- Department of Agricultural, Food and Nutritional Science, University of Alberta, Edmonton, AB, Canada
The first objective of this study was to compare behavioral and ear temperature changes using accelerometer ear tags (CowManager system; Sensor) during the declining progesterone (P4) phase (expected estrus) and the luteal phase determined using in-line milk P4 analysis (Herd Navigator system; HNS). The second objective was to evaluate the accuracy of each Sensor metric to detect estrus compared to HNS in a commercial dairy herd. Forty-six cows (23 young [1 to 2 lactations] and 23 mature [3 to 6 lactations]) at 20 days in milk (DIM) were fitted with Sensor tags, and P4 profiles measured via HNS until 90 DIM. Sensor metrics analyzed were Resting, Ruminating, Eating, Active, High-Active, and ear temperature (Etemp). The day of milk P4 decline below the 5 ng/mL threshold in the HNS was designated as d -1 (LSM ± SEM; 3.42 ± 0.08 ng/mL) and the day of expected estrus as d 0. Significant increases (LSM ± SEM) were observed at d 0 in Active (5.01 ± 0.14 min/h) and High-Active (8.70 ± 0.25 min/h) behavior responses as well as in Etemp (29.45 ± 0.08°C) compared with the luteal phase (Active: 4.46 ± 0.13 min/h; High-Active: 6.40 ± 0.22 min/h and Etemp: 28.69 ± 0.08°C). The greatest estrus detection accuracy (Youden Index [J: performance]) single metric was achieved using Etemp (0.24 J) followed by Resting (0.20 J) and High-Active (0.17 J) in all cows. Greater accuracy was observed in Young cows (Etemp: 0.44 J; Resting: 0.33 J; and High-Active: 0.25 J) than in Mature cows (Etemp: 0.09 J; Resting: 0.12 J; and High-Active: 0.13 J). Similarly, accuracy was greater when only healthy cows (cows with no postpartum health events) were compared (Etemp: 0.33 J; Resting: 0.31 J; High-Active: 0.20 J) to unhealthy cows (Etemp: 0.11 J; Resting: 0.02 J; High-Active: 0.02 J). The combination of behavior and Etemp metrics optimized the estrus detection accuracy in all the cows (0.30 J), Young (0.46 J), Mature (0.26 J), Healthy (0.45 J), and Unhealthy (0.11 J) cows compared to a single metric approach. Age and postpartum health affected the estrus detection accuracy using Sensor tags.
Introduction
Estrus detection is a pivotal element of reproductive management that determines the continuity of a herd’s lactation cycle. Traditionally, the observation of sexual receptivity behavior (e.g., standing-to-be-mounted) has been used to identify cyclic cows to be artificially inseminated within 60 to 100 days in milk (DIM) to maintain an optimum calving interval, thereby sustainable dairy production (De Vries, 2006). However, visual observation of estrus behaviors is only approximately 50% accurate (i.e., estrus detection rate) in lactating cows of 60 to 100 DIM (LeBlanc, 2005).
There is evidence that physiological challenges (e.g., negative energy balance and increased rate of steroid hormone clearance) and changes in behavior (e.g., increased eating and rumination time) to cope with the greater demands of milk production during early lactation are potential reasons for low visual detection of standing estrus events (Lopez et al., 2004). Other authors attribute the deficit in estrus detection to the lack of experienced workforce needed to observe cows (Pfeiffer et al., 2020), non-optimal environments [e.g., concrete flooring (Britt et al., 1986); tie-stalls (Felton et al., 2012), and heat stress (> 25°C; Hansen and Aréchiga, 1999)] that hinder cows from expressing normal sexual behaviors. In addition, dairy herds have increased in size, in recent decades (242% in Western Canada, and 37% in Eastern Canada; Jelinski et al., 2015), making estrus detection in larger herds more difficult due to the lack of workforce to perform visual observations of estrus.
To address low estrus detection rates, technology developers and scientists have been working together to create automated technologies that can measure biological traits (e.g., physiological and behavioral changes) to determine when to inseminate cows. Automated technologies that have been developed include heat mount detectors (e.g., HeatWatch system: DDx, Inc., Denver, CO) that create estrus alerts based on mounting events (Rorie et al., 2002), reticulo-rumen temperature loggers (e.g., DVM bolus; DVM Systems, LLC, Greeley, CO) that identify cows in estrus based on expected increases in core body temperature during estrus (Burnett et al., 2020), and 3-D accelerometers (e.g., Afitag S.A.E. Afikim, Kibbutz Afikim, Israël) that record the duration of rumination, resting, and walking activity (Dolecheck et al., 2015). On the other hand, technology that measures physiological traits include in-line milk analysis (e.g., Herd Navigator™, DeLaval International, Tumba, Sweden & Lattec I/S, Hillerød, Denmark) that indicate when cows are in estrus based on milk progesterone (P4) concentrations (Bruinjé et al., 2019), volatile chemical compounds using electronic nose detectors (Sanderink et al., 2017), vaginal mucus impedance and electrical resistance (Zuluaga et al., 2008), and increases in skin temperature measured with infrared thermography cameras (Perez Marquez et al., 2019).
Most automated technologies have greater estrus detection rates (≈80%) than visual observation of estrus behaviors with minimal labor input (Sauls et al., 2017). However, the evaluation of automated technologies has been often conducted under research conditions (e.g., synchronized estrus, controlled experimental units, and small samples sizes), using standing-to-be-mounted as the gold standard (low estrus detection accuracy), and using cows beyond 100 DIM (Dolecheck, 2015). These conditions do not always represent the circumstances in which producers will use automated estrus detection technologies leaving a gap in the knowledge regarding the actual accuracy in commercial conditions.
Our objectives were: 1) to compare behavioral (i.e., Resting, Ruminating, Eating, Active, and High-Active) and ear temperature (Etemp) changes using 3-D accelerometer ear tags (CowManager system; Sensor) during the declining P4 phase (i.e., proestrus and estrus) with non-estrus periods (i.e., diestrus or luteal phase) determined using in-line milk P4 analysis (Herd Navigator system; HNS), and 2) to evaluate the accuracy of each Sensor metric to detect estrus compared to HNS in a commercial dairy herd. We hypothesized that behavior and Etemp changes (either increases or decreases) and estrus detection accuracy via Sensor can be influenced by parity, postpartum health, time of day, and between 1st and 2nd estrous cycle in a commercial dairy herd.
Materials and methods
Animals and housing
Forty-six Holstein cows (n = 46) at different parities (23 young [1 to 2 lactations] and 23 mature [3 to 6 lactations]) were used in this study following the sample size calculation using alpha (α = 0.05), power (β = 0.95), and a correlation coefficient (r = 0.60) parameters for clinical research (Hulley et al., 2013). Cows were followed from 20 to 90 DIM (early lactation) and produced 43.9 ± 9.85 (mean ± SEM) kg of milk per day during peak lactation. The study was conducted from February to October 2020 at the Lakeland College Dairy Learning Centre (Vermilion, Alberta, Canada), a 121-cow free-stall facility, that simulates a commercial dairy operation. All lactating cows in this study were milked by a voluntary (robotic) milking system (De Laval Inc.). Partial mixed ration provided in the feed bunk included corn silage, rolled barley-corn, grass hay, mineral supplements, in addition to a concentrate mixture offered at the voluntary milking system. Cows had free access to potable water, and the ration formulation was based on NRC guidelines (National Research Council, 2001) for lactating dairy cows.
