- Animal Welfare Program, IRTA, Monells, Spain
Extensive farming is often considered very beneficial to animals for its potential to enhance animal welfare, providing animals with free-range access to their natural environment where they can engage in innate behaviors like grazing and exploration. However, despite these benefits, extensive production still faces welfare and health challenges due to unpredictable weather conditions and limited supervision by stockholders. Moreover, increasing consumer demand for information regarding food quality, safety, and production conditions poses a challenge for extensive farming, where animals are less controlled. Precision livestock farming (PLF) emerges as a possible solution by enabling the continuous real-time monitoring of the health, welfare, and behavior of animals. A novel approach combining geolocation collars for cows and Bluetooth low energy (BLE) ear tags for calves appears promising to enhance traceability and monitoring in extensive farming. Nevertheless, challenges persist, including limitations in the data transmission capacity and associated costs. This study evaluated the effectiveness of combining geolocation collars and BLE ear tags for monitoring calf traceability and cow–calf relationships across three scenarios: 1) Equilibrated: same collar/ear tag ratio; good coverage; 2) intermediate: more collars than ear tags; fair coverage; 3) worst: more ear tags than collars; lousy coverage. Our results indicate that the equilibrated scenario (ES) with an equal number of geolocation collars and BLE ear tags, was the best scenario, demonstrating the highest fix rate (22%) and the longest mean consecutive days of detecting the same ear tag (22.30 days), followed by the intermediate scenario (IS) and the worst scenario. In all scenarios, there was a mean period of 14–15 d without detecting a calf. However, this shortcoming can be overcome as calves usually graze alongside their mothers, ensuring comprehensive traceability in farm breeding. Additionally, by comparing differences in the number of ear tags received from offspring compared to other calves, the BLE ear tags successfully associated every mother with their calf in the ES and IS. Finally, this valuable information, would enable the development of a traceability system that ensures transparency and reliability throughout the supply chain and could allow consumers to access to product information related to animal welfare.
1 Introduction
Traditionally, farm animal welfare research has predominantly focused on issues related to intensive production, while extensive farming has received less attention. This discrepancy may stem from the prevailing assumption that extensive farming inherently guarantees animal welfare. It is true that extensive environments are more likely to provide the conditions that animals require to perform innate behavioral needs (Hemsworth et al., 1995), such as grazing and exploration. Nevertheless, the ability to exhibit these behaviors may be constrained by environmental limitations. While extensive systems offer numerous welfare advantages to animals, they suffer from a wide range of welfare issues that are hard to predict, difficult to detect due to infrequent animal inspections, and often difficult to mitigate. These environments are highly variable regarding climate conditions, food quality, high-quality water access, and wildlife coexistence; thus, serious welfare concerns related to chronic hunger and thirst, thermal stress, and high mortality rates may arise. Additionally, other problems may arise such as more difficulties treating diseases compared to intensive systems, more peripartum deaths due to the uncontrolled natural conditions during parturition, difficulties reliably identifying cow–calf pairs, early exposure of calves to an environment with predators, and less animal supervision by human caretakers. Mitigating these welfare issues is challenging because of large pasture areas and, in some cases, poor access. Furthermore, overcoming these issues is time and money consuming for farmers, further decreasing profits from the already low profit margins that are common in extensive systems (McLean and Holmes, 2015; Hocquette et al., 2018).
Detection and monitoring of welfare issues in extensive systems are essential for implementing practical solutions. In this context, precision livestock farming (PLF) aims to manage individual animals by continuously monitoring their health, welfare, production/reproduction, and/or environmental impact in real-time (or close to real-time) (Berckmans, 2017). PLF can monitor animals with greater detail than farmers (Daigle, 2014). With increasingly reliable animal welfare measures and the decreasing costs of on-animal sensors, technology adoption will likely expand, mainly if algorithm development ensures validity and reliability (Manning et al., 2021). For instance, animal location and tracking allow users to evaluate animal movements around landscapes (Gordon, 2001); animal performance, activity, and behavior (Turner et al., 2000); or social affiliations within the herd (Veissier et al., 1998; Senneke et al., 2004). PLF has the potential to provide significant information to support better animal welfare practices and deliver early alerts regarding potential well-being issues (Trotter, 2018). Initial GPS-based systems utilized high-temporal resolution GPS trackers that recorded locations every few seconds or minutes. While these devices have proven to be suitable for research purposes, their practicability in commercial farm settings is limited by various factors such as lack of robustness, limited battery life, absence of wireless data transmission, and high costs, which often outweigh their value to the animals and farmers (Polojärvi et al., 2010; Bailey et al., 2018). However, significant advancements have been achieved in recent years in commercial geolocation tracking devices. These newer devices can record fixes over extended periods, spanning months or even years, and transmit real-time data through low-power wide-area (LPWA) networks (Maroto-Molina et al., 2019). Additionally, the typical temporal resolution range of these commercial devices is 15 min–2 h to prolong battery life. These devices are part of the Internet of Things (IoT) technology that can collect massive amounts of data at almost any time in any place and environmental conditions. Using these data, it is possible to analyze and evaluate the status of the monitored objects. Therefore, geolocation tracking devices with IoT technology and integrated sensors, actuators, and smart devices—key to the development of real-time applications—can be widely used in livestock farming. Geolocation tracking devices provide long-range communication up to 10–40 and 1–5 km in rural and urban areas, respectively. Thus, an entire city or several farms can be covered by a single base station (Mekki et al., 2019). In addition, LPWA networks are highly energy efficient and inexpensive, where a radio chipset costs less than 2 € and operating it costs 1 € (Raza et al., 2017). In recent years, despite significant advancements in geolocation technology for animal tracking, several constraints have impeded their utilization as PLF tools, particularly in extensive production systems. One major hurdle is the complexity of wireless data transmission, especially in rural or mountainous regions. While IoT offers a potential solution to this challenge, IoT developments dedicated to animal tracking are scarce (Dieng et al., 2017; Nóbrega et al., 2018). Additionally, deploying coverage through an antenna network entails an initial investment. Among the LPWA networks, Sigfox emerges as one of the most widely utilized options. Sigfox employs binary phase-shift keying modulation within an ultra-narrow band (100 Hz) and unlicensed ISM bands, such as 868 MHz in Europe (Mekki et al., 2019). However, Sigfox has a maximum message size of 12 bytes and constraints on transmission technology duty cycles. Moreover, the ISM band of Sigfox is limited to a maximum utilization of 1%/h, equating to a maximum of 140 messages/day at 10-min intervals. These constraints shape the operational parameters and scalability of Sigfox-enabled systems for animal tracking applications (Maroto-Molina et al., 2019). Furthermore, because the maximum number of messages is related to energy consumption, a year of continuous recording requires the system to send a message every 30 min; thus, a maximum of 48 messages can be sent per day. Conversely, the cost of geolocation tracking systems (generally 100–200 €/unit) and antennas (approximately 1000 €), could hinder the widespread adoption of these technologies, as it may be unfeasible to provide a device for all the animals from the herd (Davis et al., 2011).
Despite these limitations, geolocation collars are seen as a feasible technology to be implemented with blockchain technology for traceability applications. Blockchain technology offers a higher level of data security using decentralized and distributed ledger systems (Azizi et al., 2021) that are immutable, avoiding fraud. Combining IoT devices, geolocation collars, and blockchain technologies into a system can provide comprehensive, reliable, and cost-effective tools to monitor beef cattle. This system should ensure livestock traceability throughout the entire supply chain by identifying in real-time, through a cloud platform, the location and status (i.e. position and animal presence) of herds in the field. This information can be made visible through a QR code that can easily be added to the labelling of the products, thus enhancing trust among participants involved in the food system (Menon and Jain, 2021). This information might affect the perception and preference of consumers and add extra value to the products of animals reared in specific conditions, such as outdoors. However, implementing this technology presents certain challenges notably with geolocation collars that are unsuitable for calves because the neck size of growing animals increases during their developmental stages, which could produce lesions or even asphyxia if a not adjustable collar is used. This presents a problem because consumers demand full traceability of veal products from farm to fork, what means traceability from calves rather than from their mothers. To address this issue, a new PLF technology has emerged in the form of low-cost Bluetooth low energy (BLE) ear tags. These BLE ear tags can be seamlessly connected to geolocation collars and configured to transmit advertisement messages containing device identification and owner-specific codes. This streamlined approach facilitates the creation of smaller devices with reduced battery consumption, making them suitable for calf ears. In this setup, each geolocation collar collects data regarding the animal’s location, while also recording the identification of the BLE ear tags in its vicinity. The BLE ear tags transmit advertisement messages asynchronously and at regular intervals, with each message limited to eight identifier-power pairs of BLE ear tags owing to the limitations of the Sigfox message size. This enables users to approximate the location of animals equipped with BLE ear tags and provide useful data of animal affiliation that could be achieved by quantifying social contacts between cows and calves to enable accurate maternal identification (Senneke et al., 2004). Social interactions of cows and calves within larger groups are more complex and will vary depending on the age of the calf and the strength of the bond with the mother (Hirata et al., 2003). Moreover, calves are not always close to their mothers and often distance themselves from their mothers as they mature.
