- 1Laboratory of Systems Microbiology and Applied Genomics, Department of Sustainable Agriculture, University of Patras, Agrinio, Greece
- 2Biology Department, University of Crete, Heraklion, Greece
- 3Department of Research & Development, Avramar Aquaculture SA, Paiania, Greece
- 4Department of Aquaculture and Fish Diseases, Faculty of Veterinary Science, School of Health Sciences University of Thessaly, Karditsa, Greece
- 5Department of Pathology of Aquatic Organisms, Athens Veterinary Center, Ministry of Rural Development and Food, Athens, Greece
Introduction: The availability of high-quality gilthead seabream (Sparus aurata) larvae in larviculture represents a major bottleneck to the aquaculture. This challenge can be compounded by losses resulting from the emergence of a clinical disorder’s symptomatology. In this study, the observed syndrome included a rotated positioning of the larval sagittal plane and in most cases leading eventually to death.
Methods: Herein, we examined using amplicon sequencing of the 16S rDNA the bacterial communities associated with S. aurata larvae at different conditions (including both fish with no evidence of the syndrome and fish with syndrome) during the early developmental stages along with the contribution of rearing water, organic matter and provided feed in the microbiota during an experiment of 18 days.
Results: Over the development of larvae, differences were observed in the bacterial composition between healthy and symptomatic larvae as well as between components of the system. A remarkable presence of members of the Psychrobacter genus was observed in symptomatic larvae with a significant increase at 18dph. The healthy larvae harbor different bacterial profile with a dominance of Vibrio and Bacillus genera during 3-8dph, various members of Alphaproteobacteria during 11-14dph and Marinifilum at 18dph. The rearing water showed a different bacterial profile compared to the other components, with a slight effect of healthy larvae at 3-8dph. The bacteriome of feeds provided during the rearing of larvae was found to be diverse. Chlorella was mainly dominated by members of Firmicutes, while Rotifer and Artemia were mainly dominated by members of Proteobacteria including different species of Psychrobacter. At 18dph, Artemia feed seems to affect the symptomatic larvae bacteriome, where it enhanced the presence of Psychrobacter spp.
Discussion: Interestingly, the comparative network analysis at 18dph indicated that the bacterial associations in symptomatic larvae were primarily driven by members of the Psychrobacter genus. While various phyla contributed to bacterial associations in healthy larvae with different frequencies including mainly members of Proteobacteria, Bacteroidota and Firmicutes phyla. Finally, the Gammaproteobacteria/Alphaproteobacteria ratio was found to be related to the health condition of larvae. This study provides valuable information which can be used as indicators for monitoring the health status of S. aurata larvae.
Introduction
Over the last two decades, the fish farming sector has been increasingly recognized for its crucial contribution to global food security and nutrition challenges. In 2020, it was estimated to provide 49% of the global aquatic animal production and is expected to increase to 53% by 2030 (FAO, 2022). This trend is expected to continue as the sector grows, with a focus on increasing the quantity and improving the quality of fish farming products (Ottinger et al., 2016; Freitas et al., 2020; FAO, 2022). The Mediterranean region is home to a thriving fish farming industry, with Greece being one of the top producers in the region where it has developed a large aquaculture sector, representing a major share of national seafood production with gilthead seabream (Sparus aurata) accounts for 54% of the production volume (Llorente et al., 2020; HAPO, 2021; FAO, 2023).
One aspect of fish farming in Greece and the Mediterranean area that has gained significant attention in recent years is larviculture, which involves providing suitable environmental conditions and high-quality live prey for the fish larvae until they reach a size that is suitable for transfer to larger grow-out systems (Shields, 2001; Gopakumar et al., 2008; Araujo et al., 2022). Despite improvements in the efficiency of raising adult fish, land-based larviculture remains a major production bottleneck of the fish farming process, as it sets the foundation for the entire production cycle. Disease outbreaks at larval stages represent a major concern in larviculture facilities, as they can cause significant economic losses and even lead to the collapse of the entire production system (Meyer, 1991; Subasinghe and Bernoth, 2000; Muniesa et al., 2020). Marine fish larvae, such as S. aurata, are highly vulnerable during the larval stages due to their small size, immaturity, and fragility upon hatching. The control of the environment, particularly the bacterial one, seems to be of particular importance during the rearing of the early developmental stages. Recently, a concerning disorder has been observed in S. aurata larviculture, where the larvae display various abnormal signs and behaviors, indicating an underlying health issue that led to death (Figure S1). The observed symptoms include a rotated positioning of the larval sagittal plane (rolling), orienting the one of two eyes to the water surface and the light source, surface fish distribution, empty digestive tract, normally developed swim bladder (without any signs of overinflation), and lethargy, in most cases leading eventually to death (Figure S1C). Macroscopically, this larval abnormal orientation was evident due to the characteristic light reflection on the dorsally oriented eye. This symptomatology typically manifests approximately 12-days post-hatching (dph). The current description is based on the experience of rearing sea bream larvae and studying their remarkable behavioral ability (positioning, swimming and orientation) during the challenging period of morphological and physiological changes. To our knowledge, this is the first study demonstrating and describing this symptomatology in sea bream larviculture.
The exact cause of this disorder is yet to be determined, however, numerous studies on S. aurata and a variety of farmed larvae of sub-class Teleostei indicated that the mortality rates, abnormalities and poor performance observed during this phase are thought to be due to disease incidence potentially caused by specific pathogens, opportunistic bacteria that may proliferate excessively or by gut dysbiosis (Haché and Plante, 2011; Llewellyn et al., 2014; Derome et al., 2016; Borges et al., 2021). However, studies that approach the structure of bacterial communities in healthy versus symptomatic or diseased larvae are still relatively scarce. This limits our ability to understand the well-being and symptoms of larvae during this critical land-based rearing stage, as well as our ability to uncover the origin of any future disease that might emerge in later developmental stages.
The larviculture ecosystem has been identified as fertile ground for a wide range of microorganisms. The high bacterial load in this ecosystem could be intensified by the fact that the larvae, feed and the excreted waste coexist within the same environment, namely the rearing water (Savaş et al., 2005; Vadstein et al., 2018a; Borges et al., 2021). This shared environment provides favorable conditions for the proliferation of microorganisms including those that are beneficial, neutral, or even pathogenic. Previous investigations have revealed that commensal microbiota in farmed fish larva may harbor opportunistic pathogenic bacteria as a minor component (Pujalte et al., 2003; Vestrum et al., 2022). Under normal circumstances, these bacteria do not cause harm and coexist with the host in a healthy balance. However, the intensive rearing conditions and alteration of environmental factors (e.g., oxygen, light, and nutrient availability) could trigger the emergence of these bacteria as pathogens, resulting in diseases and infections (Derome et al., 2016; Madeira et al., 2016; Vadstein et al., 2018a; Mariana and Badr, 2019; Manchanayake et al., 2023).
On the other hand, microbiome research using next-generation sequencing (NGS) technique revealed alterations occurring during larval development along with a significant influence of different larviculture practices such as the water-rearing systems and different diets provided on a variety of Teleostei species including gilthead seabream (S. aurata) (Savaş et al., 2005; Califano et al., 2017; Nikouli et al., 2019), sea bass (Dicentrarchus labrax) (Savaş et al., 2005; Lamari et al., 2013), Atlantic cod (Gadus morhua) (Bakke et al., 2015; Vestrum et al., 2018), yellowtail kingfish (Seriola lalandi) (Wilkes Walburn et al., 2019), rabbitfish (Siganus guttatus) (Le et al., 2020), rainbow trout (Oncorhynchus mykiss) (Ingerslev et al., 2014).
This study aimed to examine the dynamics of the bacterial communities between healthy larvae and those exhibiting abnormal orientation (symptomatic) along with the associated bacteriome of the larviculture ecosystem during the early larval developmental stages in an industrial production facility. Using high-throughput Illumina sequencing of 16S rRNA gene, the bacterial communities present in healthy and symptomatic S.aurata larvae at various developmental stages spanning from 3 to 18 days post-hatching (dph) were compared. To determine the impact of various environmental bacterial sources on early microbiome development, we also examined the bacterial communities present in both the rearing water, organic matter and provided feed. Characterization and understanding the composition and interactions of the microbiome in healthy and symptomatic larvae during the early stages of development along with the impact of their surrounding environment lays the groundwork for early monitoring and control of the larvae’s microbiome. This, in turn, has the potential to enhance yields and facilitate the creation of new strategies to develop and improve the aquaculture industry.
Materials and methods
Rearing conditions and sample collections
The experiment was conducted under a commercial farming condition of S. aurata for 18-days period between February and June 2019 at a Greek larviculture commercial unit. Fertilized eggs were placed in rearing tanks with a density of around 100 eggs/L and growth of larvae was performed in a continuous water flow-through. Throughout the experiment, daily monitoring of physical and chemical parameters such as temperature, pH, salinity, light intensity and dissolved oxygen was carried out. These variables remained stable and fell within the optimal ranges recommended by Moretti et al. guidelines for S. aurata larviculture (Moretti et al., 2005). The sampling scheme for bacterial community analysis of S. aurata larvae aimed to identify the bacteria present in both healthy and symptomatic larvae at each developmental stage that coincides with changes in larval feeding. Despite that the symptoms typically manifest approximately 12-days post-hatching (dph), larvae from all developmental stages in tanks where the symptoms appeared were deemed symptomatic. This includes 3-8dph larvae that do not exhibit any apparent symptoms. The investigation targeted the bacterial community in healthy and symptomatic S. aurata larvae (LH and LS respectively), rearing water from healthy and symptomatic tanks (WH and WS respectively), feeds (separately: Chlorella, Rotifer and Artemia) and organic matter from 18dph (OM).
S. aurata larvae were collected from four different tanks at three different times points during each of the established stages of development: Chlorella feeding stage at 3-8dph (n=24), Rotifer feeding at 11-14dph (n=24) and Artemia feeding at 18dph (n=12). Due the small size of larvae, the analysis was conducted on the whole-body microbiota. From each tank, a sample of water and larvae was taken with a 5ml sterile plastic pipette, minimizing the contamination between the tanks and transferred to sterile containers. To prepare each larval sample, a triplicate of 1.5 mL of larvae in water was taken and centrifuged to separate the rearing water from the larvae. The resulting solid materials were then subjected to DNA extraction. Triplicate samples of organic matter and each feed type (Chlorella, Rotifers and Artemia) underwent a similar process to larvae samples. Rearing water samples (1 L) were collected at the same time points as the larvae, then filtered through a 0.22μm pore-size filter, and the filter was used for subsequent DNA extraction.
DNA extraction, 16S rRNA gene amplicon library preparation and sequencing
Total DNA extraction from all the materials namely, whole larva, water filters, organic matter and feeds was performed following a modified CTAB protocol (Doyle and Doyle, 1987). The quality of DNA preparations and the concentration of double-stranded DNA were estimated using a Q5000 micro-volume UV Vis spectrophotometer (Quawell Technology, San Jose, CA, USA). DNA samples were preserved in Eppendorf tubes at −20°C until further use.
Polymerase chain reaction amplification of the hypervariable V3–V4 region of the 16S rRNA gene was performed on each DNA sample using barcoded fusion primers U341F-MiSeq 5’ -CCTACGGGRSGCAGCAG-3’ and 805R-MiSeq 5’-GACTACHVGGGTATCTAATCC-3’ (Klindworth et al., 2013). The PCRs were performed in 25 μL reactions containing KAPA Taq Buffer (10X) at a final concentration of 1X, dNTP mix solution at 200 μM each, forward and reverse primer solution at 0.4 μM, 0.5 U of KAPA Taq DNA polymerase (5 U/μl), ≤ 250 ng from the template DNA solution, and sterile deionized water. The amplification was carried out as follows: an initial denaturation at 95°C for 5 min and 35 cycles of denaturation at 95°C for 30 s, annealing at 54°C for 30 s, and extension at 72°C for 45 s, followed by final extension step at 72°C for 2 min. Negative and positive controls were always included in parallel. To examine the presence and size of the amplified fragments, PCR products were electrophoresed on a 1.5% (w/v) of agarose gel in TAE buffer (1X) (40 mM Tris–acetate, 1 mM EDTA). Approximately, 550 bp amplification products were visualized in Bio-Rad’s Gel Doc™ XR+ system. Positive PCR products of the correct size were then purified using polyethylene glycol (20% PEG, 2.5 M NaCl) (Hartley and Bowen, 1996).
