- School of Animal Sciences, Virginia Tech, Blacksburg, VA, United States
This study sought to evaluate the accuracy of a PPG (photoplethysmography) sensor designed to measure human heart rates in monitoring the distal limb pulse of healthy adult horses. We hypothesized that the PPG sensor is sensitive to placement location and orientation, and that measurement accuracies depend on placement and orientation on the limb. To evaluate this hypothesis, a completely randomized block design with a factorial treatment structure was used. Horses were considered as the block. Limb type (right front, left front, right hind, and left hind) and position of sensor (medial or lateral) were treatments, with levels arranged in a complete (4x2) factorial design. Data were collected by placing the PPG sensor on the limb of each horse (n = 6), with placement location according to the treatment (limb type and location) combination, and taking pulse readings for 60 seconds. Manual heart rates were collected concurrently using a stethoscope. Data were analyzed by calculating root mean square errors (RMSE) for the PPG measurements with the manual heart rates as a gold standard. Variation in RMSE associated with limb and location of sensor were evaluated using a general linear model with fixed effects for limb and location and a random effect for horse. Our results indicated that the PPG sensor was ineffective at measuring horse heart rates, and that the device was insensitive to placement location and orientation. Future work should focus on developing alternative analytics to interpret the data from PPG sensors to better reflect horse heart rates.
1 Introduction
The application of wearable technologies in animal health management is gradually increasing in popularity (Halachmi et al., 2019; Neethirajan and Kemp, 2021). These sensor devices, if designed well and implemented properly, can help detect physiological alterations that could indicate disease, potentially decreasing economic loss for owners and producers. Unlike manual measurements which are labor-intensive and may vary in accuracy due to the evaluator’s training or lack thereof, an ideal sensor technology should provide reliable, continuous, and real-time data reflecting the physiological condition of an individual animal (Neethirajan, 2017). Sensor technology has shown to be successful in helping improve the welfare of poultry (Zhuang et al., 2018), pigs (D’Eath et al., 2018), and sheep (McLennan and Mahmoud, 2019). Useful applications of sensor technology have also been demonstrated in detecting gait abnormalities and disruptions in eating behavior of dairy cows (Beer et al., 2016; Alsaaod et al., 2019; Eckelkamp, 2019). Despite these successes in livestock species, wearable technologies for the equine industry are still comparatively limited. This may be due, in part, to challenges with the practical applicability of sensor technologies, which can include insufficient sample sizes, technical or equipment issues, and high sensitivity to external factors (e.g., weather variables, presence of hair, and between-day variation) (Egan et al., 2019). Alternatively, the slow adoption could be because horses are still largely managed as individuals that are (typically) handled and visually evaluated by their owners or other personnel on a daily basis. Therefore, the perception may exist that sensor technologies would be fairly unnecessary in this type of management situation.
One area where sensor technology may be particularly useful to the equine industry is in heart rate detection. This is because heart rates are not easily observed visually, and rapid fluctuation of physiological importance may occur in the short hours between human interaction. Examples of beneficial applications include monitoring of recovery from physical exertion (Flethøj et al., 2016) and detecting responses to physical or emotional stress (von Borell et al., 2007). Heart rate monitoring technologies for horses exist; however, they typically are focused on core heart rate determination through sensors attached to equipment leveraged during exercise (Nery et al., 2018; Williams et al., 2019). The Polar Equine heart rate monitor has been validated during exercise and at rest (McDuffee et al., 2019; Frippiat et al., 2021); however, it is fairly expensive and requires the horse to wear a band around the thorax much like a girth. Although the majority of currently available technologies work well for exercise investigation, besides the Polar system, they are not feasible for long-term, continuous heart rate monitoring. Photoplethysmography (PPG) technology has been used to create small, simple, and affordable heart rate sensors that are well-suited to this continuous monitoring application in cattle (Salzer et al., 2022), sheep (Cui et al., 2019), and pigs (Youssef et al., 2020). Since fitness is important in the equine industry, a continuous heart rate monitor may also be useful in exercise settings, much like its use in humans for fitness tracking during workouts as well as day-to-day monitoring. These PPG sensors typically use visual and infrared light in the wavelength range of around 600 to 900 nm to measure blood volume changes in tissue beds to determine heart rate and pulse oximetry (Tamura et al., 2014; Välisuo, 2015). Unlike electrocardiograms (ECG) that use electrodes on patient chests, PPG technology is non-invasive and low-cost. As such, evaluation of the opportunities to leverage PPG technology for equine heart rate detection is needed to understand how placement and preparation of equine PPG sensors may influence the accuracy of this technology in detecting horse pulse rates.
