- 1Carrington Research Extension Center, North Dakota State University, Carrington, ND, United States
- 2Department of Animal and Ranges Sciences, New Mexico State University, Las Cruces, NM, United States
- 3Panhandle Research and Extension Center, University of Nebraska-Lincoln, Scottsbluff, NE, United States
- 4Institute for Future Farming Systems, School of Health, Medical, and Applied Sciences, CQUniversity, Rockhampton, QLD, Australia
- 5University of Maine Cooperative Extension, University of Main, Orono, ME, United States
Advances in technology have led to precision livestock management, a developing research field. Precision livestock management has potential to improve sustainable meat production through continuous, real-time tracking which can help livestock managers remotely monitor and enhance animal welfare in extensive rangeland systems. The combination of global positioning systems (GPS) and accessible data transmission gives livestock managers the ability to locate animals in arduous weather, track animal patterns throughout the grazing season, and improve handling practices. Accelerometers fitted to ear tags or collars have the potential to identify behavioral changes through variation in the intensity of movement that can occur during grazing, the onset of disease, parturition or responses to other environmental and management stressors. The ability to remotely detect disease, parturition, or effects of stress, combined with appropriate algorithms and data analysis, can be used to notify livestock managers and expedite response times to bolster animal welfare and productivity. The “Five Freedoms” were developed to help guide the evaluation and impact of management practices on animal welfare. These freedoms and welfare concerns differ between intensive (i.e., feed lot) and extensive (i.e., rangeland) systems. The provisions of the Five Freedoms can be used as a conceptual framework to demonstrate how precision livestock management can be used to improve the welfare of livestock grazing on extensive rangeland systems.
Introduction
Projections have shown that the world population will increase 2.9% annually to 9 billion people by the year 2050 (FAO, 2007; FAO, 2009). The increase in population will require an increase in food and fiber production of nearly 50%, with a need to increase meat production by 70%, roughly 200 million tonnes (OECD - FAO, 2018). Increasing animal production while maintaining and improving animal welfare will be necessary to fulfill consumer demand and expectations. However, there is resistance toward increasing the intensity of agricultural production to meet this growing demand for food because of concerns about animal welfare and environmental limitations.
Global grazing ruminant livestock populations, approximately 3.5 billion animals (FAOSTAT, 2020), are produced on 77% (40 million km2) of the total agricultural lands (51 million km2) (Ritchie and Roser, 2013) which includes both rangelands and croplands. Rangelands provide biological diversity, ecological function, carbon storage, and habitat for wild flora and fauna above livestock production (Lund, 2007). Extensive livestock operations, especially ruminant livestock such as cattle, sheep, and goats, throughout the world rely on pasturelands, rangelands, and forested lands, which are not suited for farming due to limited precipitation and/or poor tillage feasibility (Lund, 2007; Reeves and Mitchell, 2011; Bailey et al., 2021). The symbiotic relationship of micro-organisms and ruminants and non-ruminant herbivores (e.g., horses, camels and rabbits) allow these livestock species to utilize vegetation that contains significant levels of cellulose (Van Soest, 1994). Grasses, forbs and shrubs that are adapted to non-tillable semi-arid, arid, rocky and steep lands can be eaten by livestock and help feed the increasing human population. Livestock managed under extensive systems often forage on lands which are expansive, rugged, and/or mountainous (Bailey, 2016) and the ability for managers to evaluate the well-being and health of these grazing livestock is often limited. On such extensive landscapes, frequent observations are typically not practical because of large travel distances and rough terrain. Sensor technology, including real-time global positioning systems (GPS), can be used to improve a livestock managers’ ability to locate livestock and determine their well-being (Bailey et al., 2021).
Development and improvement of technologies has been key to increasing farm productivity (Balaine et al., 2020). Cameras, real-time GPS tracking, and sensors have been successfully used by livestock managers to remotely monitor livestock and detect animal well-being concerns (Herlin et al., 2021). Many sensors, such as accelerometers, are difficult for livestock managers to use in their management systems because they produce large and complex data sets that are difficult to process, analyze, interpret, which limits their ability to provide effective decision support tools (Werkheiser, 2018). Technology that remotely monitors livestock and implements that data into precision livestock management (PLM) schemes are quickly being developed at commercial levels for large- and small-scale livestock managers (Bailey et al., 2021).
Precision livestock management (PLM) has the potential to benefit both the livestock manager and the animal. Research focusing on PLM can improve our understanding of animal behavior changes facilitating management action before performance and well-being of the animal are compromised (Ikurior et al., 2020). The objective of PLM is to create “a management system based on continuous automatic real-time monitoring and control of production/reproduction, animal health and welfare, and the environmental impact of livestock production” (Berckmans, 2014). Termed “precision”, PLM provides livestock managers opportunities to improve data driven and proactive decisions, compared to traditional management where monitoring data are limited and management actions on extensive rangelands are typically reactionary. The motivation behind PLM is to observe the individual animal (fine-grained) rather than pens, buildings, or herds because the individual has needs (e.g., nutrition, health and physiological needs, etc.) that are different from the herd average (Werkheiser, 2018).
During the last 20 years, livestock grazing behavior research has been enhanced due to the development of GPS tracking (Bailey et al., 2018). Commercially available GPS tracking collars are often cost prohibitive to use within a commercial livestock operation with some costing up to $2,000 USD or more per unit (Anderson et al., 2013). However, relatively inexpensive GPS models are being developed with readily available GPS components that can be purchased off the shelf and retrofitted with larger batteries and homemade collars (McGranahan et al., 2018; Knight et al., 2018a; Karl and Sprinkle, 2019). Karl and Sprinkle (2019) built their collars for less than $60 USD per unit, but indicated that their design had some problems with reliability, battery life, and GPS fix frequency. The cost of tracking is not only the purchase price of the GPS unit, but the time and skill required to process data which can be time consuming and require unique expertise (Knight et al., 2018b). With improved technologies, increased availability, and reduced cost, cost-effective units can be used and more animals in a herd can be tracked, which increases the ability of livestock managers to monitor more individuals within commercial scale livestock herds. Until recently, GPS location data were usually only used in research settings with data stored on the device. Data storage on the device prevented access to information unit the GPS unit was removed from the animal, which may be only once or twice per year (Bailey et al., 2018). Greater access to data with LoRaWan technology and satellite transmission will improve the real-time nature and applicability of PLM technology (Casas et al., 2021). Livestock managers need frequent and accurate tracking data to utilize the information in their decision-making process.
In contrast to GPS that tracks movement, accelerometers have helped researchers monitor activity and behavior of sheep (Alvarenga et al., 2016; Guo et al., 2018; Barwick et al., 2018b; Fogarty et al., 2020a), beef cattle (Poulopoulou et al., 2019; Sprinkle et al., 2021; Chang et al., 2022), and dairy cattle (Thorup et al., 2015; Bar et al., 2019; O’Leary et al., 2020). Accelerometers typically record animal movement in three axes (triaxial), measuring both gravitational and inertial acceleration (Watanabe et al., 2008; Brown et al., 2013; Alvarenga et al., 2016; Barwick et al., 2018b; Fogarty et al., 2020b). For example, accelerometers mounted to ear tags and halters can detect side to side (x axis), forward –aft (y axis) and up and down movements (z axis). Currently available accelerometers monitor these movements at 12 to 25 hertz (12 to 25 data points per second). Algorithms can integrate measurements of these three axes to create metrics such as intensity of movement, energy, pitch, and roll (Campbell et al., 2013; Alvarenga et al., 2016). These metrics may be more useful for predicting livestock behavior with machine learning, or other types of artificial intelligence, than the separate analysis of each axis (Fogarty et al., 2020c). While potential for using accelerometers is high in practice, researchers must continue to develop and refine accelerometer based algorithms used on extensive rangelands to identify and predict specific behaviors (e.g., grazing vs resting) and inform managers of animal concerns (e.g., parturition or sickness).
The use of real-time GPS and accelerometer devices could improve livestock managers’ ability to identify livestock welfare issues on extensive rangeland pastures which require large tracts of land to provide sufficient forage for their herds. Precision livestock management is also being implemented in many intensive operations such as dairies and feedlots (Hartung et al., 2017; Abeni et al., 2019; Rosa, 2021). As opposed to extensive operations, intensive livestock operations usually have access to electrical power and internet availability (wifi), which facilitates transfer of data from the sensors to internet or computers for processing and analyses. Recent developments in Long Range Wide Array Networks (LoRaWAN), Internet of Things (IoT), and satellite technologies is making transmission of data from sensors to the internet for processing and delivery to livestock managers more feasible and cost effective (Tzounis et al., 2017; Sanchez-Iborra et al., 2018; Suji Prasad et al., 2021). Although these technologies are evolving there are many challenges. Mountainous and rugged topography limits line of site transmission and can decrease the range and viability of LoRaWAN gateway and cellular reception of tracking and sensor data. LoRaWAN gateways can be strategically placed to help overcome the impact of rough terrain on line of site transmissions, but this increases initial costs and maintenance. Satellite based transmission may work well in mountainous and remote locations with limited or no cellular reception, but the initial cost and data transfer fees (often a subscription) are more expensive that other solutions. The continued development of asset tracking will facilitate development of technologies to transmit sensor data from livestock grazing extensive pastures to the location for data processing (e.g., internet) and subsequently to the manager (Elijah et al., 2018).
With new advances in technology allowing for PLM, livestock producers and researchers now can further our understanding of best management practices and enhance animal welfare. The “Five Freedoms” were developed to guide the standards and management practices within intensive livestock production systems (Farm Animal Welfare Council, 2009). These five freedoms for animal welfare include 1) freedom from thirst, hunger, and malnutrition, 2) freedom from discomfort, 3) freedom from pain, injury and disease, 4) freedom to express normal behavior, and 5) freedom from fear and distress. The use of the five freedoms and concerns for animal welfare differ between extensive systems and intensive systems (Petherick, 2005) because of the effort and cost to observe animals on the rugged topography and large paddocks that are typical for rangeland livestock production. Rutter (2014) introduced the concept of using smart technologies to monitor and manage livestock on rangeland systems, but little research was available at that time to explore how livestock well-being could be monitored remotely. A systematic review by Fogarty et al. (2019) evaluated the use of on-animal sensors to monitor sheep welfare using the five domains model. From this review, authors identified that the number of studies specifically which used on-animal sensors to detect welfare concerns of sheep are limited. Manning et al. (2021) uses the five domains to evaluate the potential of on-animal sensors to remotely assess animal behavior and well-being and to help monitor compliance with legislation directed at livestock welfare. The objectives of this paper are to discuss the potential of specific applications of PLM within extensive livestock production systems to remotely monitor animal wellbeing using the concepts first described by the “five freedoms of animal welfare” so that managers can prevent issues and promptly resolve concerns (Table 1).
