- 1Department of Camel Research, Fujairah Research Centre (FRC), Fujairah, United Arab Emirates
- 2Department of Theriogenology, Faculty of Veterinary Medicine, Kafrelsheikh University, Kafr El-Sheikh, Egypt
Introduction: This study addresses the need for automated monitoring solutions to evaluate the daily activity patterns of camels, which is critical for improving animal welfare and farm management practices. By leveraging advanced deep learning techniques, this research aims to identify and analyze five key daily activities—sleeping, sitting, standing, eating, and drinking—using video recordings from a camel farm in Fujairah, United Arab Emirates.
Methods: The dataset was collected over two 7-day phases in November and December 2022. In Phase 1, video recordings were analyzed to monitor the activities of two camels and measure the duration of each activity. In Phase 2, the study expanded to include six camels, enabling an evaluation of individual behavioral variations. The YOLOv7 object detection algorithm was used to train and validate the model on images extracted from the recordings, achieving high accuracy in detecting and classifying the defined activities.
Results: The results showed notable variations in activity patterns between Phases 1 and 2. Average standing time decreased from 9.8 hours (40.8%) to 6.0 hours (25.1%), and sleeping time dropped from 4.3 hours (18.0%) to 2.8 hours (11.7%). Conversely, sitting time increased from 6.2 hours (25.8%) to 9.9 hours (41.5%), and eating time rose from 3.1 hours (12.8%) to 4.6 hours (19.2%). Drinking time remained consistent at an average of 37 minutes (2.6%) across both phases. Activity peaks were observed during early mornings and after 16:00, with midday hours dominated by resting in shaded areas. Evening and nighttime activities primarily included sitting, minimal head movements, and occasional standing or walking.
Discussion: The established deep learning framework demonstrated reliable performance in detecting and analyzing camel activity patterns, offering a practical solution for continuous monitoring and improved farm management. However, further research is recommended to validate the model’s performance across different seasons and environmental conditions to enhance its robustness and adaptability.
Introduction
Camels play crucial roles in the Arabian Gulf, significantly contributing to the food biosecurity sector by providing milk and meat. Additionally, they are used for transport, entertainment, and are integral to the social structure of pastoral societies (Faye, 2016). Naturally, camels are active animals capable of walking 20-25 km daily in search of food, water, and shelter in their harsh desert habitat (Bouaouda et al., 2014). They exhibit remarkable resilience to pathogens, can survive up to a month without food and several days without water, and their body temperature fluctuates between 36-39°C throughout the day (Tibary and El Allali, 2020; Hussen and Al-Sukruwah, 2022).
With the impact of global warming leading to reduced rainfall and natural vegetation, and an increase in camel numbers, many camel farms have been established. However, restricted housing conditions can agitate and stress camels, causing changes in their behavior, performance, and various body systems, including the immune system (Aubè et al., 2017; Kutty and Yousuf, 2017). Notably, significant changes in the blood immune system have been observed in dromedary camels kept under restricted housing compared to those on free land (Hussen and Al-Sukruwah, 2022). Additionally, reproductive efficacy declines, with a calving interval exceeding three years and a 27% mortality rate among camel calves reported under farm conditions (Kutty and Yousuf, 2017; Tibary and El Allali, 2020).
Camel farms are often situated in remote areas, far from veterinary services. The delay between the onset of disease symptoms and veterinary intervention can seriously impact camel health management (Padalino and Menchetti, 2021). Advanced deep-learning techniques are becoming increasingly significant in animal research for the continuous monitoring of camel health and welfare (Padalino and Menchetti, 2021). In daily activity research, numerous hours of video are systematically examined, requiring meticulous observation of animal behavior over weeks or months. These videos are then visually inspected and automatically analyzed to assess daily activities. Changes in movement patterns over time can provide insights into animal well-being and health, particularly when detected automatically (Rice et al., 2017). An early warning system can be developed to issue alerts upon identifying abnormal daily activities (Fernández-Carrión et al., 2017). The objective of this study was to apply machine learning techniques to automate the identification and analysis of five specific daily activities in camels: sleeping, sitting, standing, eating, and drinking (collectively referred to as 3SED activities). The experiment was carried out in two phases with varying numbers of camels to (a) measure the duration of each activity and (b) assess the variations in these activities across individual camels as well as different group sizes.
Materials and methods
Animal keeping and the experiment setup
Six immature dromedary camels (3 males and 3 females), aged 2 years and free from any physical abnormalities, were housed in a half-shaded pen measuring 76 m² at Bulaida Farm in Fujairah, United Arab Emirates. The pen was equipped with tanks for water and food, and the camels were fed twice daily with wheat bran, flaked maize, flaked barley, and dried alfalfa at rates of 1.0, 0.6, 0.4, and 2.0 kg per head per day, respectively. Additionally, the camels had free access to Rhodes grass, water, and mineral blocks. The feed quantity and quality were maintained consistently throughout the experiment.
Two Reolink RLC-810A cameras were installed at diagonal angles in the pen to cover the entire area, recording approximately 478 GB of video data over 24 days. The experiment was conducted in two phases: Phase 1 involved two camels (one male and one female) to measure the average time spent on each activity. In Phase 2, four additional camels of the same age group (two males and two females) were introduced, bringing the total to six camels in the pen, to compare the daily activities among different individuals. Each phase consisted of 7 non-consecutive days of testing (with 3-day intervals) during November and December 2022.
