- 1Laboratory of Biodiversity, Ecology, and Genome, Department of Biology, Faculty of Sciences, Mohammed V University in Rabat, Rabat, Morocco
- 2Department of Animal and Dairy Science, University of Georgia, Athens, GA, United States
- 3African Sustainable Agriculture Research Institute (ASARI), Mohammed VI Polytechnic University (UM6P), Laayoune, Morocco
Relatively high milk-producing cows in Morocco are culled at an elevated rate due to poor fertility. This situation is due to genetic, environmental, and management-related factors. To remedy this situation or prevent an additional decline in reproductive performance, using locally produced replacement heifers with good fertility and yield traits was proposed as a solution. Identifying these animals requires estimating the genetic parameters of the relevant reproduction and production traits in order to develop a genetic evaluation. Three reproduction traits (number of inseminations per conception, success of first insemination, and days open) and 305-d milk yield were used in this study. Two datasets of 4,186 records (first parity cows) and 5,511 records (first and multi-parity cows) were used. The pedigree files for both datasets consisted of 8,758 and 9,935 animals, respectively. A threshold-linear model was used for the analyses. For the first parity, estimates of heritability for 305-day milk yield (MY), days open (DO), number of inseminations per conception (NIC), and success of first insemination (SFI) were 0.26 ± 0.04, 0.17 ± 0.04, 0.10 ± 0.03, and 0.10 ± 0.04, respectively. For multi-parity data, the estimates were 0.19 ± 0.03, 0.12 ± 0.02, 0.10 ± 0.02, and 0.09 ± 0.02 for MY, DO, NIC, and SFI, respectively. The genetic correlations between MY and reproduction traits were 0.15 ± 0.11, 0.38 ± 0.12, and -0.43 ± 0.11 for DO, NIC, and SFI respectively. Overall, the heritability estimates of fertility traits were low. The genetic correlations of DO, NIC, and SFI with MY were moderately negative allowing for further selection for milk production without an additional deterioration in reproductive performance. The relative impact of using high fertility bulls compared to low fertility bulls on the success of first insemination ranged between 1.2% and 6.3% depending on the production environment. Collectively, these results confirm the possibility of implementing a viable selection program based on an appropriate weighted selection index.
1 Introduction
Over the last two decades, milk production in Morocco has witnessed a significant increase largely due to the import of purebred heifers and semen of elite sires, the integration of artificial insemination, and the enhancement of on-farm data recording practices. Increased milk production and the multiple environmental and management-related obstacles have led to a deterioration in reproductive performance. This situation has increased the early involuntary culling resulting in a marked negative impact on dairy farms’ profitability and sustainability (Boujenane, 2017). In fact, imported heifers rarely reach their third lactation in the Moroccan production environment (Sraïri and Baqasse, 2002). To remedy this situation or prevent additional decline in reproductive performance, locally produced replacement heifers with good fertility and yield traits was proposed as a solution. Identifying these animals requires estimating the genetic parameters of the relevant reproduction and production traits in order to develop a genetic evaluation.
The majority of fertility traits phenotypes suffer from a high noise level (Rodriguez et al., 2008) due to several reasons including inconsistent management practices, preferential treatment, and incomplete reporting. This has often resulted in low heritability estimates for these traits (Berry et al., 2019). Additionally, several reproductive traits are discrete (e.g., success of first insemination). Furthermore, these traits have moderate to high antagonistic genetic correlations with milk yield (Windig et al., 2006). Consequently, an accurate genetic evaluation for fertility and milk yield traits requires the availability of high-quality field data and an appropriate statistical model. Linear-threshold models are recommended for the joint analysis of fertility and production traits to maximize the amount of information and to accommodate discrete traits (Abdollahi-Arpanahi et al., 2013; Ayalew et al., 2017).
Three fertility traits (DO, NIC, and SFI) were systematically collected at the dairy herds used in this study. However, estimates of their heritabilities and correlations with milk yield are seldom available. This information is needed to assess the traits to include in a potential future genetic evaluation. The objectives of this study are to estimate the genetic parameters of 305-day milk yield, days open, number of inseminations per conception, and success of first insemination using an appropriate multivariate linear-threshold model in a Moroccan Holstein population and to assess the benefits of using semen from high fertility bulls on the SFI across different production environments.
2 Material and methods
2.1 Data
The data was collected at four herds owned by the Les Domaines Agricoles company. All herds are situated in two regions in north Morocco (Rabat-Salé-Kénitra, and Fez-Meknès). These regions are characterized by a Mediterranean climate with mild and humid winters and hot and dry summers. Cows were housed in open stalls and fed a total mixed ration twice a day. Artificial insemination (AI) was the sole breeding method utilized and it was administered by specialized AI technicians using frozen semen imported from Europe and North America. Semen quality is routinely assessed at artificial insemination (AI) centers for production and marketing purposes. However, at the farm level, while semen quality may be checked prior to purchase, it is neither quantified, assessed, nor recorded after thawing for use in artificial insemination procedures. Consequently, this critical parameter is excluded from data collection and subsequent analyses, despite its potential significant role and high correlation with multiple fertility traits in females, such as conception rate and non-return rate (Gebreyesus et al., 2021). However, the stringent assessment of the quality of semen used in AI will likely reduce the impact of male contribution on reproduction traits as supported by several studies (Averill et al., 2006; Miglior et al., 2017).