Ear-tag accelerometer data collection
Behavioral traits were collected and analyzed using 3-D accelerometer ear tag Sensors (CowManager Sensor™ Agis Automatisering, Harmelen, Netherlands) placed on all 46 cows at 20 to 90 DIM (Figure 1). Sensor tags were attached to the radio frequency identification (i.e., RFID) tag located at the right ear, between the auricular cartilages, which captured data every min and sent data every 60 min using a wireless router antenna to a coordinator antenna connected to a computer. Data for the first 7 d after placement were excluded from our analysis as per manufacturer recommendation to build up a reference period. Sensor tag data are based on ear and head movements and head position, which are collected and classified into 1 of 5 behavioral traits as follows: Resting (lying-standing inactive), Ruminating (lying-standing ruminating), Eating (muzzle on feed), Active (head movements while standing), and High-Active (walking with head movements), reported as minutes of behavior per hour (min/h) and previously validated by Bikker et al. (2014); Resting [0.86 ± 0.02 Kappa coefficient (K)], Ruminating [0.85 ± 0.01K], Eating [0.77 ± 0.03K], Active [0.47 ± 0.03K], and High-Active [0.49 ± 1.17K). Complementary to the behavior metrics, Sensor tags provide ear-surface temperature (Etemp: °C) hourly, although manufacturer algorithms do not consider ear temperature to detect estrus. Raw data files were received via email from the manufacturer on a daily basis. Ambient temperature (Atemp °C) was recorded every hour (average) using a temperature logger (iButtonLink, LLC, Whitewater, WI, United States) located outside the voluntary milking system robot to identify possible associations between Atemp and Etemp and behavioral responses to Atemp changes. Estrus alerts, behavior and Etemp data were displayed using Agis CowManager (sensor.cowmanager.com) and the IOS 14.8 Smartphone CowManager App.
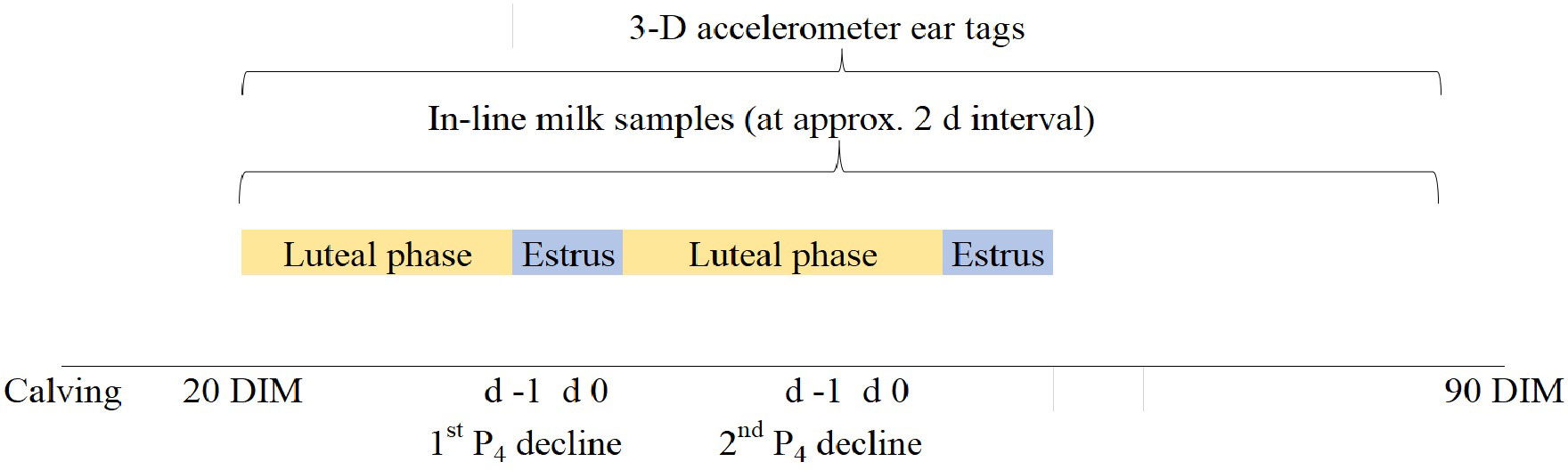
Figure 1 Timeline of the study shown using the example of a hypothetical cow that had her first ovulation at approximately 20 days in milk (DIM). The first estrus event after calving, based on milk P4 decline, occurred on average at (LSM ± SEM) 56.7 ± 4.24 DIM. In-line milk samples (HNS; Herd Navigator system) began at 20 DIM and sample collection continued at approximately 2-d intervals to monitor progesterone (P4) concentrations. The day after the decline in P4 was defined as d 0 which was used to estimate the expected estrus period following the P4 decline below 5 ng/mL after being higher in the previous 2 sampling events. Three-dimension accelerometer sensor tags (Sensor; CowManager Sensor System) were placed in 46 eligible cows at 20 DIM to measure behavioral metrics and ear temperature changes at the decrease in milk P4 measured using HNS.
In-line milk progesterone analysis
Milk P4 concentrations were collected automatically via the HNS to flag the estrus occurrence. Milk samples were taken at set (≈2 d) intervals starting at day 21 after calving; however, this study evaluated the P4 concentrations at d -14, d-7, d -2, d -1, d 0, and d 4 to maintain consistency through sample collection per cow. The estrus period was confirmed in this study based on the decline in P4 concentrations (i.e., below 5 ng/mL) for 2 consecutive sampling events after being greater than 5 ng/mL. The estrus alert accuracy of HNS was previously validated (99% accuracy; 93.3% Sensitivity, and 93.7% Specificity) against visual examination of estrus behaviors by Friggens et al. (2008). Progesterone profiles and estrus alerts were displayed using the herd management software (DeLaval Delpro™, International, Tumba, Sweden). In this study, the estrus period (d 0) was defined as the day following P4 decline (d -1) in milk below 5 ng/mL as per HNS recommendations (See Figure 1). No visual mounting or standing-to-be-mounted events were observed or recorded in this study.
Statistical analysis
Data were analyzed using SAS software (ver. 9.4, SAS, Cary, NC, USA) to identify changes in behavior traits and Etemp during the estrus period (i.e., d 0) compared to the luteal phase (i.e., d -14 to d -7). The Univariate procedure was used to test the normality assumption using Kolmogorov-Smirnov test in each metric. Behavioral data did not comply with the normality assumptions, and models were fitted using a Generalized Linear Mixed Model approach (GLIMMIX procedure) with Poisson distribution specified (Note: Etemp data were normally distributed). Independent variables included Sample Day (d -21 to d 7), Sample time (0 to 23 h), Parity group (Young: 1-2 and Mature: 3-6), and estrous cycle number (1st and 2nd). Data were tested using a Type 3-test with the inverse (ilink) function specified and the ar(1) statement to account for the lack of independent and homogeneous data. All results are presented as least squares means (LSM) and standard error means (SEM) using a Bonferroni means separation test. To test the effect of Atemp on behavioral traits and Etemp, a regression analysis was performed to estimate the expected behavioral response and Etemp changes due to different barn Atemp. Differences were considered significant if P < 0.05, a tendency if P > 0.05 but < 0.10 and not significant if P ≥ 0.10. Milk P4 concentrations were analyzed using Sample Day as a fixed variable.
To evaluate the accuracy of Sensor tags to detect estrus, Receiver Operating Characteristics (ROC) curve analyses were performed using SAS software. A logistic regression procedure was used to calculate the number of cows in estrus (detected by Sensor) divided by the number of cows confirmed in estrus by HNS [Se: Sensitivity], and the number of cows detected not to be in estrus (detected by Sensor tags) by the number confirmed not to be in estrus (Sp: Specificity). In addition, a Youden J index (J) was used to identify the most optimum reference value (i.e., threshold value) with a balance Se and Sp level to determine the proportion of false positives and false negatives expected in a diagnostic test. Receiver Operating Character curve analysis was performed in all cows, parity groups (i.e., Young-Mature), cows with no postpartum disease events during the data collection (Healthy), and cows with postpartum disease events during the current lactation (Unhealthy; mastitis, lameness, ketosis, metritis, hypocalcemia, etc.). The evaluation of accuracy was performed in single behavior and Etemp metrics as well as all behaviors and Etemp combined (i.e., Resting, Ruminating, Eating, Active, High-Active, and Etemp).
