- 1Department of Animal Science, University of Tennessee, Knoxville, TN, United States
- 2Department of Large Animal Clinical Sciences, University of Tennessee, Knoxville, TN, United States
With an expected increase in population by 2050, the demand for high-quality protein for human consumption will increase. To improve the sustainability of US cattle production, producers and researchers have historically focused on improving the feed efficiency of steers through improvements in genetics, nutrition, and microbiome management, producing a more marketable beef product. However, without a successful pregnancy, there would be no marketable animal to feed. The objective of this study was to examine the impact of pregnancy on the rumen environment, microbial communities, and the correlation to feed efficiency status in Angus heifers. Utilizing 17 cannulated Angus heifers, feed efficiency status was determined using the GrowSafe 8000 feed intake monitoring system to monitor feed intake for a 70d trial period to calculate residual feed intake. Following the trial, heifers were bred and 40mL rumen fluid and content samples were collected every two weeks during gestation. Metagenomic DNA was extracted from the rumen samples, sequencing libraries were prepared targeting the bacterial V4 region of the 16S rRNA gene, and resultant libraries were sequenced on an Illumina MiSeq. All microbial analyses were conducted in the R environment v2.6.2 where alpha diversity, beta diversity, and differential abundances were determined. Metabolites were identified using ultra high-performance liquid chromatography high resolution mass spectrometry, and Metabolomic Analysis and Visualization Engine was used to select metabolite peaks. Differential abundance analyses using “ANCOMBC” indicated 10 amplicon sequence variants (ASVs), assigned to seven Prevotella and three Succiniclasticum, significantly varied among weeks of gestation (P<0.05). Based on a one-way ANOVA, 90 metabolites were determined significant throughout pregnancy (P<0.05). The 10 metabolites with the highest variable importance of projection scores from a partial least squares discriminant analysis were used to calculate correlations between. Of the 10 ASVs and 10 metabolites, significant correlations with feed efficiency status were found at various time points (P<0.05). The rumen microbiome and its fermentative profile were different at various time points during pregnancy with several correlations to feed efficiency status. Identifying pregnancy-related microbial and metabolite changes may aid producers to anticipate nutritional or management adjustments, ultimately contributing to the sustainability of beef production.
1 Introduction
Improving sustainability across the US beef industry is important due to the expected increased demand for high-quality protein sources. The beef industry must continue to produce environmentally sound, socially responsible, and economically viable beef (Beef USRfS, 2022). Roughly 70% of beef cattle production costs are associated with feed procurement and forage production (Stewart et al., 2010). To improve upon the profit margins and sustainability of beef production, the efficient use of these inputs are necessary for effective production, including costs, arable land, and natural resources (Beef USRfS, 2022). With rising input costs and decreases in arable land to produce feed and forages, the importance of feed efficient cattle is paramount for sustainable agriculture.
Bovine feed efficiency considers their unique ability to convert forages, a low-quality nutrient source for humans, into a high-quality end product, such as meat or milk. Ultimately, the rumen microbiome is responsible for the fermentation, conversion, and processing of feedstuffs into usable compounds for the ruminant to absorb and utilize for energy (Russell et al., 1992; Mackie et al., 2000). Research regarding the rumen microbiome to date has focused heavily on defining the relationship between feed efficiency and the rumen microbiome to further describe sources of variation in feed efficiency (Myer et al., 2015; Clemmons et al., 2019a; Li et al., 2019). Myer and others (Myer et al., 2015) identified specific genera such as Succiniclasticum, Lactobacillus, Ruminococcus, multiple Prevotella, and the family Veillonellaceae with abundance differences in steers divergent in feed efficiency. Metabolic and microbial biomarkers have also been implicated to impact feed efficiency. Abundances of the microbially-derived metabolite pantothenate and pantothenate-producing Flavobacteriia were significantly different in steers with differing feed efficiency status (Clemmons et al., 2019a). To date, research has been thorough in defining the relationship of the rumen environment in steers and fed cattle.
To be considered productive, a cow must raise a calf every year. Reproductive failures, such as failure to conceive or pregnancy losses, account for $471 million annually (Bellows et al., 2002). The emphasis on maintaining health during pregnancy has the utmost importance from a profitability standpoint, as without the resultant calf, there would be no opportunity for income. Additionally, a more feed efficient dam has the potential to reduce her own management costs while providing genetic improvement in nutrient utilization in resultant offspring. The physiological changes that occur during pregnancy (Winters et al., 1942; Swett et al., 1948; Lyne, 1960; Ferrell et al., 1976; Prior and Laster, 1979), and ultimately the suggested changes in nutritional requirements and intake (LeBlanc, 2006), could influence the gut microbiome leading to alterations in metabolic outputs, but the relationship between pregnancy and the rumen environment remains unclear. Understanding this relationship may offer insights that can enhance cattle management practices, particularly by informing tailored nutritional strategies to meet the heightened physiological demands during gestation. These insights can improve reproductive success and feed efficiency, supporting healthier, more productive animals with fewer resources.
The research focus on the rumen microbiome of fed cattle has likely been driven by economics, as the majority of revenue in the US beef industry is derived from the sale of beef as a consumable commodity (United States Department of Agriculture ERC, 2021). However, limited work exists regarding rumen microbiome impacts on the cow calf sector of the beef industry. The effect of pregnancy on rumen health, especially in relation to the feed efficiency status of the female has yet to be clarified. Understanding of the cow’s feed efficiency status and rumen microbiome will inform genetic selection and enhance ability to produce the next generation of highly productive beef cattle required to meet the increasing demand for a quality protein source such as red meat. The objectives of this study were to determine key changes in the rumen microbiome and environment during pregnancy in Angus heifers and determine the correlation of key ruminal environment changes to their previously established feed efficiency status.
2 Methods
This study was approved and carried out in accordance with the recommendations of the Institutional Animal Care and Use Committee at the University of Tennessee, Knoxville (protocol number 2639-0818).
2.1 Animals and sampling
2.1.1 Feed efficiency trial
For this study, 17 previously cannulated Angus heifers of approximately 2 years of age and 678 ± 54 kg were placed on a 70-day feed efficiency trial at the Plateau Research and Education Center, (Crossville, TN). Rumen fistulated and cannulated heifers were used to facilitate comprehensive rumen sampling. The trial utilized a GrowSafe System© 8000 (GrowSafe Systems Ltd., Airdrie, Alberta, Canada) to determine individual animal feed intake to calculate resultant feed efficiency status based on residual feed intake (RFI). As described by Clemmons et al (Clemmons et al., 2022), the diet was composed of corn silage and a custom blend based on guidelines from the National Research Council for beef cattle growth (National Academies of Sciences, Engineering, and Medicine, 2016). Body weights were recorded on days -1, 0, 35, 70, and 71 throughout the feed efficiency trial. Day 0 denoted the first day of the feed efficiency trial and was used to calculate average daily gain (ADG). Average daily feed intake (ADFI) and ADG were used to determine RFI and feed efficiency status (Koch et al., 1963; Clemmons et al., 2022).
2.1.2 Pregnancy samples
At the completion of the feeding study, heifers were transported to the East Tennessee Research and Education Center (Louisville, TN) and underwent a 7-day co-synch + CIDR protocol (Andersen et al., 2020). All heifers were artificially inseminated and exposed to two Angus bulls. Open cows (n = 4) were removed from the study after pregnancy confirmation via quantification of circulating pregnancy associated glycoproteins on day 30 (Oliveira Filho et al., 2020), and 13 heifers carried full term. After pregnancy confirmation, heifers were maintained on mixed cool season pasture with ad libitum access to a loose mineral supplement (#678, Co-op Supreme Cattle Mineral, Protrition Feeds, LaVergne, TN) and supplemented with mixed grass hay in winter months. Prior to breeding (Weeks -1 and 0) and beginning at week 4 post conception through calving, heifers had rumen content and fluid collected biweekly during gestation. Rumen content (40 mL) was removed from the ventral sac of the rumen via cannula access, placed into a conical tube, immediately flash frozen, and stored at -80°C. A second sample of 15 mL of rumen fluid was also collected for metabolomic profiling. Samples were not collected from animals that failed to meet health and wellness criteria on sampling days for reasons that include adverse behaviors, illness, and labor.