Therefore, this study aims to assess the effectiveness of combining two technologies: geolocation collars and BLE ear tags. The geolocation collars are fitted to the cows and are connected to the BLE ear tags on the calves. The study compares three distinct scenarios with varying coverage, geolocation collar and BLE ear tag ratios, and time periods to identify the most efficient system for accurately monitoring the conditions of calves. For all scenarios, the study is performed in mountainous areas, where using this type of devices is more challenging. Simultaneously, the work investigates the effectiveness of the combined technologies to evaluate aggregating behaviors between cow–calf interactions and the rest of the herd. As a potential ultimate objective, the obtained information could be integrated into a reliable traceability system containing an integrated blockchain procedure that may be accessed by consumers.
2 Materials and methods
2.1 Study trials
Three commercial farms served as test sites to validate the performance of the proposed system across diverse zones, coverage areas, collar/tag ratios, and period times. These trials were conducted in Garrotxa province in Catalonia, Spain, characterized by a Mediterranean climate with one dry season in winter. The average temperature hovers around 12°C, with an annual precipitation range of 850–1,100 mm (METEOCAT, 2023). The primary forage resource consists of natural grass, especially prevalent during fall and spring when rainfall is abundant. Additionally, all farms are situated in oak forests (Quercus ilex) and natural meadows, housing Angus beef cattle.
We defined three scenarios to analyze the efficacies of these technologies, all in a mountainous area. a) The equilibrated scenario (ES) had an equal collar/ear tag ratio (1/1) and a good Sigfox coverage. The ES was conducted in the Can Casademunt farm located in Vidrà (42.12° N, 2.32° W). The validation study involved a herd of 54 animals, in which 23 randomly selected cows had geolocation collars (Digitanimal Ltd., Madrid, Spain) and 23 calves had BLE ear tags (Digitanimal Ltd., Madrid, Spain) (ratio=23/23). b) The intermediate scenario (IS) had a greater collar/ear tag ratio. Thus, there were more possibilities for an individual calf to find an adult cow with a collar (including their mother) and a fair Sigfox coverage. The IS was conducted in the Can Carrera farm situated in Sant Esteve d’en Bas (42.10° N, 2.47° W) with a total herd of 47 animals, in which 16 cows had geolocation collars and 13 calves had BLE ear tags (ratio=16/13). The cows and calves in the ES and IS grazed freely on 146 hectares during 5 months from May to September 2021 for 150 consecutive days. c) The worst scenario (WS) had a lower collar/ear tag ratio and a poor Sigfox coverage. The WS was conducted in Font Rubí located in Camprodon (42.32° N, 2.41° W) with a herd of 59 animals, in which 10 cows had geolocation collars and 20 calves had BLE ear tags (ratio=10/20). In this case, the herd was tracked in a 100-hectare free-range area for 75 consecutive days from May to July 2021.
2.2 Devices components and configuration
The system operates by fusing two devices: geolocation collars affixed to cows and linked to an LPWA network (Sigfox, Labège, France), and BLE ear tags affixed to calves and connected to the cow geolocation collars. Prior to the study, we fitted various adult animals with geolocation collars, and their respective calves were equipped with BLE ear tags upon identification and registration. This allowed us to compare the information obtained from the offspring of the cows with that of the other calves.
The geolocation collars were equipped with various hardware components, including a Sigfox-based long-range IoT communications module for message transmission, a BLE-based short-range communications module for reading BLE ear tag advertisements, a positional device unit for location data collection, a microcontroller running low-level firmware, a lithium battery pack, and an IP67 enclosure. These collars operated in two modes: idle mode, consuming less than 20 µA, and active mode, in which power consumption varied owing to the different components. The hot-start mechanism optimized consumption during the operation of the geolocation collar. A coverage search required over 30 mA, sending Sigfox messages required 30 mA, and receiving BLE ear tag advertisements required 15 mA. The temporal resolution was set at 30 min, allowing the geolocation collars to send a maximum of 48 messages daily and ensuring a battery life exceeding 365 days. The BLE ear tags comprised a Bluetooth 4.2 communications module, a microcontroller, a coin battery, and an IP67 enclosure. Each BLE ear tag transmitted an advertisement containing the device identification and owner code. Power consumption of the BLE ear tag in the idle state was 2.0 µA, which rose to 7.5 mA during transmission to optimize coverage. There was a trade-off between power consumption and network performance as broadcasting with higher power widens coverage but reduces operational life. Considering the need for prolonged operational life, the BLE ear tags were configured with a 1-s message interval, ensuring an average operational life of 280 days. This configuration prioritized power efficiency, crucial for the intended use of the system in commercial farms, where continuous animal tracking demands sustained location data availability.
2.3 System operation
The system operated as follows: (1) when the geolocation collar was inactive, it remained in the idle mode, consuming minimal power; (2) every 30 minutes, the geolocation collar activated to collect location data; (3) simultaneously, the BLE communications module, configured to maximize the reading range (over 75 m, depending on habitat characteristics), scanned the surrounding BLE ear tags for several seconds; (4) BLE ear tags on nearby animals sent advertisement messages asynchronously and regularly, at predefined intervals of several seconds, when they were close to a geolocation collar. To ensure reception, the operation window lasted for several cycles; (5) the geolocation collar combined data from its unit and the BLE module, encoding it to be transmitted through the Sigfox communications module. Each message included geolocation data and identifier-power pairs corresponding to detected BLE ear tags; and (6) messages sent by geolocation collars were received by the cloud server, where they were extracted and analyzed (Figure 1).
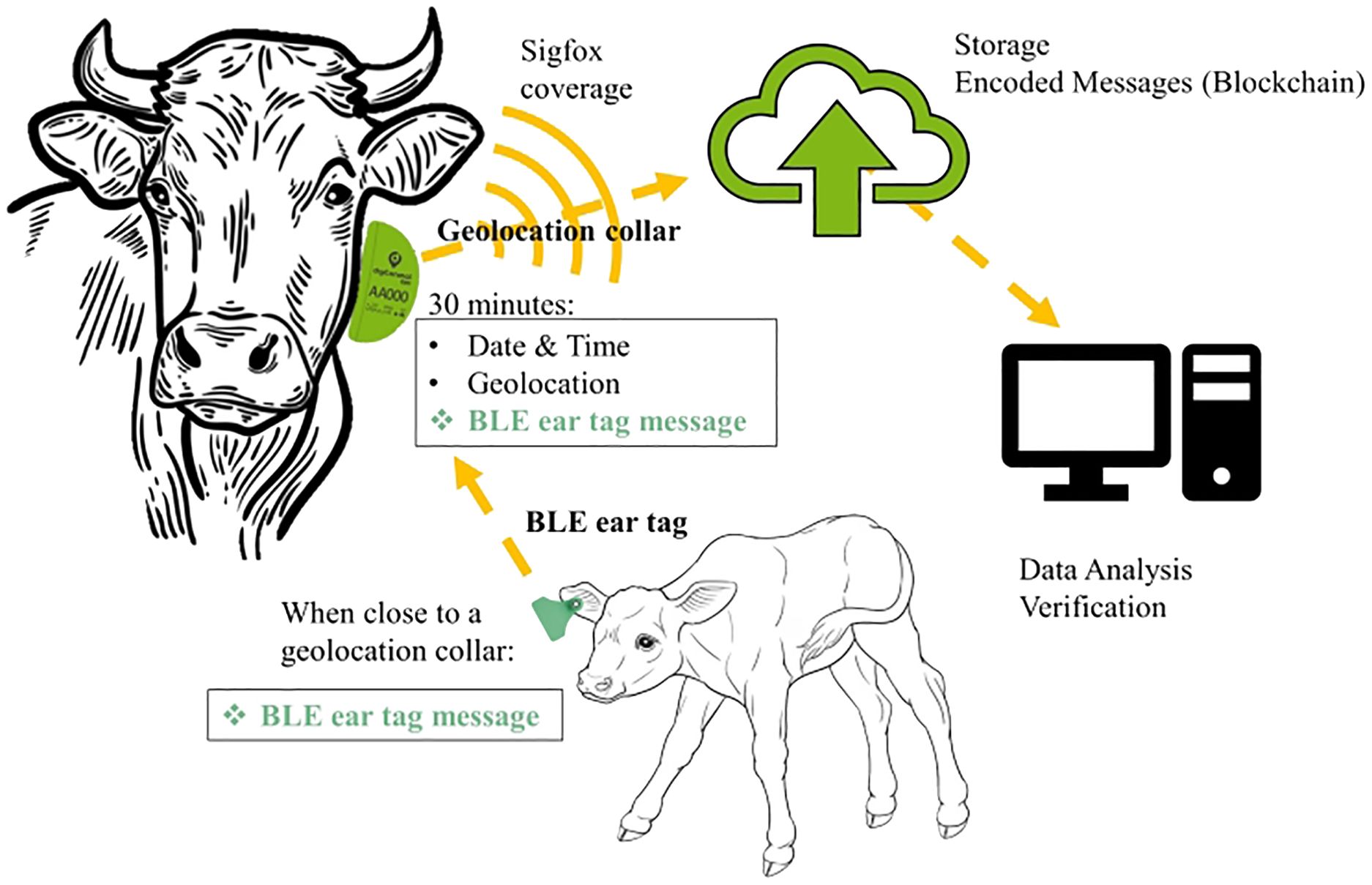
Figure 1. Diagram of system operation through the interaction of geolocation collars and BLE ear tags.