Results
The P4 concentration in milk (LSM ± SEM) for all the cows in the study at d -1 was 3.4 ± 0.08 ng/mL, and the P4 peak was found at d -7 (21.8 ± 0.43 ng/mL). The first estrus event after calving, based on milk P4 decline, occurred on average at 56.65 ± 4.24 DIM, when all cows were considered. Duration of the estrous cycle between P4 nadirs averaged 24.9 ± 0.99 d ranging from 20 to 43 d. A total of 96 estrus alerts were created by HNS during the study period with no difference (P = 0.10) in the estrus alert time of day (i.e., AM – PM).
Behavioral traits
Resting (Figure 2A; P = 0.40) decreased at d 0 (13.8 ± 0.45 min/h) and d 2 (4.28 ± 0.46 min/h) compared to d -7 (15.5 ± 0.45 min/h). Ruminating (Figure 2B; P = 0.19) and Eating (Figure 2C; P = 0.98) did not differ at d 0 compared to d -7. Active and High-Active behaviors had a significant change in Sample Day behavior response duration during d 0 (Figures 2D, E). In particular, Active behavior (P = 0.01) was observed to increase at d 0 (5.0 ± 0.14 min/h) compared to d -7 (4.5 ± 0.13 min/h), similarly, High-Active (P = 0.01) increased at 24 h (d 0; 8.7 ± 0.25 min/h) and 48 h after the decline of P4 (d 2; 8.2 ± 0.24 min/h) compared to d -7 (6.4 ± 0.22 min/h).
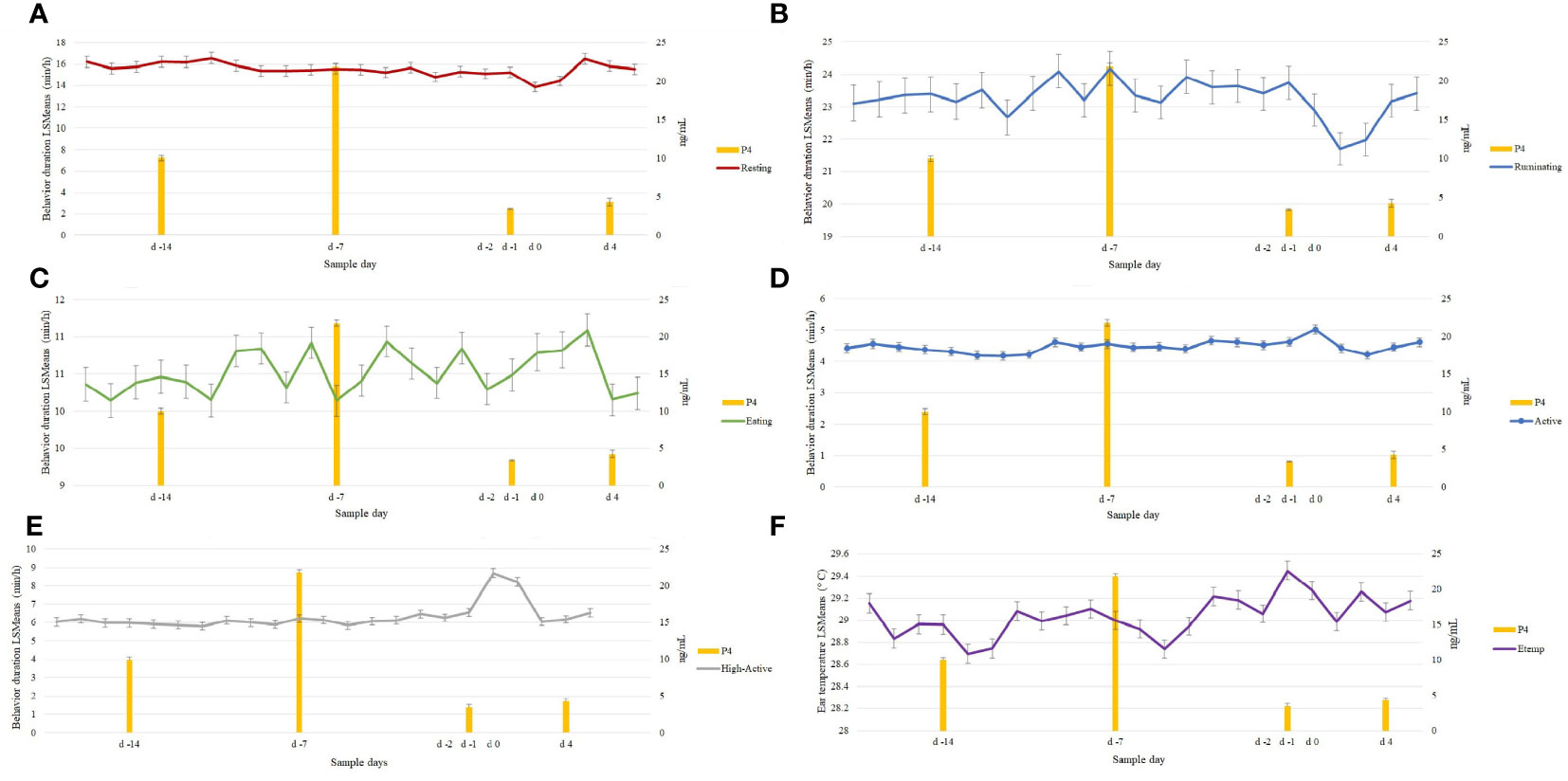
Figure 2 Behavioral changes in 96 estrous cycles (n=46; P < 0.05) preceding the decrease in progesterone (P4). Resting (A) behavior decreased at d 0 while Active (D) and High-Active (E) behaviors increased. Decrease in Resting and increase in High-Active continued at d 2. No behavioral changes (P > 0.05) were observed in Ruminating (B) and Eating (C) during the decline in P4 (d -1). Ear temperature (F: Etemp) increase (P = 0.01) at d 0 (decrease of Progesterone: P4) and lowest Etemp reported during the luteal phase (d -13 and d -5) in 96 estrous cycles.
Sample time had a significant effect on all behaviors (P < 0.01) as expected (i.e., changes related to the cow’s daily routine). Resting increased from 0500 to 1900 (17.2 ± 0.46 min/h) with a decrease at 1100 (14.8 ± 0.47 min/h). Ruminating increased from 0400 to 1200 (26.3 ± 0.49 min/h) and decreased from 1300 to 0300 (21.34 ± 0.45 min/h). Similarly, Eating increased late in the evening 2000 to early morning 0300 (13.40 ± 0.49 min/h) and decreased from 0400 to 1900 (9.09 ± 0.49 min/h). Active and High-Active behaviors were increased at 0300 (Active: 5.30 ± 0.49 min/h; High-Active: 8.34 ± 0.49 min/h) and at 2200 (Active: 5.28 ± 0.13 min/h; High-Active: 8.13 ± 0.22 min/h) compared to the rest of the day (Active: 4.46 ± 0.12 min/h; High-Active: 6.39 ± 0.20 min/h). In addition, parity groups differed (P < 0.05) for all behavioral traits. Young cows had longer durations of Ruminating, Eating, and High-Active behaviors compared to Mature cows (Table 1). However, Mature cows had longer Resting and Active durations compared to Young cows (Table 1). Behaviors neither differed (P > 0.10) between the 1st and 2nd estrous cycle, nor was any association found between Atemp and behavioral traits (R2 < 0.03).