Estrus detection was conducted through visual inspection. The dataset consisted of 13,255 records collected on 7,928 cows between 2015 and 2023. The number of lactations per cow ranged between 1 and 5. Daily records including calving events, veterinary interventions, health issues, abortions, and dry-off dates were logged using herd management software.
Only the first 3 lactations were retained to reduce inconsistencies and non-random missingness. 305-d milk yield (MY), days open (DO), and number of inseminations per conception (NIC) were considered as continuous traits in the analysis, and success of first insemination (SFI) as a binary response. Parities with matching calving and abortion dates were removed. DO and NIC were considered missing when the conception date was missing, and no subsequent calving was reported. NIC and DO ranged between 1 and 17, and 20 and 574 days, respectively. Animals with first calving before 530 days of age were excluded. After editing, the final multi-parity data consisted of 7,600 records collected on 5,511 cows. The dataset for the first parity included 4,186. For the four-trait analysis, the pedigree file included 9,935 purebred Holstein animals, with cows born between 2006 and 2023. The majority of the animals (6,146 animals) have at least one parent known. The animals with records were born between 2016 and 2023. The average number of progenies was 4.05 and 1.16 for sires and dams, respectively. All inseminations were carried out using imported semen. The paternal grandsires were not available on all animals and the ages of the bulls were not documented. The average age of cows was 777, 1,219, and 1,655 days for first, second, and third parity, respectively.
2.2 Statistical model
A Bayesian multivariate linear-threshold model was used to jointly analyze four productive and reproductive traits for both first parity and multiparity cows. The linear model assumed at the liability scale included the fixed effects of herd (4 levels), calving month (12 classes), health status (2 levels), abortion status (3 levels), and two covariates (age at calving and days in milk at first insemination). Herd and calving month were included as separate factors and not as a contemporary group to prevent extreme-category problems (ECP) classes (all observations within a fixed effect class are either 0 or 1) for SFI. Health status was defined as healthy if no health issues (e.g., mastitis, metritis, and lameness), excluding abortion, occurred during lactation; otherwise, the cow was considered non-healthy regardless of the number of health problem episodes. Abortion status was coded 1 if no abortion occurred, 2 if one or more abortions occurred during the milk production period, and 3 if one or more abortions occurred during the dry period. In addition, the parity number was included as a fixed effect for the repeatability model.
At the liability scale, the following mixed linear model was used to analyze the data of the first three parities
where ′ is the vector of phenotypes for milk yield (, days open (, number of inseminations to conception (, and the liabilities for the binary response (success of first insemination). , , and e are the vectors of fixed, additive effects, random permanent environmental effects, and error terms. , , are known incidence matrices with the appropriate dimensions.
Conditionally on the model parameters, the joint distribution of the three continuous traits and the liability of the binary response were assumed to be normal
where is a 4x4 residual covariance matrix between the three continuous traits and the liability for the success of first insemination and is the identity matrix with dimensions equal to the number of animals with data. Note that the last diagonal element of the residual covariance matrix is fixed to 1 to make the model identifiable (Gianola, 1982).
When using only the first parity data, the permanent effect and the parity number were dropped from the model presented in Equation 1. To finalize the Bayesian formulation, the following priors are assumed for the position parameters
where and are 4x4 genetic and permanent environmental covariance matrices, respectively. A is the additive relationship matrix.
For the genetic and permanent environment covariance matrices, scaled inverse Wishart distribution priors were assigned
where and are 4x4 covariance matrices and and are the degrees of belief a priori for the genetic permanent environmental covariances, respectively.
The residual covariance matrix , is not completely random due to the fixation of the fourth diagonal element (corresponding to the binary traits) to 1. Consequently, direct sampling of is not feasible. To overcome the problem of sampling of the residual (co) variance, the methods described by Rekaya et al. (2013) were used.
Briefly, the model in [1] is multiplied by a matrix where is a 4x4 diagonal matrix, and is an identity matrix with the appropriate dimensions, yielding an equivalent model:
where , , with , , and are the vectors of fixed, additive, and permanent environmental effects for the trait i in the identifiable model in [1], respectively, and is the diagonal element i of the matrix . The resulting model in [2] is not identifiable because is unknown. The residual (co)variance matrix of the non-restricted model in [2] is given by:
where is the original restricted residual (co)variance matrix in [1], and is a 4x4 residual (co)variance matrix of the non-restricted model in [2]. Thus, estimates of the restricted residual covariance matrix could be easily obtained using Equation 3 and the non-restricted matrix . The lack of restrictions in enormously facilitates the Bayesian implementation via Markov Chain Monte Carlo (MCMC) methods. However, to obtain the parameters of the identifiable model in [1] from the draws of the non-identifiable parameters, the matrix needs to be defined. The identifiable parameters, based on expressions in [2] and [3], can be retrieved as:
Given that the diagonal element of corresponding to the binary response is fixed to 1, the last diagonal element of the matrix must be equal to the square root of the corresponding element in , and the first 3 diagonal elements of corresponding to the continuous traits are set equal to 1 as indicated in Rekaya et al. (2013) and Chang et al. (2017). A flat bounded prior was assumed for .
