- 1Veterinary Veterinary Medicine Teaching and Research Center, School of Veterinary Medicine, University of California, Davis, Tulare, CA, United States
- 2Department of Population Health and Reproduction, School of Veterinary Medicine, University of California, Davis, Davis, CA, United States
Introduction: The current global concern over increasing antimicrobial resistance among animal and human pathogens has motivated efforts to reduce antimicrobial drug use in food animals and its impact on antimicrobial resistance. One such strategy is to use selective dry cow therapy (SDCT) in dairy cows, which involves treating only cows with intramammary infection (IMI) at dry-off. However, efficient methods are needed to identify cows with IMI at dry-off to implement SDCT. Automatic Milking Systems (AMS) data may help farmers identify cows with IMI when individual Somatic Cell Count (SCC) is not routinely tested. This study assessed the correlation between cow-level and quarter-level AMS parameters and IMI at dry-off.
Methods & Results: A total of 733 udder quarters (comprising both Primiparous [PRIM] and Multiparous [MULT] cows) were sampled and categorized for IMI based on bacterial growth and SCC. Data were aggregated both daily and into 7-day and 15-day intervals preceding dry-off. The quarter-level prevalence of bacterial growth at dry-off was 24.28% overall. When stratified by parity, logistic regression analysis at 15 days to dry-off revealed that the average difference in mastitis detection index (MDi) in PRIM, MDi, and standard deviation milk flow rate in MULT were associated with increased odds of IMI at dry-off. Similarly, data from 7 days to dry-off revealed that average peak milk flow rate in PRIM, and MDi in MULT were associated with increased odds of IMI at dry-off. However, an increase in average milk yield was associated with decreased odds of IMI.
Discussion & Conclusion: Our findings underscore the significance of MDi, milk flow rate, peak milk flow rate, and milk yield in predicting IMI at dry-off. Notably, stronger associations were observed with data collected 7 days preceding dry-off. Further research is warranted to refine and validate algorithms amalgamating these variables for precise IMI prediction in cows at dry-off.
Introduction
Bovine mastitis is an inflammation of the mammary gland, typically caused by bacterial infection through the teat canal (Bradley, 2002; Pyörälä, 2003). Mastitis is a painful condition that not only affects animal welfare but also causes substantial economic losses to dairies. These losses are primarily due to reduced milk yield, the cost of discarded milk, and other expenses related to diagnosis, treatment, culling, and labor (Cha et al., 2011; Khatun et al., 2017). On average, a single case of mastitis on United States (US) dairies cost between $110 to $440 (Liang et al., 2017; Rollin et al., 2015). The predominant bacterial pathogens causing mastitis are from the Enterobacteriaceae, Staphylococcaceae, or Streptococcaceae families. Mastitis is categorized into two types based on its symptoms: subclinical and clinical mastitis (Forsbäck et al., 2009). Subclinical mastitis is the more common form of the condition, affecting 20 - 50% of cows in a herd. It is characterized by an increase in somatic cell count (SCC) with no obvious clinical signs. Clinical mastitis, on the other hand, is characterized by visible changes in the milk or udder (Gonçalves et al., 2018).
Dry cow therapy (DCT) is an essential aspect of many dairy herds’ mastitis control program. This practice involves the intramammary infusion of long-acting antimicrobials into the teats of dairy cows at the end of lactation. The goal of DCT is to cure existing infections and prevent new ones during the dry period and subsequent lactation. Typically, intramammary antimicrobials are administered to all four udder quarters of every cow at dry-off, also referred to as blanket dry cow therapy (BDCT) (Higgins et al., 2017). In the US, BDCT has been adopted as a management practice by 80% of dairy herds (NAHMS, 2014) and is associated with high IMI cure rates of up to 87.5% in the dry period (Rowe et al., 2020). However, the global concern over increasing antimicrobial resistance in animal and human pathogens has motivated efforts to reduce antimicrobial drug use in food animals and its impact on antimicrobial resistance (Garcia et al., 2019). One such strategy is selective dry cow therapy (SDCT) which involves treating only cows with IMI at dry-off. However, efficient methods are needed to identify cows with IMI at dry-off for the implementation of SDCT (Cameron et al., 2015; McParland et al., 2019). Detection methods for IMI at dry-off include bacterial culture (Bradley et al., 2015; Patel et al., 2017), increase in somatic cell count (SCC) (Lipkens et al., 2019), California Mastitis Test (CMT; (Godden et al., 2017), and culture free algorithms that use individual cow and farm data, among others.
Culture-free algorithms require data at both cow and herd-level, such as records from Automatic Milking Systems (AMS). AMS involves the use of robots to milk cows multiple times a day without human interference (Kamphuis et al., 2008). This technology is gaining popularity in the dairy industry of many countries (De Koning, 2010) due to its ability to reduce labor costs, increase the number of times a cow is milked over a lactation period and improve animal welfare (Hovinen and Pyörälä, 2011). The AMS collects data on milk properties such as electrical conductivity, presence of red blood cells in the milk, as well as milk flow and cow behavior including time between milkings, feed consumption. This data is crucial for guiding both cow and milking management practices. Delaval® AMS uses information on electrical conductivity, blood, and milk interval, to generate the Mastitis Detection Index (MDi), which predicts IMI at the cow level (Khatun et al., 2018). Dairy operators may rely on the MDi alerts to identify IMI suspect cows since cows are not individually checked during milking (Bonestroo et al., 2022; Khatun et al., 2018).
Several studies have reported on using AMS alerts to detect IMI during lactation (Bonestroo et al., 2022; Inzaghi et al., 2021; Jensen et al., 2016). However, we did not find any research investigating the use of AMS data to detect cows with IMI at dry-off. In this cross-sectional study, we aimed to examine the relationship between cow-level and quarter-level data at the end of lactation and the presence of IMI at dry-off. We hypothesized that AMS data may help identify cows at risk of IMI at dry-off, thus guiding the implementation of SDCT on the herds. This information would help dairy operations that use AMS to implement SDCT and improve the health of their cows.
Materials and methods
Study population
The study enrolled two commercial dairy herds (A and B) that use Automated Milking Systems (AMS) with the voluntary consent of their owners. Both herds were located in the San Joaquin Valley of California and raised Holstein-Friesian cows in free-stall barns. Farm A had 984 lactating cows, out of which 818 were milked using 14 AMS. Farm B had 5,342 lactating cows, and 787 of them were milked using 13 AMS. All the cows enrolled on both farm A were milked using AMS throughout the lactation. On both farms, a maximum of 62 cows were assigned to a single automatic milking machine. The average daily milking frequency was 2.0 milking/cow/day on Farm A and 2.2 milking/cow/day on Farm B.
Cows on both farms were given commercial pelleted concentrate feed during milking, with the amount determined by the cow’s lactation stage. To keep the cows cool, the AMS pens were equipped with heat abatement systems such as fans and soakers placed over the beds and feed bunks. Both farms used a combination of dry manure and almond shells as bedding, but the ratio of dry manure to almond shells was 1:1 for Farm A and 3:1 for Farm B.
The cows were separated into primiparous (PRIM) and multiparous (MULT) pens by parity. Both farms utilized the AMS alerts to identify cows with intramammary infections (IMI) during lactation. On Farm A, mastitis was identified using a criterion of a 3-day average MDi of above 1.8, coupled with milk diversion and electrical conductivity (EC) above 125 mS/cm. On the other hand, Farm B identified cows with intramammary infections (IMI) by noting an increase in EC above 0.5 mS/cm following an AMS alert and a decrease in milk yield per quarter. The two farms both conducted on-farm cultures, where Farm A treated only quarters with Gram-positive bacteria, while Farm B treated all quarters, both Gram-positive and Gram-negative bacteria.
Enrollment
All the cows in the AMS pens that were eligible for enrollment had four functional quarters, regardless of their parity. Any cows that exhibited obvious clinical illnesses at dry-off were excluded from the study. The enrolled cows were dried off between August 23 and December 27, 2021. The study included a total of 218 cows, with 105 in the PRIM group and 113 in the MULT group. A total of 733 quarter milk samples were collected, with 389 samples from primiparous cows and 344 from multiparous cows. A maximum of 4 and minimum of 3 quarter milk samples were collected per cow, averaging 3.36 quarters samples per cow.
Sample collection
Trained study personnel collected aseptic quarter milk samples following the guidelines of the National Mastitis Council (NMC, 2017). Whenever necessary, farm personnel assisted with sampling. The personnel wore disposable gloves throughout the process, which were changed between cows. First, the teats were wiped with a clean hand towel to remove gross contamination, and then dipped in a Povidone Iodine solution. A contact time of at least 30 seconds was allowed before wiping the teat ends with gauze soaked in 70% ethanol. The initial 1-3 squirts of milk from each teat were stripped onto the ground. Then, each quarter milk sample was collected in a sterile 2-ounce plastic vial held at a 45° angle. Sampling of quarters was done in chronological order, starting from Right Rear, Left Rear, Right Front, and Left Front. During the sampling process, the udder hygiene scores (UHS) were recorded using a 3-point scale. UHS-1 was given for a clean udder, free or nearly free of dirt, manure, or stains. UHS-2 was given for a slightly dirty udder with flecks of dirt, manure, or stains, while UHS-3 was given for an udder largely covered with dirt and manure. All the collected samples were transported in cold boxes with ice packs to Sequoia Veterinary Services milk quality laboratory (Tulare CA) within 4 hours of sampling for SCC, culture, and organism speciation by MALDI-TOF. Data from both dairies and enrolled cows were de-identified and stored in Microsoft Excel® 2019. Herd management data was obtained from Dairycomp305 and DeLaval DelPro software 5.6 (DeLaval International AB).
Bacterial culture and species identification
Bacteriological culture and bacterial organism speciation were conducted in accordance with the guidelines set by the National Mastitis Council (NMC, 2017). The milk vials were inverted several times to ensure proper mixing. Each sample was streaked onto the entire bovine blood agar plate using a sterile cotton-tipped applicator. The cultured plates were kept in an incubator at 37°C, and a visual examination of the colony growth (morphology, and hemolysis) was done after 24 and 48 hours. The Matrix-Assisted Laser Desorption/Ionization Time of Flight (MALDI/MALDI-TOF) extended direct transfer method was used for bacterial species identification of each colony type. If the species were too similar for speciation MALDI/MALDI-TOF, biochemical analysis (catalase reaction and gram stain) was performed.
Somatic cell count
Somatic cell counts were determined using a Fossomatic™ FC analyzer (FOSS North America, Eden Prairie, MN) on the day of sample collection. The fossomatic method is based on somatic cell DNA recognition. Each milk sample was mixed with a staining solution and the resultant mixture was placed in a flow cell where stained somatic cells were exposed to light of a specific wavelength. The cells then emit fluorescent light pulses of a different wavelength that are counted and displayed (Schwarz et al., 2020).
Definition of intramammary infection
Quarter-level intramammary infection (IMI) at dry-off was determined based on culture-positive results and an SCC ≥ 100,000 cells/ml for primiparous cows and or SCC ≥ 200,000 cells/ml for multiparous cows (Godden et al., 2016). A positive culture result was defined as ≥1 colony for all pathogens except for coagulase-negative Staphylococcus (CNS) (positive if ≥ 2 colonies) (Dohoo et al., 2011) and Bacillus species (positive if ≥ 5 colonies) (Arruda et al., 2013). This adjustment was performed to increase specificity thus reducing the occurrence of biased measures of association (Haine et al., 2018).