2.2 DNA extraction, library preparation, and sequencing of rumen content
Rumen samples were processed at the University of Tennessee, Knoxville for DNA extraction and purification. Microbial DNA was extracted and purified by the rumen digesta extraction protocol detailed by Yu and Morrison (2004). Briefly, approximately 0.2 g of rumen content was placed into a 2 mL beaded screw cap tube with 0.5 mm ZR bead bashing matrix (Zymo Research, Irvine, CA, USA). A total of 1 mL of lysis buffer (500 mM NaCl, 50mM Tris-HCl, pH 8.0, 50 mM EDTA, and 4% sodium dodecyl sulfate [SDS]) was added and homogenized for 3 minutes at 21 Hz and incubated at 70°C for 15 minutes with inversions of sample tubes at 5 minutes intervals. Samples were then centrifuged at 16,000 x g for 5 minutes and supernatants were transferred into separate 2 mL microcentrifuge tubes. The 300 μL of lysis buffer was added into the beaded screw cap tube, and the steps were repeated. After the final centrifugation, individual samples were pooled, and lysis tubes were discarded. A total of 260 μL of 10-M ammonium acetate was added to each individual sample for nucleic acid precipitation. Samples were mixed and placed on ice for 5 minutes. Tubes were then centrifuged at 4°C for 10 minutes at 16,000 x g. The supernatant was divided evenly into two fresh tubes and the same volume of isopropanol was added and mixed well. Samples were put on ice for 30 minutes before being centrifuged 4°C for 15 minutes at 16,000 x g resulting in a nucleic acid pellet formation. Excess supernatant was discarded, and the pellet was washed with 70% ethanol and dried for 5 minutes. The pellet was then dissolved in 100 μL of Tris-EDTA (TE) buffer and aliquots were pooled. After completion of nucleic acid precipitation, the QIAamp DNA Stool Mini Kit (QIAGEN, Valencia, CA, USA) was utilized for purification. A total of 15 μL of proteinase K and 200 μL of Buffer AL were added to eliminate protein contamination. Samples were then incubated at 70°C for 10 minutes and 200 μL of 100% ethanol was added and mixed before being transferred into a QIAamp column (QIAGEN, Valencia, CA, USA). Columns were centrifuged for 1 minute at 4°C at 16,000 x g. Flow through was discarded, and the process was repeated twice, with the addition of 500 μL of Buffer AW1 in the first repetition and 500 μL of Buffer AW2 the second. After discarding final flow through, columns were centrifuged for 1 minute at 16,000 x g to dry the column. Columns were then placed into fresh 1.5 mL tubes and 70 μL of Buffer AE was added to the column and incubated at room temperature for 2 minutes. Following the 2 minutes, 30 μL of Buffer AE was added and incubated at room temperature for 2 minutes. Finally, samples were centrifuged at 4°C for 1 minute at 16,000 x g and DNA was retained in 1.5 mL tubes. The quality of extracted DNA was confirmed via 2% agarose gel electrophoresis. Samples were stored at -20°C until amplification and library preparation.
Samples were thawed and diluted to 10 ng/μL in 1.5mL cryovials. Amplicon library preparation for the 16S rRNA gene was performed at the University of Tennessee Genomics Core Laboratory, following their standard operating procedures of a two-step polymerase chain reaction (PCR). The V4 hypervariable region of the 16S rRNA gene was amplified from extracted DNA, using primers 515Fb (GTGYCAGCMGCCGCGGTAA) (Parada et al., 2016) and 806Rb (GGACTACNVGGGTWTCTAAT) (Apprill et al., 2015), modified with adapters for Illumina MiSeq sequencing. The first PCR used the V4 amplicon primers (2 mM), Kapa HiFi master mix (Roche, Indianapolis, IN, USA), and 2.5 μL of DNA. The reaction consisted of 3 min at 95°C, followed by 25 cycles of 95°C for 30 s, 55°C for 30 s, and 72°C for 30 s, with a final extension at 72°C for 5 min. The PCR product was purified with 20 μL Agencourt Ampure XP beads (Beckman Coulter, Brea, CA, USA) and eluted in 50 μL of 10 mM Tris, then each sample was indexed with a unique combination of forward and reverse Nextera XT v2 indexes (Illumina, Inc., San Diego, CA, USA) using Kapa HiFi master mix, and 8 cycles of the above PCR. The indexed PCR product was again purified with 56 μL of Agencourt Ampure XP beads and eluted in 25 μL of Tris. Final libraries were visualized for quality control and quantified with a combination of nanodrop and Agilent Bioanalzyer (Agilent Technologies, Inc., Santa Clara, CA, USA). The libraries were sequenced at a final loading concentration of 4 pM on a v2 2 × 250 bp kit for the Illumina MiSeq.
2.3 Metabolite extraction and analysis of rumen content
After thawing from storage, 2 mL of rumen fluid were placed into two 2 mL microcentrifuge tubes and centrifuged at 6,000 x g for 15 minutes at 4°C. Following, the supernatant was aspirated from both tubes and pooled before being filtered through a 0.22 μM syringe filter (MidSci, St. Louis, MO, USA) and placed into a 2 mL cryovial. All samples were stored at -20°C until further analysis.
Samples were processed by the Biological and Small Molecule Mass Spectrometry Core (BSMMSC), University of Tennessee, Knoxville, TN (RRID: SCR_021368). Water soluble metabolites were extracted from 100 μL rumen content using 4:4:2 acetonitrile/methanol/water with 0.1 M formic acid as previously described by Domingo-Almenara and others (Domingo-Almenara et al., 2019). Following extraction, the supernatant was dried under N2 then resuspended in 300 μL water. All solvents used were HPLC grade and purchased from Fisher Scientific (Hampton, NH, USA).
To identify metabolites, ultra-high performance liquid chromatography high resolution mass spectrometry (UHPLC-HRMS) (Thermo Scientific, San Jose, CA, USA) was utilized using an untargeted metabolomics method by Lu and others (Lu et al., 2010). Metabolites were separated based on polarity by using reverse-phase (RP), ion-paring chromatography. Chromatographic separation utilized a Synergi Hydro RP column (100 mm x 2.1 mm, 2.6 μm, 100 Å) and an UltiMate 3000 pump (Thermo Fisher Scientific, Waltham, MA). Finally, mass analysis was performed by using Exactive Plus Orbitrap MS (Thermo Fisher Scientific, Waltham, MA) in conjunction with the UHPLC system.
2.4 16S rDNA gene sequence processing and analysis
An R pipeline as described by Callahan and others (Callahan et al., 2016b) was used to process resultant fastq reads. The R package ‘fastqcr’ v0.1.2 (Alboukadel, 2019) was used to assess quality. Samples with less than 10,000 reads were removed from the data (n=7). Open-source R packages ‘phyloseq’ v1.40.0 (McMurdie and Holmes, 2013) and ‘DADA2’ (Callahan et al., 2016a) were used to filter low-quality reads, call amplicon sequence variants (ASVs), and assign taxa. The parameters for filterandTrim were set with a truncLen of 240 for forward and reverse reads. Forward and reverse reads without a quality score of 25 or greater, more than 2 expected errors in a forward read, and more than 2 expected errors in a reverse read were removed (Edgar and Flyvbjerg, 2015). The learnErrors function in the ‘DADA2’ package v.1.24.0 (Callahan et al., 2016a) was utilized to identify error rates. ASVs were called by the dada function. ‘DADA2’ was then used to correct for amplicon errors, and forward and reverse reads were merged using the mergePairs function with a 12 base pair overlap requirement. Chimeras were removed from resultant sequence table by the removeBimeraDenova function. Taxonomy to the genus level was assigned using the SILVA v138.1 database (Quast et al., 2012) using assignTaxonomy. All assigned data were input into a phyloseq object for further analysis.
Alpha diversity was measured using the estimate_richness function from the ‘phyloseq’ package in R to calculate the observed ASVs, Chao1 metric, and Shannon Diversity Index. Beta diversity was measured by using a Bray-Curtis Dissimilarity Matrix to identify distances for a principal coordinate analysis (PCoA) in the ‘vegan’ package in R v2.6.2 (Oksanen et al., 2007).
2.5 Statistical analyses
Downstream analysis for bacterial reads, alpha- and beta-diversity were performed in R v2.6.2. A histogram was created to visually assess the normality, quality, and distribution of scores.
2.5.1 Alpha diversity
Visual assessment of alpha diversity measurements of Observed ASVs, Chao1, and the Shannon Diversity Index were performed before using a Shapiro-Wilks test (W) to test for normality at a W>0.85 and P>0.05. To identify differences in alpha diversity measurements, a linear mixed effects model was used. The resultant alpha diversity index including observed, Chao1, or Shannon, were tested as a response of time point, being week of measurement, and blocked by individual animal to account for repeated measurements. An ANOVA was then used to test differences across means in alpha diversity measurements. Pairwise comparisons between weeks were calculated using linear hypothesis testing and Tukey’s honest significance test. Alpha was set at 0.05 for all analyses.
2.5.2 Beta diversity
Samples were transformed from total counts and a Bray-Curtis Dissimilarity Matrix was used to determine distance between samples for beta diversity measurements. Dispersion estimates were calculated and a permutational multivariate analysis of variance (PERMANOVA) with 999 permutations was performed using the adonis function in the ‘vegan’ package in R (Oksanen et al., 2007) with significance of P<0.05 for all analyses. To test pairwise comparisons in the Bray-Curtis Dissimilarity Matrix between time points, the pairwise.adonis function was used (Martinez Arbizu, 2020).