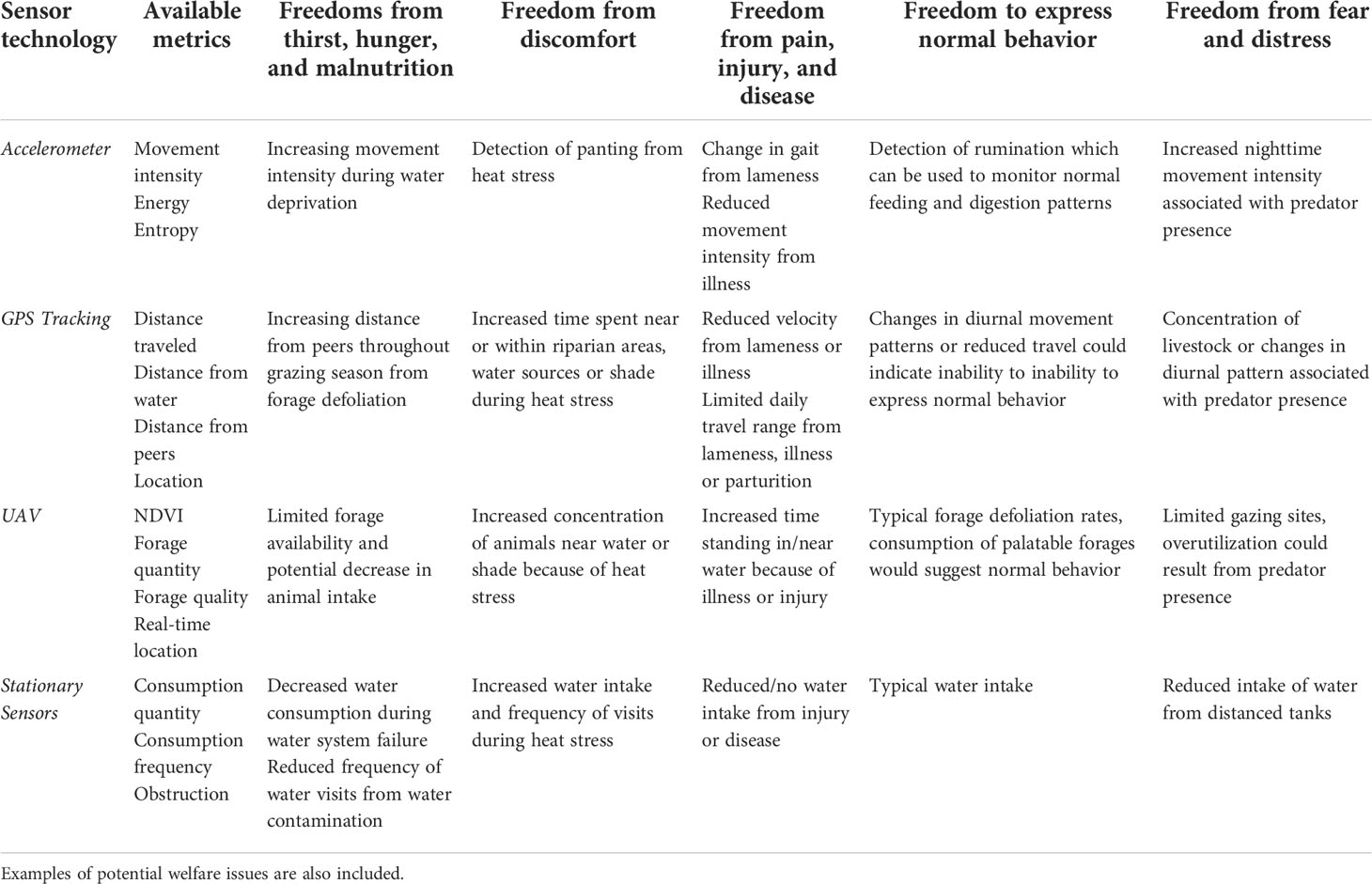
Table 1 Non-exhaustive listing of precision livestock management technologies, derivable metrics from each device, and examples of metrics which could identify issues related to each of the five freedoms.
Application of PLM to the five freedoms
The Five Freedoms have been criticized and alternative systems such as the Five Provisions, Five Domains and a Life Worth Living have been developed (Mellor, 2016a; Mellor, 2016b; Mellor, 2017). One of the criticisms of the Five Freedoms is that they concentrate on negative effects (McCulloch, 2013). Originally developed as an animal welfare assessment process (Mellor and Reid, 1994), the Five Domains now includes positive effects (Mellor and Beausoleil, 2015). To ensure livestock have “Lives Worth Living”, Mellor (2016b) suggests that negative experiences should be minimized and positive experiences should be emphasized. Mellor (2016a; 2016b, and 2017) also contends that the advancements in the understanding of biological process are not included in the Five Freedoms concepts. Webster (2016) argues that the Five Freedoms continue to have value, because they are simple and clearly outline appropriate management actions. For this paper, we do not attempt to access mental state or directly measure welfare status. Instead, we describe how tracking, accelerometers and other sensors may be able to help managers identify situations when rangeland livestock may need attention and allow them to respond more quickly. The Five Freedoms facilitates that discussion and allows to organize the paper in a concept that readers are familiar with.
Freedom from thirst, hunger, and malnutrition
The most important nutrient for livestock is water and the most critical livestock well-being concern is water availability on extensive rangelands (Bailey, 2016). Typically, water requirements range from 3-6 liters per day for sheep and goats (Sykes, 2017), 20 liters per day for horses (Swinker, 2014) to more than 100 liters per day for lactating cattle (Rasby and Walz, 2011). Water in many rangeland livestock operations is provided to livestock from remote wells powered by electricity, windmill, or solar power. Failure of these water systems can lead to devastating consequences and must be addressed immediately to maintain animal welfare. The ability of livestock managers to visually monitor water availability is time consuming and, at times, labor intensive. Water tanks are often located in areas that require travel on unimproved and primitive roads or on horseback. Use of PLM technologies to monitoring livestock water systems remotely could improve managers’ ability to identify issues sooner and minimize the chance the livestock not having access to adequate water.
Desert adapted cattle can turn over roughly one-third of their body water per day during the summer months (Siebert and Macfarlane, 1969). Water deprivation within the first 24 h is rarely fatal but there is a loss of appetite and mobilization of body fat reserves (Marques et al., 2012). Effects of water deprivation on cattle become more critical after 24 h. Cows can lose 20% body weight within 3 d and die within 5 d of water deprivation (Siebert and Macfarlane, 1975). During periods of dehydration and heat stress, animals will increase sweating and panting. Panting allows the blood passing through the nasal area to cool, keeping the brain temperature lower than the body (Robertshaw and Damiel, 1983; Baker, 1989).
Panting and other observations such as decreased movement, heavy breathing, and lack of coordinated movement are signs of heat stress. These behaviors could potentially be identified by accelerometers and GPS-tracking and used to remotely monitor livestock for heat stress. Williams et al. (2019) used an accelerometer placed within neck collars on 12 tropical beef heifers to determine if drinking behavior of cattle could be identified remotely. The accelerometer was able to identify drinking, walking, standing (head up) and standing (head down) behaviors. Accelerometers proved to be a viable tool to record cattle drinking behavior.
Tobin et al. (2021b) provides the background and proof of concept for the use of real-time GPS to detect water infrastructure failure. To our knowledge, this is the first research that uses on-animal sensors to identify water system failures. The research was conducted near Prescott, AZ in a pasture with one water source. Store-on-board GPS tracking data detected differences in minimum animal distances from water, where animals remained near water during a simulated drinker failure. After cattle initially approached the water location, GPS tracking verified that distance to water was less during simulated water failure compared to normal watering events when cattle traveled out of the watering area after drinking and rested several hundred meters away. Movement intensity (MI) calculated from accelerometer data showed an increase in activity during the simulated water failure trials compared to the previous normal watering events.
Stationary sensors, such as radio frequency identification (RFID) readers and cameras, provide valuable animal behavior data in intensive systems including drinker and feeder visit duration, intake rates, and drinking rates (Chapinal et al., 2007; Allwardt et al., 2017). In an extensive system, these stationary sensors can identify time of day, frequency, water intake, and associated differences due to weather variability and water availability (Williams et al., 2020). A potential issue with utilizing stationary sensors includes animals’ refusal to drink due to contaminated water caused by stagnation or death of other animals. The combinations of on-animal and stationary sensors can bolster livestock managers’ ability to monitor water intake of their herds
In addition to water, livestock require adequate supplies of forage. Livestock on extensive rangelands can roam freely in search of forage to satisfy their nutritional requirements. Unlike intensive systems, where feed is typically provided to livestock (e.g., feed bunk), ranchers must determine if there is sufficient forage and if the grazing use has reached a desired level. Failure to observe grazing levels can lead to overgrazing and associated adverse impacts to vegetation. Monitoring forage availability and vegetative utilization is time consuming. Sampling methods such clipping small plots to determine biomass availability and measurement of stubble heights to determine forage utilization provide quantitative data, but they are time consuming and estimates often lack precision because grazing use within extensive rangeland systems is often highly heterogenous based on topography, distance from water, and other variables (Raynor et al., 2021). Qualitative visual estimates for forage availability and utilization require skill and experience to provide accurate information, and these techniques still require time to travel and observe pasture conditions. Remote sensing can be used to determine available forage and potentially estimates of nutrient content (Jansen et al., 2021b). Currently, the cost of acquiring aerial or satellite imagery and cost and expertise required for processing and interpreting remote sensing data limits its application to commercial livestock operations that rely on extensive rangeland, forest lands and pastures (Knox et al., 2012; Pringle et al., 2021). However, programs like the rangeland analysis platform are getting closer to providing frequent updates on biomass availability across wide regions of the United States based on remote sensing (Jones et al., 2021; Jansen et al., 2021a).
Determining when to move livestock out of a pasture is a balance between using available forage throughout the pasture and preventing overuse of preferred forage and grazing areas. The use of PLM could help managers determine if forage availability was reaching a level that may limit animal intake and result in resource damage (Bailey et al., 2021). Determining when to move livestock to a new pasture is a critical issue for rangeland livestock operations. Cheleuitte-Nieves et al. (2020) used GPS tracking to determine environmental and landscape influences on cattle distribution in South Texas. They GPS tracked 31 free ranging Angus x Bonsmara cows (Bos taurus) on 457-ha and detected seasonal differences in animal dispersion. During the growing season, when forage is more abundant animals disperse more than during the dormant season (Sato, 1982; Owens et al., 1991; Cheleuitte-Nieves et al., 2018). Findings from other regions, particularly northern, mountainous and arid locations, indicate that cattle tend to congregate more during the growing season and disperse during the winter, dormant season (Dudzınski et al., 1982; Lazo, 1994; Howery et al., 1996; Harris et al., 2002; Harris et al., 2007). Uneven grazing distribution limits the effective carrying capacity of a pasture (Holechek, 1988; Bailey, 2004). In addition to resource damage, livestock performance typically declines when forage availability becomes limited in preferred areas and animals are forced to travel farther from water and use steeper terrain to find forage (Hart et al., 1993; Bailey and Brown, 2011). Remote real-time tracking systems could inform managers if livestock are concentrating in preferred locations, and managers could then implement management strategies to manipulate grazing distribution to help prevent resource degradation and to ensure animals have sufficient forage (Bailey et al., 2021).
Livestock social interactions can also be monitored using GPS tracking (Stephenson and Bailey, 2017; Xu et al., 2020) and may provide insight into foraging conditions (Cheleuitte-Nieves et al., 2020). Tobin et al. (2021a) studied how the time cattle spent in close proximity to each other in the pastures was affect by stocking density in central Arizona to determine if social associations were affected by forage availability and utilization. The two cattle herds of similar size (132 to 135 animals) were tracked simultaneously across two adjacent pastures, high stock density pasture (312 ha pasture at 0.417 animal units (AU) ha-1) and a low stock density pasture (1096 ha pasture at 0.123 AU ha-1), over a six week period. As utilization of the forages increased, association levels for both herds decreased and distance traveled from water increased (Figure 1). These metrics for greater herd dispersion could potentially be used as indicators to inform the timing of pasture rotations with real time GPS tracking in conjunction with visual and quantitative estimates of vegetation utilization.