The objective of the study was to assess the ability of a wearable PPG-based sensor originally designed for human heart rate detection to accurately monitor the distal limb pulse of healthy adult horses. We hypothesized that the PPG sensor would be sensitive to placement location and orientation, and that measurement accuracies would depend on sensor placement and orientation.
2 Materials and methods
2.1 Animals, husbandry, and data collection
All procedures in this study were approved by the Virginia Tech Institutional Animal Care and Use Committee. Six randomly selected horses were used from Virginia Tech’s collegiate equestrian program herd. Horses ranged in age from 9 to 22. The sample of horses included geldings (n=5) and a mare (n=1) of various breeds including two thoroughbreds, two appendix quarter horses, one warmblood, and one Morab. Prior to enrollment, all horses in the study were determined to be clinically healthy with no known or observed signs of cardiac abnormalities. All animals were housed in Virginia Tech barn facilities, were given water ad libitum, and offered diets comprised of commercial grain mixes, grass hay, and grass pasture that were formulated to meet or exceed requirements (NRC, 2007).
One research assistant attached the PPG-based heart rate sensor on each given experimental day to avoid inconsistencies in technique. Evaluations were conducted between the hours of 0900 h and 1500 h in the facility where the horses were housed over a period of 10 days. During data collection, each horse was restrained by cross ties to restrict large amounts of movement. Prior to data collection, all horses had an acclimation period during which a fetlock exercise boot was placed around their limb. This exercise boot was similar in size and attachment method to the PPG-based heart rate sensor and was used to discourage the horses from reacting excessively to the PPG-based sensor, allowing for more consistent data collection. The PPG sensor device was positioned just above the fetlock on the lateral or medial side of each limb (Figure 1) in order to measure the distal limb pulse for the duration of data collection. Hair on the horses’ limbs was left unshaved. Eight readings were taken per horse per day: one reading for the medial and lateral side of each leg. Each reading was taken for 60 seconds. Over the course of 10 days, each horse was subjected to a total of 80 readings: 20 readings per limb with 10 readings on each side of the limb. The heart rate measured by the sensor was compared with the heart rate measured by a trained technician with a stethoscope at concurrently with each sensor recording.
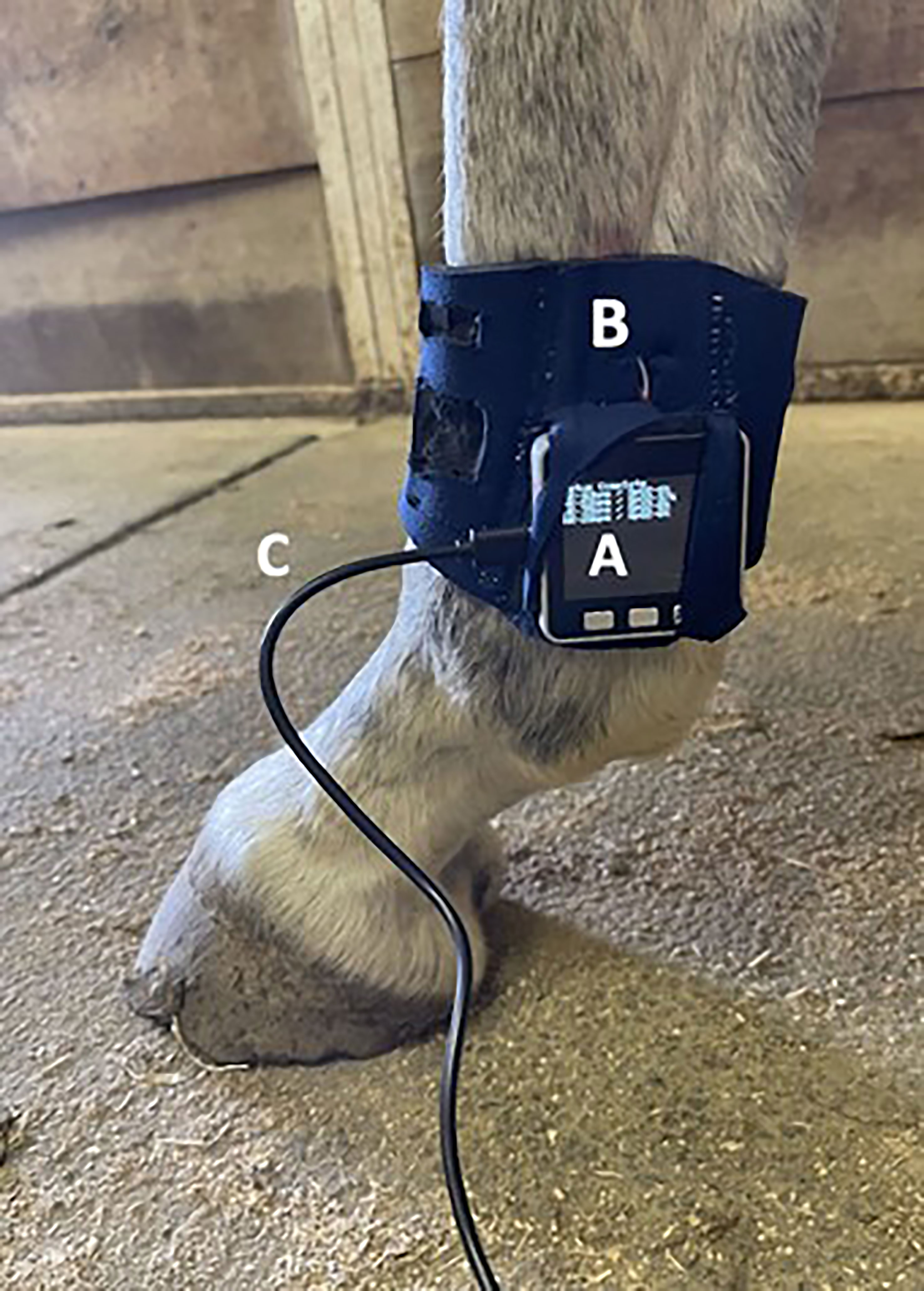
Figure 1 The PPG-based heart rate sensor attached to a hind limb during data collection. (A) M5Stack GRAY kit (M5Stack, Guangdong, China), which has an ESP-32 core and integrated OLED screen used for data integration and visualization. (B) Attachment of the sensor to the horse using flexible neoprene with a Velcro strap. (C) USB charging cable connected to an external battery source.