2.3 Effects of the genetic component on the probability of success of first insemination
The success of the first insemination was scored as a binary response. Under the assumed model, the liability for animal i, conditionally on the model parameters is given by:
where is the liability for animal i, , and are the vector of solutions for the fixed effects, the animal breeding value, and the permanent environmental effect, respectively. is a known incidence vector relating the fixed effects to the liability.
The relationship between the observed ordered categorical response and the liabilities is given by:
where is the binary response for animal i is equal to , and the threshold value () was assumed to be equal to zero.
Given the distribution in Equation 6 and the relationship in Equation 7, the probability of observing class for animal i is given by:
where is the cumulative distribution function for the normal distribution with mean equal to and variance equal to evaluated at .
The impact of using the best (highest breeding value for SFI) versus the worst bull on the probability of success of first insemination was assessed across three production environments (bad: =-1; average= ; and good: =+1).
3 Results and discussion
3.1 Descriptive statistics for the traits
Table 1 presents a summary description of the four traits. Around 43% of the records were removed during the data editing for several reasons. Observation loss increased with the parity number. This trend can be attributed to the elevated culling rate and the dependence on imported heifers resulting in a substantial portion of cows failing to progress beyond their initial lactation due to fertility and health issues. The 305-d milk production increased with the parity and it was comparable to the results reported by Fahim et al. (2021). However, it was slightly higher than what has been reported by Ouarfli and Chehma (2021). Overall, the cows’ reproductive performance across all parities were notably subpar. Across the three first parities, DO ranged between 153 to 161 days (Table 1). These results align closely with findings from Tunisia where M’Hamdi et al. (2009) reported a similar average DO interval of 151 days. Boujenane and Draga (2021), reported a shorter interval of 125 days, while El-Sherief et al. (2022) reported a much longer DO interval (173 days).
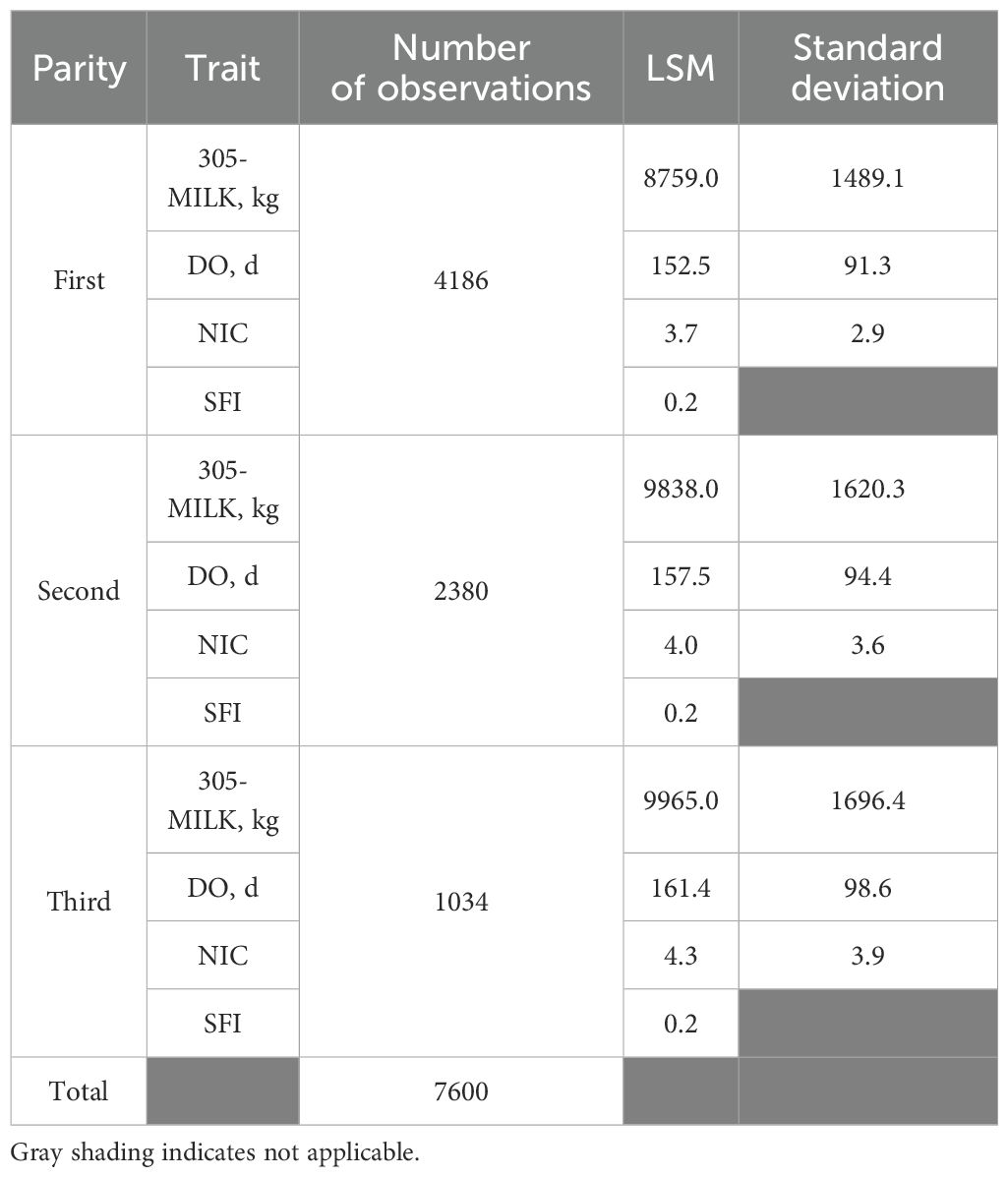
Table 1. Least square means (LSM) and standard deviations for 305d-milk yield (305-MILK), days open (DO), number of inseminations per conception (NIC), and success of first insemination (SFI) across the first three paities.