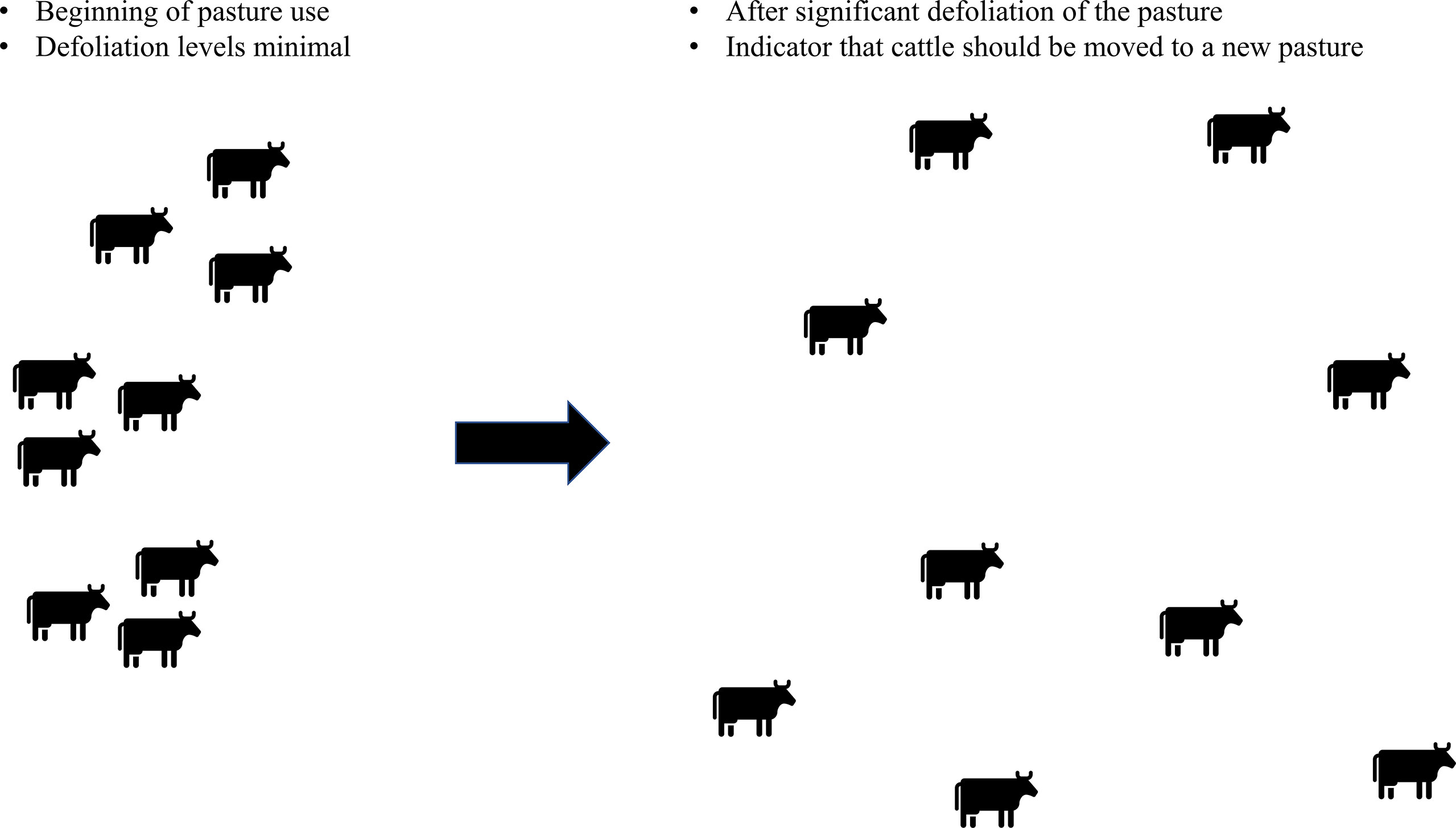
Figure 1 Example of the informative value of associations among animals within the herd. Tobin et al. (2021a) found that as utilization level of forages increased, the assocation between animals decreased for both high and low stocking density managed herds. Tracking data and precsion livestock management (PLM) techniques could potentially inform the timing of pasture rotations through changes in behavior of individuals within the herd.
Livestock managers largely rely on visual estimations of biomass quantities and utilization levels. In-field estimation methods are subjective, can have high observer variability and bias (Smith et al., 2007), and costly to collect (Caughlan and Oakley, 2001). Linking tracking and in-field forage monitoring systems can help inform ranchers where livestock are congregating and higher levels of defoliation are occurring compared to other areas. Jansen et al. (2021a) utilized in-field forage utilization measurements, livestock GPS tracking data, and pre- and post-grazing Landsat satellite images in northeastern Oregon to identify appropriate sampling areas for repeatable and objective monitoring of targeted monitoring locations. The use of all three methods identified similar broad patterns of livestock utilization within the pasture including increased congregation near stock ponds and other key areas (Laurence-Traynor, 2020). The incorporation of these systems will not only improve short-term livestock management but also help livestock managers make long-term herd-based decisions regarding changing the breed/species of grazing animal, improving watering/supplement locations, and pasture rotations to provide adequate nutrition for their herd.
Freedom from discomfort
During times of extreme heat and cold, grazing livestock must find shelter in extensive systems to prevent hyperthermia or hypothermia (Bailey, 2016). Knowing an animal is in discomfort and then providing supplemental feed, water, and shelter could be vital to many animals’ survival and well-being. Known movements of livestock and their use of landscape resources can be monitored with GPS tracking.
Body temperature is the most important factor for assessing heat stress in domestic livestock and is closely associated with health, well-being, and reproductive success (Lewis Baida et al., 2021). Livestock may not travel as far during hot temperatures (Herbel and Nelson, 1966) or spend as much time grazing (Findlay, 1958). Bar et al. (2019) utilized accelerometer-based tags affixed to collars, which were previously used to classify ruminating, resting, and grazing behaviors, to successfully detect heavy breathing/panting in dairy cattle when subjected to heat stress. Rumen boli can be useful for monitoring body core temperature of livestock and would be useful for detecting heat stress (Lewis Baida et al., 2021). Livestock responses to heat stress could potentially be determined by real-time GPS because animals may move to areas with water (e.g., riparian areas) and shade in response to high temperatures (DelCurto et al., 2005; Cheleuitte-Nieves et al., 2020). Providing shade can improve production in high temperatures and humidity (McIlvain and Shoop, 1971) and reduce radiant heat load (Bear et al., 2012). However, Davison and Neufeld (2005) found that the construction of shade structures did not reduce cattle use of riparian areas. Real-time accelerometers, GPS, and remote sensing have the potential to identify symptoms associated with heat stress and inform livestock managers so management decisions can be made instantaneously.
Cold stress is also a concern for animal discomfort. During periods of extreme cold, wind, and rain, cattle will gather together near shelter and stand with their hind quarters facing the wind (Vandenheede et al., 1992; Gregory, 1995). In extensive rangeland systems, livestock typically seek sheltered or protected terrain during cold and windy weather (Black Rubio et al., 2008). Livestock tend to also decrease feed consumption during cold stress (Gregory, 1995). Real-time GPS and accelerometers could potentially be able to detect when livestock are not responding well to cold stress during periods of low temperatures and high wind speeds. If livestock do not travel and graze in preferred areas based off historic information and congregate with limited movement it might be a sign of cold stress and indicate a need for additional management to provide supplemental feeding or shelter. Taylor et al. (2011) found that recently shorn sheep utilized shelter significantly more than the remainder of the paddock. Beaver and Olson (1997) found that older, more experienced cows used protected areas during cold and windy weather more than younger, less experienced cows. If identified, cattle could be moved to pastures with more natural protection with rough topography (Olson and Wallander, 2002) or areas with evergreen tree cover (Black Rubio et al., 2008). They could also be moved to areas with constructed shelters.
The potential for real-time GPS tracking and accelerometer monitoring to detect cold and heat stress needs to be studied in more detail, and there is a great opportunity for improving our understanding. Tracking and physiologic status of livestock should be examined in a variety of weather conditions and in landscapes with variable topographic features and availabilities of natural and man-make shelter and shade. Sensor data could provide another managerial tool to improve livestock’s freedom from discomfort on rangelands.
Freedom from pain, injury and disease
Application of PLM has the potential to significantly benefit livestock and livestock managers. Prey animals, including livestock, will hide signs of stress and pain, and fail to show symptoms to livestock managers (Underwood, 2002). The use of sensors, such as accelerometers and GPS, could detect subclinical issues related to disease and parasitism before production is affected (Ikurior et al., 2020; Tobin et al., 2020). The effects of disease cause a wide range of behavior changes in livestock.
One of the leading livestock welfare concerns is lameness (O’Callaghan et al., 2003; Rushen et al., 2007; Adams et al., 2017). The use of tri-axial accelerometers could be beneficial in the detection of abnormal gait patterns due to lameness. Barwick et al. (2018a) used an accelerometer attached to an ear tag to detect lameness in sheep. Quadratic discriminant analysis of the accelerometer data predicted selected behaviors including lameness with accuracies > 95%. Lameness could be categorized as a change in behavioral state and with the addition of real-time systems could alert livestock managers within hours rather than the historical timeline of days needed by visual observation. Williams et al. (2016) used GPS tracking and machine learning to detect lameness in dairy cows grazing pastures. Discriminant analyses were able to detect lameness in pasture-based dairy cows using the combination of GPS tracking and accelerometers (Riaboff et al., 2021).
Accelerometer applications could help determine the onset of diseases by monitoring changes in livestock behavioral patterns. Tobin et al. (2020) identified reduction in daily movement intensity (MI) during the day of observation and diagnosis of bovine ephemeral fever (BEF) in two tropical beef heifers compared to their behavior two days prior to diagnosis. Results from this study suggest that detection should be based on changes from an individual’s normal behavior rather than comparison to other animals. Ikurior et al. (2020) assessed sheep movement intensity and performance when inhabited by gastrointestinal nematode infection using accelerometers. Ram lambs, aged 9-10 months, were fitted with a mating harness with attached accelerometers recording at 30hz. Analyzing MI, they found a reduction in activity in untreated lambs compared to lambs treated with anthelmintic. Heavier lambs at the beginning of the study were affected more by nematodes and displayed less activity (lower MI) than lighter lambs.
Parturition requires increased observation and livestock management to reduce offspring susceptibility to disease, decrease offspring mortality, and improve animal well-being (Meijering, 1984; Mee, 2008; Cornou and Kristensen, 2014; Chang et al., 2020). The most common complication during parturition is dystocia. Dystocia births refers to the an abnormal, prolonged, and/or difficult labor (Meijering, 1984; Mee, 2008) which can decrease offspring vigor and increase the likelihood of stillborn offspring (Riley et al., 2004; Barrier et al., 2012). Monitoring of the animal during all stages of parturition may allow for identification and earlier intervention by the producer (Dobos et al., 2014). Holmøy et al. (2012) found that odds of neonatal loss were 30% greater in flocks where farm staff were not regularly present compared to flock which were continuously monitored. Using accelerometer sensors and GPS tracking have the potential to identify common unobservable behaviors associated with parturition including reduction in drinking and feeding, seeking sheltered areas, increasing standing, and reduction in rumination (Chang et al., 2020).