Data collection and management
Over the course of 15 days prior to dry-off, we collected daily reports generated by DeLaval® AMS systems. These reports contained quarter-level information on each cow for every milking. Additionally, we downloaded and stored weekly backups from DairyComp 305 during the study period. The backups contained cow-level data. Altogether, our final dataset consisted of 936,754 data points from a total of 218 cows, including 105 primiparous (PRIM) cows and 113 multiparous (MULT) cows.
A description of the variables and how they were aggregated is summarized in Table 1. The cow-level variables included parity (PRIM and MULT), number of mastitis cases in current lactation (NMAST), last total milk yield before dry-off (LAST_MY_TOTAL, pounds), days in milk at dry-off date (DIM_DO), mastitis detection index (MDi), and the udder hygiene score (UHS). Quarter-level variables were bacterial culture (BACT_CULT), somatic cell count (SCC, cells/mL), electrical conductivity (EC, mS/cm), milk yield (QT_MY, pounds), milk flow rate (MILK_FLOW, lbs/min), peak milk flow rate (PEAK_MILK_FLOW, lbs/min), milking duration (MILK_DUR, min), and presence of blood in milk (BLOOD, a numerical variable, ppm). When needed, these variables were aggregated by day (when more than one milking was recorded for the same cow), as described in Table 1. A daily sum was calculated for the variables quarter milk yield and blood (DAILY_QT_MY and DAILY_BLOOD, respectively). The averages within a day were calculated for the variables EC, MILK_FLOW, PEAK_MILK_FLOW, and MILK_DUR. Additionally, the standard deviation was calculated for the same variables above for the days with more than one milking per cow. Besides daily minimum (MIN_MDi), maximum (MAX_MDi), and the difference between minimum and maximum (DIF_MDi) were calculated for the MDi variable.
Finally, cow-level and quarter-level continuous variables were categorized according to the DeLaval® AMS. An AMS alert was triggered if MDi > 1.4, and no alert was triggered if MDi < 1.3. The DAILY_BLOOD was categorized as negative if no blood was detected or positive blood if DAILY_BLOOD > 0. Somatic cell count was categorized into three groups: Group 1 (SCC ≤ 100,000 cells/mL), Group 2 (100,000 - 200,000 cells/mL), and Group 3 (SCC > 200,000 cells/mL). The number of mastitis cases in current lactation (NMAST) was categorized as 0 if NMAST = 0, 1 if NMAST = 1, and 2 if NMAST ≥ 2.
Datasets for the association between cow-level and quarter-level data and intramammary infection at dry-off
Two datasets (datasets 1 and 2) were generated based on data aggregation as described in the previous section and the time point before dry-off (Figure 1). Dataset 1 contained daily aggregated milking information for each enrolled cow for the last 15 days before dry-off. Dataset 1 included the daily averages and daily standard deviations of the following variables: EC, MILK_FLOW, PEAK_MILK_FLOW, MILK_DUR, DAILY_QT_MY, DAILY_BLOOD, MIN_MDi, MAX_MDi, and DIF_MDi. Additionally, the maximum number of days blood was in milk (MAX_BLOOD_15) and the total number of days blood was in milk (NDAYS_BLOOD_15) during the 15 days was calculated. Furthermore, the total number of days a quarter received an MDi alert (N_MDi_ALERT_15) during the 15 days were calculated. In dataset 2, the variables in dataset 1 were considered but for only the last 7 days before dry-off. However, because daily standard deviations could not be calculated, only information on the daily variation for this period (average and standard deviations of the daily standard deviations) was calculated.
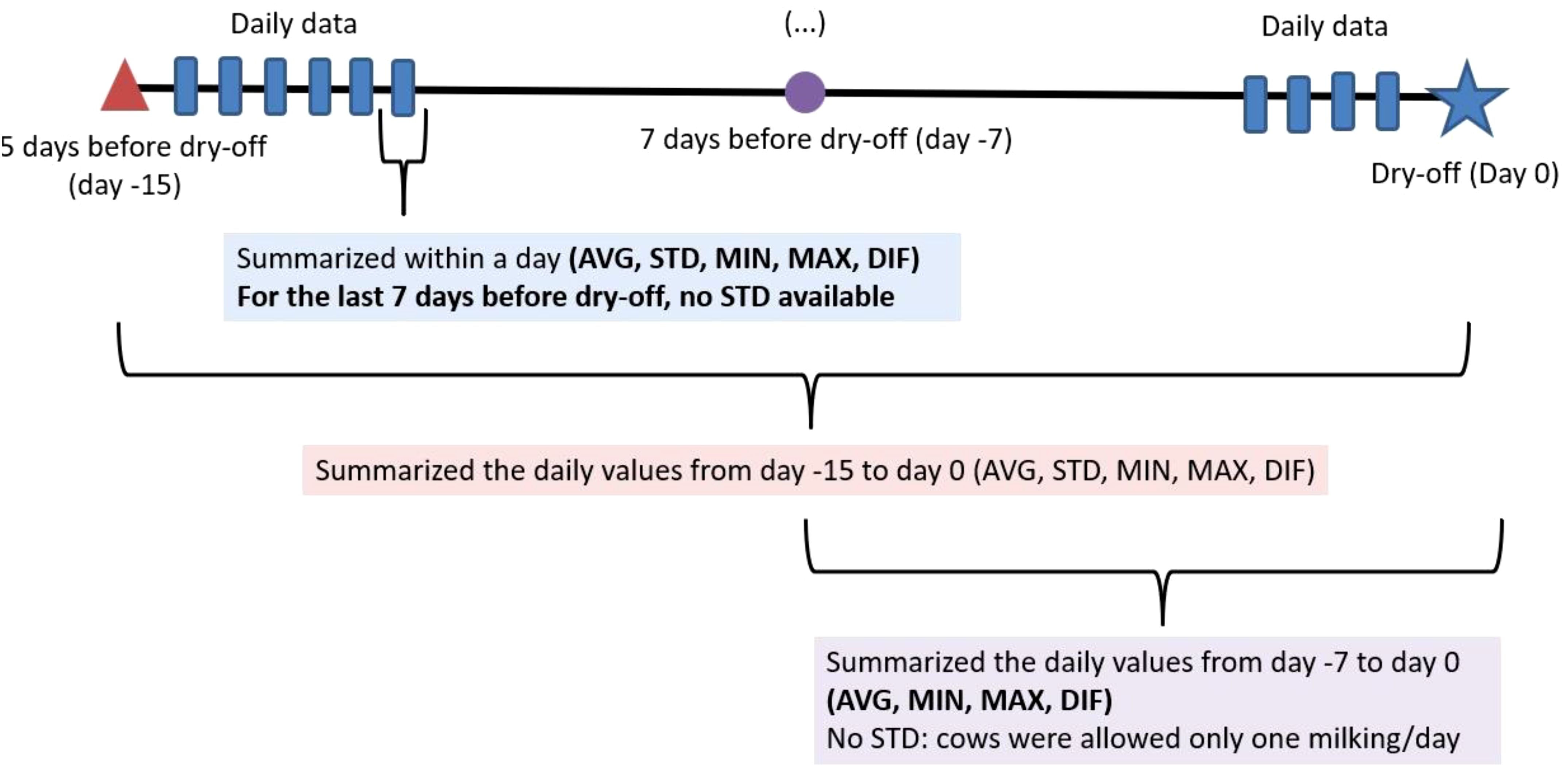
Figure 1. Data management and summarization. The data were first summarized within a day. Later the data for the 15 days and 7 days before dry-off were independently summarized. AVG, Average; STD, Standard deviation; DIF, the difference between minimum and maximum.
Statistical analysis
All analyses were stratified by parity due to known differences in risks for mastitis in primiparous and multiparous cows (Compton et al., 2007). Descriptive analysis of the cow-level and quarter-level variables was performed in both datasets 1 and 2 (stratified by parity, PROC FREQ, and MEANS, SAS 9.4). Following the descriptive analysis of the data, the dependent variable IMI was determined as previously defined (culture-positive results and an SCC ≥ 100,000 cells/ml for primiparous cows and or SCC ≥ 200,000 cells/ml for multiparous cows). Mixed univariate analyses were performed for all the cow-level and quarter-level variables using PROC GLIMMIX on SAS 9.4. Explanatory variables with P < 0.20 were included in the multivariate analyses (Bursac et al., 2008). The farm was included in all models due to known differences in management practices and the associated effect on the prevalence of mastitis. The farm was considered a fixed effect. The udder quarter was considered the experimental unit. The random effects were the cow and quarter within cows. Eligible predictors from the univariable models were offered to a multivariable mixed model. The forward stepwise selection method and the Akaike Information Criteria (AIC) were used for model selection with the method Laplace. If exclusion of a variable from the model resulted in >15% change in the estimate of association with IMI, the variable was considered as a confounder and retained in the model. The final model was run using PROC GLIMMIX (SAS 9.4) with the default Quasi-Newton method and final significance was declared at P <0.05.
Results
Description of cow-level and quarter-level variables
A total of 105 primiparous and 113 multiparous cows were enrolled at dry-off. Table 1 shows the distribution of cow-level and quarter-level variables during the last 15 days before dry-off for primiparous (PRIM) and multiparous (MULT) cows, respectively.
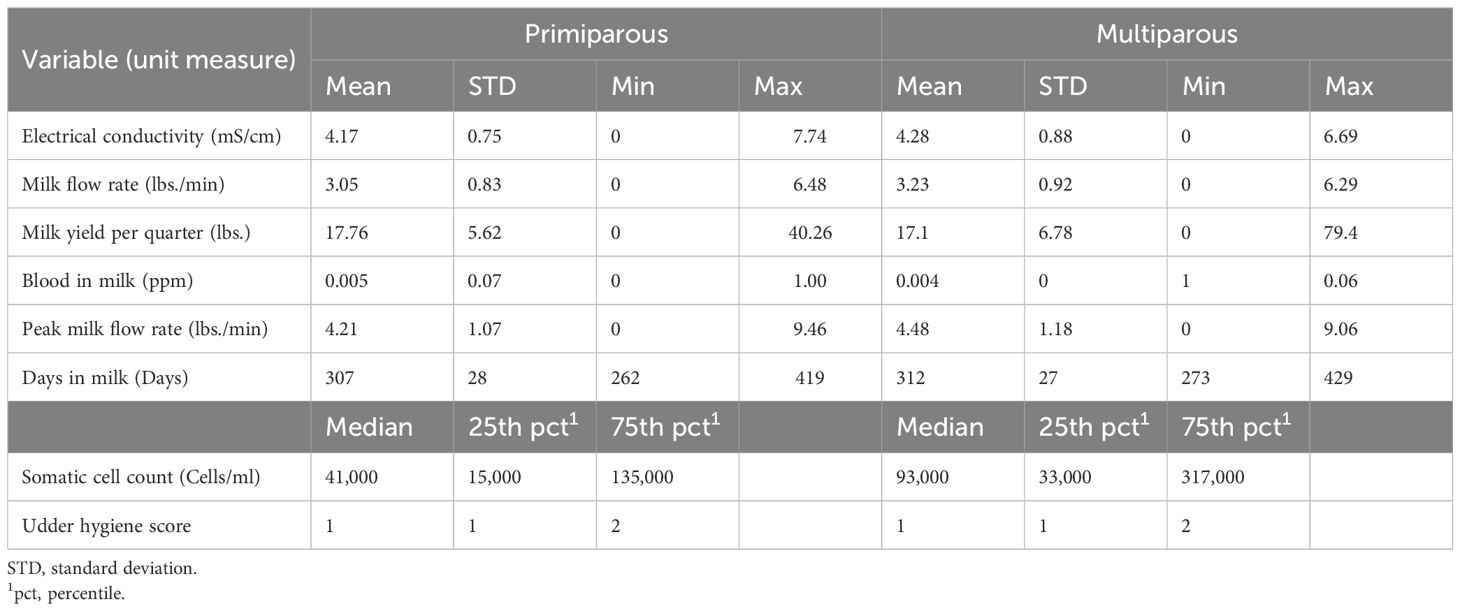
Table 1. Distribution of cow level and quarter level variables of primiparous (n = 105 cows) and multiparous (n = 113 cows) for the 15 days before dry-off.