Daily activities and video data sets
Sitting, sleeping, standing, eating, and drinking were the five daily studied activities of the camels. The daily activities were previously identified (Mansour et al., 2024) and briefly as follows: Sitting: having all four legs tucked under the body and the brisket on the ground; Sleeping: camel was either in sitting posture and the head was on the ground or the camel was laying on one side and its head was also on the ground; Standing: the camel was either fully in an upright quadrupedal stance or walking throughout the pen; Eating: camel was in the standing posture and its head was inside the feeding tank; Drinking: similar to the eating activity but the head was inside the water tank (White et al., 2008).
The video recordings were captured at 5 frames per second (fps) with a resolution of 1920x1080 pixels. From these recordings, single frames were extracted to create a comprehensive image set representing various camel activities. A total of 8,000 frames were initially extracted from videos capturing the camels’ full range of motion both day and night. These frames were manually reviewed to identify relevant instances where camels were observed performing behaviors such as sitting, standing, eating, drinking, and sleeping. From these frames, 14,380 images were ultimately extracted for further analysis., The cameras operated continuously, and random images were selected for training the model. All camels in the images were manually labeled using the Roboflow program.
The image set was divided into three parts: a training set (70%, 5600 frames), a test set (10%, 800 frames), and a validation set (20%, 1600 frames), ensuring the balance of the three labeled classes (Table 1). The training set was used to train the YOLOv7 (You Only Look Once version 7) object detection algorithm, while the test set was utilized to evaluate the model’s effectiveness in detecting camels (Bochkovskiy et al., 2020; Redmon and Farhadi, 2018; Schütz et al., 2021).
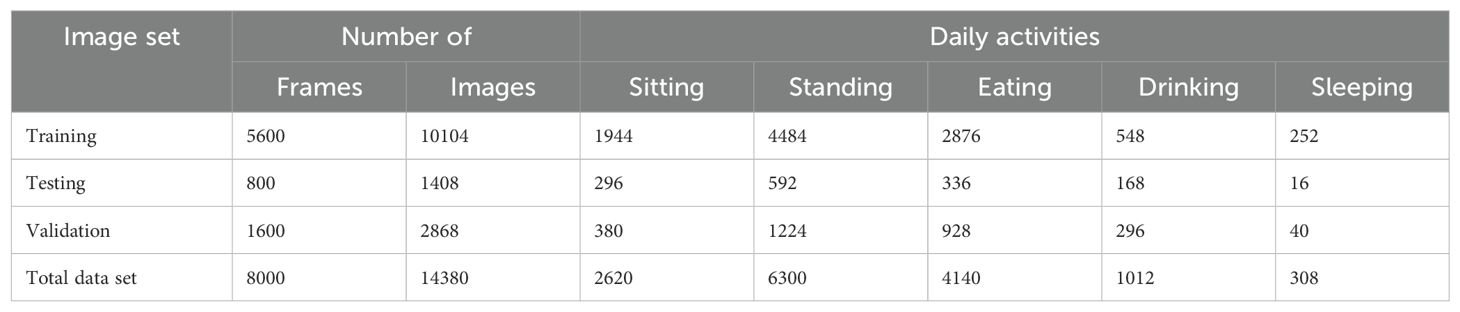
Table 1. Distribution of image set into training, testing, and validation subsets (70%, 10%, and 20%, respectively) with frame counts for each daily activity.
Automatic detection and evaluation of the model performance
The YOLOv7 algorithm, leveraging a Convolutional Neural Network (CNN), was implemented for the automatic detection of camel activities, demonstrating high efficiency and accuracy as a real-time object detection system (Bochkovskiy et al., 2020; Redmon and Farhadi, 2018; Robert et al., 2009). The model was trained using Python 3.6.8 in a Jupyter Notebook environment, with input images specifically selected for clarity and a low learning rate applied over 2000 batches to ensure accurate training.
To evaluate the model’s performance, key metrics such as average precision (AP), precision, and recall were calculated by comparing the predicted bounding boxes to manually labeled ground-truth bounding boxes (Everingham et al., 2009; Fernández-Carrión et al., 2020). The Intersection over Union (IoU) metric was used to classify detections as either true positives (IoU ≥ 0.5) or false positives (IoU < 0.5), with undetected activities marked as false negatives.
Precision is defined as the percentage of correctly identified activities within a class (e.g., the correct classification of standing), while recall measures the proportion of actual activities that were accurately identified. Average precision (AP) represents the overall percentage of correct predictions across all classes, providing a comprehensive evaluation of the model’s effectiveness in detecting camel activities throughout the experiment. The tested data set was used to check how well the final model could identify and classify camel activities, measuring accuracy through precision, recall, and average precision. The validation data set was used during training to make sure the model was learning correctly and to adjust settings as needed. This helped prevent the model from becoming too focused on the training data and improved its overall performance.
Automated video evaluation workflow
To evaluate the videos, we utilized the trained camel detector depicted in Figure 1. The video analysis process involved several steps: collecting video data, dividing the videos into sets, and storing the detected camel activities after model training.