The NIC was relatively high across all three lactations, averaging around 4 inseminations per conception. Similar results were reported by Fahim et al. (2021) for Egyptian Holstein cows, and smaller estimates were reported by El-Sherief et al. (2022) and Boujenane and Draga (2021). It’s worth noting that the number of inseminations per conception is directly linked to Days Open (DO), as both metrics reflect similar aspects of cow reproductive efficiency. Nevertheless, DO fails to account for the time elapsed between calving and first insemination, a gap addressed by the number of inseminations per conception (NIC) as suggested by Abdollahi-Arpanahi et al. (2013). However, DO is recommended for populations that lack a good recording scheme (González-Recio et al., 2006). Therefore, NIC emerges as a more comprehensive indicator of fertility. This comparison underscores the importance of considering multiple metrics to gain a holistic understanding of dairy cows’ reproductive performance.
The success of the first insemination, a reflection of the pregnancy rate and closely linked to the non-return rate, was remarkably low across all parties, particularly in the first parity. This finding is unexpected, considering that the first insemination success rate typically tends to be higher for first parity cows compared to older ones. On top of the limited number of observations for older cows, one possible explanation for this discrepancy is the strong culling rate for cows failing the first 2 or 3 inseminations if they calved in fall or early winter. Thus, older cows are heavily selected for their ability to conceive early. The observed conception rate in this study appears to be lower than the 0.46 and 0.41 reported by Boujenane and Draga (2021) and El-Sherief et al. (2022), respectively.
3.2 Estimates of variance and genetic parameters
Table 2 presents the estimates of the posterior means (PM) and the posterior standard deviations (PSD) of heritabilities, genetic, and residual correlations for the four traits in the first parity. For fertility traits, heritability estimates were 0.17, 0.10, and 0.10 for DO, NIC, and SFI, respectively. The negative correlations of DO and NIC with milk production and the small correlations between SFI and the other traits should be noted. However, these results must be interpreted with caution given the wide high posterior density intervals associated with these estimates spanning from negative to positive values due to the limited size of the dataset and the sparseness of pedigree information.

Table 2. Heritability estimates (diagonal), genetic correlations (above), and residual correlations (below) for 305d-milk yield (305-MY), days open (DO), number of inseminations per conception (NIC), and success of first insemination (SFI) in first parity.