The median udder hygiene scores of the PRIM and MULT cows at dry-off were 1 and 2, respectively. The mean number of DIM at dry-off was 307 ± 28 days and 312 ± 27 days for PRIM and MULT cows, respectively. The median SCC at the quarter level for PRIM and MULT cows were 41,000 cells/ml (15,000 - 135,000) and 93,000 cells/mL (33,000 - 317,000) respectively (Table 1).
The mean EC, MILK_FLOW, PEAK_MILK_FLOW for the PRIM cows for the last 15 days was 4.17 ± 0.75 mS/cm, 3.05 ± 0.83 lbs/min, 4.21 ± 1.07 lbs/min, respectively. For the MULT cows, mean EC, MILK_FLOW, and PEAK_MILK_FLOW was 4.28 ± 0.88 mS/cm, 3.23 ± 0.92 lbs./min, and 4.48 ± 1.18 lbs/min, respectively. The mean QT_MY was 17.76 ± 5.62 lbs for PRIM and 17.1 ± 6.78 lbs for MULT.
Milk flow parameters
Figures 2–5 show the pattern of daily quarter-level average milk flow rate (AVG_MILK_FLOW), average peak milk flow rate (AVG_PEAK_MILK_FLOW), the sum of milk yield per quarter (DAILY_QT_MY), and the difference between the daily maximum and MDi (DIF_MDi), respectively. Whereas the data were collected 15 days to dry-off, the majority of cow level and quarter level parameters were not statistically significant at 15 days to dry-off (Table 2), thus subsequent downstream analysis only focused on the last 7 days to dry-off. The AVG_MILK_FLOW for PRIM during the last 7-days before dry-off was 3.29 lbs/min in healthy quarters and 2.81 lbs/min in quarters with intramammary infection, while in MULT cows, the respective values were 3.17 and 3.06 lbs/min. The AVG_PEAK_MILK_FLOW during the last 7-days before dry-off for the healthy quarters and quarters with IMI infected were 4.42 and 3.97 lbs/min in PRIM and 4.41 and 3.56 lbs/min in MULT, respectively. For the 7 days prior to drying off, the average DAILY_QT_MY for healthy quarters and those with IMI for PRIM were 15.99 and 14.32 lbs, respectively, while for MULT these values were 17.12 and 10.06 lbs. Lastly, the average DIF_MDi during 7 days before dry-off for healthy and quarters with intramammary infection were 0.06 and 0.03 for PRIM and 0.11 and 0.13 for MULT.
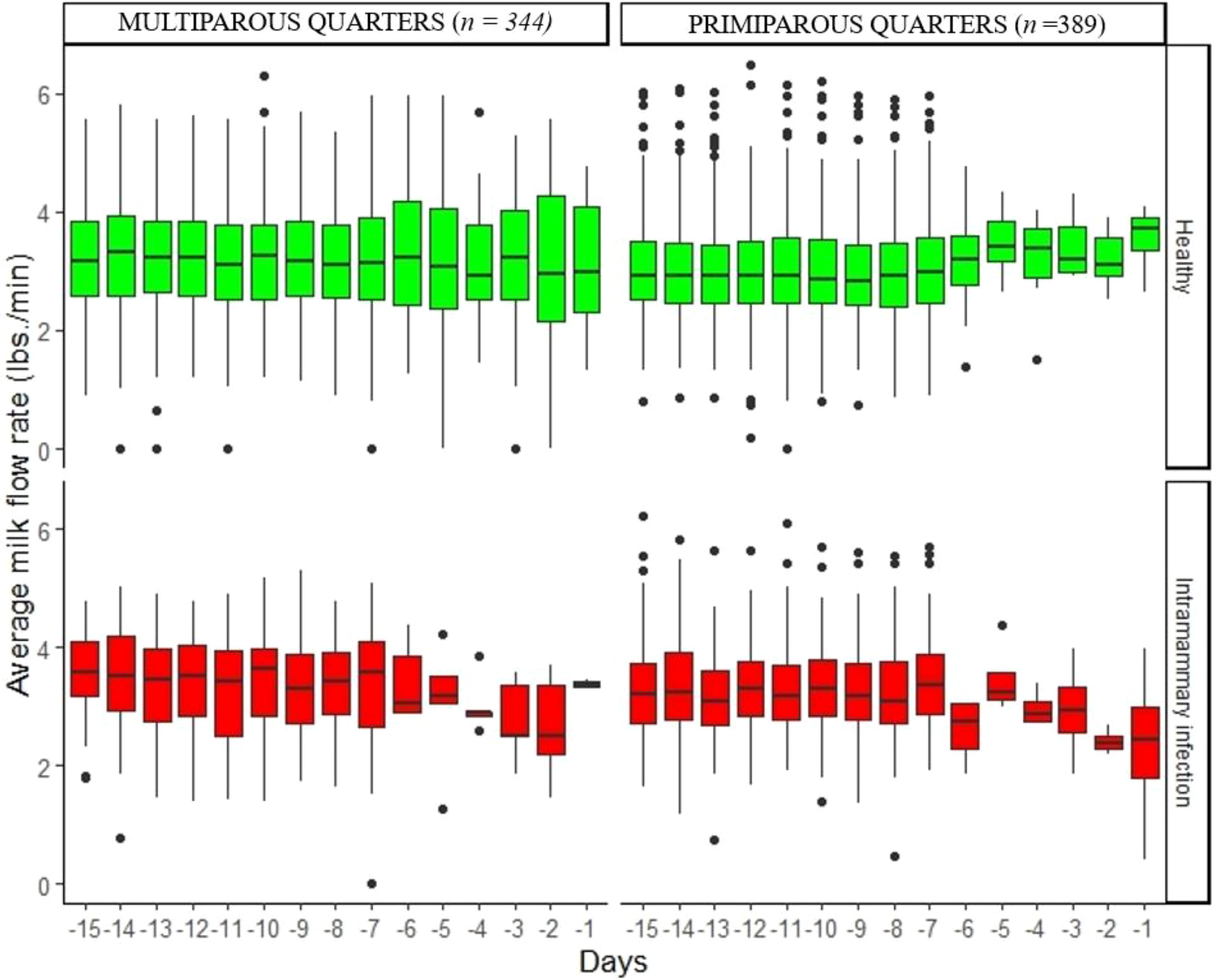
Figure 2. Variation of daily average milk flow rate in healthy quarters and those with intramammary infection during the 15 days before dry-off.
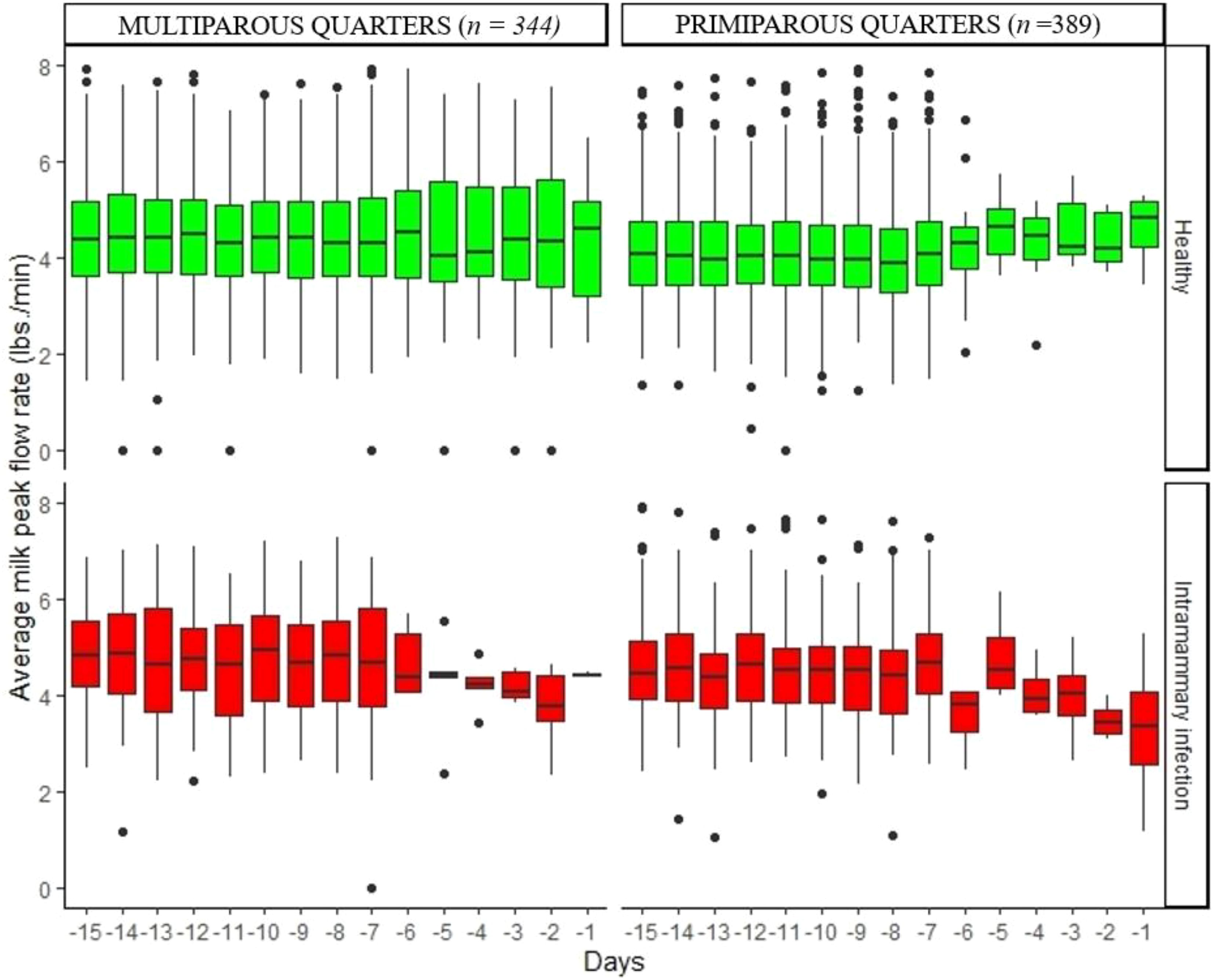
Figure 3. Variation of daily average peak milk flow rate in healthy quarters and those with intramammary infection during the 15 days before dry-off.
