair objective and subjective metrics of hearing aid technology level in socially involved situations. When considering the subjective results, results indicate that adult hearing aid users can reliably complete EMA survey tasks in real-world scenarios related to location, activity classification, and preference; however, further research aimed at optimizing EMA data collection methods is warranted. The findings from this study can be applied to future research and clinical application to inform in-the-moment performance with pre-determined hearing aid program comparisons and/or targeted listening situations. Technology levels including mid to premium features resulted in higher preference ratings when evaluated in socially involved listening situations. Including an assessment of hearing aid users' activity profile, and information related to the presence of communication partners, may help inform technology selection; this type of assessment information may also inform the use of individualized programs. In the research context of this study, listeners were willing to complete EMA surveys comparing manual programs; this application of EMA methods could translate to clinical practice for evaluation of program preference or other technology level comparisons.
Future research is aimed at exploring the relationship between the objective data captured by the hearing aid classifier in the study-provided hearing aids, with the subjective participant responses obtained during the EMA surveys. This will yield information related to hearing aid classification by technology level which could be used for individualization of device configuration and settings. Furthermore, EMA can be used as a way of determining benefit (or lack thereof) resulting from automatic hearing aid classification, guiding future advancements around the functionality and adjustment of hearing aid technology in different listening environments.
The original contributions presented in the study are included in the article/Supplementary material, further inquiries can be directed to the corresponding author.
This study, involving humans, was approved by the Western University Health Sciences Research Ethics Board. The study was conducted in accordance with local legislation and institutional requirements. The participants provided their written informed consent to participate in this study.
DG: Conceptualization, Data curation, Formal analysis, Funding acquisition, Investigation, Methodology, Project administration, Resources, Supervision, Validation, Visualization, Writing – original draft, Writing – review & editing. RO: Conceptualization, Data curation, Formal analysis, Investigation, Methodology, Project administration, Resources, Validation, Visualization, Writing – original draft, Writing – review & editing. KB: Data curation, Investigation, Resources, Writing – review & editing. JC: Data curation, Formal analysis, Resources, Supervision, Validation, Visualization, Writing – original draft, Writing – review & editing. SS: Conceptualization, Funding acquisition, Project administration, Resources, Writing – review & editing. LC: Conceptualization, Data curation, Formal analysis, Funding acquisition, Methodology, Resources, Validation, Writing – original draft, Writing – review & editing.
The author(s) declare financial support was received for the research, authorship, and/or publication of this article. This study was funded by an Ontario Research Fund Research Excellence Award (ORF RE08-072), with collaboration by four Ontario-based companies including Unitron. The collaboration is governed by a Collaborative Research Agreement that was developed through Western's office of Research Services, and has appropriate protections for data, intellectual property, student right to publish and graduate, and other key elements of ethical collaboration with industry.
The authors would like to thank Paula Folkeard, Shruthi Sundararaman, and Christina Boakye-Gyan for their assistance with various aspects of data management/visualization support and Prudence Allen who is co-PI of the grant that supported this work.
LC is an employee of Unitron (Sonova) and supported the supply of hearing instruments and software that were used in this study.
The remaining authors declare that the research was conducted in the absence of any commercial or financial relationships that could be construed as a potential conflict of interest.
All claims expressed in this article are solely those of the authors and do not necessarily represent those of their affiliated organizations, or those of the publisher, the editors and the reviewers. Any product that may be evaluated in this article, or claim that may be made by its manufacturer, is not guaranteed or endorsed by the publisher.
The Supplementary Material for this article can be found online at: https://www.frontiersin.org/articles/10.3389/fauot.2024.1430992/full#supplementary-material
4PTA, Four frequency pure-tone average; CI, Credible interval; Db HL, Decibels Hearing Level; DB SPL, Decibels Sound pressure level; EMA, Ecological Momentary Assessment; ELPD, Expected log predictive density; ESM, Experience Sampling Methods; ICF, International Classification of Functioning, Disability and Health; MCMC, Markov chain Monte Carlo; P, Program; PSIS-LOO, Pareto smoothed importance sampling leave-one-out cross-validation; RECD, Real ear to coupler difference; RIC, Receiver-in-the-canal; SE, Standard error.