Estimates of heritability and repeatability using the first three parities data are presented in Table 3. The 305-day milk yield heritability estimate (0.19 ± 0.03) was comparable to the findings reported by Bakri et al. (2022). However, it was slightly lower than those reported in several studies conducted in arid and Mediterranean regions (Fahim et al., 2021; M’Hamdi et al., 2009; Ojango and Pollott, 2001; Sadek et al., 2021; Wahinya et al., 2020; Windig et al., 2006). Heritability estimates for fertility traits were low and within the range of reported estimates in the literature (Biffani et al., 2005; González-Recio and Alenda, 2005; Inchaisri et al., 2011; Jamrozik et al., 2005; M’Hamdi et al., 2009; Ojango and Pollott, 2001; Sadek et al., 2021; Wahinya et al., 2020) with a posterior mean of 0.12, 0.07, and 0.08 for DO, NIC, and SFI, respectively. Repeatability estimates (Table 3) ranged between moderate for milk yield (0.29) and low for fertility traits (0.08 to 0.014). Estimates of genetic correlations using the threshold-linear model indicated positive associations between 305-day milk yield, days open, and number of inseminations per conception, suggesting that high milk-producing cows often require more inseminations to conceive and exhibit prolonged days open. Conversely, a negative correlation was observed between the success of first insemination and all other traits, indicating that high milk-yield producers are more likely not to conceive soon after calving. This is likely due to high milk production around the peak of the lactation leading to a negative energy balance. The genetic correlations between SFI, DO, and NIC ranged between -0.97 and -0.89 (Table 4). The correlation between NIC and DO was high and positive (0.83). The residual correlations between the four traits were similar in trend and magnitude to those observed for the genetic correlations (Table 4) except for the correlation between 305-d milk yield and SFI which was substantially smaller (in absolute value) than the genetic correlation (-0.12 vs -0.43). Notably, the standard deviations associated with the estimates of genetic correlations between milk yield and fertility traits are relatively large indicating potential heterogeneity in managing cows based on their milk yield or/and reproductive performance. However, despite the small size of the dataset, most of the estimates of the genetic parameters are reasonably accurate as reflected by the relatively small associated standard errors. This could be due in part to relatively better pedigree information.
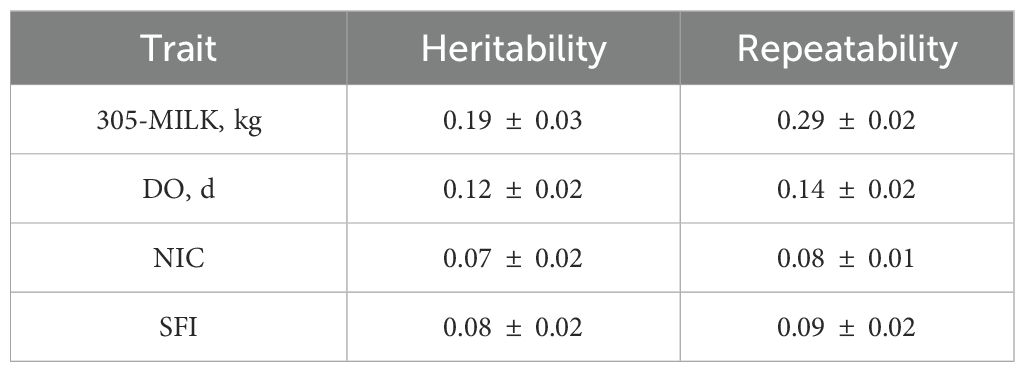
Table 3. Heritability and repeatability estimates for 305d-milk yield (305-MY), days open (DO), number of inseminations per conception (NIC), and success of first insemination (SFI) using the first three parities.
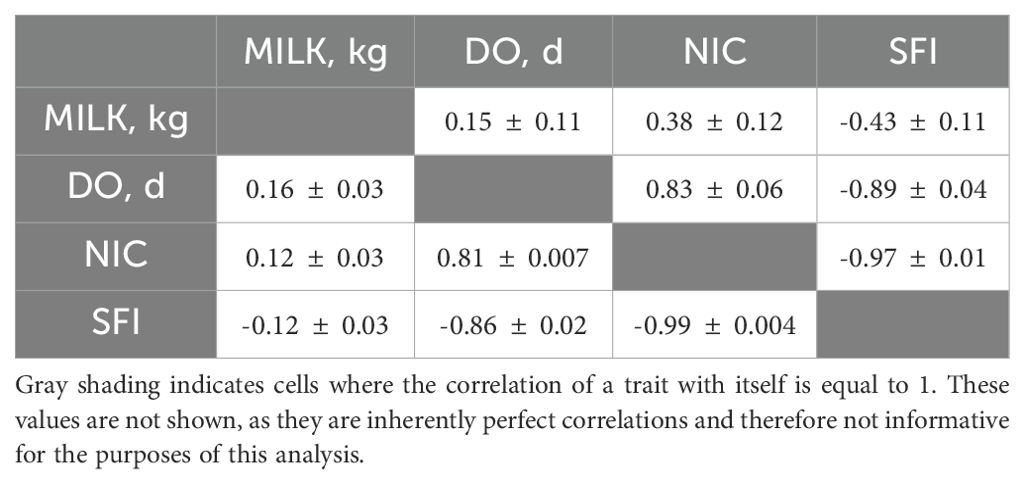
Table 4. Genetic (above the diagonal) and residual correlations (below diagonal) between 305d-milk yield (305-MILK), days open (DO), number of inseminations per conception (NIC), and success of first insemination (SFI).
Figure 1 presents the percentage gain in the success of first insemination (SFI) in the hypothetical case of using the best sire (based on estimated breeding values) compared to using the worst sire across three production environments (bad, average, and good). The data reveals a notable difference in impact depending on the environment type. In a bad production environment, the gain in SFI is the more substantial (6.3%) indicating that using a top sire for fertility traits can greatly enhance reproductive success under challenging conditions. Conversely, in a good production environment, the benefit of using a top sire will result only in a 1.2% increase in SFI suggesting that under favorable conditions, the choice of sire has limited impact in the SFI in concordance with previously reported results (Averill et al., 2006). In an average production environment, an intermediate benefit of 3.3% was observed. These findings highlight that the advantage of using sires with superior breeding values is most pronounced in suboptimal conditions, while in optimal settings, the relative gain diminishes.
