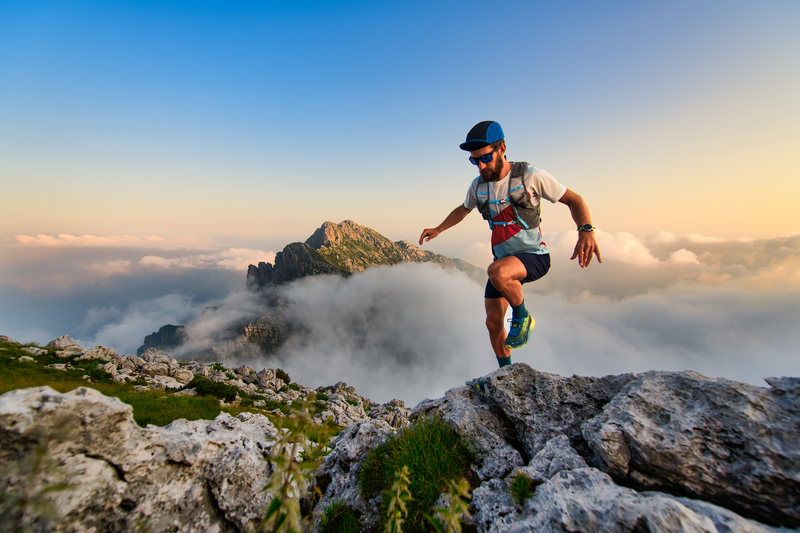
94% of researchers rate our articles as excellent or good
Learn more about the work of our research integrity team to safeguard the quality of each article we publish.
Find out more
ORIGINAL RESEARCH article
Front. Anim. Sci. , 12 November 2024
Sec. Animal Physiology and Management
Volume 5 - 2024 | https://doi.org/10.3389/fanim.2024.1485447
This study leverages Shotgun metagenomics to assess the rumen microbial community and functionality in Angus bulls with differing residual feed intake-expected progeny difference (RFI-EPD) values, aiming to elucidate the microbial contributions to feed efficiency. Negative RFI-EPD bulls (NegRFI: n=10; RFI-EPD= -0.3883 kg/d) and positive RFI-EPD bulls (PosRFI: n=10; RFI-EPD=0.2935 kg/d) were selected from a group of 59 Angus bulls (average body weight (BW) = 428 ± 18.8 kg; 350 ± 13.4 d of age) fed a high-forage total mixed ration after a 60-d testing period. At the end of the 60-d period, rumen fluid samples were collected for bacterial DNA extraction and subsequent shotgun metagenomic sequencing. Results of the metagenome analysis revealed greater gene richness in NegRFI bulls, compared to PosRFI. Analysis of similarity revealed a small but noticeable difference (P =0.052; R-value = 0.097) in the rumen microbial community of NegRFI and PosRFI bulls. Linear Discriminant Analysis effect size (Lefse) was utilized to identify the differentially abundant taxa. The Lefse results showed that class Fibrobacteria (LDA = 5.1) and genus Fibrobacter (LDA = 4.8) were greater in NegRFI bulls, compared to PosRFI bulls. Relative abundance of the carbohydrate-active enzymes was also compared using Lefse. The results showed greater relative abundance of glycoside hydrolases and carbohydrate-binding modules such as GH5, CBM86, CBM35, GH43, and CBM6 (LDA > 3.0) in NegRFI bulls whereas GH13 and GT2 were greater in PosRFI bulls. The distinct metabolic and microbial profiles observed in NegRFI, compared to PosRFI bulls, characterized by greater gene richness and specific taxa such as Fibrobacter, and variations in carbohydrate-active enzymes, underscore the potential genetic and functional differences in their rumen microbiome. These findings contribute to a deeper understanding of the interplay between rumen microbiota and feed efficiency in Angus bulls, opening avenues for targeted interventions and advancements in livestock management practices.
In the cattle industry, feed costs make up the largest part of production expenses causing efficient bull breeding is becoming increasingly more important (Wang et al., 2012). Even though feed costs are the main factor in profitability, genetic selection programs have usually focused on increasing weight gain rather than reducing feed intake (Alende et al., 2016). Furthermore, the beef cattle industry is facing more scrutiny due to environmental and economic concerns, leading to a push for more sustainable practices.
Residual feed intake (RFI), defined as the difference between actual and expected dry matter intake, is moderately heritable (h2 ≈ 0.35). This indicates that selecting for efficient or negative-RFI cattle will result in progeny that consume less feed compared to their less efficient or positive-RFI counterparts (Kenny et al., 2018; Arthur and Herd, 2008). Additionally, RFI is associated with various cattle traits such as mitochondrial efficiency and growth patterns (Idowu et al., 2022; Taiwo et al., 2022b). Expected Progeny Differences (EPDs) predict the genetic potential of offspring, and recent efforts have incorporated RFI into EPDs to select for more feed-efficient cattle. This integration removes environmental influences, allowing for comparisons within breeds across environments (Rossi et al., 2022; Beck, 2022). Research in this area offers opportunities for optimizing progeny without affecting bull reproductive parameters (Rossi et al., 2022).
While diet has long been considered the primary determinant of gut microbiota composition, recent research highlights the heritability of the cattle microbiome as a significant factor (Gonzalez-Recio et al., 2018). Ruminal fermentation is crucial for energy provision from feed, emphasizing the importance of feed efficiency (Guan et al., 2008). Progress in microbiome research has previously underscored the genetic influence on ruminal microbial composition and function. This research may further identify microbial signatures associated with feed efficiency, aiding targeted breeding and microbial selection programs to enhance overall beef cattle productivity (Li et al., 2019a).
Metagenomics is a powerful tool for unraveling microbial communities and their functional potential in diverse ecosystems (Handelsman, 2004; Yung et al., 2009). While next-generation sequencing has revolutionized genomics, choosing between Shotgun metagenomics sequencing and 16S rRNA gene sequencing is crucial. Although 16S rRNA gene sequencing provides taxonomic information, it is limited in resolving biological functions (Pace et al., 1986; Sharpton, 2014). Shotgun metagenomics sequencing offers a broader view of microbial diversity and functional capabilities, making it a preferred method for in-depth microbial studies (Basbas et al., 2023; Robinson et al., 2021). The integration of shotgun metagenomics into ruminant science has been reported to yield a more precise evaluation of microbial diversity and functional potential, as well as their impact on feed efficiency (Delgado et al., 2019; Xie et al., 2022). Thus, the objective of this study was to leverage deep Shotgun metagenomics sequencing to assess differences in the rumen microbial community and function in Angus bulls with negative or positive RFI-EPD.
