- 1Animal Breeding and Husbandry in the Tropics and Subtropics, University of Hohenheim, Stuttgart, Germany
- 2Livestock Genetics, International Livestock Research Institute, Nairobi, Kenya
- 3Animal and Veterinary Science, Scotland’s Rural College, Edinburgh, United Kingdom
The current review examines potential solutions to enhance the sustainability and productivity of the dairy sector in sub-Saharan Africa (SSA) with an emphasis on breeding for resilience. Additionally, the paper explores various indicators for measuring resilience and provides insights into the data that can be utilized to quantify resilience in SSA’s dairy production systems. Dairy production contributes significantly to food and nutritional security and employment in SSA. However, besides the general lack of enabling policy and institutional environments, production is negatively affected by environmental challenges such as high temperatures and heat stress, diseases and parasites, unreliable rainfall patterns, shortages of feeds and forages and undue preference for taurine cattle breeds regardless of their poor adaptability to prevailing local conditions. Fostering the resilience capacity of dairy animals is imperative to combat climate-related adversities and maintain productivity. This can only be achieved if reliable and practical methods for quantifying and analyzing resilience in SSA are described and undertaken. This study has reviewed variance of deviations, root mean square of deviations, autocorrelation of deviations, skewness of deviations, slope of the reaction norm and its absolute value as possible indicators of resilience in SSA. While previous research has reported genetic variation and favorable correlations of these indicators with health, fitness, and fertility traits, their potential in SSA environments requires further investigation. Besides, labor- and cost-effective phenotypic data collection is essential for characterization of resilience using these indicators. Through this study, we propose frequently collected data on milk production traits, body fat-related traits, and activity patterns as suitable in the sub-Saharan Africa context. The African Asian Dairy Genetic Gains Project by the International Livestock Research Institute (ILRI) offers a valuable opportunity to collate data from diverse dairy systems in SSA for testing the potential of these indicators. Insights from this study are helpful in improving resilience of dairy animals in SSA, which would contribute to poverty alleviation, animal welfare improvement, and better preparedness in lieu of climate change in SSA.
1 Introduction
Livestock production in sub-Saharan Africa (SSA) contributes to the global livestock landscape in terms of number of live animals, their global carbon footprint, number of their keeper and number of people who extract direct and indirect benefit from them (Poole et al., 2013). However, due to growth in human population and increased urbanization, the arable land has significantly decreased, leading to a reduced growth in livestock production at a time when the demand is persistently growing (Nardone et al., 2010; Britt et al., 2018; Hernández-Castellano et al., 2019). The demand for meat and dairy products in SSA is projected to double over the next two decades (Nyameasem et al., 2018).
Dairy production significantly serves various purposes, including contributing to food and nutritional security, income, and non-market purposes (Moll et al., 2007). Despite considerable growth witnessed in the sector in recent years, the demand for dairy products still surpasses the production level (Marshall et al., 2019; Ojango et al., 2019). However, for sustainable milk production with the ongoing climate change, attention on dairy production must shift from increased production per se to increased profitability, efficiency, and resilience. Sustainable practices that ensure food security for the growing population and overcome the negative impacts of climate change on dairy cattle need to be adopted.
Sub-Saharan Africa experiences environmental challenges including severe droughts, high heat loads, diseases and parasites, and shortages of feeds and forages, all of which negatively affect dairy production. The naturally occurring challenges (i.e., intrinsic characteristics of the environment) such as droughts and high temperatures, cannot be easily averted through husbandry interventions. It is therefore vital to breed for improved resilience and productivity to enable animals to cumulatively weather the environmental stressors while maintaining or even improving their productivity (Oloo et al., 2022a).
An animal’s degree of resilience to an environmental disturbance is demonstrated by its capacity to be minimally affected by that perturbation or rapidly return to the state pertained before exposure to the disturbance (Berghof et al., 2019b). The degree of resilience to environmental disturbance is influenced by various factors, including but not limited to its genetics, sex, and age (von Holst, 1998; Cockram, 2004; Veissier and Miele, 2014). However, resilience is not well defined or measured in livestock populations of SSA as information on the related functional traits is limited. This study thus reviews dairy production in SSA and the challenges it faces, the importance of resilience in dairy cattle to environmental disturbances, and how resilience can be harnessed to impact the sub-Saharan Africa dairy industry positively and sustainably.
2 Status of dairy production in sub-Saharan Africa
Significant growth in the dairy sector has been witnessed in recent years because of collaborative performance enhancement strategies involving various stakeholders in the industry (Marshall et al., 2019; Ojango et al., 2019). However, with the demand for milk and dairy products in this region increasing by more than 2% per annum (Alexandratos and Bruinsma, 2012), production is still below the demand. Though SSA hosts approximately 14.3% of the world’s human population, it produces less than 4% of the global milk (FAOSTAT, 2023). The average consumption of locally produced milk per head per day in SSA is the lowest globally and the rate of increase remains minimal (Figure 1A), (FAOSTAT, 2023). Although the average per capita milk consumption increased from 40.6g/head/day in 2001 to 60.3g/head/day in 2012 in SSA, thereafter it assumed a declining trend. FAO statistics show that the average milk yield produced per dairy cow per day in SSA is less than 2kg, a figure that has not changed for the last two decades (Figure 1B). Reversing the declining trend needs to be addressed promptly through research and innovation.
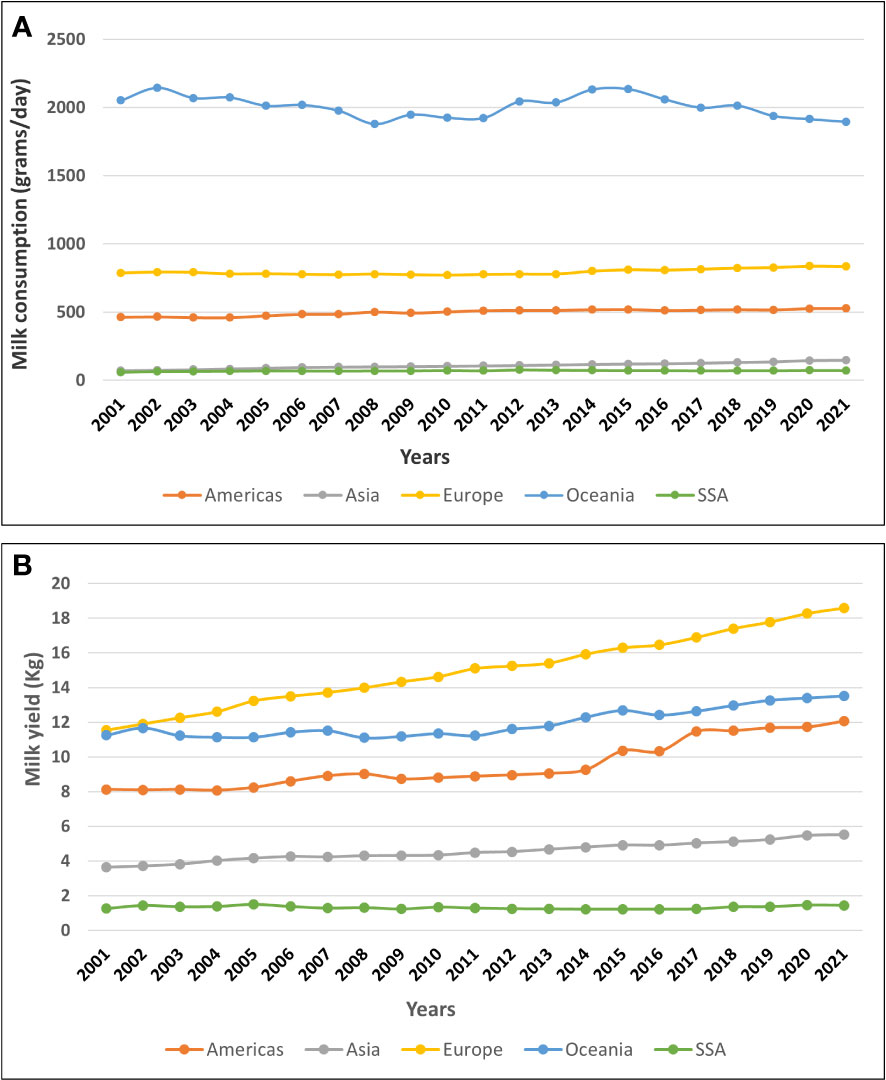
Figure 1 (A) A trend of average consumption of locally-produced milk per person per day within different regions of the world from 2001 to 2021 based on FAOSTAT estimate data. (B) A trend of average milk yield produced per cow per day in different regions of the world from 2001 to 2021 based on FAOSTAT estimate data.
The dairy sector, through dairy products, provides a source of nourishment and income to millions of people in SSA. It also contributes to non-marketable intangible benefits roles including social-cultural needs such as dowry payments, while cull cow and fattened male calves are slaughtered for cultural occasions and during important community fetes. The animals are also viewed as security assets and serve as insurance against uncertainties. They are also used to finance other needs, for example, proceeds from milk and cattle sales are used to pay school fees and medical bills. In many communities, cattle serve as status symbols for their owners, offering respect and reputation, depending on the number and types of cattle an individual has (Moll et al., 2007). Products such as refined milk are used as an avenue of blessing in the Maasai community in Kenya (Arhem, 1989), while manure is used as an organic fertilizer to support food/feed crop and pasture production and as fuel for cooking.
In SAA, livestock are raised under different production systems, ranging from small-scale subsistence farming to large-scale dairy farms. The descriptions of these systems have been documented by Chagunda et al. (2016) and Ojango et al. (2017). Distinctive features of these systems include: the land size, number of cattle owned, cattle genotype raised, and the level of management. Smallholder dairy systems have a landholding capacity that is rarely above 4 ha and herd sizes that vary between one to ten cattle. The genotypes of cattle reared depend on the level of economic investment and scale of operation. Smallholder systems mostly keep indigenous breeds and their crosses with exotic commercial dairy breeds, whereas large-scale commercial dairy farms mostly keep commercial exotic dairy breeds and their crosses with indigenous breeds (Ojango et al., 2016).
Smallholder systems in SSA supply the bulk of the milk needed, which is approximated to be 80%. Besides dairy cattle, these farmers keep a range of livestock species and practice mixed crop-livestock rainfed production (Herrero et al., 2010). Animals are grazed in communal pastures and occasionally receive leafy crop residues after harvesting as a supplement. Cow-calf milking is usually practiced whereby the calf is allowed to suckle in short intervals during the milking process. This is mainly because zebu cows only freely let down milk in the presence of their calves. Hand-milking is the common practice twice a day (Duguma and Janssens, 2015), mostly early in the morning before animals are taken out for grazing and in the evening when they are returned for overnight kraaling/corralling.
Overall, most dairy cattle in sub-Saharan Africa are crossbreds of highly adapted zebu breeds and high-production potential exotic breeds such as Ayrshire, Guernsey, Holstein Friesian, and Jersey (Ojango et al., 2017; Oloo et al., 2022b). This is because indigenous cattle produce little milk and exotic commercial cattle remain largely unaffordable to, and unmanageable by majority of farmers because they are delicate and require high management costs. Progressive upgrading of indigenous cattle through crossbreeding using exotic dairy bulls or semen through Artificial insemination (AI) is the most common strategy for increasing milk production. Although the uptake of artificial insemination is currently low (30%), most mating events occur through natural services using uncertified bulls. While farmers prefer to use AI services, they are mostly not readily available, and when available, they are unreliable and expensive compared to bull service (Mwanga et al., 2019). Bull ownership is dependent on the herd size; farmers with small herd sizes rarely own a bull and mostly rely on their neighbor’s or communal bulls while those with large herd sizes have their bulls for mating (Omer et al., 2021). In cases where genetic evaluation is not routinely done to inform bull choices, the choice of the breeding bull is mostly dependent on the proximity of locally available bull to the farmer (Lukuyu et al., 2019) and a few observable traits such as color, body formation, and size, as proxies to breed characteristics (Aman et al., 2021).
3 Management practices limiting dairy systems of sub-Saharan Africa
Performance of dairy breeds in SSA is often limited by the management practices adopted across the different farming systems as outlined below.
3.1 Inadequate animal husbandry
Since most dairy farmers in this region practice both crop and livestock production, they are usually busy attending to other farm chores and involving themselves with other income-generating activities to make ends meet. This prevents them from dedicating quality time to tend to their animals, notably ensuring good herd-health, estrus detection, adequate feed and water intake. Preventative herd health practices are rare and often ineffective. Late detection of health disorders increases the cost of treatment, worsens animal welfare, and reduces milk production (Stachowicz and Umstätter, 2021). Undetected estrus or conducting AI past the fertility window results in late ages at first calving and longer calving intervals (O’Connor, 2016). Farmers mainly manage their herds based on their knowledge, memory, and experiences, which sometimes may be inaccurate and misleading (Ojango et al., 2017). Also, poor housing is a major concern in SSA, which compromises the animals’ welfare leading to low production. Housing structures are made of different materials, with roofs of thatch grass, iron sheets, or combination of plastic sheets and grass thatch. Floors vary from mud, concrete, to sand. Bedding may or may not be provided. Stalls are mainly wooden and often poorly drained, leading to slurry accumulation, notably during rainy seasons. This creates challenges in maintaining cleanliness and poses risks as pathogens proliferate in the slurry (Ojango et al., 2017).
3.2 Inadequate and poor-quality feeds
In smallholder systems, cattle are grazed on natural pastures often on communal land (roadsides, beside riverbeds) and harvested lands to feed on crop residues with little or no supplementation with concentrates (Ojango et al., 2017). These farmers are sometimes not keen on the quality of feed that their dairy cows are fed, and even when they do, the capacity to assess feed quality before giving it to their herds if often low or lacking altogether. Where bought feeds are used, their qualities are not always assured (Lukuyu et al., 2009). In most cases, all animals are grazed together in the same field with no supplementary feeding, irrespective of the different nutritional needs of animals of different physiological groups. Furthermore, tropical pastures are known to be of lower nutritional quality compared to those in temperate regions (Ørskov, 2005). Therefore, without supplemental feeding, animals are often malnourished and predisposed to diseases, resulting in lower reproductive performance and production yields.
3.3 Inappropriate breeding practices
Few farmers have clearly defined breeding objectives for their dairy enterprises. With little information and supportive policies, inappropriate dairy cattle genotypes that do not match the local production environments are aggressively promoted by “sellers” of global dairy genetic companies. This has been precipitated by a lack of appropriate and comprehensive herd-recording (Hoffmann et al., 2012; Dürr, 2013; Mrode et al., 2020), hence inability to locally evaluate and select for milk production and resilience traits within the existing cattle population in this region. Therefore, most farmers tend to hitch-hike from selections undertaken in developed European and North and South American systems. Although most of the imported dairy genetics have high milk production potential, they have failed to perform to their genetic potentials in SSA because of inadequate management levels and poor adaptability to sub-Saharan African climate and production systems (Mwai et al., 2015). Moreover, as compared to indigenous breeds, exotic commercial dairy breeds generally have higher body weights and maintenance requirements in terms of feed and water and are thus unsuitable for the smallholder dairy SSA and tropical systems. Another bottleneck within SSA is unsystematic crossbreeding, which results in unpredictable results, and often under-performance (Galukande et al., 2013). If well implemented, crossbreeding can produce cattle that best match local systems.