As parturition begins in livestock, the dam undergoes several behavioral and physiological changes. The beginning of parturition can be observed by an increase in time standing compared to levels pre and post-parturition (Huzzey et al., 2005), an increase in standing compared to lying (Chang et al., 2020; Gurule et al., 2021b), and isolation from the group (Dobos et al., 2014). The application of accelerometers and GPS tracking can improve livestock managers’ ability to identify parturition behaviors and provide assistance to both dam and offspring (Fogarty et al., 2021). Dobos et al. (2014) utilized Global navigation satellite system technology to track 20 pregnant ewes grazing a 1.6 ha paddock. Results from that study suggest that location tracking can help identify individual isolation from the flock during the day of parturition, decrease daily speed during the day of and up to three days after parturition. Gurule et al. (2021b) attached accelerometers to ear tags which were affixed to 13 pregnant ewes, observed behaviors including feeding, laying, licking lamb, licking salt, contractions, standing, and walking, and then applied machine learning to predict parturition and behavior. Results from this study suggest that some behaviors may be difficult to predict but changes in MI and individual accelerometer axis data can be used to help detect parturition.
Rumination is a key behavior for several grazing livestock species. The process of chewing and ruminating assists in reducing feed particle sizes, lubricating the feed bolus, and providing saliva as a rumen buffer and is vital to maintain efficient digestive function (Beauchemin, 2018). Rumination time can be reduced by disease (Soriani et al., 2012; Soriani et al., 2013; Calamari et al., 2014; Stangaferro et al., 2016a; Stangaferro et al., 2016b; Stangaferro et al., 2016c), heat stress (Soriani et al., 2013) or calving (Calamari et al., 2014; Chang et al., 2020). Traditional methods of observing rumination are time consuming, require dedicated personnel, and are nearly impossible at the large scale of most pastures throughout the central and western US and Australia. Chang et al. (2022) conducted a study utilizing accelerometers affixed to 8 beef cows to compare different machine learning techniques and epoch lengths to detect rumination. The results show that individual rumination can be detected with high accuracy (98.4%) using an ear tag placed accelerometer. Future research should evaluate if deviations in an individual’s’ normal rumination to improve real-time identification of disease, pain, and injury detection.
Freedom to express normal behavior
In extensive rangeland systems, livestock have ample opportunities to express normal behaviors (Bailey, 2016). Cattle are social animals and associate with other individuals on rangelands, often creating a synchronization of behaviors. The expression of normal behavior is unhindered unless management practices, such as herding and supplementation (Stephenson et al., 2017; Sprinkle et al., 2021), affect the social interactions among individual animals. The use of PLM could improve our ability to identify, and categorize, normal behaviors in diverse situations and determine if the environment or management is adversely impacting this behavior.
Many behaviors can be predicted from traveling velocities calculated from GPS tracking (Augustine and Derner, 2013; Nyamuryekung’e et al., 2020). Daily activity budgets can be calculated from predicted behaviors based from GPS tracking or accelerometers. Daily activity patterns can be affected by season (Cheleuitte-Nieves et al., 2018; Cheleuitte-Nieves et al., 2020), forage quality and quantity (Tobin et al., 2021a), and physiological states of the animals (Werkheiser, 2018). Distance traveled per day, distance from water, and changes in grazing locations can be used to determine normal behavior and also changes in behavior resulting from environmental factors and management actions. Fogarty et al. (2020b) was able to classify behavior, determine activity, and detect posture from ear tag accelerometer data derived from extensively grazing sheep. Deviations from normal diurnal activity patterns would suggest an inability to express normal behavior.
Another opportunity to remotely assess livestock’s ability to express normal behavior may be to monitor rumination. Rumination is part of a ruminant’s normal behavior. Changes in rumination patterns can be used to monitor the physiologic state of livestock and identify metabolic disorders (Stangaferro et al., 2016a). Abnormal rumination patterns may be a result of several factors such disease, but abnormal patterns may also suggest that livestock are consuming diets with excessive or insufficient levels of fiber (White et al., 2017). Accelerometers can remotely monitor rumination patterns (Chang et al., 2022) and potentially grazing livestock can be monitored. Unusual rumination patterns may be useful for determining conditions when the diet does not allow livestock to express normal behavior. On pastures and rangeland this might occur when forages are very low quality, and managers could potentially resolve this concern by providing protein supplements (Huston et al., 2002).
The well-being of adapted cattle is likely better than for unadapted cattle (Bailey, 2016). Adapted animals may be able to use rangelands more efficiently and with less stress than unadapted animals. Adaptation is the result of nature and nurture. Learning, experiences, and genotype and environmental interactions allow animals to adapt to local conditions (Provenza, 2008). For example, Hereford cattle did not travel as far Santa Gertrudis cows during the summer in the Chihuahuan Desert (Herbel and Nelson, 1966). Santa Gertrudis cattle are 3/8 Brahman and likely to be more heat tolerant than Hereford cows. Some cows use steeper and higher terrain than others (Bailey et al., 2004) and are likely more adapted to mountainous terrain. Real-time tracking has the potential to provide managers data to identify cows that readily use steep terrain.
Freedom from fear and distress
Extensive systems allow animals to roam free and generally livestock are free from fear or stress. However, during certain periods, mangers must interact with livestock. Pregnancy detection, calving, branding, and weaning require livestock managers to interact with their animals (Bailey, 2016). Due to varying management styles and skills, these interactions could cause distress and fear during handling and processing for the livestock (Grandin, 1997). Interactions between livestock and livestock managers that increase stress and discomfort are detrimental to an animal’s wellbeing and livestock managers’ attitudes toward their animals. Real-time accelerometers could indicate higher activity levels resulting from increased anxiety and stress upon the animal, similar to what is addressed in Tobin et al. (2021b) when cattle did not have access to water. Hibbard (2012) describes a method developed by Bud Williams and termed low stress stockmanship that can reduce stress livestock incur during handling. Changing handlers or improving handler skills (e.g., from traditional to low stress stockmanship) would likely change livestock anxiety and stress levels. Such changes potentially could be monitored with GPS tracking and accelerometers similar to Tobin et al. (2021b).
Identification of fearful and distressed animals on rangelands is vital to evaluate welfare and performance. Monitoring changes in heart rate can help livestock managers determine the causes of fearful and distressful situations. Piette et al. (2016) utilized wearable technology to evaluate mental state of horses using the actual versus predicted heart rates. The researchers monitored heart rates during basic metabolism (resting), exercise, and mental state or stress and found that heart rate peaks could be matched to stress and behavior with sensitivity and precision of 77% and 78, respectively. Though this method to identify stress may be difficult for grazing animals, it could provide additional opportunities to expedite intervention.
Another form of stress livestock experience is predation, which almost certainly impacts animal welfare and increases fear and distress of livestock. When experiencing predation, ungulates may aggregate into larger groups which reduces the probability of being preyed upon (Hebblewhite and Pletscher, 2002; Figure 2). Clark et al. (2017) found livestock that grazed mountainous areas and were exposed to wolf presence traveled less each day than cattle that were not exposed to wolves. Livestock fidelity to safe habitats could restrict daily movements and impact livestock diets (Lima and Dill, 1990; Clark et al., 2017). Cows whose calves were preyed upon by wolves were more vigilant and spent less time grazing than cows whose calves were not harmed (Kluever et al., 2008). Evans et al. (2022) found that ewes traveled further each day during periods when exposed to predation by wild dogs compared to periods when the dogs were absent. The application of sensors such as real-time GPS tracking and accelerometers could be used to identify when predators may be present. Clark et al. (2020) found that 84% of cattle –wolf encounters occurred in areas identified as “high risk areas” from resource selection models of cattle and wolf tracking data. Congregation of livestock and reduction in daily distances traveled could be used to alert managers to implement strategies to minimize the impacts of predation (e.g., Breck et al., 2011; Breck et al., 2012).
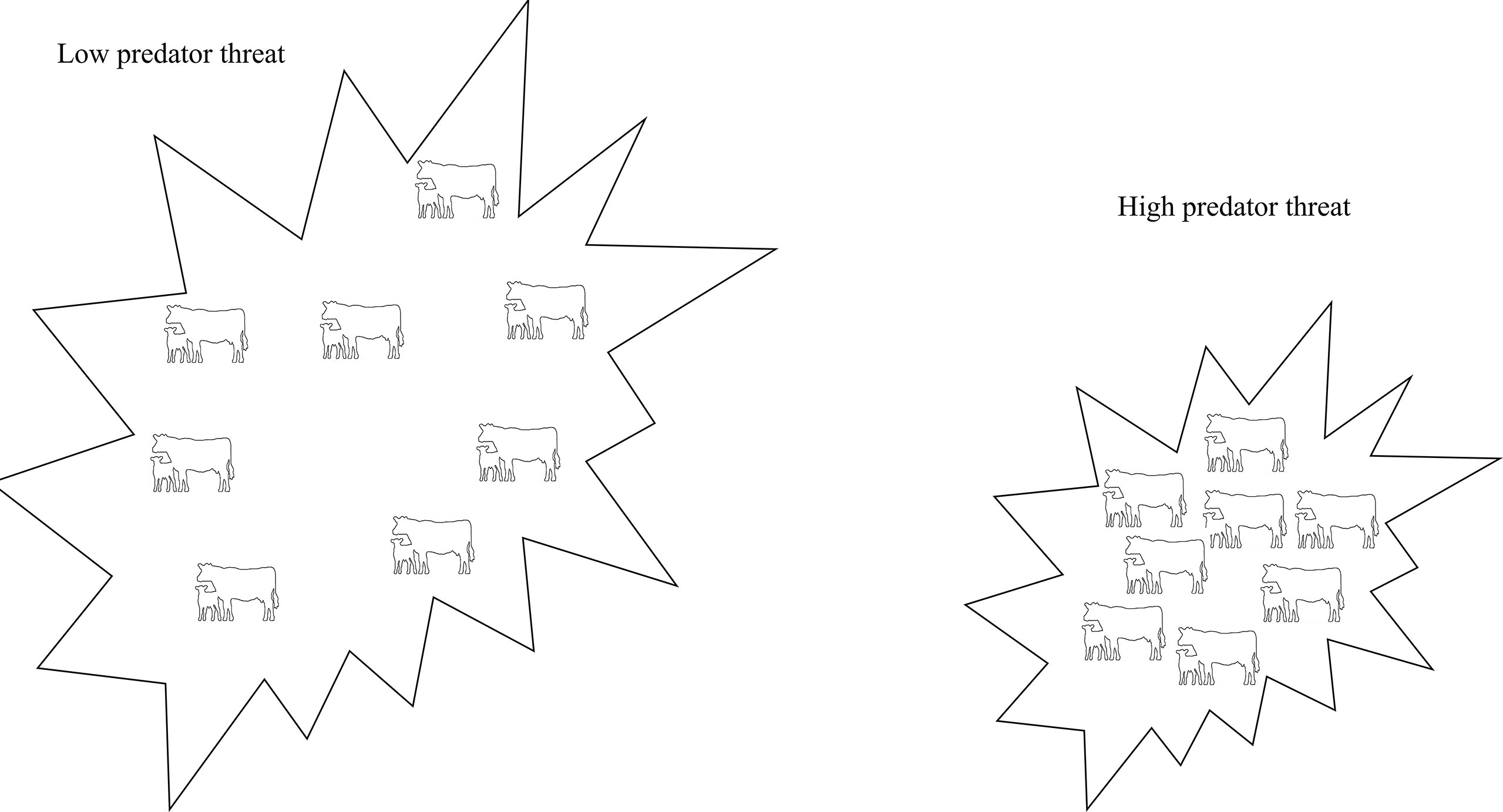
Figure 2 Example of the aggregation of ungulates into larger groups (Hebblewhite and Pletscher, 2002) and reduction in grazing sites (Lima and Dill, 1990; Clark et al., 2017) which could be identified by livestock managers via real-time global positioning system (GPS) dashboards. The identification of predator presence could prompt livestock managers to change grazing location or increase anti-predator management to improve the welfare of livestock.