The research procedures were approved by the West Virginia University’s Institutional Animal Care and Use Committee (IACUC Protocol Number: 2206054350). A group of 59 Angus bulls (average body weight (BW) = 428 ± 18.8 kg; 350 ± 13.4 d of age) were fed a high-forage total mixed ration (TMR; primarily consisting of corn silage, hay, cracked corn, and a ration-balancing supplement; see Table 1) for 60 days in two pens (pen 1: n = 31; pen 2: n = 28). Each pen was equipped with two GrowSafe8000 intake nodes (GrowSafe Systems Ltd., Airdrie, Alberta, Canada) to measure individual feed intake and In-Pen Weighing Positions (IPW, Vytelle LLC), positioned at a water trough in each pen to measure the body weight (BW) of individual animals several times daily (Wells et al., 2021). The use of IPW to measure BW has enabled the measurement of feed efficiency with sufficient accuracy over a test period of 59 days (MacNeil et al., 2021; Wells et al., 2021; Taiwo et al., 2022a). Following this test period, growth performance and feed intake data were collected. The phenotypic RFI values of the bulls were determined as described previously by Taiwo et al., 2022a. Briefly, the daily BW was regressed on time to calculate the beginning BW, mid-test BW, and average daily gain (ADG). Thereafter, ADG and metabolic mid-test BW (mid-test BW0.75) were regressed against individual daily DMI, and RFI was calculated as the difference between the predicted value of the regression and the actual measured value using the following equation; Y =β0 + β1X1 + β2X2 + ϵ, where Y is the dry matter intake (DMI; kg/d), β0 is the regression intercept, β1 and β2 are partial regression coefficients, X1 represents the metabolic mid-test BW (MMTW = mid test BW0.75; kg), and X2 is the average daily gain (ADG; kg/d) (Durunna et al., 2011; Taiwo et al., 2022a). Genetic evaluation of the bulls was performed by Vytelle (Vytelle Insight Beef Genetics) using data collected by Vytelle SENSE systems and Vytelle INSIGHT analytics services, which include at least three generations of pedigrees to determine the RFI-EPD values. At the conclusion of the trial and subsequent calculations, the bulls were ranked based on their Residual Feed Intake Expected Progeny Difference (RFI-EPD) coefficients. The bulls with the most negative RFI-EPD values (NegRFI; genetically efficient; n = 10) and the most positive RFI-EPD values (PosRFI; genetically inefficient; n = 10) were identified for further evaluation.
Rumen fluid samples were collected prior to morning feeding on day 60 of the testing period from all the bulls using an orally administered stomach tube connected to a vacuum pump (Ruminator; profs-products.com). The first 200 mL of rumen fluid was discarded to prevent saliva contamination. Then, 200 mL of fluid was collected from each animal and placed into 50-mL polypropylene conical bottom tubes. The samples were immediately placed on ice after collection and subsequently stored at −80°C until DNA extraction and sequencing were performed.
Before DNA extraction, rumen fluid samples from Angus bulls identified as NegRFI and PosRFI were thawed at room temperature. Microbial DNA was extracted from 500 µL of rumen fluid samples using the Qiagen DNeasy Powersoil Pro DNA Isolation Kit following the manufacturer’s instructions (Qiagen; catalog number: 47014, Germantown, MD, USA). Using a NanoDrop 2000 spectrophotometer (Thermo Fisher Scientific, Waltham, MA, USA), total DNA purity was measured, with an A260/A280 ratio ranging from 1.8 to 2.0. To prevent contamination, Kimtech wipes (Fisher scientific; catalog number 06-666, Pittsburg, PA, USA) were utilized throughout spectrophotometry quantification steps prior to each sample measurement. DNA was stored at −80°C until sequencing.
All subsequent steps were performed at Novogene Bioinformatics Technology (UC Davis Sequencing Center, CA; Batch ID: X202SC23094803-Z01-F001; Contract ID: H202SC23094803). For library preparation and construction, quality control (QC) of DNA samples was performed using agarose gel electrophoresis to assess DNA degradation and potential contamination. The concentration of DNA in the library was measured using a Qubit® 2.0 Fluorometer (Life Technologies, Carlsbad, CA, USA) with a Qubit® dsDNA Assay Kit (Thermo Fisher Scientific, Waltham, MA, USA). The purity of samples ranged from 1.8 to 2.0, with concentrations greater than 1 µg used to construct the library. Following library construction, the DNA was measured using the Qubit Fluorometric Quantification system (Thermo Fisher Scientific, Waltham, MA, USA). Genomic DNA of each sample was randomly sheared into short fragments (350 bp) for sequencing and processed using the Illumina NovaSeq 6000 PE150bp sequencing platform according to its effective concentration and expected data volume, as described by Li et al. (2022).
Preprocessing of raw data from the Illumina sequencing platform was performed to obtain clean data for subsequent analysis. Clean data were obtained by removing low-quality bases (default quality ≥ 38) exceeding the default length of 40 bp, reads containing N bases exceeding the default length of 10 bp, and reads with overlaps with adapters exceeding the default length of 15 bp. Subsequently, clean data were BLASTed against the host database (B. Taurus ARS-UCD2.0)., using Bowtie2 software (http://bowtie-bio.sourceforge.net/bowtie2/index.shtml) by default, followed by MEGAHIT software for assembly analysis of clean data. For Scaftigs without N assembly, resulting Scaffolds from the N junction were broken as previously reported (Qin et al., 2010; Li et al., 2015).
DIAMOND software (https://github.com/bbuchfink/diamond/; Buchfink et al., 2015) was used to align unigenes to the sequences of bacteria, fungi, archaea, and viruses from the non-redundant National Center for Biotechnology Information (NCBI) database. To prevent multiple alignment results and ensure species annotation and abundance of the sequences, a Lossless Compression Algorithm (LCA) was applied in the taxonomic software MEGAN. To form leveled taxonomic abundance tables, a relative abundance overview, and an abundance clustering heatmap, principal coordinate analysis (PCA) was performed. To identify differences between groups, analysis of similarities (ANOSIM), Metastat, and linear discriminant analysis (LDA, default score of 4) effect size (LEfSe) were conducted.
To evaluate the rumen microbial function, DIAMOND software (https://github.com/bbuchfink/diamond/) was used to BLAST Unigenes alignment with the functional database according to the default parameter settings of BLAST. The functional databases include KEGG (http://www.kegg.jp/kegg/) and CAZy (http://www.cazy.org/). Additionally, LEfSe analyses (LDA ≤ 2) of the functional differences between the two groups were conducted.
The growth performance data (DMI, average daily gain (ADG), initial and final BW) and RFI values of the PosRFI and NegRFI Angus bulls were analyzed using the GLIMMIX procedure of SAS version 9.4 (SAS Institute Inc., Cary, NC). Animals were included as a random effect nested within RFI-EPD. The RFI-EPD status was included as a fixed effect, and initial body weight values were included as a covariate for the final body weight. Significant effects were reported at P ≤ 0.05.
The results of the growth performance of the NegRFI and PosRFI bulls are shown in Table 2. The average RFI-EPD values of the NegRFI and PosRFI bulls were -0.39 kg/d and 0.29 kg/d, respectively. The initial and final body weights and ADG were similar (P > 0.05) between the groups. However, the DMI was lower (P = 0.0002) in the NegRFI bulls (7.74 kg/d) compared to the PosRFI bulls (9.90 kg/d).