4 Climate and environmental disturbances to dairy production in sub-Saharan Africa
Environmental disturbances are changes in environmental conditions that impair the normal functioning of a biological system (Capucchio et al., 2019). The SSA’s climate is characterized by high ambient temperatures, solar radiation, wind speed, and relative humidity, as well as seasonal and unpredictable rainfall. These climatic conditions present several environmental disturbances that impair the performance and production level of dairy cattle. The most common disturbances in SSA include heat stress, seasonal and scarcity in supply of water, diseases, and disease pressure.
4.1 Heat stress
Every animal has a thermal range within which it can comfortably operate without its normal physiological functions being altered or compromised. Heat stress in animals occurs when the rate of heat energy gain is higher than the rate of heat dissipation (Gantner et al., 2015). Animals gain heat either from metabolic processes such as respiration, digestion, and lactation or from their environment. They dissipate excess heat through evaporative heat loss mechanisms to maintain their thermal comfort zone (Angel et al., 2018). When ambient temperatures are high, animals accumulate extra radiant energy that they do not need. If coupled with high relative humidity, as commonly witnessed in many parts of SSA, evaporative loss of heat is compromised, making the animal to be heat-stressed (Bohmanova et al., 2007). Heat stress is quantified based on the temperature-humidity index (THI); high air temperature and high relative humidity increase the THI. Due to increasing global warming as a result of climate change, heat stress on animals in SSA is expected to increase (Ekine-Dzivenu et al., 2020; Ekine-Dzivenu et al., 2022). Heat stress has various negative impacts on the dairy production industry.
Heat stress affects the quality of milk by reducing the fat content of milk and causing low-fat milk syndrome. During rumen fermentation, rumen microbiota metabolizes almost all hexoses into pyruvate through the glycolytic pathway. Pyruvate is then broken down in different pathways into mainly three volatile fatty acids: acetate, propionate, and butyrate (Ungerfeld, 2020). The resulting ratio of acetate to propionate is an indicator of milk fat synthesis and is greatly affected by the diet of animals. Heat stress changes the diet preference and composition of cows significantly by increasing the intake of more concentrates compared to roughages (Uyeno et al., 2010) due to a higher amount of total digestible nutrients in concentrates. Feeding in diets rich in readily fermentable carbohydrates lowers the pH in the rumen, which leads to lower acetate and higher propionate production and an unbalanced ratio of acetate to propionate (National Research Council, 1988). Acetate is a precursor of milk fat; hence, reduction of its production volume results in reduced fat content in the milk (Baumgard and Rhoads, 2013; Angel et al., 2018).
Animals acclimatize to heat stress by adopting physiological responses that alleviate the negative effects of thermal challenge. One such response is a reduction in feed intake, which ensures that less feed is digested, hence decreasing metabolic heat produced through digestion process. Although this response helps the animal to immediately weather the stress, it reduces the milk production volume as less nutrients are available for milk production (Summer et al., 2019). Thermal stress also reduces the plantation cover including those utilized by dairy cattle for nutrients, which leads to a shortage of feed for dairy cows resulting in reduced dry matter intake.
Decreased feed intake in heat-stressed animals lowers the quantity of hexoses in feed to be broken down to release energy. As a result, these animals tend to metabolize their reserves to obtain energy to support basic metabolic processes. Therefore, the demand for glucose for energy is increased, which increases the rate at which glucose enters cells for glycolysis (Baumgard et al., 2007). Also, a heat-stressed lactating cow has increased concentrations of basal insulin (Baumgard and Rhoads, 2013) which decreases the rate of gluconeogenesis (Hatting et al., 2018). The upshot of reduced synthesis of glucose in the liver, less intake of hexoses, and high demand of glucose for energy is lower availability of glucose in the mammary gland to support the synthesis of lactose (Nardone et al., 1997; Nardone et al., 2010). Lactose is the prime sugar present in milk and, owing to its primary role in milk osmoregulation, it is the main determinant of milk production volume (Holsinger, 1997). Therefore, limited availability of glucose lowers the milk lactose content and milk yield and could account for reduced milk yield not directly explained by reduced feed intake under hot and humid conditions.
Thermal stress causes animals to be more susceptible to sub-clinical and acute rumen acidosis. A study by West (2003) demonstrated high respiratory rates in heat-stressed animals. Hyperventilation increases the rate at which carbon dioxide (CO2) is exhaled and induces a decrease in blood CO2 levels. The body of the animals responds to low blood CO2 levels through secretion of bicarbonate ions by kidney to level up this ratio (Schneider et al., 1988). However, this creates another problem by reducing the available bicarbonate ions that can be used in saliva to buffer and maintain a healthy rumen pH. Moreover, ruminants drool when panting, and this lowers the quantity of saliva that would otherwise be deposited in the rumen (Nardone et al., 2010). Decreased feed intake and reduced forage-to-concentrate ratio cause cattle to ruminate less, hence produce less saliva. These reductions in the salivary bicarbonate ions concentration, quantity of saliva produced, the amount of saliva entering the rumen, and forage intake make thermal-stressed cattle to be much more exposed to rumen acidosis (Kadzere et al., 2002; Plaizier et al., 2008).
High temperatures reduce the reproductive performance of the cows. Exposure of heifers and cows to heat-stressing conditions suppresses progesterone production and decreases its plasma concentration (Wolfenson et al., 2000). Low plasma progesterone concentrations impair follicular development, leading to aberrant maturation of oocytes in the ovulatory follicle, and consequently resulting in low conception rates (De Rensis and Scaramuzzi, 2003). It also interferes with ovarian steroidogenesis, a process by which ovarian cells produce hormones for the maintenance of reproductive tissues, regulation of ovarian function and ovulation, and the establishment of pregnancy. This subsequently affects formed corpus luteum and alters endometrial morphology and function in the ensuing cycle. This may lead to increased rates of embryo losses following successful insemination (Wolfenson et al., 2000). Besides, reduced feed intake in heat-stressed cows may result in negative energy balance which causes a decreased concentration of glucose and insulin-like growth factor-I. These metabolites play important roles in oocyte quality, follicular growth, and implantation (Madhusoodan et al., 2019). Hot conditions also compromise the estrus behavior of cows and might lead to up to 80% rate of undetected estrus (De Rensis and Scaramuzzi, 2003; Hernández-Castellano et al., 2019). Heat stress reduces growth rates and eventually mature body sizes in dairy cattle through reduction of available feeds and dry matter intake (Angel et al., 2018). This results in delayed sexual maturity and the onset of puberty (Le Cozler et al., 2008; Krpálková et al., 2014; Duplessis et al., 2015).
4.2 Water constraints
Clean water is one of the most important nutrients for a dairy cow and should always be available. Animal performance deteriorates more quickly and drastically under deficient water intake than any other nutrient deficiency. Cattle and any other domesticated animal can live for about 2 months without food but cannot cope for more than a week without water (Lardy et al., 2008). Water plays multiple physiological roles in the body of the animal, for instance, during lactation, water forms the major component of the milk as it comprises 87–88% of the milk. In metabolism, water is critical in the transportation of nutrients and metabolites to and from the tissue by the circulatory system. It plays a vital role in excretion, electrolyte balance, and thermoregulation. During pregnancy, water in form of amniotic fluid provides fluidity and cushioning environment for the developing fetus in the uterus (Giri et al., 2020).
Water intake requirement of a dairy cow is influenced by body weight, lactation stage, milk production potential, physiological state, dry matter intake, composition of diet, and climatic factors (Wakchaure et al., 2015; Wagner and Engle, 2021). Water consumption is directly associated with the body weight and size of the animals. Lactating and pregnant cows require more water than non-lactating and nonpregnant cows to support milk synthesis and fetus development, respectively. Dairy cows of higher genetic merits require a large amount of water compared to animals with low milk production potential. Likewise, cows in early stage of lactation require more water as compared to those in mid or late lactation. Physiological stress such as sickness may lead to reduced water intake. Newly acquired animals may show low water intake simply because they are not accustomed to the new environment or due to differences in the taste of water (Lardy et al., 2008). Diets with increased salt or protein concentrations such as concentrate feed trigger increased water intake by increasing the frequency of urination and urine volume of urine, both of which are needed to excrete the extra salt and urea. Cows fed on total mixed rations were found to drink more water than those depending on pastures (Jago et al., 2005). The higher the feed intake, the higher the amount of water intake required for proper digestion.
Despite the importance of water to dairy production, sub-Saharan Africa faces several water challenges that mainly include shortage and seasonality in supply, poor quality, and long distances to watering points. A baseline survey on dairy farming systems was carried out by the African Asian Dairy Genetic Gains (AADGG) project of the International Livestock Research Institute (ILRI) in Ethiopia and Tanzania (https://portal.adgg.ilri.org/). It found that seasonality in supply (44%), poor water quality (34%), and long distances to the water source (22%) were the most common water constraints experienced by farmers in Ethiopia (Figure 2). In Tanzania, long distances to the water points (49%), seasonality in supply (28%), and poor water quality (23%) were the most common challenges.
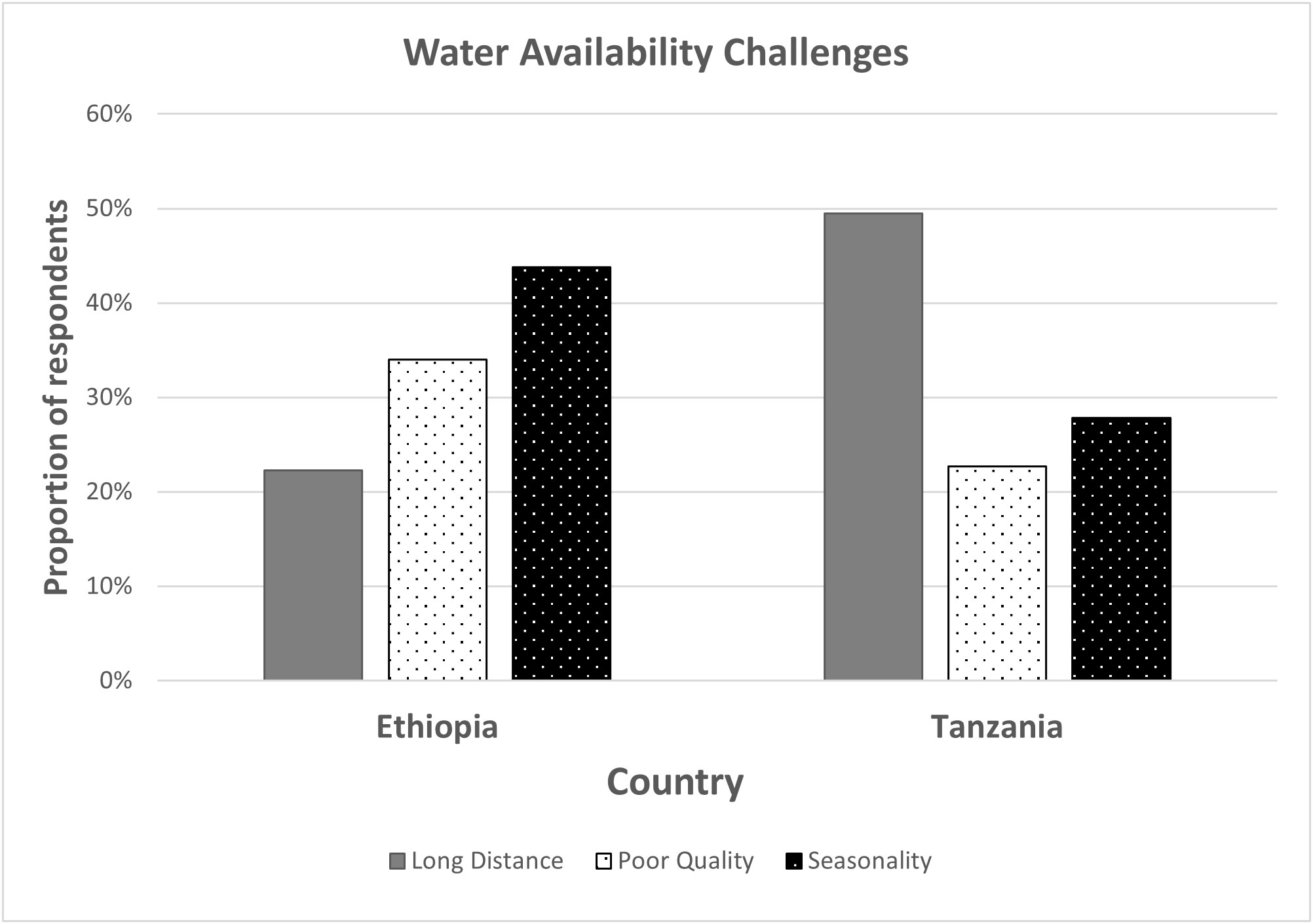
Figure 2 Main challenges affecting water availability as reported by farmers registered under the African Dairy Genetic Gains (ADGG) project of the International Livestock Research Institute (ILRI).
In SSA, shortages of clean water supply limit the development and adoption of technologies in the mixed crop-livestock systems. Farmers in this region depend on boreholes and wells, rivers, seasonal streams, and rainfall for water for domestic use and farming activities. Irrigation schemes are only practiced by large-scale and capital-intensive mechanized farms. A bigger section of sub-Saharan Africa experiences low, unreliable, and seasonal rainfall patterns on a yearly basis. This scarcity and seasonality of rainfall reduces the flow and amount of water in the rivers, causing a general shortage in the supply of water. Low rainfall results in long periods of droughts that deprive the nature of its ability to supply the nutritional needs of humans and animals. It causes a scarcity of vegetative cover, which leads to animal feed unavailability. For example, it is common practice for the pastoral communities in the northern part of Kenya to engage in deadly fights for pasture grounds and water sources for their herds during the dry season (Mkutu, 2004; Schilling et al., 2012). Owing to poor infrastructure, limited investments are made to harvest and preserve water during the rainy seasons. In most smallholder systems of SSA, the amount of water provided to dairy cattle is normally rationed depending on availability, thus severely limiting the production potential of the animals (Ojango et al., 2017).