2.2 Sensor description
To avoid a rigid structure that could interfere with capillary pressure, our monitoring system (Figure 1) was constructed using flexible neoprene with Velcro attachments. This made the PPG sensor system lightweight, compact, and sturdy. The tightness of application was industry standard for a boot, and was as close to uniform as possible among animals. We used a MAX30101 (Maxim Integrated, San Jose, California) health sensor platform with optical elements, photodetectors, internal LEDs, and low-noise electronics with light rejection for PPG sensing. This platform utilizes a triple-wavelength PPG sensor with green (537 nm), red (660 nm), and infrared (880 nm) emission wavelengths (Maxim Integrated, 2020). The sensor operates by emitting light from the LEDs which is altered by blood pulsation under the skin. The reflected light wave is then captured by the photodetectors and translated into an electrical charge (Brazhnikova et al., 2022). Greater blood volume would cause more light scattering, which would result in a proportionally greater voltage output (Välisuo, 2015). In this study, the electrical signals obtained from the sensor were integrated, analyzed, and displayed using an M5Stack GRAY kit (M5Stack, Guangdong, China), which has an ESP-32 core and integrated OLED screen used for data integration and visualization, respectively. Data was stored directly to an SD card.
2.3 Statistical analysis
All data were analyzed in R version 4.1.2 (R Core Team, 2021). Accuracy of the sensor in estimating heart rate was assessed by way of the root mean square error (RMSE) and RMSE-observations standard deviation ratio (RSR) that are given as follows:
where N is the total number of observations, and and are the estimated heart rates measured by the heart rate sensor and stethoscope, respectively. Both the overall RMSE and RSR, as well as RMSE and RSR for individual limb and position measurement units were evaluated. Variation in RMSE associated with limb and location of sensor was evaluated using a general linear model with fixed effects for limb and location and a random effect for horse. Significance was declared at P< 0.05.
To better evaluate whether the sensor system was effective at classifying horse heart rates (e.g., low, normal, high), we classified both the measured and sensed heart rates based on whether they fell within the normal range of 28 to 40 beats per minute (Scott and Martin, 2016), above, or below the normal range using a random forest algorithm (Liaw and Wiener, 2002). A confusion matrix was generated to evaluate these responses using the caret package (Kuhn, 2022) and five metrics were calculated per class to evaluate the quality of the sensor classification. These metrics are given as follows:
where TP is the number of true positives, TN is the number of true negatives, FP is the number of false positives, and FN is the number of false negatives.