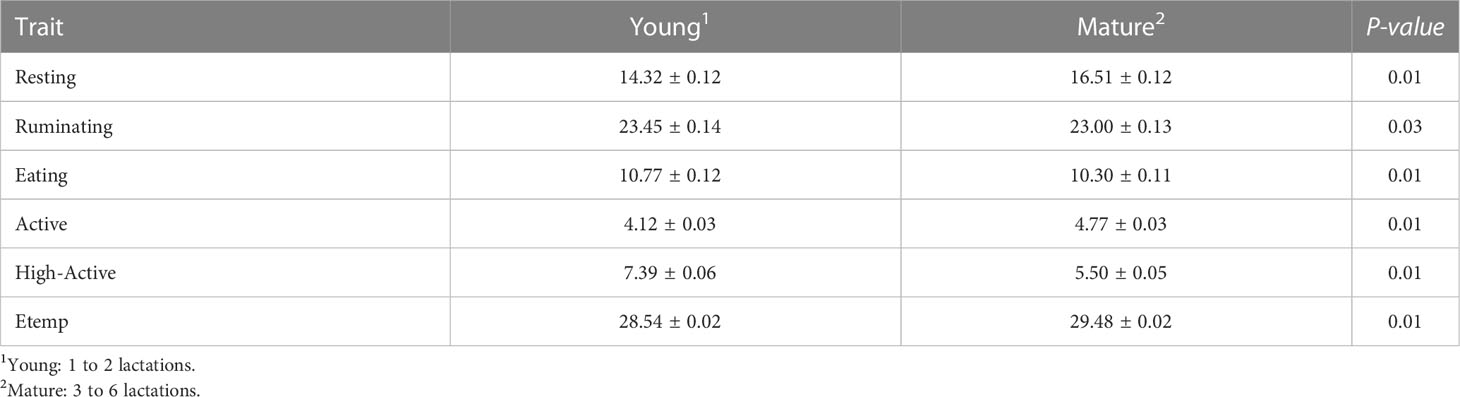
Table 1 Behavior traits (LSM ± SEM; min/h) and ear temperature (Etemp: °C) comparison between Young and Mature cows at the confirmation of the estrus period (d 0).
Ear temperature
Ear temperature increased (P = 0.01) during the estrus period (d 0: 29.45 ± 0.08°C) compared to the average of non-estrus days [d -21 to d -1; 29.02 ± 0.08°C. (Figure 2F)]. The lowest Etemp was found at d -13 (28.69 ± 0.09°C) and d -5 (28.74 ± 0.08°C). In addition, Etemp was lower during midday (1100 to 1400: 28.10 ± 0.07°C) compared to 1500 to 2400 (29.23 ± 0.07°C). Despite the Sample time effect, the increase at d 0 was greater than the other Sample Days. In addition, Mature cows had higher Etemp (29.48 ± 0.02°C) compared to Young cows (28.54 ± 0.02°C) when observed at d 0 (Mature: 29.86 ± 0.10°C; Young: 28.98 ± 0.12°C). Furthermore, the associations between ATemp and Etemp were evident (R2 = 0.54); however, despite the Atemp effect, Etemp increased at d 0 (30.37 ± 0.01°C) at similar Atemp (17.36 ± 0.02°C) compared to d -7 (Etemp 29.01 ± 0.01°C; Temp: 17.36 ± 0.02°C). No significant changes in Etemp were found (P > 0.10) between estrous cycles (1st and 2nd).
Estrus detection evaluation
Reference values for each behavior and Etemp, and the corresponding Se, Sp, and J index are presented in Table 2. The greatest accuracy based on J index was achieved by Etemp (0.24 J) followed by Resting (0.20 J) and High-Active (0.17 J) when ROC curve analyses were performed for all the cows in the study. Differences between Young and Mature cows were noted in accuracy. Overall Young cows had greater estrus detection accuracies (Resting: 0.33 J; High-Active: 0.25 J; Etemp: 0.44 J) compared to Mature cows (Resting: 0.12 J; High-Active: 0.13 J; Etemp: 0.09 J). In addition, when Unhealthy cows (n = 12) were removed from the ROC analysis, the accuracy of all traits increased despite the parity group (Resting: 0.31 J; High-Active: 0.20 J; Etemp: 0.33 J, See Table 2). Accuracy of estrus detection increased when ROC curves were generated using the combination of behavior and Etemp metrics for all cows (0.30 J), Young (0.46 J), Mature (0.26 J), Healthy (0.45 J), and Unhealthy (0.11 J) cows (Figures 3A1 to 5) compared to the individual metrics of Resting (Figures 3B1 to 5), High-Active (Figures 3C1 to 5), and Etemp (Figures 3D1 to 5) for the corresponding groups.
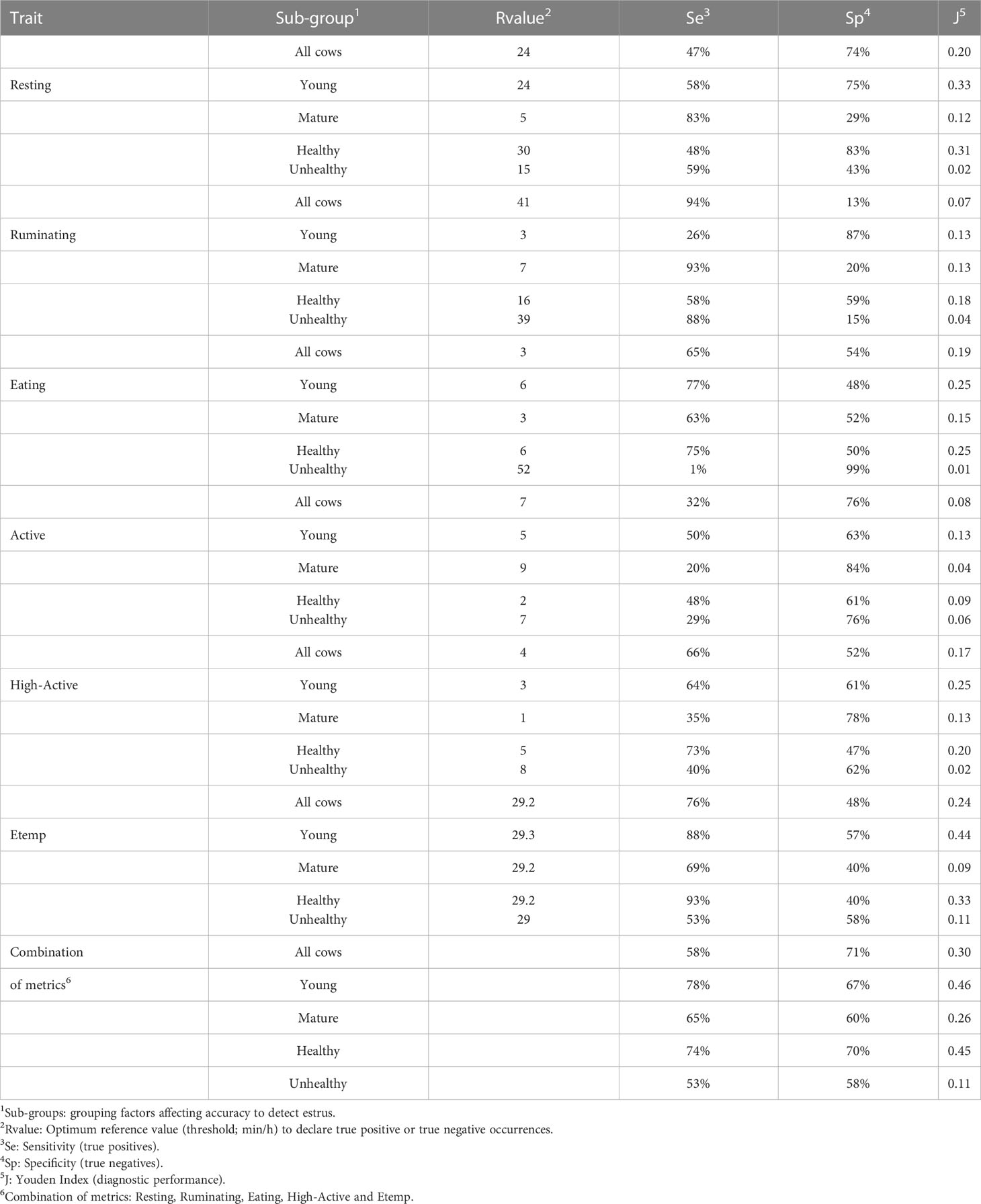
Table 2 Accuracy evaluation (ROC- curve analysis) results from all behaviors and ear temperature (Etemp) in 96 estrus events (2 estrous cycles per eligible cow).