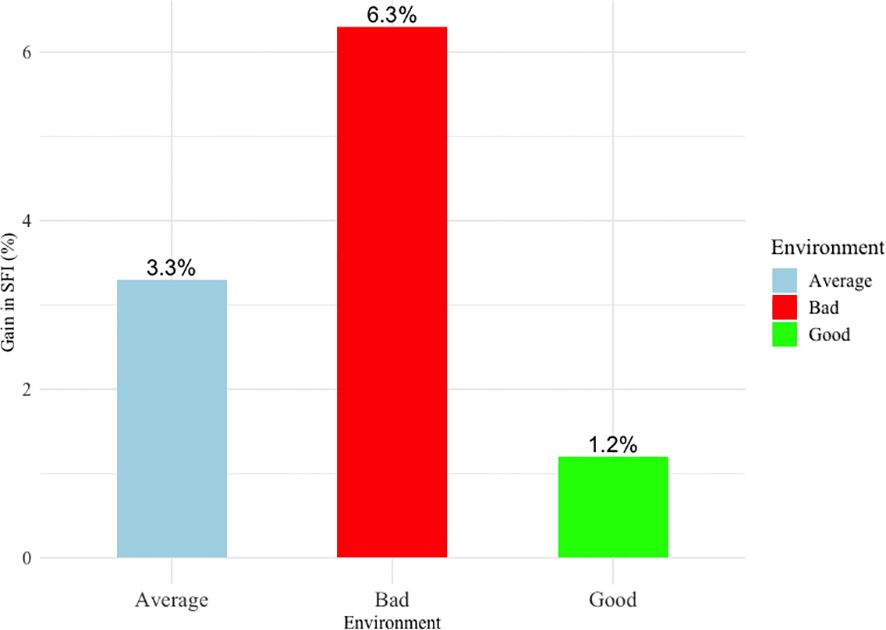
Figure 1. The relative gain in the success of the first insemination in a hypothetical scenario of using the best and the worst bulls across three different production environments.
In general, the results of this study align with previous research indicating the relatively low heritability of fertility traits in Holstein cows (Fahim et al., 2021; Inchaisri et al., 2011; M’Hamdi et al., 2009; Ojango and Pollott, 2001; Sadek et al., 2021; Wahinya et al., 2020). Additionally, our investigation reveals a strong positive correlation between days open and the number of inseminations per conception. This correlation suggests that cows experiencing prolonged intervals between calving and conception tend to require more inseminations to conceive. Notably, the repeatability of days open was higher than that of NIC. This can be attributed to the fact that the length of DO interval is more susceptible to management interventions, such as delaying the first insemination for high-yielding cows. In fact, it was argued against using days open as a trait for fertility evaluation, particularly in settings with comprehensive recording schemes and management practices (González-Recio et al., 2006). However, in contexts where such schemes are lacking, days open may still offer valuable insights into reproductive performance and management strategies (González-Recio et al., 2006). The success of first insemination is an important economic determinant, encompassing the cost of semen and the labor associated with monitoring heat cycles and conducting inseminations. Integrating SFI into the fertility index of a herd holds considerable merit, as it allows for a more comprehensive evaluation of reproductive performance and cost-effectiveness. Furthermore, the outcome of each insemination can be assessed as a longitudinal binary variable, as demonstrated by (Averill et al., 2006). This modeling approach incorporates all breeding information within a certain period, allowing for more precise estimations.
Accurate reproductive data is hard to collect especially under Moroccan dairy production and management conditions. Similarly, the pedigree information is often incomplete or inaccurate. Thus, stringing data pre-processing and editing is needed. This process often leads to loss of information resulting in relatively small datasets to carry out genetic analyses. Although the estimates of genetic parameters in this study seem to be accurate based on their associated standard errors, they should be interpreted with some caution. Overall, despite the limitations of this study, the results provide valuable insights while we accumulate additional data.
4 Conclusion
Female fertility is crucial for the profitability of dairy herds, particularly for Holstein cows. However, this relationship is often marred by an antagonistic correlation with milk yield. The moderate genetic correlations between milk yield and the three fertility traits considered in this study support the possibility to attenuate the deterioration or even improve dairy cows’ fertility in Morocco without sacrificing the selection for higher milk yield. This could be achieved through a selection index that includes all relevant traits with their relative weights. Defining appropriate traits to include in selection indices is paramount. A particular emphasis should be put on traits directly impacting profitability, such as the number of inseminations per conception and the success rate at first insemination, which include information on veterinary, breeding, and labor costs. A fundamental hurdle in fertility trait analysis lies in the noisy field datasets and the non-normal distribution of some traits, notably the discrete nature of the success rate of first insemination.