3 Results and discussion
We evaluated the accuracy of a wearable PPG sensor designed to detect human heart rates in terms of its ability to detect heart rates of adult horses. The sensor system leveraged was designed to be non-invasive, compact, and low-cost. The current costs for the MAX30101 health system platform ($5.00) and M5 Stack GRAY kit used for data integration and visualization ($45.00) show promise for the future application of these devices to be affordable to a range of consumers. Despite the potential promise in terms of affordability, however, the PPG-based sensor was ineffective at measuring horse heart rates (Table 1). This demonstrates that although similarly designed PPG heart rate sensors have been applied in other species like cattle (Jun et al., 2013) and swine (Youssef et al., 2020), using this technology in the equine industry will require further work. In particular, specific tailoring of the algorithms to interpret voltage readings from the PPG sensor in terms of actual pulses of blood though horse capillary beds will be necessary to enhance the effectiveness of this technology for equine subjects. The poor coherence between the sensor readings and the measured heart rates is underpinned by our global RMSE (69.3%), which suggested the sensor had high error compared with measured value. In addition to the challenges associated with interpreting sensor signals in a species-specific manner, some studies indicate that poor accuracy when using PPG sensors on animals could be attributed to the inability to keep good contact between the sensor and animal (Youssef et al., 2020). Improvements on sensor attachment for horses could therefore also be an important future research objective to consider.
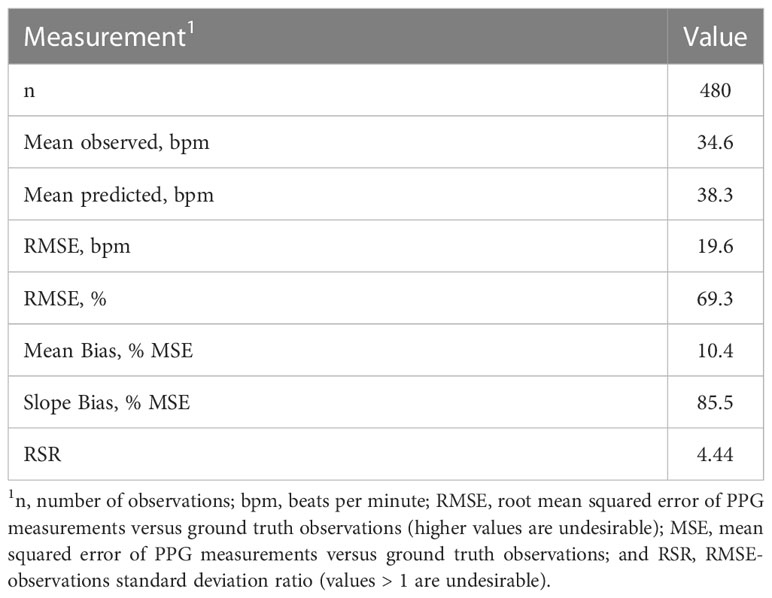
Table 1 Descriptive statistics for the comparison of sensor-measured heart rates and heart rates determined manually.
In addition to poor overall coherence with measured heart rates, the accuracy of our PPG sensing device was not sensitive to lateral or medial placement on the limb (Table 2). Sensor-measured and manually-measured heart rates averaged by sensor orientation and limb are located in Supplementary Table S1. Although we had postulated that PPG sensing would be sensitive to placement, our results suggest that the sensor was ineffective at determining heart rates even when placed in different orientations on the limb. Given that PPG sensors are difficult to apply in environments that are not controlled, it was expected that the sensor readings, and thereby sensor accuracy, would be sensitive to external factors such as placement location and limb type (i.e., front versus hind). However, it is possible that the sensitivity of the sensor to these factors was masked by the poor overall accuracy of the readings and the high variability among sensor measurements. Other studies of the use of PPG sensors on non-anesthetized and anesthetized animals help to further describe this finding. For example, sensors used on anesthetized pigs in controlled environments had less motion artifacts in heart rate data compared to those not anesthetized (Youssef et al., 2020). As such, the high variability among readings observed in this study could be partially due to motion of the non-anesthetized horses, which do fidget frequently, even when calmly standing during restraint. Accounting for variability induced by horse motion is a challenge that must be addressed by further research if PPG technology is going to be used as a viable heart rate monitoring strategy for these animals. Successes in heart rate monitoring during exercise, both in human technologies leveraging PPG sensing, and in existing horse technologies, suggest that with improved data analytics, there will be opportunity to address this challenge (Hebenbrock et al., 2005; Weiler et al., 2017).