A total of 132, 526, 840 raw reads were obtained from the 20 rumen fluid samples of the Angus bulls, with an average of 6,626,342.00 ± 815,282.6 raw reads per sample. After quality control and removal, 131,849, 910 clean reads, with 6,592,455 ± 803,592 reads per sample, were retained (Supplementary Table 1). Using Megahit, a total number of 6,993,174 scaftigs with an average of 349,659 ± 72,350 scaftigs per sample were obtained by interrupting scaffolds at the N-site, resulting in an average max length of 170,278bp (Supplementary Table 2).
The results revealed numerically greater gene richness in NegRFI bulls compared to PosRFI bulls (Figure 1). ANOSIM results revealed a small but noticeable difference (P = 0.052; R = 0.097) between the rumen microbial communities of the two groups (Figure 2). However, the results of the Metastats analysis identified a total of 35 differentially abundant taxa at the species level. The relative abundance of 29 species such as Fibrobacter UWB5, UWT2, UWB1, Trepomena C6AB and Trepomena JC4 were greater in NegRFI bulls, whereas 6 species such as Frischella japonica, Prevotella veroralis, and Helicobacter cetorum were more abundant in PosRFI bulls (Supplementary Figure 1). Abundance heatmaps showing the distribution of the 35 dominant taxa at the phylum and species levels are reported in Supplementary Figures 2, 3. Figure 3 shows the principal coordinate analysis (PCA) plot based on the differentially abundant (P < 0.05) species. Results of the LEfSe analysis showed the class Fibrobacteria (LDA = 5.1) and genus Fibrobacter (LDA = 4.8) as the most differentially enriched taxa in NegRFI bulls compared to PosRFI bulls (Figure 4).
Figure 1. Total number of non-redundant genes in PosRFI and NegRFI bulls. X-axis coordinate stands for each group and the Y-axis coordinate stands for the number of non-redundant genes. NegRFI, Angus bulls with negative residual feed intake; PosRFI, Angus bulls with positive residual feed intake.
Figure 2. Analysis of similarity (ANOSIM) of the rumen microbiome of NegRFI and PosRFI Angus bulls. NegRFI, Angus bulls with negative residual feed intake; PosRFI, Angus bulls with positive residual feed intake.
Figure 3. Principal component analysis (PCA) plot of the species whose abundance were significantly different between NegRFI and PosRFI (P ≤ 0.05). NegRFI, Angus bulls with negative residual feed intake; PosRFI, Angus bulls with positive residual feed intake.
Figure 4. Least discriminant analysis effects size (LEfSe) analysis of the rumen microbiota of NegRFI and PosRFI Angus bulls. The linear discriminant analysis (LDA) plot indicates the most differentially abundant taxa by ranking according to their effect size (LDA ≥ 4). NegRFI, Angus bulls with negative residual feed intake; PosRFI, Angus bulls with positive residual feed intake.
Utilizing the first-level KEGG orthology database, 46 core pathways belonging to 6 functional categories were identified within the rumen microbiome of the bulls. Predominant genes involved in carbohydrate metabolism, amino acid metabolism, translation, replication and repair, and folding, sorting and degradation were identified as the top 5 most abundant functions (Figure 5). No differences in KEGG functional pathways were detected between the two groups.
To identify the relative abundance of carbohydrate-active enzymes (CAZy), predicted genes were annotated to the CAZy database containing the following 6 classes: glycoside hydrolases (GH), glycosyltransferases (GT), polysaccharide lyases (PL), carbohydrate-binding modules (CBM), carbohydrate esterases (CE), and auxiliary activities (AA). The relative distribution of all annotated CAZy genes is shown in Figure 6. The LEfSe analysis of CAZy genes revealed that the relative abundance of GH5, CBM86, CBM35, GH43, and CBM6 (LDA ≥ 2.0; P ≤ 0.05) was greater in NegRFI bulls, whereas the relative abundance of GH13 and GT2 was greater in PosRFI bulls (LDA ≥ 2.0; P ≤ 0.05) (Figure 7).
Figure 7. Least discriminant analysis effects size (LEfSe) plot showing the differentially abundant carbohydrate-active enzymes between PosRFI (green) and NegRFI (red) Angus bulls (. NegRFI, Angus bulls with negative residual feed intake; PosRFI, Angus bulls with positive residual feed intake; GH13, glycoside hydrolases family 13; GT2, glycoside transferases family 2; GH5, glycoside hydrolases family 5; CBM86, carbohydrate-binding modules family 86; CBM35, carbohydrate-binding modules family 35; CBM43, glycoside hydrolases family 43; and CBM6, carbohydrate-binding modules family 6.
While the beef cattle production industry provides food and nutrition globally, feed efficiency is economically important for creating sustainable practices (de Ondarza and Tricarico, 2017; Stewart et al., 2018). Within ruminants, the influence of the rumen microbiome is crucial in facilitating feed intake, metabolism of carbohydrates, and energy utilization through the production of VFAs and nitrogen (Wang et al., 2013; Shabat et al., 2016; Wang et al., 2020). In this study, the quantitative gene abundance was greater in bulls identified as NegRFI. While little has been reported regarding a tendency of different gene richness in the rumen of cattle selected for feed efficiency, a study by Lima et al. (2019) reported a substantial link between rumen microbial abundances and feed intake, associating efficient cattle with greater gene abundance in the rumen while consuming less feed. Our research indicates an association between ruminal gene richness and genetic feed efficiency.
In cattle, the rumen serves as a station for diet fermentation, as it harbors a diverse microbial community that contributes to the breakdown of complex plant materials into absorbable nutrients, mediated by several mechanisms. These processes all contribute to cattle metabolic homeostasis (Sakata and Tamate, 1979; Lima et al., 2019). Relative to gene abundance, the expression of genes related to feed metabolism within the rumen microbiome significantly influences nutrient conversion and energy acquisition, ultimately impacting overall metabolic efficiency and production outcomes in cattle. The results of this study indicate greater gene richness in NegRFI bulls, which could suggest a greater ability to metabolize feed, requiring quantitatively less feed to exhibit the same production gain as their less efficient counterparts. However, conclusive results surrounding microbial diversity and gene richness in feed-efficient cattle are far from finalized. In a study conducted by Shabat et al. (2016), cattle identified as feed efficient revealed a less diverse and genetically rich microbial community. Nevertheless, while these cattle were reported as such, they were accompanied by a significantly higher dominance of certain taxa involved in energy metabolism and VFA production at the genus and species level, which complements our results. While the results surrounding microbial community and gene richness are still widely variable, feed-efficient cattle consistently possess dominant taxa that contribute to energy metabolism and production (Myer et al., 2015; Shabat et al., 2016; Auffret et al., 2020).