Use of multiple sensors to improve behavior detection
Grazing livestock on rangelands presents challenges when collecting data for PLM. Rangelands are large, heterogeneous, and highly dynamic (Wishart et al., 2015; Morgan-Davies et al., 2018). Livestock in extensive pastures can damage and lose tracking equipment which is affixed to their ears and neck (e.g. Tobin et al., 2021a; Tobin et al., 2021b). Normal grooming, scratching, and fighting can impact structural integrity while environmental factors, such as ultraviolet radiation, temperature, and precipitation can deteriorate cases and internal electronic connections.
Adding additional sensors to animals will ensure continuous monitoring and improve accurate readings. The combination of remote sensing imagery and GPS tracking could help livestock managers determine management of herds (Jansen et al., 2021a). The combination of GPS tracking and accelerometer data appear to be more useful for detecting changes of normal animal behavior than one type of sensor data. Issues such as water infrastructure failures could likely be identified sooner using real-time GPS and accelerometer systems (Tobin et al., 2021b). Fogarty et al. (2021) incorporated GNSS tracking collars, accelerometer ear tags, and local weather data into a machine learning algorithm to detect parturition events in sheep flock over two consecutive years. The combination of metrics was able to detect up to 55% of lambing events within 3 hours of parturition with no false positive prediction alerts. If one or two false positive predictions were permitted, detection rates of lambing events were up to 91%.
With additional equipment development, researchers and livestock managers must scrutinize the size and weight of on-animal sensors. The acceptable standard for maximum tracking collar weight has been suggested as less than 5% bodyweight (Macdonald, 1978). Fit and weight of tracking devices can have an effect on natural behaviors. Brooks et al. (2008) found that heavier, ill-fitting tracking collars slowed zebra traveling speeds during grazing bouts. Ill-fitting collars and additional head mass may increase foraging costs and reduce bite rates by resting the tracking device on the lower jaw (Brooks et al., 2008). The use of tracking devices on the animal body may result in hair loss and skin abrasions, and must be adjusted if necessary to reduce strangulation. The weight of ear tags used for tracking and monitoring with accelerometers must not be excessive or the tag will damage the ear and fall off. Observations by the authors suggest that heavy tags can work down the ear causing the ear to split and resulting in tissue damages. Ongoing studies by the authors and other researchers are being conducted to determine the maximum weight for ear tag sensors.
Current use of these devices is often limited due to costs. With advancements in battery capabilities, connectivity to cellular and satellite networks, and product competition, the cost of these devices will become more affordable to users. An alternative to monitoring the entire herd would be to track sentinel animals (Neo and Tan, 2017). Utilizing sentinel animals would be helpful to track and monitor herd health issues, such as water infrastructure failure (Tobin et al., 2021b) or exposure to moldy feed (Gurule et al., 2021a) at a cost of losing the majority of the individual animal behaviors (Bailey et al., 2021).
Enabling intervention
Precision livestock management has the potential to detect numerous issues with livestock by tracking deviations from normal behavior. A goal of PLM on extensive rangelands is to improve animal welfare by expediting treatment of affected animals. The application of sensors and their associated notification systems need to work efficiently to notify livestock managers of location and potential issues to supply preparation.
One added benefit of utilizing PLM and real-time sensors would be identifying locations of comprised animals. On extensive rangelands, there is no possible way in which animals can be consistently and promptly located to evaluate and treat without GPS tracking. Although not as accurate and useful, placement of RFID readers near water or supplement locations could help identify the time and general area of the animal by recording the time it approaches the device.
Along with locating compromised animals, the devices could potentially give managers detected symptoms, such as lameness or heat stress, to help them prepare supplies for treatment. Intervention and correction of the wide range of diseases and welfare concerns that can affect livestock on extensive rangeland is much more efficient if managers can be prepared. For example, livestock managers need to take immediate action when dystocia occurs and intervention is needed. Schuenemann et al. (2011) found that livestock manager intervention should take place approximately 65 minutes after feet appearance or immediately if malposition is evident. Identifying the onset and length of parturition could improve detection of dystocia to improve health and welfare for both dam and offspring. Also, treatment of disease often requires antibiotics which herdsman must have available to facilitate intervention in extensive pastures.
During the breeding season, male livestock can be affected by disease (e.g. hoof and foot rot), weather, and other males from within the pasture and adjacent pastures. These stressors can limit movement and agility, which can compromise their ability to mate and lead to poor reproductive performance of the entire herd (Drake et al., 2011) if the remaining herd sires cannot compensate. The application of PLM potential could identify sire injuries during the breeding season for expedited treatment and replacement.
In some cases, livestock illness and/or injury cannot be successfully treated and euthanasia is the only practical option, and is required for welfare of the animal when quality of life has diminished (Woods et al., 2010). The ability to locate such animals on extensive and mountainous rangeland with real-time tracking prevents excessive suffering because euthanasia can be provided promptly.
Conclusion
It is difficult, time consuming, and sometimes impractical for ranchers to visually observe individual livestock on a daily basis. Correspondingly, many animals grazing extensive rangelands may become ill and are not treated for several days to weeks. Real and near-real time sensors could potentially assess diseases and other well-being concerns remotely and reduce the time required to find and treat animals. Technology innovations are facilitating the transition from store-on-board to real-time sensors, which has facilitated the development of precision livestock management. Improved systems will decrease location errors, data transmission, and the data transfer interval between recording animal locations. Development of algorithms using current and historic data has potential to detect changes in animal behavior and welfare concerns in real-time. The use of these sensors will provide valuable information to help improve productivity through improved disease response, infrastructure repairs, and grazing management. Though PLM systems will vary among operations, the integration of technologies should improve animal well-being while grazing rangelands. The use of on-animal sensors could promptly send alerts of altered behavior of individual animals rather than relying only on visual observations to monitor livestock wellbeing.
Author contributions
CT and DB prepared the initial draft. All authors contributed to the research used in the development of this paper. All authors edited and improved the paper.
Funding
Research and concepts described in this paper by the authors were supported by funding from several sources: the Harold James Family Trust (Prescott, Arizona), the New Mexico Agricultural Experiment Station, NDSU Carrington Research Extension Center, and USDA National Institute of Food and Agriculture, Hatch project (NMBailey-15H).
Conflict of interest
The authors declare that the research was conducted in the absence of any commercial or financial relationships that could be construed as a potential conflict of interest.
Publisher’s note
All claims expressed in this article are solely those of the authors and do not necessarily represent those of their affiliated organizations, or those of the publisher, the editors and the reviewers. Any product that may be evaluated in this article, or claim that may be made by its manufacturer, is not guaranteed or endorsed by the publisher.
References
Abeni F., Petrera F., Galli A. (2019). A survey of italian dairy farmers’ propensity for precision livestock farming tools. Animals 9, 1–13. doi: 10.3390/ani9050202
Adams A. E., Lombard J. E., Fossler C. P., Román-Muñiz I. N., Kopral C. A. (2017). Associations between housing and management practices and the prevalence of lameness, hock lesions, and thin cows on US dairy operations. J. Dairy Sci. 100, 2119–2136. doi: 10.3168/jds.2016-11517
Allwardt K., Ahlberg C., Broocks A., Bruno K., Taylor A., Place S., et al. (2017). Technical note: Validation of an automated system for monitoring and restricting water intake in group-housed beef steers. J. Anim. Sci. 95, 4213. doi: 10.2527/jas2017.1593
Alvarenga F. A. P., Borges I., Palkovic L., Rodina J., Oddy V. H., Dobos R. (2016). Using a three-axis acceleromter to identify and classify sheep behaviour at pasture. Appl. Anim. Behav. Sci. 181, 91–99.
Anderson D. M., Estell R. E., Cibils A. F. (2013). Spatiotemporal cattle data–a plea for protocol standardization. Positioning 04, 115–136. doi: 10.4236/pos.2013.41012
Augustine D. J., Derner J. D. (2013). Assessing herbivore foraging behavior with GPS collars in a semiarid grassland. Sensors (Switzerland) 13, 3711–3723. doi: 10.3390/s130303711
Bailey D. W. (2004). Management strategies for optimal grazing distribution and use of arid rangelands in. J. Anim. Sci. 82, 147–153. doi: 10.2527/2004.8213_supplE147x
Bailey D. W. (2016). Grazing and animal distribution. In: Villalba J. J. (Ed). Ani- mal welfare in extensive production systems Sheffield, UK: 5M Publishing, 59–81. The Animal Welfare Series
Bailey D. W., Brown J. R. (2011). Rotational grazing systems and livestock grazing behavior in shrub-dominated semi-arid and arid rangelands. Rangel. Ecol. Manage. 64, 1–9. doi: 10.2111/REM-D-09-00184.1
Bailey D. W., Keil M. R., Rittenhouse L. R. (2004). Research observation: Daily movement patterns of hill climbing and bottom dwelling cows. J. Range Manage. 57, 20–28.