2.4 Data analysis
We performed a general system performance analysis for every scenario (ES, IS, and WS) with the mean number of geolocation collar and BLE ear tag messages received per day, the fix rate of the geolocation collars as the number of signals sent per day divided by the maximum number of signals that the system can send in a day (48), the mean number of devices working over the days, and the mean number of messages per hour sent by the devices over the whole day.
To prove that calf activity could be detected with the BLE ear tags in the three scenarios, we analyzed the number of BLE ear tags detected by all geolocation collars over the day, the number of BLE ear tags included in each message, the time between two consecutive messages from the same BLE ear tag, the maximum number of consecutive days that a single BLE ear tag was detected, and the maximum number of days that no BLE ear tags were detected. Additionally, we calculated the number of days that all the BLE ear tags were detected by the geolocation collars and the percentage of active days of the BLE ear tags. The mean and standard deviation were calculated for each variable.
To study aggregative relationships between the cows and their calves, we compared, for each cow, the total, mean, and percentage of signals received daily by the BLE ear tag of their calf with those of the unrelated calves. Owing to the non-normal distribution of the error variability in the number of BLE ear tag signals, we employed the Kruskal-Wallis test to identify variations among the signals emitted by different BLE ear tags. In cases where differences were detected, we applied a post-hoc Dunn test for post-hoc comparison among every BLE ear tag.
For the line graphics included in the present paper, a trend line was drawn to improve the clarity of the charts. R Project (R Core Team, 2014) was used for data handling, calculations, and graphics via the following R packages: “dunn.test” (Dinno, 2017) and “ggpubr” (Kassambara, 2023).
3 Results
3.1 System validation
During the experiment, a total of 23,356 geolocation collar messages were gathered from the three scenarios. In the ES, IS, and WS 18,178, 4,529, 649 geolocation collar messages were collected, respectively. Each of these messages contained data about the collar location and the BLE ear tags within close proximity. Altogether, 47,255 BLE ear tag messages were gathered: 37,757 (29.1 ± 19.2 reads/BLE ear tag/day) from the ES, 7,851 (15.1 ± 11.00 reads/BLE ear tag/day) from the IS, and 1,647 (3.63 ± 3.65 reads/BLE ear tag/day) from the WS. To validate the system, it was necessary to analyze different parameters such as the mean geolocation collar messages per day, the fix rate of the geolocation collars, and the number of functional devices per day. The mean geolocation collar messages sent per day were 10.5 ± 8.06 (22% fix rate), 6.71 ± 5.61 (14% fix rate), and 2.91 ± 2.73 (6% fix rate) for the ES, IS and WS, respectively over a maximum of 48 attempts (every 30 min).
As shown in Figure 2, in the ES, the mean messages per day of the geolocation collars and BLE ear tags decreased over the time, where 16.90 and 4.11 messages/day were sent before and after mid-July, respectively. In the IS, the number of geolocation collar and BLE ear tag messages sent was irregular during the study and the BLE ear tags showed a progressive reduction in the messages sent at the end of the study. In contrast, in the WS, the mean number of messages from both the geolocation collars and BLE ear tags was regular, with a notable rise during July, although we could only record data from late May to July.
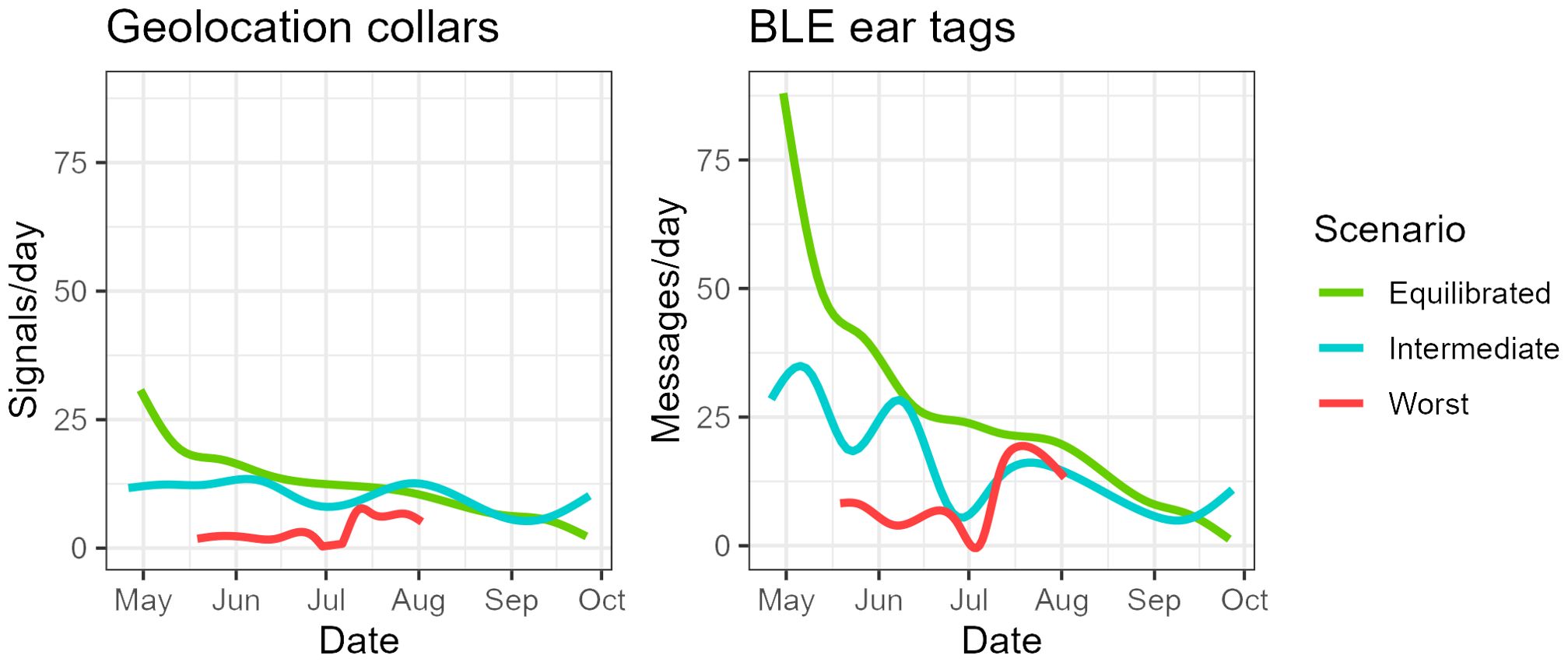
Figure 2. Mean number of signals and messages received daily from (A) geolocation collars and (B) BLE ear tags over study period in three scenarios: equilibrated (green line), intermediate (blue line), and worst (red line).
Generally, more geolocation collars were active than the detected BLE ear tags in all three scenarios since the BLE ear tags depended on the geolocation collars to send their messages; however, the BLE ear tags sent more messages than the geolocation collars (Figure 2). Therefore, an undetected BLE ear tag did not mean that the BLE ear tag was dysfunctional. Furthermore, the number of activated and detected devices decreased over time in the ES and IS, while it increased in the WS (Figure 3).
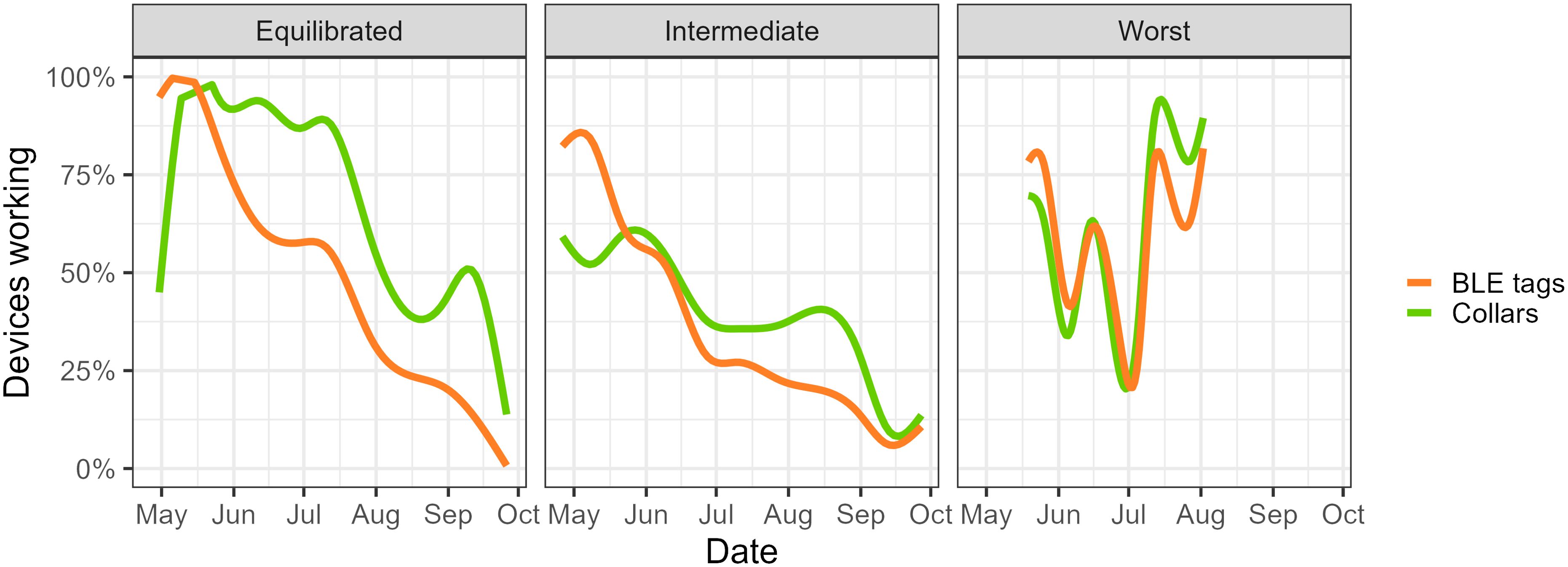
Figure 3. Progress of number of geolocation collars activated (green lines) and BLE ear tags detected (orange lines) during study in three scenarios (equilibrated, intermediate, and worst).