American Speech-Language-Hearing Association (2020). American Speech-Language-Hearing Association. Degree of Hearing Loss. Available from: https://www.asha.org/public/hearing/Degree-of-Hearing-Loss/ (accessed January 14, 2020).
Baker, S., and Jenstad, L. (2017). Matching real-ear targets for adult hearing aid fittings: NAL-NL1 and DSL v5.0 prescriptive formulae. Can. J. Speech Lang. Pathol. Audio.l. 41:9. Available at: https://cjslpa.ca/files/2017_CJSLPA_Vol_41/No_02/CJSLPA_Vol_41_No_2_2017_Baker_Jenstad_227-235.pdf
Boisvert, I., Clemesha, J., Lundmark, E., Crome, E., Barr, C., McMahon, C. M., et al. (2017). Decision-making in audiology: balancing evidence-based practice and patient-centered care. Trends Hear. 21:233121651770639. doi: 10.1177/2331216517706397
Brennan, M. A., Lewis, D., McCreery, R., Kopun, J., and Alexander, J. M. (2017). Listening effort and speech recognition with frequency compression amplification for children and adults with hearing loss. J. Am. Acad. Audiol. 28, 823–837. doi: 10.3766/jaaa.16158
Brennan, P. F., and Strombom, I. (1998). Improving health care by understanding patient preferences: the role of computer technology. J. Am. Med. Inform. Assoc. 5, 257–262. doi: 10.1136/jamia.1998.0050257
Bridges, J. F. P., Lataille, A. T., Buttorf, C., White, S., and NIparko, J. K. (2012). Consumer preferences for hearing aid attributes: a comparison of rating and conjoint analysis methods. Trends Amplif. 16, 40–48. doi: 10.1177/1084713811434617
Carpenter, B., Gelman, A., Hoffman, M. D., Lee, D., Goodrich, B., Betancourt, M., et al. (2017). Stan : a probabilistic programming language. J. Stat. Softw. 76:i01. doi: 10.18637/jss.v076.i01
Christensen, J. H., Rumley, J., Gil-Carvajal, J. C., Whiston, H., Lough, M., Saunders, G. H., et al. (2024b). Predicting individual hearing-aid preference from self-reported listening experiences in daily life. Ear Hear. 45, 1313–1325. doi: 10.1097/AUD.0000000000001520
Christensen, J. H., Whiston, H., Lough, M., Gil-Carvajal, J. C., Rumley, J., and Saunders, G. H. (2024a). Evaluating real-world benefits of hearing aids with deep neural network–based noise reduction: an ecological momentary assessment study. Am. J. Audiol. 33, 242–253. doi: 10.1044/2023_AJA-23-00149
Cox, R. M., Johnson, J. A., and Xu, J. (2016). Impact of hearing aid technology on outcomes in daily life I: the patients' perspective. Ear Hear. 37, 224–237. doi: 10.1097/AUD.0000000000000277
Critchlow, D., and Flinger, M. (1991). Paired comparison, triple comparison, and ranking experiments as generalized linear models, and their implementation on GLIM. Psychometrika 56, 517–533. doi: 10.1007/BF02294488
Dao, A., Folkeard, P., Baker, S., Pumford, J., and Scollie, S. (2021). Fit-to-targets and aided speech intelligibility index values for hearing aids fitted to the DSL v5-adult prescription. J. Am. Acad. Audiol. 32, 090–098. doi: 10.1055/s-0040-1718707
Dawes, P., and Munro, K. J. (2017). Auditory distraction and acclimatization to hearing aids. Ear. Hear. 38, 174–183. doi: 10.1097/AUD.0000000000000366
Gabry, J., and Cešnovar, R. R. (2021). Interface to CmdStan. Available at: https://mc-stan.org/cmdstanr/ (accessed March, 6, 2023).