Bailey D. W., Trotter M. G., Knight C. W., Thomas M (2018). Use of GPS tracking collars and accelerometers for rangeland livestock production research. G. Transl. Anim. Sci. 2, 81–88. doi: 10.1093/tas/txx006
Bailey D. W., Trotter M. G., Tobin C. T., Thomas M. G. (2021). Opportunities to apply precision livestock management on rangelands. Front. Sustain. Food Syst. 5. doi: 10.3389/fsufs.2021.611915
Baker M. A. (1989). EFFECTS OF DEHYDRATION AND REHYDRATION ON THERMOREGULATORY SWEATING IN GOATS. J. Physiol. 417, 421–435. doi: 10.1097/00005768-200110000-00013
Balaine L., Dillon E. J., Läpple D., Lynch J. (2020). Can technology help achieve sustainable intensification? evidence from milk recording on Irish dairy farms. Land Use Policy 92, 104437. doi: 10.1016/j.landusepol.2019.104437
Bar D., Kaim M., Flamenbaum I., Hanochi B., Toaff-Rosenstein R. L. (2019). Technical note: Accelerometer-based recording of heavy breathing in lactating and dry cows as an automated measure of heat load. J. Dairy Sci. 102, 3480–3486. doi: 10.3168/jds.2018-15186
Barrier A. C., Ruelle E., Haskell M. J., Dwyer C. M. (2012). Effect of a difficult calving on the vigour of the calf, the onset of maternal behaviour, and some behavioural indicators of pain in the dam. Prev. Vet. Med. 103, 248–256. doi: 10.1016/j.prevetmed.2011.09.001
Barwick J., Lamb D., Dobos R., Schneider D., Welch M., Trotter M. (2018a). Predicting lameness in sheep activity using tri-axial acceleration signals. Animals 8, 1–16. doi: 10.3390/ani8010012
Barwick J., Lamb D. W., Dobos R., Welch M., Trotter M. (2018b). Categorising sheep activity using a tri-axial accelerometer. Comput. Electron. Agric. 145, 289–297. doi: 10.1016/j.compag.2018.01.007
Bear D. A., Russell J. R., Morrical D. G. (2012). Physical characteristics, shade distribution, and tall fescue effects on cow temporal/spatial distribution in midwestern pastures. Rangel. Ecol. Manage. 65, 401–408. doi: 10.2111/REM-D-11-00072.1
Beauchemin K. A. (2018). Invited review: Current perspectives on eating and rumination activity in dairy cows. J. Dairy Sci. 101, 4762–4784. doi: 10.3168/jds.2017-13706
Beaver J. M., Olson B. E. (1997). Winter range use by cattle of different ages in southwestern Montana. Appl. Anim. Behav. Sci. 51, 1–13. doi: 10.1016/S0168-1591(96)01081-7
Berckmans D. (2014). Precision livestock farming technologies for welfare management in intensive livestock systems. OIE Rev. Sci. Tech. 33, 189–196. doi: 10.20506/rst.33.1.2273
Black Rubio C. M., Cibils A. F., Endecott R. L., Petersen M. K., Boykin K. G. (2008). Piñon-juniper woodland use by cattle in relation to weather and animal reproductive state. Rangel. Ecol. Manage. 61, 394–404. doi: 10.2111/07-056.1
Breck S., Clark P., Howery L., Johnson D., Kluever B., Smallidge S., et al. (2012). A perspective on livestock wolf interactions on western rangelands. Rangelands 34, 6–11. doi: 10.2111/RANGELANDS-D-11-00069.1
Breck S. W., Kluever B. M., Panasci M., Oakleaf J., Johnson T., Ballard W., et al. (2011). Domestic calf mortality and producer detection rates in the Mexican wolf recovery area: Implications for livestock management and carnivore compensation schemes. Biol. Conserv. 144, 930–936. doi: 10.1016/j.biocon.2010.12.014
Brooks C., Bonyongo C., Harris S. (2008). Effects of global positioning system collar weight on zebra behavior and location error. J. Wildl. Manage. 72, 527–534. doi: 10.2193/2007-061
Brown D. D., Kays R., Wikelski M., Wilson R., Klimley A. P. (2013). Observing the unwatchable through acceleration logging of animal behavior. Anim. Biotelemetry 1, 1–16. doi: 10.1186/2050-3385-1-20
Calamari L., Soriani N., Panella G., Petrera F., Minuti A., Trevisi E. (2014). Rumination time around calving: An early signal to detect cows at greater risk of disease. J. Dairy Sci. 97, 3635–3647. doi: 10.3168/jds.2013-7709
Campbell H. A., Gao L., Bidder O. R., Hunter J., Franklin C. E. (2013). Creating a behavioural classification module for acceleration data: using a captive surrogate for difficult to observe species. J. Exp. Biol. 216, 4501–4506. doi: 10.1242/jeb.089805
Casas R., Hermosa A., Marco Á., Blanco T., Zarazaga-Soria F. J. (2021). Real-time extensive livestock monitoring using lpwan smart wearable and infrastructure. Appl. Sci. 11, 1–18. doi: 10.3390/app11031240
Caughlan L., Oakley K. L. (2001). Cost considerations for long-term ecological monitoring. Ecol. Indic. 1, 123–134. doi: 10.1016/s1470-160x(01)00015-2
Chang A. Z., Fogarty E. S., Moraes L. E., García-Guerra A., Swain D. L., Trotter M. G. (2022). Detection of rumination in cattle using an accelerometer ear-tag: A comparison of analytical methods and individual animal and generic models. Comput. Electron. Agric. 192, 106595. doi: 10.1016/j.compag.2021.106595
Chang A. Z., Swain D. L., Trotter M. G. (2020). Towards sensor-based calving detection in the rangelands: A systematic review of credible behavioral and physiological indicators. Transl. Anim. Sci. 4, 1–18. doi: 10.1093/tas/txaa155
Chapinal N., Veira D. M., Weary D. M., Von Keyserlingk M. A. G. (2007). Technical note: Validation of a system for monitoring individual feeding and drinking behavior and intake in group-housed cattle. J. Dairy Sci. 90, 5732–5736. doi: 10.3168/jds.2007-0331
Cheleuitte-Nieves C., Perotto-Baldivieso H. L., Wu X. B., Cooper S. M. (2018). Association patterns reveal dispersal-aggregation dynamics among cattle in a south Texas rangeland, USA. Ecol. Process 7, 1–10. doi: 10.1186/s13717-018-0141-9
Cheleuitte-Nieves C., Perotto-Baldivieso H. L., Wu X. B., Cooper S. M. (2020). Environmental and landscape influences on the spatial and temporal distribution of a cattle herd in a south Texas rangeland. Ecol. Process 9, 1–18. doi: 10.1186/s13717-020-00245-6
Clark P. E., Chigbrow J., Johnson D. E., Larson L. L., Nielson R. M., Louhaichi M., et al. (2020). Predicting spatial risk of wolf-cattle encounters and depredation. Rangel. Ecol. Manage. 73, 30–52. doi: 10.1016/j.rama.2019.08.012
Clark P. E., Johnson D. E., Larson L. L., Louhaichi M., Roland T., Williams J. (2017). Effects of wolf presence on daily travel distance of range cattle. Rangel. Ecol. Manage. 70, 657–665. doi: 10.1016/j.rama.2017.06.010
Cornou C., Kristensen A. R. (2014). Monitoring individual activity before, during and after parturition using sensors for sows with and without straw amendment. Livest. Sci. 168, 139–148. doi: 10.1016/j.livsci.2014.08.002
Davison J. C., Neufeld J. D. (2005). Can shade structures help riparian areas?: a look at using constructed shades to pull cattle off riparian lands in northeastern Nevada. Rangelands 27, 24–30. doi: 10.2458/azu_rangelands_v27i2_davison
DelCurto T., Porath M., Parsons C. T., Morrison J. A. (2005). Management strategies for sustainable beef cattle grazing on forested rangelands in the pacific Northwest. Rangel. Ecol. Manage. 58, 119–127. doi: 10.2111/1551-5028(2005)58<119:MSFSBC>2.0.CO;2
Dobos R. C., Dickson S., Bailey D. W., Trotter M. G. (2014). The use of GNSS technology to identify lambing behaviour in pregnant grazing merino ewes. Anim. Prod. Sci. 54, 1722–1727. doi: 10.1071/AN14297
Drake D. J., Weber K. L., Van Eenennaam A. L. (2011). What Are Herd Bulls Accomplishinb in Multiple Ssire Breeding Pastures?, in Proceedings, applied reproductive strategies in beef cattle august 31-September 1, 2011 (Joplin, MO), 305–319.
Dudzınski M. L., Muller W. J., Low W. A., Schuh H. J. (1982). Relationship between dispersion behaviour of free-ranging cattle and forage conditions. Appl. Anim. Ethol. 8, 225–241.
Elijah O., Rahman T. A., Orikumhi I., Leow C. Y., Hindia M. N. (2018). An overview of Internet of things (IoT) and data analytics in agriculture: Benefits and challenges. IEEE Internet Things J. 5, 3758–3773. doi: 10.1109/JIOT.2018.2844296
Evans C. A., Trotter M. G., Manning J. K. (2022). Sensor-based detection of predator influence on Livestock : A case study exploring the impacts of wild dogs (Canis familiaris) on rangeland sheep. Animals 12, 1–11.
Farm Animal Welfare Council (2009). Farm animal welfare in great Britain: Past, present and future. London, United Kingdom
Findlay J. D. (1958). Physiological reactions of cattle to climatic stress. Proc. Nutr. Soc 17, 186–190. doi: 10.1079/pns19580037
Food and Agriculture Organization of the United Nations (FAO) (2009). How to feed the world in 2050. Rome: FAO. Available at: http://www.fao.org/fileadmin/templates/wsfs/
Food and Agriculture Organization of the United Nations (FAO) (2007). FAO Corporate Document Repository, Global and regional food consumption patterns and trends, Agriculture and Consumer Protection report. Rome: FAO.
FAOSTAT (2020). Food and agriculture data. Retrieved on 15 January 2022 from http://www.fao.org/faostat/en/#home
Fogarty E. S., Swain D. L., Cronin G. M., Trotter M. (2019). A systematic review of the potential uses of on-animal sensors to monitor the welfare of sheep evaluated using the five domains model as a framework. Anim. Welf 28, 407–420. doi: 10.7120/09627286.28.4.407
Fogarty E. S., Swain D. L., Cronin G. M., Moraes L. E., Bailey D. W., Trotter M. (2021). Developing a simulated online model that integrates GNSS, accelerometer and weather data to detect parturition events in grazing sheep: A machine learning approach. Animals 11, 1–22. doi: 10.3390/ani11020303
Fogarty E. S., Swain D. L., Cronin G. M., Moraes L. E., Trotter M. (2020a). Behaviour classification of extensively grazed sheep using machine learning. Comput. Electron. Agric., 169. doi: 10.1016/j.compag.2019.105175
Fogarty E. S., Swain D. L., Cronin G. M., Moraes L. E., Trotter M. (2020b). Can accelerometer ear tags identify behavioural changes in sheep associated with parturition? Anim. Reprod. Sci. 216, 1–13. doi: 10.1016/j.anireprosci.2020.106345
Fogarty E. S., Swain D. L., Cronin G. M., Moraes L. E., Trotter M. (2020c). Potential for autonomous detection of lambing using global navigation satellite system technology. Anim. Prod. Sci. doi: 10.1071/AN18654
Grandin T. (1997). Assessment of stress during handling and transport. J. Anim. Sci. 75, 249–257. doi: 10.2527/1997.751249x
Gregory N. G. (1995). The role of shelterbelts in protecting livestock: A review. New Zeal. J. Agric. Res. 38, 423–450. doi: 10.1080/00288233.1995.9513146
Guo L., Welch M., Dobos R., Kwan P., Wang W. (2018). Comparison of grazing behaviour of sheep on pasture with different sward surface heights using an inertial measurement unit sensor. Comput. Electron. Agric. 150, 394–401. doi: 10.1016/j.compag.2018.05.004
Gurule S. C., Aguirre M., Forrest K., Flores V. V., Wenzel J., Tobin C. T., et al. (2021a). Potential of sensor technology to detect changes in behavior related to health status of ewes following exposure to mold-contaminated feed. J. Anim. Sci. 99, 313.