Cattle possess a diverse microbial community in their rumen, characterized by dominant phylogenetic taxa such as Firmicutes, Fibrobacterota, Bacteroidetes, and Proteobacteria, which are crucial for efficient digestion and nutrient metabolism (Jami and Mizrahi, 2012; Myer et al., 2015). While a core microbiome exists in the rumen, research has exhibited altered rumen microbial profiles in cattle selected for feed efficiency (Li et al., 2019b; Idowu et al., 2023). In our study, the microbial communities between PosRFI and NegRFI bulls were different and can be explained by the greater gene abundance in NegRFI bulls. Additionally, the relative abundance of 35 species belonging to taxa such as Fibrobacter, Lentisphaerae, Bacteroidetes, and Treponema were found to be different between the two groups of bulls.
The relative abundance of the genus Treponema and its species T. C6A8, T. JC4, and T. porcinum were greater in bulls identified as NegRFI, compared to PosRFI. Many Treponema species have been reported within the rumen of cattle and play a crucial role in the complex microbial ecosystem of the rumen; furthermore contributing to the efficient breakdown of fibrous plant materials and the production of energy-rich VFAs for the host animal (Radolf, 1996; Nordhoff et al., 2005; Rosewarne et al., 2012). The relative abundance of Bacteroidetes bacterium sp. Adurb.BinA104, Candidatus Endolissoclinum, and Lentisphaerae bacterium sp. GWF2528 were greater in NegRFI bulls compared to PosRFI bulls. These bacteria are classified as symbiotic proteobacteria and their phylogeny has previously been identified within the rumen of cattle divergent in feed efficiency (Kwan et al., 2012; Myer et al., 2015; Reis et al., 2023). Symbiotic proteobacteria contribute to ruminal fermentation through hydrolysis of complex polysaccharides, contributing to the formation and fermentation of biofilms, and have been previously reported to be involved in nucleotide, carbohydrate, and nitrogen metabolism (Hart et al., 2018; Zhang et al., 2021; Hernández et al., 2022). Schwartzia sp. succinovorans is a gram-negative bacterium that can ferment succinate to propionate in the rumen and was found in greater abundance in NegRFI bulls compared to PosRFI bulls (van Gylswyk et al., 1997). In ruminants, the production of VFAs is crucial in anaerobic digestion processes, serving as substrates for microbial metabolism (Bergman, 1990). Propionate and succinate use acetate: succinate transferases, which feed into the tricarboxylic acid (TCA) cycle, thereby potentially enhancing energy generation and microbial growth efficiency in the rumen (Kwong et al., 2017).
The relative abundance of species such as Fibrobacter UWB5, F. UWT2, and F. UWB were greater in NegRFI bulls compared to PosRFI bulls. Additionally, the genus Fibrobacter and the class Fibrobacteria were identified as the most enriched taxa in NegRFI Angus bulls. Fibrobacteria, particularly represented by the genus Fibrobacter and its species F. UWB5, F. UWT2, and F. UWB, are major cellulose-degrading bacteria. They break down plant material, including cellulose and hemicellulose, through the secretion of fibrolytic enzymes (Suen et al., 2011; Cammack et al., 2018). This enzymatic activity facilitates the breakdown of complex polysaccharides into simpler compounds, enhancing the accessibility of nutrients for both the microbial community and the host animal (Comtet-Marre et al., 2017; Jewell et al., 2013). A study by Neumann and Suen (2018) highlighted the importance of herbivore-associated Fibrobacter spp. within the gut microbiome of ruminant livestock, noting that species F. UWB5, F. UWT2, and F. UWB are associated with lignocellulose degradation.
Lignocellulose, an important building block in plant cell walls, is among the most abundant natural feedstocks in agriculture (Vázquez-Vuelvas et al., 2021). Cattle heavily rely on microbial digestion of plant lignocellulosic roughages, which make up major parts of their diets and consist mostly of lignin and three polysaccharides: cellulose, hemicellulose, and pectin (Naraian and Gautam, 2018; Gharechahi et al., 2023). The breakdown and conversion of complex plant material into polysaccharides within the rumen are suggested to support up to 70% of a ruminant animal’s daily energy requirements (Flint et al., 2008). This suggests that NegRFI bulls are able to break down and utilize feed more efficiently for increased energy production and metabolism, which likely explains their better feed efficiency.
The increased abundance of fiber-degrading species in the rumen of NegRFI bulls suggests an enhanced capacity to break down and utilize fibrous components of high-forage diets, compared to PosRFI bulls. This improved fiber degradation likely supports the ability of these bulls to grow at similar rates while consuming less feed compared to NegRFI bulls. Improved fiber degradative species in cattle selected for feed efficiency have previously been reported (McGovern et al., 2018; Elolimy et al., 2018; Liu et al., 2022). McGovern et al. (2018) employed 16S rRNA gene sequencing to further explore the relationship between the cellulolytic rumen microbiome and feed efficiency in bulls. Their analysis revealed a higher abundance of operational taxonomic units (OTUs) associated with fiber-degrading phyla in efficient RFI bulls, suggesting that the rumen bacterial community was adapted for enhanced fiber degradation, which may influence the feed efficiency phenotype. In a separate study, Elolimy et al. (2018) reported a higher abundance of cellulose-degrading bacteria in the rumen of low RFI beef steers, suggesting their role in improving feed digestibility and energy production in feed-efficient steers. These findings collectively indicate the importance of ruminal fiber-degrading communities in enhancing feed efficiency in cattle, suggesting that selecting for these microbial traits may contribute to the development of more feed-efficient herds fed high-forage diets.
Carbohydrate-active enzymes are essential for breaking down and utilizing complex carbohydrates in cattle feed (Abbott et al., 2018). In this study, we observed differences in the levels of certain enzymes, including glycoside hydrolases, glycoside transferases, and carbohydrate-binding modules, between two groups of Angus bulls. These enzymes are crucial components of the rumen microbiome and have been extensively studied for their biochemical and enzymatic properties (Lombard et al., 2014; Amin et al., 2021). Glycoside hydrolases, also known as glycosidases or glycosyl hydrolases, break glycosidic bonds in complex carbohydrates and play roles in antibacterial defense and pathogenesis in living organisms (Davies and Henrissat, 1995; Sjögren and Collin, 2014).
In our study, we found that the levels of GH5 and GH43 were higher in NegRFI bulls, while GH13 was more abundant in PosRFI bulls. GH5 proteins are one of the largest families and are commonly found in various environments (Henrissat et al., 1989; Dai et al., 2012). GH43 enzymes are known for their ability to break down biomass and are important in degrading plant cell walls (Cantarel et al., 2009; Mewis et al., 2016). Both GH5 and GH43 are involved in breaking down mannan bonds, a type of hemicellulose found in plant cell walls, into usable sugars (Aspeborg et al., 2012; Wang et al., 2013). This breakdown increases fermentable substrates available to rumen microorganisms, potentially increasing VFA production and microbial protein availability (Wang et al., 2019; Hua et al., 2022). The higher levels of GH5 enzymes in NegRFI bulls suggest increased energy substrates and nutrient availability, enhancing ruminal fermentation efficiency and nutrient utilization.