2.5.3 Differential abundance
The ‘ANCOMBC’ package v2.0.2 (Lin and Peddada, 2020) was used to test for differential abundances of bacterial communities from each sample during progression of pregnancy. The phyloseq object was utilized in the ancombc2 function. The fixed effect was time point in pregnancy and the random effect was individual animal to adjust for repeated measures. Further, ASVs with prevalence less than 10% across samples were removed from the analysis. For this data set, there were no assumed structural zeroes. Pairwise comparisons were conducted and Benjamini-Hochberg (Benjamini and Hochberg, 1995) was used to adjust for multiple testing. The adjusted p-value was set to Q<0.05 for all analyses.
2.5.4 Metabolites
Metabolomic Analysis and Visualization Engine (MAVEN) was used to visualize raw UHPLC-HRMS data and identify rumen metabolites. Metabolites were identified based on the exact mass and retention time in accordance with an in-house database (Oksanen et al., 2007). Variable importance of projection (VIP) scores were determined in MetaboAnalyst v5.0 via partial least squares discriminant analysis.
Visualizations for ASV and metabolite abundances by week based on continuous RFI were generated in JMP v15.2.0 (SAS Institute Inc., Cary, NC, USA, 1989–2023).
2.5.5 Feed efficiency correlation analyses
Analyses to determine the relationship between ASV abundance, metabolite prevalence, and residual feed efficiency status were performed in MetaboAnalyst v5.0. The pattern search function using the Pearson R method was used to identify correlation patterns P<0.05 and Q<0.05.
3 Results
3.1 Animal data
At the completion of a 70-day feed efficiency trial, 13 cows received RFI values based on the metrics calculated from the GrowSafe 8000 system (GrowSafe Systems Ltd., Airdrie, Alberta, Canada). These values fell on a continuous scale from -3.3 to 3.5. A standard deviation of ±0.5 was used to determine RFI classification, where three animals ranked in the High-RFI category, eight animals ranked in the Mid-RFI category, and two animals ranked in the Low-RFI category (Table 1). However, for the analyses of the current study, the animals were not binned into respective groups, but analyzed on a continuous scale (Clemmons et al., 2022).
3.2 Sequencing results
An average of 80,353 read pairs per sample were produced from the libraries sequenced on the Illumina MiSeq. The minimum number of reads was 23,809 and the maximum was 164,552. A total of 32 unique phyla and 372 unique genera were identified from 207 samples. Overall, 88,410 taxa were identified.
3.3 Alpha diversity of bacterial communities
Alpha diversity analyses examining the observed ASVs (richness), Chao1 (anticipated richness), and Shannon Diversity Index (richness and evenness) among all weeks are displayed (Figures 1–3, respectively). The linear hypothesis of the pairwise comparisons of observed ASVs, Chao1, and Shannon Diversity Indices indicated numerous significant differences among weeks (P<0.05; Tables 2–4, respectively). The number of pairwise differences among weeks in all alpha diversity metrics increased throughout pregnancy.
3.4 Beta diversity of bacterial communities
When analyzing beta diversity using Bray-Curtis-based principal coordinates analysis, there was a significant difference among weeks after PERMANOVA analysis with 999 permutations (P<0.05; Figure 4). Circles represent a 95% confidence interval around the centroids of each time point. Pairwise comparisons of Bray-Curtis Dissimilarity Matrices indicated numerous significant differences among weeks (Table 5). Overall, fewer differences occurred during the end of the gestation period from Weeks 26-38, specifically when compared to weeks during the third trimester, indicating stage-dependent changes of the microbiome.
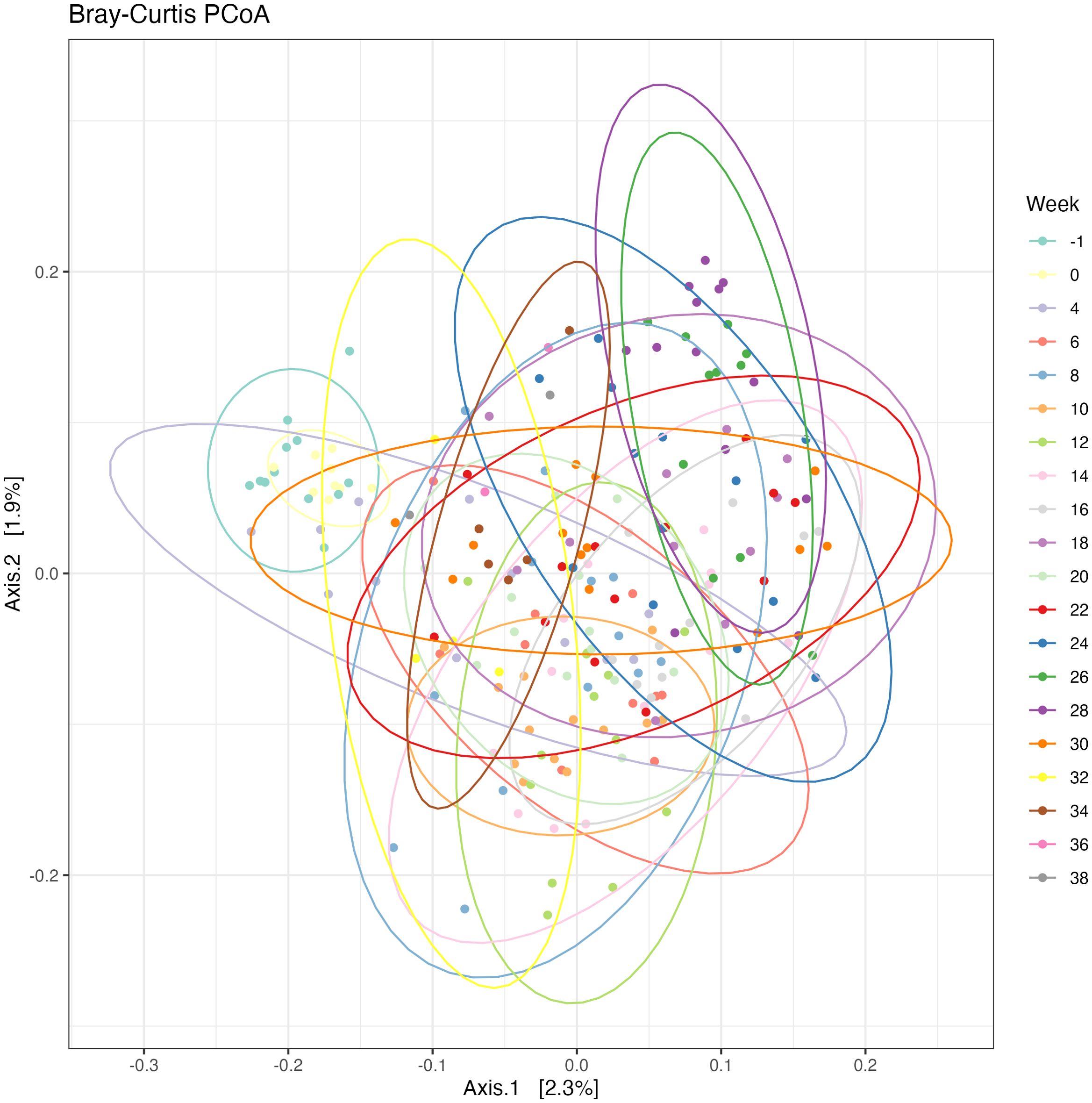
Figure 4. Bray-Curtis Dissimilarity Matrix PCoA with 95% confidence intervals. Bray-Curtis principal coordinates analysis (PCoA) describing the dissimilarity between weeks of pregnancy. Testing differences with a PERMANOVA with 999 permutations revealed differences in between sample diversity (P < 0.05).
3.5 Differential abundance analysis
Based on the global test in the ANCOM-BC analysis, 10 ASVs were confirmed to be differentially abundant across time points (Table 6; Q<0.05). At the genus level, seven ASVs were taxonomically classified as Prevotella and three as Succiniclasticum. Differential abundance pairwise comparisons indicated 25 significant comparisons (Table 7).