Gurule S. C., Tobin C. T., Bailey D. W., Hernandez Gifford J. A. (2021b). Evaluation of the tri-axial accelerometer to identify and predict parturition-related activities of debouillet ewes in an intensive setting. Appl. Anim. Behav. Sci. 237, 105296. doi: 10.1016/j.applanim.2021.105296
Harris N. R., Johnson D. E., George M. R., Mcdougald N. K. (2002). “The effect of topography, vegetation, and weather on cattle distribution at the San Joaquin experimental range, California,” in Proceedings of the Fifth Symposium on Oak Woodlands: Oaks in California’s Challenging Landscape. Gen. Tech. Rep. PSW-GTR-184, Standiford R. B., McCreary D., Purcell K. L. eds. (Albany, CA: Pacific Southwest Research Station, Forest Service, U.S. Department of Agriculture), 53–63. doi: 10.2737/PSW-GTR-184
Harris N. R., Johnson D. E., McDougald N. K., George M. R. (2007). Social associations and dominance of individuals in small herds of cattle. Rangel. Ecol. Manage. 60, 339–349. doi: 10.2111/1551-5028(2007)60
Hart R. H., Bissio J., Samuel M. J., Waggoner J. W. (1993). Grazing systems, pasture size, and cattle grazing behavior, distribution and gains. J. Range Manage. 46, 81–87. doi: 10.2307/4002452
Hartung J., Banhazi T., Vranken E., Guarino M. (2017). European Farmers’ experiences with precision livestock farming systems. Anim. Front. 7, 38–44. doi: 10.2527/af.2017.0107
Hebblewhite M., Pletscher D. H. (2002). Effects of elk group size on predation by wolves. Can. J. Zool. 80, 800–809. doi: 10.1139/z02-059
Herbel C. H., Nelson A. B. (1966). Activities of Hereford and Santa gertrudis cattle on a southern new Mexico range. J. Range Manage. 19, 173–176. doi: 10.2307/3895975
Herlin A., Brunberg E., Hultgren J., Högberg N., Rydberg A., Skarin A. (2021). Animal welfare implications of digital tools for monitoring and management of cattle and sheep on pasture. Animals 11, 1–20. doi: 10.3390/ani11030829
Holmøy I. H., Kielland C., Marie Stubsjøen S., Hektoen L., Waage S. (2012). Housing conditions and management practices associated with neonatal lamb mortality in sheep flocks in Norway. Prev. Vet. Med. 107, 231–241. doi: 10.1016/j.prevetmed.2012.06.007
Howery L. D., Provenza F. D., Banner R. E., Scott C. B. (1996). Differences in home range and habitat use among individuals in a cattle herd. Appl. Anim. Behav. Sci. 49, 305–320. doi: 10.1016/0168-1591(96)01059-3
Huston J., Forbes R., Ellis W., Lippke H., Forbes T. (2002). Supplementation of grazing beef cattle. Texas A&M Univ. Agr. Exp. Sta. Tech. Monogr. 12, 12.
Huzzey J. M., Von Keyserlingk M. A. G., Weary D. M. (2005). Changes in feeding, drinking, and standing behavior of dairy cows during the transition period. J. Dairy Sci. 88, 2454–2461. doi: 10.3168/jds.S0022-0302(05)72923-4
Ikurior S. J., Pomroy W. E., Scott I., Corner-Thomas R., Marquetoux N., Leu S. T. (2020). Gastrointestinal nematode infection affects overall activity in young sheep monitored with tri-axial accelerometers. Vet. Parasitol. 283, 1–6. doi: 10.1016/j.vetpar.2020.109188
Jansen V. S., Kolden C. A., Schmalz H. J., Karl J. W. (2021a). Using satellite-based vegetation data for short-term grazing monitoring to inform adaptive management. Rangel. Ecol. Manage. 76, 30–42. doi: 10.1016/j.rama.2021.01.006
Jansen V., Traynor A. C. E., Karl J. W., Lepak N., Sprinkle J. (2021b). Monitoring grazing use: Strategies for leveraging technology and adapting to variability. Rangelands 1–14, 1–14. doi: 10.1016/j.rala.2021.07.005
Jones M. O., Robinson N. P., Naugle D. E., Maestas J. D., Reeves M. C., Lankston R. W., et al. (2021). Annual and 16-day rangeland production estimates for the Western united states. Rangel. Ecol. Manage. 77, 112–117. doi: 10.1016/j.rama.2021.04.003
Karl J. W., Sprinkle J. E. (2019). Low-cost livestock global positioning system collar from commercial off-the-Shelf parts. Rangel. Ecol. Manage. 72, 954–958. doi: 10.1016/j.rama.2019.08.003
Kluever B. M., Breck S. W., Howery L. D., Krausman P. R., Bergman D. L. (2008). Vigilance in cattle: The influence of predation, social interactions, and environmental factors. Rangel. Ecol. Manage. 61, 321–328. doi: 10.2111/07-087.1
Knight C. W., Bailey D. W., Faulkner D. (2018a). Low-cost global positioning system tracking collars for use on cattle. Rangel. Ecol. Manage. 71, 506–508. doi: 10.1016/j.rama.2018.04.003
Knight C. W., Bailey D. W., Grubb K., Mandeville W., Wilker J. (2018b). Data correction and analysis of spatial data using lower-cost global positioning system tracking collars. 71st Soc. Range Management Sparks NV (Reno NV). doi: 10.1016/j.rama.2018.04.003
Knox N. M., Skidmore A. K., Prins H. H. T., Heitkönig I. M. A., Slotow R., van der Waal C., et al. (2012). Remote sensing of forage nutrients: Combining ecological and spectral absorption feature data. ISPRS J. Photogramm. Remote Sens. 72, 27–35. doi: 10.1016/j.isprsjprs.2012.05.013
Laurence-Traynor A. (2020) Evaluating field-based grazing intensity measurements for adaptive rangeland monitoring. Available at: http://www.akrabjuara.com/index.php/akrabjuara/article/view/919.
Lazo A. (1994). Social segregation and the maintenance of social stability in a feral cattle population. Anim. Behav. 48, 1133–1141.
Lewis Baida B. E., Swinbourne A. M., Barwick J., Leu S. T., van Wettere W. H. E. J. (2021). Technologies for the automated collection of heat stress data in sheep. Anim. Biotelemetry 9, 1–15. doi: 10.1186/s40317-020-00225-9
Lima S. L., Dill L. M. (1990). Behavioral decisions made under the risk of predation: a review and prospectus. Can. J. Zool. 68, 619–640. doi: 10.1139/z90-092
Lund H. G. (2007). Accounting for the world’s rangelands. Rangelands 29, 3–10. doi: 10.2111/1551-501X(2007)29
Macdonald D. W. (1978). ““Radio-tracking: some applications and limitations,”,” in Animal marking: recognition marking of animals in research. Ed. Stenhouse G. B. (London, United Kingdom: Macmillan), 192–204.
Manning J., Power D., Cosby A. (2021). Legal complexities of animal welfare in australia: Do on-animal sensors offer a future option? Animals 11, 1–15. doi: 10.3390/ani11010091
Marques R. S., Cooke R. F., Francisco C. L., Bohnert D. W. (2012). Effects of twenty-four hour transport or twenty-four hour feed and water deprivation on physiologic and performance responses of feeder cattle. J. Anim. Sci. 90, 5040–5046. doi: 10.2527/jas.2012-5425
McCulloch S. P. (2013). A critique of FAWC’s five freedoms as a framework for the analysis of animal welfare. J. Agric. Environ. Ethics 26, 959–975. doi: 10.1007/s10806-012-9434-7
McGranahan D. A., Geaumont B., Spiess J. W. (2018). Assessment of a livestock GPS collar based on an open-source datalogger informs best practices for logging intensity. Ecol. Evol. 8, 5649–5660. doi: 10.1002/ece3.4094
McIlvain E. H., Shoop M. C. (1971). Shade for improving cattle grains and rangeland use. J. Range Manage. 24, 181–184.
Mee J. F. (2008). Prevalence and risk factors for dystocia in dairy cattle: A review. Vet. J. 176, 93–101. doi: 10.1016/j.tvjl.2007.12.032
Meijering A. (1984). Dystocia and stillbirth in cattle - a review of causes, relations and implications. Livest. Prod. Sci. 11, 143–177. doi: 10.1016/0301-6226(84)90057-5
Mellor D. J. (2016a). Moving beyond the “Five freedoms” by updating the “five provisions” and introducing aligned “animal welfare aims”. Animals 6, 1–7. doi: 10.3390/ani6100059
Mellor D. J. (2016b). Updating animal welfare thinking: Moving beyond the “five freedoms” towards “A lifeworth living”. Animals 6, 1–20. doi: 10.3390/ani6030021
Mellor D. J. (2017). Operational details of the five domains model and its key applications to the assessment and management of animal welfare. Animals 7, 1–20. doi: 10.3390/ani7080060
Mellor D. J., Beausoleil N. J. (2015). Extending the “Five domains” model for animal welfare assessment to incorporate positive welfare states. Anim. Welf. 24, 241–253. doi: 10.7120/09627286.24.3.241
Mellor D. J., Reid C. S. W. (1994). Concepts of animal well-being and predicting the impact of procedures on experimental animals. Improving the well-being of animals in the research environment, 3–18.
Morgan-Davies C., Lambe N., Wishart H., Waterhouse T., Kenyon F., McBean D., et al. (2018). Impacts of using a precision livestock system targeted approach in mountain sheep flocks. Livest. Sci. 208, 67–76. doi: 10.1016/j.livsci.2017.12.002
Neo J. P. S., Tan B. H. (2017). The use of animals as a surveillance tool for monitoring environmental health hazards, human health hazards and bioterrorism. Vet. Microbiol. 203, 40–48. doi: 10.1016/j.vetmic.2017.02.007
Nyamuryekung’e S., Cibils A. F., Estell R. E., VanLeeuwen D., Steele C., Estrada O. R., et al. (2020). Do young calves influence movement patterns of nursing raramuri criollo cows on rangeland? Rangel. Ecol. Manage. 73, 84–92. doi: 10.1016/j.rama.2019.08.015
O’Callaghan K. A., Cripps P. J., Downham D. Y., Murray R. D. (2003). Subjective and objective assessment of pain and discomfort due to lameness in dairy cattle. Anim. Welf. 12, 605–610.
OECD - FAO (2018). OECD-FAO agricultural outlook 2018-2027 (Rome, Italy: OECD Publsihing). doi: 10.1787/agr_outlook-2018-en
O’Leary N. W., Byrne D. T., Garcia P., Werner J., Cabedoche M., Shalloo L. (2020). Grazing cow behavior’s association with mild and moderate lameness. Animals 10, 1–11. doi: 10.3390/ani10040661
Olson B. E., Wallander R. T. (2002). Influence of winter weather and shelter on activity patterns of beef cows. Can. J. Anim. Sci. 82, 491–501. doi: 10.4141/A01-070
Owens M. K., Launchbaugh K. L., Holloway J. W. (1991). Pasture characteristics affecting spatial distribution of utilization by cattle in mixed brush communities. J. Range Manage. 44, 118–123. doi: 10.2307/4002308
Petherick J. C. (2005). Animal welfare issues associated with extensive livestock production: The northern Australian beef cattle industry. Appl. Anim. Behav. Sci. 92, 211–234. doi: 10.1016/j.applanim.2005.05.009
Piette D., Exadaktylos V., Berckmans D. (2016). Presenting a methodology for continuous and automated monitoring of mental state in horses using wearable technology. in. Proc. 12th Int. Equitation Sci. Conf. 31, 1.
Poulopoulou I., Lambertz C., Gauly M. (2019). Are automated sensors a reliable tool to estimate behavioural activities in grazing beef cattle? Appl. Anim. Behav. Sci. 216, 1–5. doi: 10.1016/j.applanim.2019.04.009
Pringle M. J., O’Reagain P. J., Stone G. S., Carter J. O., Orton T. G., Bushell J (2021). Using remote sensing to forecast forage quality for cattle in the dry savannas of northeast Australia. J. Ecol. Indic. 133, 108426. doi: 10.1016/j.ecolind.2021.108426
Provenza F. D. (2008). What does it mean to be locally adapted and who cares anyway? J. Anim. Sci. 86. doi: 10.2527/jas.2007-0468
Rasby R. J., Walz T. (2011)Water requirements for beef catle. In: Univ. Nebraska-Lincoln ext. Available at: https://extensionpublications.unl.edu/assets/pdf/g2060.pdf (Accessed February 2, 2022).