The GH13 family, also known as the α-amylase family, is responsible for rapidly converting starch into glucose and maltose (Bourne and Henrissat, 2001; Stam et al., 2006). Interestingly, despite being fed the same diet as NegRFI bulls, PosRFI bulls had higher levels of GH13, indicating differences in carbohydrate utilization mechanisms between the groups (Lin et al., 2019; Zhao et al., 2022). The relative abundance of GT2 was higher in PosRFI bulls. These enzymes are involved in the biosynthesis of structural molecules from sugar moieties, affecting the overall utilization of carbohydrates for energy substrates and production (Keenleyside et al., 2001).
The relative abundance of CBM86, CBM35, and CBM6 was higher in NegRFI bulls compared to PosRFI bulls. Carbohydrate-binding modules influence carbohydrate binding, degradation, and utilization by facilitating GH binding to carbohydrate structures (Boraston et al., 2004; Ficko-Blean and Boraston, 2006). The greater relative abundance of these enzymes in NegRFI bulls suggests increased enzymatic recognition and hydrolysis, potentially providing additional energy substrates to support better nutrient utilization.
The insights gleaned from this study carry significant implications for beef bull production and progeny, particularly concerning feed efficiency and the observed outcomes in our feed-efficient bulls. Understanding the intricate associations between rumen microbiome composition, host genetics and feed efficiency traits can inform targeted breeding strategies to select bulls with superior feed efficiency characteristics. Notably, Angus bulls classified as NegRFI exhibit higher gene richness, microbial diversity, and enzymatic functionality, indicative of a thriving microbiome adept at facilitating fermentative metabolism and enhancing nutrient availability. This microbial profile suggests the potential for more efficient energy partitioning towards growth, health, and production in NegRFI bulls. However, we acknowledge that this study does not allow for observing rumen microbiome changes over time, which could arise from shifts in diet and environmental factors. Longitudinal studies would provide a clearer understanding of how ruminal microbial communities evolve and influence feed efficiency under changing conditions. Additionally, broader metagenomic analyses across diverse breeds, age groups, and dietary regimes will provide valuable insights into optimizing beef cattle production systems for enhanced feed efficiency and sustainability.
The datasets presented in this study can be found in online repositories. The names of the repository/repositories and accession number(s) can be found below: https://www.ncbi.nlm.nih.gov/, sraPRJNA1151032.
The animal study was approved by Institutional Animal Care and Use Committee of West Virginia University (IACUC Protocol Number: 2206054350). The study was conducted in accordance with the local legislation and institutional requirements.
TS: Data curation, Formal analysis, Investigation, Methodology, Writing – original draft, Writing – review & editing. ET: Data curation, Investigation, Methodology, Writing – review & editing. GT: Data curation, Investigation, Methodology, Writing – review & editing. EF: Writing – review & editing. PF: Writing – review & editing. IO: Conceptualization, Data curation, Investigation, Methodology, Project administration, Resources, Supervision, Validation, Visualization, Writing – review & editing.
The author(s) declare financial support was received for the research, authorship, and/or publication of this article. The research study was funded by the U.S. Department of Agriculture hatch multi-state regional project W-3010 and West Virginia University Animal Health hatch project #923.
The authors declare that the research was conducted in the absence of any commercial or financial relationships that could be construed as a potential conflict of interest.
All claims expressed in this article are solely those of the authors and do not necessarily represent those of their affiliated organizations, or those of the publisher, the editors and the reviewers. Any product that may be evaluated in this article, or claim that may be made by its manufacturer, is not guaranteed or endorsed by the publisher.
The Supplementary Material for this article can be found online at: https://www.frontiersin.org/articles/10.3389/fanim.2024.1485447/full#supplementary-material
Abbott W., Alber O., bayer E., Berrin J.-G., Boraston A., Brumer H., et al. (2018). Ten years of CAZypedia: A living encyclopedia of carbohydrate-active enzymes. Glycobiology 28, 3–8. doi: 10.1093/glycob/cwx089
Alende M., Pordomingo A., Andrae J. G. (2016). RESIDUAL FEED INTAKE IN CATTLE: PHYSIOLOGICAL BASIS. A review. Rev. Argent. Producción Anim. 36, 49–56.
Amin K., Tranchimand S., Benvegnu T., Abdel-Razzak Z., Chamieh H. (2021). Glycoside hydrolases and glycosyltransferases from hyperthermophilic archaea: insights on their characteristics and applications in biotechnology. Biomolecules 11, 1557. doi: 10.3390/biom11111557
Arthur J. P. F., Herd R. M. (2008). Residual feed intake in beef cattle. Rev. Bras. Zootecnia 37, 269–279. doi: 10.1590/S1516-35982008001300031
Aspeborg H., Coutinho P. M., Wang Y., Brumer H., Henrissat B. (2012). Evolution, substrate specificity and subfamily classification of glycoside hydrolase family 5 (GH5). BMC Evolutionary Biol. 12, 186. doi: 10.1186/1471-2148-12-186
Auffret M. D., Stewart R. D., Dewhurst R. J., Duthie C.-A., Watson M., Roehe R. (2020). Identification of microbial genetic capacities and potential mechanisms within the rumen microbiome explaining differences in beef cattle feed efficiency. Front. Microbiol. 11. doi: 10.3389/fmicb.2020.01229