Results
Model training and evaluation
The model achieved a detection speed of 73.3 milliseconds per frame, and the output for camel detection in a single frame is illustrated in Figure 2. The performance of the trained model for detecting the five camel activity classes (eating, standing, drinking, sitting, and sleeping) demonstrated high levels of precision, recall, and average precision (AP) across all categories was shown in Table 2. The model achieved a precision of 95.4% for detecting eating activities, with a recall of 86.6% and an AP of 94.5%. For standing, the precision and recall were both 90%, with an AP of 93.8%. The drinking class exhibited the highest performance, with a precision of 97.7%, recall of 99.9%, and an AP of 99.5%. Sitting activities were similarly well-detected, with a precision of 98.9%, recall of 99.9%, and an AP of 99.6%. Sleeping activities achieved a precision of 91.1%, with recall and AP both reaching 99.9% and 99.5%, respectively.
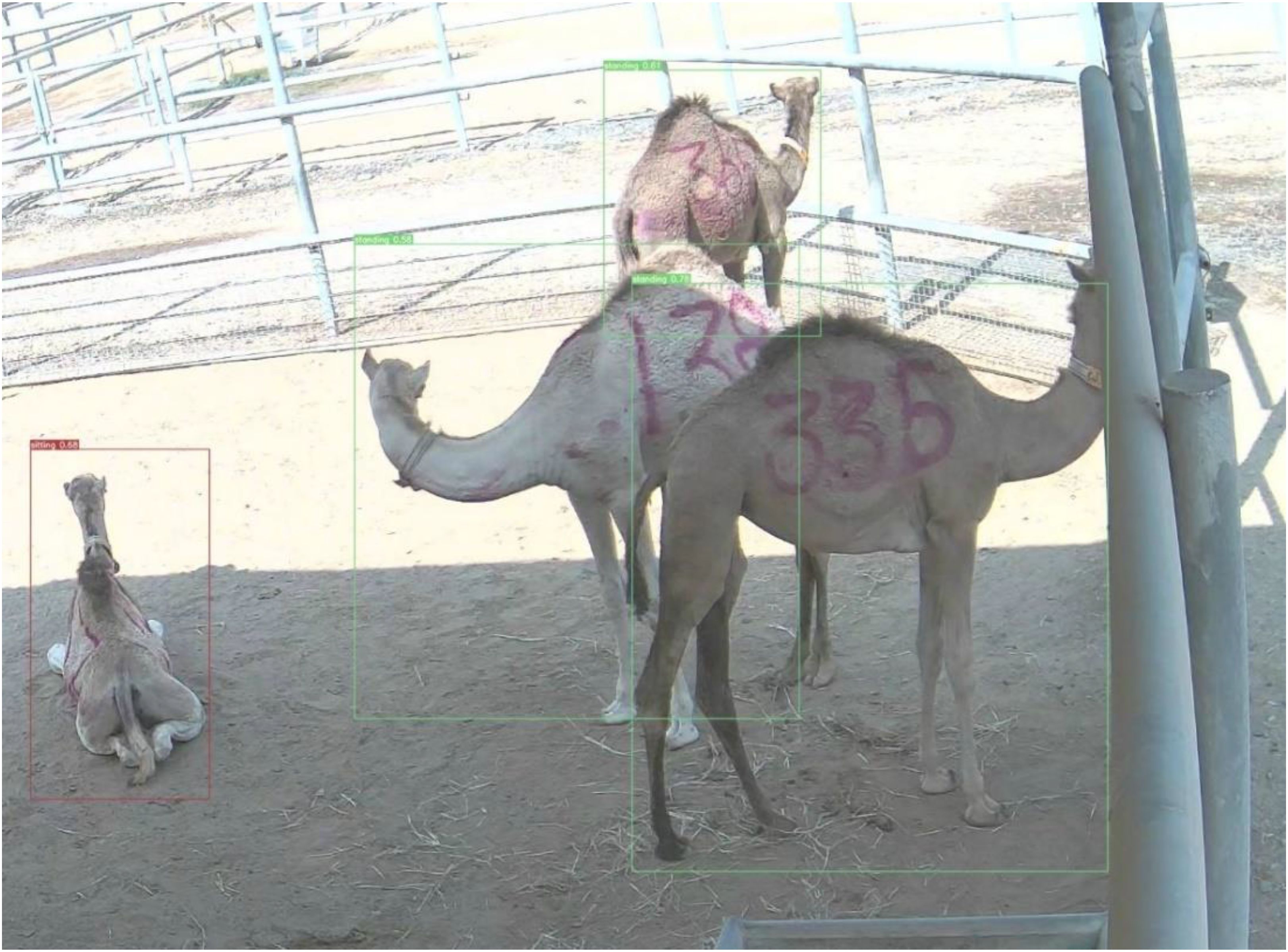
Figure 2. Detection of four camels in single frames showed three of them were standing and the fourth was sitting.