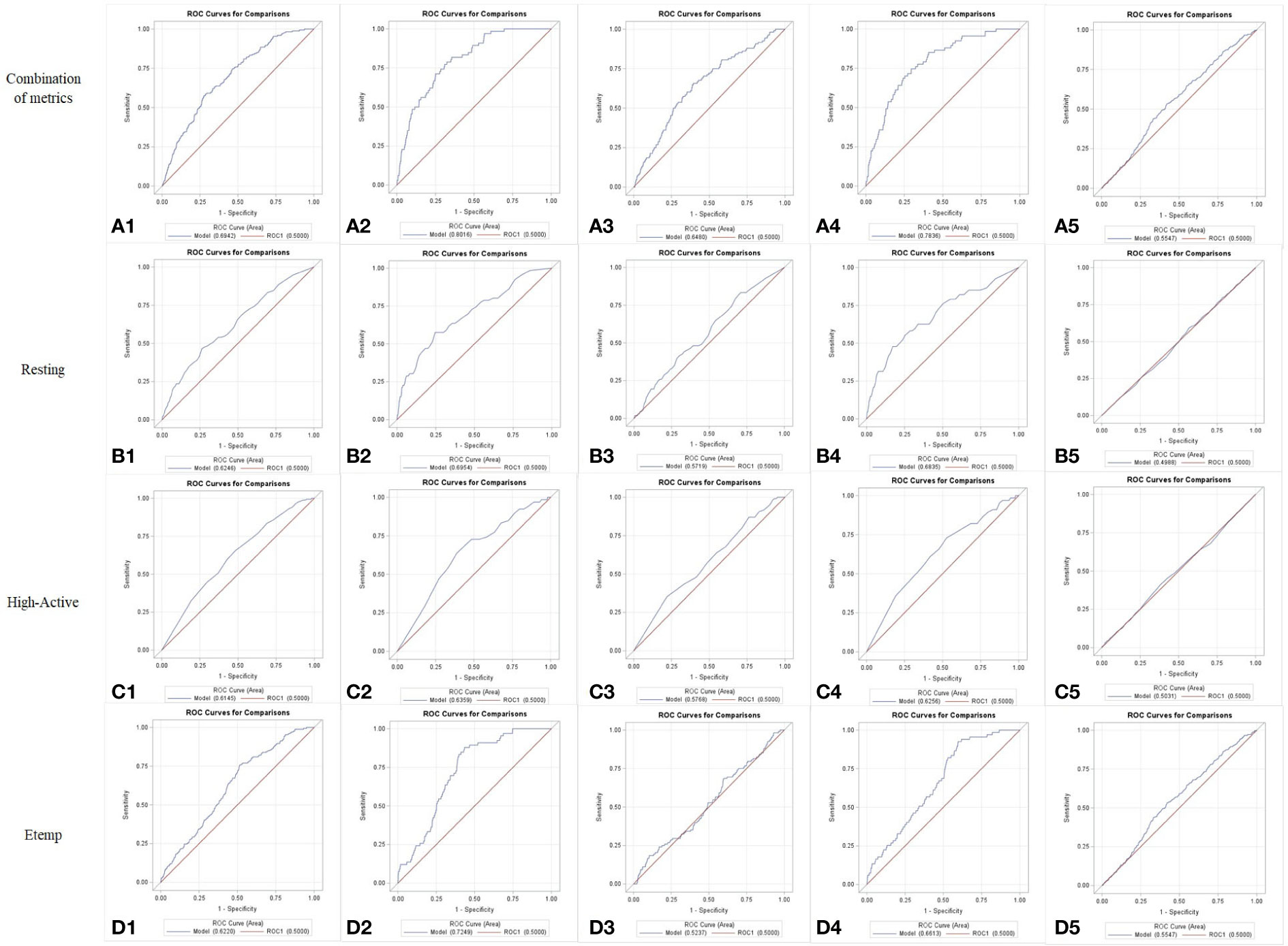
Figure 3 Receiver Operating Characteristics (ROC) curves for the combination of behavior and Ear temperature (Etemp) metrics (A) or for Resting (B), High-Activity (C), and Etemp (D), individually, presented for all cows (1), Young cows [2], Mature cows [3], cows with no postpartum health events [Healthy (4)], and for cows with at least one postpartum health event [Unhealthy (5)] during the current lactation. Greater accuracies (Sensitivity, Specificity and Youden J index) were evident when the metrics were combined (A1 to A5).
Discussion
The objectives of this study were to compare behavioral traits and ear temperature changes using 3-D accelerometer ear tags that corresponded with the decline in P4 using HNS and to evaluate the accuracy of each Sensor metric to detect estrus compared to HNS in a commercial dairy herd. We found that locomotory behaviors (i.e., Active, and High-Active) and ear temperature increased during the estrus period (d -1 to d 0) compared to other days of the estrous cycle (d -14, d -7, and d 4).
The decrease in Resting behavior observed at d 0 and d 2 compared to non-estrus days is inversely proportional to the increase in Active and High-Active behaviors observed at d 0 as observed by Stevenson (2021). Resting behavior was defined as lying-down or standing with no head-ear movements by Bikker et al. (2014), which means that an increase in other behaviors (e.g., Active and High-Active) should result in decreased Resting. The increase in restless movements (e.g., mounting or attempting to mount behaviors) during the onset of estrus have been widely reported in the scientific literature as secondary signs of estrus (Van Vliet and van Eerdenburg, 1996; Kerbrat and Disenhaus, 2004; Sveberg et al., 2011). The CowManager system is not configured to measure mounting events since the 3-D accelerometers measure behaviors based on the position or orientation of a sensor placed on a cow’s ear when it is moved relative to an inertial system (e.g., earth; Haslwanter, 2018). Sensors can measure the frequencies of ear and head movement while walking, which suggests that secondary estrus behaviors like mounting involve walking and head-ear movements observed at lower frequency during non-estrus periods than the estrus period. In addition to mounting behavior, other accessory sexual behaviors associated with head-ear movements could be vulva sniffing, urine sniffing and female-to-female chasing, frequently found prior to standing-to-be-mounted in Bos taurus (Price, 2008).
Active and High-Active increases (d 0) did not perfectly align with the decline in milk P4 at d 0 (24 h prior to the increase of Active and High-Active). The ≥24 h delay in the onset of increased activity was expected because the mean interval from induction of luteolysis to the onset of peak activity in dairy cows was 71 ± 17 h (Valenza et al., 2012), whereas the interval between plasma P4 decline and the occurrence of ovulation has been 24 to 48 h (Perez Marquez et al., 2019). The HNS recommends inseminating eligible cows 36 h after milk P4 decline triggering an estrus alert (Bruinjé et al., 2017) compared to the interval between activity increases and ovulation of ≈28.7 h (Valenza et al., 2012) which coincides with the onset of standing-to-be-mounted prior ovulation (28-30 h; Roelofs et al., 2005). In contrast, Ruminating and Eating did not change relative to the P4 concentration as other studies reported using 3-D accelerometers (74 min/24 h reduced rumination than base level: Reith and Hoy, 2012; 84 min/24 h reduced rumination than base level and 58 min/24 h fewer eating time than base level: Pahl et al., 2015). The differences in Ruminating and Eating could be attributed to the difference in 3-D accelerometer manufacturer (e.g., neck collar sensors compared to ear sensors), the stage in lactation (lower feed intake and rumination during early lactation [20 to 90 DIM] compared to >100 DIM in Pahl et al., 2015), and behavior data unit recorded (e.g., min/h compared to min/24 h, Dolecheck et al., 2015; Stevenson, 2021).