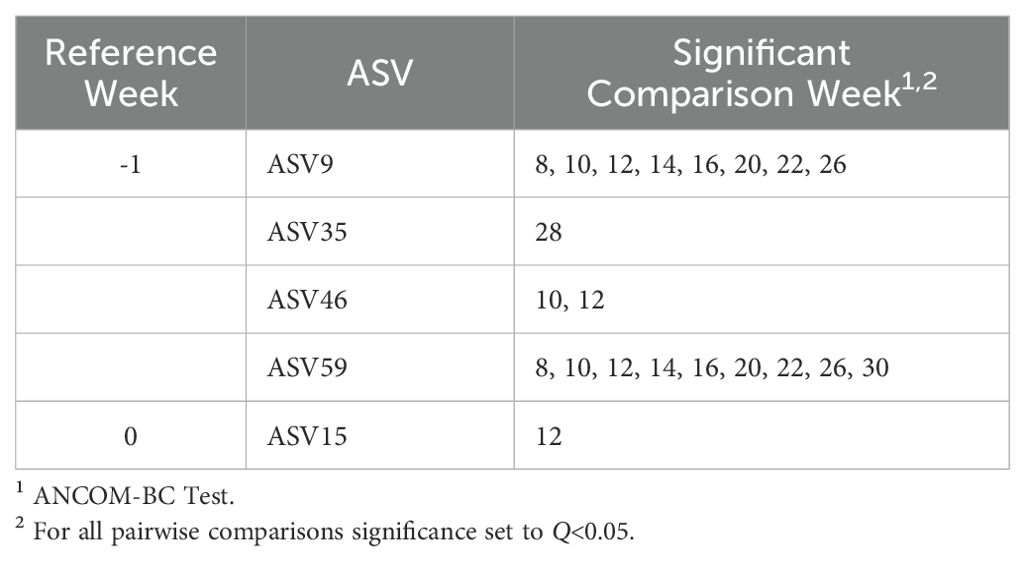
Table 7. Significant amplicon sequence variants (ASV) differential abundance pairwise comparison of weeks.
3.6 Rumen metabolites
After determining abundances of metabolites, a total of 90 metabolites were identified with significant difference across time points (Q<0.05; Supplementary Figure 1). The 10 metabolites with the highest VIP scores of the current study were utilized in final analysis (Figure 5) and included 3-hydroxyisovalerate, pimelic acid, 3-methylphenylacetic acid, salicylate, methyl succinic acid, ribose phosphate, 2_3-dihydroxybenzoate, myo-inositol, phenyllactic acid, and pantothenate. Metabolite abundance ratios changed by week, with reduced abundances overall during weeks 26-30.
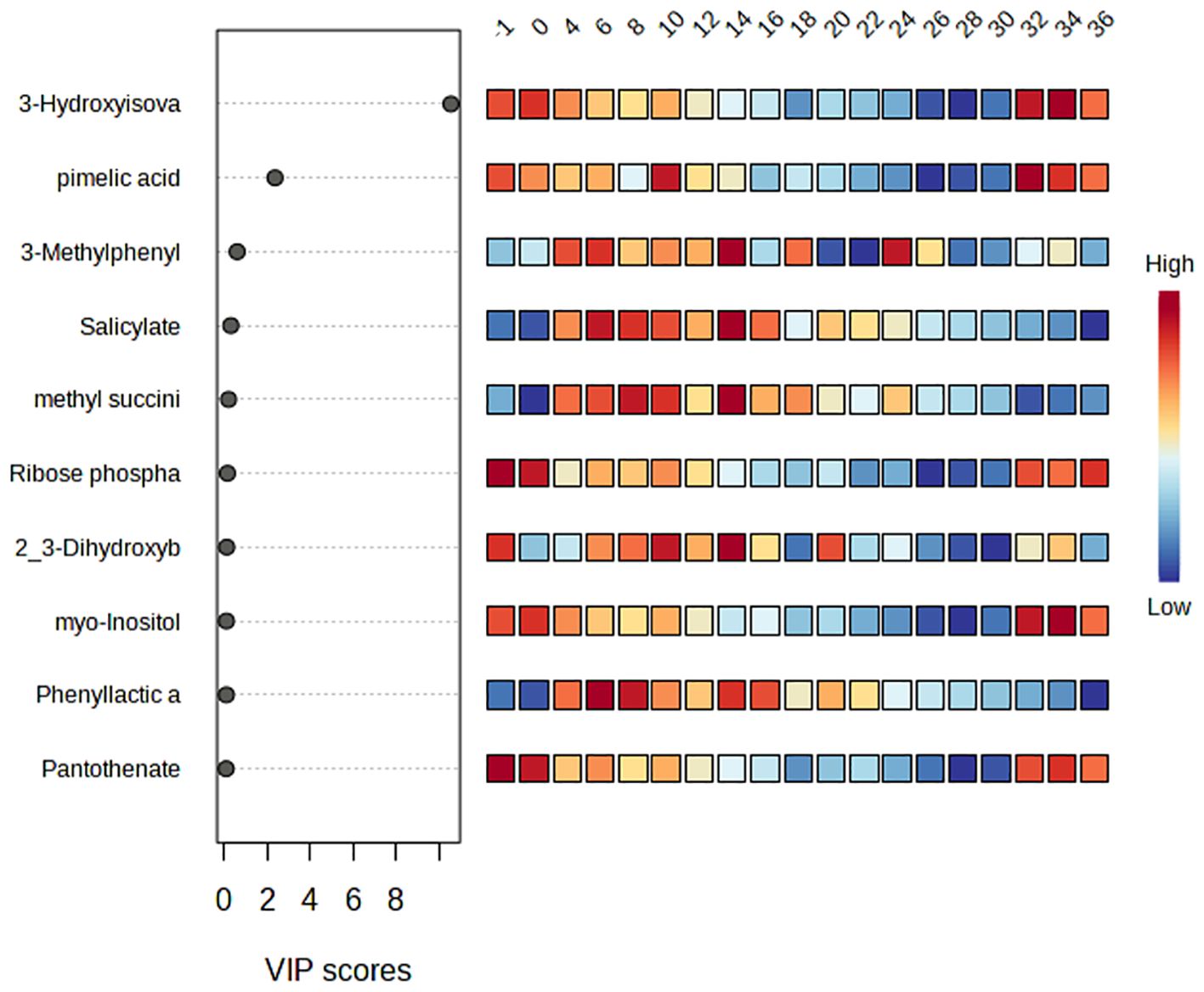
Figure 5. Variable Importance of Projection (VIP) scores of the top ten metabolites identified from Partial Least Squares Discriminant Analysis (PLS-DA). The corresponding heat map on the right indicates high and low abundance ratios by week.
3.7 Correlation with feed efficiency status
The 10 significant ASVs identified in the ANCOMBC global test and top 10 metabolites identified by VIP scores in MetaboAnalyst v5.0 were used for correlation analyses for the assessment of trends in ASV and metabolite abundances by RFI status (Supplementary Figures 2-21). The pattern search function in MetaboAnalyst v5.0 identified correlation values across multiple weeks for ASVs and metabolites. Significant correlations occurred in Weeks 4 (phenyllactic acid and myo-inositol), 12 (3-hydroxyisovalerate, ASV59 (Prevotella), ASV106 (Prevotella), and ASV9 (Prevotella)), 16 (myo-inositol), 18 (pimelic acid), 20 (ASV59 (Prevotella), ASV83 (Succiniclasticum), ASV9 (Prevotella), and ASV245 (Succiniclasticum)), 22 (ASV106 (Prevotella), 3-hydroxyisovalerate, and ASV83 (Succiniclasticum)), 24 (ASV46 (Prevotella) and 3-methylphenylacetic acid), 26 (pantothenate, ASV46 (Prevotella), ASV59 (Prevotella), 3-hydroxyisovalerate, salicylate, myo-inositol, pimelic acid, phenyllactic acid, 2_3-dihydroxybenzoate, and ASV106 (Prevotella)), and 30 (ASV1166 (Prevotella) and ASV15 (Prevotella)) (P<0.05; Table 8; Figure 6A–I). When adjusting for false discovery rate, significant correlations existed for Week 26 for both ASVs and metabolites (Q<0.05; Table 8; Figure 6H), and included ASV46 (Prevotella), ASV59 (Prevotella), 3-hydroxyisovalerate, salicylate, myo-inositol, pimelic acid, phenyllactic acid, 2_3-dihydroxybenzoate, and ASV106 (Prevotella). All significant correlations in Week 26 were positively correlated with RFI.