Animals also move for long distance in search of drinking water, thus predisposing them to diseases such as lameness and Foot and Mouth disease which easily spread because of mixing with animals from different places at the water source (Fèvre et al., 2006). It also affects the growth and milk production of the cows as a greater portion of energy is expended in walking, making it difficult to meet the maintenance energy requirement for growth and milk production (McLennan et al., 2017; Fust and Schlecht, 2018). During the dry season, it is hard to recover the energy lost in walking through feeding as the feed quality normally declines. Moreover, cattle need to drink about 3 to 4 litres of water for every kilogram of feed dry matter ingested. While fresh grasses in the rainy season supply much of this needed water given their high moisture content (Turner and Schlecht, 2019), enough water intake is imperative in dry seasons to help the animal digest the dry fibrous forage. Failure to meet the demand for water intake during this season would greatly impair animal health and nutrition. The movement of animals in search of water also reduces feed intake. Time spent by animals while walking for long distances to get water diminishes the grazing time, especially when no or limited feed resources are available on their route. Besides, extended waiting times at water points reduce the time available for grazing.
Poor quality of water affects health, reproduction, growth, and production performance of animals. When the quality of water is compromised, lactating cows reduce their water intake, and consequently their milk production, thus resulting in significant economic losses (Van Eenige et al., 2013). Poor quality of drinking water can also reduce body growth indirectly by causing the animal to reduce its feed intake. Analyses by Willms et al. (2002) found that cattle drinking clean water had greater weight gains because they spent more time grazing than resting or standing. Poor-quality water can also serve as a reservoir for toxins and waterborne microorganisms. Stagnant water that is contaminated with manure and other nutrients may favor the proliferation of blue-green algae, which can cause poisoning to animals or even death. Some Leptospirosis-causing microorganisms, such as Leptospira species that are spread through the urine of carrier animals, can use stagnant water sources to spread from one animal to another (Pfost et al., 2001). The disease presents itself with reproductive problems such as abortions, infertility, and low milk production (Lilenbaum and Martins, 2014).
4.3 Diseases and disease pressure
A disease is a condition that alters the normal functioning of the animal’s body. Cattle can host many infectious agents, including bacteria, viruses, fungi, and a wide range of parasites such as ectoparasites, protozoa, and worms. Most of these pathogens cause disease in humans or are closely related to human pathogens. Bovine diseases cost the economy of sub-Saharan Africa billions of US$ per annum, promote food insecurity, and alter socioeconomic development (MacGregor et al., 2021). Examples of the main parasitic infections in cattle include animal African trypanosomiasis (AAT) caused by Trypanosoma spp, and East Coast Fever (ECF) caused by Theileria parva, while an example of viral infection is the Foot and Mouth Disease (FMD), a highly contagious viral infection of cloven-hoofed animals including domesticated ruminants and swine and is caused by a virus belonging to the Aphthovirus genus, in the Picornaviridae family (Belsham, 1993).
Mastitis is the inflammation of the mammary glands and udder tissues as a result of either an immune response to a bacterial infection of the teat canal or physical injury of the udder of the cow (The Cattle Site, 2021). It is one of the most common production-related bacterial caused infections for cows in SSA and results in huge economic losses, especially in small-scale dairy systems (Chagunda et al., 2016). Two types of mastitis are distinguished: infectious/contagious mastitis is acquired by the transmission of the infectious bacteria from one cow to another, and environmental mastitis acquired from bacteria present in the environment of the animals. Major bacterial species that cause mastitis include Staphylococcus aureus, Escherichia coli, Streptococcus agalactiae, Streptococcus uberis, and Streptococcus dysgalactiae (Bradley and Green, 2005). Subclinical mastitis does not show any noticeable symptoms. Clinical mastitis presents with painful swelling, redness, and hardness of the udder, fever, loss of appetite, reduction in activity, and reduction in milk yield.
The climatic conditions of sub-Saharan Africa favor proliferation of diseases in various ways. High temperatures in this region increase the growth rate and multiplication of most parasites and pathogens that spend part of their life cycle outside their animal host (Harvell et al., 2002). It has been previously shown that the ability of some arthropod vectors to get and remain infected with viruses increases with an increase in global temperature (Wittmann and Baylis, 2000). This increases the rate at which these viral diseases spread from one host to another. High temperature also increases the feeding frequency of arthropod vectors (Thornton et al., 2009). Thus, warmer temperatures of SSA might increase the successful transmission of diseases by increasing the rate at which vectors acquire and transmit the infection by feeding from one host to another. The spread of some pathogens through their vectors is further aided by the speed and direction of wind, which can be high in some part of SSA (Thornton et al., 2009).
Inadequate management practices also contribute to the spread of diseases in this region. Intensification of livestock in a small piece of land leads to overcrowding and when not well-managed, increases the spread of disease from one animal to another. Failure to properly clean and regularly sanitize the cattle houses offers the infectious agents a chance to multiply and infect the animals. Grazing on communal pastures leads to the spread of bovine diseases across the cattle herds within that community. Because of poor disease prevention, detection, and monitoring knowledge and capacity (Machila et al., 2003; Hernández-Castellano et al., 2019), sick animal ends up spreading infectious diseases to other animals before the farmer notices the presence of the disease. In most cases, animals have to fight and recover from undetected diseases on their own without any medical intervention, which negatively impacts their welfare. For instance, the baseline survey of the AADGG project indicated that most farmers did not practice any disease-preventative measures, especially in Ethiopia (Table 1). Lack of quality and enough feed and fodder to supply the nutritional needs of the cattle causes them to be nutrient deficient and weakens their immune system. This increases susceptibility to new diseases, reduces the capacity to fight acquired infections, and increases the mortality rates in calves and mature cattle.

Table 1 Status of the practice of preventative measures of cattle diseases by Ethiopian and Tanzanian farmers registered under the African Dairy Genetic Gains project of the International Livestock Research Institute at the time they joined the project.
Due to increased human population growth and rate of urbanization in SSA, the natural areas are increasingly being encroached on and used for agricultural production. Forests are perceived as legitimate areas for grazing animals. Such encroachments result in increased interaction between livestock and wildlife and diseases being transmitted between wild and domesticated animals, hence increasing the incidence of zoonotic diseases among livestock and humans (Bengis et al., 2002).
5 Response of the animals to stressors in their environment
Animals must continually adapt to the changing nature of their environment during their lives. The major coping mechanism to the disturbances in the environment that the animals adopt can be classified as either proactive and reactive coping styles (Koolhaas et al., 1999; Colditz and Hine, 2016). The proactive coping style is characterized by increased behavioral activity, higher sensitivity of the dopaminergic reward system, and higher reactivity of the sympathetic autonomic nervous system (SAM) in a stressful situation. On the other hand, reactive coping is characterized by lower behavioral activity, such as low aggressivity and withdrawal, higher hypothalamic-pituitary-adrenal (HPA) axis, and elevated parasympathetic reactivity (Cooper, 2008). When animals are exposed to increasing levels of disturbance, their capacity to cope is reduced. They, then, invest much of their energies and efforts towards their survival, which often increases the cost of maintaining their physiological normal functionality (Kadzere et al., 2002; Roberts N, 2007).
Adaptation is broadly described as a change that reduces the physiological strain that is caused by a stressful component of the total environment on an individual animal. Two main forms of adaptation have been described: Physiological and Biological adaptation (Gaughan et al., 2009). Physiological/phenotypic adaptation is the ability of an animal to respond to acute or sudden environmental change behaviorally or metabolically. Using heat stress as an example, physiological adaptations include increased respiration rate for enhanced evaporative cooling, as well as higher pulse and heart rates. Animals also increase sweating to release excessive heat (Rashamol et al., 2018). Besides, animals spend much of their time standing and changing orientations to circumvent direct solar and ground radiation (Madhusoodan et al., 2019), and expose more surface area to convection thus, increasing evaporative heat loss (Berman, 2006; Allen et al., 2015). Milk production also decreases as cows prioritize survival over milk production. And during water shortages, animals adjust their feed intake to match water intake level for efficient digestion (Willms et al., 2002).
Genetic/biological adaptation involves cumulative evolutionary processes and results from cumulative genetic changes over generations and time, and through environmental stimulation and experiences during the lifetime of an animal. Animals that have evolved to survive in adverse conditions have generally high resistance to stress and longer lifespan, coupled with low metabolic rates, late maturation, smaller mature sizes, and a slower rate of development (Parsons, 1994; Hansen, 2004; Gaughan et al., 2009). This form of adaptation is influenced by genetic makeup, and it determines an animal’s ability to tolerate harsh conditions such as drought, high temperature, pests, and diseases (Tsegaye and Ebrahim, 2018).
Most of indigenous SSA’s cattle breeds have developed many adaptive traits that increase their survivability in prevailing and increasingly harsh conditions (Kim et al., 2017). Bos indicus breeds evolved to acquire genes for thermotolerance and they have a higher degree of heat tolerance than Bos taurus cattle and can perform equally in hot climates (Sejian et al., 2013). These breeds have heat proteins 70 (Hsp 70) that are expressed to protect the animals from heat shock (Naskar et al., 2012). The skin properties also differ among breeds. Zebu cattle have smooth and shiny hair coats that act to reduce heat absorption via radiation (Hutchinson and Brown, 1969). Many zebu breeds have hair of light color that helps them reflect much of solar radiation. Zebu cattle have more and larger sweat glands that are closer to the skin than taurine cattle (Dowling, 1955; Nay and Haymani, 1956; Hansen, 2004). Resistance to one or more pests and diseases is another form of genetic adaptation used by animals, especially in areas with high disease pressure. Zebu cattle tend to be more resistant to tick infestation than taurine cattle. For instance, Sahiwal cattle are resistant to parasitic infection of Theileria annulata and N’Dama cattle to Anaplasma marginale and Haemonchus contortus (Naskar et al., 2012). African breeds are well known for total (e.g., N’Dama) or some level (e.g., Orma Boran) of tolerance and resistance against trypanosomiasis (Mwai et al., 2015).
6 Resilience of dairy cattle to environmental disturbances
An animal’s degree of resilience to a disturbance is demonstrated by its capacity to be minimally affected by the perturbation or rapidly return to the state pertained before exposure to the disturbance (Berghof et al., 2019b). These disturbances are normally situation-specific, episodic, or sporadic and not permanent attributes of the environment (Colditz and Hine, 2016). Degree of resilience, therefore, compares the differences in the magnitudes of phenotypes associated with resilience among individuals after exposure to environmental challenges (Rutter, 2012) and is a measure of better adaptability or lower sensitivity to a challenging state of affairs. This means that, when exposed to a disturbance, the performance of a resilient animal need not be the same as when it is under no disturbance, but rather, the negative change in its performance would be relatively lower compared to less resilient individuals that are exposed to similar disturbances.
6.1 Significance of resilience of dairy cattle in SSA
Breeding for resilience of cattle genotypes in SSA would help increase production efficiency and profitability of dairy production. Resilient animals are generally healthier, more fertile, and have a longer productive life. Resilient dairy cattle, therefore, have lower veterinary and disease management costs, produce more from similar levels of inputs, and give higher total lifetime returns. A less-resilient herd, on the other hand, results in high production losses due to high mortality and morbidity rates and lower-than-optimal production yields. Extra resources are usually used to care for less resilient cows (Berghof et al., 2019b).
Improving the resilience of cattle to environmental stressors enables better animal welfare experiences. Dairy cattle in good welfare experience less or no disturbance and thus are likely to perform optimally. Besides benefits related to fertility and production, animals in good welfare attract better prices in the market and hence fetch more income for the farmer.
Degree of intrinsic resilience prepares the animals for the future unseen consequences of climate change and ensures future food security for human beings. Global climatic conditions have been predicted to become warmer, with the temperature rising by up to 4.8°C by the next century (IPCC 2014). With the world becoming warmer, shortages of water and forage (feed and fodder) available for dairy cattle as well as the emergence of novel diseases and resistant infectious agents are expected to rise. Continuous improvement of the resilience capacity of animals would ensure that animals are able to survive future adversaries and continue to supply dietary needs to the rising human population.
6.2 Indicators of resilience
6.2.1 Indicators of general resilience
These are indicators that capture more resilience to microenvironmental disturbances than macroenvironmental stressors and are based on fluctuations from the normal performance of the animal. They include variance of deviations, root mean square deviations, lag-1 autocorrelation of deviations, and skewness of deviations. These indicators have been shown to be genetically variable (Tables 2 and 3) hence can be used to select for resilience in livestock. They have been shortly described below.
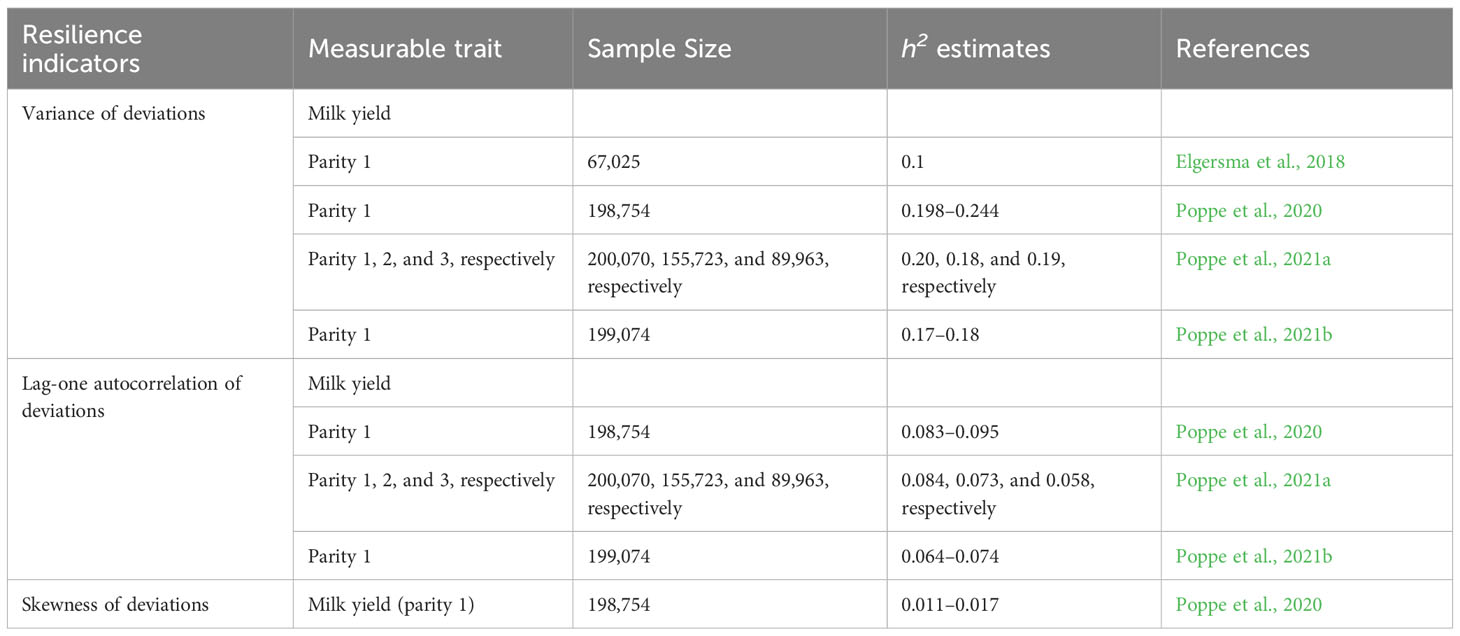
Table 2 Published heritability estimates of some of the indicators of resilience using different types of longitudinal data collected from cattle.