To include the indexes and the Illumina adaptors, a second PCR was performed in a final volume of 50 μL. Each reaction contained 10 μL of KAPA Taq Buffer (10X), 0.4 μL of dNTPs solution (25 mM each), 5 μL of the forward/reverse indexing primer (10 μM), 5 μL of KAPA Taq DNA Polymerase (5 U/μL), and 2 μL from the diluted purified PCR product (10 ng/μL) and sterile deionized water. The temperature profile used for the amplification was: an initial denaturation at 95°C for 3 min and 8 cycles of denaturation at 95°C for 30 s, annealing at 55°C for 30 s, and extension at 72°C for 30 s and a final 5 min terminator reaction at 72°C. The resulting amplicons were purified using the NucleoMag NGS Clean-up and Size Selection kit (Macherey-Nagel, Düren, Germany) following the manufacturer’s recommendation. Indexed amplicons from different samples were quantified with a Quawell Q5000 micro-volume UV-Vis spectrophotometer and mixed in equimolar concentrations (8 nM). Samples were sequenced by Macrogen using a 2x300bp pair-end kit on a MiSeq platform.
Bioinformatic data processing
After sequencing, raw sequencing reads were demultiplexed and converted to FASTQ files and the Illumina adapters were trimmed using Illumina standard algorithm. Resulting sequences were analyzed and processed using a combination of USEARCH version 11 (Edgar, 2010) and QIIME2 distribution 2019.1 (Bolyen et al., 2019). Forward and reverse reads of each sample were assembled into paired-end fragments and merged in a single Fastq file using fastq_mergepairs command. Fragments were excluded from the analysis if they were < 400 bp in length. The quality of assembled sequences was improved using fastq_filter command, followed by removing duplicated sequences using fastx_uniques command. Unique paired-end fragments were then clustered into operational taxonomic units (OTUs) with cluster_otus command based on 97% OTU clustering using UPARSE algorithm (Edgar, 2013). Cross-talk errors were detected and omitted with uncross command based on UNCROSS2 algorithm (Edgar, 2018). Extremely rare OTUs (<0.001% of total sequences across all samples) were discarded using otutab_trim command. Based on 16S rRNA gene SILVA 138 release database (Pruesse et al., 2007), taxonomy was assigned to the representative sequences of the identified OTUs using BLAST+ algorithm (Camacho et al., 2009) as implemented in QIIME2. Phylogenetic tree was constructed using FastTree algorithm (Price et al., 2009) and rooted using midpoint-root method as implemented in QIIME2.
Bacterial composition and diversity analysis
Alpha diversity indices as well as indices depicting the population structure were calculated using vegan R package (Oksanen et al., 2020) based on a normalized OTU table at a depth of 5,000 sequences/sample (Observed species, Chao1, Shannon, Simpson and Evenness). Good’s coverage was calculated based on the count OTU table. Pairwise ANOVA was used to identify significant differences of alpha diversity indices between the different groups.
Between samples diversity was calculated based on Generalized UniFrac distance using GUniFrac R package (Chen et al., 2021). Overall differences in community structure were shown using the constrained ordination technique: Canonical analysis of principal coordinates (CAP) based on 999 permutations. While similarities in bacterial community structures were presented using unconstrained ordination techniques: Principal coordinates analyses (PCoA). Statistically significant differences between samples were identified with permutational multivariate analysis of variance (PERMANOVA) (Anderson, 2001) using 999 permutations and Monte Carlo tests. CAP, PCoA analyses and PERMANOVA test were performed and visualized on PRIMER version 6 and PERMANOVA+ for PRIMER routines (Clarke and Gorley, 2006; Anderson et al., 2008). A p-value < 0.05 was considered indicative of statistical significance. Pairwise comparisons of mean relative abundance of OTUs between samples were performed using non-parametric Wilcoxon rank sum test (Bauer, 1972). The obtained significance values were corrected for multiple testing using the Benjamini-Hochberg method (Benjamini and Hochberg, 1995).
Network analysis
To account for the heterogeneity of the data, the bacterial community network associated with the larval samples was evaluated by splitting the larval OTU matrix into multiple datasets based on the feeding stages (Chlorella, Rotifer and Artemia within 3-8dph, 11-14dph, and 18dph respectively) and health status (healthy and symptomatic). Each dataset (abundance matrix with OTUs in rows and larval samples in columns) was loaded into CoNet plugging available in Cytoscape (Shannon et al., 2003) to identify the co-presence and mutual exclusion interactions among the OTUs selected. In all the groups, data were normalized by column to reduce compositionality issues associated to different sampling efforts. Pearson and Spearman correlation coefficients along with the Bray-Curtis dissimilarity index were chosen to infer the relationships between OTUs, with correlation thresholds set to retain 25% to top-scoring edges (top positives and negatives). Statistical significance of each interaction was tested using the row-shuffle randomization followed by bootstrap score distributions options with 1,000 iterations. Edges with original scores outside the 0.95 range of their bootstrap distribution were discarded, and the merged p-values (using brown method) were corrected using the Benjamini–Hochberg method.
Results
Dataset overview
To characterize the bacterial community associated with S. aurata larvae and their connection to the observed symptoms, we sampled larvae, rearing water at various feeding stages along with samples of Chlorella, Rotifer and Artemia and organic matter at 18dph. The sequencing of hypervariable region V3-V4 of the 16S rRNA gene resulted in a total of 4,010,458 raw reads. After quality filtering and removal of chimeric sequences, 2,860,970 high-quality reads were clustered into OTUs at a 97% sequence similarity cut-off with an average of 17.770 reads/sample. The mean coverage estimate was 0.98, indicating a good sampling of the microbial communities (Table S1). OTUs with an abundance below 0.1% in all the samples were removed, resulting in a total of 118 OTUs classified in 11 phyla, 14 classes and 81 genera with 37 OTUs identified at the species level (Table S2). Proteobacteria was the most abundant taxonomic group in all the studied materials (72%), followed by Bacteroidota and Firmicutes where the three phyla represented more than 92% of the total bacterial community. At the class level, Proteobacteria was represented by Gammaproteobacteria and Alphaproteobacteria (37.1% and 35.4% respectively), Bacteroidota with Bacteroidia class (13.2%) and Firmicutes with Bacilli and Clostridia (5.8% and 1.3%). Various taxa were detected at the genus level, with Pseudophaeobacter (15.3%) being the most dominant, followed by Psychrobacter (12.4%). The remaining taxa constituted separately less than 5% of the entire bacterial community.
Diversity of bacterial communities in healthy and symptomatic larviculture system along with growth stages
During the initial stage of larval development (3-8dph), the healthy larvae exhibited a marginally higher bacterial diversity in comparison to the larvae that developed disorder symptoms (ANOVA; p<0.05; Figure S2A). However, no differences were observed in terms of species richness. As symptoms began to manifest between 11dph and 14dph, a decrease in bacterial richness and diversity became evident in symptomatic larvae compared to their healthy counterparts (Figure S2B). With the full appearance of symptoms at 18dph, a significant decrease in both species richness and diversity was observed among the larvae showing symptoms (ANOVA; p<0.001; Figure S2C). Interestingly, across the developmental stages, the rearing water of symptomatic larvae harbors significantly higher levels of bacterial species richness and diversity compared to S. aurata larvae. Conversely, in the healthy tanks, no significant differences in bacterial richness and diversity were observed between the rearing water and larvae. However, a slight decrease was observed in bacterial diversity of rearing water at 18dph (ANOVA; p<0.05; Figure S2C). Compared to the larvae and rearing water, the feed used at each stage exhibited lower bacterial richness and diversity (Figure S2).
As evident from the CAP along with PERMANOVA analysis, a remarkable difference was observed in the bacterial composition between healthy and symptomatic larvae as well as between components of the larviculture system namely the rearing water, live feed and organic matter (Figure 1; Table 1). During the early larval stage (3-8dph), significant differences were observed between larvae, rearing water and Chlorella (PERMANOVA; p<0.01). PCoA and CAP analyses indicated a slight overlapping of healthy larvae and rearing water clusters, suggesting a minor influence of water bacteriome on the larvae bacteriome (Figures 1A, S3A; Table S3). Between 11-14dph, when Rotifer live feed was introduced, the bacterial profile of the different components of the larviculture remains to display significant differences (Figures 1B, S3B; Table S3). However, the bacterial profile of Rotifers showed a resemblance to that of symptomatic larvae (p=0.062), while showing a marginal difference to that of healthy larvae (p=0.033). At a later developmental stage (18dph) with the introduction of Artemia live feed, the healthy and symptomatic larvae tend to harbor different bacterial profiles (p<0.05). However, both profiles seem to be influenced to some extent by Artemia live feed bacteriome (Figures 1C, S3C; p>0.1; Table S3). The rearing water from healthy and symptomatic tanks along with organic matter samples formed significantly separated clusters (PERMANOVA; p<0.05; Figure 1C; Table S3).
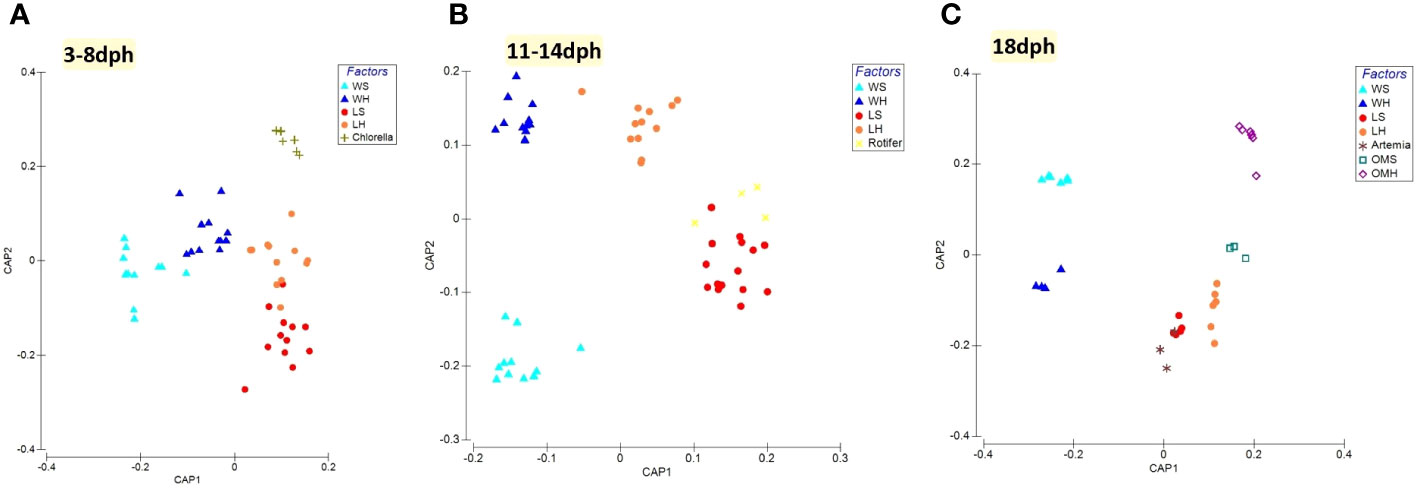
Figure 1 Canonical analysis of principal coordinates (CAP) of beta-diversity based on GUnifrac distance between different components of the larviculture at each S. aurata developmental stage. (A) 3-8dph, (B) 11-14dph and (C) 18dph.