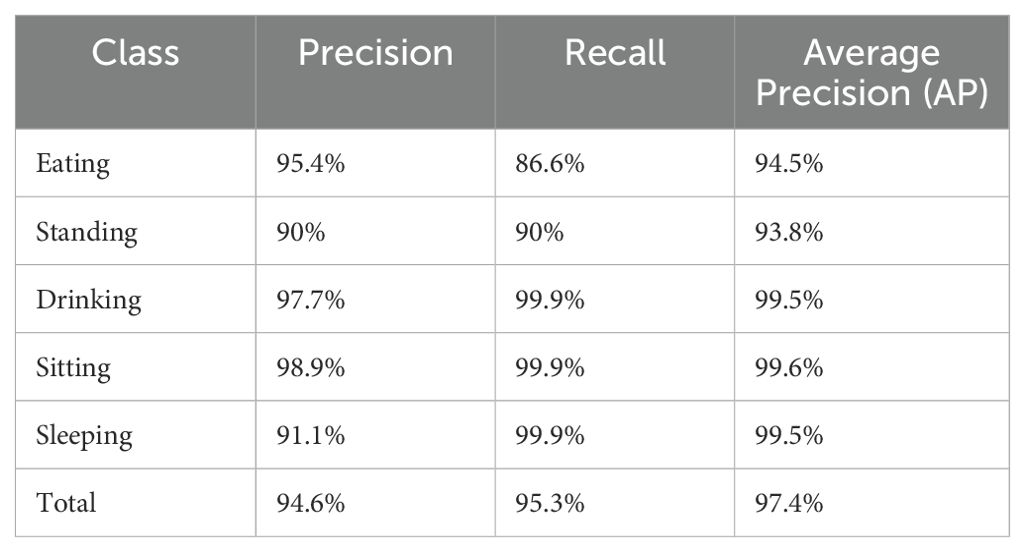
Table 2. Performance of the trained model for the classes ‘eating’, ‘sitting’, ‘standing’, ‘drinking’, and ‘sleeping’ and their total.
Detection of daily activities
The average daily activities exhibited notable differences between Phase 1 and Phase 2 of the experiment. Specifically, the average time allocated to standing and sleeping decreased from 9.8 hours (40.8%) to 6.0 hours (25.1%), and from 4.3 hours (18.0%) to 2.8 hours (11.7%) across the two phases, respectively. Conversely, the time spent sitting and eating increased from 6.2 hours (25.8%) to 9.9 hours (41.5%) and from 3.1 hours (12.8%) to 4.6 hours (19.2%) in Phases 1 and 2, respectively. The drinking activity remained consistent at an average of 37 minutes (2.6%) per day for all camels in both phases, as detailed in Figure 3.
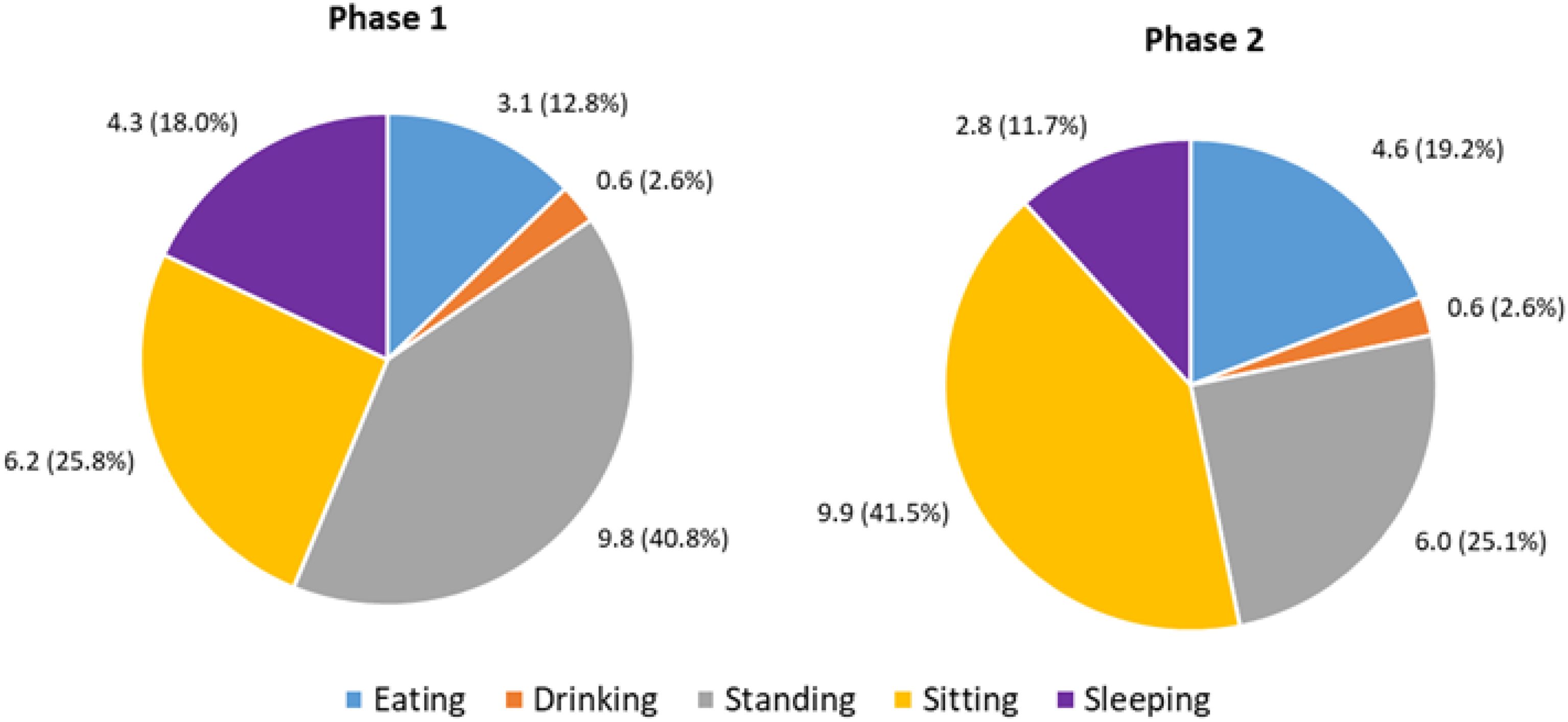
Figure 3. The difference between the two phases of the experiment is demonstrated by comparing the average duration and percentage of time spent on five activities over seven days for two camels in Phase 1 and six camels in Phase 2.