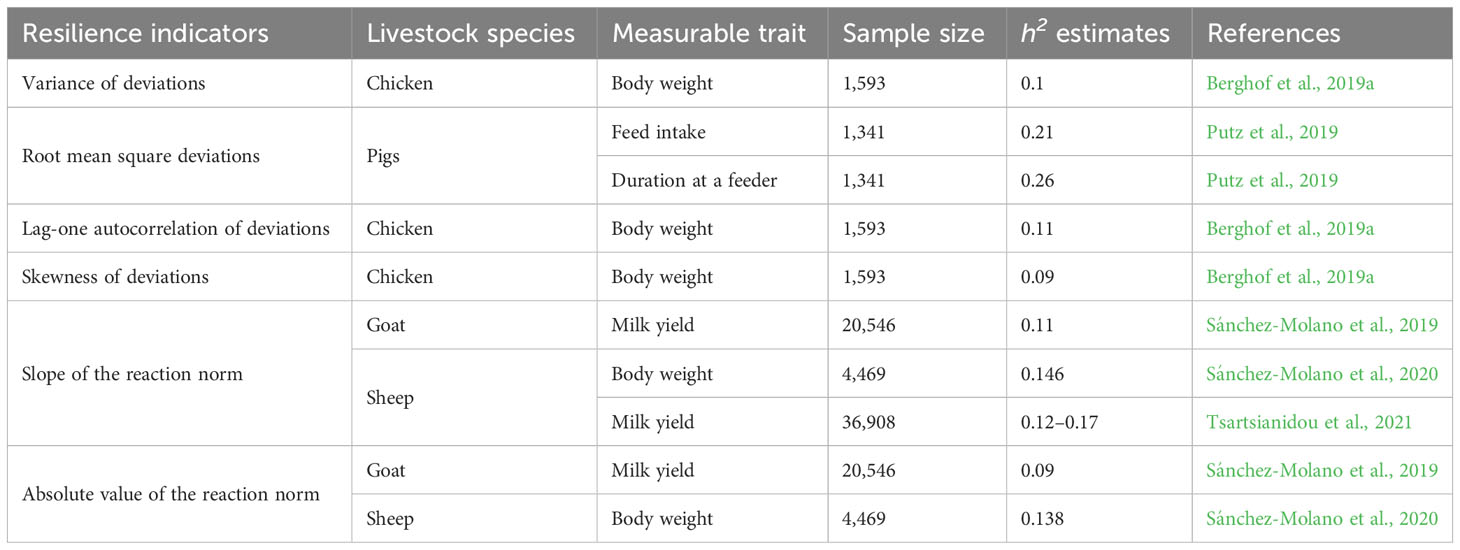
Table 3 Published heritability estimates of some of the indicators of resilience using different types of longitudinal data collected from other livestock species.
6.2.1.1 Variance of deviations
Variance of deviations indicates the impact of the disturbance on the performance of an individual animal. It is sometimes known as inherited variability, uniformity, environmental or residual variance (Berghof et al., 2019b). The biological functioning of resilient animals is less affected by disturbances in the environment. As such, resilient animals have a smaller range of deviation from their expected performance, hence a low variance of deviations. The performance of less resilient animals tends to be variable because they are more affected by the stressors in their environment, therefore, they have a higher variance of deviations (Berghof et al., 2019b). Most studies have reported significant negative genetic correlations between variance of deviation (derived from different types of longitudinal data) and fertility and fitness traits (Table 4). This points toward the possibility for utilizing this indicator to measure the general resilience of dairy animals.
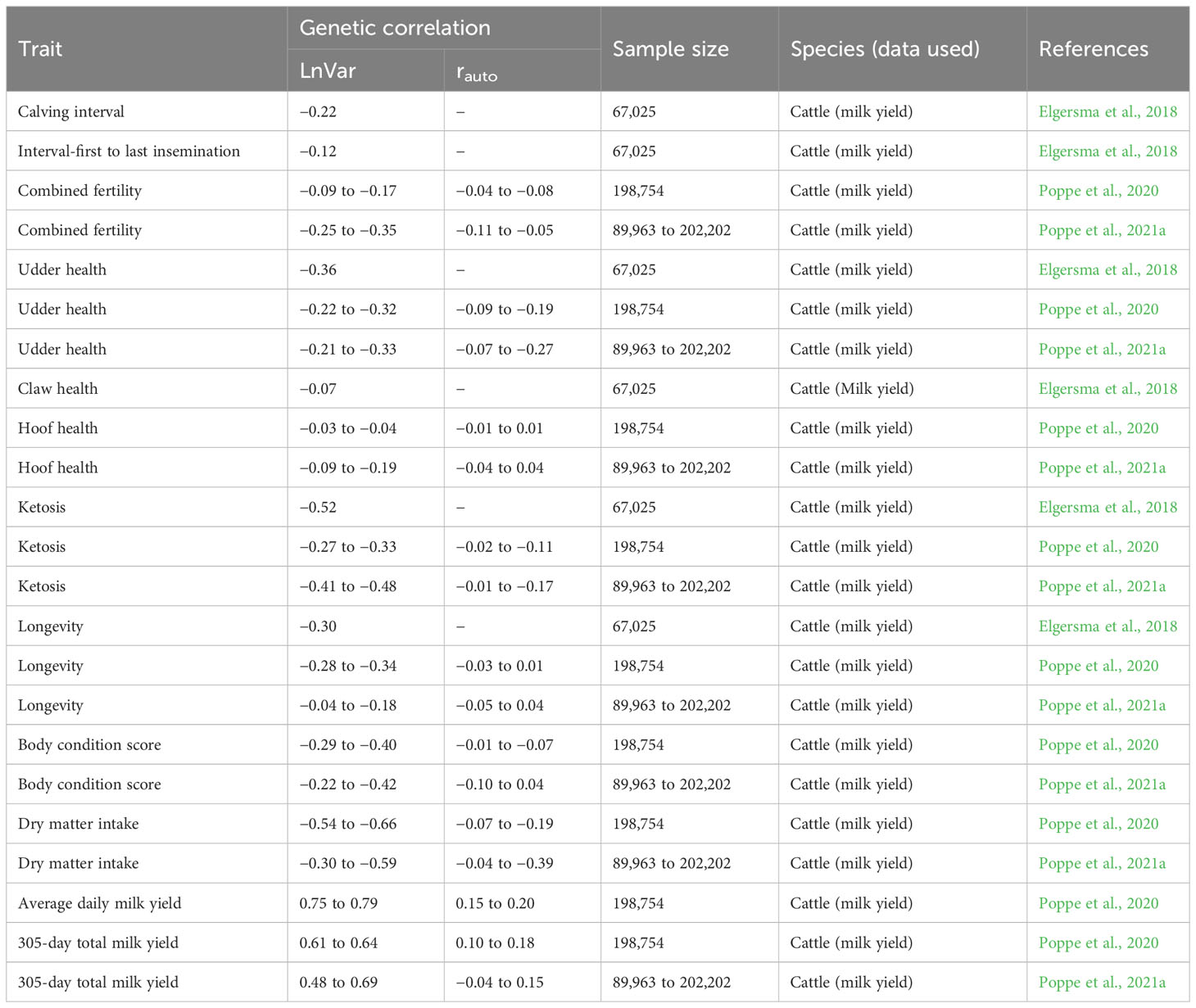
Table 4 Published genetic correlations of log-transformed variance (LnVar) and lag-1 autocorrelation (rauto) of deviations in cow milk yield with fertility, health, metabolic, and production traits.
6.2.1.2 Root mean square deviations
Just like variance of deviations, root mean square deviations (RMSD), or root mean square error (RSME), indicates the impact of disturbance on the performance of an individual animal. It is a square root of the raw variance of deviation. A larger RMSD value is expected from a less resilient animal and smaller values for resilient animals. Given the mathematical attribute of this indicator, a large number of records is needed in order to avoid erroneous grouping of animals with fewer records as more resilient (Putz et al., 2019). Although not on cattle, this indicator using feed intake and duration on pigs was found to have moderate heritability estimates (0.21 and 0.26, respectively) and to be favorably correlated with the number of treatments (0.56 and 0.62) and mortality (0.37 and 0.60) in a health-challenged environment (Putz et al., 2019).
6.2.1.3 Lag-1 autocorrelation of deviations
This indicator shows the duration of the impact of disturbance or the rate of recovery from the disturbance. The biological principle behind Lag-1 autocorrelation (rauto) is that a disturbance would mostly cause animals to deviate from their normal performance and animals would recover from the disturbance at different rates depending on their degree of resilience. Resilient animals are expected to recover faster from disturbances and thus tend to have shorter and fewer stretches of negative deviations than less resilient animals. As a result, the similarity between subsequent deviations is low. The opposite is the case for less resilient animals. An autocorrelation around one indicates that deviations are because of a similar stressor thus, an animal is influenced by the disturbance and has a slower rate of recovery from the disturbance. An autocorrelation around −1 indicates that the deviations are opposite; although an animal is affected by the perturbation, it has a quick and overcompensating response to the perturbation. Past studies have reported low but significant heritability estimates for Lag-1 autocorrelation on both cattle (Table 2) and other livestock species (Table 3) denoting that it contains information on genetic variation among the individuals in the population. Weak and negligible genetic correlations between health traits and rauto have been observed in past studies (Table 4).
6.2.1.4 Skewness of deviations
This indicates the direction of the deviation and captures the level of severity of the disturbance experienced by an individual animal. Less resilient animals are more influenced by disturbances and thus have more negative than positive deviations, which leads to a negative skewness around −1. Resilient animals have skewness around zero because they have almost equal numbers of negative and positive deviations. An animal that is responding positively to environmental improvement should show a positive skewness due to positive deviations. Past studies have reported low heritability estimates (Tables 2 and 3) and unexpected genetic correlations with fitness and health traits for skewness of deviations. Skewness of deviations around zero is expected to show good resilience. However, in a study by Poppe et al. (2020), it was observed that skewness of deviations around zero was genetically associated with a shorter productive life span, lower body condition score, and higher ketosis in dairy cattle. Berghof et al. (2019a) also could not predict mortality or lesion scores of chickens using estimated breeding values for skewness. For this reason, skewness might not be a promising indicator of resilience.
The indicators listed and discussed above having generally low to moderate heritabilities, most of them have moderate genetic correlations with fitness-related traits such as fertility, health, and longevity, signifying their importance in dairy production. Direct assessment of fertility, longevity, and health is expensive in terms of time, cost, and labor because it requires more and different datasets to be collected. These empirical indicators can easily be integrated into selection indices for dairy cattle in SSA, since they use the same data that are used to assess other performance traits, such as growth and production.
6.2.2 Indicators of specific resilience
These indicators capture resilience of animals to macroenvironmental disturbances. Macroenvironmental stressors are environmental disturbances such as heat stress, inadequate supply of water and feed, and disease pressure that affect the entire population. These indicators capture the severity of macroenvironmental disturbances and are specific to that type of disturbance. They measure how stable the animal performs in different intensities of a disturbance. Some of these indicators include:
6.2.2.1 Slope of the reaction norm
A reaction norm is the spectrum of phenotypic variation produced when individuals of the same genotype are exposed to varying environmental conditions. The slope of the reaction norm is the number that describes both the direction and the steepness of the reaction norm. It measures the phenotypic change in the performance of an individual animal in response to disturbances in the field (phenotypic plasticity). It indicates the severity of macro-environmental disturbance experienced by an individual animal.
Under the assumption that a stressor is reducing the trait values, animals that are not influenced by these disturbances are expected to have a positive slope or a slope of zero, whereas those affected by the disturbance should have slopes below zero. The steeper the negative slope, the more the animal is influenced by the respective disturbance, and the more the animal is less resilient (Berghof et al., 2019b). The slope of the reaction norm has a weak but significant (p<0.01) positive genetic correlation (0.04 to 0.05) with total lifetime milk yield in goats (Sánchez-Molano et al., 2019). This shows that animals with high milk production potential are more likely to have their production influenced by the changes in their environments.
6.2.2.2 Absolute value of the slope of reaction norm
Absolute value of the reaction norm is the distance of the slope of the reaction norm from zero. It indicates the stability or volatility of animal performance in relation to a disturbance in the environment. The closer the absolute value to zero, the more stable and resilient the animal is. Previous studies showed that the absolute value of the reaction norm has a moderate genetic correlation with total lifetime milk yield (0.46) in dairy goats (Sánchez-Molano et al., 2019) and a weak negative correlation (−0.152) with weight gain in sheep (Sánchez-Molano et al., 2020). This implies that resilient animals do not allocate resources to the production of milk at the expense of their health and welfare. Resilient animals (with low absolute values of the reaction norm) have also higher average weight gain than less resilient animals.
7 Adapting resilience in dairy systems of SSA
Availability of large volumes of performance data on the animals is critical for quantification and genetic improvement of resilience to environmental disturbances. Nevertheless, routine collection of data is a major drawback in livestock production systems in SSA. Some of the reasons put forward for the lack of data include poor infrastructure, unsupportive policies, unavailability of data recording organizations to support the farmers, small herds, limited knowledge among farmers and institutions on recording and processing of data as well as feedback generation, and inadequate mobilization and allocation of resources to support farmers (Ojango et al., 2017; Ojango et al., 2022).
Perhaps the resources being allocated to improve resilience in a trait-by-trait manner could be channeled to the frequent collection of quality longitudinal data, such as milk yield, growth traits, and activity patterns. Improved data quality and quantity would not only help to assess resilience but also improve production performance. Projects such as African and Asian Dairy Genetic Gains (AADGG, https://portal.adgg.ilri.org/) program have come up with innovative digital recording and farmer education systems and solutions to help address these situations. AADGG program led by ILRI is funded by the Bill & Melinda Gates Foundation (BMGF) and is an integral part of the CGIAR Initiative on Sustainable Animal Productivity for Livelihoods, Nutrition and Gender Inclusion (SAPLING). It is capturing and managing large volumes of performance data from both small- and large-scale farmers in eastern Africa and is pulling related weather data to derive indicative resilience traits. Future efforts aim to capture resilience related traits using various sensor-based technologies more directly. Over time, accumulated data can be used in genetic evaluations. Another ILRI-led BMGF funded project, EnviroCow, is developing phenotyping tools as well as genetic selection tools in SSA for novel animal traits such as methane emission intensity, feed requirements for maintenance and milk production, calf survival rate, and stability of milk. These projects aim to grant small-scale farmers in SSA access to more productive, feed-efficient, and resilient cattle genotypes, ultimately increasing their income levels.