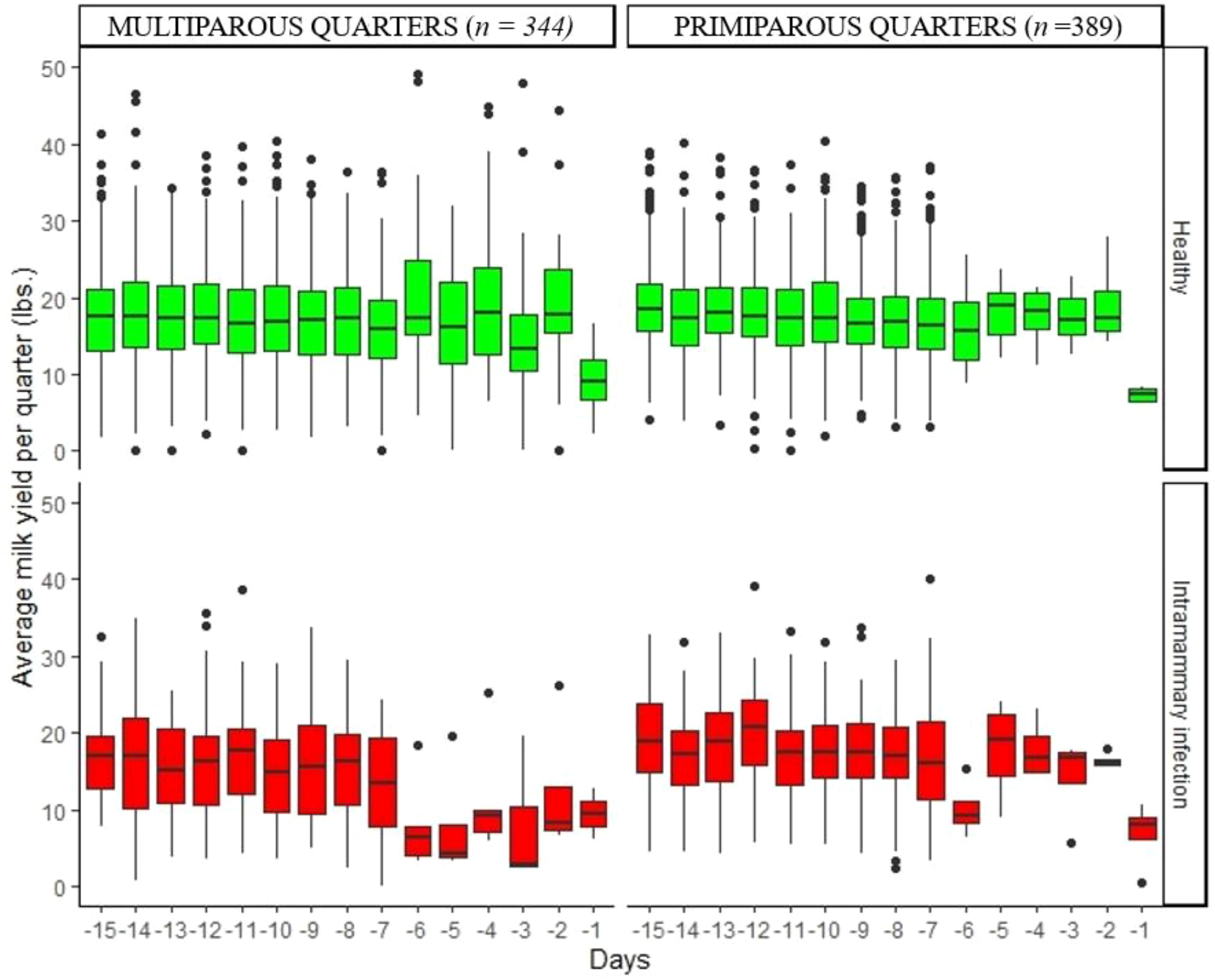
Figure 4. Variation of daily average milk yield per quarter in healthy quarters and those with intramammary infection during the 15 days before dry-off.
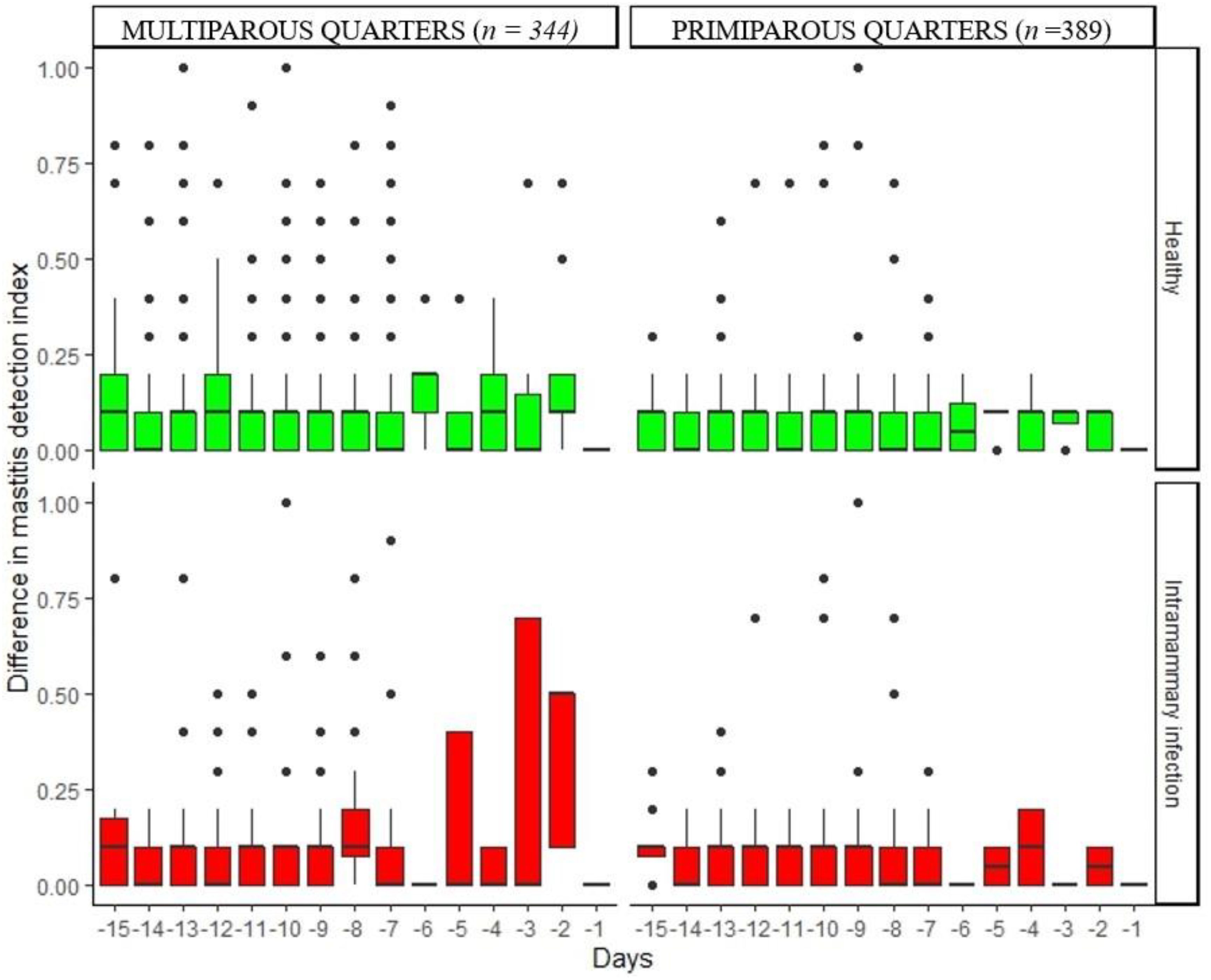
Figure 5. Variation of daily difference in mastitis detection index in healthy quarters and those with intramammary infection during the 15 days before dry-off.
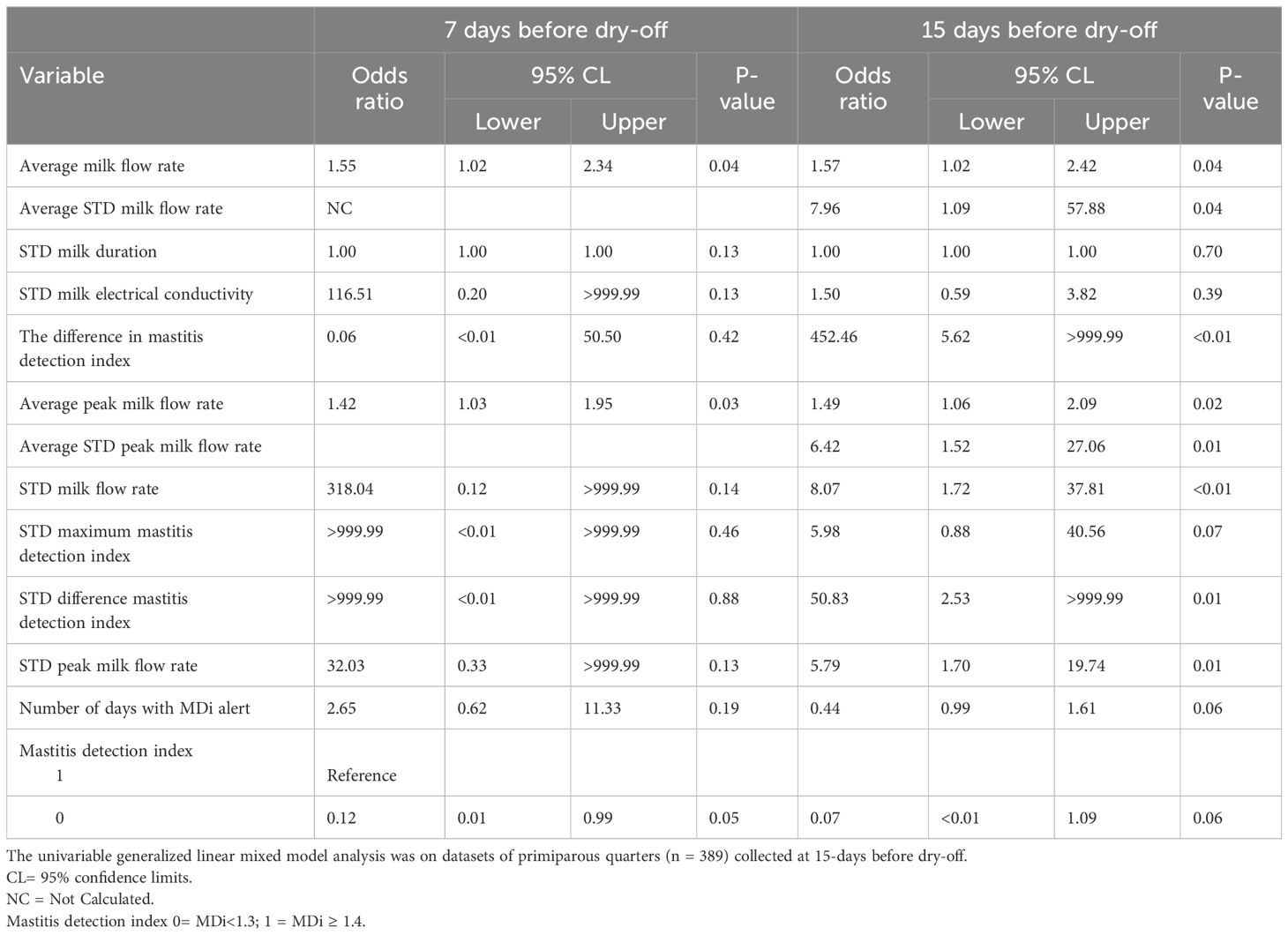
Table 2. Association between cow-level and quarter-level variables and intramammary in primiparous cows at dry-off.