The messages sent in the different hours over the day remained similar in all scenarios; however, fewer ear tag messages were sent at night (Figure 4).
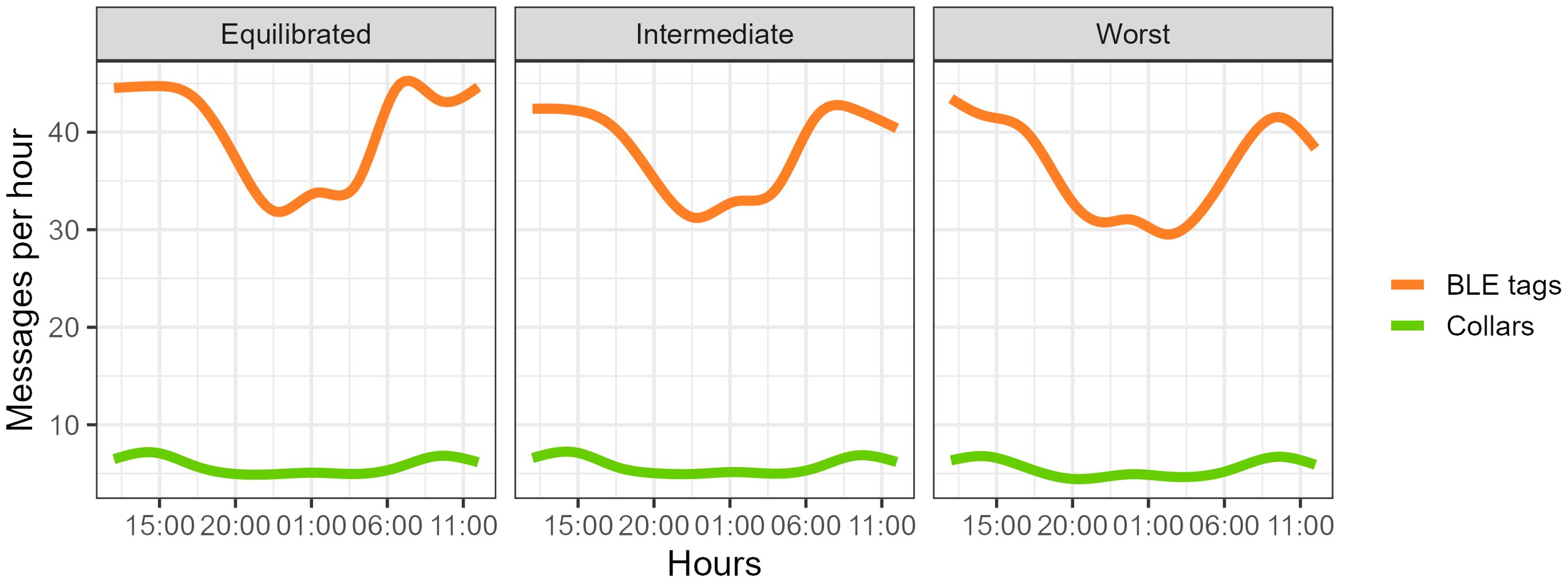
Figure 4. Progress of number of messages from geolocation collars sent hourly (green lines) and BLE ear tags detected (orange lines) hourly during study in three scenarios (ES, IS, and WS).
3.2 Calf activity detection
We found that the number of BLE ear tags read at least once every day was higher in the ES and WS (Table 1). In the ES, 17 days was the longest that all BLE ear tags were detected, while the other scenarios never detected all the BLE ear tags in one day. Eventually, the mean number of BLE ear tags included in each geolocation collar message was the same in all scenarios. This indicates that regardless of the number of geolocation collars and BLE ear tags used, each signal from a geolocation collar typically transmits a mean of three and a maximum of nine BLE ear tag messages, showing a maximum capacity of the collars to detect nine different ear BLE tags during the study.
To verify that the BLE ear tags obtained enough information from the calves during the pasture season, we determined the capacity of the devices to detect the calves over time continuously. The ES was the best scenario compared with the IS and WS, with 37.80% of active BLE ear tags over time versus 28.20 and 30.30%, respectively (p < 0.05), 63.50 min between BLE ear tag readings versus 136 and 1,054 min, respectively (p < 0.05), and 22.30 consecutive days that BLE ear tags were detected versus 19.17 and 4.23 days, respectively (p < 0.05) (Table 2). Nonetheless, the ES had the highest variability in the active days of the BLE ear tags (Figure 5).
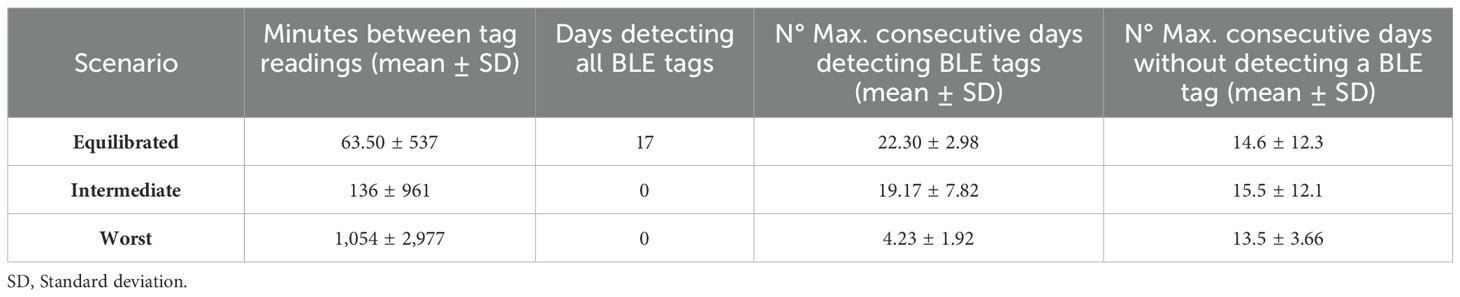
Table 2. System capacity of monitoring BLE ear tag messages through geolocation collars for each scenario.
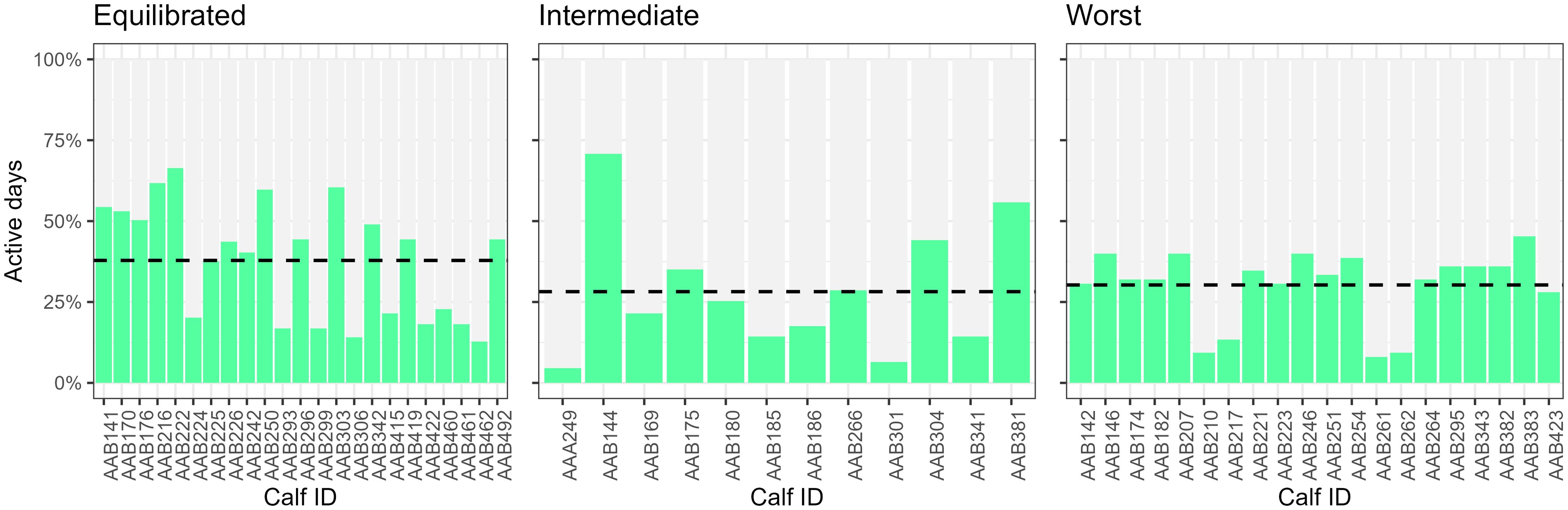
Figure 5. Percentage of active days that BLE ear tags were detected during study in three scenarios (equilibrated, intermediate, and worst). Dashed line in black indicates mean percentage of active days for each scenario.