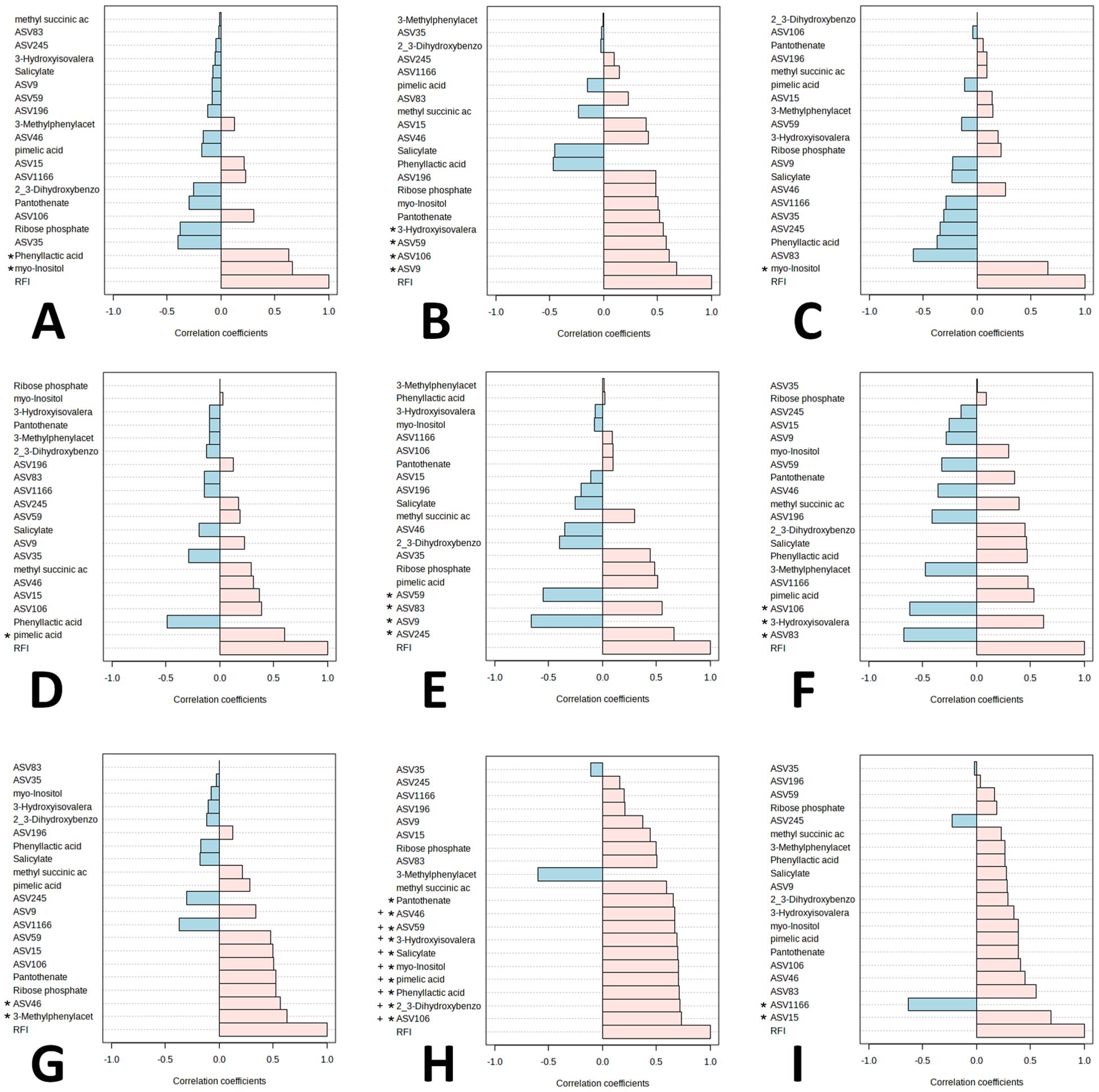
Figure 6. Significant correlations of Amplicon Sequence Variants (ASV) and metabolites with Residual Feed Intake (RFI) by Week. (A) Week 4, (B) Week 12, (C) Week 16, (D) Week 18, (E) Week 20, (F) Week 22, (G) Week 24, (H) Week 26, (I) Week 30. Asterisk (*) indicates significant correlation P<0.05. (+) indicates significant correlation FDR<0.05.
4 Discussion
Defining the rumen environment response to pregnancy is important for improving feed efficiency in beef production. This study identified rumen environment changes during pregnancy and determined correlations with established feed efficiency status in Angus heifers. The heifers in this study had numerous ASVs and metabolites that were different over time throughout pregnancy, with the majority identified in the third trimester. Of the 10 ASV abundances that were significantly different across all weeks, several were consistently significantly correlated with RFI across weeks in pregnancy, and several of the 10 metabolites with the greatest VIP scores correlated with RFI across weeks in pregnancy. Notably, however, both positive and negative correlations emerged among certain ASVs and metabolites at different time points, indicating a mixed correlation pattern. This complex pattern could imply multifaceted microbial and metabolic interactions influenced by feed efficiency and pregnancy, necessitating further investigation to fully understand the underlying dynamics. These data suggest an interaction between pregnancy and feed efficiency that shapes the rumen microbiome and its fermentative activity in heifers.
Differences in all alpha diversity metrics occurred throughout pregnancy and there was notable increase in the number of significant comparisons through the progression of gestation. The changes that occur throughout gestation tend to be physiologically more demanding beginning in the third trimester, or roughly week 26 of gestation. At this point in pregnancy, there is rapid fetal development of organs, skin, and hair as well as the maternal preparation for parturition (Winters et al., 1942). The natural progression throughout pregnancy is congruent with the rumen environment changes occurring in this study with the increasing number of significant comparisons of bacterial richness and evenness throughout gestation. Interestingly, approximately every 10 weeks during pregnancy, there was a decrease in species richness and evenness, followed by a progressive increase. Notable variation occurred throughout gestation due to the fluctuation in alpha diversity measurements; however, more extreme variation between individuals was measured from weeks 22-32. Possible environmental changes (i.e., weather, pasture quality) throughout gestation could influence alpha diversity indices. Outside of external influences on the ruminal bacterial diversity, the cyclical changes in richness and evenness could also relate to the individual differing energy demands on the cow, as the physiological demand increases towards parturition (Goff and Horst, 1997). The variation in means across time points seen in alpha diversity measurements within the current study indicate the need to perform additional longitudinal analyses to characterize changes in diversity in rumen microbiome. Temporal studies conducted in steers have indicated that alpha diversity is predominantly stable throughout trials, with major shifts due to changes in diet or year of sampling (Clemmons et al., 2019b; Snelling et al., 2019). However, another study assessing the stability of the microbiome in cows from birth to three years of age indicated that there were patterns in microbial diversity that appeared repetitively independent of diet (Furman et al., 2020). However, no studies to the author’s knowledge investigating the longitudinal changes of alpha diversity in pregnant cows are available. As previous studies have indicated more stability in richness and evenness over periods of time, there is potential that the fluctuations in alpha diversity noted within the current study are due to pregnancy rather than environmental changes. The mean averages for all three alpha diversity metrics show a decrease in species richness and evenness later in gestation which is supported by previous work from Shabat (Shabat et al., 2016), indicating that an efficient cow’s rumen microbiome tends to be less diverse and more specialized to efficiently meet the animals energy requirements.
The dominance of the abundance of Prevotella in this study aligns with previous work indicating the role and function in the rumen. Prevotella has been reported as the dominant genus of the rumen and a large abundance of Prevotella is linked with a healthy rumen microbiome across different species, including domestic cattle (Flint and Duncan, 2014), sheep (Wang et al., 2017), and buffalo (Singh et al., 2014; O’Hara et al., 2020). Prevotella are unique in their ability to further process complex polysaccharides and produce propionate, the most important substrate for gluconeogenesis (Strobel, 1992). Additionally, Succiniclasticum also has a primary role converting succinate into propionate (van GYLSWYK, 1995). Propionate is a critical energy source for ruminant animals (Yost et al., 1977), and is considered the primary substrate for gluconeogenesis in the liver (Armstrong, 1965; Ford, 1965). The production of glucose in the liver is critical as ruminants do not consume adequate amounts in their diet and rely on microbial activity to fuel a variety of physiological processes, including pregnancy. Increases in Prevotella and Succiniclasticum are commonly seen in animals on a more energy-intensive diet, rather than those grazing forages, similar to the heifers in this study (van GYLSWYK, 1995; Huo et al., 2014). Myer and others (Myer et al., 2015) indicated an association of increased Prevotella in greater feed efficient steers. The same study indicated the abundance of Succiniclasticum was greater in the lesser feed efficient steers. However, Auffret (Auffret et al., 2020) demonstrated greater abundances of Succiniclasticum in the more feed efficient steers. The conflicting data regarding the abundances of Succiniclasticum among feed efficiency studies and the current study likely derive from the physiological pressure and increased energy demand of pregnancy, confounded with feed efficiency status, as even prepartum and postpartum rumen samples from dairy cattle were variable and also distinguished in a diet-dependent manner (Lima et al., 2015). Further, metagenome analyses have indicated genes related to succinate-pathway propionate production were downregulated in a severely feed restricted pregnant sheep model (Xue et al., 2020), indicating the importance of the Prevotella and Succiniclasticum genera in the current study.