Basbas C., Garzon A., Schlesener C., van Heule M., Profeta R., Weimer B. C., et al. (2023). Unveiling the microbiome during post-partum uterine infection: A deep shotgun sequencing approach to characterize the dairy cow uterine microbiome. Anim. Microbiome 5, 59. doi: 10.1186/s42523-023-00281-5
Bergman E. N. (1990). Energy contributions of volatile fatty acids from the gastrointestinal tract in various species. Physiol. Rev. 70, 567–590. doi: 10.1152/physrev.1990.70.2.567
Boraston A. B., Bolam D. N., Gilbert H. J., Davies G. J. (2004). Carbohydrate-binding modules: Fine-tuning polysaccharide recognition. Biochem. J. 382, 769–781. doi: 10.1042/BJ20040892
Bourne Y., Henrissat B. (2001). Glycoside hydrolases and glycosyltransferases: Families and functional modules. Curr. Opin. Struct. Biol. 11, 593–600. doi: 10.1016/s0959-440x(00)00253-0
Buchfink B., Xie C., Huson D. H. (2015). Fast and sensitive protein alignment using DIAMOND. Nat. Methods 12, 59–60. doi: 10.1038/nmeth.3176
Cammack K. M., Austin K. J., Lamberson W. R., Conant G. C., Cunningham H. C. (2018). RUMINANT NUTRITION SYMPOSIUM: Tiny but mighty: the role of the rumen microbes in livestock production. J. Anim. Sci. 96, 752–770. doi: 10.1093/jas/skx053
Cantarel B. L., Coutinho P. M., Rancurel C., Bernard T., Lombard V., Henrissat B. (2009). The Carbohydrate-Active EnZymes database (CAZy): An expert resource for Glycogenomics. Nucleic Acids Res. 37, D233–D238. doi: 10.1093/nar/gkn663
Comtet-Marre S., Parisot N., Lepercq P., Chaucheyras-Durand F., Mosoni P., Peyretaillade E., et al. (2017). Metatranscriptomics reveals the active bacterial and eukaryotic fibrolytic communities in the rumen of dairy cow fed a mixed diet. Front. Microbiol. 8. doi: 10.3389/fmicb.2017.00067
Dai X., Zhu Y., Luo Y., Song L., Liu D., Liu L., et al. (2012). Metagenomic insights into the fibrolytic microbiome in yak rumen. PloS One 7, e40430. doi: 10.1371/journal.pone.0040430
Davies G., Henrissat B. (1995). Structures and mechanisms of glycosyl hydrolases. Structure (London England: 1993) 3, 853–859. doi: 10.1016/S0969-2126(01)00220-9
Delgado B., Bach A., Guasch I., González C., Elcoso G., Pryce J. E., et al. (2019). Whole rumen metagenome sequencing allows classifying and predicting feed efficiency and intake levels in cattle. Sci. Rep. 9, 11. doi: 10.1038/s41598-018-36673-w
de Ondarza M. B., Tricarico J. M. (2017). Review: advantages and limitations of dairy efficiency measures and the effects of nutrition and feeding management interventions. Prof. Anim. Sci. 33, 393–400. doi: 10.15232/pas.2017-01624
Durunna O. N., Mujibi F. D. N., Goonewardene L., Okine E. K., Basarab J. A., Wang Z., et al. (2011). Feed efficiency differences and reranking in beef steers fed grower and finisher diets1. J. Anim. Science. 89, 158–167. doi: 10.2527/jas.2009-2514
Elolimy A. A., Abdelmegeid M. K., McCann J. C., Shike D. W., Loor. J. J. (2018). Residual feed intake in beef cattle and its association with carcass traits, ruminal solid-fraction bacteria, and epithelium gene expression. J. Anim. Sci. Biotechnol. 9, 67. doi: 10.1186/s40104-018-0283-8
Ficko-Blean E., Boraston A. B. (2006). The interaction of a carbohydrate-binding module from a Clostridium perfringens N-acetyl-beta-hexosaminidase with its carbohydrate receptor. J. Biol. Chem. 281, 37748–37757. doi: 10.1074/jbc.M606126200
Flint H. J., Bayer E. A., Rincon M. T., Lamed R., White B. A. (2008). Polysaccharide utilization by gut bacteria: Potential for new insights from genomic analysis. Nat. Rev. Microbiol. 6, 121–131. doi: 10.1038/nrmicro1817
Gharechahi J., Vahidi M. F., Sharifi G., Ariaeenejad S., Ding X.-Z., Han J.-L., et al. (2023). Lignocellulose degradation by rumen bacterial communities: New insights from metagenome analyses. Environ. Res. 229, 115925. doi: 10.1016/j.envres.2023.115925
Gonzalez-Recio O., Zubiria I., García-Rodríguez A., Hurtado A., Atxaerandio R. (2018). Short communication: Signs of host genetic regulation in the microbiome composition in 2 dairy breeds: Holstein and Brown Swiss. J. Dairy Sci. 101, 2285–2292. doi: 10.3168/jds.2017-13179
Guan L. L., Nkrumah J. D., Basarab J. A., Moore S. S. (2008). Linkage of microbial ecology to phenotype: Correlation of rumen microbial ecology to cattle’s feed efficiency. FEMS Microbiol. Lett. 288, 85–91. doi: 10.1111/j.1574-6968.2008.01343.x
Handelsman J. (2004). Metagenomics: application of genomics to uncultured microorganisms. Microbiol. Mol. Biol. Rev. 68, 669–685. doi: 10.1128/MMBR.68.4.669-685.2004
Hart E. H., Creevey C. J., Hitch T., Kingston-Smith A. H. (2018). Meta-proteomics of rumen microbiota indicates niche compartmentalisation and functional dominance in a limited number of metabolic pathways between abundant bacteria. Sci. Rep. 8, 10504. doi: 10.1038/s41598-018-28827-7
Henrissat B., Claeyssens M., Tomme P., Lemesle L., Mornon J. P. (1989). Cellulase families revealed by hydrophobic cluster analysis. Gene 81, 83–95. doi: 10.1016/0378-1119(89)90339-9
Hernández R., Chaib De Mares M., Jimenez H., Reyes A., Caro-Quintero A. (2022). Functional and phylogenetic characterization of bacteria in bovine rumen using fractionation of ruminal fluid. Front. Microbiol. 13. doi: 10.3389/fmicb.2022.813002
Hua D., Hendriks W. H., Xiong B., Pellikaan W. F. (2022). Starch and cellulose degradation in the rumen and applications of metagenomics on ruminal microorganisms. Animals: Open Access J. MDPI 12, 3020. doi: 10.3390/ani12213020
Idowu M. D., Taiwo G., Pech Cervantes A., Bowdridge S. A., Ogunade I. M. (2022). Effects of a multicomponent microbial feed additive containing prebiotics and probiotics on health, immune status, metabolism, and performance of newly weaned beef steers during a 35-d receiving period. Trans. Anim. Sci. 6, txac053. doi: 10.1093/tas/txac053