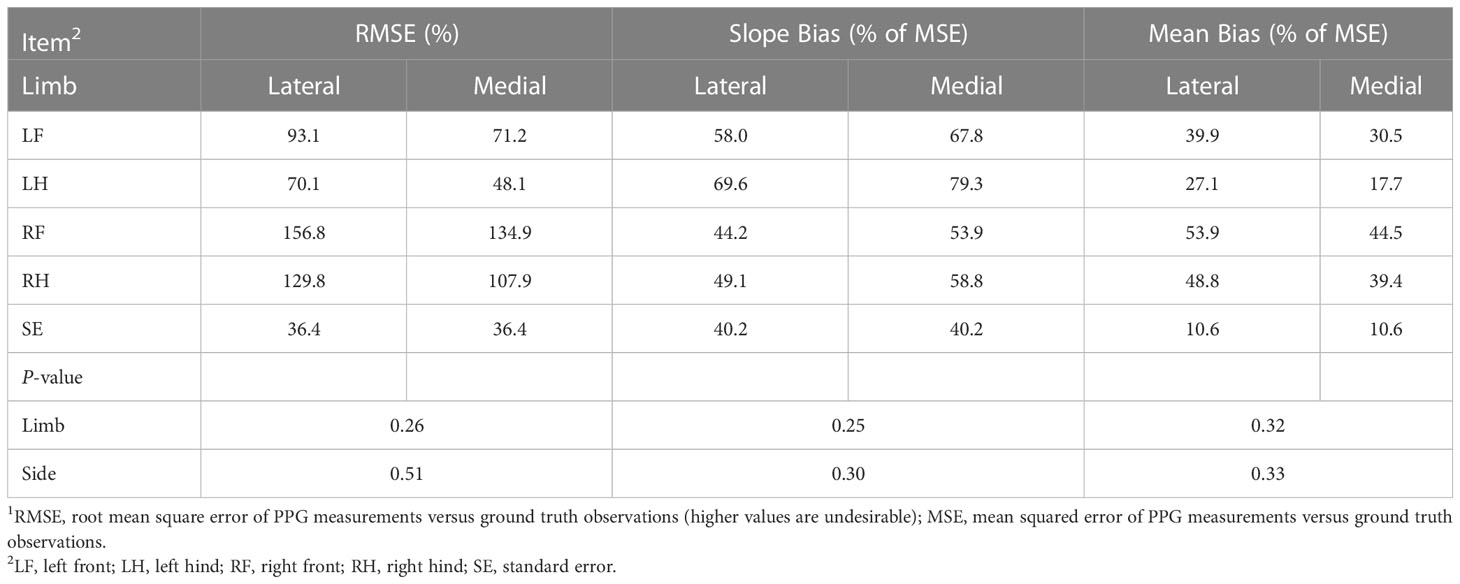
Table 2 Fit statistics for the comparison of sensor-measured heart rates and heart rates determined manually when stratified by limb and positioning on the limb1.
Although prediction of exact heart rates is an ideal goal for PPG sensing, a minimum viable performance level would require the ability to identify when a horse’s heart rate extends outside the normal range. For example, sensing that the heart rate is 85 beats per minute may be only marginally more useful than being able to determine that the horse’s heart rate is elevated. We therefore additionally evaluated whether the heart rate classifications (low, normal, high) by the sensor had improved coherence with measured values. Unfortunately, a high error rate was associated with the sensor’s attempts to distinguish between low, normal, and high heart rates (Table 3). As such, the precision, accuracy, and sensitivity of heart rate classification data were poor (Table 4). The classification-based analysis suggested that the PPG sensor, in its current form, was not able to reliably classify heart rates as normal, low, or high. This finding further supports the need for more horse-specific analytics to interpret and understand PPG data obtained from equines. PPG sensors for humans are designed to measure through skin layers that average 1.2 mm (Southwood, 1955). Unlike humans, horse skin layers are much thicker and can be up to 7.0 mm (Wakuri et al., 1995). This increase in thickness, and the addition of a hair layer, may dampen the absorbance of light occurring with capillary bed filling, meaning that the signals obtained by the PPG sensor readings in horses may be decreased compared to humans. Other studies indicating high accuracy with PPG sensor technology on animals may be due to more anatomical and physiological similarities of their subjects to humans. For instance, it has been reported that the orientation, thickness, and vessel distribution in the porcine dermis is like that of humans which could contribute to better measurement accuracy in pigs (Nie et al., 2020).