During the morning hours, all camels were dynamically engaged in eating, drinking, and standing, moving intermittently throughout the pen. From 12:00 to 16:00, their activity levels decreased significantly, with most camels resting in shaded areas. At night, the primary activities were sitting and occasional standing or walking.
The detailed breakdown of the five daily activities for the six camels during the morning, afternoon, evening, and nighttime periods in Phase 2 are presented in Figure 4. The overall averages for eating, standing, and sleeping activities during these periods did not show obvious variations among the six camels throughout the study (Figures 4A, C, E). However, there were notable variations in other activities. Camels 4 and 6 engaged in drinking activity less frequently than the other camels during the morning period (6:00-12:00), as illustrated in Figure 4B.
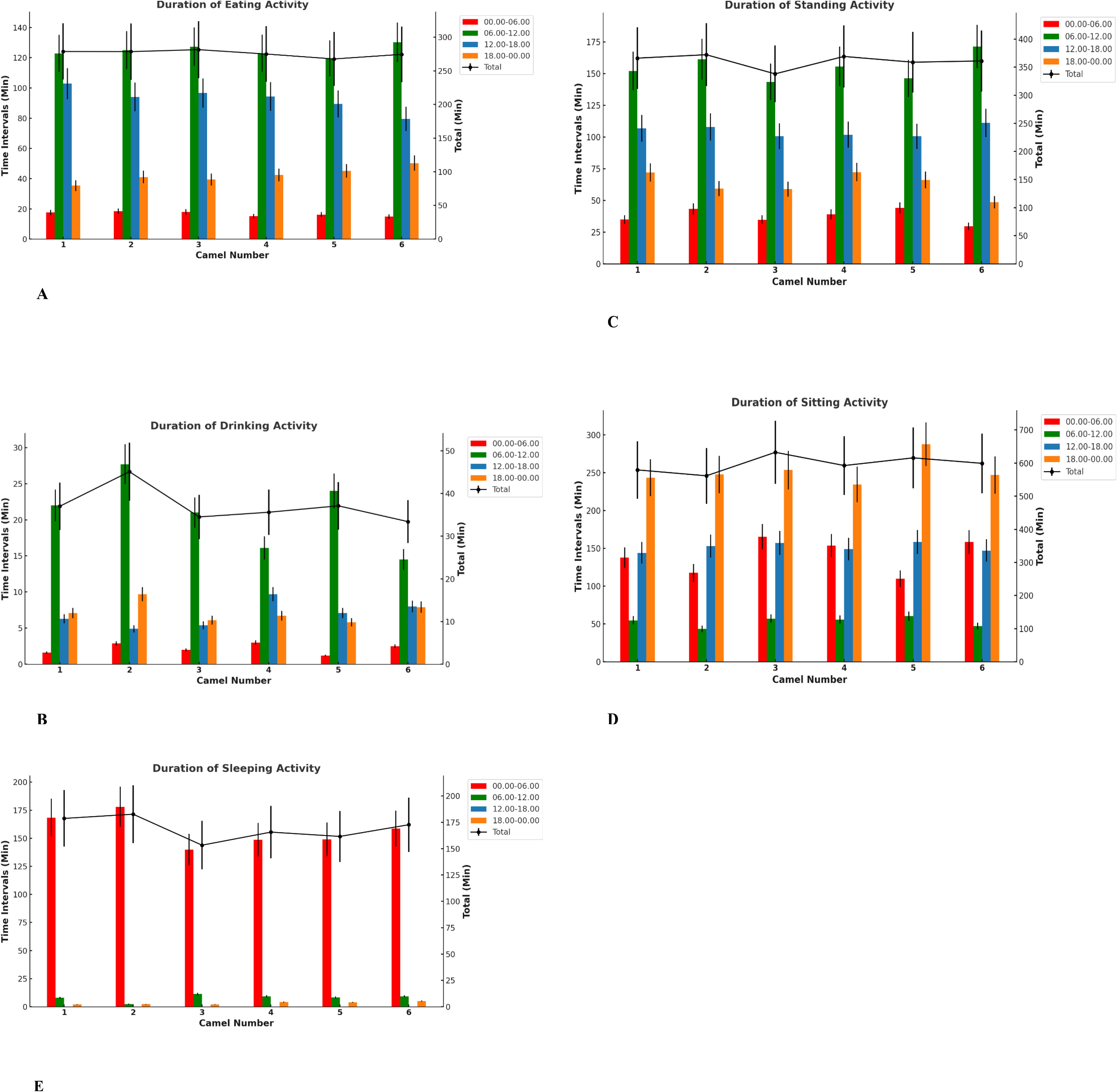
Figure 4. Duration, in minutes, that six camels spent during the five activities over the course of seven days. Columns represent the activity during certain daily periods; Solid line represents the total daily activity. Data are mean ± standard deviation (shown as vertical bars). (A) Eating; (B) Drinking; (C) Standing; (D) Sitting; (E) Sleeping.