7.1 Examples of phenotypes related to resilience
These are phenotypes that can be utilized to quantify resilience of the animals either directly or by analyzing them using one of the methods named above. The phenotypes to be used to quantify resilience in SSA should be easy, and cost and labor effective to measure. The tools used to collect these phenotypes should be easily available to make their use scalable. Some of the phenotypes that meet such criteria include body energy-related traits, physical activity patterns, and milk production profiles.
7.1.1 Body energy-related traits
These include body weight and related body linear measurements such as heart girth and body condition at a specific stage of life. They can indirectly inform about body fat mobilization, dry matter intake, and feed efficiency. Lactating cows mobilize their body fats to support milk production and other metabolic energy requirement deficiencies. When the energy obtained from dry matter intake is not enough, the animal is likely to catabolize some of its energy reserves to compensate for the deficit. These phenotypes include body weight and body condition score of animals.
7.1.1.1 Body weight
The weight of the animal assesses the growth rate of the animals and determines the feed requirements and the response of animals to changes in their environment (Lukuyu et al., 2016). The most globally accepted and accurate method of measuring weight is the use of a calibrated mechanical or electronic scale. However, this method is expensive and not readily available in sub-Saharan Africa, especially in smallholder dairy systems. Estimation of body weight from the visual assessment is always subjective and is associated with a lot of errors (Machila et al., 2008; Rahal et al., 2017; Mehdid et al., 2018). Therefore, the use of inexpensive, direct measuring tapes to measure the heart girth and body length for estimation of live weight is currently the most reliable method in SSA. Even though the body weights of animals vary, changes from the ideal weight can be utilized to inform about the resilience of the animals. Previous studies have shown genetic variation in fluctuations of body weight in different livestock species (Berghof et al., 2019a; Sánchez-Molano et al., 2020).
7.1.1.2 Body condition score
Body condition score (BCS) is used to estimate the level of mobilization of body fat reserves. Various scoring procedures, ranging from a visual and tactile assessment of the fat reserves on the back and pelvic region of the animals, to the use of photographic mobile applications are applied. Animals have different levels of body fat mobilization depending on their genetics, health status, climatic condition, lactation stage, and level of farm management. Therefore, BCS varies with animal and time of assessment. Scoring of body condition can be used to provide information on the well-being, nutrition, production, and reproductive performance, hence the robustness of dairy herd (Bewley and Schutz, 2008; Kellogg, 2010; Heinrichs et al., 2016). Deviations from ideal BCS negatively affect the production, reproduction, and health status of dairy cows (Garnsworthy and Topps, 1982).
There is no single recommended BCS that applies across all stages of lactation as the energy requirement varies with stages of lactation. An ideal BCS at a given stage of lactation is that which optimizes milk production and reproductive performance and minimizes health disorders, thereby ensuring optimal profitability (Bayram et al., 2012). Dairy cows maintaining an ideal body condition score curve throughout the lactation, dry, and transition period are likely to have better reproductive performance and lower occurrence of disease (Roche et al., 2009; Gomez et al., 2018). From different recommendations in the literature, an ideal body condition of cows on a scale of 1–5 should range between 2.5 to 3.75 with the lowest point (nadir BCS) witnessed at the early stage of the lactation (Table 5 and Figure 3).
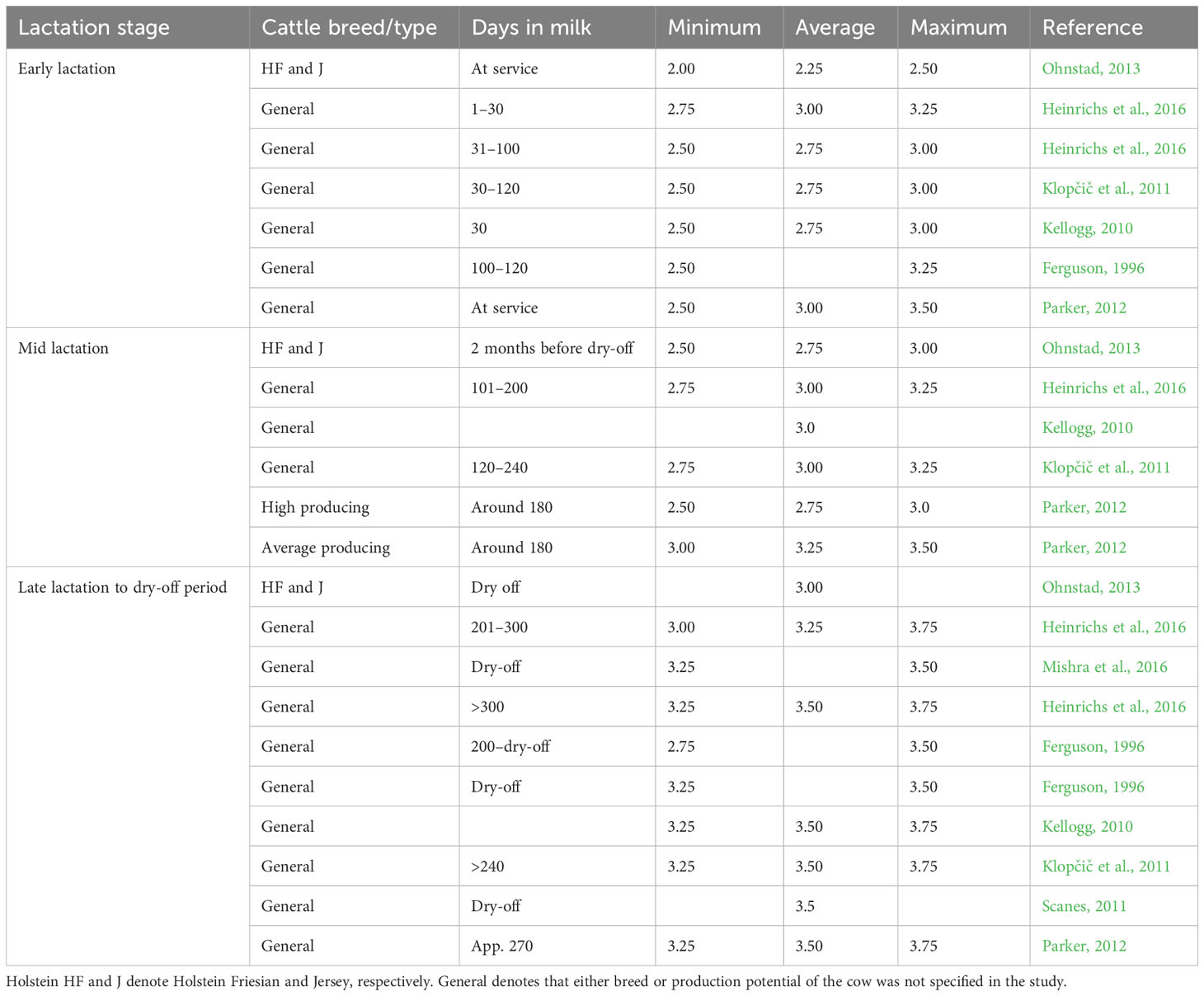
Table 5 Recommended body condition score for dairy cows at different stages of lactation generated from published literature on a 1–5 scale.
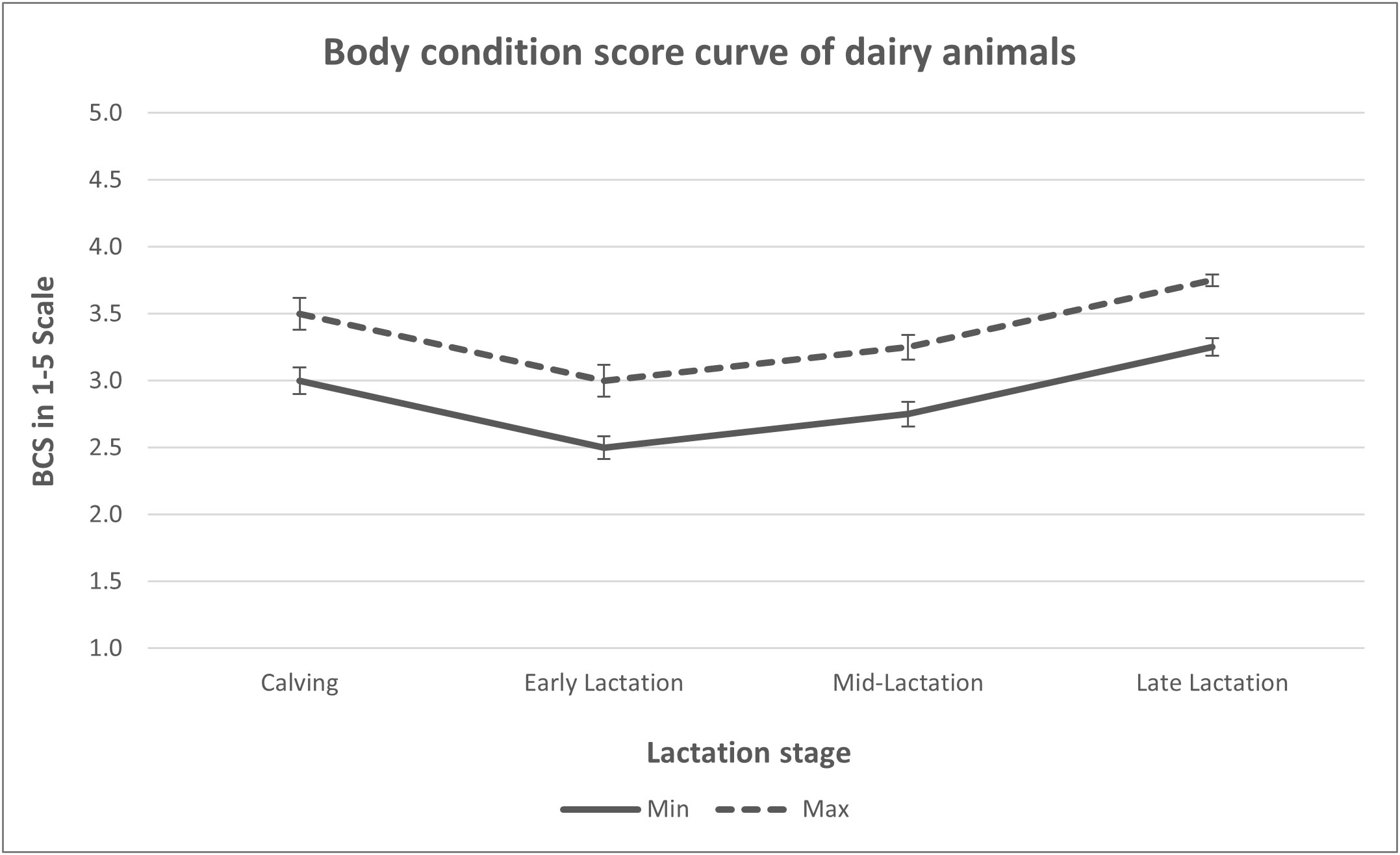
Figure 3 Ideal body condition curve of dairy cattle generated from various BCS recommendations in the literature.
Despite the potential of BCS in assessing the robustness of dairy herds, its assessment through visual observation is subjective as it is affected by the training and experience of the evaluator. The score an animal gets would still vary among the experienced evaluators and could be influenced by previously observed cows (Bercovich et al., 2013). Advances in technology have led to development and wide use of smartphone applications with photographic sensors in developed countries to directly score body condition of animals. Nonetheless, care should be taken before using such applications to score animals in sub-Saharan Africa as the body conformation of zebu and their crosses are different from that of taurine cattle and their crosses.
7.1.2 Animal activity patterns
The activity patterns of animals differ depending on individual, management practices, and regional differences (Ito et al., 2014; Krawczel, 2014). Changes in activity patterns can help in detecting health and welfare issues of animals (EIP-AGRI, 2018). Using activity pattern data, the resilience of animals can be estimated based on the average daily measurement after adjusting for encountered fixed effects. Depending on the required measurement, whether more or less, animals that perform below or above the population average could be more resilient. Another way of quantifying resilience would be based on the fluctuations from their normal activity pattern. Animals adjust their activity patterns, such as lying, mobility, and feeding behavior, in response to the stressors in their environment. The ways and forms of these adjustments can be used to define resilience indicators to estimate degree of resilience of animals to these disturbances. Resilient animals are expected to have limited deviations from their normal activity patterns. Precision livestock farming (PLF) sensor-based technology has allowed data on the physical activities of the animals to be easily collected using activity meters and analyzed. Some of the activity data includes but not limited to lying, standing, and stepping behaviors.
Lying behavior includes total lying time and the number of lying bouts. Generally, cows spend from 4 to 19.5 hours lying with 1 to 28 lying bouts per day (Ito et al., 2009). Limited lying is associated with low productivity and poor welfare, whereas more than usual lying behavior could be an indicator of health issues. Animals stay in a standing posture when feeding, drinking water, socializing, being milked, or moving from one point to another. Spending more than 11 hours per day standing could be a sign of heat stress and might increase the risk of lameness and decreased standing time could be an indicator of physical injuries, lameness, and other sicknesses (Temple et al., 2016). Animals move around in search of feed, mates, and resting areas as well as to the milking parlors. The stepping behavior during grazing could inform about feed efficiency and grazing type (Gregorini et al., 2015). A higher step count confers physical health benefits but is a risk factor for lameness. A lower than usual step count could indicate a health disorder.
Mobility scoring is used to assess lameness. Industry-standard four-point mobility scoring on a scale from 0 to 3 is the commonly used scale with 0 signifying sound/good mobility and 3 indicating severely impaired mobility (Whay et al., 2003). Lameness leads to low milk production, poor reproductive performance, compromised animal welfare, and an increased risk of premature culling (Archer et al., 2010). It is also associated with physical injury and different kinds of clinical diseases (Murray et al., 1996). The incidence of lameness is influenced by the genetics of the animal (such as temperament and body conformation), management practices, and geographical region. The animals with constant high mobility scores are deemed to be less resilient as their movement in search of feed and water is impaired, thus requiring extra labor to feed.
7.1.3 Milk production traits
These include milk yield, milk chemical composition, especially fat and protein content, and somatic cells. Disturbances in the environment such as diseases and harsh climatic conditions are expected to cause a decrease in milk yield. Less resilient animals are highly affected by the perturbations and will deviate greatly from their expected milk production levels. For instance, animals that are greatly affected by heat stress will reduce their feed intake and consequently produce less milk yield and of lower quality than anticipated. Therefore, deviations in milk yield from the expected lactation curve of the animal can inform the resilience of the animals. Indeed, studies have already used the fluctuation in the milk yield to indicate resilience of the animals (Sánchez-Molano et al., 2019; Poppe et al., 2020; Poppe et al., 2021a; Poppe et al., 2021b; Tsartsianidou et al., 2021).