Data availability statement
The data analyzed in this study is subject to the following licenses/restrictions: The data used in this study are not publicly available due to restrictions imposed by the company that provided the data. Consequently, we are unable to share the data as the company does not permit its dissemination. Requests to access these datasets should be directed to Ym91YWJpZGJhZGFvdWlAZ21haWwuY29t.
Author contributions
NC: Conceptualization, Data curation, Formal analysis, Methodology, Writing – original draft, Writing – review & editing. BB: Conceptualization, Project administration, Resources, Supervision, Validation, Writing – review & editing. RR: Conceptualization, Formal analysis, Methodology, Project administration, Supervision, Validation, Writing – review & editing.
Funding
The author(s) declare that financial support was received for the research, authorship, and/or publication of this article. NC gratefully acknowledges financial support for this publication by the Fulbright U.S. Student Program, which is sponsored by the U.S. Department of State and The Moroccan American Commission for Educational & Cultural Exchange (MACECE). Its contents are solely the responsibility of the author and do not necessarily represent the official views of the Fulbright Program, the Government of the United States, or the MACECE.
Acknowledgments
NC and BB thank “Les Domaines Agricoles” for providing the analyzed data.
Conflict of interest
The authors declare that the research was conducted in the absence of any commercial or financial relationships that could be construed as a potential conflict of interest.
Publisher’s note
All claims expressed in this article are solely those of the authors and do not necessarily represent those of their affiliated organizations, or those of the publisher, the editors and the reviewers. Any product that may be evaluated in this article, or claim that may be made by its manufacturer, is not guaranteed or endorsed by the publisher.
References
Abdollahi-Arpanahi R., Peñagaricano F., Aliloo H., Ghiasi H., Urioste J. I. (2013). Comparison of Poisson, probit and linear models for genetic analysis of number of inseminations to conception and success at first insemination in Iranian Holstein cows. Livestock Sci. 153, 20–26. doi: 10.1016/j.livsci.2013.01.009
Averill T., Rekaya R., Weigel K. (2006). Random regression models for male and female fertility evaluation using longitudinal binary data. J. Dairy Sci. 89, 3681–3689. doi: 10.3168/jds.S0022-0302(06)72408-0
Ayalew W., Aliy M., Negussie E. (2017). Estimation of genetic parameters of the productive and reproductive traits in Ethiopian Holstein using multi-trait models. Asian-Australasian J. Anim. Sci. 30, 1550–1556. doi: 10.5713/ajas.17.0198
Bakri N. E., Djemali M., Sarti F. M., Benyedder M., Pieramati C. (2022). Genetic evaluation to design a reference cow population for the Holstein breed in Tunisia: a first step toward genomic selection. Animal Production Science 62, 774–782. doi: 10.1071/AN20688
Berry D. P., Twomey A. J., Evans R. D., Cromie A. R., Ring S. C. (2019). Heritability–what is it, and what is it not; implications for improving cattle health. Cattle Pract. 27, 1–11.
Biffani S., Canavesi F., Samoré A. (2005). Estimates of genetic parameters for fertility traits of Italian Holstein-Friesian cattle. Stockbreeding (Hrvatsko-Agronomsko-Drustvo@zg.t-Com.Hr) 59, 145–153.
Boujenane I. (2017). Reasons and risk factors for culling of Holstein dairy cows in Morocco. J. Livestock Sci. Technol. 1, 25–31.
Boujenane I., Draga B. (2021). Non-genetic factors affecting reproductive performance of Holstein dairy cows. Livestock Res. Rural Dev. 33, Article 10. doi: 10.22103/jlst.2017.1661
Chang L.-Y., Toghiani S., Ling A., Hay E. H., Aggrey S. E., Rekaya R. (2017). Analysis of multiple binary responses using a threshold model. J. Agricultural Biol. Environ. Stat 22, 640–651. doi: 10.1007/s13253-017-0305-6
El-Sherief A. A., El-Komy S. M., Rashad A., El-Hedainy D. K. (2022). Reproductive performance of lactating Holstein cows as influenced by season of calving and parity under subtropical conditions. J. Advanced Veterinary Res. 12, Article 1.