For total sitting activity, Camel 3 followed by Camel 5 spent more time compared to the other camels, as shown in Figure 4D. Camel 5 exhibited increased sitting time during the evening (18:00-00:00) periods than the other camels. Additionally, Camels 3, 4 and 6 spent more time sitting during the night (00:00-06:00) compared to Camels 2 and 5 (Figure 4D).
Discussion
This study introduced a method to estimate the daily activities of camels using individual snapshots instead of long-term observational windows. This approach yielded greater precision and accuracy compared to traditional manual observation. A significant advantage of this method is that it does not require the attachment of collars or sensors to the camels, unlike previous studies (Fernández-Carrión et al., 2020; Robert et al., 2009), which enhances animal comfort and reduces logistical complexities. Recently, the integration of machine and deep learning technologies, with or without embedded artificial intelligence of things (AIoT) systems, has been instrumental in assessing and identifying animal behavior. These technologies provide critical insights into health, welfare, and productivity, particularly in farm animals (Maekawa et al., 2020; Arablouei et al., 2023). The use of a deep learning model to automate the analysis of camels’ daily activities provides significant improvements in farm management practices. In the current study, the trained model exhibited high accuracy in detecting and classifying the five camel activity classes: eating, standing, drinking, sitting, and sleeping. With an overall precision of 94.6%, recall of 95.3%, and an average precision (AP) of 97.4%, the model proved effective in monitoring camel daily activities. The strong performance across all activity classes highlights the model’s potential for reliable and automated behavioral analysis, making it a valuable tool for detecting and recognizing camel activities in remote farm settings. In intensive housing systems, alterations in behavioral activity can indicate unusual or challenging situations triggered by internal or external stimuli (Wechsler, 1995). Factors such as restricted space, frequent human handling, and social disturbances under farming conditions can result in the development of abnormal behaviors and may negatively affect the overall daily behavior and welfare of camels (Kutty and Yousuf, 2017).
Observational data from several days indicated that the camels were actively eating, drinking, and standing during the early morning hours, and from 12:00 to 16:00, they tended to seek shade and sit. In the evenings and at night, they primarily sat, with occasional standing and moving. Similar behavioral patterns are noted in male camels, where inactive behaviors like rumination and lying down peak during the evening and nighttime, whereas active behaviors such as feeding, walking, and stereotypical movements are most prevalent during daylight hours (Aubè et al., 2017). In response to the heat in their native desert environments, camels become less active, cluster together, and reduce their food intake to combat heat stress (Bouaouda et al., 2014). Moreover, the daily ambient temperature cycle acts as a strong zeitgeber, influencing the rhythm of camel locomotor activity, which typically begins early in the photo-phase and peaks midday (Farsi et al., 2020).
The highest levels of eating and drinking were observed between 06:00 and 12:00, and again after 16:00, extending into the evening. Given that camels are ruminants, their food and water intake is crucial as it directly impacts their physiological state and susceptibility to diseases, with any decline in these activities often indicating underlying health issues (Fesseha and Desta, 2020). Consequently, the model developed in this study can promptly notify veterinarians of any decrease in eating or drinking, potentially indicating health problems.
Sitting and standing were the predominant activities observed, suggesting that camels generally prefer to remain seated after their meals. In a recent study on farmed milking camels (Aoun et al., 2024), it was observed that the camels spent 54% of their time standing and 38% lying down, without differentiating between sitting and sleeping. Additionally, walking accounted for only 2% of their daily time and was not considered part of the standing activity. In comparison, dairy cattle are known to lie down for 8 to 16 hours per day, an activity that is positively associated with rumination (Schirmann et al., 2012). Previous studies have indicated that rumination and lying down in cattle typically commence around 70 minutes after feeding concludes (Pilatti et al., 2019). Sleep, rumination, and rest are interconnected activities that regulate metabolic functions and immunity in ruminant animals, thereby affecting their health and performance (Smutný et al., 2013; Aoun et al., 2024). In this study, camels predominantly slept at night, while daytime was characterized by eating, standing, moving around the enclosure, and sitting for rest and rumination. Camels in their natural habitats typically roam extensively in search of food and water, leading to different activity patterns compared to those observed on farms where movement is restricted (Fesseha and Desta, 2020). Activities like sleeping and sitting are less frequent in the wild, as camels expend considerable effort searching for sustenance and shade (Schmidt-Nielsen et al., 1956).