Taxonomic structure of bacterial communities in healthy and symptomatic larviculture system along with growth stages
The bacterial community associated with healthy and symptomatic S. aurata larvae along with their rearing water and feeds clearly changed with the developmental stage at different taxonomic levels. During the initial stage 3-8dph, the bacterial community consisted of four phyla: Proteobacteria, Firmicutes, Bacteroidota and Cyanobacteria. Proteobacteria was the most dominant phylum for both healthy and symptomatic larvae representing more than 80% of the bacterial community, followed by Firmicutes (10.9 ± 6.8%, 15.1 ± 7.9% respectively), Bacteroidota (8.4 ± 4.2%, 1.3 ± 0.4% respectively) and Cyanobacteria with less than 0.2% of the bacterial community (Figure 2A). The most significantly abundant OTUs in larvae that developed the observed symptoms belonged to Gammaproteobacteria (79.4 ± 8.1%) including Psychrobacter_OTU8 (26.1 ± 9.2%), Pseudomonas (24.5 ± 11.4%) and Vibrio (15.8 ± 7.4%) followed by Bacillus member of Bacilli class (14.0 ± 8.0%). The bacterial community of healthy larvae was dominated by members of Alphaproteobacteria mainly by Pseudophaebacter (32.3 ± 9.0%) followed by Vibrio (17.9 ± 3.9%), Bacilli (10.8 ± 6.8%) and NS3a-marine-group (8.0 ± 4.2%) (Figures 2B, 3). As Rotifer live feed was introduced, the relative abundance of Gammaproteobacteria was decreased and replaced with Alphaproteobacteria for both healthy and symptomatic 11-14dph larvae (80.4 ± 4.5%, 60.6 ± 7.2% respectively; Figure 2B). At Genus level, healthy larvae showed a significant decrease in Pseudophaebacter (15.4 ± 1.3%) and an increase in Leisingera and Nautella genera (29.6 ± 2.7%, 18.9 ± 2.8% respectively). While in symptomatic larvae an increase of members of Alphaproteobacteria was observed, mainly Pseudophaebacter and Nautella (26.3 ± 4.9%, 14.4 ± 3.2% respectively). Among members of Gammaproteobacteria, a significant increase was observed in Psychrobacter_OTU4 (10.2 ± 5.3%; Wilcoxon’s rank sum test: p<0.05) that was assigned to Psychrobacter marincola species, and a decrease in Psychrobacter_OTU8 (6.4 ± 2.7%). Interestingly, a strong shift in bacterial composition was observed with the full appearance of symptoms at 18dph, where a sharp increase of Gammaproteobacteria was observed in symptomatic larvae which represent 99.7 ± 0.06% of the bacterial community, which was shared between two Psychrobacter species, namely Pychrobacter marincola (OTU4; 48.0 ± 13.5%) and an undefined species of Psychrobacter (OTU8; 49.9 ± 14.1%). Conversely, a decrease of members of Proteobacteria was observed in the healthy larvae, which was replaced by an increase in Marinifilum members of Bacteroidota (40.5 ± 2.7%) followed by Fusibacter members of Firmicutes (7.4 ± 1.4%), in addition to members of various phyla including Campilobacterota and Desulfobacterota (13.8 ± 1.3% and 13.0 ± 2.8% respectively) (Figures 2A, 3). Variation of the proportion of these phyla (such as Proteobacteria: Bacteroidota) may be a useful metric to assess changes between healthy and symptomatic larvae. The dominance of Proteobacteria, mainly members of Gammaproteobacteria in symptomatic larvae at 18dph was reflected in the Proteobacteria (P) to Bacteroidota (B) ratio which shows a higher mean of P:B compared to healthy larvae (Figure 4A). While this difference was not evident during the earlier developmental stages. Furthermore, the proportion of Proteobacteria at class level [Gammaproteobacteria (G):Alphaproteobacteria (A)] could be also a reliable marker for detecting anomalies in larvae, where the G:A ratio was significantly higher in symptomatic larvae from the beginning of the symptom’s manifestation during 11-14dph and the difference increased with the development of the symptoms at 18dph (Figure 4B).
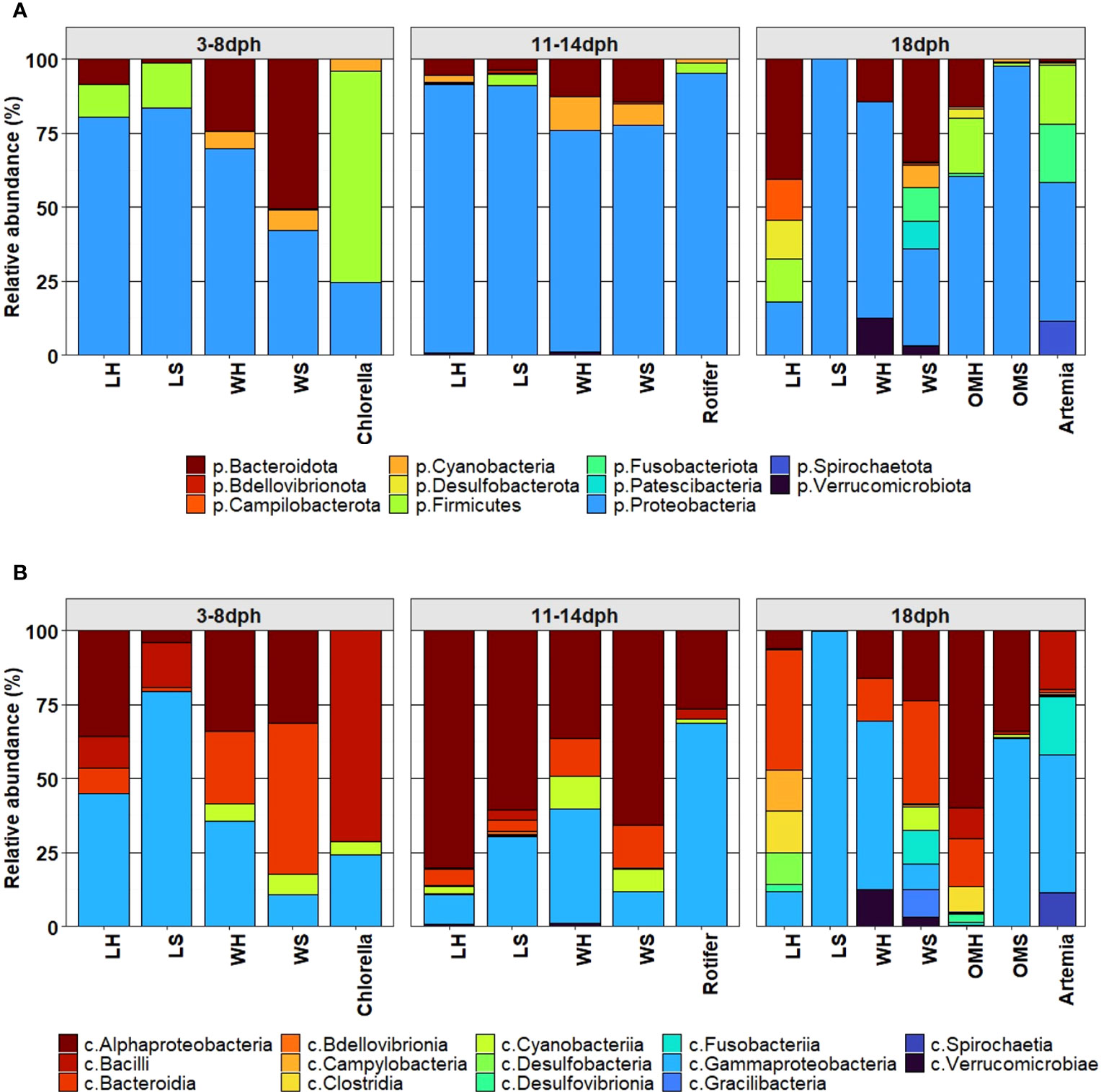
Figure 2 Comparison of microbial composition associated with S. aurata larvae, rearing water, feed (Chlorella, Rotifer and Artemia) and organic matter at (A) phylum level, (B) class level.
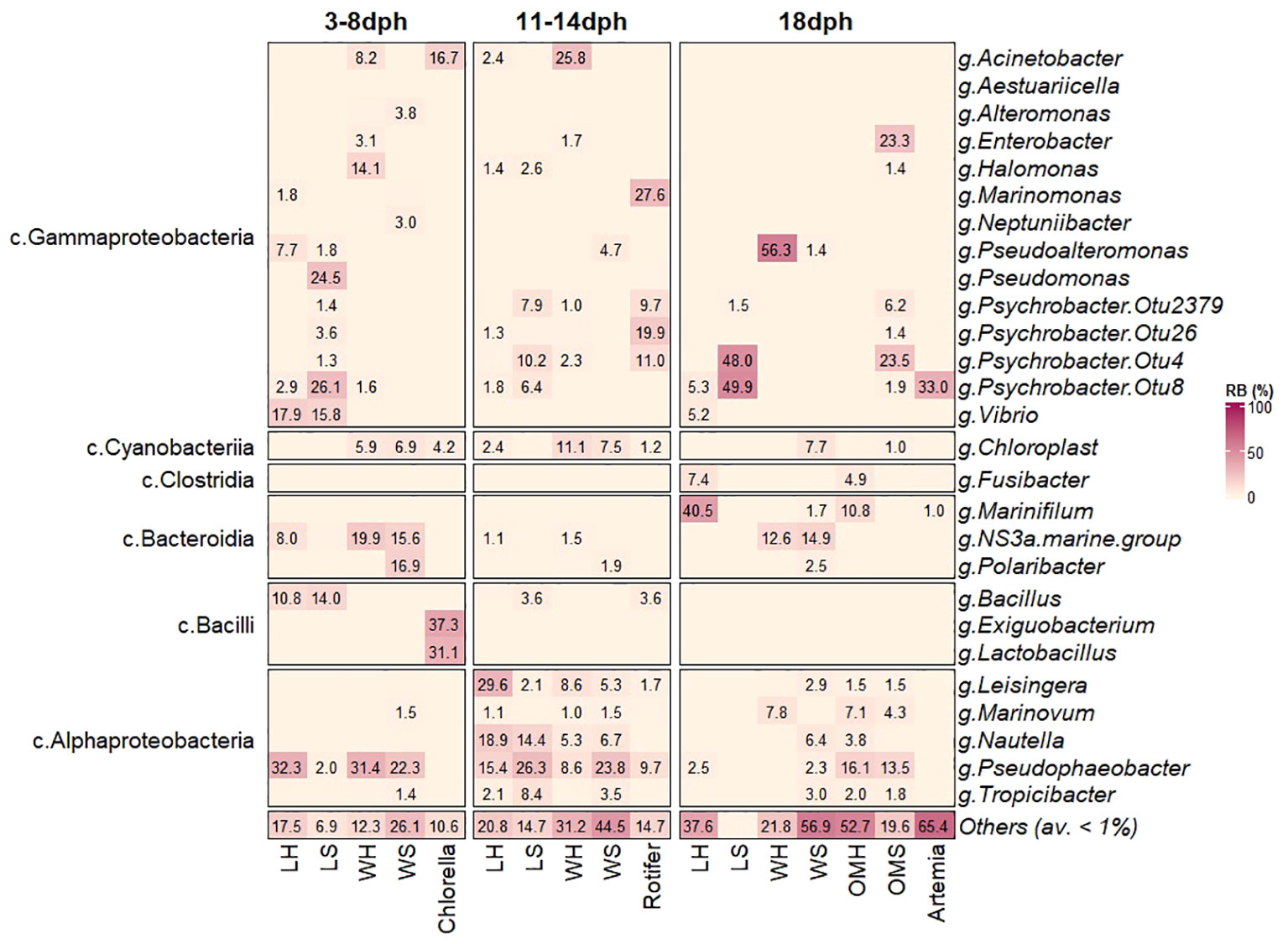
Figure 3 Comparison of microbial composition associated with S. aurata larvae, rearing water, feed (Chlorella, Rotifer and Artemia) and organic matter at genus level.