Gallagher, K., Charvin, K., Nielsen, S., Sambridge, M., and Stephenson, J. (2009). Markov chain Monte Carlo (MCMC) sampling methods to determine optimal models, model resolution and model choice for Earth Science problems. Mar. Pet. Geol. 26, 525–535. doi: 10.1016/j.marpetgeo.2009.01.003
Galvez, G., Turbin, M. B., Thielman, E. J., Istvan, J. A., Andrews, J. A., Henry, J. A., et al. (2012). Feasibility of ecological momentary assessment of hearing difficulties encountered by hearing aid users. Ear Hear. 33, 497–507. doi: 10.1097/AUD.0b013e3182498c41
Glista, D., O'Hagan, R., Van Eeckhoutte, M., Lai, Y., and Scollie, S. (2021). The use of ecological momentary assessment to evaluate real-world aided outcomes with children. Int. J. Audiol. 60, S68–78. doi: 10.1080/14992027.2021.1881629
Hausladen, J., Plyler, P. N., Clausen, B., Fincher, A., Norris, S., Russell, T., et al. (2022). Effect of hearing aid technology level on new hearing aid users. J. Am. Acad. Audiol. 33, 149–157. doi: 10.1055/a-1673-9989
Holman, J. A., Drummond, A., and Naylor, G. (2021). Hearing aids reduce daily-life fatigue and increase social activity: a longitudinal study. Trends Hear. 25, 23312165211052786. doi: 10.1177/23312165211052786
Holube, I., von Gablenz, P., and Bitzer, J. (2020). Ecological Momentary Assessment in hearing research: current state, challenges, and future directions. Ear Hear. 41(Suppl. 1), 79S−90S. doi: 10.1097/AUD.0000000000000934
Houmøller, S. S., Wolff, A., Tsai, L. T., Narayanan, S. K., Hougaard, D. D., Gaihede, M. L., et al. (2023). Impact of hearing aid technology level at first-fit on self-reported outcomes in patients with presbycusis: a randomized controlled trial. Front Aging. 4:1158272. doi: 10.3389/fragi.2023.1158272
Howard, T. (2014). Putting the Focus on Conversations. Unitron. Available at: https://www.unitron.com/content/dam/unitron-2014/documents/english/moxi-north/whitepaper/027-5892-02_Classifier_SoundNav_Whitepaper_ENG.pdf (accessed August 28, 2023).
Huxley, K. (2020). Content analysis, quantitative. In: Atkinson P, Delamont S, Cernat A, Sakshaug JW, Williams RA, editors. SAGE Research Methods Foundations. SAGE Publications Ltd. Available at: https://methods.sagepub.com/foundations/content-analysis-quantitative (accessed October 22, 2024).
Johnson, J. A., Xu, J., and Cox, R. M. (2016). Impact of hearing aid technology on outcomes in daily life II: speech understanding and listening effort. Ear Hear. 37, 529–540. doi: 10.1097/AUD.0000000000000327
Khare, S. R., and Vedel, I. (2019). Recall bias and reduction measures: an example in primary health care service utilization. Fam. Pract. 36, 672–676. doi: 10.1093/fampra/cmz042
Kruschke, J. (2014). Doing Bayesian Data Analysis: A Tutorial with R, JAGS, and Stan. 2nd Edn. London: Academic Press, 673.