Because many days without a message from the calf may negatively affect the reliability of the data, we analyzed the maximum number of consecutive days without information from a calf. In this case, the results indicated differences among the three scenarios; however, if we compare the means, there was a one-day difference among the scenarios, and all scenarios had a maximum mean of 14–15 days without detecting a calf (Figure 6).
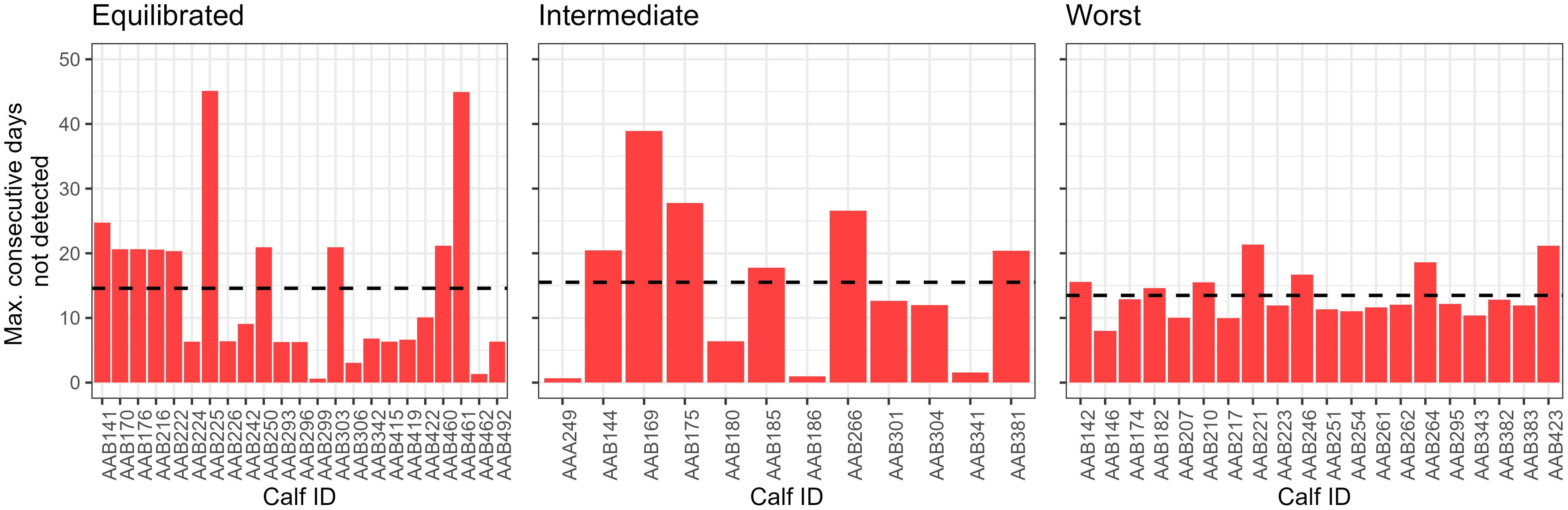
Figure 6. Number of maximum consecutive days without detecting a BLE ear tag during study in three scenarios (equilibrated, intermediate, and worst). Dashed line in black indicates mean percentage of active days for each scenario.
Additionally, in the ES, the maximum consecutive days without information from a calf was 45 days in two different calves (AAB225 and AAB461), which means that, for 30% of the days, the two calves were not detected by a geolocation collar (Supplementary Material Table 1). The calf age was found to be unrelated to the undetected calves because there was a wide age range of 1–16 months between the detected calves. Moreover, the mean messages per day did not differ (R2 = 0.01; d.f = 46; p = 0.52).
3.3 Cow–calf affiliation behavior
To study the degree of cow–calf affiliation, we compared a total of 14 cows with their corresponding offspring. Each cow had a geolocation collar and their offspring had a BLE ear tag. In the ES, IS, and WS, every cow received a mean of 11.69 ± 5.23, 7.18 ± 1.03, and 1.56 ± 0.68 messages/day from their offspring, respectively.
To determine if we were able to identify each calf and its mother in the three scenarios, we compared the mean signals per day that the cows received from their offspring with the mean signals received from unrelated calves. The results show that in the ES and IS, the cows with geolocation collars consistently received a statistically higher number of signals from their offspring compared to all unrelated calves (p < 0.05) as can be seen in Table 3 (see Figure 7 for a graphical visualization of four different cows as examples). However, in the WS, the most challenging trial, the number of signals received was notably lower, and it was impossible to distinguish the cows and their offspring from unrelated calves. Comparing the three different cows with geolocation collar, we found that they received statistically similar mean signals from their offsprings and from others unrelated calves (Table 3).
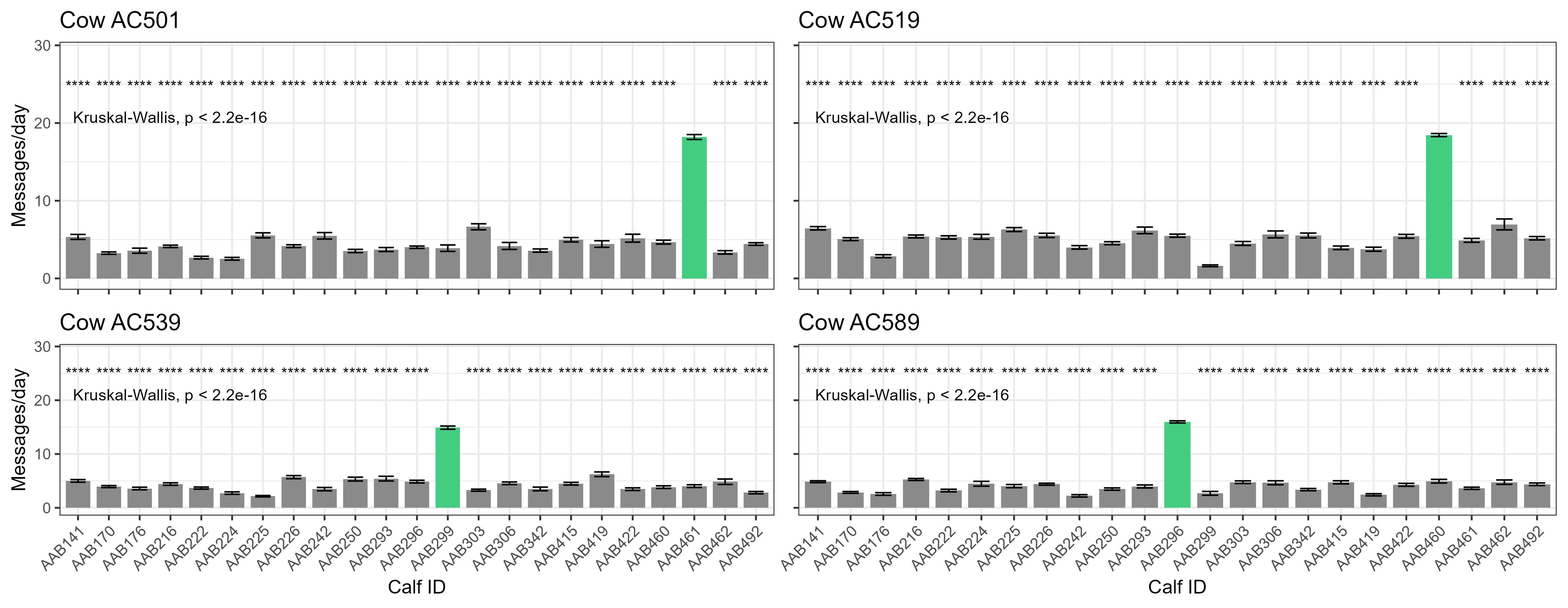
Figure 7. Graphical visualization of the data presented in Table 3 as the mean number of messages received from different BLE ear tags from four different geolocation collars from equilibrated scenario (cows’ calf in green). Asterisks indicate statistical significance in post-hoc comparisons according to Dunn test. Test P < 0.10(*); P < 0.05(**); P < 0.01(***); P < 0.001(****).
4 Discussion
In this study, we proposed a system to monitor animals in extensive livestock farming for different conditions using geolocation collars in adult animals and BLE ear tags in growing animals. The monitoring of growing animals is highly demanded by consumers since veal products are produced from these animals (Dickinson and Bailey, 2002; Gracia and Zeballos, 2005).