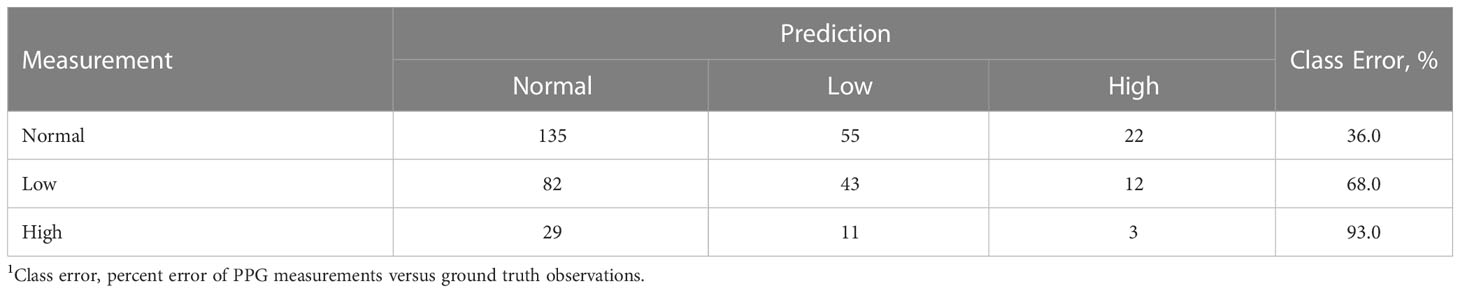
Table 3 Confusion matrix for classification of heart rate data given as numbers of observations obtained from a sensor classified into categorical responses reflecting “Normal”, “Low”, and “High” heart rates1.

Table 4 Confusion matrix metrics summary for heart rate data obtained from a sensor classified into categorical responses reflecting “Normal”, “Low”, and “High” heart rates1.
The readings within this study were, on average, higher than those obtained with the stethoscope (Table 1), suggesting more peaks within the data being identified as heart beats than were actually occurring. This elevated number of peaks could reflect higher variability due to motion, sensor movement or slippage, or variability induced by the thicker skin and presence of hair (dos Reis and White, 2022). Future work should isolate these factors and attempt to train better analytics to convert PPG readings to reliable and robust measurement of equine heart rates.
While our experiment was modeled after successful evaluations of heart rate sensors in other species that utilized 1 to 6 animals (Cui et al., 2019; Youssef et al., 2020; Salzer et al., 2022), the sampling size used (n = 6) may be considered as a limitation of this work. When examined on a per animal basis, it was observed that the sensor readings resulted in greater residual error for some horses compared to others (Supplementary Table S2). High between-animal variation has been encountered with heart rate sensors in equines previously (von Borell et al., 2007). Larger sample sizes for future work would be advisable, but more meaningful improvement of the PPG sensor performance in horses will likely occur through better adaptation of the technology itself for this species. A major aim of the present study was to highlight that the manufacturer algorithms used by the PPG sensor are insufficient for application in horses. Because we were only able to access the heart rate data post-hoc, analysis of the raw data generated by the sensor would be a beneficial next step in this investigation.
4 Conclusions
This study evaluated an inexpensive human heart rate sensor in terms of its ability to detect heart rates of healthy adult horses at rest. Although simplistic, practical, and affordable, the designed sensor was ineffective in accurately measuring horse heart rates. This could be due, in part, to challenges with the practical applicability of sensor technologies, including sensitivity to environmental noise and animal movements. The differences in horse anatomy compared with humans and other similar livestock species could also have contributed to the sensor’s poor performance. Future endeavors of designing PPG sensors that can accurately detect horse heart rates should focus on better and more robust data analytics to translate PPG signals obtained from horse measurements into heart rate observations.
Data availability statement
The raw data supporting the conclusions of this article will be made available by the authors, without undue reservation.
Ethics statement
The animal study was reviewed and approved by the Virginia Tech Institutional Animal Care and Use Committee.
Author contributions
SN was responsible for animal monitoring, data collection, data analysis, and manuscript preparation. CG was responsible for manuscript editing. CL was responsible for experimental design and student supervision. RW guided data analysis and contributed to manuscript editing. All authors contributed to the article and approved the submitted version.
Funding
This research was supported by funding from the U.S. Department of Agriculture—National Institute of Food and Agriculture (2019-67021-29007 and 2018-67007-28452) and the John Lee Pratt Fellowship of Virginia Tech.
Conflict of interest
The authors declare that the research was conducted in the absence of any commercial or financial relationships that could be construed as a potential conflict of interest.
Publisher’s note
All claims expressed in this article are solely those of the authors and do not necessarily represent those of their affiliated organizations, or those of the publisher, the editors and the reviewers. Any product that may be evaluated in this article, or claim that may be made by its manufacturer, is not guaranteed or endorsed by the publisher.