Lansbergen, S., and Dreschler, W. A. (2020). Classification of hearing aids into feature profiles using hierarchical latent class analysis applied to a large dataset of hearing aids. Ear Hear. 41, 1619–1634. doi: 10.1097/AUD.0000000000000410
Leijon, A., von Gablenz, P., Holube, I., Taghia, J., and Smeds, K. (2023). Bayesian analysis of Ecological Momentary Assessment (EMA) data collected in adults before and after hearing rehabilitation. Front. Digit. Health 5:1100705. doi: 10.3389/fdgth.2023.1100705
Pasta, A., Petersen, M. K., Jensen, K. J., Pontoppidan, N. H., Larsen, J. E., Christensen, J. H., et al. (2022). Measuring and modeling context-dependent preferences for hearing aid settings. User Model. User Adapt. Interact. 32, 977–998. doi: 10.1007/s11257-022-09324-z
Plyler, P. N., Hausladen, J., Capps, M., and Cox, M. A. (2021). Effect of hearing aid technology level and individual characteristics on listener outcome measures. J. Speech Lang. Hear. Res. 64, 3317–3329. doi: 10.1044/2021_JSLHR-21-00111
R Core Team (2022). R: A Language and Environment for Statistical Computing. Vienna: R Foundation for Statistical Computing. Available at: https://www.R-project.org/ (accessed March 6, 2023).
Saleh, H. K., Folkeard, P., Van Eeckhoutte, M., and Scollie, S. (2022). Premium versus entry-level hearing aids: using group concept mapping to investigate the drivers of preference. Int. J. Audiol. 61, 1003–1017. doi: 10.1080/14992027.2021.2009923
Sawyer, C. S., Armitage, C. J., Munro, K. J., Singh, G., and Dawes, P. D. (2019). Correlates of hearing aid use in UK adults: self-reported hearing difficulties, social participation, living situation, health, and demographics. Ear Hear. 40, 1061–1068. doi: 10.1097/AUD.0000000000000695
Schinkel-Bielefeld, N., Kunz, P., Zutz, A., and Buder, B. (2020). Evaluation of hearing aids in everyday life using ecological momentary assessment: what situations are we missing? Am. J. Audiol. 29, 591–609. doi: 10.1044/2020_AJA-19-00075
Scollie, S., Glista, D., Seto, J., Dunn, A., Schuett, B., Hawkins, M., et al. (2016). Fitting frequency-lowering signal processing applying the American academy of audiology pediatric amplification guideline: updates and protocols. J. Am. Acad. Audiol. 27, 219–236. doi: 10.3766/jaaa.15059
Scollie, S., Seewald, R., Cornelisse, L., Moodie, S., Bagatto, M., Laurnagaray, D., et al. (2005). The desired sensation level multistage input/output algorithm. Trends Amplif. 9, 159–197. doi: 10.1177/108471380500900403
Searchfield, G. D., McAuliffe, M. J., Fok, C., Kyaw, T. A., Williams, E., Burton-Harris, L., et al. (2024). The CogniAid trial. the impact of two hearing aid signal processing strategies on cognition. Front. Audiol. Otol. 2:1285496. doi: 10.3389/fauot.2024.1285496
Shiffman, S., Stone, A. A., and Hufford, M. R. (2008). Ecological momentary assessment. Annu. Rev. Clin. Psychol. 4, 1–32. doi: 10.1146/annurev.clinpsy.3.022806.091415
Timmer, B. H. B., Hickson, L., and Launer, S. (2017). Ecological momentary assessment: feasibility, construct validity, and future applications. Am. J. Audiol. 26, 436–442. doi: 10.1044/2017_AJA-16-0126
Vercammen, C., Oosthuizen, I., Manchaiah, V., Ratinaud, P., Launer, S., Swanepoel, D. W., et al. (2023). Real-life and real-time hearing aid experiences: Insights from self-initiated ecological momentary assessments and natural language analysis. Front. Digit. Health 5:1104308. doi: 10.3389/fdgth.2023.1104308
von Gablenz, P., Schinkel-Bielefeld, N., and Holube, I. (2023). Hearing aid satisfaction in real-life environments in momentary and short-term retrospective assessments. In: Forum Acusticum 2023. Turin.