Variability of fat and protein content of the milk measured on test days has also been shown to have a genetic variance (Ehsaninia et al., 2019). As an example, variations in fat content may indicate resilience to rumen acidosis or ketosis. It would be interesting though, to use more frequent records of fats and protein content to define resilience indicators and estimate genetic correlations with health and fitness traits.
Somatic cell count (SCC) is the count of cells in a millilitre (mL) of milk sample. Its log-transformed form is called somatic cell score (SCS). SCC in the milk is used to indicate the status of udder health and mastitis infection. Generally, a healthy cow is expected to have up to 100,000 somatic cells per 1 mL of milk. A somatic cell count above 200,000 cells/mL is considered an indicator of mastitis infection (El-Tahawy and El-Far, 2010; Cinar et al., 2015). Variations in somatic cell scores could be used as an indication of resilience to mastitis (De Haas et al., 2008; Urioste et al., 2012). A resilient animal is expected to have few incidences and frequencies of mastitis infection or a high recovery rate from the infection.
Light and portable milk analyzers are commercially available and can be used to quickly measure milk chemical composition and SCC directly from the field. This reduces the cost, time, and labor needed for laboratory analysis.
7.2 Broad-based selection objective
While individual traits are important components of resilience, they capture different aspects of resilience and might be having different genetic background (Berghof et al., 2021b, Poppe et al., 2021a). Thus, the potential for applying different resilience indicators to measure resilience of animals in SSA needs to be tested and those indicators with substantial genetic variability be appropriately combined into a composite resilience measure that can be used to inform selection for resilience.
The long-term goal of dairy production in SSA should aim at producing robust and resilient animals, with the ability to express their production potential in a wide range of, and varying environmental conditions without compromising their reproduction, health, and wellbeing (Knap, 2005; Colditz and Hine, 2016). A broad-based breeding objective that incorporates a range of traits on production, health, reproduction, efficiency, and adaptation is fundamental in SSA. Such objectives have a commonplace for dairy cattle in developed countries (Miglior et al., 2005) but limited in SSA. Currently, the selection objective for dairy cattle for Tanzania implemented by AADGG accounts for productivity and efficiency for body maintenance through the use of breeding values for milk yield and body weight (Mrode et al., 2021) but further research to incorporate fertility, milk quality and reproductive traits is under way.
8 Conclusions
Breeding dairy cattle for improved productivity and resilience can be implemented in SSA using different indicators and phenotypes that quantify the degree of resilience. Indicators proposed are broadly categorized into two; those that capture resilience to microenvironmental disturbances (general resilience) and those that measure resilience to macro-environmental disturbances (specific resilience). All the indicators have genetic variation and heritability estimates that are significant from zero and hence can be used to select for resilience. In addition, easier to measure phenotypes that are routinely collected using available tools, including body weight, body condition score, milk chemical composition and somatic cell count, and animal activity patterns, offer opportunities for characterizing the resilience of dairy cattle in SSA. However, using these indicators and phenotypes to quantify resilience requires that a large volume of related data be collected on animals and appropriately analyzed. Appropriate application of emerging genomic tools might allow for improvement for both resilience and production and simultaneously address the problem of poor adaptability and low production of dairy cattle in SSA. There is, therefore, a need to develop broad-based selection objectives that incorporate a wide range of traits on production, health, reproduction, efficiency, and adaptation to effectively improve resilience of dairy cattle. Whereas, breeding for resilience might help to improve adaptability and dairy productivity, farmers need to be continuously trained on feed management, good animal husbandry and management practices. This will ultimately ensure that dairy cattle have conducive environment to optimally express their production potential.
Author contributions
RO: Conceptualization, Data curation, Investigation, Validation, Visualization, Writing – original draft, Writing – review & editing. JO: Data curation, Validation, Writing – review & editing. CE-D: Data curation, Validation, Writing – review & editing. GG: Data curation, Writing – review & editing. RM: Data curation, Resources, Supervision, Writing – review & editing, Conceptualization. OM: Conceptualization, Data curation, Funding acquisition, Resources, Supervision, Writing – review & editing. MC: Conceptualization, Funding acquisition, Resources, Supervision, Writing – review & editing.
Acknowledgments
The authors acknowledge financial support from the Academy for International Agricultural Research (ACINAR) and African Asian Dairy Genetic Gains (AADGG) projects. ACINAR work is commissioned by the German Federal Ministry for Economic Cooperation and Development (BMZ) and carried out by the Council for Tropical and Subtropical Agricultural Research (ATSAF e.V) on behalf of the Deutsche Gesellschaft für Internationale Zusammenarbeit (GIZ) GmbH. AADGG project is led by the International Livestock Research Institute (ILRI) and funded by Bill and Melinda Gates Foundation (BMGF). This work was conducted as part of the CGIAR Research Initiative on Sustainable Animal Productivity for Livelihoods, Nutrition, and Gender Inclusion (SAPLING). CGIAR research is supported by contributors to the CGIAR Trust Fund. CGIAR is a global research partnership for a food-secure future dedicated to transforming food, land, and water systems in a climate crisis.
Conflict of interest
The authors declare that the research was conducted in the absence of any commercial or financial relationships that could be construed as a potential conflict of interest.
Publisher’s note
All claims expressed in this article are solely those of the authors and do not necessarily represent those of their affiliated organizations, or those of the publisher, the editors and the reviewers. Any product that may be evaluated in this article, or claim that may be made by its manufacturer, is not guaranteed or endorsed by the publisher.
References
Alexandratos N., Bruinsma J. (2012). World agriculture towards 2030/2050: the 2012 revision (Rome: ESA).
Allen J. D., Hall L. W., Collier R. J., Smith J. F., et al. (2015). Effect of core body temperature, time of day, and climate conditions on behavioral patterns of lactating dairy cows experiencing mild to moderate heat stress. J. Dairy Sci. 98 (1). doi: 10.3168/jds.2013-7704
Aman G., Tadele M., Tesfaye K. (2021). Traditional cattle breeding practices and rate of inbreeding in the mid rift valley of Oromia, Ethiopia. Int. J. Veterinary Sci. Res. 7, 077–082. doi: 10.17352/ijvsr.000084
Angel S. P., Amitha J. P., Rashamol V. P., Vandana G. D., Savitha S. T. (2018). Climate change and cattle production: impact and adaptation. J. Veterinary Med. Res. 5 (4), 1134. doi: 10.47739/2378-931X/1134
Archer S. C., Green M. J., Huxley J. N. (2010). Association between milk yield and serial locomotion score assessments in UK dairy cows. J. Dairy Sci. 93 (9). doi: 10.3168/jds.2010-3062
Arhem K. (1989). Maasai food symbolism: the cultural connotations of milk, meat, and blood in the pastoral maasai diet. Anthropos 84 (3), 1–23. doi: 10.2307/40461671
Baumgard L. H., Wheelock J. B., O’Brien M., Shwartz G., Zimbelman R. B., Sanders S. R., et al. (2007). “The differential effects of heat stress vs. underfeeding on production and post-absorptive nutrient partitioning,” in 22nd Annual Southwest Nutrition & Management Conference.
Baumgard L. H., Rhoads R. P. (2013). Effects of heat stress on postabsorptive metabolism and energetics. Annu. Rev. Anim. Biosci. 1, 311–337. doi: 10.1146/annurev-animal-031412-103644
Bayram B., Aksakal V., Akbulut O. (2012). Effect of the body condition score on some reproduction and milk yield traits of Swedish red and white cows. J. Anim. Plant Sci. 22 (3), 545–551.
Belsham G. J. (1993). Distinctive features of foot-and-mouth disease virus, a member of the picornavirus family; aspects of virus protein synthesis, protein processing and structure. Prog. Biophys. Mol. Biol. doi: 10.1016/0079-6107(93)90016-D
Bengis R. G., Kock R. A., Fischer J. (2002). Infectious animal diseases: The wildlife/livestock interface. OIE Rev. Scientifique Technique 21 (1). doi: 10.20506/rst.21.1.1322
Bercovich A., Edan Y., Alchanatis V., Moallem U., Parmet Y., Honig H., et al. (2013). Development of an automatic cow body condition scoring using body shape signature and Fourier descriptors. J. Dairy Sci. 96 (12), 8047–8059. doi: 10.3168/jds.2013-6568
Berghof T. V. L., Bovenhuis H., Mulder H. A. (2019a). Body weight deviations as indicator for resilience in layer chickens. Front. Genet. 10 (December). doi: 10.3389/fgene.2019.01216
Berghof T. V. L., Poppe M., Mulder H. A. (2019b). Opportunities to improve resilience in animal breeding programs. Front. Genet. 10 (JAN). doi: 10.3389/fgene.2018.00692
Berman A. (2006). Extending the potential of evaporative cooling for heat-stress relief. J. Dairy Sci. 89 (10). doi: 10.3168/jds.S0022-0302(06)72423-7
Bewley J. M., Schutz M. M. (2008). An interdisciplinary review of body condition scoring for dairy cattle. Prof. Anim. Scientist 24 (6), 507–529. doi: 10.15232/s1080-7446(15)30901-3
Bohmanova J., Misztal I., Cole J. B. (2007). Temperature-humidity indices as indicators of milk production losses due to heat stress. J. Dairy Sci. 90 (4), 1947–1956. doi: 10.3168/jds.2006-513
Bradley A., Green M. (2005). Use and interpretation of somatic cell count data in dairy cows. In Pract. 27 (6), 310–315. doi: 10.1136/inpract.27.6.310
Britt J. H., Cushman R. A., Dechow C. D., Dobson H., Humblot P., Hutjens M. F., et al. (2018). Invited review: Learning from the future—A vision for dairy farms and cows in 2067. J. Dairy Sci. 101 (5), 3722–3741. doi: 10.3168/jds.2017-14025
Capucchio M. T., Colombino E., Martina T., Biagini D., Alborali L. G., Maisano A. M., et al. (2019). “The disturbed habitat and its effects on the animal population,” in Habitats of the world - biodiversity and threats. Eds. Musarella C. M., Ortiz A. C., Canas R. Q. (IntechOpen), 137–144. doi: 10.5772/intechopen.84872
Chagunda M. G. G., Mwangwela A., Mumba C., Dos Anjos F., Kawonga B. S., Hopkins R., et al. (2016). Assessing and managing intensification in smallholder dairy systems for food and nutrition security in Sub-Saharan Africa. Regional Environ. Change 16 (8), 2257–2267. doi: 10.1007/s10113-015-0829-7
Cinar M., Serbester U., Ceyhan A., Gorgulu M. (2015). Effect of somatic cell count on milk yield and composition of first and second lactation dairy cows. Ital. J. Anim. Sci. 14 (1), 105–108. doi: 10.4081/ijas.2015.3646
Cockram M. S. (2004). A review of behavioural and physiological responses of sheep to stressors to identify potential behavioural signs of distress. Anim. Welfare. 13 (3). doi: 10.1017/S0962728600028396
Colditz I. G., Hine B. C. (2016). Resilience in farm animals: Biology, management, breeding and implications for animal welfare. Anim. Production Sci. 56 (12), 1961–1983. doi: 10.1071/AN15297
Cooper S. J. (2008). From Claude Bernard to Walter Cannon. Emergence of the concept of homeostasis. Appetite. doi: 10.1016/j.appet.2008.06.005
De Haas Y., Ouweltjes W., Ten Napel J., Windig J. J., De Jong G. (2008). Alternative somatic cell count traits as mastitis indicators for genetic selection. J. Dairy Sci. 91 (6). doi: 10.3168/jds.2007-0459
De Rensis F., Scaramuzzi R. J. (2003). Heat stress and seasonal effects on reproduction in the dairy cow - A review. Theriogenology 60 (6). doi: 10.1016/S0093-691X(03)00126-2
Dowling D. F. (1955). The thickness of cattle skin. Aust. J. Agric. Res. 6 (5). doi: 10.1071/AR9550776
Duguma B., Janssens G. P. J. (2015). Assessment of dairy farmers’ Hygienic milking practices and awareness of cattle and milk-borne zoonoses in Jimma, Ethiopia. Food Sci. Qual. Manage. 45, 114–121.
Duplessis M., Cue R. I., Santschi D. E., Lefebvre D. M., Lacroix R. (2015). Weight, height, and relative-reliability indicators as a management tool for reducing age at first breeding and calving of dairy heifers. J. Dairy Sci. 98 (3), 2063–2073. doi: 10.3168/jds.2014-8279
Dürr J. (2013). “Animal identification, recording and evaluation–Interbull’s perspectives,” in ICAR technical series, vol. 15. , 127–133. Available at: http://www.icar.org/Documents/technical_series/tec_series_15_Santiago_Chile.pdf#page=143.
Ehsaninia J., Ghavi Hossein-Zadeh N., Shadparvar A. A. (2019). Estimation of genetic variation for macro- and micro-environmental sensitivities of milk yield and composition in Holstein cows using double hierarchical generalized linear models. J. Dairy Res. 86 (2), 145–153. doi: 10.1017/S0022029919000293
EIP-AGRI (2018) Robust and resilient dairy production systems. Available at: https://ec.europa.eu/eip/agriculture/sites/agri-eip/files/eip-agri_fg_robust_resilient_dairy_farming_final_report_2018_en.pdf.
Ekine-Dzivenu C., Mrode R., Oloo D., Komwihangilo D., Lyatuu E., Msuta G., et al. (2022). “Genomic analysis of milk yield and heat tolerance in small holder dairy system of sub-Saharan Africa,” in Proceedings, 12th World Congress of Genetics Applied to Livestock Production (WCGALP)(Rotterdam, The Netherlands), 1709–12. doi: 10.3920/978-90-8686-940-4_410
Ekine-Dzivenu C. C., Mrode R., Oyieng E., Komwihangilo D., Lyatuu E., Msuta G., et al. (2020). Evaluating the impact of heat stress as measured by temperature-humidity index (THI) on test-day milk yield of small holder dairy cattle in a Sub-Sahara African climate. Livestock Sci. 242 (January). doi: 10.1016/j.livsci.2020.104314
Elgersma G. G., Jong G., van der Linde R., Mulder H. A. (2018). Fluctuations in milk yield are heritable and can be used as a resilience indicator to breed healthy cows. J. Dairy Sci. 101 (2), 1240–1250. doi: 10.3168/jds.2017-13270
El-Tahawy A. S., El-Far A. H. (2010). Influences of somatic cell count on milk composition and dairy farm profitability. Int. J. Dairy Technol. 63 (3), 463–469. doi: 10.1111/j.1471-0307.2010.00597.x
FAOSTAT (2023). FAO Statistical Database. Available at: http://www.fao.org/faostat/en/#data/QCL (Accessed 17 May 2023).
Ferguson J. D. (1996). “Implementation of a Body Condition Scoring Program in Dairy Herds.” In Penn Conference.