Raynor E. J., Gersie S. P., Stephenson M. B., Clark P. E., Spiegal S. A., Boughton R. K., et al. (2021). Cattle grazing distribution patterns related to topography across diverse rangeland ecosystems of north America. Rangel. Ecol. Manage. 75, 91–103. doi: 10.1016/j.rama.2020.12.002
Reeves M. C., Mitchell J. E. (2011). Extent of coterminous US rangelands: Quantifying implications of differing agency perspectives. Rangel. Ecol. Manage. 64, 585–597. doi: 10.2111/REM-D-11-00035.1
Riaboff L., Relun A., Petiot C. E., Feuilloy M., Couvreur S., Madouasse A. (2021). Identification of discriminating behavioural and movement variables in lameness scores of dairy cows at pasture from accelerometer and GPS sensors using a partial least squares discriminant analysis. Prev. Vet. Med. 193, 105383. doi: 10.1016/j.prevetmed.2021.105383
Riley D. G., Chase C. C., Olson T. A., Coleman S. W., Hammond A. C. (2004). Genetic and nongenetic influences on vigor at birth and preweaning mortality of purebred and high percentage Brahman calves. J. Anim. Sci. 82, 1581–1588. doi: 10.2527/2004.8261581x
Ritchie H., Roser M. (2013) Meat and dairy production. l. use. Available at: https://ourworldindata.org/meat-production (Accessed November 10, 2021).
Robertshaw D., Damiel R. (1983). The effect of dehydration on the control of panting and sweating in the black bedouin goat. Physiol. Zool. 56, 412–418.
Rosa G. J. M. (2021). Grand challenge in precision livestock farming. Front. Anim. Sci. 2. doi: 10.3389/fanim.2021.650324
Rushen J., Haley D., De Passille A. M. (2007). Effect of softer flooring in tie stalls on resting behavior and leg injuries of lactating cows. J. Dairy Sci. 90, 3647–3651. doi: 10.3168/jds.2006-463
Rutter S. M. (2014). Smart technologies for detecting animal welfare status and delivering health remedies for rangeland systems. OIE Rev. Sci. Tech. 33, 181–187. doi: 10.20506/rst.33.1.2274
Sanchez-Iborra R., Sanchez-Gomez J., Ballesta-Viñas J., Cano M. D., Skarmeta A (2018). Performance evaluation of lora considering scenario conditions. F. Sensors (Switzerland) 18, 1–19. doi: 10.3390/s18030772
Sato S. (1982). Leadership during actual grazing in a small herd of cattle. Appl. Anim. Ethol. 8, 53–65. doi: 10.1016/0304-3762(82)90132-8
Schuenemann G. M., Nieto I., Bas S., Galvão K. N., Workman J. (2011). Assessment of calving progress and reference times for obstetric intervention during dystocia in Holstein dairy cows. J. Dairy Sci. 94, 5494–5501. doi: 10.3168/jds.2011-4436
Siebert B. D., Macfarlane W. V. (1969). Body water content and water turnover of tropical bos taurus, bos indicus, bibos banteng, and bos bubalus bubalis. Austrailian J. Agric. Res. 20, 613–622.
Siebert B. D., Macfarlane W. V. (1975). Dehydration in desert cattle and camels. Physiol. Zool. 48, 36–48.
Smith L., Ruyle G., Maynard J., Barker S., Meter W., Stewart D., et al. (2007). Principles of obtaining and interpreting untilization data on rangelands. Univ. Arizona Ext. Publ. AZ1375, 1–12.
Soriani N., Panella G., Calamari L. (2013). Rumination time during the summer season and its relationships with metabolic conditions and milk production. J. Dairy Sci. 96, 5082–5094. doi: 10.3168/jds.2013-6620
Soriani N., Trevisi E., Calamari L. (2012). Relationships between rumination time, metabolic conditions, and health status in dairy cows during the transition period. J. Anim. Sci. 90, 4544–4554. doi: 10.2527/jas.2012-5064
Sprinkle J. E., Sagers J. K., Hall J. B., Ellison M. J., Yelich J. V., Brennan J. R., et al. (2021). Predicting cattle grazing behavior on rangeland using accelerometers. Rangel. Ecol. Manage. 76, 157–170. doi: 10.1016/j.rama.2020.10.001
Stangaferro M. L., Wijma R., Caixeta L. S., Al-Abri M. A., Giordano J. O. (2016a). Use of rumination and activity monitoring for the identification of dairy cows with health disorders: Part i. metabolic and digestive disorders. J. Dairy Sci. 99, 7395–7410. doi: 10.3168/jds.2`016-10907
Stangaferro M. L., Wijma R., Caixeta L. S., Al-Abri M. A., Giordano J. O. (2016b). Use of rumination and activity monitoring for the identification of dairy cows with health disorders: Part II. mastitis. J. Dairy Sci. 99, 7411–7421. doi: 10.3168/jds.2016-10908
Stangaferro M. L., Wijma R., Caixeta L. S., Al-Abri M. A., Giordano J. O. (2016c). Use of rumination and activity monitoring for the identification of dairy cows with health disorders: Part II. mastitis. J. Dairy Sci. 99, 7411–7421. doi: 10.3168/jds.2016-10908
Stephenson M. B., Bailey D. W. (2017). Do movement patterns of GPS-tracked cattle on extensive rangelands suggest independence among individuals? Agriculture 7, 1–17. doi: 10.3390/agriculture7070058
Stephenson M. B., Bailey D. W., Bruegger R. A., Howery L. D. (2017). Factors affecting the efficacy of low-stress herding and supplement placement to target cattle grazing locations. Rangel. Ecol. Manage. 70, 202–209. doi: 10.1016/j.rama.2016.08.007
Suji Prasad S. J., Thangatamilan M., Suresh M., Panchal H., Rajan C. A., Sagana C., et al. (2021). An efficient LoRa-based smart agriculture management and monitoring system using wireless sensor networks. Int. J. Ambient Energy. doi: 10.1080/01430750.2021.1953591
Swinker A. (2014)How much drinking waer does your horse need? In: Penn State ext. Available at: https://extension.psu.edu/how-much-drinking-water-does-your-horse-need (Accessed February 2, 2022).
Sykes S. (2017)Water for small ruminants. In: North Carolina coop. ext. Available at: http://go.ncsu.edu/readext?476919 (Accessed February 2, 2022).
Taylor D. B., Schneider D. A., Brown W. Y., Price I. R., Trotter M. G., Lamb D. W., et al. (2011). GPS Observation of shelter utilisation by merino ewes. Anim. Prod. Sci. 51, 724–737. doi: 10.1071/AN11025
Thorup V. M., Munksgaard L., Robert P.-E., Erhard H. W., Thomsen P. T., Friggens N. C. (2015). Lameness detection via leg-mounted accelerometers on dairy cows on four commercial farms. Animal 9, 1704–1712. doi: 10.1017/s1751731115000890
Tobin C. T., Bailey D. W., Stephenson M. B., Trotter M. G. (2021a). Temporal changes in association patterns of cattle grazing at two stocking densities in a central Arizona rangeland. Animals 11, 1–14. doi: 10.3390/ani11092635
Tobin C. T., Bailey D. W., Trotter M. G. (2021b). Tracking and sensor-based detection of livestock water system failure : A case study simulation. Rangel. Ecol. Manage. 77, 9–16. doi: 10.1016/j.rama.2021.02.013
Tobin C. T., Bailey D. W., Trotter M. G., O’Connor L. (2020). Sensor based disease detection : A case study using accelerometers to recognize symptoms of bovine ephemeral fever. Comput. Electron. Agric. 175, 1–5. doi: 10.1016/j.compag.2020.105605
Tzounis A., Katsoulas N., Bartzanas T., Kittas C. (2017). Internet Of things in agriculture, recent advances and future challenges. Biosyst. Eng. 164, 31–48. doi: 10.1016/j.biosystemseng.2017.09.007
Underwood W. J. (2002). Pain and distress in agricultural animals. J. Am. Vet. Med. Assoc. 221, 208–211. doi: 10.2460/javma.2002.221.208
Vandenheede M., Nicks B., Canart B., Dufrasne I., Biston R., Lecomte P. (1992). Modalites d’utilisation d’un abri per des taurillons au paturage. Ann. Med. Vet. 136, 321–325.
Van Soest P. J. (1994). Nutritional ecology of the ruminant (Ithaca, NY: Cornell Univeristy Press). doi: 10.7591/9781501732355
Watanabe N., Sakanoue S., Kawamura K., Kozakai T. (2008). Development of an automatic classification system for eating, ruminating and resting behavior of cattle using an accelerometer. Grassl. Sci. 54, 231–237. doi: 10.1111/j.1744-697x.2008.00126.x
Webster J. (2016). Animal welfare: Freedoms, dominions and “A life worth living”. Animals 6, 2–7. doi: 10.3390/ani6060035
Werkheiser I. (2018). Precision livestock farming and farmers’ duties to livestock. J. Agric. Environ. Ethics 31, 181–195. doi: 10.1007/s10806-018-9720-0
White R. R., Hall M. B., Firkins J. L., Kononoff P. J. (2017). Physically adjusted neutral detergent fiber system for lactating dairy cow rations. I: Deriving equations that identify factors that influence effectiveness of fiber. J. Dairy Sci. 100, 9551–9568. doi: 10.3168/jds.2017-12765
Williams L. R., Bishop-Hurley G. J., Anderson A. E., Swain D. L. (2019). Application of accelerometers to record drinking behaviour of beef cattle. Anim. Prod. Sci. 59, 122–132. doi: 10.1071/AN17052
Williams M. L., Mac Parthaláin N., Brewer P., James W. P. J., Rose M. T. (2016). A novel behavioral model of the pasture-based dairy cow from GPS data using data mining and machine learning techniques. J. Dairy Sci. 99, 2063–2075. doi: 10.3168/jds.2015-10254
Williams L. R., Moore S. T., Bishop-Hurley G. J., Swain D. L. (2020). A sensor-based solution to monitor grazing cattle drinking behaviour and water intake. Comput. Electron. Agric. 168, 1–9. doi: 10.1016/j.compag.2019.105141
Wishart H., Morgan-Davies C., Waterhouse A. (2015). A PLF approach for allocating supplementary feed to pregnant ewes in an extensive hill sheep system. Precis. Livest. Farming 15, 256–265.
Woods J., Shearer J. K., Hill J. (2010). ““Recommended on-farm euthanasia practices,”,” in Improving animal welfare: A practical approach. Ed. Grandin T. (Cambridge, MA: CABI), 186–213.
Keywords: animal well-being, grazing livestock, GPS tracking, accelerometer, disease, five freedoms
Citation: Tobin CT, Bailey DW, Stephenson MB, Trotter MG, Knight CW and Faist AM (2022) Opportunities to monitor animal welfare using the five freedoms with precision livestock management on rangelands. Front. Anim. Sci. 3:928514. doi: 10.3389/fanim.2022.928514
Received: 25 April 2022; Accepted: 11 July 2022;
Published: 02 August 2022.
Edited by:
Carolina Pugliese, University of Florence, ItalyReviewed by:
Danila Marini, Commonwealth Scientific and Industrial Research Organisation (CSIRO), AustraliaHayley Randle, Charles Sturt University, Australia
Copyright © 2022 Tobin, Bailey, Stephenson, Trotter, Knight and Faist. This is an open-access article distributed under the terms of the Creative Commons Attribution License (CC BY). The use, distribution or reproduction in other forums is permitted, provided the original author(s) and the copyright owner(s) are credited and that the original publication in this journal is cited, in accordance with accepted academic practice. No use, distribution or reproduction is permitted which does not comply with these terms.
*Correspondence: Colin T. Tobin, Y29saW4udG9iaW5AbmRzdS5lZHU=