Idowu M., Taiwo G., Sidney T., Treon E., Leal Y., Ologunagba D., et al. (2023). Effects of rumen-bypass protein supplement on growth performance, hepatic mitochondrial protein complexes, and hepatic immune gene expression of beef steers with divergent residual feed intake (p. 2023.10.19.563076). bioRxiv 19 (7), e0293718. doi: 10.1101/2023.10.19.563076
Jami E., Mizrahi I. (2012). Composition and Similarity of Bovine Rumen Microbiota across Individual Animals. PLOS ONE. 7, e33306. doi: 10.1371/journal.pone.0033306
Jewell K. A., Scott J. J., Adams S. M., Suen G. (2013). A phylogenetic analysis of the phylum Fibrobacteres. Systematic Appl. Microbiol. 36, 376–382. doi: 10.1016/j.syapm.2013.04.002
Keenleyside W. J., Clarke A. J., Whitfield C. (2001). Identification of residues involved in catalytic activity of the inverting glycosyl transferase wbbE from salmonella enterica serovar borreze. J. Bacteriology 183, 77–85. doi: 10.1128/JB.183.1.77-85.2001
Kenny D. A., Fitzsimons C., Waters S. M., McGee M. (2018). Invited review: Improving feed efficiency of beef cattle - the current state of the art and future challenges. Animal: Int. J. Anim. Bioscience 12, 1815–1826. doi: 10.1017/S1751731118000976
Kwan J. C., Donia M. S., Han A. W., Hirose E., Haygood M. G., Schmidt E. W. (2012). Genome streamlining and chemical defense in a coral reef symbiosis. Proc. Natl. Acad. Sci. United States America 109, 20655–20660. doi: 10.1073/pnas.1213820109
Kwong W. K., Zheng H., Moran N. A. (2017). Convergent evolution of a modified, acetate-driven TCA cycle in bacteria. Nat. Microbiol. 2, 17067. doi: 10.1038/nmicrobiol.2017.67
Li F., Hitch T. C. A., Chen Y., Creevey C. J., Guan L. L. (2019a). Comparative metagenomic and metatranscriptomic analyses reveal the breed effect on the rumen microbiome and its associations with feed efficiency in beef cattle. Microbiome 7, 6. doi: 10.1186/s40168-019-0618-5
Li F., Li C., Chen Y., Liu J., Zhang C., Irving B., et al. (2019b). Host genetics influence the rumen microbiota and heritable rumen microbial features associate with feed efficiency in cattle. Microbiome 7, 92. doi: 10.1186/s40168-019-0699-1
Li D., Liu C.-M., Luo R., Sadakane K., Lam T.-W. (2015). MEGAHIT: An ultra-fast single-node solution for large and complex metagenomics assembly via succinct de Bruijn graph. Bioinformatics 31, 1674–1676. doi: 10.1093/bioinformatics/btv033
Li L., Yang K., Li C., Zhang H., Yu H., Chen K., et al. (2022). Metagenomic shotgun sequencing and metabolomic profiling identify specific human gut microbiota associated with diabetic retinopathy in patients with type 2 diabetes. Front. Immunol. 13. doi: 10.3389/fimmu.2022.943325
Lima J., Auffret M. D., Stewart R. D., Dewhurst R. J., Duthie C.-A., Snelling T. J., et al. (2019). Identification of rumen microbial genes involved in pathways linked to appetite, growth, and feed conversion efficiency in cattle. Front. Genet. 10. doi: 10.3389/fgene.2019.00701
Lin L., Xie F., Sun D., Liu J., Zhu W., Mao S. (2019). Ruminal microbiome-host crosstalk stimulates the development of the ruminal epithelium in a lamb model. Microbiome 7, 83. doi: 10.1186/s40168-019-0701-y
Liu Y., Wu H., Chen W., Liu C., Meng Q., Zhou Z. (2022). Rumen microbiome and metabolome of high and low residual feed intake angus heifers. Front. Vet. Sci. 9. doi: 10.3389/fvets.2022.812861
Lombard V., Golaconda Ramulu H., Drula E., Coutinho P. M., Henrissat B. (2014). The carbohydrate-active enzymes database (CAZy) in 2013. Nucleic Acids Res. 42, D490–D495. doi: 10.1093/nar/gkt1178
MacNeil M. D., Berry D. P., Clark S. A., Crowley J. J., Scholtz M. M. (2021). Evaluation of partial body weight for predicting body weight and average daily gain in growing beef cattle. Trans. Anim. Sci. 5, txab126. doi: 10.1093/tas/txab126
McGovern E., Kenny D. A., McCabe M. S., Fitzsimons C., McGee M., Kelly A. K., et al. (2018). 16S rRNA sequencing reveals relationship between potent cellulolytic genera and feed efficiency in the rumen of bulls. Front. Microbiol. 9. doi: 10.3389/fmicb.2018.01842
Mewis K., Lenfant N., Lombard V., Henrissat B. (2016). Dividing the large glycoside hydrolase family 43 into subfamilies: A motivation for detailed enzyme characterization. Appl. Environ. Microbiol. 82, 1686–1692. doi: 10.1128/AEM.03453-15
Myer P. R., Smith T. P. L., Wells J. E., Kuehn L. A., Freetly H. C. (2015). Rumen microbiome from steers differing in feed efficiency. PloS One 10, e0129174. doi: 10.1371/journal.pone.0129174
Naraian R., Gautam R. (2018). “Penicillium enzymes for the saccharification of lignocellulosic feedstocks,” in New and Future Developments in Microbial Biotechnology and Bioengineering. doi: 10.1016/B978-0-444-63501-3.00006-5
Neumann A. P., Suen G. (2018). The phylogenomic diversity of herbivore-associated fibrobacter spp. Is correlated to lignocellulose-degrading potential. mSphere 3, e00593–e00518. doi: 10.1128/mSphere.00593-18
Nordhoff M., Taras D., Macha M., Tedin K., Busse H.-J., Wieler L. H. (2005). Treponema berlinense sp. Nov. And Treponema porcinum sp. Nov., novel spirochaetes isolated from porcine faeces. Int. J. Systematic Evolutionary Microbiol. 55, 1675–1680. doi: 10.1099/ijs.0.63388-0
Pace N. R., Stahl D. A., Lane D. J., Olsen G. J. (1986). “The analysis of natural microbial populations by ribosomal RNA sequences,” in Advances in Microbial Ecology. Ed. Marshall K. C. (Springer, Boston, MA: Springer US), 1–55. doi: 10.1007/978-1-4757-0611-6_1
Qin J., Li R., Raes J., Arumugam M., Burgdorf K. S., Manichanh C., et al. (2010). A human gut microbial gene catalogue established by metagenomic sequencing. Nature 464, 59–65. doi: 10.1038/nature08821
Radolf J. D. (1996). “Treponema,” in Medical Microbiology, 4th ed. Ed. Baron S. (Galveston, TX: University of Texas Medical Branch at Galveston). Available at: http://www.ncbi.nlm.nih.gov/books/NBK7716/.