Milk culture and microbial identification
The proportion of quarters with bacterial growth at dry-off was generally similar in both PRIM (24.42%) and MULT cows (24.13%) (Table 3). Overall, the most predominant organisms recovered from the quarter milk samples at dry-off were coagulase-negative staphylococci (CNS) for both primiparous (47.41%) and multiparous (35.35%), followed by Corynebacterium spp. at 13.79% in primiparous and 19.19% in multiparous. Brachybacterium spp., Aerococcus spp., and Enterococcus spp. were the least common microorganisms (0.86%), among the primiparous, while Enterococcus spp (1.01%), Brevibacterium spp (1.01%) and other Gram-negative bacteria (1.01%) were the least common among the multiparous cows. Cows that had bacterial growth in at least one quarter sample at dry-off were 71.4% and 46% for primiparous and multiparous, respectively. There was no bacterial growth in 211 (54.2%) and 172 (50%) quarter milk samples from all primiparous and multiparous cows, respectively.
The proportion of PRIM and MULT quarters with high SCC (PRIM: >100,000 cells/ml and MULT: >200,000 cells/ml) were 72.3% and 61%, respectively. Cows with at least one quarter with high SCC were 72% and 96.6% for PRIM and MULT, respectively. The proportion of quarters with an IMI (based on high SCC and positive culture results) at dry-off was 24% for the MULT and 23% for the PRIM. The proportion of cows with an intramammary infection in at least one quarter at dry-off was 44.8% and 70.8% for PRIM and MULT, respectively.
Association between cow-level and quarter-level variables and IMI at dry-off
Variables from two datasets (datasets 1 and 2) were investigated for association with IMI. A total of 10 variables of dataset 1 demonstrated association with intramammary infection at dry-off for PRIM cows (P < 0.20) (Table 2). On the other hand, only 4 variables in multiparous cows showed an association with IMI (P < 0.20) and were included in the multivariable model (Table 4). In dataset 2, up to 8 PRIM variables were associated with IMI at dry-off (P < 0.20) (Table 4), while 5 MULT variables were associated with IMI at dry-off (Table 5).
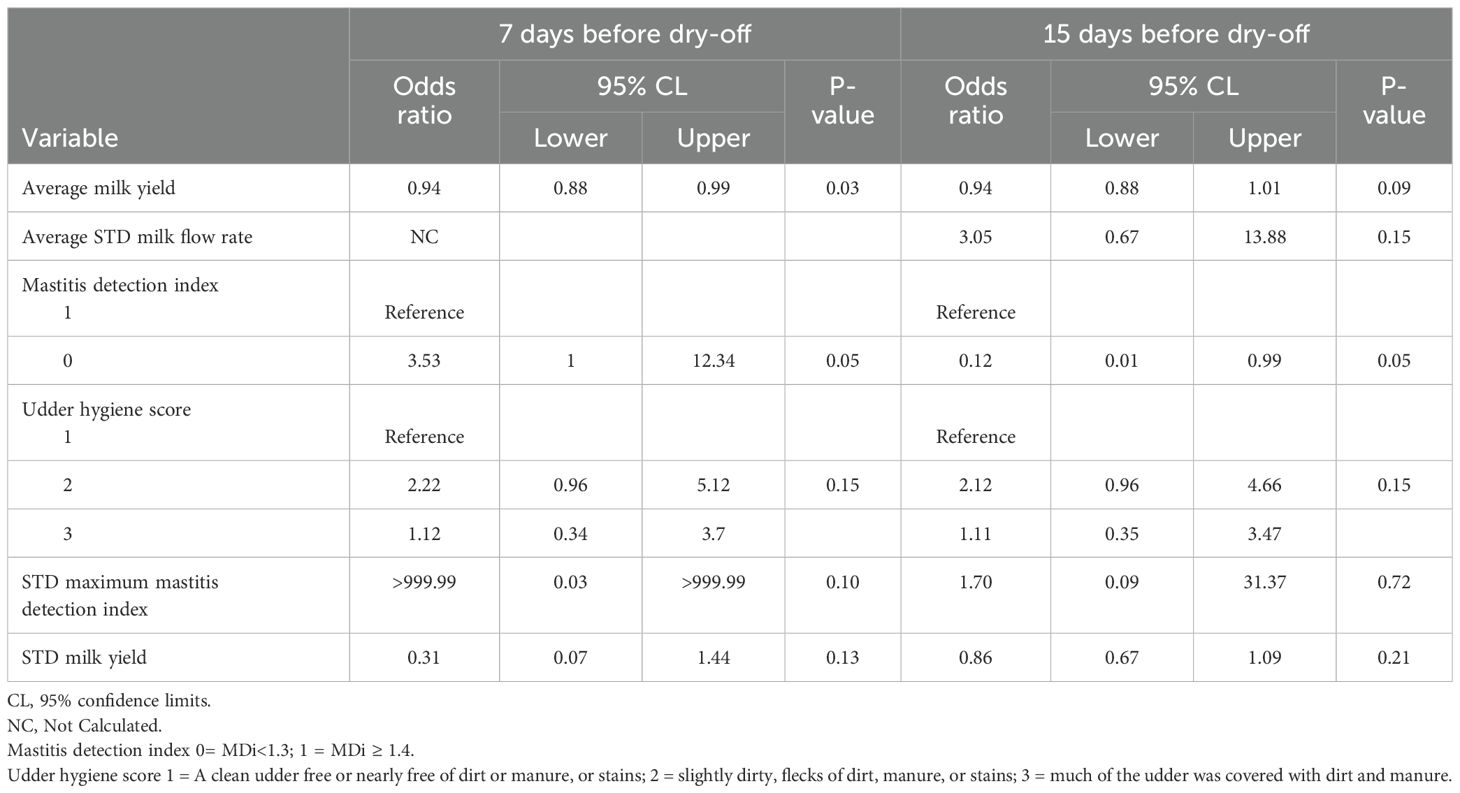
Table 4. Variables associated with intramammary infection at dry-off by univariable generalized linear mixed models of multiparous quarters (n = 389) for the 15 days before dry-off.
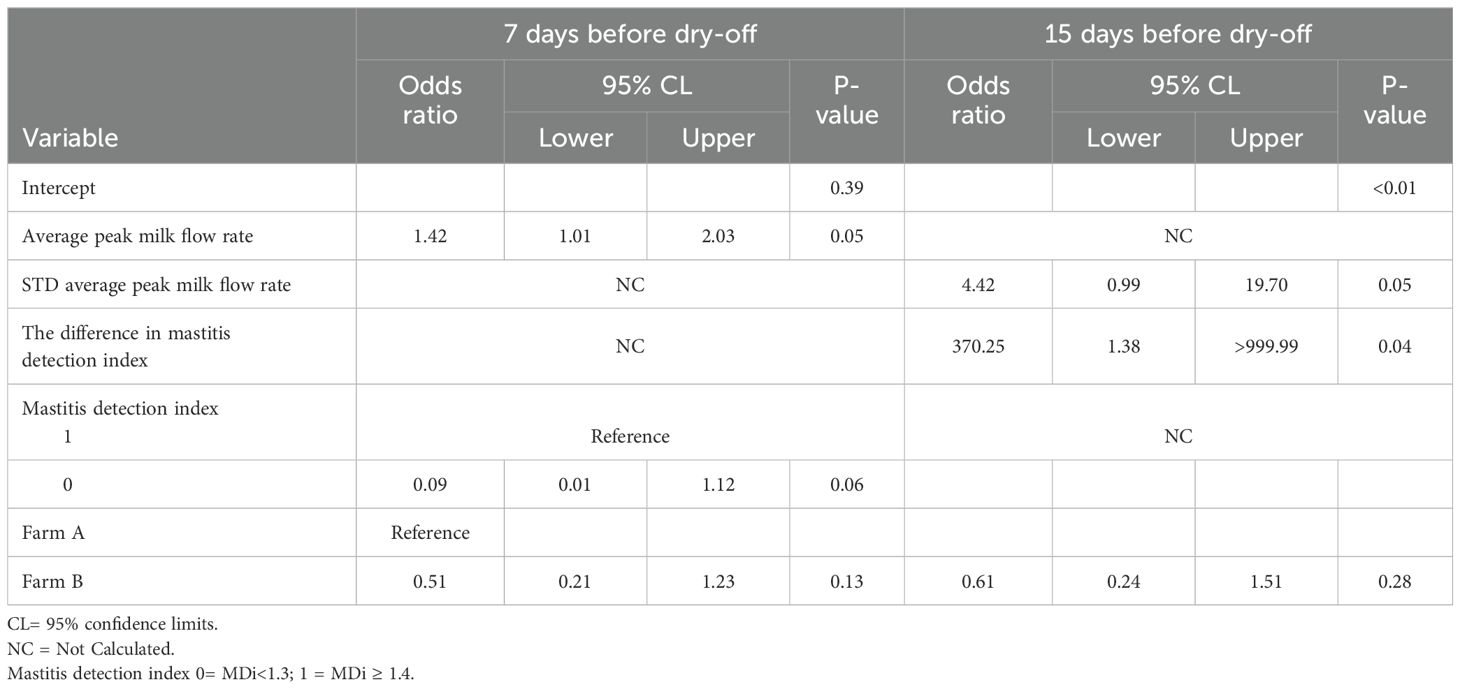
Table 5. Final multivariable logistic regression model describing the association of AMS variables and intramammary infection of primiparous quarters (n = 389) for the 15-days before dry-off.
Table 5 shows the results of the multivariable analysis for primiparous cows. Only the difference in mastitis detection index (DIF_MDi_15) was significantly associated with IMI in PRIM for dataset 1. The PRIM quarters with a greater difference in DIF_MDi_ranging from mean 0.07 to 1.07 had 370.25 greater odds of IMI at dry-off (CI:1.39->999.999). The statistical analysis found no significant association between IMI at dry-off and the standard deviation of the daily average peak milk flow rate during the 15 days (STD_PEAK_MILK_FLOW_15). Similarly, there was no significant association between IMI at dry-off and PRIM quarters with a one-unit increase in standard deviation during the 15 days of daily average peak milk flow rate (STD_PEAK_MILK_FLOW_15) (OR: 4.42, CI: 0.99-19.70). (Table 6). In multiparous cows, the variables mastitis detection index (MDi_CAT), average daily milk yield per quarter (AVG_DAILY_QT_MY_15), and the average daily standard deviation of milk flow rate during the 15-day period (AVG_STD_MILK_FLOW_15) were found to have a significant association with intramammary infection (IMI) at dry-off (P <0.05). The MULT quarters with MDi values >1.4 had 6.21 (CI: 1.63-23.65) greater odds of IMI at dry-off than quarters with MDi values <1.3. The MULT quarters with a one-unit increase in the AVG_STD_MILK_FLOW_15 from 0.31 to 1.31 lbs/min had 5.53 (CI: 1.08-28.47) greater odds of IMI at dry-off. A one-unit increase in average milk yield per quarter (AVG_DAILY_QT_MY_15) from 17.2 to 18.2 lbs was not significantly associated with IMI at dry-off (OR:0.95, CI: 0.88-1.02).