The heifers in this study weighed approximately 678 kg at the beginning of the trial and were of greater body condition score (BCS) than ideal, although exact BCS were not recorded. Less than 25% of all females conceived via artificial insemination by three different AI sires and 76% total were confirmed pregnant after a 60-day breeding season with two Angus bulls. The excess conditioning of the heifers on this trial likely impacted conception rates. Body condition score is a direct reflection of adipose fat deposition. Adipose fat tissue produces leptin (Wathes, 2012). Increases in leptin levels in obese women inhibits estradiol production and secretion and ovarian response to IGF-1, therefore, providing no signal for the initiation of LH surges (Caprio et al., 2001). This chain of events from increased adipose fat prevents ovulation and therefore pregnancy (Caprio et al., 2001). This connection is further supported by other research indicating a BCS greater than 4 at first service negatively impacts first service conception rates in dairy cattle (Heuer et al., 1999; Roche et al., 2018). In beef cattle however, research has indicated a BCS>8 negatively effects conception, in addition to cyclicity, mobility, and dystocia (Selk et al., 1986; Eversole et al., 2005). Leptin also inhibits insulin production and promotes insulin resistance. Insulin in turn stimulates leptin production and secretion (Paz-Filho et al., 2012). Insulin also works to regulate blood glucose levels. Glucose is a key nutrient in fetal development (Sletmoen-Olson et al., 2000), and during pregnancy, increased levels of leptin (Tessier et al., 2013) promote insulin resistance to allow greater systemic glucose and transported to the fetus.
This exchange of glucose and other nutrients becomes the most critical in the third trimester, to support rapid fetal growth. In this study, the shift in rumen microbial and metabolomic profiles around week 26 in gestation may indicate ruminal acclimation to the subsequent change of nutrient requirements. This increased nutritional demand during the third trimester is further indicated by the overall reduction in metabolite relative abundance ratios observed during weeks 26-30. Fetal growth is dependent on nutrient availability and its ability to be transported from the maternal source through the placenta to the fetus (Lager and Powell, 2012). In humans, glucose uptake is dependent on maternoplacental and fetal placental blood flow (Illsley et al., 1987). Inhibition of glucose exchange through the maternal-fetus placenta has been demonstrated with both maternal overnutrition and undernutrition in ewes (Bell and Ehrhardt, 2002). Restriction of nutrients in sheep prevents the production of substrates needed for nitric oxide dependent vasodilation to enhance blood flow to the conceptus (Bell and Ehrhardt, 2002). Conversely, overfed ewes exhibited a reduction in uterine and umbilical blood flow as well as glucose and oxygen uptake (Wallace et al., 2003). Therefore, an optimal ruminal environment may allow for maternal nutrient requirements to be better met.
The increased body weight of the heifers in this trial also likely influenced metabolic outputs. The metabolite 3-Hydroxyisovalerate had the highest VIP score of the top 10 metabolites. 3-Hydroxyisovalerate is a product of leucine degradation in an attempt to produce ATP for the Krebs cycle (Palomino-Schätzlein et al., 2018). In human studies, increased levels of 3-Hydroxyisovalerate from pregnant women were likely due to altered biotin, amino acid, and gut metabolism status, and potentially related to increased BMI status (Diaz et al., 2011). Based on identified abundances in this trial, 3-Hydroxyisovalerate is the most abundant in the beginning of pregnancy. In early pregnancy, biotin serves as an important precursor for normal fetal growth (Watanabe, 1983) as well as an important factor for glucose regulation (Dakshinamurti and Cheah-Tan, 1968). In several species, a biotin deficiency in pregnancy can be teratogenic on the fetus (Mock, 2008). Mock concluded that biotin metabolism is impacted by the alteration of steroid hormone concentrations that occur with the onset of pregnancy (Mock, 2005). The production of 3-Hydroxyisovalerate regarding pregnancy in heifers and cows may be of interest for future research with regard to its impact on gut function, gut metabolism, biotin, and BCS.
The rumen environment changes and metabolic outputs from the heifers in this study correlated with feed efficiency status. While feed efficiency was measured before pregnancy, feed efficiency status may persist throughout physiological and environmental changes (Lancaster et al., 2021). Many of the abundances of ASVs and metabolites were in a positive relationship with an increase in RFI. Metabolites and bacterial communities correlated to a less efficient animals could be an indicator of metabolic inefficiency. Although positively correlated metabolites and microbes may have other functions or relationships with feed inefficiency (Myer et al., 2015), their increased prevalence may indicate production increases, decreases in absorption, production exceeding absorption capacity of the host (Li et al., 2019), or reduced metabolism of the fermentative products and microbial growth (Kong et al., 2016) and lead to low feed efficiency. These inefficiencies may provide insight to the metabolic response to pregnancy in beef cattle.
In conclusion, several metabolites and bacterial communities in Angus heifers changed in abundance throughout pregnancy and were potentially associated with nutrient requirements and physiological changes. These metabolic and microbial changes were correlated with the previously established feed efficiency status of the heifers. Future work should focus on the long-term effects of changes in the rumen microbiome during pregnancy on both maternal health and calf development, as well as how dietary modifications can further optimize microbial diversity and feed efficiency. Additionally, research exploring the interactions between specific microbial communities and metabolites during various stages of gestation could provide deeper insights into enhancing cattle management practices and sustainability in beef production.
Data availability statement
The datasets presented in this study can be found in online repositories. The names of the repository/repositories and accession number(s) can be found below: https://www.ncbi.nlm.nih.gov/, PRJNA1040764.
Ethics statement
The animal study was approved by Institutional Animal Care and Use Committee at the University of Tennessee, Knoxville. The study was conducted in accordance with the local legislation and institutional requirements.
Author contributions
MM: Formal analysis, Investigation, Methodology, Writing – original draft, Writing – review & editing. MH: Formal analysis, Investigation, Methodology, Software, Validation, Writing – review & editing. BC: Conceptualization, Investigation, Methodology, Writing – review & editing. TA: Investigation, Methodology, Writing – review & editing. JC: Investigation, Methodology, Writing – review & editing. BV: Conceptualization, Investigation, Resources, Supervision, Writing – review & editing. KM: Investigation, Methodology, Project administration, Resources, Writing – review & editing. PYM: Investigation, Methodology, Resources, Writing – review & editing. DA: Investigation, Methodology, Resources, Writing – review & editing. PRM: Conceptualization, Funding acquisition, Investigation, Resources, Supervision, Validation, Writing – original draft, Writing – review & editing.
Funding
The author(s) declare that financial support was received for the research, authorship, and/or publication of this article. This research was funded by the Tennessee Beef Promotion Board and the USDA-NIFA Hatch/Multistate Project W-3010-TEN00578: Integrated Approach to Enhance Efficiency of Feed Utilization in Beef Production Systems; Accession Number: 1025826. The funding bodies had no role in the design of the study, collection, analyses, interpretation of data, or writing the manuscript.
Acknowledgments
The authors would like to thank the farm staff at the East Tennessee Research and Education Center as well as the farm staff at the Plateau Research and Education Center for their support throughout this project.
Conflict of interest
The authors declare that the research was conducted in the absence of any commercial or financial relationships that could be construed as a potential conflict of interest.
Publisher’s note
All claims expressed in this article are solely those of the authors and do not necessarily represent those of their affiliated organizations, or those of the publisher, the editors and the reviewers. Any product that may be evaluated in this article, or claim that may be made by its manufacturer, is not guaranteed or endorsed by the publisher.
Supplementary material
The Supplementary Material for this article can be found online at: https://www.frontiersin.org/articles/10.3389/fanim.2024.1484821/full#supplementary-material
References
Andersen C., Bonacker R., Smith E., Spinka C., Poock S., Thomas J. (2020). Evaluation of the 7 & 7 Synch and 7-day CO-Synch+ CIDR® protocols for estrus synchronization of beef cows prior to fixed-time artificial insemination with conventional or sex-sorted semen. bioRxiv. 2020-08. doi: 10.1101/2020.08.25.266783
Apprill A., McNally S., Parsons R., Weber L. (2015). Minor revision to V4 region SSU rRNA 806R gene primer greatly increases detection of SAR11 bacterioplankton. Aquat. Microb. Ecol. 75, 129–137. doi: 10.3354/ame01753
Armstrong D. (1965). Carbohydrate metabolism in ruminants and energy supply. Physiol. Digest. Ruminant, 272–288.