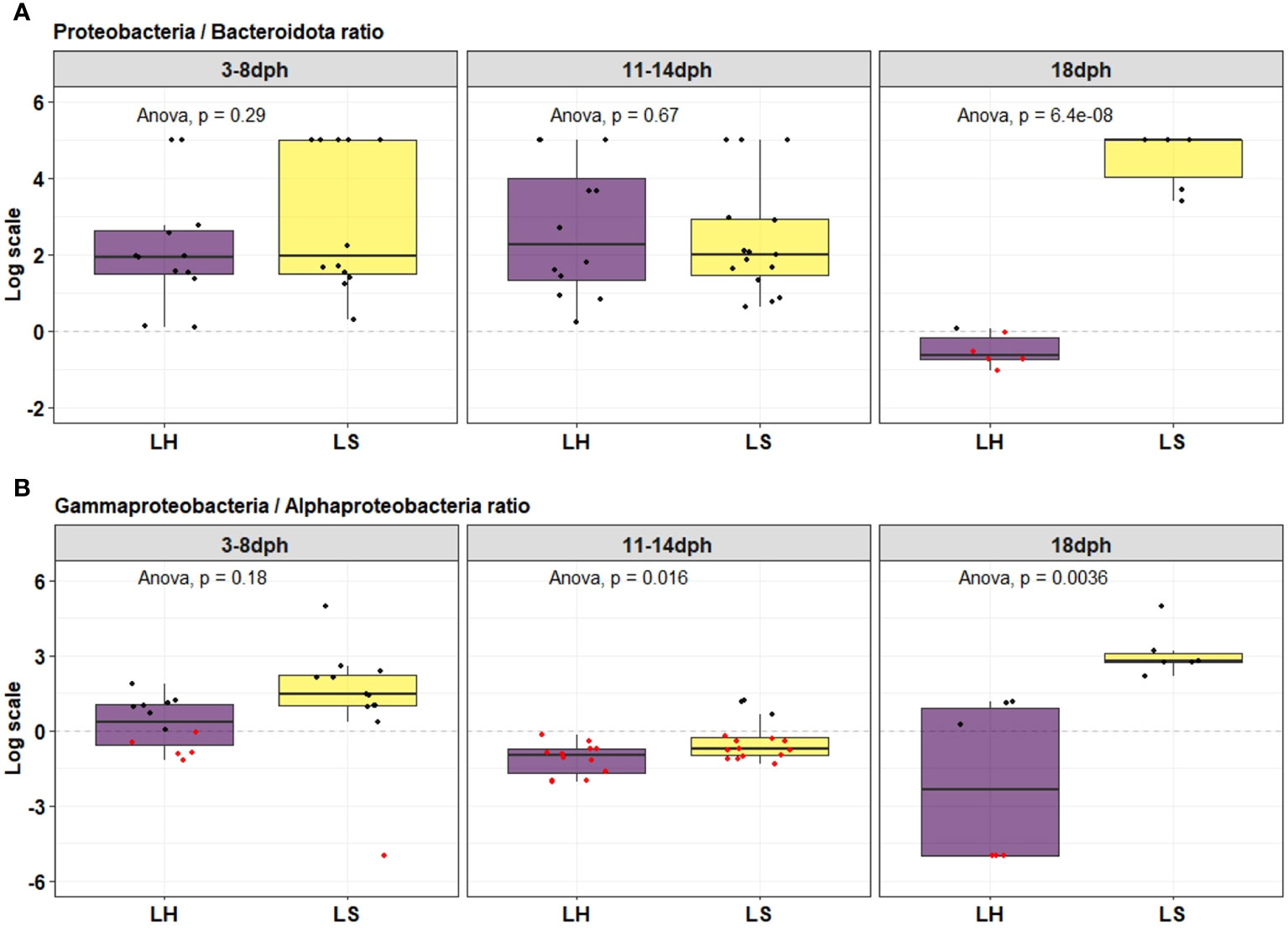
Figure 4 Proteobacteria/Bacteroidota ratio (A) and Gamma-/Alpha-proteobacteria ratio (B) through the development of healthy (LH) and symptomatic (LS) S. aurata larvae.
During the earlier developmental stages of larvae (3-8dph), the rearing water in both healthy and symptomatic tanks was dominated by Pseudophaebacter (31.4 ± 6.2%, 22.3 ± 5.3% respectively), followed by NS3a-marine-group (19.9 ± 4.8%, 15.6 ± 6.3% respectively) and Polaribacter (16.9 ± 4.7%) mainly in symptomatic tanks (Figure 3). This reflects that certain genera identified in healthy larvae including NS3a-marine-group and Pseudophaebacter were likely acquired from the rearing water. In contrast, the larvae that were going to develop symptoms displayed a susceptibility to the water bacteriome. From 11dph to 14dph, a significant increase in members of Alphaproteobacteria was observed in the rearing water in both healthy and symptomatic tanks (Figures 2B, 3), while at 18dph the rearing water from healthy tanks showed a significant increase of Pseudoalteomonas represented mainly by P. tetraodonis species (OTU3; 56.3 ± 9.7%), whereas tanks displaying symptoms demonstrated a broad range of distinct taxa.
The bacterial taxa present in the larvae feeds were found to be diverse. Chlorella was mainly dominated by members of Firmicutes phylum namely Exiguobacterium and Lactobacillus (37.3 ± 7.5% and 31.1 ± 11.8 respectively) followed by members of Proteobacteria (24.4 ± 3.2%) and Cyanobacteria (4.2 ± 0.4%) (Figure 3). Rotifer was dominated mainly by members of Proteobacteria (95%) including Marinomonas (24.6 ± 8.2) followed by different species of Psychrobacter in addition to a minor community of Firmicutes and Cyanobacteria. In contrast, Artemia, has a slightly lower diversity compared to Rotifer with the dominance of members of Proteobacteria mainly by Psychrobacter (OTU8; 33.0 ± 7.7%) followed by Enterococcus member of Firmicutes phylum (19.7 ± 8.8%), and Fusobacterium member of Fusobacteriota (18.0 ± 8.0%) (Figure 3).
The organic matter at 18dph reflects the diversity between the healthy and the symptomatic tanks. In heathy tanks the organic matter shows higher bacterial diversity compared to the symptomatic tanks with dominance of member of Alphaproteobacteria represented mainly by Pseudophaeobacter (16.1± 0.87%) followed by Marinifilum (10.8 ± 3.5%) members of Bacteroidota (Figures 2B, 3). In contrast, the organic matter in the symptomatic tanks where mainly dominated by members of Gammaproteobacteria namely by Psychrobacter marincola (OTU4) and Enterobacter (23.5 ± 8.3% and 23.3 ± 16.4% respectively).
Bacterial networks of healthy and symptomatic larvae
To assess the bacterial association patterns in S. aurata larvae, we performed a comparative network analysis between healthy and symptomatic larvae across their developmental in the larviculture environment. Marked differences were observed between networks in terms of size and topological features (Table 2). The network analysis indicates that the bacterial community in healthy larvae is highly diverse with more associations between their components compared to the symptomatic larvae. The symptomatic larvae, on the other hand, exhibited a limited number of interactions and nodes, which decreased considerably following the appearance of symptoms at 18dph (Table 2). In detail, across the developmental stages, the healthy larvae networks were represented with a comparable number of nodes, ranging from 91 to 100 nodes. However, the symptomatic larvae showed variations in the number of nodes, with an increase from 77 during 3-8dph to 93 during 11-14dph, then sharply decreased to 30 at 18dph. Similar patterns were also observed in terms of the number of associations, which increased from 285 during 3-8dph to 374 during 11-14dph, then sharply decreased to 72 at 18dph. Conversely, the number of associations in healthy larvae decreased from 735 during 3-8dph to 373 during 11-14dph, then a steep rise to 1233 associations at 18dph. In addition, most of the associations were negative (Mutual Exclusion) for most of the networks, except for symptomatic larvae at 3-8dph (Table 2).
During the earlier developmental stage (3-8dph), the healthy and symptomatic larval networks showed a quite comparable taxonomic profile consisting of the same major three phyla, Proteobacteria, Bacteroidetes and Firmicutes with comparable node frequencies (Figure S4). Proteobacteria contributed with the largest number of nodes followed by Firmicutes (Bacilli class) in healthy larvae and Bacteroidota (Bacteroidia class) in symptomatic larvae (Figures 5A, S4). The associations in the healthy larvae were mainly conducted by members of Gammaproteobacteria namely Pseudoalteromonas, Vibrio and Colwellia, followed by members of Alphaproteobacteria such as Pseudophaeobacter and Ponticoccus (Figure 5B). In contrast, the bacterial associations in larvae that were going to develop symptoms were primarily driven by Gammaproteobacteria members, with more associations linked to various OTUs of the Psychrobacter genus (OTU8, OTU4, OTU26, Otu2379 and OTU1981) compared to healthy larvae (OTU8) (Figures 5A, B).
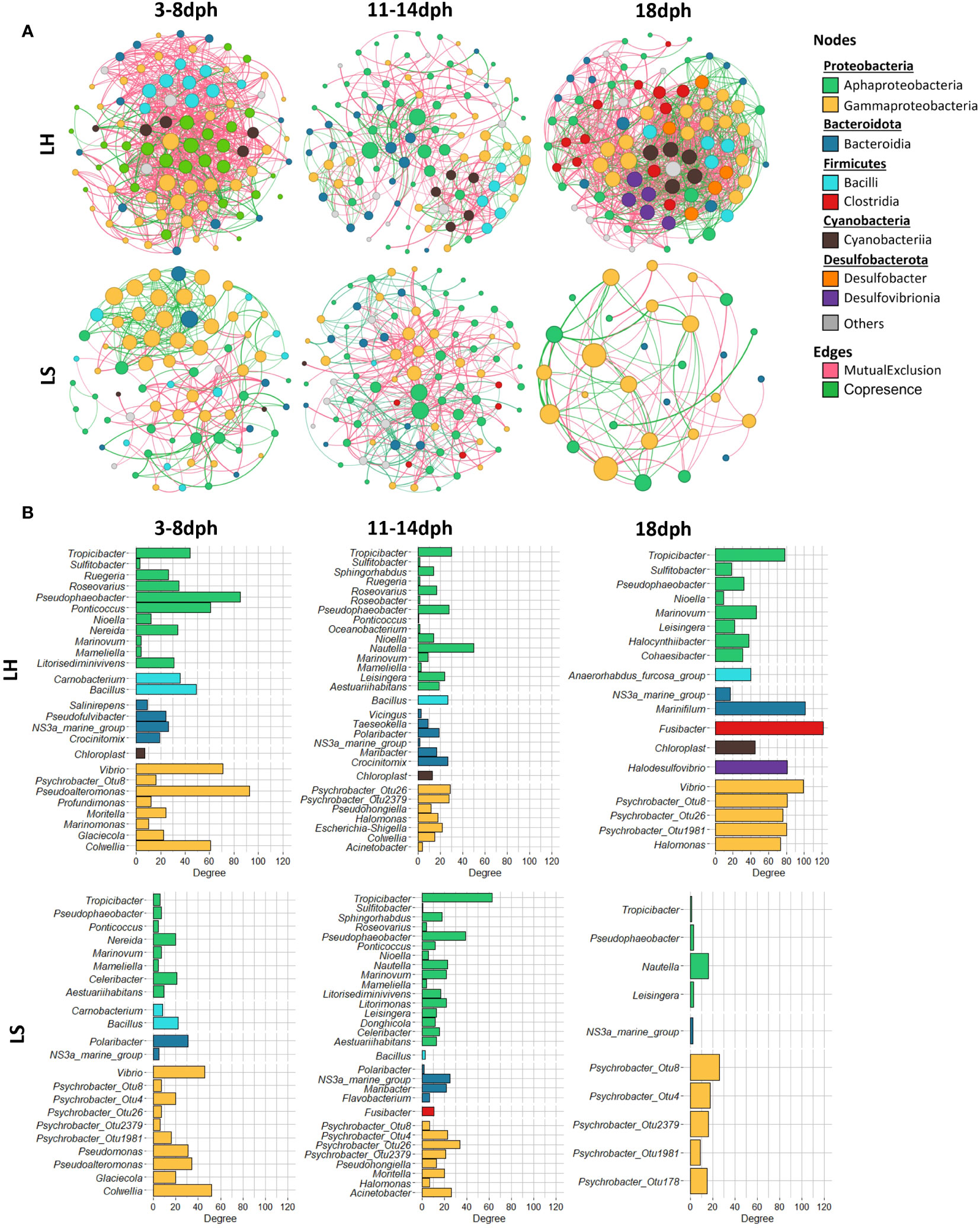
Figure 5 Co-occurrence and mutual exclusion network analysis. (A) Bacterial community networks during the development of healthy (LH) and symptomatic (LS) S. aurata larvae. For each developmental stage, the nodes correspond to the present OTUs colored according to class level. The size of each node corresponds to its degree of connection (the number of edges associated with the node). (B) Bar charts indicate the number of connections nodes at the genus level.
Despite the similarities in the number of nodes and associations, different bacterial profiles were observed between healthy and symptomatic larvae during 11-14dph. In both networks, the associations were primarily established by members of Alphaproteobacteria namely Nautella, Tropicibacter and Pseudophaeobacter followed by members of Gammaproteobacteria mainly with members of the Psychrobacter genus with the contribution of more Psycrobacter species in symptomatic larvae (OTU8, OTU4, OTU26 and Otu2379) compared to healthy larvae (OTU26, OTU2379) (Figure 5B).