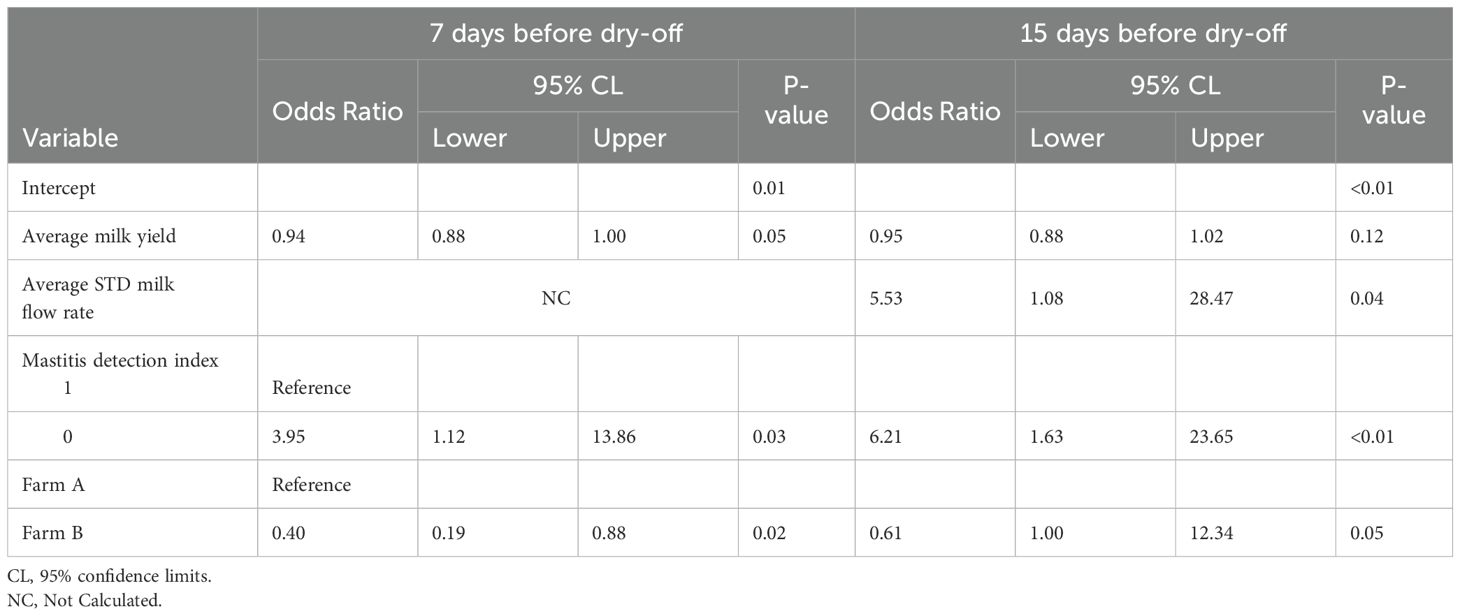
Table 6. Final multivariable logistic regression model describing the association of AMS variables and intramammary infection of multiparous quarters (n = 344) for the 15-days before dry-off.
In dataset 2, the multivariate regression analysis for PRIM revealed that the mastitis detection index categorical variable (MDi_CAT), and average peak milk flow rate (AVG_PEAK_MILK_FLOW_7) were associated with IMI. A quarter with a mastitis detection index of >1.4 was not significantly associated with IMI at dry-off (OR: 0.09, CI: 0.01-1.12). Compared to quarters with an average peak milk flow rate (AVG_PEAK_MILK_FLOW_7) during the period of (4.25 lbs/min), PRIM quarters with a one-unit increase in average peak milk flow had 1.42 (CI: 1.01-2.03) greater odds of IMI at dry-off (Table 5). MULT quarters showed a significant association between IMI and MDi_CAT, AVG_DAILY_QT_MY_7, and farm (Table 6). A one-unit increase in average milk yield during the 7 days (AVG_DAILY_QT_MY_7) from 16.14 to 17.14 lbs was associated with a 9.4% decrease in the odds (CI: 0.88-1.00) of IMI at dry-off. The MULT quarters with mastitis detection index (MDi_CAT) ≥1.4 had 3.95 (CI: 1.12-13.86) greater odds of IMI at dry-off as compared to quarters with MDi values <1.3.
Discussion
The aim of the study was to identify cow-level and quarter-level variables associated with intramammary infection at dry-off in cows milked using automatic milking systems. In dairy operations that utilize AMS, individual cow milk is not routinely tested for somatic cell count (SCC). However, this information is essential in guiding the selection of cows for treatment when implementing selective dry cow therapy (SDCT) on a farm. Hence, the results of this study could serve as an alternative source of information to help such operations implement an effective SDCT program. SDCT is considered a judicious approach to using antimicrobial drugs treatment and control intramammary infection in dairy cows. In the SDCT method, cows with IMI or at high risk for IMI during the dry period and early during the next lactation are selected to receive intramammary antimicrobial infusion and sealant, while normal and low-risk cows receive only teat sealants at dry-off (NMC, 2017; Schwarz et al., 2020). Dairy herds using AMS rely on the alerts generated from the AMS algorithms to identify cows with mastitis during the lactation period. Such cows are segregated for further examination and treatment if warranted. The AMS algorithm utilizes milk properties such as electrical conductivity, milk yield milk flow, peak milk flow, and mastitis detection index among others (Haine et al., 2018; Hogeveen et al., 2021; Khatun et al., 2018). Although the AMS has enabled farmers to manage mastitis and udder health, the wide range of sensitivity (45-83%) and specificity (91-99%) of AMS algorithms remains challenging (Dohoo et al., 2011; Godden et al., 2016) and, thus subject to possible misclassification of a cow with or without IMI. More so, the AMS algorithm is not optimized for the detection of subclinical mastitis, the major indication for dry cow therapy. The big data generated by the AMS system may help us improve the current algorithms for the detection of subclinical infection and make more precise management decisions at dry-off (Lokhorst et al., 2019). Such algorithm for detection of subclinical IMI can be developed by incorporating all the validated AMS parameters that are associated with IMI such as electrical conductivity, milk yield milk flow, peak milk flow, and mastitis detection index among others (Haine et al., 2018; Khatun et al., 2018).
The present study focused on two farms with AMS systems. The median Udder Hygiene Score (UHS) for primiparous and multiparous cows on both farms was 1, indicating that enrolled cows had clean udders that were free or nearly free of dirt, manure, or stains. The most predominant organisms in both the primiparous and multiparous quarters were Coagulase-negative staphylococci (CNS) followed by Corynebacterium species. Bradley et al., 2015, reported similar organisms on 12 herds from 6 European countries, with CNS (19.49%) and Corynebacterium species (37.83%) dominating. On the contrary, lower proportions of Corynebacterium spp. (6.7%) and CNS (13.1%) were reported in on Brazilian cow herds (Gonçalves et al., 2020). The low number of Staphylococcus aureus isolates in our study could be due to the adoption of good mastitis control program on these farms (Buggiotti et al., 2021; Grindal et al., 1991). In addition, non-aureus Staphylococcus and Corynebacterium spp have been reported as minor organisms and lack the ability to cause severe IMI cases (Taponen and Pyörälä, 2009). Blagitz et al. (2013) also suggested that they have a protective nature against major pathogens like Staphylococcus aureus. In our study, intramammary infection (IMI) was determined based on a quarter sample having both a culture-positive result and somatic cell count higher than the set threshold of 100,000 cells/ml for primiparous and 200,000 cells for multiparous cows. This definition of IMI enabled us to appropriately allocate healthy and IMI quarter samples thus reducing the likelihood of classifying quarters as false negatives (Malek dos Reis et al., 2011).
Furthermore, our results showed that four AMS parameters (mastitis detection index, quarter milk yield, quarter milk flow rate, peak milk flow rate) were associated with the presence of IMI at dry-off. Quarter milk flow rate is one of the most important parameters in predicting IMI, as a decrease in milk yield has been attributed to the onset of pathological changes in the mammary gland which reduces the milk production and milk yield concurrently (Gonçalves et al., 2020). This is attributed to the fact that inflammation of the mammary gland due to IMI induces cortisone production, which in turn inhibits milk letdown (Penry et al., 2017). Cows approaching the end of a lactation cycle also have reduced milk production, milk yield, milk flow rate, peak milk flow rate, and average milk duration. Reduced milk production at the end of the lactation cycle is more pronounced in the multiparous compared to the primiparous cows, possibly due to physiological nutrient channeling toward both milk production and growth in primiparous cows, while multiparous utilizes nutrients majorly for production (Wathes et al., 2007). Also, Buggiotti et al. (2021) argued that leucocytes of multiparous have stronger immune responses than primiparous.
With regards to milk flow rate, our results showed that an increased quarter peak milk flow rate was associated with greater odds of IMI. Similarly, previous studies also suggested that a high average peak milk flow rate (>4.4 lbs/min) occurring 7-14 days before dry-off due to weakening of the teat canal muscles, is associated with higher odds for IMI since the refractoriness in teat canal refolding post milking favors the invasion of the quarter by mastitis-causing pathogens (Inzaghi et al., 2021; Steeneveld et al., 2010). Furthermore, Grindal et al. (1991) suggested that cows with greater peak milk flow rates are more susceptible to infection. Our study shows different information for average and peak milk flow rate, but studies have revealed that they are strongly correlated (Zucali et al., 2021). On the contrary, a low quarter-level average peak milk flow rate (2.2 lbs/min) was reported to be associated with higher odds of IMI 7-14 days before mastitis occurrence (Hammer et al., 2012). Our statistical analysis revealed that IMI was associated with both low and high peak milk flow rate during 0-7 days before dry-off and a similar association was also reported by (Zucali et al., 2021).
Our results also revealed that increased milk yield was associated with lower odds of IMI. This finding was similar to earlier findings from a study in Canada (Pinedo et al., 2012) which revealed that lower milk yield before dry-off in dairy cattle may be attributed to poor udder health already in that lactation, especially in dairy cattle with higher risk of developing new IMI after calving. Wagemann-Fluxá et al. (2024) hypothesized that farmers manage AMS herds with higher quantities of milk at dry-off better to achieve higher production levels. As a result, improved udder health decreases the odds of IMI at dry-off. Furthermore, a higher concentration of lactoferrin, a multifunctional glycoprotein with antibacterial properties has been reported in cattle producing lower milk yields at dry-off (Vilar and Rajala-Schultz, 2020). On the contrary, a previous study in Belgium showed a higher milk yield in CNS-infected udder quarters as compared to healthy quarters (Piepers et al., 2013). The authors hypothesized that this could be attributed to a protective effect of current CNS infections against a future occurrence of infection caused by major mastitis pathogens (Piepers et al., 2013).