Supplementary material
The Supplementary Material for this article can be found online at: https://www.frontiersin.org/articles/10.3389/fanim.2023.1103812/full#supplementary-material
References
Alsaaod M., Fadul M., Steiner A. (2019). Automatic lameness detection in cattle. Vet. J. 246, 35–44. doi: 10.1016/j.tvjl.2019.01.005
Beer G., Alsaaod M., Starke A., Schuepbach-Regula G., Müller H., Kohler P., et al. (2016). Use of extended characteristics of locomotion and feeding behavior for automated identification of lame dairy cows. PLoS One 11, e0155796. doi: 10.1371/journal.pone.0155796
Brazhnikova A. K., Semenova A. N., Zyulin V. A., Denisova O. V. (2022). “Determination of pulse oximetric parameters of the body by photoplethysmography,” in 2022 Conference of Russian Young Researchers in Electrical and Electronic Engineering (ElConRus) (IEEE). Saint Petersburg, Russian Federation: Institute of Electrical and Electronics Engineers 123–126. doi: 10.1109/ElConRus54750.2022.9755665
Cui Y., Zhang M., Li J., Luo H., Zhang X., Fu Z. (2019). WSMS: Wearable stress monitoring system based on IoT multi-sensor platform for living sheep transportation. Electronics 8, 441. doi: 10.3390/electronics8040441
D’Eath R. B., Jack M., Futro A., Talbot D., Zhu Q., Barclay D., et al. (2018). Automatic early warning of tail biting in pigs: 3D cameras can detect lowered tail posture before an outbreak. PLoS One 13, e0194524. doi: 10.1371/journal.pone.0194524
dos Reis B. R., White R. R. (2022). Brief research report: evaluation of photoplethysmographic heart rate monitoring for sheep under heat-stressed conditions. Front. Anim. Sci. 3, 1046557. doi: 10.3389/fanim.2022.1046557
Eckelkamp E. A. (2019). Invited review: current state of wearable precision dairy technologies in disease detection. Appl. Anim. Sci. 35, 209–220. doi: 10.15232/aas.2018-01801
Egan S., Brama P., McGrath D. (2019). Research trends in equine movement analysis, future opportunities and potential barriers in the digital age: a scoping review from 1978 to 2018. Equine Vet. J. 51, 813–824. doi: 10.1111/evj.13076
Flethøj M., Kanters J. K., Haugaard M. M., Pedersen P. J., Carstensen H., Balling J. D., et al. (2016). Changes in heart rate, arrhythmia frequency, and cardiac biomarker values in horses during recovery after a long-distance endurance ride. J. Am. Vet. Med. Assoc. 248, 1034–1042. doi: 10.2460/javma.248.9.1034
Frippiat T., van Beckhoven C., Moyse E., Art T. (2021). Accuracy of a heart rate monitor for calculating heart rate variability parameters in exercising horses. J. Equine Vet. Sci. 104, 103716. doi: 10.1016/j.jevs.2021.103716
Halachmi I., Guarino M., Bewley J., Pastell M. (2019). Smart animal agriculture: application of real-time sensors to improve animal well-being and production. Annu. Rev. Anim. Biosci. 7, 403–425. doi: 10.1146/annurev-animal-020518-114851
Hebenbrock M., Düe M., Holzhausen H., Sass A., Stadler P., Ellendorff F. (2005). A new tool to monitor training and performance of sport horses using global positioning system (GPS) with integrated GSM capabilities. DTW Dtsch. Tierarztl. Wochenschr. 112, 262–265.
Jun H.-B., Kim H.-J., Kim J.-O. (2013). Development of pulse measurement method for health monitoring of dairy cows. J. Korea Contents Assoc. 13, 27–37. doi: 10.5392/JKCA.2013.13.12.027
Kuhn M. (2022) Caret: Classification and regression training. Available at: https://CRAN.R-project.org/package=caret.
Liaw A., Wiener M. (2002). Classification and regression by randomForest. R News 2, 18–22. Available at: https://www.r-project.org/doc/Rnews/Rnews_2002-3.pdf
Maxim Integrated (2020) MAX30101: High-sensitivity pulse oximeter and heart-rate sensor for wearable health. Available at: https://datasheets.maximintegrated.com/en/ds/MAX30101.pdf (Accessed February 15, 2023).
McDuffee L., Mills M., McNiven M., Montelpare W. (2019). Establishing statistical stability for heart rate variability in horses. J. Vet. Behav. 32, 30–35. doi: 10.1016/j.jveb.2019.05.003
McLennan K., Mahmoud M. (2019). Development of an automated pain facial expression detection system for sheep (Ovis aries). Anim. Open Access J. MDPI 9, E196. doi: 10.3390/ani9040196
Neethirajan S. (2017). Recent advances in wearable sensors for animal health management. Sens. Bio-Sens. Res. 12, 15–29. doi: 10.1016/j.sbsr.2016.11.004
Neethirajan S., Kemp B. (2021). Digital livestock farming. Sens. Bio-Sens. Res. 32, 100408. doi: 10.1016/j.sbsr.2021.100408
Nery J., Racioppi F., Valle E., Assenza A., Bergero D. (2018). Proof of concept on energy expenditure assessment using heart rate monitoring and inertial platforms in show-jumping and riding school horses. J. Equine Vet. Sci. 61, 1–6. doi: 10.1016/j.jevs.2017.10.014
Nie L., Berckmans D., Wang C., Li B. (2020). Is continuous heart rate monitoring of livestock a dream or is it realistic? a review. Sensors 20, 2291. doi: 10.3390/s20082291
R Core Team (2021) R: A language and environment for statistical computing. Available at: https://www.R-project.org/.