At 18dph, various phyla contributed to forming bacterial associations in healthy larvae with different node frequencies (Figures 5A, S4). Fusibacter member of Bacilli had the highest number of associations followed by Marinifilum member of Bacteroidia and members of Gammaproteobacteria including Vibrio and Psychrobacter genus (OTU8, OTU26, and OTU1981) (Figures 5A, B). However, the bacterial associations in symptomatic larvae were primarily driven by members of Proteobacteria mainly by member Psychrobacter genus (OTU8, OTU4, Otu2379, OTU1981 and OTU178) (Figure 5B).
Discussion
The fish-microbiome interactions play a vital role in determining the overall health and well-being of farmed fish (de Bruijn et al., 2018; Raulo et al., 2018; Perry et al., 2020; Luan et al., 2023). Numerous studies have examined the fish-associated microbiome throughout various life stages, from juvenile and adult stages along with their dynamics with the rearing environment including factors such as water quality and feed composition (Wang et al., 2018; Krotman et al., 2020; Zeng et al., 2020; Nikouli et al., 2021; Roquigny et al., 2021; Sehnal et al., 2021; Karlsen et al., 2022; Quero et al., 2022; Rabelo-Ruiz et al., 2022). However, so far, little is known about the diversity and function of these microorganisms, particularly in relation to disease occurrence during the earlier rearing stages. Here, we compared bacterial communities associated with healthy and symptomatic larvae (lateral positioning, abnormal orientation) and the related rearing water, feeds and organic matter along with sea bream development. Using high throughput sequencing of the hyper-variable region V3-V4 of the 16S rRNA gene, the current analysis reveals that various factors significantly influence the bacterial communities of S. aurata larvae with a clear distinction between the microbiome of symptomatic and healthy larvae, indicating that some specific bacterial groups might be applied as indicators for monitoring the health status of sea bream larvae in hatchery. The causative agent responsible for the observed symptoms does not appear to belong to a specific known pathogen. Instead, it seems to be associated with variations in the diversity and interactions within the bacterial communities. Interestingly, the influence of feed and developmental stage factors cannot be dismissed, as they consistently align. Nevertheless, both healthy and symptomatic larvae have been sampled across the developmental stages and with all the feed provided. The structure of the communities of both healthy and symptomatic larvae can be characterized as dynamic, as evident from clear fluctuations that are observed as the larvae progress through different developmental stages. Moreover, the rearing water, live feed and organic matter which are integral components of the larviculture ecosystem, have demonstrated a certain level of influence, particularly on symptomatic larvae compared to healthy ones.
Microbiota of symptomatic vs healthy larvae across the developmental stages
Although the symptoms typically appear between 11- and 14-days post-hatching, distinct bacterial communities were observed at earlier stages of development between symptomatic and healthy larvae. This suggests that the microbial composition may play a role in the development of symptoms, even before they become visibly apparent. On the other hand, the larval-associated microbiome is shaped by a variety of internal factors such as species, genetics and developmental stage (Bakke et al., 2015; Wilkes Walburn et al., 2019; Borges et al., 2021; Medina-Félix et al., 2023), and external factors such as rearing water, live feed provided and the environmental carrying capacity (De Schryver and Vadstein, 2014; Vadstein et al., 2018a; Vestrum et al., 2018). Therefore, to investigate the relationship between the bacterial profile of larvae and the observed symptoms, we ensured uniformity across all tanks used in this study by standardizing the aforementioned factors such as the water-rearing system and the provided feed (Ingerslev et al., 2014; Vestrum et al., 2018; Wilkes Walburn et al., 2019).
The bacterial community in symptomatic S. aurata larvae was characterized by lower species richness and diversity mainly with the full appearance of symptoms at 18dph compared to healthy larvae which present an opposite pattern. The bacterial richness and diversity remains generally stable during the development of healthy larvae which aligns with findings from a previous study that focused on characterizing bacterial populations during the early stages of healthy larvae (Califano et al., 2017; Nikouli et al., 2019).
Taxonomic characterization of both symptomatic and healthy S. aurata larvae consists of three dominant phyla: Proteobacteria, Firmicutes and Bacteroidota with fluctuation on relative abundance across the developmental stages and the health status. In other studies, Proteobacteria also seems to be the preponderant phyla of the bacterial community found in both European seabass and gilthead seabream eggs (before or after disinfection) and also in broodstock water across different hatcheries (Najafpour et al., 2021). During the early developmental stage (3-8dph), the symptomatic were mainly dominated by members of Gammaproteobacteria including the genera Pseudomonas, Psychrobacter and Vibrio while the healthy were dominated by members of Alphaproteobacteria such as the genus Pseudophaeobacter that is most likely acquired from the rearing water. The presence of potentially pathogenic bacteria in production systems such as some Pseudomonas, Psychrobacter and Vibrio species may be related to mass mortality of fish larvae and juveniles. The introduction of Chlorella has no impact on the bacterial communities of larvae and no major differences were observed in the bacterial composition of the rearing water in healthy and symptomatic tanks. Therefore, the difference in the bacterial profile between the healthy larvae and those that will develop symptoms may be likely related to the bacterial composition of the eggs. In the case of S. aurata, (Nikouli et al., 2019) suggested a vertical transmission of bacteria from heathy fertilized eggs to early developmental stage of larvae (5dph) with 59 shared OTUs between these consecutive stages. Since our study did not include bacterial characterization during the egg stage, a similar pattern could potentially be suggested for symptomatic larvae. The eggs used in aquaculture industry under certain circumstances might carry opportunistic pathogens as it has been reported for the presence of genera such as Pseudomonas spp. and Vibrio spp. in Atlantic cod eggs (Hansen and Olafsen, 1999) and Aeromonas spp., Vibrio spp. and Yersinia spp. in Atlantic salmon eggs (Liu et al., 2014). Therefore, Pseudomonas and Vibrio characterized in symptomatic S. aurata larvae were likely transmitted vertically from the reared eggs. However, their abundance was not maintained or relatively reduced during the rearing process. This could probably be due to the treatment and water-rearing system used in the larviculture facility (Sekkin and Kum, 2011; Rigos et al., 2021).
With the introduction of Rotifer feed and the manifestation of symptoms during 11-14dph, a significant shift was observed for both healthy and symptomatic larvae with an increase of the relative abundance of various members of Alphaproteobacteria mainly members of Rhodobacteraceae which is in line to some extent with the results of Nikouli et al. (Nikouli et al., 2019). Alongside the increase of members of Alphaproteobacteria in symptomatic larvae, a significant increase of Psychrobacter marincola (OTU4) and an uncultured Psychrobacter sp. (OTU2379) was also observed which suggest potential correlation between members of this genus with the observed symptoms. Unlike the findings of Nikouli et al. (Nikouli et al., 2019), our study suggests that the bacterial community of healthy larvae was unaffected by Rotifer live feed (PERMANOVA, p=0.035). This difference could potentially be attributed to variations in sampling time, as Nikouli et al. collected larvae at 15dph, whereas our study utilized samples taken between 11-14dph. While the symptomatic larvae were more susceptible to bacterial profile of Rotifer where it mainly enhanced the presence of Psychrobacter genus (OTU4 and OTU2379). The transition from Chlorella to animal-based protein, Rotifer, is likely have a significant impact on early-stage mainly for the symptomatic larvae where Rotifer feed showed a significant impact. Consequently, we can infer that the susceptibility to diet bacteriome is higher in symptomatic larvae compared to their healthy counterparts.
With the full manifestation of symptoms at 18dph, the S. aurata larvae show a significant decrease in species richness and diversity which was due to the dominance of Psychrobacter marincola (OTU4) and an uncultured Psychrobacter sp. (OTU8) representing together around 98% of the total bacterial community. The significant increase of members of Psychrobacter at this stage and the previous stage (11-14dph) raises intriguing possibilities regarding their role in the observed symptoms as it has been recently described to be phylogenetically close to pathobiont bacteria (Welter et al., 2021). Certain Psychrobacter spp. have been reported to be infectious to multiple fish species, including Oncorhynchus mykiss (rainbow trout), Salmo salar, Rhabdosargus haffara (haffara sea bream) (Hisar et al., 2002; McCarthy et al., 2013; El-Sayed et al., 2023). On the other hand, it has been isolated from different organs of apparently healthy fish species, such as the gastrointestinal tract, skin, gills, and kidney (Wilson et al., 2008; Svanevik and Lunestad, 2011; Floris et al., 2013; McCarthy et al., 2013). Some specific strains of Psychrobacter have even been used as novel probiotics, showing a positive effects on feed utilization, digestive enzyme activity, and innate immune responses in fish like grouper Epinephelus coioides and Oreochromis niloticus (Nile tilapia) (Yang et al., 2011; Makled et al., 2017). Hence, the correlation between the increased relative abundance of Psychrobacter spp. and the emergence of symptoms in S. aurata larvae do not necessarily imply causation. Therefore, further investigations are required to elucidate the role of Psychrobacter in S. aurata larviculture and the larval rearing of other species.
Beside these, the role of rearing water and live feed may not be conclusively established, it is plausible that these factors could exert some degree of influence on the overall environment experienced by the larvae (Savaş et al., 2005; Chen et al., 2019; Gundersen et al., 2022). For instance, Pseudoalteromonas identified mainly in rearing water of healthy tanks at 18dph is a known probiotics with the effects of reducing the larval mortality of fish by inhibiting vibrio and controlling biofilm formation in sea bass (Rahmani et al., 2023). In contrast, the rearing water of symptomatic larvae exhibited distinct characteristics, including the presence of members from the family Rhodobacteraceae such as the genus Pseudophaeobacter and members of the family Flavobacteriaceae such as Polaribacter, that are known to be frequently surface associated and metabolize a wide variety of organic compounds (Dang and Lovell, 2015; Rud et al., 2017), which are supposed to be highly concentrated in symptomatic tanks. This suggests a potential correlation with the symptomatic tanks, indicating that the abundance of members of these families might be a response to the health status of the larvae, rather than contributing to the development of symptoms.
Biomarkers of S. aurata larval lateral positioning symptomatology
The causative agent responsible for the observed symptoms does not appear to belong to a specific known pathogen. Instead, it seems to be associated with variations in the diversity and interactions within the bacterial communities (Olafsen, 2001; Vadstein et al., 2004; De Schryver and Vadstein, 2014; Vadstein et al., 2018b). For yellowtail kingfish adults, (Legrand et al., 2018) has recently suggested the ratio of the relative abundances of Proteobacteria to Bacteroidota (P/B) as an indicator of fish disease where they demonstrate a decrease in P/B ratio at early stages of the enteritis disease. Such approach has already been proposed in humans and mammals, where the Firmicutes to Bacteroidota ratio has been used as a biomarker for assessing intestinal dysbiosis (Stojanov et al., 2020). However, in the case of fish larvae, Firmicutes and Bacteroidetes are not always the predominant phyla. In fact, as observed in the present and previous studies, during the early developmental stages, Proteobacteria were often more dominant (Bakke et al., 2015; Nikouli et al., 2019; Wilkes Walburn et al., 2019). Thus, we propose a Gammaproteobacteria to Alphaproteobacteria ratio which is based on members of Proteobacteria phylum. Herein, for S. aurata larvae the G/A ratio was found to significantly increase in symptomatic samples from the early manifestation of symptoms during 11-14dph comparing to P/B ratio that show differences only at 18dph with full appearance of symptoms. Alphaproteobacteria such as members of Rhodobacteraceae have often mutual beneficial relationships with their host larvae (Vadstein et al., 2018b; Pettersen et al., 2021). In contrast, members of Gammaproteobacteria including many genera known for their pathogenicity in aquaculture including Vibrio, Pseudomonas, Alteromonas, and Enterobacter (Vadstein et al., 2018b) are characterized by their ability to rapidly reproduce and colonize new environments with high resources. Their high growth rate is often associated with opportunistic behavior and may be prone to cause dysbiosis when conditions are suboptimal or when the host’s immune system is immature like the case of in the land-based rearing of larvae (Andrews and Harris, 1986; Vadstein et al., 1993; Vadstein et al., 2018b). Therefore, the abundance of Gammaproteobacteria over Alphaproteobacteria could be a useful biomarker for assessing larval health at an early stage of development. To this end, further studies are required to understand the normal range of G/A ratio in healthy fish populations. This includes the variation of the ratio under different conditions including the key factors of fish health, such as growth rates and disease prevalence.