Contrary to previous reports (Bonestroo et al., 2022; Hammer et al., 2012; Khatun et al., 2017), the present study revealed that electrical conductivity was not associated with IMI. This could be attributable to the fact that electrical conductivity varies depending on the prior treatment and cure process of the cows which alters the physical properties of milk (Norberg et al., 2004). Given that the studied cows were subjected to BDCT in the previous lactation, it was possible that the electrical conductivity couldn’t significantly increase to an IMI predictive magnitude. A previous study revealed that alveoli milk had a lower electrical conductivity than teat milk therefore fore stripping the teats before actual milking could have led to the recording of lower electrical conductivity values by the sensors (Khatun et al., 2019). The use of electrical conductivity along with different AMS algorithms has been reported in previous studies with varying sensitivity (99%) and specificity (60%); sensitivity of 90% and specificity of 90% were reported in a recent study (Khatun et al., 2018).
For this study, we collaborated with DeLaval® AMS manufacturer to provide data access. Farmers using DeLaval® AMS routinely use the mastitis detection index (MDi) to check for mastitis. MDi is a composite variable that incorporates electrical conductivity, blood in the milk, and milk interval. The mastitis detection index threshold 1.4 is used by farmers to flag a cow as having IMI, and it has been proven to predict IMI accurately. The present study revealed that an increase in the mastitis detection index above the 1.4 threshold used by farmers for the selection of cows suspected of IMI was associated with higher odds of IMI (OR:6.21, CI: 1.63-23.65). It has been suggested that the performance of the mastitis detection index might be attributed to the influence of milk fat, breed, and color due to blood or color in milk (Rasmussen and Bjerring, 2005). Based on the results revealed in our study, it might be misleading for farmers to rely solely on mastitis detection index alerts as a criterion to select cows suspected of having IMI at dry-off. Further studies evaluating the sensitivity, specificity, and other performance parameters of MDi for cows at dry-off are needed.
We would like to mention some of the limitations of this study. Firstly, we only used one brand of AMS which might have yielded different results if we had used different brands. Secondly, the samples were collected only during the summer and early fall of one year. Previous studies have shown that the increase in temperature during this season can adversely reduce the population of bacteria in the environment, which could have affected our study’s findings (Amimoto et al., 2021; Hillerton et al., 1987). Furthermore, only two dairy farms were enrolled in our study. However, the present study revealed that farm B was significantly associated with lower odds of IMI at dry-off than farm A (OR = 0.40, CI: 0.19-0.88). It has been suggested that this could be due to the significant increase in bacteriological cure due to the treatment of Gram-negative bacteria (Schukken et al., 2011). We, however, used MALDI-TOF techniques to classify our microbial population. Given its high level of classification accuracy, the MALDI-TOF technique has been utilized in large and small-scale studies (Barreiro et al., 2010). This, therefore, prevents the misclassification of closely related organisms like the Aerococcus species and Lactococcus species as Streptococcus species (Murray, 2010).
Conclusion
Our results show that the most predominant organisms were Coagulase-negative staphylococci (CNS) followed by Corynebacterium species irrespective of parity. The isolation of Staphylococcus aureus was however low in our study. Our results indicate that mastitis detection index, milk flow rate, and peak milk flow rate were associated with higher odds of IMI at dry-off. An increase in milk yield per quarter was associated with lower odds of IMI at dry-off. In the primiparous, mastitis detection index was not associated with intramammary infection 7 days before dry-off. Stronger associations were observed with data collected 7 days before dry-off. Further studies are warranted to develop and validate algorithms integrating these variables to predict intramammary infections in cows at dry-off.
Data availability statement
Data collected for this study is subject to California Food and Agriculture Code (FAC) Sections 14400 to 14408. FAC Section 14407 requires that data collected be held confidential to prevent the identification of a farm or business. Requests for raw data may be made to the authors, who will consult with the California Department of Food and Agriculture (CDFA) on fulfilling the request. Unaggregated data that would identify a farm or business from this study are not able to be shared.
Ethics statement
The animal study was approved by University of California, Davis, Institutional Animal Care, and Use Committee. The study was conducted in accordance with the local legislation and institutional requirements.
Author contributions
JO: Investigation, Methodology, Writing – review & editing, Visualization, Data curation, Formal analysis, Validation, Writing – original draft. HO: Supervision, Visualization, Writing – original draft, Writing – review & editing. FF: Writing – review & editing, Conceptualization, Formal analysis, Funding acquisition, Investigation, Methodology, Project administration, Resources, Software, Supervision, Validation. EO: Conceptualization, Funding acquisition, Investigation, Methodology, Project administration, Resources, Software, Supervision, Writing – review & editing, Visualization.
Funding
The author(s) declare financial support was received for the research, authorship, and/or publication of this article. This study was supported by a research contract award from the California Department of Food and Agriculture’s Antimicrobial Use and Stewardship program (Contract # 20-1085–000-SG). Additional support was obtained from UC Davis Library Open Access Funds. The funders did not participate in study design, data collection, analysis, manuscript preparation, or decision to publish.
Acknowledgments
The authors express their gratitude to the owners and staff of the two dairy farms for their contributions to the study. Additionally, Kaitlyn McFarlan is acknowledged for her contribution to sample collection.
Conflict of interest
The authors declare that the research was conducted in the absence of any commercial or financial relationships that could be construed as a potential conflict of interest.
Publisher’s note
All claims expressed in this article are solely those of the authors and do not necessarily represent those of their affiliated organizations, or those of the publisher, the editors and the reviewers. Any product that may be evaluated in this article, or claim that may be made by its manufacturer, is not guaranteed or endorsed by the publisher.
References
Amimoto M., Ouchi Y., Okita M., Hirota T., Imura Y., Bungo T. (2021). Application of a simple measuring method to evaluate the fecal microflora of dairy cows in the summer season. J. Adv Vet. Anim. Res. 8, 307–311. doi: 10.5455/javar.2021.h516
Arruda A. G., Godden S., Rapnicki P., Gorden P., Timms L., Aly S. S., et al. (2013). Randomized noninferiority clinical trial evaluating 3 commercial dry cow mastitis preparations: I. Quarter-level outcomes. J. Dairy Sci 96(7), 4419–4435. doi: 10.3168/jds.2012-6502
Barreiro J. R., Ferreira C. R., Sanvido G. B., Kostrzewa M., Maier T., Wegemann B., et al. (2010). Short communication: Identification of subclinical cow mastitis pathogens in milk by matrix-assisted laser desorption/ionization time-of-flight mass spectrometry. J. Dairy Sci. 93, 5661–5667. doi: 10.3168/jds.2010-3614
Blagitz M. G., Souza F. N., Santos B. P., Batista C. F., Parra A. C., Azevedo L. F. F., et al. (2013). Function of milk polymorphonuclear neutrophil leukocytes in bovine mammary glands infected with corynebacterium bovis. J. Dairy Sci. 96, 3750–3757. doi: 10.3168/jds.2012-6370
Bonestroo J., Van Der Voort M., Hogeveen H., Emanuelson U., Klaas C., Fall N. (2022). Forecasting chronic mastitis using automatic milking system sensor data and gradient-boosting classifiers. Comput. Electron. Agric. 198, 107002. doi: 10.1016/j.compag.2022.107002
Bradley A. J. (2002). Bovine mastitis: an evolving disease. Vet. J. 164, 116–128. doi: 10.1053/TVJL.2002.0724
Bradley A. J., De Vliegher S., Green M. J., Larrosa P., Payne B., Van de Leemput E. S., et al. (2015). An investigation of the dynamics of intramammary infections acquired during the dry period on European dairy farms. J. Dairy Sci. 98, 6029–6047. doi: 10.3168/jds.2014-8749
Buggiotti L., Cheng Z., Salavati M., Wathes C. D., Fahey A., Crisà A., et al. (2021). Comparison of the transcriptome in circulating leukocytes in early lactation between primiparous and multiparous cows provides evidence for age-related changes. BMC Genomics 22, 1–17. doi: 10.1186/s12864-021-07977-5
Bursac Z., Gauss C. H., Williams D. K., Hosmer D. W. (2008). Purposeful selection of variables in logistic regression. Source Code Biol. Med. 3. doi: 10.1186/1751-0473-3-17
Cameron M., Keefe G. P., Roy J. P., Stryhn H., Dohoo I. R., McKenna S. L. (2015). Evaluation of selective dry cow treatment following on-farm culture: Milk yield and somatic cell count in the subsequent lactation. J. Dairy Sci. 98, 2427–2436. doi: 10.3168/jds.2014-8876
Cha E., Bar D., Hertl J. A., Tauer L. W., Bennett G., González R. N., et al. (2011). The cost and management of different types of clinical mastitis in dairy cows estimated by dynamic programming. J. Dairy Sci. 94, 4476–4487. doi: 10.3168/JDS.2010-4123
Compton C. W. R., Heuer C., Parker K., McDougall S. (2007). Epidemiology of mastitis in pasture-grazed peripartum dairy heifers and its effects on productivity. J. Dairy Sci. 90, 4157–4170. doi: 10.3168/jds.2006-880
De Koning C.J.A.M. (2010). “Automatic milking – common practice on dairy farms,” in University of Minnesota: The First North American Conference on Precision Dairy Management, Vol. 16.