Salzer Y., Lidor G., Rosenfeld L., Reshef L., Shaked B., Grinshpun J., et al. (2022). Technical note: a nose ring sensor system to monitor dairy cow cardiovascular and respiratory metrics. J. Anim. Sci. 100, skac240. doi: 10.1093/jas/skac240
Scott B., Martin M. (2016). Understanding vital life signs in horses. TAMU AgriLife Ext., 1–5. Available at: https://texashelp.tamu.edu/wp-content/uploads/2016/02/understanding-vital-life-signs-in-horses.pdf
Southwood W. F. (1955). The thickness of the skin. Plast. Reconstr. Surg. 15, 423–429. doi: 10.1097/00006534-195505000-00006
Tamura T., Maeda Y., Sekine M., Yoshida M. (2014). Wearable photoplethysmographic sensors–past and present. Electronics 3, 282–302. doi: 10.3390/electronics3020282
Välisuo P. (2015). “Optical methods for assessing skin flap survival,” in Biophotonics for medical applications woodhead publishing series in biomaterials. Ed. Meglinski I. V. (Sawston, Cambridge, UK: Woodhead Publishing), 331–346.
von Borell E., Langbein J., Després G., Hansen S., Leterrier C., Marchant-Forde J., et al. (2007). Heart rate variability as a measure of autonomic regulation of cardiac activity for assessing stress and welfare in farm animals — a review. Physiol. Behav. 92, 293–316. doi: 10.1016/j.physbeh.2007.01.007
Wakuri H., Mutoh K., Ichikawa H., Liu B. (1995). Microscopic anatomy of the equine skin with special reference to the dermis. Okajimas Folia Anat. Jpn. 72, 177–183. doi: 10.2535/ofaj1936.72.2-3_177
Weiler D. T., Villajuan S. O., Edkins L., Cleary S., Saleem J. J. (2017). Wearable heart rate monitor technology accuracy in research: a comparative study between PPG and ECG technology. Proc. Hum. Factors Ergon. Soc Annu. Meet. 61, 1292–1296. doi: 10.1177/1541931213601804
Williams J., Kenworthy K., Jones T., Marlin D., Tabor G. (2019). The role of heart rate monitoring to assess workload during maintenance interval training in national hunt racehorses. J. Vet. Behav. 30, 54–60. doi: 10.1016/j.jveb.2018.12.003
Youssef A., Peña Fernández A., Wassermann L., Biernot S., Wittauer E.-M., Bleich A., et al. (2020). An approach towards motion-tolerant PPG-based algorithm for real-time heart rate monitoring of moving pigs. Sensors 20, E4251. doi: 10.3390/s20154251
Keywords: health monitoring, heart rate, horses, precision technology, sensors
Citation: Naughton SG, Gleason CB, Leeth CM and White RR (2023) Brief research report: Photoplethysmography pulse sensors designed to detect human heart rates are ineffective at measuring horse heart rates. Front. Anim. Sci. 4:1103812. doi: 10.3389/fanim.2023.1103812
Received: 21 November 2022; Accepted: 13 March 2023;
Published: 21 March 2023.
Edited by:
Patrick Christian Pageat, Institut de Recherche en Sémiochimie et Ethologie Appliquée (IRSEA), FranceReviewed by:
Jose Antonio Diaz-Olivares, KU Leuven Campus Geel, BelgiumMalin Connysson, Swedish University of Agricultural Sciences, Sweden
Alessandro Cozzi, Institut de Recherche en Sémiochimie et Ethologie Appliquée (IRSEA), France
Copyright © 2023 Naughton, Gleason, Leeth and White. This is an open-access article distributed under the terms of the Creative Commons Attribution License (CC BY). The use, distribution or reproduction in other forums is permitted, provided the original author(s) and the copyright owner(s) are credited and that the original publication in this journal is cited, in accordance with accepted academic practice. No use, distribution or reproduction is permitted which does not comply with these terms.
*Correspondence: Robin R. White, cnJ3aGl0ZUB2dC5lZHU=