Windle, R., Dillon, H., and Heinrich, A. (2023). A review of auditory processing and cognitive change during normal ageing, and the implications for setting hearing aids for older adults. Front. Neurol. 14:1122420. doi: 10.3389/fneur.2023.1122420
Wolters, F., Smeds, K., Schmidt, E., Christensen, E. K., and Norup, C. (2016). Common sound scenarios: a context-driven categorization of everyday sound environments for application in hearing-device research. J. Am. Acad. Audiol. 27, 527–540. doi: 10.3766/jaaa.15105
World Health Organization (2004). International Classification of Functioning, Disability and Health. WHO. Available at: https://apps.who.int/iris/bitstream/handle/10665/42407/9241545429_tha+eng.pdf?sequence=7
Wu, Y. H., Stangl, E., Chipara, O., Gudjonsdottir, A., Oleson, J., Bentler, R., et al. (2020). Comparison of in-situ and retrospective self-reports on assessing hearing aid outcomes. J. Am. Acad. Audiol. 31, 746–762. doi: 10.1055/s-0040-1719133
Wu, Y. H., Stangl, E., Chipara, O., Hasan, S. S., DeVries, S., Oleson, J., et al. (2019). Efficacy and effectiveness of advanced hearing aid directional and noise reduction technologies for older adults with mild to moderate hearing loss. Ear. Hear. 40, 805–822. doi: 10.1097/AUD.0000000000000672
Wu, Y. H., Stangl, E., Chipara, O., Hasan, S. S., Welhaven, A., Oleson, J., et al. (2018). Characteristics of real-world signal to noise ratios and speech listening situations of older adults with mild to moderate hearing loss. Ear. Hear. 39, 293–304. doi: 10.1097/AUD.0000000000000486
Wu, Y. H., Stangl, E., Dunn, C., and Oleson, J. (2023). Entropy as a measure of Auditory Environment Diversity: An Ecological Momentary Assessment (EMA) approach. Ear Hear. 44, 917–923. doi: 10.1097/AUD.0000000000001334
Wu, Y. H., Stangl, E., Zhang, X., and Bentler, R. A. (2015). Construct validity of the ecological momentary assessment in audiology research. J. Am. Acad. Audiol. 26, 872–884. doi: 10.3766/jaaa.15034
Xu, J., Wu, Y. H., Stangl, E., Crukley, J., Pentony, S., Galster, J., et al. (2020). Using smartphone-based Ecological Momentary Assessment in audiology research: the participants' perspective. Am. J. Audiol. 29, 935–943. doi: 10.1044/2020_AJA-20-00057
Yadav, A. K., Ahsan, A., and Kumar, V. (2023). Impact of hearing aid usage on emotional and social skills in persons with severe to profound hearing loss. J Audiol Otol. 27, 10–15. doi: 10.7874/jao.2022.00290
Keywords: Ecological Momentary Assessment, hearing aids, listening effort, loudness perception, self-report, patient preference
Citation: Glista D, O'Hagan R, Beh K, Crukley J, Scollie S and Cornelisse L (2024) Real-world assessment of listener preference for hearing aid technology levels in socially involved situations. Front. Audiol. Otol. 2:1430992. doi: 10.3389/fauot.2024.1430992
Received: 11 May 2024; Accepted: 30 November 2024;
Published: 19 December 2024.
Edited by:
Grant Searchfield, The University of Auckland, New ZealandReviewed by:
Niels Henrik Pontoppidan, Eriksholm Research Centre, DenmarkCopyright © 2024 Glista, O'Hagan, Beh, Crukley, Scollie and Cornelisse. This is an open-access article distributed under the terms of the Creative Commons Attribution License (CC BY). The use, distribution or reproduction in other forums is permitted, provided the original author(s) and the copyright owner(s) are credited and that the original publication in this journal is cited, in accordance with accepted academic practice. No use, distribution or reproduction is permitted which does not comply with these terms.
*Correspondence: Danielle Glista, ZGFnbGlzdGFAbmNhLnV3by5jYQ==
Disclaimer: All claims expressed in this article are solely those of the authors and do not necessarily represent those of their affiliated organizations, or those of the publisher, the editors and the reviewers. Any product that may be evaluated in this article or claim that may be made by its manufacturer is not guaranteed or endorsed by the publisher.
Research integrity at Frontiers
Learn more about the work of our research integrity team to safeguard the quality of each article we publish.