The changes in behavior responses per sample time observed may be related to the dairy cow’s circadian rhythm and the nature of Bos taurus behavior. Lactating dairy cows are often milked at 12 h intervals AM and PM, which can influence cow behavior during the day. However, in this study, cows were milked via voluntary milking system, which implies that the time of milking is voluntary. In particular, Resting increased between 0500 to 1900 with a decrease during 1100 which implies that cows were lying down or standing idle, which coincides with Bos taurus crepuscular activity (i.e., most active at the sunrise and sunset) and preferences to be active during cooler temperatures (Jensen, 2017). Crepuscular behavior of cattle may also explain the increase in Active, High-Active, and Eating behaviors early in the morning (0300) and late at night (2200). In addition, rumination time increased from 0400 to 1200 (6 to 8 h per day, Jensen, 2017). The differences observed between Young and Mature cows (longer Ruminating, Eating, and High-Active in Young cows compared to Mature cows and longer Resting and Active in Mature cows compared to Young cows) were similar to reported feed intake and rumination activity (Maekawa et al., 2002; Kowsar et al., 2008) which could be attributed to the morphological attributes of younger animals such as smaller rumen size, body weight, and body size compared to Mature cows.
The Etemp was observed to be higher at d 0 (29.45 ± 0.08°C) compared to any other Sample Days, and the minimum Etemp reported during the estimated luteal phase based on P4 profiles (d -14: 28.69 ± 0.09°C; d -7: 28.74 ± 0.08°C). The increase of Etemp could be associated with the increase of body movement (e.g., walking, head-ear movements, and mounting prior ovulation, Walton and King, 1986). However, the increase in High-Active and Active behaviors were observed 24 h after (d 0) the increase of Etemp (d -1). Another study suggested that the elevated presence of estradiol expected at the decrease of P4, as well as the GnRH and LH response during estrus could be associated with the increase of temperature in dairy cows (Talukder et al., 2014). Furthermore, increases in skin temperature using infrared thermography at different body locations (e.g., eyes, neck, vulva, flank, and rump) of dairy cows have been reported 48 and 24 h before the decline in P4 concentrations (<3.17 nmol/L in plasma; Perez Marquez et al., 2019). Other research studies using the same accelerometer (Sensor tags) found increases in ear temperature during the estrus period (24.17°C ± 1.2°C) compared to non-estrus days (23.0 ± 1.20°C); however, the differences were not significant (P = 0.20) most likely due to the smaller degrees of freedom in that study (df = 17: Dolecheck et al., 2015) compared to this study (df = 95). Similarly, Dolecheck et al. (2015) found significant increases in temperature during estrus (0.43°C) compared to non-estrus periods using reticulum-rumen bolus temperature logger (i.e., DVM bolus) with similar results presented in this study (0.43°C) using Etemp.
Factors affecting Etemp measurement were sample time and parity groups (i.e., Young and Mature cows). Ear temperature was observed to be higher during the afternoon and the morning (29.2 ± 0.07°C) compared to midday (28.1 ± 0.07°C) when the ambient temperature is expected to be the highest. The temperature increases between the morning and evening coincided with an increase in Active, High-Active, and Eating behaviors which coincided with crepuscular activity expected in Bos taurus. In addition, increases in Etemp could be attributed to heat production after feeding intake due to metabolism (e.g., protein synthesis and energy intake) demonstrated to increase body core temperature (Kyle et al., 1998). No feed intake data or metabolic data were collected in this experiment. Younger cows had lower Etemp overall (28.54°C ± 0.02) compared to Mature cows (29.48°C ± 0.02) as well during d 0 (Young: 28.98 ± 0.12°C; Mature: 29.86 ± 0.10°C) regardless of higher activity observed in Young cows compared to Mature cows at d 0 (Young: 7.39 ± 0.06 min/h; Mature: 5.50 ± 0.05 min/h). The higher Etemp in Mature cows may have been associated with greater body weight, increased feed intake, and possibly higher blood volume compared to Young cows, although body weight, feed intake, and blood volume were not determined in this study.
Additionally, Etemp were positively correlated with the Atemp inside the dairy barn (R2 = 0.54) during the study timeline. The current study was subjected to changes in Atemp due to the seasonality effects (e.g., colder temperature during winter compared to summer). It was expected that body core temperatures in dairy cows would fluctuate according to ambient conditions depending on coat, subcutaneous fat, and metabolic rate if exposed to critical temperatures (e.g., critical low -5°C; critical high 25°C, Knızkova et al., 2002). Although the critical warm temperatures were reached several times during the summer (>25°C), the highest Etemp registered did not coincide with the highest summer Atemp, rather with the decrease in P4 (d 0: 30.37 ± 0.01°C).
Our second objective was to evaluate the accuracy of each parameter measured with Sensor compared to the decrease in P4 as an indirect sign of estrus for all the eligible cows in a commercial dairy herd. This study found increases in activity behaviors (Active and High-Active) and skin temperature at the ear (Etemp) during the decrease of P4 that indicate the occurrence of estrus confirmed by obtaining the most efficient reference value (i.e., cut off value). The evaluation of each behavior and ear temperature was completed using the balanced contribution of true estrus positives (i.e., sensitivity) and true estrus negatives (i.e., specificity) represented as a performance index (i.e., Youden J Index). In particular, the proportion of true estrus positives represents the ability of each parameter to detect estrus (i.e., estrus detection rate) with the minimum number of false positive alerts possible (e.g., the proportion of true estrus negatives not flagged using a particular cut off value) which indicates the accuracy of a parameter measured by Sensor with a given cut off value. In this study, Etemp was found to be the most accurate parameter to flag eligible cows in estrus (76% Se, 48% Sp, 0.24 J), followed by Resting (47% Se, 74% Sp, 0.20 J) and High-Active behaviors (66% Se, 52% Sp, 0.17 J). Other studies had reported greater accuracies for ear accelerometer tags (100% CowManager system: Dolecheck et al., 2015; 97% SMARTBOW system: Schweinzer et al., 2019) compared to our results in dairy cows under hormone-based ovulation synchronization treatments. The accuracy differences observed using similar equipment could be related to the absence of hormone treatments and the DIM when the cows were monitored (20-90 DIM). Some studies indicate estrus detection is difficult during the first 100 DIM due to physiological demands placed on lactating cows after parturition (e.g., increasing milk production, negative energy balance, Butler, 1998; Lopez et al., 2005). Nevertheless, the current study aimed to evaluate the accuracy of Sensor tags during the most economically sustainable period (60 to 100 DIM; De Vries and Conlin, 2003), in all the eligible (i.e., non-pregnant) cows in a commercial dairy herd.
Some of the factors affecting the accuracy of Sensor were the parity group and the postpartum health events of the eligible cows during the study period. Young cows had greater accuracies compared to Mature cows. Accuracy differences by parity could be associated with the difference in high active behavior expression in Young cows (7.39 ± 0.06 min/h) compared to Mature cows (5.50 ± 0.05 min/h), also found using other accelerometer technologies (e.g., Heatime and Boumatic; Madureira et al., 2015) as well the minimum cut off value used to differentiate estrus and non-estrus (Young: 3 min/h compared to Mature: 1 min/h). The potential reason for less high active behaviors in mature cows could be attributed to the larger body mass compared with younger cows and possible previous injuries impacting the locomotory system (e.g., lameness). Another risk factor associated with the accuracy of results was the prevalence of clinical disease (e.g., mastitis, metritis, lameness, leukosis) in some of the cows (n = 12) during the study period. When cows with postpartum health events were removed from the accuracy evaluation analysis, the overall performance index (Youden J Index) increased for all the traits measured. In particular, Etemp sensitivity increased up to 93%, which was the highest estrus detection rate achieved by Sensor in this study. The increased accuracy when removing cows with clinical cases may be because of the increase in Etemp due to fever associated with disease and inflammation. Similarly, the presence of fever could negatively affect the expression of normal behavior during estrus and non-estrus periods. The combination of behavior and Etemp increased the accuracy of evaluation in all cows, Young vs. Mature cows, and healthy vs. unhealthy cows, demonstrating that a multi-metric approach reduces the false-positive and false negative proportions in an estrus alert. Results indicate that combining all Sensor metrics (ear temperature plus activity behaviors) increases the accuracy of estrus detection during the most economically efficient period (<100 DIM) to inseminate lactating dairy cows in a commercial free-stall herd, although estrus detection efficiency was greatest only in cows with no history of postpartum illness.