The average duration of drinking observed in the current study was only 37 minutes per day. This limited drinking behavior might be attributed to the winter season during which the study was conducted, as camels typically consume less water during colder months (Schmidt-Nielsen et al., 1956). The periods when camels drank the most coincided with their feeding times. Naturally, in cooler months, camels can go 4 to 5 days without water, drinking copiously only when a water source is found (Farsi et al., 2020; Fesseha and Desta, 2020). However, camels on a farm, with constant access to shade and water, tend to drink small quantities multiple times throughout the day, unlike their desert-dwelling counterparts who experience more significant water loss.
Eating, standing and sleeping activity were relatively consistent among camels, but there was some variation among individuals, especially in drinking and sitting activities. Such variation among individual camels, is expected (Schulte and Klingel, 1991).
Conclusion
The established deep learning model enables accurate and efficient monitoring of camels’ daily activities, making it highly suitable for managing remote farms where direct human supervision is limited. Future studies are recommended to further assess its performance across different seasons with varying weather conditions to enhance its applicability.
Data availability statement
The raw data supporting the conclusions of this article will be made available by the authors, without undue reservation.
Ethics statement
The animal studies were approved by Fujairah Research Centre scientific committee. The studies were conducted in accordance with the local legislation and institutional requirements. Written informed consent was obtained from the owners for the participation of their animals in this study.
Author contributions
RA-K: Writing – original draft, Conceptualization, Data curation, Investigation, Methodology, Software, Supervision, Writing – review & editing. NM: Conceptualization, Supervision, Writing – review & editing, Data curation, Investigation, Methodology, Software, Writing – original draft. SBM: Resources, Funding acquisition, Investigation, Visualization, Writing – original draft, Writing – review & editing. FL: Project administration, Validation, Data curation, Formal analysis, Methodology, Resources, Supervision, Writing – original draft, Writing – review & editing.
Funding
The author(s) declare that no financial support was received for the research, authorship, and/or publication of this article.
Acknowledgments
The authors wish to thank, H.H. Sheikh Mohammed bin Hamad Al-Sharqi, the Crown Prince of Fujairah Emirate, UAE for offering the research facilities and for their continuous encouragement. Our grateful thanks are extended to the team of Bulaida Farms for their cooperation.
Conflict of interest
The authors declare that the research was conducted in the absence of any commercial or financial relationships that could be construed as a potential conflict of interest.
Publisher’s note
All claims expressed in this article are solely those of the authors and do not necessarily represent those of their affiliated organizations, or those of the publisher, the editors and the reviewers. Any product that may be evaluated in this article, or claim that may be made by its manufacturer, is not guaranteed or endorsed by the publisher.
References
Aoun C. C., Atigui M., Brahmi M., Gherairi E., Hammadi M. (2024). Time budgets and 24 h temporal patterns variation of activities in stabled dairy dromedary camels. Appl. Anim. Behav. Sci. 275, 106295. doi: 10.1016/j.applanim.2024.106295
Arablouei R., Wang L., Currie L., Yates J., Alvarenga F. A. P., Bishop-Hurley G. J. (2023). Animal behavior classification via deep learning on embedded systems. Comput. Electron. Agric. 207, 107707. doi: 10.1016/j.compag.2023.107707
Aubè L., Fatnassi M., Monaco D., Khorchani T., Lacalandra G. M., Hammadi M., et al. (2017). Daily rhythms of behavioral and hormonal patterns in male dromedary camels housed in boxes. PeerJ 5, e3074. doi: 10.7717/peerj.3074
Bochkovskiy A., Wang C. Y., Liao H. Y. M. (2020). YOLOv4: Optimal speed and accuracy of object detection (Ithaca, NY, USA: Cornell University). arXiv:2004, 10934.
Bouaouda H., Achaaban M., Ouassat M., Oukassou M., Piro M., Challet E. (2014). Daily regulation of body temperature rhythm in the camel (Camelus dromedarius) exposed to experimental desert conditions. Physiol. Rep. 2, e12151. doi: 10.14814/phy2.12151
Everingham M., van Gool L., Williams C. K. I., Winn J., Zisserman A. (2009). The pascal visual object classes (voc) challenge. Int. J. Comput. Vision 88, 303–308. doi: 10.1007/s11263-009-0275-4
Farsi H., Achaâban M. R., Piro M., Bothorel B., Ouassat M., Challet E., et al. (2020). Entrainment of circadian rhythms of locomotor activity by ambient temperature cycles in the dromedary camel. Sci. Rep. 10, 19515. doi: 10.1038/s41598-020-76535-y
Faye B. (2016). The camel, new challenges for a sustainable development. Trop. Anim. Health Prod. 48, 689–692. doi: 10.1007/s11250-016-0995-8
Fernández-Carrión E., Barasona J.Á., Sánchez Á., Jurado C., Cadenas-Fernández E., Sánchez-Vizcaíno J. M. (2020). Computer vision applied to detect lethargy through animal motion monitoring: a trial on African swine fever in wild boar. Animals 10, 2241. doi: 10.3390/ani10122241
Fernández-Carrión E., Martínez-Avilés M., Ivorra B., Martínez-López B. ,. M., Ramos Á., Sánchez-Vizcaíno J. M. (2017). Motion-based video monitoring for early detection of livestock diseases: The case of African swine fever. PloS One 12, e0183793. doi: 10.1371/journal.pone.0183793
Fesseha H., Desta W. (2020). Dromedary camel and its adaptation mechanisms to desert environment. Int. J. Zool. Stud. 5, 23–28.