Auffret M. D., Stewart R. D., Dewhurst R. J., Duthie C.-A., Watson M., Roehe R. (2020). Identification of microbial genetic capacities and potential mechanisms within the rumen microbiome explaining differences in beef cattle feed efficiency. Front. Microbiol. 11, 1229. doi: 10.3389/fmicb.2020.01229
Beef USRfS (2022). USRSB High-Priority Indicator Goals and Sector Targets 2022. Available online at: https://www.usrsb.org/about. (Accessed May 24, 2023)
Bell A. W., Ehrhardt R. A. (2002). Regulation of placental nutrient transport and implications for fetal growth. Nutr. Res. Rev. 15, 211–230. doi: 10.1079/NRR200239
Bellows D., Ott S., Bellows R. (2002). Cost of reproductive diseases and conditions in cattle. Prof. Anim. Scientist 18, 26–32. doi: 10.15232/S1080-7446(15)31480-7
Benjamini Y., Hochberg Y. (1995). Controlling the false discovery rate: a practical and powerful approach to multiple testing. J. R. Stat. Soc.: Ser. B (Methodol.) 57, 289–300. doi: 10.1111/j.2517-6161.1995.tb02031.x
Callahan B. J., McMurdie P. J., Rosen M. J., Han A. W., Johnson A. J. A., Holmes S. P. (2016a). DADA2: High-resolution sample inference from Illumina amplicon data. Nat. Methods 13, 581–583. doi: 10.1038/nmeth.3869
Callahan B. J., Sankaran K., Fukuyama J. A., McMurdie P. J., Holmes S. P. (2016b). Bioconductor workflow for microbiome data analysis: from raw reads to community analyses. F1000Research 5:1492. doi: 10.12688/f1000research
Caprio M., Fabbrini E., Isidori A. M., Aversa A., Fabbri A. (2001). Leptin in reproduction. Trends Endocrinol. Metab. 12, 65–72. doi: 10.1016/S1043-2760(00)00352-0
Clemmons B. A., Martino C., Powers J. B., Campagna S. R., Voy B. H., Donohoe D. R., et al. (2019a). Rumen bacteria and serum metabolites predictive of feed efficiency phenotypes in beef cattle. Sci. Rep. 9, 19265. doi: 10.1038/s41598-019-55978-y
Clemmons B. A., Martino C., Schneider L. G., Lefler J., Embree M. M., Myer P. R. (2019b). Temporal stability of the ruminal bacterial communities in beef steers. Sci. Rep. 9, 9522. doi: 10.1038/s41598-019-45995-2
Clemmons B. A., Mulon P.-Y., Anderson D. E., Ault-Seay T. B., Henniger M. T., Schneider L. G., et al. (2022). Ruminal bacterial communities and metabolome variation in beef heifers divergent in feed efficiency. Ruminants 2, 282–296. doi: 10.3390/ruminants2020019
Dakshinamurti K., Cheah-Tan C. (1968). Biotin-mediated synthesis of hepatic glucokinase in the rat. Arch. Biochem. Biophys. 127, 17–21. doi: 10.1016/0003-9861(68)90195-1
Diaz S. O., Pinto J., Graça G., Duarte I. F., Barros A. S., Galhano E., et al. (2011). Metabolic biomarkers of prenatal disorders: an exploratory NMR metabonomics study of second trimester maternal urine and blood plasma. J. Proteome Res. 10, 3732–3742. doi: 10.1021/pr200352m
Domingo-Almenara X., Montenegro-Burke J. R., Guijas C., Majumder E. L. W., Benton H. P., Siuzdak G. (2019). Autonomous METLIN-guided in-source fragment annotation for untargeted metabolomics. Anal. Chem. 91, 3246–3253. doi: 10.1021/acs.analchem.8b03126
Edgar R. C., Flyvbjerg H. (2015). Error filtering, pair assembly and error correction for next-generation sequencing reads. Bioinformatics 31, 3476–3482. doi: 10.1093/bioinformatics/btv401
Eversole D. E., Browne M. F., Hall J. B., Dietz R. E. (2005). Body condition scoring beef cows. Virginia Cooperative Extension.
Ferrell C. L., Garrett W. N., Hinman N. (1976). Growth, development and composition of the udder and gravid uterus of beef heifers during pregnancy. J. Anim. Sci. 42, 1477–1489. doi: 10.2527/jas1976.4261477x
Flint H. J., Duncan S. (2014). Bacteroides and prevotella. In Encyclopedia of Food Microbiology: Second Edition (pp. 203-208). Elsevier Inc. Available at: https://doi.org/10.1016/B978-0-12-384730-0.00031-8
Ford E. (1965). The importance of glucose in ruminant metabolism Vol. 21 (New York: Energy Metabolism Academic Press).
Furman O., Shenhav L., Sasson G., Kokou F., Honig H., Jacoby S., et al. (2020). Stochasticity constrained by deterministic effects of diet and age drive rumen microbiome assembly dynamics. Nat. Commun. 11, 1904. doi: 10.1038/s41467-020-15652-8
Goff J. P., Horst R. L. (1997). Physiological changes at parturition and their relationship to metabolic disorders1, 2. J. Dairy Sci. 80, 1260–1268. doi: 10.3168/jds.S0022-0302(97)76055-7
Heuer C., Schukken Y., Dobbelaar P. (1999). Postpartum body condition score and results from the first test day milk as predictors of disease, fertility, yield, and culling in commercial dairy herds. J. Dairy Sci. 82, 295–304. doi: 10.3168/jds.S0022-0302(99)75236-7
Huo W., Zhu W., Mao S. (2014). Impact of subacute ruminal acidosis on the diversity of liquid and solid-associated bacteria in the rumen of goats. World J. Microbiol. Biotechnol. 30, 669–680. doi: 10.1007/s11274-013-1489-8
Illsley N. P., Hall S., Stacey T. (1987). The modulation of glucose transfer across the human placenta by intervillous flow rates: An in vitro perfusion study. Cell. Biol. Pharmacol. Placenta: Techniques Appl., 535–544. doi: 10.1007/978-1-4757-1936-9_38
Koch R. M., Swiger L. A., Chambers D., Gregory K. E. (1963). Efficiency of feed use in beef cattle. J. Anim. Sci. 22, 486–494. doi: 10.2527/jas1963.222486x
Kong R. S., Liang G., Chen Y., Stothard P., Guan L. L. (2016). Transcriptome profiling of the rumen epithelium of beef cattle differing in residual feed intake. BMC Genomics 17, 1–16. doi: 10.1186/s12864-016-2935-4
Lager S., Powell T. L. (2012). Regulation of nutrient transport across the placenta. J. Pregnancy 2012, 179827. doi: 10.1155/2012/179827
Lancaster P. A., Davis M. E., Rutledge J. J., Cundiff L. V. (2021). Relationships among feed efficiency traits across production segments and production cycles in cattle. Trans. Anim. Sci. 5, txab111. doi: 10.1093/tas/txab111
LeBlanc S. (2006). Monitoring programs for transition dairy cows. In Proceedings of the 26th World Biuatrics Congress, Nice (pp. 460-472).
Li F., Hitch T. C. A., Chen Y., Creevey C. J., Guan L. L. (2019). Comparative metagenomic and metatranscriptomic analyses reveal the breed effect on the rumen microbiome and its associations with feed efficiency in beef cattle. Microbiome 7, 6. doi: 10.1186/s40168-019-0618-5
Lima F. S., Oikonomou G., Lima S. F., Bicalho M. L. S., Ganda E. K., JCdO F., et al. (2015). Prepartum and postpartum rumen fluid microbiomes: characterization and correlation with production traits in dairy cows. Appl. Environ. Microbiol. 81, 1327–1337. doi: 10.1128/AEM.03138-14
Lin H., Peddada S. D. (2020). Analysis of compositions of microbiomes with bias correction. Nat. Commun. 11, 3514. doi: 10.1038/s41467-020-17041-7
Lu W., Clasquin M. F., Melamud E., Amador-Noguez D., Caudy A. A., Rabinowitz J. D. (2010). Metabolomic analysis via reversed-phase ion-pairing liquid chromatography coupled to a stand alone orbitrap mass spectrometer. Anal. Chem. 82, 3212–3221. doi: 10.1021/ac902837x
Mackie R., Aminov R., White B., McSweeney C. (2000). “Molecular ecology and diversity in gut microbial ecosystems,” in Ruminant physiology: digestion, metabolism, growth and reproduction (CABI, Wallingford UK), 61–77.
Martinez Arbizu P. (2020). pairwiseAdonis: Pairwise multilevel comparison using adonis. R package version 04, Vol. 1. Available at: https://github.com/pmartinezarbizu/pairwiseAdonis
McMurdie P. J., Holmes S. (2013). phyloseq: an R package for reproducible interactive analysis and graphics of microbiome census data. PloS One 8, e61217. doi: 10.1371/journal.pone.0061217
Mock D. M. (2005). Marginal biotin deficiency is teratogenic in mice and perhaps humans: a review of biotin deficiency during human pregnancy and effects of biotin deficiency on gene expression and enzyme activities in mouse dam and fetus. J. Nutr. Biochem. 16, 435–437. doi: 10.1016/j.jnutbio.2005.03.022
Mock D. M. (2008). Marginal biotin deficiency is common in normal human pregnancy and is highly teratogenic in mice. J. Nutr. 139, 154–157. doi: 10.3945/jn.108.095273
Myer P. R., Smith T. P., Wells J. E., Kuehn L. A., Freetly H. C. (2015). Rumen microbiome from steers differing in feed efficiency. PloS One 10, e0129174. doi: 10.1371/journal.pone.0129174
National Academies of Sciences, Engineering, and Medicine. (2016). Nutrient requirements of beef cattle, 8th ed.; The National Academies Press: Washington, DC, USA, 2016; p. 494.