Fèvre E. M., Bronsvoort BM, Hamilton KA, Cleaveland S. (2006). Animal movements and the spread of infectious diseases. Trends Microbiol. 14 (3), 125–131. doi: 10.1016/j.tim.2006.01.004
Fust P., Schlecht E. (2018). Integrating spatio-temporal variation in resource availability and herbivore movements into rangeland management: RaMDry—An agent-based model on livestock feeding ecology in a dynamic, heterogeneous, semi-arid environment. Ecol. Model. 369. doi: 10.1016/j.ecolmodel.2017.10.017
Galukande E., Mulindwa H., Wurzinger M., Roschinsky R., Mwai A. O., Sölkner J. (2013). Cross-breeding cattle for milk production in the tropics: achievements, challenges and opportunities. Anim. Genet. Resources/Ressources génétiques animales/Recursos genéticos animales 52, 111–125. doi: 10.1017/s2078633612000471
Gantner V., Mijić P., Kuterovac K., Barać Z., Potočnik K. (2015). Heat stress and milk production in the first parity Holsteins – threshold determination in Eastern Croatia. Poljoprivreda 21 (1), 97–100. doi: 10.18047/poljo.21.1.sup.22
Garnsworthy P. C., Topps J. H. (1982). The effect of body condition of dairy cows at calving on their food intake and performance when given complete diets. Anim. Production 35 (1). doi: 10.1017/S0003356100000878
Gaughan J., Lacetera N., Valtorta S. E., Khalifa H. H., Hahn L., Mader T. (2009). “Response of Domestic Animals to Climate Challenges,” in Biometeorology for Adaptation to Climate Variability and Change, 131–70. doi: 10.1007/978-1-4020-8921-3_7
Giri A., Bharti V. K., Kalia S., Arora A., Balaje S. S., Chaurasia O. P. (2020). A review on water quality and dairy cattle health: a special emphasis on high-altitude region. Appl. Water Sci. 10 (3), 1–16. doi: 10.1007/s13201-020-1160-0
Gomez N. A., Conley A. J., Robinson P. H. (2018). Effects of long-term, near-term, and real-time energy balance, and blood progesterone concentrations, on the pregnancy rate of contemporary dairy cows. Anim. Reprod. Sci. 189 (October 2017), 136–145. doi: 10.1016/j.anireprosci.2018.01.001
Gregorini P., Waghorn G. C., Kuhn-Sherlock B., Romera A. J., Macdonald K. A. (2015). Short communication: Grazing pattern of dairy cows that were selected for divergent residual feed intake as calves. J. Dairy Sci. 98 (9). doi: 10.3168/jds.2015-9614
Hansen P. J. (2004). Physiological and cellular adaptations of zebu cattle to thermal stress. Anim. Reprod. Sci. 82–83, 349–360. doi: 10.1016/j.anireprosci.2004.04.011
Harvell C. D., Mitchell C. E., Ward J. R., Altizer S., Dobson A. P., Ostfeld R. S., et al. (2002). Climate warming and disease risks for terrestrial and marine biota. Science. doi: 10.1126/science.1063699
Hatting M., Tavares C. D. J., Sharabi K, Rines A. K., Puigserver P. (2018). Insulin regulation of gluconeogenesis. Ann. New York Acad. Sci. doi: 10.1111/nyas.13435
Heinrichs J., Jones C. M., Ishler V. A. (2016). Body Condition Scoring as a Tool for Dairy Herd Management (Penn State Extension), 14. Available at: http://people.vetmed.wsu.edu/jmgay/courses/documents/363eng1.pdf%0Ahttps://extension.psu.edu/body-condition-scoring-as-a-tool-for-dairy-herd-management.
Hernández-Castellano L. E., Nally JE, Lindahl J., Wanapat M., Alhidary I. A., Fangueiro D., et al. (2019). Dairy science and health in the tropics: challenges and opportunities for the next decades. Trop. Anim. Health Production 51 (5), 1009–1017. doi: 10.1007/s11250-019-01866-6
Herrero M., Thronton P. K., Notenbaert A. M., Wood S., Msangi S., Freeman H. A., et al. (2010). Smart investments in sustainable food production: revisiting mixed crop-livestock systems. Science 327 (FEBRUARY), 822–825. doi: 10.1126/science.1183700
Hoffmann I., Besbes B., Battaglia D., Wagner H. (2012). “Capacity building in support of animal identification for recording and traceability: FAO’s multipurpose and global approach,” in Proceedings of the First OIE Global Conference on Identification and Traceability ‘From Farm to Fork. 140–145. Available at: http://www.fao.org/ag/AGAinfo/home/documents/2012_Traceability_as_utility_to_animal_Identification.pdf.
Holsinger V. H. (1997). “Physical and Chemical Properties of Lactose,” in Advanced Dairy Chemistry. (Boston, MA: Springer), vol. 3. doi: 10.1007/978-1-4757-4409-5_1
Hutchinson J. C., Brown G. D. (1969). Penetrance of cattle coats by radiation. J. Appl. Physiol. 26 (4). doi: 10.1152/jappl.1969.26.4.454
IPCC. (2014). Climate Change 2014 Synthesis Report. Edited by Field C. B., Barros V., Stocker T. F., Dahe Q.. Vol.9781107025. Cambridge: Cambridge University Press. https://www.cambridge.org/core/product/identifier/CBO9781139177245A012/type/book_part.
Ito K., Chapinal N., Weary D. M., Von Keyserlingk M. A. G. (2014). Associations between herd-level factors and lying behavior of freestall-housed dairy cows. J. Dairy Sci. 97 (4), 2081–2089. doi: 10.3168/jds.2013-6861
Ito K., Weary D. M., von Keyserlingk M. A. G. (2009). Lying behavior: Assessing within- and between- herd variation in free-stall-housed dairy cows. J. Dairy Sci. 92 (9). doi: 10.3168/jds.2009-2235
Jago J. G., Roche J. R., Kolver E. S, Woolford M. W. (2005). “The drinking behaviour of dairy cows in late lactation,” in Proceedings of the New Zealand Society of Animal Production 65, 209–214.
Kadzere C. T., Murphy M. R., Silanikove N., Maltz E. (2002). Heat stress in lactating dairy cows: A review. Livestock Production Sci. 77 (1). doi: 10.1016/S0301-6226(01)00330-X
Kellogg W. (2010) Body Condition Scoring with dairy Cattle (Agriculture and Natural Resources). Available at: https://www.uaex.edu/publications/PDF/FSA-4008.pdf (Accessed 26 August 2021).
Kim J., Hanotte O., Mwai O. A., Dessie T., Salim B., Diallo B., et al. (2017). The genome landscape of indigenous African cattle. Genome Biol. 18 (1), 1–14. doi: 10.1186/s13059-017-1153-y
Klopčič M., Hamoen A., Bewley J. (2011). Body Condition Scoring of Dairy Cows. Eds. Klopčič M., Kuipers A. (University of Ljubljana, Biotechnical Faculty, Department of Animal Science). Available at: http://www.holstein.si/PDF/bodyconditionofdairycows.pdf.
Knap P. W. (2005). Breeding robust pigs. Aust. J. Exp. Agric. 45 (7–8), 763–773. doi: 10.1071/EA05041
Koolhaas J. M., Korte S. M., De Boer S. F., Van Der Vegt B. J., Van Reenen C. G., Hopster H., et al. (1999). Coping styles in animals: Current status in behavior and stress- physiology. Neurosci. Biobehav. Rev. 23 (7), 925–935. doi: 10.1016/S0149-7634(99)00026-3
Krawczel P. (2014) The Importance of Lying Behavior in the Well-Being and Productivity of Dairy Cows. Available at: https://extension.tennessee.edu/publications/Documents/W386.pdf.
Krpálková L., Cabrera V. E., Vacek M., Štípková M., Stádník L., Crump P., et al. (2014). Effect of prepubertal and postpubertal growth and age at first calving on production and reproduction traits during the first 3 lactations in Holstein dairy cattle. J. Dairy Sci. 97 (5), 3017–3027. doi: 10.3168/jds.2013-7419
Lardy G., Stoltenow C., Johnson R. (2008). Livestock and Water (North Dakota: North Dakota State University) Vol. 954.
Le Cozler Y., Lollivier V., Lacasse P., Disenhaus C. (2008). Rearing strategy and optimizing first-calving targets in dairy heifers: A review. Animal. doi: 10.1017/S1751731108002498
Lilenbaum W., Martins G. (2014). Leptospirosis in cattle: A challenging scenario for the understanding of the epidemiology. Transboundary Emerging Dis. 61 (SUPPL1.), 63–68. doi: 10.1111/tbed.12233
Lukuyu M. N., Gibson J. P., Savage D. B., Duncan A. J., Mujibi F. D. N., Okeyo A. M. (2016). Use of body linear measurements to estimate liveweight of crossbred dairy cattle in smallholder farms in Kenya. SpringerPlus 5 (1), 1–14. doi: 10.1186/s40064-016-1698-3
Lukuyu M. N., Gibson J. P., Savage D. B., Rao E. J. O., Ndiwa N., Duncan A. J., et al. (2019). Farmers’ Perceptions of dairy cattle breeds, breeding and feeding strategies: A case of smallholder dairy farmers in Western Kenya. East Afr. Agric. Forestry J. 83 (4), 351–367. doi: 10.1080/00128325.2019.1659215
Lukuyu B. A., KItalyi A., Franzel S., Duncan A., Baltenweck I. (2009). Constraints and Options to Enhancing Production of High-Quality Feeds in Dairy Production in Kenya, Uganda and Rwanda. ICRAF Working Paper No. 95. (Nairobi, Kenya).
MacGregor P., Nene V., Nisbet E. R. R. (2021). Tackling protozoan parasites of cattle in sub-Saharan Africa. PloS Pathog. 17 (10), 1–7. doi: 10.1371/journal.ppat.1009955
Machila N., Wanyangu S. W., McDermott J., Welburn S. C., Maudlin I., Eisler M. C. (2003). Cattle owners’ perceptions of African bovine trypanosomiasis and its control in Busia and Kwale Districts of Kenya. Acta Tropica 86 (1), 25–34. doi: 10.1016/S0001-706X(02)00288-7
Machila N., Fèvre E. M., Maudlin I., Eisler M. C. (2008). Farmer estimation of live bodyweight of cattle: Implications for veterinary drug dosing in East Africa. Prev. Veterinary Med. 87 (3–4). doi: 10.1016/j.prevetmed.2008.06.001
Madhusoodan A. P., Sejian V., Rashamol V. P., Savitha S. T., Bagath M., Krishnan G., et al. (2019). Resilient capacity of cattle to environmental challenges – An updated review. J. Anim. Behav. Biometeorol. 7 (3), 104–118. doi: 10.31893/2318-1265jabb.v7n3p104-118
Marshall K., Gibson J. P., Mwai O., Mwacharo J. M., Haile A., Getachew T., et al. (2019). Livestock genomics for developing countries - African examples in practice. Front. Genet. 10. doi: 10.3389/fgene.2019.00297
McLennan S. R., Bolam M. J., Kidd J. F., Chandra K. A., Poppi D. P. (2017). Responses to various protein and energy supplements by steers fed low-quality tropical hay. 1. Comparison of response surfaces for young steers. Anim. Production Sci. 57 (3). doi: 10.1071/AN15659
Mehdid B., Benyarou M., Ameur Ameur A., Gaouar S. B. S. (2018). Contribution to the study of two local bovine breeds in wilaya of tlemcen: morphometric characterization and DNA biobank. Genet. Biodiver. J. 2 (1), 44–55. doi: 10.46325/gabj.v2i1.114
Miglior F., Muir B. L., Van Doormaal B. J. (2005). Selection indices in Holstein cattle of various countries. J. Dairy Sci. 88 (3), 1255–1263. doi: 10.3168/jds.S0022-0302(05)72792-2
Mishra S., Kumari K., Dubey A. (2016). Body condition scoring of dairy cattle: A review’, research & Reviews. J. Veternary Sci. 2 (1), 1–8.
Mkutu K. (2004). Pastoralism and conflict in the Horn of Africa (Bradford: African Peace Forum/Safeworld University of Bradford). Available at: http://kms1.isn.ethz.ch/serviceengine/Files/ISN/124889/ipublicationdocument_singledocument/ee10725a-716d-4ef9-9edd-5bf2eedcfd16/en/WebLaikipia.pdf.
Moll H. A. J., Staal S. J., Ibrahim M. N. M. (2007). Smallholder dairy production and markets: A comparison of production systems in Zambia, Kenya and Sri Lanka. Agric. Syst. 94 (2), 593–603. doi: 10.1016/j.agsy.2007.02.005
Mrode R., Ekine Dzivenu C, Marshall K, Chagunda MGG, Muasa BS, Ojango J, et al. (2020). Phenomics and its potential impact on livestock development in low-income countries: Innovative applications of emerging related digital technology. Anim. Front. 10 (2), 6–11. doi: 10.1093/af/vfaa002
Mrode R., Ojango J, Ekine-Dzivenu C, Aliloo H, Gibson J, Okeyo MA. (2021). Genomic prediction of crossbred dairy cattle in Tanzania: A route to productivity gains in smallholder dairy systems. J. Dairy Sci. 104 (11), 11779–11789. doi: 10.3168/jds.2020-20052
Murray R. D., Downham D. Y., Clarkson M. J., Faull W. B., Hughes J. W., Manson F. J., et al. (1996). Epidemiology of lameness in dairy cattle: Description and analysis of foot lesions. Veterinary Rec. 138 (24). doi: 10.1136/vr.138.24.586
Mwai O., Hanotte O., Kwon Jun Y., Cho S. (2015). - Invited review - African indigenous cattle: Unique genetic resources in a rapidly changing world. Asian-Australasian J. Anim. Sci. 28 (7), 911–921. doi: 10.5713/ajas.15.0002R
Mwanga G., Mujibi F. D. N., Yonah Z. O., Chagunda M. G. G. (2019). Multi-country investigation of factors influencing breeding decisions by smallholder dairy farmers in sub-Saharan Africa. Trop. Anim. Health Production 51 (2), 395–409. doi: 10.1007/s11250-018-1703-7
Nardone A., Lacetera N., Bernabucci U., Ronchi B. (1997). Composition of colostrum from dairy heifers exposed to high air temperatures during late pregnancy and the early postpartum period. J. Dairy Sci. 80 (5). doi: 10.3168/jds.S0022-0302(97)76005-3
Nardone A., Ronchi B., Lacetera N., Ranieri M. S., Bernabucci U. (2010). Effects of climate changes on animal production and sustainability of livestock systems. Livestock Sci. 130 (1–3), 57–69. doi: 10.1016/j.livsci.2010.02.011
Naskar S., Gowane A., Chopra G. R., Paswan C., Prince L .L. L. (2012). “Genetic adaptability of livestock to environmental stresses,” in Environmental Stress and Amelioration in Livestock Production (Berlin, Heidelberg: Springer) 9783642292, 317–378. doi: 10.1007/978-3-642-29205-7_13
National Research Council (1988). Designing Foods: Animal Product Options in the Marketplace (Washington, DC: The National Academies Press). doi: 10.17226/1036
Nay T., Haymani R. H. (1956). Sweat glands in zebu (Bos indicus l.) and european (b. taurus l.) cattle i. size of individual glands, the denseness of their population, and their depth below the skin surface. Aust. J. Agric. Res. 7 (5). doi: 10.1071/AR9560482
Nyameasem J. K., Reinsch T., Malisch U. F., Taube F. (2018). The potential of dairy production in sub-Saharan Africa. Article 1. 223–227.