Hussen J., Al-Sukruwah M. A. (2022). The impact of the animal housing system on immune cell composition and function in the blood of dromedary camels. Animals 12, 317. doi: 10.3390/ani12030317
Kutty C. I., Yousuf A. M. (2017). Management practices and production performance of camels under organized farming in Abu Dhabi Emirate, United Arab Emirates. Indian J. Anim. Sci. 87, 1269–1273. doi: 10.56093/ijans.v87i10.75301
Maekawa T., Ohara K., Zhang Y., Fukutomi M., Matsumoto S., Matsumura K., et al. (2020). Deep learning-assisted comparative analysis of animal trajectories with DeepHL. Nat. Commun. 11, 5316. doi: 10.1038/s41467-020-19105-0
Mansour N., Al-Khateeb R., Lamghari F. (2024). Impact of parity on prepartum activities and behavior in dromedary camels under farm conditions. Reprod. Domest. Anim. 59, e14572. doi: 10.1111/rda.14572
Padalino B., Menchetti L. (2021). The first protocol for assessing welfare of camels. Front. Vet. Sci. 7, 631876. doi: 10.3389/fvets.2020.631876
Pilatti J. A., Vieira F. M. C., Rankrape F., Vismara E. S. (2019). Diurnal behaviors and herd characteristics of dairy cows housed in a compost-bedded pack barn system under hot and humid conditions. Animals 2, 399–406. doi: 10.1017/S1751731118001088
Redmon J., Farhadi A. (2018). YOLOv3: an Incremental Improvement (Cornell University: Ithaca, NY, USA).
Rice C. A., Eberhart N. L., Krawczel P. D. (2017). Prepartum lying daily activity of Holstein dairy cows housed on pasture through parturition. Animals 7, 32. doi: 10.3390/ani7040032
Robert B., White B. J., Renter D. G., Larson R. L. (2009). Evaluation of three-dimensional accelerometers to monitor and classify daily activity patterns in cattle. Comput. Electron. Agric. 67, 80–84. doi: 10.1016/j.compag.2009.03.002
Schirmann K., Chapinal N., Weary D. M., Heuwieser W., Von Keyserlingk M. A. G. (2012). Rumination and its relationship to feeding and lying daily activity in Holstein dairy cows. J. Dairy. Sci. 95, 3212–3217. doi: 10.3168/jds.2011-4741
Schmidt-Nielsen B., Schmidt-Nielsen K., Houpt T. R., Jarnum S. A. (1956). Water balance of the camel. Am. J. Physiol. 185, 185–194. doi: 10.1152/ajplegacy.1956.185.1.185
Schulte N., Klingel H. (1991). Herd structure, leadership, dominance and site attachment of the camel, Camelus Dromedarius. Behaviour 118, 103–114. doi: 10.1163/156853991X00229
Schütz A. K., Schöler V., Krause E. T., Fischer M., Müller T., Freuling C. M., et al. (2021). Application of YOLOv4 for detection and motion monitoring of red foxes. Animals 11, 1723. doi: 10.3390/ani11061723
Smutný L., Smutná Š., Kindlová J., Šoch M., Škeˇrík V., Zábranský L. (2013). The usage of information technology for evaluation of animal welfare. Anim. Sci. Biotechnol. 17, 137–144. doi: 10.2478/aucft-2013-0022
Tibary A., El Allali K. (2020). Dromedary camel: A model of heat-resistant livestock animal. Theriogenology 154, 203–211. doi: 10.1016/j.theriogenology.2020.05.046
Wechsler B. (1995). Coping and coping strategies: a behavioural view. Appl. Anim. Behav. Sci. 43, 123–134. doi: 10.1016/0168-1591(95)00557-9
Keywords: dromedary camels, deep learning, activity pattern, camel farm, welfare
Citation: Al-Khateeb R, Mansour N, Mirza SB and Lamghari F (2024) Deep learning-based analysis of daily activity patterns of farmed dromedary camels. Front. Anim. Sci. 5:1445133. doi: 10.3389/fanim.2024.1445133
Received: 06 June 2024; Accepted: 25 November 2024;
Published: 11 December 2024.
Edited by:
Paul Lelovas, National and Kapodistrian University of Athens, GreeceReviewed by:
Claudia Gianetto, University of Messina, ItalyDerek Wayne Bailey, New Mexico State University, United States
Copyright © 2024 Al-Khateeb, Mansour, Mirza and Lamghari. This is an open-access article distributed under the terms of the Creative Commons Attribution License (CC BY). The use, distribution or reproduction in other forums is permitted, provided the original author(s) and the copyright owner(s) are credited and that the original publication in this journal is cited, in accordance with accepted academic practice. No use, distribution or reproduction is permitted which does not comply with these terms.
*Correspondence: Nabil Mansour, bmFiaWwubWFuc291ckBmcmMuYWU=