First, we validated the system in three scenarios based on the collar/tag ratios and Sigfox coverage. The fix rates of the geolocation collars were 22, 14, and 6% for the ES, IS, and WS, respectively. These results show the coverage differences among the scenarios. These fix rates are very low compared to Maroto-Molina et al., 2019, Hofmann, 2022, and Ulloa-Vásquez et al., 2022 using a Sigfox (LPWA) network with fix rates of 66, 62.3, and 90%, respectively. This may be explained because in most of those studies the terrain was flat, the area was smaller, and the canopy cover was less dense. In contrast, in our study, the animals moved freely in a larger area around irregular terrain with dense canopy cover from oak forests in a mountainous area. Several authors have reported that habitat characteristics, such as topography and tree canopy, may significantly reduce location acquisition (Rempel et al., 1995; Di Orio et al., 2003). This highlights the difficulties of adopting these technologies in mountainous areas where the coverage (e.g. Sigfox) can change dramatically from one point to another. Consequently, the fix rates found in this study varied a lot among scenarios over time and depended on the animal movements. Nonetheless, these low fix rates may be enough for most applications in commercial farms and for some research studies.
According to the results obtained from the different scenarios, the ES had the most efficient combination to successfully detect calf activity, with the same number of geolocation collars and BLE ear tags, and the best Sigfox coverage (fix rate of 22%). In the ES, a major number of different BLE ear tags were detected, in which all BLE ear tags were detected for 17 d and the same BLE ear tag was detected for a maximum mean of 22.30 consecutive days. The second-best scenario was the IS with more geolocation collars than BLE ear tags, followed by the WS with less geolocation collars than ear tags, and the worst Sigfox coverage. Therefore, high fix rates are inessential as better coverage will always contribute to a higher quality and quantity of information. The results agree with those of Maroto-Molina et al. (2019), which showed an increase in the total number of messages from the BLE ear tags as the number of animals fitted with geolocation collars increased.
A common limitation observed across all scenarios was that the geolocation collars could only transmit a mean of three to a maximum of nine messages from the BLE ear tags at a time. This suggests that to enhance the amount of information collected about the calves, the number of geolocation collars should be increased. Nevertheless, because calves exhibit a stronger affiliation and proximity to their own mothers compared to other cows (Veissier et al., 1990; Swain and Bishop-Hurley, 2007; Lidfors, 2022), we found that 12–33% of the calf messages were transmitted via the geolocation collar from their mothers. However, 67–88% of the calf messages were also sent by other geolocation collars from the herd, indicating the contribution of other adult cows to calf detection, even in the WS. Moreover, when group size increases, there is the potential for a greater number of interactions between unrelated animals (Green, 1992).
In the ES and IS, it was possible to accurately identify the mother of each calf, and the experimental data revealed a significant difference (p < 0.05) in the total messages received between the cow–calf pairs and other cows. Particularly, in the ES scenario, the results were clear, indicating the potential of these technologies to establish associations between cows and their offspring without the need for direct observation at the time of birth, and allowing remote monitoring of calves through the mothers, as shown by Swain and Bishop-Hurley (2007) in beef cattle. The results also indicate that the calf age (in months) (R2 = 0.01; d.f = 46; p = 0.52) had no effect on the number of BLE ear tags messages sent to their mother in contrast to previous studies that found that as calf age increased, the amount of time spent with the mother decreased (Vitale et al., 1986; Hirata et al., 2003). This could be due to differences in data collection methods and age of calves used in the present study (1–6 months) compared with those in previous studies (from birth up to 4 months). Nonetheless, we show a decrease of messages sent to their mother by the calves over the time (R2 =0.24; d.f = 46; p < 0.05).
Notably, there was a significant drop in BLE ear tag messages during the night. However, these results were not observed by Maroto-Molina et al. (2019). Conversely, they found that the number of messages did not differ between night and day for cows, and the number was even higher at night for sheep. This may be because a smaller area (300 hectares) was used for the cows and the sheep were housed at night. Moreover, this phenomenon in our results could be attributed to reduced activity among adult animals during the night as seen by Kour et al. (2021) and a potential dispersion or separation of the calves from the group. The locations used in this study were natural areas without large predators. Thus, it would be valuable to compare these behaviors with areas inhabited by predators to confirm if similar patterns persist under predator presence. This comparison could further elucidate the dynamics of cow–calf behavior in varying ecological contexts.
As previously suggested, the system utility can vary significantly based on factors such as the number of geolocation collars, the number of BLE ear tags, and coverage provided by the LPWA networks. The system effectiveness is directly influenced by the fix rate, which determines the system precision and the amount of information gathered. For instance, in the WS, a calf could be undetected for a mean of 15 days. This highlights the importance of considering different parameters and configurations to optimize system performance according to specific needs and conditions. Certainly, from the perspective of animal welfare and health, prolonged intervals without monitoring calves could pose significant risks. In such circumstances, the absence of calf information increases the likelihood of adverse outcomes, such as injury, illness, or lameness, which could ultimately lead to fatalities (Petherick, 2005). Therefore, the fix rate serves as an inadequate indicator, as it fails to address the urgent need for prompt and regular assessments to ensure animal well-being. Accordingly, the technology and coverage should be improved to ensure higher fix rates and detect more BLE ear tags over time. Nevertheless, it appears plausible to demonstrate extensive management practices wherein calves graze alongside their mothers. Within such a context, it is illogical to assume that calves would be separated from their mothers for periods longer than 15 days by farm personnel owing to the difficulties involved in capturing, handling, and relocating calves further from the pastures within such a brief timeframe.
Instead, the system could validate calf traceability through blockchain technology. Based on the findings of this study, geolocation collars and BLE ear tags make verifying free-pasture access from the cow and their offspring possible. This implementation might be feasible in commercial farms considering the reasonable costs of geolocation collars (100–200€/collar) and BLE ear tags (10–20 €/tag), the long battery life of these devices, and the use of BLE ear tags in growing animals. Schleppe et al. (2010) described a lighter GPS device for use as an ear tag, but the battery only lasts a few weeks. In this study, the BLE ear tags were 25 g and had an average operational life of 280 days. We found that high fix rates are unnecessary for monitoring calves in a herd. Even in the WS, a message every few days could be more than satisfactory if the messages are constant. Using blockchain technology and BLE ear tags, we may be able to ensure comprehensive traceability of calves across the entire supply chain (Sander et al., 2018; Pearson et al., 2019; Patelli and Mandrioli, 2020). This entails establishing a system that enables real-time identification of herd locations and conditions through a cloud-based platform (Crosby et al., 2016; Casino et al., 2019), adding another level of traceability and transparency. Finally, to make this information accessible, a QR code can be developed, which can be easily integrated into product labelling reliably providing more information about the veal and beef production of various livestock farms (Zhang et al., 2021b). This enables consumers to effortlessly access and verify the authenticity and conditions of animal products, contributing to enhanced transparency and consumer confidence in the food supply chain (Zhang et al., 2021a) (Figure 8). Considering that access to natural environments is a central component of how the public conceptualizes animal welfare, daily access to pasture is a key attribute valued by beef consumers, where a portion of consumers are willing to pay more for beef derived from pasture (Conner and Oppenheim, 2008; Gwin et al., 2012; McCluskey et al., 2005). Most studies confirm that the public regards pasture-based production as natural and beneficial to animals (Yunes et al., 2017; Cardoso et al., 2018). This could be translated into an increase in profits based on a justifiable price increase, according to Violino et al. (2019). Furthermore, the increased profits can be invested into these technologies, facilitating improvements in the coverage areas and the deployment of numerous devices. Consequently, this investment would lead to a reduction in the number of days without detecting calves, or even enhance the quantity and quality of the information. This, in turn, would enable additional functionalities through the devices, thereby contributing to advancements in decision-making processes aimed at optimizing animal welfare and health.