Conclusions
This study found that activity behaviors and ear temperature increased following milk P4 decline preceding the estrus period compared to other days of the estrous cycle. The factors that affected behavior responses and ear temperature were time of day, postpartum health events and parity group. Greater activity was found in young cows than in mature cows, however, mature cows had higher ear temperature compared to young cows. Ear temperature, Resting and High-Active had the highest accuracy to detect estrus. Accuracy increased if only young cows or only healthy cows were analyzed using Ear temperature, Resting and High-Active behaviors. Furthermore, when activity behaviors and ear temperature were combined, in all cows, the accuracy of estrus detection increased. We conclude that parity group and postpartum health events affect the accuracy of estrus alerts using 3-D accelerometer ear tags.
Data availability statement
The raw data supporting the conclusions of this article will be made available by the authors, without undue reservation.
Ethics statement
The animal study was reviewed and approved by the University of Alberta Animal Care and Use Committee for Livestock and Animal Care Committee - Animal Use for Research: Lakeland College.
Author contributions
HP contributed to writing the original draft preparation, visualization, formal analysis, investigation, and funding acquisition. DA contributed to the conceptualization, methodology, writing-review, editing, and funding acquisition. CB contributed to the conceptualization, methodology design, lab resources, writing-review, editing, PhD supervision, project administration and served as PI on the funded grant. All authors contributed to the article and approved the submitted version
Funding
This work was supported by the Agriculture Funding Consortium (Alberta Agriculture and Forestry’s Strategic Research and Development Program) and Alberta Milk (Grant 2019F059R).
Acknowledgments
The authors thank the Agriculture Funding Consortium (Alberta Agriculture and Forestry’s Strategic Research and Development Program) and Alberta Milk for providing funding for this research. In addition, the authors acknowledge the Department of Agricultural, Food and Nutritional Science at the University of Alberta, Lakeland College and Animal InfraMetrics for providing in-kind support. We acknowledge Dr Obioha Durunna for assistance with the project logistics at Lakeland College, and the Dairy Learning Centre staff who provided assistance with animal care and handling.
Conflict of interest
The authors declare that the research was conducted in the absence of any commercial or financial relationships that could be construed as a potential conflict of interest.
Publisher’s note
All claims expressed in this article are solely those of the authors and do not necessarily represent those of their affiliated organizations, or those of the publisher, the editors and the reviewers. Any product that may be evaluated in this article, or claim that may be made by its manufacturer, is not guaranteed or endorsed by the publisher.
Abbreviations
DIM, Days in milk; P4, Progesterone; Sensor, CowManager Sensor System; HNS, Herd Navigator System; Etemp, Ear-temperature; Atemp, Ambient temperature; ROC, Receiver Operating Curve; Se, Sensitivity; Sp, Specificity; J, Youden J Index.
References
Bikker J. P., van Laar H., Rump P., Doorenbos J., van Meurs K., Griffioen G. M., et al. (2014). Technical note: evaluation of an ear-attached movement sensor to record cow feeding behavior and activity. J. Dairy Sci. 97, 2974–2979. doi: 10.3168/jds.2013-7560
Britt J. H., Scott R. G., Armstrong J. D., Whitacre M. D. (1986). Determinants of estrous behavior in lactating holstein cows. J. Dairy Sci. 69, 2195–2202. doi: 10.3168/jds.S0022-0302(86)80653-1
Bruinjé T. C., Colazo M. G., Gobikrushanth M., Ambrose D. J. (2017). Relationships among early postpartum luteal activity, parity, and insemination outcomes based on in-line milk progesterone profiles in Canadian Holstein cows. Theriogenology 100, 32–41. doi: 10.1016/j.theriogenology.2017.05.021
Bruinjé T. C., Colazo M. G., Ribeiro E. S., Gobikrushanth M., Ambrose D. J. (2019). Using in-line milk progesterone data to characterize parameters of luteal activity and their association with fertility in Holstein cows. J. Dairy Sci. 102, 780–798. doi: 10.3168/jds.2018-14654
Burnett T. A., Kaur M., Polsky L., Cerri R. L. A. (2020). Rumen-reticular temperature during estrus and ovulation using automated activity monitors in dairy cows. Front. Vet. Sci. 7, 597512 doi: 10.3389/fvets.2020.597512
Butler W. R. (1998). Review: effect of protein nutrition on ovarian and uterine physiology in dairy cattle. J. Dairy Sci. 81, 1874–1882. doi: 10.3168/jds.S0022-0302(98)70146-8
De Vries A. (2006). Economic value of pregnancy in dairy cattle. J. Dairy Sci. 89, 3876–3885. doi: 10.3168/jds.S0022-0302(06)72430-4
De Vries A., Conlin B. J. (2003). Economic value of timely determination of unexpected decreases in detection of estrus using control charts. J. Dairy Sci. 11, 3516–3526. doi: 10.3168/jds.S0022-0302(03)73956-3
Dolecheck K. A. (2015). Assessment of the technical and economic potential of automated estrus detection technologies for dairy cattle (Lexington (KY: University of Kentucky).
Dolecheck K. A., Silvia W. J., Heersche G. Jr., Chang Y. M., Ray D. L., Stone A. E., et al. (2015). Behavioral and physiological changes around estrus events identified using multiple automated monitoring technologies. J. Dairy Sci. 98, 8723–8731. doi: 10.3168/jds.2015-9645
Felton C. A., Colazo M. G., Ponce-Barajas P., Bench C. J., Ambrose D. J. (2012). Dairy cows continuously-housed in tie-stalls failed to manifest activity changes during estrus. Can. J. Anim. Sci. 92, 189–196. doi: 10.4141/cjas2011-134
Friggens N. C., Bjerring M., Ridder C., Højsgaard S., Larsen T. (2008). Improved detection of reproductive status in dairy cows using milk progesterone measurements. Reprod. Dom. Anim. 43, 113–121. doi: 10.1111/j.1439-0531.2008.01150.x
Hansen P. J., Aréchiga C. F. (1999). Strategies for managing reproduction in the heat-stressed dairy cow. J. Anim. Sci. 77, 36–50. doi: 10.2527/1997.77suppl_236x
Haslwanter T. (2018). Measurement techniques, in: 3D kinematics (Upper, Austria: Springer International). doi: 10.1007/978-3-319-75277-8
Hulley S. B., Cummings S. R., Browner W. S., Grady D., Newman T. B. (2013). Designing clinical research: an epidemiologic approach. 4th ed (Philadelphia, PA: Lippincott Williams & Wilkins).
Jelinski M. D., LeBlanc S., Kennedy R. (2015). Demographics of the Canadian dairy industry from 1991 to 2011. Can. Vet. J. 56, 701–708.
Jensen P. (2017). “Sexual behavior,” in The ethology of domestic animals: an introductory text (Linköping, Sweden: CAB).