O’Hara E., Neves A. L., Song Y., Guan L. L. (2020). The role of the gut microbiome in cattle production and health: driver or passenger? Annu. Rev. Anim. Biosci. 8, 199–220. doi: 10.1146/annurev-animal-021419-083952
Oksanen J., Kindt R., Legendre P., O’Hara B., Stevens M. H. H., Oksanen M. J., et al. (2007). The vegan package. Community Ecol. Package 10, 719.
Oliveira Filho R., Franco G., Reese S., Dantas F., Fontes P., Cooke R., et al. (2020). Using pregnancy associated glycoproteins (PAG) for pregnancy detection at day 24 of gestation in beef cattle. Theriogenology 141, 128–133. doi: 10.1016/j.theriogenology.2019.09.014
Palomino-Schätzlein M., Simo R., Hernandez C., Ciudin A., Mateos-Gregorio P., Hernandez-Mijares A., et al. (2018). Metabolic fingerprint of insulin resistance in human polymorphonuclear leucocytes. PloS One 13, e0199351. doi: 10.1371/journal.pone.0199351
Parada A. E., Needham D. M., Fuhrman J. A. (2016). Every base matters: assessing small subunit rRNA primers for marine microbiomes with mock communities, time series and global field samples. Environ. Microbiol. 18, 1403–1414. doi: 10.1111/emi.2016.18.issue-5
Paz-Filho G., Mastronardi C., Wong M.-L., Licinio J. (2012). Leptin therapy, insulin sensitivity, and glucose homeostasis. Indian J. Endocrinol. Metab. 16, S549. doi: 10.4103/2230-8210.105571
Prior R. L., Laster D. B. (1979). Development of the bovine fetus1. J. Anim. Sci. 48, 1546–1553. doi: 10.2527/jas1979.4861546x
Quast C., Pruesse E., Yilmaz P., Gerken J., Schweer T., Yarza P., et al. (2012). The SILVA ribosomal RNA gene database project: improved data processing and web-based tools. Nucleic Acids Res. 41, D590–D5D6. doi: 10.1093/nar/gks1219
Roche J., Burke C., Crookenden M., Heiser A., Loor J., Meier S., et al. (2018). Fertility and the transition dairy cow. Reprod. Fertil. Dev. 30, 85–100. doi: 10.1071/RD17412
Russell J. B., O’connor J., Fox D., Van Soest P., Sniffen C. (1992). A net carbohydrate and protein system for evaluating cattle diets: I. Ruminal fermentation. J. Anim. Sci. 70, 3551–3561. doi: 10.2527/1992.70113551x
Selk G. E., Wettemann R., Lusby K., Rasby R. (1986). The importance of body condition at calving on reproduction in beef cows (Oklahoma State University: Miscellaneous publication Agricultural Experiment Station).
Shabat S. K. B., Sasson G., Doron-Faigenboim A., Durman T., Yaacoby S., Berg Miller M. E., et al. (2016). Specific microbiome-dependent mechanisms underlie the energy harvest efficiency of ruminants. ISME J. 10, 2958–2972. doi: 10.1038/ismej.2016.62
Singh K., Reddy B., Patel A., Panchasara H., Parmar N., Patel A., et al. (2014). Metagenomic analysis of buffalo rumen microbiome: effect of roughage diet on Dormancy and Sporulation genes. Meta Gene 2, 252–268. doi: 10.1016/j.mgene.2014.01.005
Sletmoen-Olson K., Caton J., Olson K., Redmer D., Kirsch J., Reynolds L. (2000). Undegraded intake protein supplementation: II. Effects on plasma hormone and metabolite concentrations in periparturient beef cows fed low-quality hay during gestation and lactation. J. Anim. Sci. 78, 456–463. doi: 10.2527/2000.782456x
Snelling T. J., Auffret M. D., Duthie C.-A., Stewart R. D., Watson M., Dewhurst R. J., et al. (2019). Temporal stability of the rumen microbiota in beef cattle, and response to diet and supplements. Anim. Microb. 1, 1–14. doi: 10.1186/s42523-019-0018-y
Stewart R. L. Jr., Harris G. H., Lacy R. C., Ellis R. W., Hancock D. W., Silcox R. E. (2010). Cutting costs, not corners: Managing cattle in tough times (University of Georgia).
Strobel H. J. (1992). Vitamin B12-dependent propionate production by the ruminal bacterium Prevotella ruminicola 23. Appl. Environ. Microbiol. 58, 2331–2333. doi: 10.1128/aem.58.7.2331-2333.1992
Swett W. W., Matthews C. A., Fohrman M. H. (1948). Development of the fetus in the dairy cow. Washington DC: United States Dept of Agriculture.
Tessier D., Ferraro Z., Gruslin A. (2013). Role of leptin in pregnancy: consequences of maternal obesity. Placenta 34, 205–211. doi: 10.1016/j.placenta.2012.11.035
van GYLSWYK N. O. (1995). Succiniclasticum ruminis gen. nov., sp. nov., a Ruminal Bacterium Converting Succinate to Propionate as the Sole Energy-Yielding Mechanism. Int. J. Syst. Evol. Microbiol. 45, 297–300. doi: 10.1099/00207713-45-2-297
Wallace J. M., Bourke D. A., Aitken R. P., Milne J. S., Hay W. W. Jr. (2003). Placental glucose transport in growth-restricted pregnancies induced by overnourishing adolescent sheep. J. Physiol. 547, 85–94. doi: 10.1113/jphysiol.2002.023333
Wang Y., Cao P., Wang L., Zhao Z., Chen Y., Yang Y. (2017). Bacterial community diversity associated with different levels of dietary nutrition in the rumen of sheep. Appl. Microbiol. Biotechnol. 101, 3717–3728. doi: 10.1007/s00253-017-8144-5
Watanabe T. (1983). Teratogenic effects of biotin deficiency in mice. J. Nutr. 113, 574–581. doi: 10.1093/jn/113.3.574
Wathes D. (2012). Mechanisms linking metabolic status and disease with reproductive outcome in the dairy cow. Reprod. Domest. Anim. 47, 304–312. doi: 10.1111/j.1439-0531.2012.02090.x
Winters L. M., Green W. W., Comstock R. E. (1942). Prenatal development of the bovine. University of Minnesota Agricultural Experiment Station
Xue Y., Lin L., Hu F., Zhu W., Mao S. (2020). Disruption of ruminal homeostasis by malnutrition involved in systemic ruminal microbiota-host interactions in a pregnant sheep model. Microbiome 8, 1–14. doi: 10.1186/s40168-020-00916-8
Yost W. M., Young J. W., Schmidt S. P., McGilliard A. D. (1977). Gluconeogenesis in ruminants: propionic acid production from a high-grain diet fed to cattle. J. Nutr. 107, 2036–2043. doi: 10.1093/jn/107.11.2036
Keywords: rumen, bacteria, microbiome, feed efficiency, pregnancy
Citation: Martin MG, Henniger MT, Clemmons BA, Ault-Seay TB, Cordero JF, Voy BH, McLean KJ, Mulon P-Y, Anderson DE and Myer PR (2024) Pregnancy influences on the rumen environment of Angus heifers differing in feed efficiency. Front. Anim. Sci. 5:1484821. doi: 10.3389/fanim.2024.1484821
Received: 22 August 2024; Accepted: 31 October 2024;
Published: 15 November 2024.
Edited by:
Susumu Muroya, Kagoshima University, JapanReviewed by:
Mourad Ben Said, University of Manouba, TunisiaKei Imai, Rakuno Gakuen University, Japan
Copyright © 2024 Martin, Henniger, Clemmons, Ault-Seay, Cordero, Voy, McLean, Mulon, Anderson and Myer. This is an open-access article distributed under the terms of the Creative Commons Attribution License (CC BY). The use, distribution or reproduction in other forums is permitted, provided the original author(s) and the copyright owner(s) are credited and that the original publication in this journal is cited, in accordance with accepted academic practice. No use, distribution or reproduction is permitted which does not comply with these terms.
*Correspondence: Phillip R. Myer, cG15ZXJAdXRrLmVkdQ==
†Present address: Brooke A. Clemmons, Department of Agriculture, College of Agricultural Sciences and Natural Resources, Texas A&M University – Commerce, Commerce, TX, United States