Bacterial network of symptomatic and healthy S. aurata larvae
Evidently, healthy larvae exhibited a significantly larger number of interactions compared to their symptomatic counterparts. This observation suggests that multiple bacterial taxa contribute to the formation of the community, mainly at 18dph, thus ensuring the stability of the bacterial community. In other words, the presence and involvement of various taxa may indicate the resilient of the healthy larvae to external disturbances, demonstrating its robustness. Interestingly, over the development of symptomatic larvae, the observed increasing number of interactions of members of Psychrobacter suggests that the community dynamics are predominantly influenced by this genus, despite the lower abundance of certain species within it. Similarly, there is a significant number of interactions involving the Psychrobacter genus, although the contribution of certain species is less than in symptomatic larvae. These findings, indicate the importance of members of this genus that is not detected in previous studies on S. aurata larvae (Califano et al., 2017; Nikouli et al., 2021). Thus, the role and the establishment of members of this genus in relation to the health status of S. aurata larvae required further investigation and should cover diverse larviculture systems to understand their influence on later larval stages, as well as on the subsequent juvenile and adult stages of the fish. Furthermore, certain taxa with lower relative abundance show high number of interactions in a particular group, such as Tropicibacter, Vibrio in healthy larvae, Colwellia for both healthy and symptomatic larvae at 3-8dph suggesting that even low abundant taxa may play an important role in shaping the total bacterial community (Jousset et al., 2017; Lam and Ye, 2022). The nature, functional relevance, and the alterations of the observed associations within bacterial communities on healthy and symptomatic S. aurata larvae remain unclear, which required further investigation.
Factors influencing the composition of larvae microbiota
Several finfish species, including S. aurata, undergo comparable larval developmental stages. Following hatching, the larva first enters a “close-mouth” phase which represents a non-feeding stage. As the larva progresses in its development, it undergoes a transition to an “open-mouth” stage. At this stage the gastrointestinal tract is prone to acquired bacteria present in the surrounding water (Sullam et al., 2012; Vadstein et al., 2018a; Gundersen et al., 2022; Taniguchi et al., 2022). The bacterial community observed in the rearing water was distinct from that of both healthy and symptomatic larvae with higher bacterial richness and diversity across the developmental stage. However, during early developmental stage (3-8dph) the healthy larvae tend to acquire bacteria from the rearing water mainly Pseudophaeobacter which is in line with previous studies on S. aurata larvae (Nikouli et al., 2019). In contrast, the presence of this genus was observed in symptomatic larvae at a later stage (11-14dph), this might suggest that the growth of symptomatic larvae may be delayed compared to that of healthy larvae. On the other hand, distinct bacterial profile was observed in rearing water between healthy and symptomatic tanks particularly after 11dph. Acinetobacter sp. and Pseudoalteromonas tetraodonis were significantly increased in rearing water of healthy tanks during 11-14dph and 18dph respectively. The presence of Acinetobacter in aquaculture environment has recently been associated with probiotic properties (Farzanfar, 2006; Bunnoy et al., 2019; Yaylacı, 2022). However, their role in the larviculture remains unknown and requires further investigations.
Rotifers and Artemia are commonly used as live feed organisms in the larviculture systems. They provide essential nutrition and support the early growth and development of fish larvae (Lahnsteiner et al., 2023). As demonstrated in this and the previous studies, the live feed strongly overlap with the larval microbiome during the initial stages of development (Califano et al., 2017; Nikouli et al., 2019; Wilkes Walburn et al., 2019). The bacterial community associated with Rotifer was characterized by high bacterial diversity as it feeds on bacterial culture as part of their diet. Artemia on the other hand, tends to exhibit a less diverse bacterial community. This is likely because Artemia cysts are subjected to sterilization through immersion in chlorine solutions before decapsulation (Nikouli et al., 2019). The bacterial composition of both diet may differ based on the used species, and rearing conditions (Haché and Plante, 2011; Tkavc et al., 2011; Seychelles et al., 2013; Turgay et al., 2020).
The presence of organic matter in larviculture systems is generally increased by factors such as larval mortality, defecation and the uneaten live feed. This increased organic matter load can create a favorable environment for microbial interactions within the larviculture system (Vadstein et al., 2004; Vadstein et al., 2013). At 18dph the bacterial composition of organic matter seems to reflect to some extent the bacteria present in the larviculture system. This includes the presence of members of Alphaproteobacteria, which are abundant in the rearing water, and members of Psychrobacter, found mainly in symptomatic larvae and live feed. At this stage, the influence of the bacterial community associated with organic matter on both S. aurata larvae, and the rearing water is not well-established. A study by Califano et al. (Califano et al., 2017) suggests that the decrease in bacterial diversity observed at a later rearing stage (34dph) in the rearing water could be attributed to an increase in organic matter concentration within the tanks. Therefore, it is plausible that the bacterial community of the rearing water, and subsequently the larvae at late rearing stages, could be affected by the organic matter bacteriome.
The metagenomics analysis used in this study provides valuable insight into the diversity and composition of the bacterial community associated with both healthy and symptomatic S. aurata larvae, highlighting their dynamic across the developmental stage and the rearing environment. Nevertheless, the reliance on DNA sequencing may not capture the functional aspects of the microbial community, limiting our understanding of their roles in larvae health and symptoms. Complementary approaches, such as metatranscriptomics or metabolomics, would provide a more comprehensive view of the functional dynamics within the bacterial communities and their contribution to fish larvae physiology and quality.
Conclusion
The finding of the present study revealed that even before the appearance of symptoms, the symptomatic S. aurata larvae were characterized by distinct bacterial communities compared to their healthy counterpart with a remarkable shift through the rearing stages. The bacterial associations in larvae that were going to develop symptoms were primarily driven by Gammaproteobacteria members, with more associations linked to various OTUs of the Psychrobacter genus. Notably, the G:A ratio was significantly higher in symptomatic larvae from the beginning of the symptom’s manifestation and may be developed as a useful biomarker. Regardless of the health status, the influence of water bacteriome on the larval bacteriome appeared to be minimal. On the other hand, S. aurata larvae show a higher likelihood of acquiring bacteria from the provided live feed (Rotifer and Artemia), this presence does not seem to have a significant impact on the host. These results suggest that the microbial communities in both the rearing water and provided feed may not direct contribute to the observed symptoms. Overall, these findings shed light on the complex dynamics of microbial communities associated with S. aurata during the early stages of larval development. Understanding the factors influencing the larval microbiota is crucial for optimizing larviculture practices and mitigating potential risks to the health and development of the reared larvae.
Data availability statement
The datasets presented in this study can be found in online repositories. The names of the repository/repositories and accession number(s) can be found in the article/Supplementary Material.
Ethics statement
Ethical approval was not required for the study involving animals in accordance with the local legislation and institutional requirements because fish samples were sourced from commercial fisheries (Andromeda SA). No live animals were utilized in any capacity during our study.
Author contributions
NB: Formal Analysis, Writing – original draft, Writing – review & editing. GA: Formal Analysis, Writing – review & editing. GK: Conceptualization, Writing – review & editing. KTz: Writing – review & editing. KTo: Writing – review & editing. EG: Writing – review & editing. PS: Formal Analysis, Methodology, Supervision, Writing – review & editing. GT: Conceptualization, Methodology, Resources, Supervision, Writing – review & editing.
Funding
The author(s) declare financial support was received for the research, authorship, and/or publication of this article. The research leading to these results has received funding from the Hellenic Ministry of Rural Development and Food (2018-2021) from the project titled “Study of the genome and microbial communities in the development and production of aquacultured sea bream and sea bass (FishμBiome)”.
Acknowledgments
The authors are thankful to Andromeda S.A. for collecting the samples of the analyzed larviculture system.
Conflict of interest
KTz and KTo were employed by Avramar Aquaculture SA.
The remaining authors declare that the research was conducted in the absence of any commercial or financial relationships that could be construed as a potential conflict of interest.
The author(s) declared that they were an editorial board member of Frontiers, at the time of submission. This had no impact on the peer review process and the final decision.
Publisher’s note
All claims expressed in this article are solely those of the authors and do not necessarily represent those of their affiliated organizations, or those of the publisher, the editors and the reviewers. Any product that may be evaluated in this article, or claim that may be made by its manufacturer, is not guaranteed or endorsed by the publisher.
Supplementary material
The Supplementary Material for this article can be found online at: https://www.frontiersin.org/articles/10.3389/faquc.2023.1270932/full#supplementary-material
References
Anderson M. J. (2001). A new method for non-parametric multivariate analysis of variance. Austral Ecol. 26, 32–46. doi: 10.1111/j.1442-9993.2001.01070.pp.x
Anderson M. J., Gorley R. N., Clarke K. R. (2008). PERMANOVA+ for PRIMER: Guide to Software and Statistical Methods (Plymouth, UK: PRIMER-E).
Andrews J. H., Harris R. F. (1986). “r- and K-Selection and Microbial Ecology,” in Advances in Microbial Ecology Advances in Microbial Ecology. Ed. Marshall K. C. (Boston, MA: Springer US), 99–147. doi: 10.1007/978-1-4757-0611-6_3
Araujo G. S., da Silva J. W. A., Cotas J., Pereira L. (2022). Fish farming techniques: current situation and trends. J. Mar. Sci. Eng. 10, 1598. doi: 10.3390/jmse10111598
Bakke I., Coward E., Andersen T., Vadstein O. (2015). Selection in the host structures the microbiota associated with developing cod larvae (Gadus morhua). Environ. Microbiol. 17, 3914–3924. doi: 10.1111/1462-2920.12888
Bauer D. F. (1972). Constructing confidence sets using rank statistics. J. Am. Stat. Assoc. 67, 687–690. doi: 10.2307/2284469
Benjamini Y., Hochberg Y. (1995). Controlling the false discovery rate: A practical and powerful approach to multiple testing. J. R. Stat. Soc. Ser. B (Methodological) 57, 289–300. doi: 10.1111/j.2517-6161.1995.tb02031.x
Bolyen E., Rideout J. R., Dillon M. R., Bokulich N. A., Abnet C. C., Al-Ghalith G. A., et al. (2019). Reproducible, interactive, scalable and extensible microbiome data science using QIIME 2. Nat. Biotechnol. 37, 852–857. doi: 10.1038/s41587-019-0209-9
Borges N., Keller-Costa T., Sanches-Fernandes G. M. M., Louvado A., Gomes N. C. M., Costa R. (2021). Bacteriome structure, function, and probiotics in fish larviculture: the good, the bad, and the gaps. Annu. Rev. Anim. Biosci. 9, 423–452. doi: 10.1146/annurev-animal-062920-113114
Bunnoy A., Na-Nakorn U., Srisapoome P. (2019). Probiotic Effects of a Novel Strain, Acinetobacter KU011TH, on the Growth Performance, Immune Responses, and Resistance against Aeromonas hydrophila of Bighead Catfish (Clarias macrocephalus Günther 1864). Microorganisms 7, 613. doi: 10.3390/microorganisms7120613
Califano G., Castanho S., Soares F., Ribeiro L., Cox C. J., Mata L., et al. (2017). Molecular taxonomic profiling of bacterial communities in a gilthead seabream (Sparus aurata) hatchery. Front. Microbiol. 8. doi: 10.3389/fmicb.2017.00204
Camacho C., Coulouris G., Avagyan V., Ma N., Papadopoulos J., Bealer K., et al. (2009). BLAST+: architecture and applications. BMC Bioinf. 10, 421. doi: 10.1186/1471-2105-10-421
Chen Z., Chang Z., Zhang L., Jiang Y., Ge H., Song X., et al. (2019). Effects of water recirculation rate on the microbial community and water quality in relation to the growth and survival of white shrimp (Litopenaeus vannamei). BMC Microbiol. 19, 192. doi: 10.1186/s12866-019-1564-x
Chen J., Zhang X., Zhou H. (2021) GUniFrac: Generalized UniFrac istances, Distance-Based Multivariate Methods and Feature-Based Univariate Methods for Microbiome Data Analysis. Available at: https://CRAN.R-project.org/package=GUniFrac.