Kerbrat K., Disenhaus C. (2004). A proposition for an updated behavioural characterization of the oestrus period in dairy cows. Appl. Anim. Behav. Sci. 87, 223–238. doi: 10.1016/j.applanim.2003.12.001
Knızkova I., Kunc P., Koubkova M., Flusser J., Dolezal O. (2002). Evaluation of naturally ventilated dairy barn management by a thermographic method. Lives. Prod. Sci. 77, 349–353. doi: 10.1016/S0301-6226(02)00062-3
Kowsar R., Ghoribani G. R., Alikhani M., Khorvash M., Nikkhah A. (2008). Corn silage partially replacing short alfalfa hay to optimaze forage use in total mixed rations for lactating cow. J. Dairy Sci. 91, 4755–4764. doi: 10.3168/jds.2008-1302
Kyle B. L., Kennedy A. D., Small J. A. (1998). Measurement of vaginal temperature by radiotelemetry for the prediction of estrus in beef cows. Theriogenology 49, 1437–1449. doi: 10.1016/S0093-691X(98)00090-9
LeBlanc S. (2005). Overall reproductive performance of Canadian dairy cows: challenges we are facing. Adv. Dairy Technol. 17, 137–148.
Lopez H., Satter L. D., Wiltbank M. C. (2004). Relationship between level of milk production and estrous behavior of lactating dairy cows. Anim. Reprod. Sci. 81, 209–223. doi: 10.3168/jds.S0022-0302(05)72958-1
Lopez H., Caraviello D. Z., Satter L. D., Fricke P. M., Wiltbank M. C. (2005). Relationship between level of milk production and multiple ovulations in lactating dairy cows. J. Dairy Sci. 88, 2783–2793. doi: 10.3168/jds.S0022-0302(05)72958-1
Madureira A. M., Silper B. F., Burnett T. A., Polsky L., Cruppe L. H., Veira D. M., et al. (2015). Factors affecting expression of estrus measured by activity monitors and conception risk of lactating dairy cows. J. Dairy Sci. 98, 7003–70014. doi: 10.3168/jds.2015-9672
Maekawa M., Beauchemin K. A., Christensen D. A. (2002). Chewing activity, saliva production, and ruminal pH of primiparous and multiparous lactating dairy cows. J. Dairy Sci. 85, 1176–1182. doi: 10.3168/jds.S0022-0302(02)74180-5
National Research Council (2001). Nutrient requirements of dairy cattle (Washington: The National Academies Press). doi: 10.17226/9825
Pahl C., Hartung E., Mahlkow-Nerge K., Haeussermann A. (2015). Feeding characteristics and rumination time of dairy cows around estrus. J. Dairy Sci. 98, 148–154. doi: 10.3168/jds.2014-8025
Perez Marquez H. J., Ambrose D. J., Schaefer A. L., Cook N. J., Bench C. J. (2019). Infrared thermography and behavioral biometrics associated with estrus indicators and ovulation in estrus-synchronized dairy cows housed in tiestalls. J. Dairy Sci. 102, 4427–4440. doi: 10.3168/jds.2018-15221
Pfeiffer J., Gandorfer M., Ettema J. F. (2020). Evaluation of activity meters for estrus detection: a stochastic bioeconomic modeling approach. J. Dairy Sci. 103, 492–506. doi: 10.3168/jds.2019-17063
Price E. O. (2008). Principles and applications of domestic animal behavior: an introductory text (MA.CAB International: Cambridge).
Reith S., Hoy S. (2012). Relationship between daily rumination time and estrus of dairy cows. J. Dairy Sci. 95, 6416–6420. doi: 10.3168/jds.2012-5316
Roelofs J. B., Kemp B., Soede N. M., van Eerdenburg F. J. C. M. (2005). Various behavioral signs of estrous and their relationship with time of ovulation in dairy cattle. Theriogenology 63, 1366–1377. doi: 10.1016/j.theriogenology.2004.07.009
Rorie R. W., Bilby T. R., Lester T. D. (2002). Application of electronic estrus detection technologies to reproductive management of cattle. Theriogenology 57, 137–148. doi: 10.1016/S0093-691X(01)00663-X
Sanderink F. E. P., Gerritsen J. W., Groot Koerkamp P. W. G., Mourik S. V. (2017). Automatic detection of oestrus cows via breath sampling with an electronic nose: a pilot study. Biosystem Eng. 156, 1–6. doi: 10.1016/j.biosystemseng.2017.01.004
Sauls J. A., Voelz E. E., Hill S. L., Mendonça L. G. D., Stevenson J. S. (2017). Increasing estrus expression in the lactating dairy cows. J. Dairy Sci. 100, 807–820. doi: 10.3168/jds.2016-11519
Schweinzer V., Gusterer E., Kanz P., Krieger S., Süss D., Lidauer L., et al. (2019). Evaluation of an ear-attached accelerometer for detecting estrus events in indoor housed dairy cows. Theriogenology 130, 19–25. doi: 10.1016/j.theriogenology.2019.02.038
Stevenson J. S. (2021). Daily activity measures and milk yield immediately before and after a fertile estrus and during the period of expected return to estrus after insemination in dairy cows. J. Dairy Sci. 104, 11277–11290. doi: 10.3168/jds.2021-20325
Sveberg G., Refsdal A. O., Erhard H. W., Kommisrud E., Aldrin M., Tvete I. F., et al. (2011). Behavior of lactating Holstein-Friesian cows during spontaneous cycles of estrus. J. Dairy Sci. 94, 1289–1301. doi: 10.3168/jds.2010-3570
Talukder S., Kerrisk K. L., Ingenhoff L., Thomson P. C., Garcia S. C., Celi P. (2014). Infrared technology for estrus detection and as a predictor of time of ovulation in dairy cows in a pasture-based system. Theriogenology 81, 925–935. doi: 10.1016/j.theriogenology.2014.01.009
Valenza A., Giordano J. O., Lopes J. G., Vincenti L., Amundson M. C., Fricke P. M. (2012). Assessment of an accelerometer system for detection of estrus and treatment with gonadotropin-releasing hormone at the time of insemination in lactating dairy cows. J. Dairy Sci. 12, 7115–7127. doi: 10.3168/jds.2012-5639
Van Vliet J. H., van Eerdenburg F. J. C. M. (1996). Sexual activities and oestrus detection in lactating Holstein cows. Appl. Anim. Behav. Sci. 50, 57–69. doi: 10.1016/0168-1591(96)01068-4
Walton J. S., King G. J. (1986). Indicators of estrus in Holstein cows housed in tie stalls. J. Dairy Sci. 69, 2966–2973. doi: 10.3168/jds.S0022-0302(86)80754-8
Zuluaga J. F., Saldarriaga J. P. D., Cooper A., Cartmill J. A., Williams G. L. (2008). Evaluation of vaginal electrical resistance as an indicator of follicular maturity and suitability for timed artificial insemination in beef cows subjected to a synchronization of ovulation protocol. Anim. Reprod. Sci. 109, 17–26. doi: 10.1016/j.anireprosci.2007.10.002
Keywords: bio-sensors, estrus detection, activity, ear-temperature, dairy cattle, estrus-behavior, milk progesterone
Citation: Perez Marquez HJ, Ambrose DJ and Bench CJ (2023) Behavioral changes to detect estrus using ear-sensor accelerometer compared to in-line milk progesterone in a commercial dairy herd. Front. Anim. Sci. 4:1149085. doi: 10.3389/fanim.2023.1149085
Received: 21 January 2023; Accepted: 03 July 2023;
Published: 03 August 2023.
Edited by:
Marcia Endres, University of Minnesota Twin Cities, United StatesReviewed by:
Michael Iwersen, University of Veterinary Medicine Vienna, AustriaMiel Hostens, Utrecht University, Netherlands
Copyright © 2023 Perez Marquez, Ambrose and Bench. This is an open-access article distributed under the terms of the Creative Commons Attribution License (CC BY). The use, distribution or reproduction in other forums is permitted, provided the original author(s) and the copyright owner(s) are credited and that the original publication in this journal is cited, in accordance with accepted academic practice. No use, distribution or reproduction is permitted which does not comply with these terms.
*Correspondence: Hector J. Perez Marquez, cGVyZXptYXJAdWFsYmVydGEuY2E=