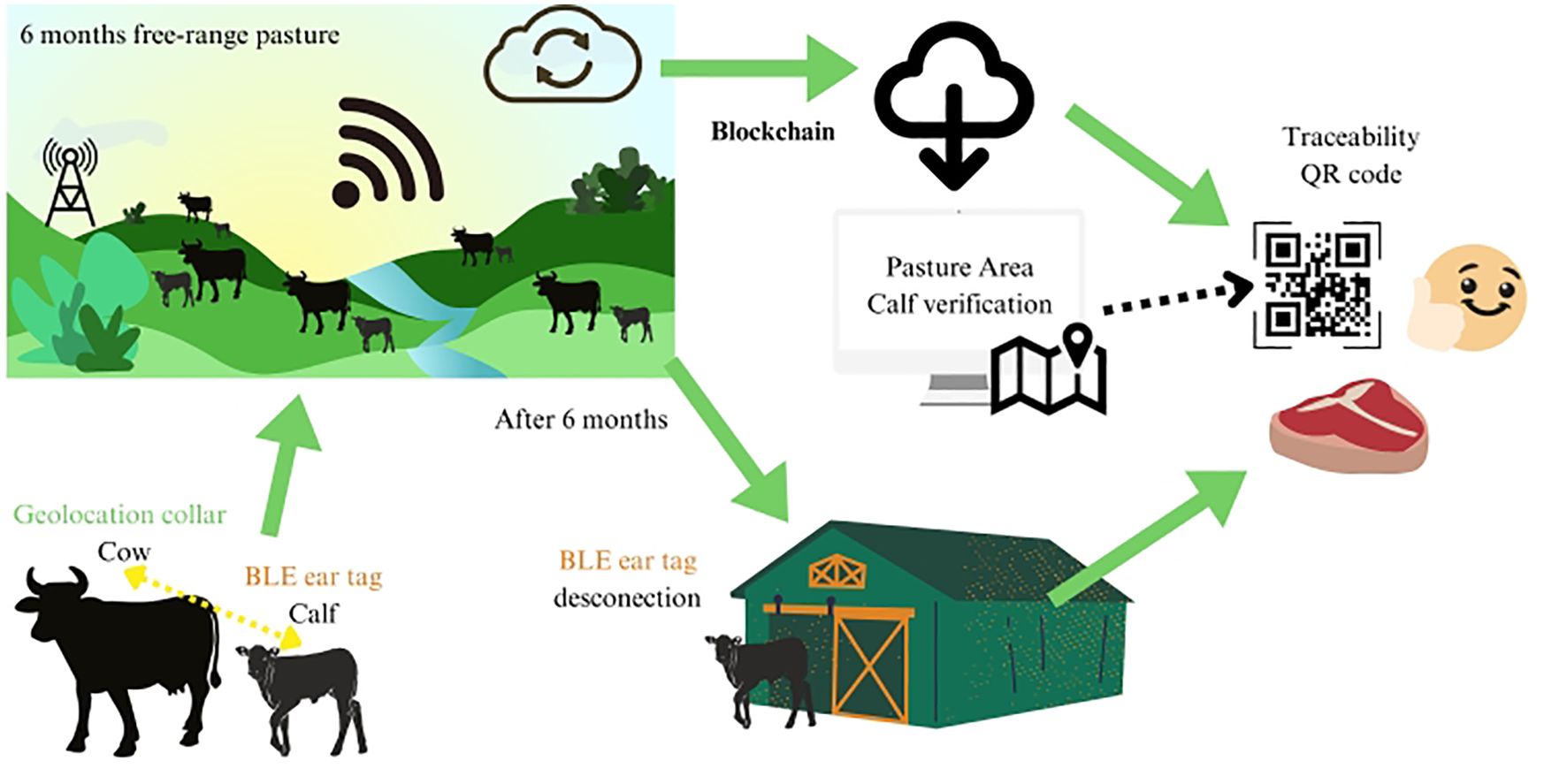
Figure 8. Diagram of possible traceability across entire supply chain using blockchain and QR code to make information accessible to consumers.
5 Conclusions
The solution proposed in this study, which integrates geolocation collars and short-range (BLE) sensor networks via LPWA (Sigfox), was found effective not only in monitoring growing animals, like calves, but also in detecting cow–calf affiliations. This valuable information, along with blockchain technology, will allow for the development of a novel traceability system to ensure complete transparency and reliability throughout the supply chain. This system can be made accessible to consumers through a QR code, offering them unprecedented insight into the pasture areas ranged by cows and their calves. However, further research is needed to explore the full potential of the system, particularly in addressing more complex challenges such as welfare and health issues affecting adult and growing animals.
Data availability statement
The original contributions presented in the study are included in the article/Supplementary Material. Further inquiries can be directed to the corresponding author.
Ethics statement
The animal study was approved by Institute of Research & Technology Food & Agriculture’s ethical committee. The study was conducted in accordance with the local legislation and institutional requirements.
Author contributions
RV-C: Conceptualization, Data curation, Formal analysis, Investigation, Validation, Visualization, Writing – original draft, Writing – review & editing. EF: Writing – original draft, Writing – review & editing, Investigation, Funding acquisition. AD: Conceptualization, Funding acquisition, Investigation, Methodology, Project administration, Resources, Supervision, Writing – original draft, Writing – review & editing.
Funding
The author(s) declare financial support was received for the research, authorship, and/or publication of this article. This project was funded by the “Ministerio de Ciencia e Innovación” of Spain, under the program for Ecological and Digital Transformation within the Spanish Plan for Transformation and Resilience and using Next Generation Funds of the EU (TED2021-129315B-C21). The first author of this paper has a PhD grant from the “Ministerio de Ciencia e Innovación”.
Acknowledgments
The authors would like to thank the farmer that participated in the system validation, and the academic editors and the reviewers for their valuable comments. We would like to thank Charrise M. Ramkissoon for English language editing.
Conflict of interest
The authors declare that the research was conducted in the absence of any commercial or financial relationships that could be construed as a potential conflict of interest.
Publisher’s note
All claims expressed in this article are solely those of the authors and do not necessarily represent those of their affiliated organizations, or those of the publisher, the editors and the reviewers. Any product that may be evaluated in this article, or claim that may be made by its manufacturer, is not guaranteed or endorsed by the publisher.
Supplementary material
The Supplementary Material for this article can be found online at: https://www.frontiersin.org/articles/10.3389/fanim.2024.1435729/full#supplementary-material
References
Azizi N., Malekzadeh H., Akhavan P., Haass O., Saremi S., Mirjalili S. (2021). IoT–blockchain: harnessing the power of internet of thing and blockchain for smart supply chain. Sensors 21, 6048. doi: 10.3390/s21186048
Bailey D. W., Trotter M. G., Knight C. W., Thomas M. G. (2018). Use of GPS tracking collars and accelerometers for rangeland livestock production research. Trans. Anim. Sci. 2, 81–88. doi: 10.1093/tas/txx006
Berckmans D. (2017). General introduction to precision livestock farming. Anim. Front. 7, 6–11. doi: 10.2527/af.2017.0102
Cardoso C. S., von Keyserlingk M. A., Hötzel M. J. (2018). Views of dairy farmers, agricultural advisors, and lay citizens on the ideal dairy farm. J. Dairy Sci. 201, 1811–1821. doi: 10.3168/jds.2018-14688
Casino F., Dasaklis T. K., Patsakis C. (2019). A systematic literature review of blockchain-based applications: Current status, classification and open issues. Telemat. Inform. 36, 55–81. doi: 10.1016/j.tele.2018.11.006
Conner D. S., Oppenheim D. (2008). Demand for pasture-raised livestock products: Results from Michigan retail surveys. J. Agribusiness 26, 1–20. doi: 10.22004/ag.econ.90550
Crosby M., Pattanayak P., Verma S., Kalyanaraman V. (2016). Blockchain technology: Beyond bitcoin. Appl. Innovation 2, 71. doi: 10.1145/2994581
Daigle C. L. (2014). Incorporating the philosophy of technology into animal welfare assessment. J. Agric. Environ. Ethics 27, 633–647. doi: 10.1007/s10806-013-9482-7
Davis J. D., Darr M. J., Xin H., Harmon J. D., Russell J. R. (2011). Development of a GPS herd activity and well-being kit (GPS HAWK) to monitor cattle behavior and the effect of sample interval on travel distance. Appl. Eng. Agric. 27, 143–150. doi: 10.36062/ijah.2022.01422
Dickinson D. L., Bailey D. (2002). Meat traceability: Are US consumers willing to pay for it? J. Agric. Resour. Econ. 7, 348–364. Available at: https://www.jstor.org/stable/40987840
Dieng O., Diop B., Thiare O., Pham C. (2017). A study on IoT solutions for preventing cattle rustling in African context. ICC 17, 1–13. doi: 10.1145/nnnnnnn.nnnnnnn
Dinno A. (2017). _dunn.test: Dunn's Test of Multiple Comparisons Using Rank Sums_. R package version 1.3.5. Available online at: https://CRAN.R-project.org/package=dunn.test.
Di Orio A. P., Callas R., Schaefer R. J. (2003). Performance of two GPS telemetry collars under different habitat conditions. Wildlife Soc. Bull. 31, 372–379. Available at: https://www.jstor.org/stable/3784315
Gordon I. J. (2001). Tracking animals with GPS: an international conference held at the Macaulay Land Use Research Institute (Aberdeen, Scotland: Macaulay Land Use Research Institute), III. doi: 10.1109/MetroAgriFor52389.2021.9628711
Gracia A., Zeballos G. (2005). Attitudes of retailers and consumers toward the EU traceability and labeling system for beef. J. Food Distrib. Res. 36, 45–56. doi: 10.22004/ag.econ.27763
Green W. C. (1992). Social influences on contact maintenance interactions of bison mothers and calves: group size and nearest-neighbour distance. Anim. Behav. 43, 775–785. doi: 10.1016/S0003-3472(05)80200-3
Gwin L., Durham C. A., Miller J. D., Colonna A. (2012). Understanding markets for grass-fed beef: taste, price, and purchase preferences. J. Food Distrib. Res. 43, 91–111. doi: 10.22004/ag.econ.145331
Hemsworth P. H., Barnett J. L., Beveridge L., Matthews L. R. (1995). The welfare of extensively managed dairy cattle: A review. Appl. Anim. Behav. Sci. 42, 161–182. doi: 10.1016/0168-1591(94)00538-P
Hirata M., Nakagawa M., Funakoshi H., Iwamoto T., Otozu W., Kiyota D., et al. (2003). Mother–young distance in Japanese Black cattle at pasture. J. Ethology 21, 161–168. doi: 10.1007/s10164-002-0092-5
Hocquette J. F., Ellies-Oury M. P., Lherm M., Pineau C., Deblitz C., Farmer L. (2018). Current situation and future prospects for beef production in Europe—A review. Asian-Australasian J. Anim. Sci. 31, 1017. doi: 10.5713/ajas.18.0196
Hofmann W. A. (2022). An evaluation of GPS technology as a tool to aid pasture management. Hamilton, New Zealand: The University of Waikato.