Clarke K. R., Gorley R. N. (2006). PRIMER v6: user manual/tutorial. PRIMER-E Plymouth Routines in Multivariate Ecological Research. (Plymouth: PRIMER-E).
Dang H., Lovell C. R. (2015). Microbial surface colonization and biofilm development in marine environments. Microbiol. Mol. Biol. Rev. 80, 91–138. doi: 10.1128/mmbr.00037-15
de Bruijn I., Liu Y., Wiegertjes G. F., Raaijmakers J. M. (2018). Exploring fish microbial communities to mitigate emerging diseases in aquaculture. FEMS Microbiol. Ecol. 94, fix161. doi: 10.1093/femsec/fix161
Derome N., Gauthier J., Boutin S., Llewellyn M. (2016). "Bacterial opportunistic pathogens of fish." in Rasputin effect: When commensals symbionts become parasitic. 81, 81–108. doi: 10.1007/978-3-319-28170-4_4
De Schryver P., Vadstein O. (2014). Ecological theory as a foundation to control pathogenic invasion in aquaculture. ISME J. 8, 2360–2368. doi: 10.1038/ismej.2014.84
Doyle J. J., Doyle J. L. (1987). A rapid DNA isolation procedure for small quantities of fresh leaf tissue. Phytochem. Bull. 19, 11–15.
Edgar R. C. (2010). Search and clustering orders of magnitude faster than BLAST. Bioinformatics 26, 2460–2461. doi: 10.1093/bioinformatics/btq461
Edgar R. C. (2013). UPARSE: highly accurate OTU sequences from microbial amplicon reads. Nat. Methods 10, 996–998. doi: 10.1038/nmeth.2604
Edgar R. C. (2018). UNCROSS2: identification of cross-talk in 16S rRNA OTU tables. bioRxiv, 400762. doi: 10.1101/400762
El-Sayed M. R., Emam A. M., Osman A. E., Abd El-Galil M. A. E.-A. A., Sayed H. H. (2023). Detection and description of a novel Psychrobacter glacincola infection in some Red Sea marine fishes in Hurghada, Egypt. BMC Vet. Res. 19, 23. doi: 10.1186/s12917-022-03542-8
FAO (2022). The State of world Fisheries and Aquaculture 2022. Towards Blue Transformation. (Rome, Italy: FAO Rome).
FAO (2023). National Aquaculture Legislation Overview, Greece. Fisheries and Aquaculture Division. (Rome, Italy: FAO Rome).
Farzanfar A. (2006). The use of probiotics in shrimp aquaculture. FEMS Immunol. Med. Microbiol. 48, 149–158. doi: 10.1111/j.1574-695X.2006.00116.x
Floris R., Manca S., Fois N. (2013). Microbial ecology of intestinal tract of gilthead sea bream (Sparus aurata Linnaeus 1758) from two coastal lagoons of Sardinia (Italy). Trans. Waters Bull. 7, 4–12. doi: 10.1285/i1825229Xv7n2p4
Freitas J., Vaz-Pires P., Câmara J. S. (2020). From aquaculture production to consumption: Freshness, safety, traceability and authentication, the four pillars of quality. Aquaculture 518, 734857. doi: 10.1016/j.aquaculture.2019.734857
Gopakumar G., Madhu K., Jayasankar R., Madhu R., Kizhakudan J. K., Josileen J., et al. (2008). Live feed research for larviculture of marine finfish and shellfish. Mar. Fish. Inf. Service T&E Ser. 197, 1–6.
Gundersen M. S., Vadstein O., De Schryver P., Attramadal K. J. K. (2022). Aquaculture rearing systems induce no legacy effects in Atlantic cod larvae or their rearing water bacterial communities. Sci. Rep. 12, 19812. doi: 10.1038/s41598-022-24149-x
Haché R., Plante S. (2011). The relationship between enrichment, fatty acid profiles and bacterial load in cultured rotifers (Brachionus plicatilis L-strain) and Artemia (Artemia salina strain Franciscana). Aquaculture 311, 201–208. doi: 10.1016/j.aquaculture.2010.11.034
Hansen G. H., Olafsen J. A. (1999). Bacterial interactions in early life stages of marine cold water fish. Microb. Ecol. 38, 1–26. doi: 10.1007/s002489900158
HAPO (2021). Aquaculture in Greece 2021, annual report (Greece: Hellenic Aquaculture Producers Organization).
Hartley L. J., Bowen H. (1996). PEG precipitation for selective removal of small DNA fragments. Focus 18, 27.
Hisar O., Yanik T., Hisar S. A. (2002) Clinical And Pathological Investigation Of Psychrobacter Immobilis Infection In Rainbow Trour (Oncorhynchus Mykiss, Walbaum). Available at: http://hdl.handle.net/10524/19062 (Accessed May 24, 2023).
Ingerslev H.-C., von Gersdorff Jørgensen L., Lenz Strube M., Larsen N., Dalsgaard I., Boye M., et al. (2014). The development of the gut microbiota in rainbow trout (Oncorhynchus mykiss) is affected by first feeding and diet type. Aquaculture 424–425, 24–34. doi: 10.1016/j.aquaculture.2013.12.032
Jousset A., Bienhold C., Chatzinotas A., Gallien L., Gobet A., Kurm V., et al. (2017). Where less may be more: how the rare biosphere pulls ecosystems strings. ISME J. 11, 853–862. doi: 10.1038/ismej.2016.174
Karlsen C., Tzimorotas D., Robertsen E. M., Kirste K. H., Bogevik A. S., Rud I. (2022). Feed microbiome: confounding factor affecting fish gut microbiome studies. ISME Commun. 2, 1–4. doi: 10.1038/s43705-022-00096-6
Klindworth A., Pruesse E., Schweer T., Peplies J., Quast C., Horn M., et al. (2013). Evaluation of general 16S ribosomal RNA gene PCR primers for classical and next-generation sequencing-based diversity studies. Nucleic Acids Res. 41, e1. doi: 10.1093/nar/gks808
Krotman Y., Yergaliyev T. M., Alexander Shani R., Avrahami Y., Szitenberg A. (2020). Dissecting the factors shaping fish skin microbiomes in a heterogeneous inland water system. Microbiome 8, 9. doi: 10.1186/s40168-020-0784-5
Lahnsteiner F., Lahnsteiner E., Duenser A. (2023). Suitability of different live feed for first feeding of freshwater fish larvae. Aquacult. J. 3, 107–120. doi: 10.3390/aquacj3020010
Lam T. J., Ye Y. (2022). Meta-analysis of microbiome association networks reveal patterns of dysbiosis in diseased microbiomes. Sci. Rep. 12, 17482. doi: 10.1038/s41598-022-22541-1
Lamari F., Castex M., Larcher T., Ledevin M., Mazurais D., Bakhrouf A., et al. (2013). Comparison of the effects of the dietary addition of two lactic acid bacteria on the development and conformation of sea bass larvae, Dicentrarchus labrax, and the influence on associated microbiota. Aquaculture 376–379, 137–145. doi: 10.1016/j.aquaculture.2012.11.016
Le D., Nguyen P., Nguyen D., Dierckens K., Boon N., Lacoere T., et al. (2020). Gut microbiota of migrating wild rabbit fish (Siganus guttatus) larvae have low spatial and temporal variability. Microb. Ecol. 79, 539–551. doi: 10.1007/s00248-019-01436-1
Legrand T. P. R. A., Catalano S. R., Wos-Oxley M. L., Stephens F., Landos M., Bansemer M. S., et al. (2018). The inner workings of the outer surface: skin and gill microbiota as indicators of changing gut health in yellowtail kingfish. Front. Microbiol. 8. doi: 10.3389/fmicb.2017.02664
Liu Y., de Bruijn I., Jack A. L., Drynan K., van den Berg A. H., Thoen E., et al. (2014). Deciphering microbial landscapes of fish eggs to mitigate emerging diseases. ISME J. 8, 2002–2014. doi: 10.1038/ismej.2014.44
Llewellyn M. S., Boutin S., Hoseinifar S. H., Derome N. (2014). Teleost microbiomes: the state of the art in their characterization, manipulation and importance in aquaculture and fisheries. Front. Microbiol. 5. doi: 10.3389/fmicb.2014.00207
Llorente I., Fernández-Polanco J., Baraibar-Diez E., Odriozola M. D., Bjørndal T., Asche F., et al. (2020). Assessment of the economic performance of the seabream and seabass aquaculture industry in the European Union. Mar. Policy 117, 103876. doi: 10.1016/j.marpol.2020.103876
Luan Y., Li M., Zhou W., Yao Y., Yang Y., Zhang Z., et al. (2023). The fish microbiota: research progress and potential applications. Engineering 29 (10), 137–146. doi: 10.1016/j.eng.2022.12.011
Madeira D., Costa P. M., Vinagre C., Diniz M. S. (2016). When warming hits harder: survival, cellular stress and thermal limits of Sparus aurata larvae under global change. Mar. Biol. 163, 1–14. doi: 10.1007/s00227-016-2856-4
Makled S. O., Hamdan A. M., El-Sayed A.-F. M., Hafez E. E. (2017). Evaluation of marine psychrophile, Psychrobacter namhaensis SO89, as a probiotic in Nile tilapia (Oreochromis niloticus) diets. Fish Shellfish Immunol. 61, 194–200. doi: 10.1016/j.fsi.2017.01.001
Manchanayake T., Salleh A., Amal M. N. A., Yasin I. S. M., Zamri-Saad M. (2023). Pathology and pathogenesis of Vibrio infection in fish: A review. Aquacult. Rep. 28, 101459. doi: 10.1016/j.aqrep.2022.101459
Mariana S., Badr G. (2019). Impact of heat stress on the immune response of fishes. J. Survey Fish. Sci., 149–159. doi: 10.18331/SFS2019.5.2.14
McCarthy Ú., Stagg H., Donald K., Garden A., Weir S. J. (2013). Psychrobacter sp. isolated from the kidney of salmonids at a number of aquaculture sites in Scotland. Bull. Eur. Assoc. Fish Pathol. 33, 67–72.
Medina-Félix D., Garibay-Valdez E., Vargas-Albores F., Martínez-Porchas M. (2023). Fish disease and intestinal microbiota: A close and indivisible relationship. Rev. Aquacult. 15, 820–839. doi: 10.1111/raq.12762
Meyer F. P. (1991). Aquaculture disease and health management. J. Anim. Sci. 69, 4201–4208. doi: 10.2527/1991.69104201x
Moretti A., Pedini Fernandez-Criado M., Vetillart R. (2005). Manual on hatchery production of seabass and gilthead seabream (Rome: FAO).
Muniesa A., Basurco B., Aguilera C., Furones D., Reverté C., Sanjuan-Vilaplana A., et al. (2020). Mapping the knowledge of the main diseases affecting sea bass and sea bream in Mediterranean. Transboundary Emerg. Dis. 67, 1089–1100. doi: 10.1111/tbed.13482
Najafpour B., Pinto P. I. S., Moutou K. A., Canario A. V. M., Power D. M. (2021). Factors driving bacterial microbiota of eggs from commercial hatcheries of european seabass and gilthead seabream. Microorganisms 9, 2275. doi: 10.3390/microorganisms9112275
Nikouli E., Meziti A., Antonopoulou E., Mente E., Kormas K. A. (2019). Host-Associated Bacterial Succession during the Early Embryonic Stages and First Feeding in Farmed Gilthead Sea Bream (Sparus aurata). Genes 10, 483. doi: 10.3390/genes10070483
Nikouli E., Meziti A., Smeti E., Antonopoulou E., Mente E., Kormas K. (2021). Gut microbiota of five sympatrically farmed marine fish species in the aegean sea. Microb. Ecol. 81, 460–470. doi: 10.1007/s00248-020-01580-z
Oksanen J., Blanchet G., Friendly M., Kindt R., Legendre P., McGlinn D., et al. (2020) vegan: Community Ecology Package. Available at: https://CRAN.R-project.org/package=vegan.