Reis I. A., de Souza M. G., Granja-Salcedo Y. T., de Carvalho I. P. C., Porcionato M. A. D. F., Prados L. F., et al. (2023). Effect of post-ruminal urea supply on growth performance of grazing nellore young bulls at dry season. Animals 13, 207. doi: 10.3390/ani13020207
Robinson S. L., Piel J., Sunagawa S.. (2021) A roadmap for metagenomic enzyme discovery. Natural Product Rep. 38, 1994–2023. doi: 10.1039/d1np00006c
Rosewarne C. P., Cheung J. L., Smith W. J. M., Evans P. N., Tomkins N. W., Denman S. E., et al. (2012). Draft genome sequence of treponema sp. Strain JC4, a novel spirochete isolated from the bovine rumen. J. Bacteriology. 194, 4130–4130. doi: 10.1128/jb.00754-12
Rossi G. F., Bastos N. M., Vrisman D. P., Rodrigues N. N., Vantini R., Garcia J. M., et al. (2022). Growth performance, reproductive parameters and fertility measures in young Nellore bulls with divergent feed efficiency. Anim. Reprod. 19, e20220053. doi: 10.1590/1984-3143-AR2022-0053
Sakata T., Tamate H. (1979). Rumen epithelium cell proliferation accelerated by propionate and acetate. J. Dairy Sci. 62, 49–52. doi: 10.3168/jds.S0022-0302(79)83200-2
Shabat S. K. B., Sasson G., Doron-Faigenboim A., Durman T., Yaacoby S., Berg Miller M. E., et al. (2016). Specific microbiome-dependent mechanisms underlie the energy harvest efficiency of ruminants. ISME J. 10, 2958–2972. doi: 10.1038/ismej.2016.62
Sharpton T. (2014). An introduction to the analysis of shotgun metagenomic data. Front. Plant Sci. 6. doi: 10.3389/fpls.2014.00209
Sjögren J., Collin M. (2014). Bacterial glycosidases in pathogenesis and glycoengineering. Future Microbiol. 9, 1039–1051. doi: 10.2217/fmb.14.71
Stam M. R., Danchin E. G. J., Rancurel C., Coutinho P. M., Henrissat B. (2006). Dividing the large glycoside hydrolase family 13 into subfamilies: Towards improved functional annotations of alpha-amylase-related proteins. Protein Engineering Design Selection: PEDS 19, 555–562. doi: 10.1093/protein/gzl044
Stewart R. D., Auffret M. D., Warr A., Wiser A. H., Press M. O., Langford K. W., et al. (2018). Assembly of 913 microbial genomes from metagenomic sequencing of the cow rumen. Nat. Commun. 9, 870. doi: 10.1038/s41467-018-03317-6
Suen G., Weimer P. J., Stevenson D. M., Aylward F. O., Boyum J., Deneke J., et al. (2011). The complete genome sequence of Fibrobacter succinogenes S85 reveals a cellulolytic and metabolic specialist. PloS One 6, e18814. doi: 10.1371/journal.pone.0018814
Taiwo G., Idowu M., Collins S., Sidney T., Wilson M., Pech-Cervantes A., et al. (2022a). Chemical group-based metabolome analysis identifies candidate plasma biomarkers associated with residual feed intake in beef steers. Front. Anim. Sci. 2. doi: 10.3389/fanim.2021.783314
Taiwo G., Idowu M. D., Wilson M., Pech-Cervantes A., Estrada-Reyes Z. M., Ogunade I. M. (2022b). Residual feed intake in beef cattle is associated with differences in hepatic mRNA expression of fatty acid, amino acid, and mitochondrial energy metabolism genes. Front. Anim. Sci. 3. doi: 10.3389/fanim.2022.828591
van Gylswyk N. O., Hippe H., Rainey F. A. (1997). Schwartzia succinivorans gen. Nov., sp. Nov., another ruminal bacterium utilizing succinate as the sole energy source. Int. J. Systematic Bacteriology 47, 155–159. doi: 10.1099/00207713-47-1-155
Vázquez-Vuelvas O., Cervantes Chávez J., Delgado Virgen F., Valdez-Velazquez L., Osuna-Cisneros R. (2021). Fungal bioprocessing of lignocellulosic materials for biorefinery. Recent Adv. Microb. Biotechnol. 171–208. doi: 10.1016/B978-0-12-822098-6.00009-4
Wang Z., Colazo M. G., Basarab J. A., Goonewardene L. A., Ambrose D. J., Marques E., et al. (2012). Impact of selection for residual feed intake on breeding soundness and reproductive performance of bulls on pasture-based multisire mating. J. Anim. Sci. 90, 2963–2969. doi: 10.2527/jas.2011-4521
Wang L., Hatem A., Catalyurek U. V., Morrison M., Yu Z. (2013). Metagenomic insights into the carbohydrate-active enzymes carried by the microorganisms adhering to solid digesta in the rumen of cows. PloS One 8, e78507. doi: 10.1371/journal.pone.0078507
Wang L., Zhang G., Li Y., Zhang Y. (2020). Effects of high forage/concentrate diet on volatile fatty acid production and the microorganisms involved in VFA production in cow rumen. Animals: Open Access J. MDPI 10, 223. doi: 10.3390/ani10020223
Wang L., Zhang G., Xu H., Xin H., Zhang Y. (2019). Metagenomic analyses of microbial and carbohydrate-active enzymes in the rumen of Holstein cows fed different forage-to- concentrate ratios. Front. Microbiol. 10. doi: 10.3389/fmicb.2019.00649
Wells R. S., Interrante S. M., Sakkuma S. S., Walker R. S., Butler T. J. (2021). Accuracy of the VYTELLE SENSE in-pen weighing positions. Appl. Anim. Sci. 37, 626–634. doi: 10.15232/aas.2021-02183
Xie Y., Sun H., Xue M., Liu. J. (2022). Metagenomics reveals differences in microbial composition and metabolic functions in the rumen of dairy cows with different residual feed intake. Anim. Microbiome. 4, 19. doi: 10.1186/s42523-022-00170-3
Yung P. Y., Burke C., Lewis M., Egan S., Kjelleberg S., Thomas T. (2009). Phylogenetic screening of a bacterial, metagenomic library using homing endonuclease restriction and marker insertion. Nucleic Acids Res. 37, e144. doi: 10.1093/nar/gkp746
Zhang Y. K., Zhang X. X., Li F. D., Li C., Li G. Z., Zhang D. Y., et al. (2021). Characterization of the rumen microbiota and its relationship with residual feed intake in sheep. Animal 15, 100161. doi: 10.1016/j.animal.2020.100161
Keywords: ruminants, metagenomics, microbiome, feed efficiency, beef cattle and bull
Citation: Sidney T, Treon E, Taiwo G, Felton E, Fan P and Ogunade IM (2024) Exploring the rumen microbial function in Angus bulls with divergent residual feed intake. Front. Anim. Sci. 5:1485447. doi: 10.3389/fanim.2024.1485447
Received: 23 August 2024; Accepted: 24 October 2024;
Published: 12 November 2024.
Edited by:
Ignacio R. Ipharraguerre, University of Kiel, GermanyReviewed by:
Vishal Suthar, Gujarat Biotechnology University, IndiaCopyright © 2024 Sidney, Treon, Taiwo, Felton, Fan and Ogunade. This is an open-access article distributed under the terms of the Creative Commons Attribution License (CC BY). The use, distribution or reproduction in other forums is permitted, provided the original author(s) and the copyright owner(s) are credited and that the original publication in this journal is cited, in accordance with accepted academic practice. No use, distribution or reproduction is permitted which does not comply with these terms.
*Correspondence: Ibukun M. Ogunade, SWJ1a3VuLm9ndW5hZGVAbWFpbC53dnUuZWR1
Disclaimer: All claims expressed in this article are solely those of the authors and do not necessarily represent those of their affiliated organizations, or those of the publisher, the editors and the reviewers. Any product that may be evaluated in this article or claim that may be made by its manufacturer is not guaranteed or endorsed by the publisher.
Research integrity at Frontiers
Learn more about the work of our research integrity team to safeguard the quality of each article we publish.