Dohoo I. R., Smith J., Andersen S., Kelton D. F., Godden S. (2011). Diagnosing intramammary infections: Evaluation of definitions based on a single milk sample. J. Dairy Sci. 94, 250–261. doi: 10.3168/jds.2010-3559
Forsbäck L., Lindmark-Månsson H., Åndrén A., Kerstedt M., Svennersten-Sjaunja K. (2009). Udder quarter milk composition at different levels of somatic cell count in cow composite milk. Animal 3, 710–717. doi: 10.1017/S1751731109004042
Garcia S. N., Osburn B. I., Cullor J. S. (2019). A one health perspective on dairy production and dairy food safety. One Health 7, 100086. doi: 10.1016/j.onehlt.2019.100086
Godden S. M., Royster E., Knauer W., Sorg J., Lopez-Benavides M., Schukken Y., et al. (2016). Randomized noninferiority study evaluating the efficacy of a postmilking teat disinfectant for the prevention of naturally occurring intramammary infections. J. Dairy Sci. 99, 3675–3687. doi: 10.3168/jds.2015-10379
Godden S. M., Royster E., Timmerman J., Rapnicki P., Green H. (2017). Evaluation of an automated milk leukocyte differential test and the California Mastitis Test for detecting intramammary infection in early- and late-lactation quarters and cows. J. Dairy Sci. 100, 6527–6544. doi: 10.3168/JDS.2017-12548
Gonçalves J. L., Kamphuis C., Martins C. M. M. R., Barreiro J. R., Tomazi T., Gameiro A. H., et al. (2018). Bovine subclinical mastitis reduces milk yield and economic return. Livestock Sci. 210, 25–32. doi: 10.1016/J.LIVSCI.2018.01.016
Gonçalves J. L., Kamphuis C., Vernooij H., Araújo J. P., Grenfell R. C., Juliano L., et al. (2020). Pathogen effects on milk yield and composition in chronic subclinical mastitis in dairy cows. Vet. J. 262, 105473. doi: 10.1016/j.tvjl.2020.105473
Grindal R. J., Walton A. W., Hillerton J. E. (1991). Influence of milk flow rate and streak canal length on new intramammary infection in dairy cows. J. Dairy Res. 58, 383–388. doi: 10.1017/S0022029900029988
Haine D., Dohoo I., Dufour S. (2018). Selection and misclassification biases in longitudinal studies. Front. Vet. Sci. 5. doi: 10.3389/fvets.2018.00099
Hammer J. F., Morton J. M., Kerrisk K. L. (2012). Quarter-milking-, quarter-, udder- and lactation-level risk factors and indicators for clinical mastitis during lactation in pasture-fed dairy cows managed in an automatic milking system. Aust. Vet. J. 90, 167–174. doi: 10.1111/j.1751-0813.2012.00917.x
Higgins H. M., Golding S. E., Mouncey J., Nanjiani I., Cook A. J. C. (2017). Understanding veterinarians’ prescribing decisions on antibiotic dry cow therapy. J. Dairy Sci. 100, 2909–2916. doi: 10.3168/jds.2016-11923
Hillerton J. E., Bramley A. J., Watson C. A. (1987). The epidemiology of summer mastitis; a survey of clinical cases. Br. Vet. J. 143, 520–530. doi: 10.1016/0007-1935(87)90041-8
Hogeveen H., Klaas I. C., Dalen G., Honig H., Zecconi A., Kelton D. F., et al. (2021). Novel ways to use sensor data to improve mastitis management. J. Dairy Sci. 104, 11317–11332. doi: 10.3168/JDS.2020-19097
Hovinen M., Pyörälä S. (2011). Invited review: Udder health of dairy cows in automatic milking. J. Dairy Sci. 94, 547–562. doi: 10.3168/jds.2010-3556
Inzaghi V., Zucali M., Thompson P. D., Penry J. F., Reinemann D. J. (2021). Changes in electrical conductivity, milk production rate and milk flow rate prior to clinical mastitis confirmation. Ital. J. Anim. Sci. 20, 1552–1559. doi: 10.1080/1828051X.2021.1984852
Jensen D. B., Hogeveen H., De Vries A. (2016). Bayesian integration of sensor information and a multivariate dynamic linear model for prediction of dairy cow mastitis. J. Dairy Sci. 99, 7344–7361. doi: 10.3168/jds.2015-10060
Kamphuis C., Sherlock R., Jago J., Mein G., Hogeveen H. (2008). Automatic detection of clinical mastitis is improved by in-line monitoring of somatic cell count. J. Dairy Sci. 91, 4560–4570. doi: 10.3168/jds.2008-1160
Khatun M., Bruckmaier R. M., Thomson P. C., House J., García S. C. (2019). Suitability of somatic cell count, electrical conductivity, and lactate dehydrogenase activity in foremilk before versus after alveolar milk ejection for mastitis detection. J. Dairy Sci. 102, 9200–9212. doi: 10.3168/jds.2018-15752
Khatun M., Clark C. E. F., Lyons N. A., Thomson P. C., Kerrisk K. L., Garciá S. C. (2017). Early detection of clinical mastitis from electrical conductivity data in an automatic milking system. Anim. Prod. Sci. 57, 1226–1232. doi: 10.1071/AN16707
Khatun M., Thomson P. C., Kerrisk K. L., Lyons N. A., Clark C. E. F., Molfino J., et al. (2018). Development of a new clinical mastitis detection method for automatic milking systems. J. Dairy Sci. 101, 9385–9395. doi: 10.3168/JDS.2017-14310
Liang D., Arnold L. M., Stowe C. J., Harmon R. J., Bewley J. M. (2017). Estimating US dairy clinical disease costs with a stochastic simulation model. J. Dairy Sci. 100, 1472–1486. doi: 10.3168/jds.2016-11565
Lipkens Z., Piepers S., De Visscher A., De Vliegher S. (2019). Evaluation of test-day milk somatic cell count information to predict intramammary infection with major pathogens in dairy cattle at drying off. J. Dairy Sci. 102, 4309–4321. doi: 10.3168/JDS.2018-15642
Lokhorst C., De Mol R. M., Kamphuis C. (2019). Invited review: Big Data in precision dairy farming. Animal 13, 1519–1528. doi: 10.1017/S1751731118003439
Malek dos Reis C. B., Barreiro J. R., Moreno J. F. G., Porcionato M. A. F., Santos M. V. (2011). Evaluation of somatic cell count thresholds to detect subclinical mastitis in Gyr cows. J. Dairy Sci. 94, 4406–4412. doi: 10.3168/JDS.2010-3776
McParland S., Dillon P. G., Flynn J., Ryan N., Arkins S., Kennedy A. (2019). Effect of using internal teat sealant with or without antibiotic therapy at dry-off on subsequent somatic cell count and milk production. J. Dairy Sci. 102, 4464–4475. doi: 10.3168/JDS.2018-15195
Murray P. R. (2010). Matrix-assisted laser desorption ionization time-of-flight mass spectrometry: usefulness for taxonomy and epidemiology. Clin. Microbiol. Infect.: Off. Publ. Eur. Soc. Clin. Microbiol. Infect. Dis. 16, 1626–1630. doi: 10.1111/J.1469-0691.2010.03364.X
NAHMS (2014). National animal health monitoring system. Dairy cattle management practices in the United States. United States Department Agric. 1.
Norberg E., Hogeveen H., Korsgaard I. R., Friggens N. C., Sloth K. H. M. N., Løvendahl P. (2004). Electrical conductivity of milk: Ability to predict mastitis status. J. Dairy Sci. 87, 1099–1107. doi: 10.3168/jds.S0022-0302(04)73256-7
Patel K., Godden S. M., Royster E. E., Timmerman J. A., Crooker B. A. (2017). Pilot study: Impact of using a culture-guided selective dry cow therapy program targeting quarter-level treatment on udder health and antibiotic use. Bovine Practitioner 51, 48–57. doi: 10.21423/bovine-vol51no1p48-57
Penry J. F., Crump P. M., Ruegg P. L., Reinemann D. J. (2017). Short communication: Cow- and quarter-level milking indicators and their associations with clinical mastitis in an automatic milking system. J. Dairy Sci. 100, 9267–9272. doi: 10.3168/jds.2017-12839
Piepers S., Schukken Y. H., Passchyn P., De Vliegher S. (2013). The effect of intramammary infection with coagulase-negative staphylococci in early lactating heifers on milk yield throughout first lactation revisited. J. Dairy Sci. 96, 5095–5105. doi: 10.3168/jds.2013-6644
Pinedo P., Fleming C., Risco C. (2012). Events occurring during the previous lactation, the dry period, and peripartum as risk factors for early lactation mastitis in cows receiving 2 different intramammary dry cow therapies. J. Dairy Sci. 95, 7015–7026. doi: 10.3168/jds.2012-5398
Pyörälä S. (2003). Indicators of inflammation in the diagnosis of mastitis. Vet. Res. 34, 565–578. doi: 10.1051/VETRES:2003026
Rasmussen M. D., Bjerring M. (2005). Visual scoring of milk mixed with blood. J. Dairy Res. 72, 257–263. doi: 10.1017/S0022029905000853
Rollin E., Dhuyvetter K. C., Overton M. W. (2015). The cost of clinical mastitis in the first 30 days of lactation: An economic modeling tool. Prev. Vet. Med. 122, 257–264. doi: 10.1016/j.prevetmed.2015.11.006
Rowe S. M., Godden S. M., Nydam D. V., Gorden P. J., Lago A., Vasquez A. K., et al. (2020). Randomized controlled non-inferiority trial investigating the effect of 2 selective dry-cow therapy protocols on antibiotic use at dry-off and dry period intramammary infection dynamics. J. Dairy Sci. 103, 6473–6492. doi: 10.3168/jds.2019-17728
Schukken Y. H., Bennett G. J., Zurakowski M. J., Sharkey H. L., Rauch B. J., Thomas M. J., et al. (2011). Randomized clinical trial to evaluate the efficacy of a 5-day ceftiofur hydrochloride intramammary treatment on nonsevere gram-negative clinical mastitis. J. Dairy Sci. 94, 6203–6215. doi: 10.3168/jds.2011-4290
Schwarz D., Santschi D. E., Durocher J., Lefebvre D. M. (2020). Evaluation of the new differential somatic cell count parameter as a rapid and inexpensive supplementary tool for udder health management through regular milk recording. Prev. Vet. Med. 181, 105079. doi: 10.1016/j.prevetmed.2020.105079
Steeneveld W., van der Gaag L. C., Ouweltjes W., Mollenhorst H., Hogeveen H. (2010). Discriminating between true-positive and false-positive clinical mastitis alerts from automatic milking systems. J. Dairy Sci. 93, 2559–2568. doi: 10.3168/JDS.2009-3020
Taponen S., Pyörälä S. (2009). Coagulase-negative staphylococci as cause of bovine mastitis-Not so different from Staphylococcus aureus? Vet. Microbiol. 134, 29–36. doi: 10.1016/j.vetmic.2008.09.011
Vilar M. J., Rajala-Schultz P. J. (2020). Dry-off and dairy cow udder health and welfare: Effects of different milk cessation methods. Vet. J. 262, 105503. doi: 10.1016/J.TVJL.2020.105503
Wagemann-Fluxá C. A., Kelton D. F., DeVries T. J. (2024). Associations of cow- and herd-level factors during the dry period with indicators of udder health in early-lactation cows milked by automated milking systems. J. Dairy Sci. 107, 459–475. doi: 10.3168/jds.2023-23796
Wathes D. C., Cheng Z., Bourne N., Taylor V. J., Coffey M. P., Brotherstone S. (2007). Differences between primiparous and multiparous dairy cows in the inter-relationships between metabolic traits, milk yield and body condition score in the periparturient period. Domest. Anim. Endocrinol. 33, 203–225. doi: 10.1016/j.domaniend.2006.05.004
Keywords: automatic milking systems, intramammary infection, dairy cows, dry-off period, selective dry cow therapy
Citation: Ongom J, Okella H, Ferreira FC and Okello E (2024) Association between automatic milking system parameters and intramammary infections in dairy cows at dry-off. Front. Anim. Sci. 5:1397144. doi: 10.3389/fanim.2024.1397144
Received: 06 March 2024; Accepted: 04 September 2024;
Published: 24 September 2024.
Edited by:
Christa Egger-Danner, ZuchtData EDV-Dienstleistungen GmbH, AustriaReviewed by:
Diana Giannuzzi, University of Padua, ItalyHermann H. Swalve, Martin Luther University of Halle-Wittenberg, Germany
Copyright © 2024 Ongom, Okella, Ferreira and Okello. This is an open-access article distributed under the terms of the Creative Commons Attribution License (CC BY). The use, distribution or reproduction in other forums is permitted, provided the original author(s) and the copyright owner(s) are credited and that the original publication in this journal is cited, in accordance with accepted academic practice. No use, distribution or reproduction is permitted which does not comply with these terms.
*Correspondence: Emmanuel Okello, eokello@ucdavis.edu