Fahim N. H., Mohamed Ibrahim M. A. A., Amin A. H., Sadek R. R. (2021). Milk production and reproductive performance of retained and culled cows in a large Holstein Herd in Egypt. World’s Veterinary J. 11, Article 3. doi: 10.54203/scil.2021.wvj61
Gebreyesus G., Lund M. S., Kupisiewicz K., Su G. (2021). Genetic parameters of semen quality traits and genetic correlations with service sire nonreturn rate in Nordic Holstein bulls. J. Dairy Sci. 104, 10010–10019. doi: 10.3168/jds.2021-20403
Gianola D. (1982). Theory and analysis of threshold characters. J. Anim. Sci. 54, 1079–1096. doi: 10.2527/jas1982.5451079x
González-Recio O., Alenda R. (2005). Genetic parameters for female fertility traits and a fertility index in Spanish dairy cattle. J. Dairy Sci. 88, 3282–3289. doi: 10.3168/jds.S0022-0302(05)73011-3
González-Recio O., Alenda R., Chang Y. M., Weigel K. A., Gianola D. (2006). Selection for female fertility using censored fertility traits and investigation of the relationship with milk production. J. Dairy Sci. 89, 4438–4444. doi: 10.3168/jds.S0022-0302(06)72492-4
Inchaisri C., Jorritsma R., Vernooij J., Vos P., van der Weijden G., Hogeveen H. (2011). Cow effects and estimation of success of first and following inseminations in Dutch dairy cows. Reprod. Domest. Anim. 46, 1043–1049. doi: 10.1111/j.1439-0531.2011.01782.x
Jamrozik J., Fatehi J., Kistemaker G. J., Schaeffer L. R. (2005). Estimates of genetic parameters for Canadian Holstein female reproduction traits. J. Dairy Sci. 88, 2199–2208. doi: 10.3168/jds.S0022-0302(05)72895-2
M’Hamdi N., Aloulou R., Brar S., Mahdi B., Ben Hamouda M. (2009). Phenotypic and genetic parameters of reproductive traits in Tunisian Holstein cows. Livestock Res. Rural Dev. 11, 297–307. doi: 10.2298/BAH1006297M
Miglior F., Fleming A., Malchiodi F., Brito L. F., Martin P., Baes C. F. (2017). A 100-Year Review: Identification and genetic selection of economically important traits in dairy cattle. J. dairy Sci. 100, 10251–10271. doi: 10.3168/jds.2017-12968
Ojango J. M. K., Pollott G. E. (2001). Genetics of milk yield and fertility traits in Holstein-Friesian cattle on large-scale Kenyan farms1. J. Anim. Sci. 79, 1742–1750. doi: 10.2527/2001.7971742x
Ouarfli L., Chehma A. (2021). Effect of temperature-humidity-index on milk performances of local born Holstein dairy cows under Saharan climate. Archiva Zootechnica 24, 24–36. doi: 10.2478/azibna-2021-0010
Rekaya R., Sapp R. L., Wing T., Aggrey S. E. (2013). Genetic evaluation for growth, body composition, feed efficiency, and leg soundness. Poultry Sci. 92, 923–929. doi: 10.3382/ps.2012-02649
Rodriguez H., Hultgren J., Båge R., Bergqvist A.-S., Svensson C., Bergsten C., et al. (2008). Reproductive performance in high-producing dairy cows: Can we sustain it under current practice? IVIS Rev. Veterinary Med. R01. Available at: https://res.slu.se/id/publ/19903.
Sadek R. R., Abou-Bakr S., Nigm A. A., Abd El-Aziz Mohamed Ibrahim M., Badr M. M., Awad M. A. A. (2021). Evaluation of milk yield and reproductive performance of pure Holstein and its F1 crossbreds with montbeliarde in Egypt. World’s Veterinary J. 11, Article 3. doi: 10.54203/scil.2021.wvj63
Sraïri M. T., Baqasse M. (2002). “Devenir et performances de génisses laitières importées au Maroc,” in Prospects for a sustainable dairy sector in the Mediterranean (Wageningen, Netherlands: Wageningen Academic), 331–336. doi: 10.3920/9789086865093_043
Wahinya P. K., JeyAruban G., Swan A., Magothe T. (2020). Estimation of genetic parameters for milk and fertility traits within and between low, medium and high dairy production systems in Kenya to account for genotype-by-environment interaction. J. Anim. Breed. Genet. 137, 495–509. doi: 10.1111/jbg.12473
Keywords: heritability, genetic correlations, milk yield, fertility, Moroccan Holstein
Citation: Chafai N, Badaoui B and Rekaya R (2024) Genetic parameters of milk yield and fertility traits in Moroccan Holsteins. Front. Anim. Sci. 5:1446989. doi: 10.3389/fanim.2024.1446989
Received: 10 June 2024; Accepted: 05 December 2024;
Published: 19 December 2024.
Edited by:
Geoffrey E. Dahl, University of Florida, United StatesReviewed by:
Maria Sumampa Coria, CONICET Institute of Bionanotechnology of NOA (INBIONATEC), ArgentinaMartina Miluchová, Slovak University of Agriculture, Slovakia
Copyright © 2024 Chafai, Badaoui and Rekaya. This is an open-access article distributed under the terms of the Creative Commons Attribution License (CC BY). The use, distribution or reproduction in other forums is permitted, provided the original author(s) and the copyright owner(s) are credited and that the original publication in this journal is cited, in accordance with accepted academic practice. No use, distribution or reproduction is permitted which does not comply with these terms.
*Correspondence: Narjice Chafai, bmFyamljZWNoYWZhaUBnbWFpbC5jb20=