Olafsen J. A. (2001). Interactions between fish larvae and bacteria in marine aquaculture. Aquaculture 200, 223–247. doi: 10.1016/S0044-8486(01)00702-5
Ottinger M., Clauss K., Kuenzer C. (2016). Aquaculture: Relevance, distribution, impacts and spatial assessments – A review. Ocean Coast. Manage. 119, 244–266. doi: 10.1016/j.ocecoaman.2015.10.015
Perry W. B., Lindsay E., Payne C. J., Brodie C., Kazlauskaite R. (2020). The role of the gut microbiome in sustainable teleost aquaculture. Proc. R. Soc. B: Biol. Sci. 287, 20200184. doi: 10.1098/rspb.2020.0184
Pettersen J. P., Gundersen M. S., Almaas E. (2021). Robust bacterial co-occurence community structures are independent of r- and K-selection history. Sci. Rep. 11, 23497. doi: 10.1038/s41598-021-03018-z
Price M. N., Dehal P. S., Arkin A. P. (2009). FastTree: computing large minimum evolution trees with profiles instead of a distance matrix. Mol. Biol. Evol. 26, 1641–1650. doi: 10.1093/molbev/msp077
Pruesse E., Quast C., Knittel K., Fuchs B. M., Ludwig W., Peplies J., et al. (2007). SILVA: a comprehensive online resource for quality checked and aligned ribosomal RNA sequence data compatible with ARB. Nucleic Acids Res. 35, 7188–7196. doi: 10.1093/nar/gkm864
Pujalte M., Sitjà-Bobadilla A., Álvarez-Pellitero P., Garay E. (2003). Carriage of potentially fish-pathogenic bacteria in Sparus aurata cultured in Mediterranean fish farms. Dis. Aquat. Org. 54, 119–126. doi: 10.3354/dao054119
Quero G. M., Piredda R., Basili M., Maricchiolo G., Mirto S., Manini E., et al. (2022). Host-associated and environmental microbiomes in an open-sea mediterranean gilthead sea bream fish farm. Microb. Ecol. 86 (2), 1319–1330. doi: 10.1007/s00248-022-02120-7
Rabelo-Ruiz M., Newman-Portela A. M., Peralta-Sánchez J. M., Martín-Platero A. M., Agraso M., del M., et al. (2022). Beneficial shifts in the gut bacterial community of gilthead seabream (Sparus aurata) juveniles supplemented with allium-derived compound propyl propane thiosulfonate (PTSO). Animals 12, 1821. doi: 10.3390/ani12141821
Rahmani A., Parizadeh L., Baud M., Francois Y., Bazire A., Rodrigues S., et al. (2023). Potential of marine strains of pseudoalteromonas to improve resistance of juvenile sea bass to pathogens and limit biofilm development. Probiot. Antimicro. Prot. doi: 10.1007/s12602-023-10180-5
Raulo A., Ruokolainen L., Lane A., Amato K., Knight R., Leigh S., et al. (2018). Social behaviour and gut microbiota in red-bellied lemurs (Eulemur rubriventer): In search of the role of immunity in the evolution of sociality. J. Anim. Ecol. 87, 388–399. doi: 10.1111/1365-2656.12781
Rigos G., Kogiannou D., Padrós F., Cristòfol C., Florio D., Fioravanti M., et al. (2021). Best therapeutic practices for the use of antibacterial agents in finfish aquaculture: a particular view on European seabass (Dicentrarchus labrax) and gilthead seabream (Sparus aurata) in Mediterranean aquaculture. Rev. Aquacult. 13, 1285–1323. doi: 10.1111/raq.12523
Roquigny R., Mougin J., Le Bris C., Bonnin-Jusserand M., Doyen P., Grard T. (2021). Characterization of the marine aquaculture microbiome: A seasonal survey in a seabass farm. Aquaculture 531, 735987. doi: 10.1016/j.aquaculture.2020.735987
Rud I., Kolarevic J., Holan A. B., Berget I., Calabrese S., Terjesen B. F. (2017). Deep-sequencing of the bacterial microbiota in commercial-scale recirculating and semi-closed aquaculture systems for Atlantic salmon post-smolt production. Aquacult. Eng. 78, 50–62. doi: 10.1016/j.aquaeng.2016.10.003
Savaş S., Kubilay A., Basmaz N. (2005). Effect of bacterial load in feeds on intestinal microflora of seabream (Sparus surata) larvae and juveniles. Israeli J. Aquacult. - Bamidgeh 57, 3–9. doi: 10.46989/001c.20395
Sehnal L., Brammer-Robbins E., Wormington A. M., Blaha L., Bisesi J., Larkin I., et al. (2021). Microbiome composition and function in aquatic vertebrates: small organisms making big impacts on aquatic animal health. Front. Microbiol. 12. doi: 10.3389/fmicb.2021.567408
Sekkin S., Kum C. (2011). Antibacterial drugs in fish farms: application and its effects. Recent Adv. Fish Farms 217. doi: 10.5772/26919
Seychelles L. H., Doiron K., Audet C., Tremblay R., Pernet F., Lemarchand K. (2013). Impact of arachidonic acid enrichment of live rotifer prey on bacterial communities in rotifer and larval fish cultures. Can. J. Microbiol. 59, 189–196. doi: 10.1139/cjm-2012-0564
Shannon P., Markiel A., Ozier O., Baliga N. S., Wang J. T., Ramage D., et al. (2003). Cytoscape: A software environment for integrated models of biomolecular interaction networks. Genome Res. 13, 2498–2504. doi: 10.1101/gr.1239303
Shields R. J. (2001). Larviculture of marine finfish in Europe. Aquaculture 200, 55–88. doi: 10.1016/S0044-8486(01)00694-9
Stojanov S., Berlec A., Štrukelj B. (2020). The influence of probiotics on the firmicutes/bacteroidetes ratio in the treatment of obesity and inflammatory bowel disease. Microorganisms 8, 1715. doi: 10.3390/microorganisms8111715
Subasinghe R., Bernoth E.-M. (2000). Disease control and health management: aquaculture development, health and wealth. (NACA/FAO).
Sullam K. E., Essinger S. D., Lozupone C. A., O’connor M. P., Rosen G. L., Knight R., et al. (2012). Environmental and ecological factors that shape the gut bacterial communities of fish: a meta-analysis. Mol. Ecol. 21, 3363–3378. doi: 10.1111/j.1365-294X.2012.05552.x
Svanevik C. S., Lunestad B. T. (2011). Characterisation of the microbiota of Atlantic mackerel (Scomber scombrus). Int. J. Food Microbiol. 151, 164–170. doi: 10.1016/j.ijfoodmicro.2011.08.016
Taniguchi A., Aoki R., Inoue I., Eguchi M. (2022). Succession of the intestinal bacterial community in Pacific bluefin tuna (Thunnus orientalis) larvae. PloS One 17, e0275211. doi: 10.1371/journal.pone.0275211
Tkavc R., Ausec L., Oren A., Gunde-Cimerman N. (2011). Bacteria associated with Artemia spp. along the salinity gradient of the solar salterns at Eilat (Israel). FEMS Microbiol. Ecol. 77, 310–321. doi: 10.1111/j.1574-6941.2011.01112.x
Turgay E., Steinum T. M., Eryalçın K. M., Yardımcı R. E., Karataş S. (2020). The influence of diet on the microbiota of live-feed rotifers (Brachionus plicatilis) used in commercial fish larviculture. FEMS Microbiol. Lett. 367, fnaa020. doi: 10.1093/femsle/fnaa020
Vadstein O., Attramadal K. J. K., Bakke I., Forberg T., Olsen Y., Verdegem M., et al. (2018a). Managing the microbial community of marine fish larvae: A holistic perspective for larviculture. Front. Microbiol. 9. doi: 10.3389/fmicb.2018.01820
Vadstein O., Attramadal K. J. K., Bakke I., Olsen Y. (2018b). K-selection as microbial community management strategy: A method for improved viability of larvae in aquaculture. Front. Microbiol. 9. doi: 10.3389/fmicb.2018.02730
Vadstein O., Bergh Ø., Gatesoupe F.-J., Galindo-Villegas J., Mulero V., Picchietti S., et al. (2013). Microbiology and immunology of fish larvae. Rev. Aquacult. 5, S1–S25. doi: 10.1111/j.1753-5131.2012.01082.x
Vadstein O., Mo T. A., Bergh Ø. (2004). “Microbial Interactions, Prophylaxis and Diseases,” in Culture of Cold-Water Marine Fish (Blackwell Publishing, Oxford: John Wiley & Sons, Ltd), 28–72. doi: 10.1002/9780470995617.ch3
Vadstein O., Øie G., Olsen Y., Salvesen I., Skjermo J., Skjåk-Bræk G. (1993). “A strategy to obtain microbial control during larval development of marine fish,” in Fish farming technology (Rotterdam, Netherlands: CRC Press), 69–75.
Vestrum R. I., Attramadal K. J. K., Winge P., Li K., Olsen Y., Bones A. M., et al. (2018). Rearing water treatment induces microbial selection influencing the microbiota and pathogen associated transcripts of cod (Gadus morhua) larvae. Front. Microbiol. 9. doi: 10.3389/fmicb.2018.00851
Vestrum R. I., Forberg T., Luef B., Bakke I., Winge P., Olsen Y., et al. (2022). Commensal and opportunistic bacteria present in the microbiota in atlantic cod (Gadus morhua) larvae differentially alter the hosts’ Innate immune responses. Microorganisms 10, 24. doi: 10.3390/microorganisms10010024
Wang A. R., Ran C., Ringø E., Zhou Z. G. (2018). Progress in fish gastrointestinal microbiota research. Rev. Aquacult. 10, 626–640. doi: 10.1111/raq.12191
Welter D. K., Ruaud A., Henseler Z. M., De Jong H. N., van Coeverden de Groot P., Michaux J., et al. (2021). Free-living, psychrotrophic bacteria of the genus psychrobacter are descendants of pathobionts. mSystems 6, e00258–e00221. doi: 10.1128/mSystems.00258-21
Wilkes Walburn J., Wemheuer B., Thomas T., Copeland E., O’Connor W., Booth M., et al. (2019). Diet and diet-associated bacteria shape early microbiome development in Yellowtail Kingfish (Seriola lalandi). Microbial. Biotechnol. 12, 275–288. doi: 10.1111/1751-7915.13323
Wilson B., Danilowicz B. S., Meijer W. G. (2008). The diversity of bacterial communities associated with atlantic cod gadus morhua. Microb. Ecol. 55, 425–434. doi: 10.1007/s00248-007-9288-0
Yang H. L., Sun Y. Z., Ma R. L., Li J. S., Huang K. P. (2011). Probiotic Psychrobacter sp. improved the autochthonous microbial diversity along the gastrointestinal tract of grouper Epinephelus coioides. J. Aquac. Res. Dev. S 1, 10.4172. doi: 10.4172/2155-9546.S1-001
Yaylacı E. U. (2022). Characterization of pseudoalteromonas sp. from aquaculture environment and optimization of fermentation culture parameters by RSM-based modeling. Turkish J. Fish. Aquat. Sci. 22, TRJFAS21726. doi: 10.4194/TRJFAS21726
Keywords: Sparus aurata, larvae, microbiome, bacteriome, rotated positioning, rolling, 16S rRNA, next-generation sequencing (NGS)
Citation: Bel Mokhtar N, Apostolopoulou G, Koumoundouros G, Tzokas K, Toskas K, Gourzioti E, Stathopoulou P and Tsiamis G (2024) Bacterial community structures and dynamics associated with rotated positioning syndrome in gilthead sea bream (Sparus aurata) larviculture. Front. Aquac. 2:1270932. doi: 10.3389/faquc.2023.1270932
Received: 01 August 2023; Accepted: 13 December 2023;
Published: 04 January 2024.
Edited by:
Prapansak Srisapoome, Kasetsart University, ThailandReviewed by:
Muhammed Duman, Bursa Uludağ University, TürkiyeRossanna Rodriguez-Canul, Center for Research and Advanced Studies - Mérida Unit, Mexico
Gayatri Tripathi, Central Institute of Fisheries Education (ICAR), India
Copyright © 2024 Bel Mokhtar, Apostolopoulou, Koumoundouros, Tzokas, Toskas, Gourzioti, Stathopoulou and Tsiamis. This is an open-access article distributed under the terms of the Creative Commons Attribution License (CC BY). The use, distribution or reproduction in other forums is permitted, provided the original author(s) and the copyright owner(s) are credited and that the original publication in this journal is cited, in accordance with accepted academic practice. No use, distribution or reproduction is permitted which does not comply with these terms.
*Correspondence: George Tsiamis, gtsiamis@upatras.gr; Panagiota Stathopoulou, panstath@upatras.gr