Kassambara A. (2023). R, package version 0.6.0 (_ggpubr: 'ggplot2' Based Publication Ready Plots_). Available at: https://CRAN.R-project.org/package=ggpubr.
Kour H., Patison K. P., Corbet N. J., Swain D. L. (2021). Recording cattle maternal behaviour using proximity loggers and tri-axial accelerometers. Appl. Anim. Behav. Sci. 240, 105349. doi: 10.1016/j.applanim.2021.105349
Lidfors L. (2022). “Parental behavior in bovines,” in Patterns of Parental Behavior: From Animal Science to Comparative Ethology and Neuroscience (Springer International Publishing, Cham), 177–212.
Manning J., Power D., Cosby A. (2021). Legal complexities of animal welfare in Australia: Do on-animal sensors offer a future option? Animals 11, 91. doi: 10.3390/ani11010091
Maroto-Molina F., Navarro-García J., Príncipe-Aguirre K., Gómez-Maqueda I., Guerrero-Ginel J. E., Garrido-Varo A., et al. (2019). A low-cost IoT-based system to monitor the location of a whole herd. Sensors 19, 2298. doi: 10.3390/s19102298
McCluskey J. J., Grimsrud K. M., Ouchi H., Wahl T. I. (2005). Bovine spongiform encephalopathy in Japan: consumers' food safety perceptions and willingness to pay for tested beef. Aust. J. Agric. Res. Econ. 49, 197–209. doi: 10.1111/j.1467-8489.2005.00282.x
McLean I., Holmes P. (2015). Improving the performance of northern beef enterprises. In: Key findings for producers from the northern beef report (Meat and Livestock Australia). Available online at: https://futurebeef.com.au/wp-content/uploads/Improving-the-performance-of-northern-beef-enterprises.pdf (Accessed January 11, 2024).
Mekki K., Bajic E., Chaxel F., Meyer F. (2019). A comparative study of LPWAN technologies for large-scale IoT deployment. ICT Express 5, 1–7. doi: 10.1016/j.icte.2017.12.005
Menon S., Jain K. (2021). Blockchain technology for transparency in agri-food supply chain: Use cases, limitations, and future directions. IEEE Trans. Eng. Manage. 71, 106–120. doi: 10.1109/TEM.2021.3110903
METEOCAT. (2003). Servei Meteorològic de Catalunya (Catalan weather forecast service). Available online at: https://www.meteo.cat/ (Accessed February 4, 2024).
Nóbrega L., Tavares A., Cardoso A., Gonçalves P. (2018). “Animal monitoring based on IoT technologies,” in United States: 2018 IoT Vertical and Topical Summit on Agriculture-Tuscany (IOT Tuscany). 1–5. doi: 10.1109/IOT-TUSCANY.2018.8373045
Patelli N., Mandrioli M. (2020). Blockchain technology and traceability in the agrifood industry. J. Food Sci. 85, 3670–3678. doi: 10.1111/1750-3841.15477
Pearson S., May D., Leontidis G., Swainson M., Brewer S., Bidaut L., et al. (2019). Are distributed ledger technologies the panacea for food traceability? Global Food Secur. 20, 145–149. doi: 10.1016/j.gfs.2019.02.002
Petherick J. C. (2005). Animal welfare issues associated with extensive livestock production: The northern Australian beef cattle industry. Appl. Anim. Behav. Sci. 92, 211–234. doi: 10.1016/j.applanim.2005.05.009
Polojärvi K., Colpaert A., Matengu K., Kumpula J. (2010). “GPS collars in studies of cattle movement: Cases of northeast Namibia and north Finland,” in Engineering Earth: The Impacts of Megaengineering Projects (Springer Netherlands, Dordrecht), 173–187.
Raza U., Kulkarni P., Sooriyabandara M. (2017). Low power wide area networks: An overview. IEEE Commun. surveys tutorials 19, 855–873. doi: 10.1109/COMST.2017.2652320
R Core Team. (2014). R: A language and environment for statistical computing (Vienna, Austria: R Foundation for Statistical Computing; R Core Team).
Rempel R. S., Rodgers A. R., Abraham K. F. (1995). Performance of a GPS animal location system under boreal forest canopy (543-551: The Journal of wildlife management). doi: 10.13031/ja.14518)@2022
Sander F., Semeijn J., Mahr D. (2018). The acceptance of blockchain technology in meat traceability and transparency. Br. Food J. 120, 2066–2079. doi: 10.1108/BFJ-07-2017-0365
Schleppe J. B., Lachapelle G., Booker C. W., Pittman T. (2010). Challenges in the design of a GNSS ear tag for feedlot cattle. Comput. Electron. Agric. 70, 84–95. doi: 10.1016/j.compag.2009.09.001
Senneke S. L., MacNeil M. D., Van Vleck L. D. (2004). Effects of sire misidentification on estimates of genetic parameters for birth and weaning weights in Hereford cattle. J. Anim. Sci. 82, 2307–2312. doi: 10.2527/2004.8282307x
Swain D. L., Bishop-Hurley G. J. (2007). Using contact logging devices to explore animal affiliations: quantifying cow–calf interactions. Appl. Anim. Behav. Sci. 102, 1–11. doi: 10.1016/j.applanim.2006.03.008
Trotter M. (2018). Final report - Demonstrating the value of animal location and behaviour data in the red meat value, ACIL Allen Consulting. Australia. Available online at: https://coilink.org/20.500.12592/rsvb8p (Accessed January 27, 2024)
Ulloa-Vásquez F., Carrizo D., García-Santander L. (2022). Comparative study of the cost of implementing wireless technologies for IoT and M2M for the last mile: A case study. Ingeniare: Rev. Chil. Ingenieria 30, 422–428. doi: 10.4067/S0718-33052022000300422
Veissier I., Boissy A., Nowak R., Orgeur P., Poindron P. (1998). Ontogeny of social awareness in domestic herbivores. Appl. Anim. Behav. Sci. 57, 233–245. doi: 10.1016/S0168-1591(98)00099-9
Veissier I., Lamy D., Le Neindre P. (1990). Social behaviour in domestic beef cattle when yearling calves are left with the cows for the next calving. Appl. Anim. Behav. Sci. 27, 193–200. doi: 10.1016/0168-1591(90)90056-J
Violino S., Pallottino F., Sperandio G., Figorilli S., Antonucci F., Ioannoni V., et al. (2019). Are the innovative electronic labels for extra virgin olive oil sustainable, traceable, and accepted by consumers? Foods 8, 529. doi: 10.3390/foods8110529
Vitale A. F., Tenucci M., Papini M., Lovari S. (1986). Social behaviour of the calves of semi-wild Maremma cattle, Bos primigenius taurus. Appl. Anim. Behav. Sci. 16, 217–231. doi: 10.1016/0168-1591(86)90115-2
Yunes M. C., von Keyserlingk M. A. G., Hötzel M. J. (2017). Brazilian citizens’ opinions and attitudes about farm animal production systems. Animals 7, 75. doi: 10.3390/ani7100075
Zhang S., Liao J., Wu S., Zhong J., Xue X. (2021b). A traceability public service cloud platform incorporating IDcode system and colorful QR code technology for important product. Math. Problems Eng. 2021, 1–15. doi: 10.1155/2021/5535535
Zhang M., Wang X., Feng H., Huang Q., Xiao X., Zhang X. (2021a). Wearable Internet of Things enabled precision livestock farming in smart farms: A review of technical solutions for precise perception, biocompatibility, and sustainability monitoring. J. Cleaner Production 312, 127712. doi: 10.1016/j.jclepro.2021.127712
Keywords: precision livestock farming, geolocation collars, bluetooth low energy, traceability, cow–calf contact, animal welfare, extensive farming
Citation: Vidal-Cardos R, Fàbrega E and Dalmau A (2024) Determining calf traceability and cow–calf relationships in extensive farming using geolocation collars and BLE ear tags. Front. Anim. Sci. 5:1435729. doi: 10.3389/fanim.2024.1435729
Received: 20 May 2024; Accepted: 12 August 2024;
Published: 03 September 2024.
Edited by:
Joao R. R. Dorea, University of Wisconsin-Madison, United StatesReviewed by:
Severiano Silva, Universidade de Trás-os-Montes e Alto, PortugalEnrico Casella, The Pennsylvania State University (PSU), United States
Rafael Ehrich Pontes Ferreira, University of Wisconsin-Madison, United States
Copyright © 2024 Vidal-Cardos, Fàbrega and Dalmau. This is an open-access article distributed under the terms of the Creative Commons Attribution License (CC BY). The use, distribution or reproduction in other forums is permitted, provided the original author(s) and the copyright owner(s) are credited and that the original publication in this journal is cited, in accordance with accepted academic practice. No use, distribution or reproduction is permitted which does not comply with these terms.
*Correspondence: Antoni Dalmau, YW50b25pLmRhbG1hdUBpcnRhLmNhdA==