O’Connor M. (2016). Heat Detection and Timing of Insemination for Cattle (The Pennsylvania State University), 20.
Ohnstad I. (2013). Body condition scoring in dairy cattle: monitoring health to improve milk yield and fertility. Livestock 18 (3), 70–73. doi: 10.12968/live.2013.18.3.70
Ojango J. M. K., Wasike C. B., Enahoro D. K., Okeyo A. M. (2016). Dairy production systems and the adoption of genetic and breeding technologies in Tanzania, Kenya, India and Nicaragua. Anim. Genet. Resources/Ressources génétiques animales/Recursos genéticos animales 59, 81–95. doi: 10.1017/s2078633616000096
Ojango J. M. K., Mrode R., Okeyo A. M., Rege J. E. O., Chagunda M. G. G., Kugonza D. R. (2017). “Improving smallholder dairy farming in Africa.” In Achieving Sustainable Production of Milk, 337–62. Sawston: Burleigh Dodds Science Publishing Limited. 337–362. doi: 10.19103/as.2016.0005.38
Ojango J. M. K., Mrode R., Rege J. E. O., Mujibi D., Strucken E. M., Gibson J., et al. (2019). Genetic evaluation of test-day milk yields from smallholder dairy production systems in Kenya using genomic relationships. J. Dairy Sci. 102 (6), 5266–5278. doi: 10.3168/jds.2018-15807
Ojango J. M. K., Okeyo A. M., Mrode R., Chinyere E., Gebreyohanes G., Meseret S., et al. (2022). “459. Bridging the Gap in Data from Smallholder Dairy Systems; Developing the Africa Dairy Genetic Gains (ADGG) Data Platform.” In Proceedings of 12th World Congress of Genetics Applied to Livestock Production (WCGALP). Wageningen Academic Publishers, 1909–1912. doi: 10.3920/978-90-8686-940-4_459
Oloo R. D., Ekine-Dzivenu C. C. C., Ojango J. M. K., Mrode R. A., Chagunda M. G. G., Okeyo M. A. (2022b). “Effects of Breed Exoticness, Agro-Ecological Zone and Their Interaction on Production and Fertility Traits of Multibreed Dairy Cattle in Kenya.” ILRI Research Report. Nairobi, Kenya: ILRI. Available at: https://hdl.handle.net/10568/118429.
Oloo R. D., Ekine-Dzivenu C. C., Ojango J. M. K., Mrode R., Mwai A. O., Chagunda M. G. G. (2022a). “415. Testing Phenotypes for Degree of Resilience Using Fluctuations in Milk Yield of Dairy Cows in Sub-Saharan Africa.” In Proceedings of 12th World Congress of Genetics Applied to Livestock Production (WCGALP), 1729–32. Wageningen Academic Publishers. doi: 10.3920/978-90-8686-940-4
Omer E. A. M., Addo S., Roessler R., Schäler J., Hinrichs D. (2021). Exploration of production conditions: a step towards the development of a community-based breeding program for Butana cattle. Trop. Anim. Health Production 53 (1). doi: 10.1007/s11250-020-02459-4
Ørskov E. B. (2005). Conferencia Silvopastoral systems : technical , environmental and socio-economic challenges Sistemas silvopastoriles : retos técnicos , ambientales y socioeconómicos. Pastos y Forrajes 28 (1), 5–9.
Parker R. (2012) Using Body Condition Scoring in Dairy Herd Management, Ontario Ministry of Agriculture, Food and Rural Affairs. Available at: http://www.omafra.gov.on.ca/english/livestock/dairy/facts/94-053.htm (Accessed 26 August 2021).
Parsons P. A. (1994). Habitats, stress, and evolutionary rates. J. Evolutionary Biol. 7 (3). doi: 10.1046/j.1420-9101.1994.7030387.x
Pfost D. L., Fulhage C. D., Casteel S. (2001) Water Quality for Livestock Drinking’, Environmental Quality. Available at: https://extension.missouri.edu/publications/eq381#:~:text=Water.
Plaizier J. C., Krause D. O., Gozho G. N., McBride B.W. (2008). Subacute ruminal acidosis in dairy cows: The physiological causes, incidence and consequences. Veterinary J. 176 (1). doi: 10.1016/j.tvjl.2007.12.016
Poole N. D., Chitundu M., Msoni R. (2013). Commercialisation: A meta-approach for agricultural development among smallholder farmers in Africa? Food Policy 41 (September 2007), 155–165. doi: 10.1016/j.foodpol.2013.05.010
Poppe M., Veerkamp R. F., van Pelt M. L., Mulder H. A. (2020). Exploration of variance, autocorrelation, and skewness of deviations from lactation curves as resilience indicators for breeding. J. Dairy Sci. 103 (2), 1667–1684. doi: 10.3168/jds.2019-17290
Poppe M., Bonekamp G., Pelt van M. L., Mulder H. A. (2021a). Genetic analysis of resilience indicators based on milk yield records in different lactations and at different lactation stages. J. Dairy Sci. 104 (2), 1967–1981. doi: 10.3168/jds.2020-19245
Poppe M., Mulder H. A., Veerkamp R. F. (2021b). Validation of resilience indicators by estimating genetic correlations among daughter groups and with yield responses to a heat wave and disturbances at herd level. J. Dairy Sci. 104 (7), 8094–8106. doi: 10.3168/jds.2020-19817
Putz A. M., Harding J. C.S. S., Dyck M. K., Fortin F., Plastow G. S., Dekkers J. C. M. M., et al. (2019). Novel resilience phenotypes using feed intake data from a natural disease challenge model in wean-to-finish pigs. Front. Genet. 10 (JAN). doi: 10.3389/fgene.2018.00660
Rahal O., Aissaoui C., Elmokhefi M’hamed, Sahraoui H., Ciani E., Gaouar S. B. S. (2017). A comprehensive characterization of guelmoise, a native cattle breed from eastern Algeria. Gabj 1 (1), 31–42. doi: 10.46325/gabj.v1i1.83
Rashamol V. P., Sejian V. (2018). Physiological adaptability of livestock to heat stress: an updated review. J. Anim. Behav. Biometeorol. 6 (3), 62–71. doi: 10.31893/2318-1265jabb.v6n3p62-71
Roberts N. (2007). Links between behavioural and physiological parameters during adaptation to stress. PhD Thesis, Armidale: University of New England. Available at: https://hdl.handle.net/1959.11/2494.
Roche J. R., Friggens N. C., Kay J. K., Fisher M. W., Stafford K. J., Berry D. P. (2009). Body condition score and its association with dairy cow productivity, health, and welfare. J. Dairy Sci. 92 (12), 5769–5801. doi: 10.3168/jds.2009-2431
Rutter M. (2012). “Resilience: Causal pathways and social ecology,” in The Social Ecology of Resilience: A Handbook of Theory and Practice. (New York, NY: Springer). doi: 10.1007/978-1-4614-0586-3_3
Sánchez-Molano E., Kapsona V. V., Ilska J. J., Desire S., Conington J., Mucha S., et al. (2019). Genetic analysis of novel phenotypes for farm animal resilience to weather variability. BMC Genet. 20 (1), 1–10. doi: 10.1186/s12863-019-0787-z
Sánchez-Molano E., Kapsona V. V., Oikonomou S., McLaren A., Lambe N., Conington J., et al. (2020). Breeding strategies for animal resilience to weather variation in meat sheep. BMC Genet. 21 (1), 1–11. doi: 10.1186/s12863-020-00924-5
Scanes C. (2011). “Section 2: Livestock Production,” in Fundamentals of Animal Science. (NY, USA: Delmar Cengage Learning ALL), 448. Available at: https://books.google.com/books?id=krVmxt9biJAC&pgis=1.
Schilling J., Opiyo F. E. O., Scheffran J. (2012). Raiding pastoral livelihoods: motives and effects of violent conflict in north-western Kenya. Pastoralism 2 (1), 1–16. doi: 10.1186/2041-7136-2-25
Schneider P. L., Beede D. K., Wilcox C. J. (1988). Nycterohemeral patterns of acid-base status, mineral concentrations and digestive function of lactating cows in natural or chamber heat stress environments. J. Anim. Sci. 66 (1). doi: 10.2527/jas1988.661112x
Sejian V., Naqvi S. M. K., Ezeji T., Lakritz J., Lal R. (2013). Environmental stress and amelioration in livestock production. Heidelberg: Springer Berlin. doi: 10.1007/978-3-642-29205-7
Stachowicz J., Umstätter C. (2021). Do we automatically detect health- or general welfare-related issues? A framework. Proc. R. Soc. B: Biol. Sci. 288 (1950). doi: 10.1098/rspb.2021.0190
Summer A., Lora I, Formaggioni P, Gottardo F. (2019). Impact of heat stress on milk and meat production. Anim. Front. 9 (1). doi: 10.1093/af/vfy026
Temple D., Bargo F., Mainau E., Ipharraguerre I., Manteca X. (2016). Lying Behaviour and Performances in Dairy Cattle-Practical Case (Cerdanyola del Vallès: Farm Animal Welfare Education Centre). Available at: www.fawec.org.
The Cattle Site (2021) Cattle Disease Guide. Available at: https://www.thecattlesite.com/diseaseinfo/ (Accessed 9 October 2021).
Thornton P. K., Steeg J., Notenbaert A., Herrero M. (2009). The impacts of climate change on livestock and livestock systems in developing countries: a review of what we know and what we need to know. Agric. Syst. 101. doi: 10.1016/j.agsy.2009.05.002
Tsartsianidou V., Kapsona V. V., Sánchez-Molano E., Basdagianni Z., Carabaño M. J., Chatziplis D., et al. (2021). Understanding the seasonality of performance resilience to climate volatility in Mediterranean dairy sheep. Sci. Rep. doi: 10.1038/s41598-021-81461-8
Tsegaye S., Ebrahim H. (2018). Benefits of farm animals genetic adaptation: A review. Eur. J. Exp. Biol. 08 (04), 3–5. doi: 10.21767/2248-9215.100063
Turner M. D., Schlecht E. (2019). Livestock mobility in sub-Saharan Africa: A critical review. Pastoralism 9 (1). doi: 10.1186/s13570-019-0150-z
Ungerfeld E. M. (2020). Metabolic hydrogen flows in rumen fermentation: principles and possibilities of interventions. Front. Microbiol. 11 (April). doi: 10.3389/fmicb.2020.00589
Urioste J. I., Franzén J. J., Windig J., Strandberg E. (2012). Genetic relationships among mastitis and alternative somatic cell count traits in the first 3 lactations of Swedish Holsteins. J. Dairy Sci. 95 (6). doi: 10.3168/jds.2011-4739
Uyeno Y., Sekiguchi Y., Tajima K., Takenaka A., Kurihara M., Kamagata Y. (2010). An rRNA-based analysis for evaluating the effect of heat stress on the rumen microbial composition of Holstein heifers. Anaerobe 16 (1), 27–33. doi: 10.1016/j.anaerobe.2009.04.006
Van Eenige M. J. E. M., Counotte G. H. M., Noordhuizen J. P. T. M. (2013). Drinking water for dairy cattle: Always a benefit or a microbiological risk? Tijdschrift Voor Diergeneeskunde 138 (2), 86–97.
Veissier I., Miele M. (2014). Animal welfare: Towards transdisciplinarity - The European experience. Anim. Production Sci. 54 (9), 1119–1129. doi: 10.1071/AN14330
von Holst D. (1998). “The Concept of Stress and Its Relevance for Animal Behavior,” in Advances in the Study of Behavior. doi: 10.1016/S0065-3454(08)60362-9
Wagner J. J., Engle T. E. (2021). INVITED REVIEW: Water consumption, and drinking behavior of beef cattle, and effects of water quality. Appl. Anim. Sci. 37 (4), 418–435. doi: 10.15232/aas.2021-02136
Wakchaure R., Ganguly S., Praveen P. K. (2015). Role of water in metamorphism. Rec. Adv. Acad. Sc. 1 (November), 53–56. doi: 10.1130/spe62-p505
West J. W. (2003). Effects of heat-stress on production in dairy cattle. J. Dairy Sci. 86. doi: 10.3168/jds.S0022-0302(03)73803-X
Whay H. R., Main D. C. J., Green L. E., Webster A. J. F. (2003). Assessment of the welfare of dairy cattle using animal-based measurements: Direct observations and investigation of farm records. Veterinary Rec. 153 (7). doi: 10.1136/vr.153.7.197
Willms W. D., Kenzie O. R., Mcallister T. A., Colwell D., Veira D., Wilmshurst J. F., et al. (2002). Effects of water quality on cattle performance. J. Range Manage. 55 (5), 452–460. doi: 10.2307/4003222
Wittmann E. J., Baylis M. (2000). Climate change: effects on culicoides-transmitted viruses and implications for the UK. Veterinary J. 160 (2), 107–117. doi: 10.1053/tvjl.2000.0470
Keywords: resilience, environmental disturbances, dairy cattle, productivity, sub-Saharan Africa
Citation: Oloo RD, Ojango JMK, Ekine-Dzivenu CC, Gebreyohanes G, Mrode R, Mwai OA and Chagunda MGG (2023) Enhancing individual animal resilience to environmental disturbances to address low productivity in dairy cattle performing in sub-Saharan Africa. Front. Anim. Sci. 4:1254877. doi: 10.3389/fanim.2023.1254877
Received: 07 July 2023; Accepted: 29 August 2023;
Published: 22 September 2023.
Edited by:
Francisco Javier Navas González, University of Cordoba, SpainReviewed by:
Bradley Heins, University of Minnesota Twin Cities, United StatesSemir Bechir Suheil Gaouar, University of Tlemcen, Algeria
Copyright © 2023 Oloo, Ojango, Ekine-Dzivenu, Gebreyohanes, Mrode, Mwai and Chagunda. This is an open-access article distributed under the terms of the Creative Commons Attribution License (CC BY). The use, distribution or reproduction in other forums is permitted, provided the original author(s) and the copyright owner(s) are credited and that the original publication in this journal is cited, in accordance with accepted academic practice. No use, distribution or reproduction is permitted which does not comply with these terms.
*Correspondence: Richard D. Oloo, richard.oloo@uni-hohenheim.de