- 1University of Tennessee, Department of Animal Science, Knoxville, TN, United States
- 2USDA-ARS US Meat Animal Research Center, Clay Center, NE, United States
- 3University of Tennessee, Department of Chemistry, Knoxville, TN, United States
- 4University of Tennessee, Biological and Small Molecule Mass Spectrometry Core, Knoxville, TN, United States
The effects of growth-promoting implants have been well-defined for their ability to impact growth performance in beef cattle. Production-relevant microbes and microbiomes in the rumen have also been associated with growth traits. However, the role of implants on the rumen microbiome has not been determined. The objective of this study was to determine if different doses of implant hormones cause gain-associated ruminal microbial community changes. To assess this, a completely randomized design was used and 336 fall-born steers 450 to 470 days of age from the germplasm evaluation population at the US Meat Animal Research Center (Clay Center, NE) were divided into two treatment groups: 1) a moderate implant strategy (n = 167) of Revalor-IS (80 mg trenbolone acetate and 16 mg estradiol) followed by Revalor-S (120 mg trenbolone acetate and 24 mg estradiol) or 2) an aggressive implant strategy (n = 169) of Revalor-IS followed by Revalor-200 (200 mg trenbolone acetate and 20 mg estradiol). Steers were fed the same diet (57.0% dry-rolled corn, 30% wet distiller’s grains with solubles, 8.0% alfalfa hay, 4.25% vitamin and mineral supplement, and 0.75% urea, on a DM basis). On d 85 after implants administration, rumen contents were collected via orogastric tubing. Samples were sequenced to target and identify bacteria, archaea, and protozoa. Untargeted metabolomics was performed on rumen content using ultra high performance liquid chromatography high resolution mass spectrometry. Production data between implant strategies was analyzed using a mixed model ANOVA (SASv9.4, Cary, NC) followed by separation of least squares means. Microbial diversity between strategies did not differ for archaea or protozoa (P > 0.05). Average daily gain was different (P = 0.01; 1.72 vs 1.66 ± 0.02 kg, aggressive vs moderate, respectively); however, large microbial community shifts were not associated with implant strategy. Two metabolites, N-acetyllysine and N-acetylornithine, were found in greater abundance in the moderate implant strategy (P ≤ 0.04). Understanding associations between the rumen microbiome and implant strategies may allow improvement of growth efficiency in beef cattle.
Introduction
The effects of growth-promoting implants have been well defined for their improvement of growth performance in beef cattle. These implants not only increase average daily gain (ADG), but also can also increase feed efficiency. Implants have been demonstrated to increase ADG by 30% and feed efficiency by 15%, ultimately serving as one of the most effective growth enhancing technologies available to feedlot operations (Duckett et al., 1996; Preston, 1999). In addition, implant strategies can be categorized by their ability to affect growth through the amounts and types of growth-promoting steroid hormones present within the implants. These hormones consist of natural or synthetic versions of estradiol, progesterone, or testosterone that synergistically work to augment weight gain (Parr, 2020). Growth-promoting implant strategies often involve re-implanting cattle, especially ones on feed for more than 200 days. Using multiple implants allows producers to capture an increase in gain between 5 to 20%, making these strategies critical to feedlot operation profitability (Preston, 1999).
While multiple implant brands are available on the market, varying in potency of estrogenic and/or androgenic compounds, Revalor implants primarily consist of a synthetic testosterone, trenbolone acetate (TBA), and estradiol (E2). Revalor terminal implant strategies primarily consist of an all-around, or moderate, re-implant strategy whereas an aggressive re-implant strategy contains higher doses of the active ingredients (Merck Animal Health, Kenilworth, NJ, USA). The mechanisms by which TBA and E2 directly alter weight gain have been studied in depth (Lee et al., 1990; Hunt et al., 1991; Perry et al., 1991); impacts on muscle tissue and transcriptomes of the muscle and liver have been reported describing the effects of these growth-promoting hormones (Elgendy et al., 2016). Studies have identified the importance of estrogenic and androgenic compounds on abundances of insulin-like growth factor I (IGF-I) and growth hormones (GH) that contribute to live weight and carcass weight (Hayden et al., 1992; Johnson et al., 1996a; Johnson et al., 1996b; Smith et al., 2018; Ohnoutka et al., 2021). While direct effects of androgenic compounds increasing muscle cell proliferation are well-understood, estrogenic compounds often have indirect effects on metabolites present as well as transcriptomes in the liver to increase feed efficiency and daily gain (Elgendy et al., 2016).
Hormones, such as TBA and E2, could be interacting with receptors present on other tissues, namely the gastrointestinal tract. Steroid hormones have previously been studied for their impacts on metabolism and nutrient absorption (Arjmandi et al., 1993), as estrogen receptor (ER) subtypes ERα and ERβ and androgen receptors (AR) have been identified on multiple gastrointestinal tissues (Sauerwein et al., 1995; Pfaffl et al., 2003). When analyzing mRNA expression in the bovine gastrointestinal tract, high ERα/ERβ ratios were examined in the rumen in addition to high AR mRNA expression (Pfaffl et al., 2003). This interaction of estrogenic and androgenic compounds with rumen wall tissue could indicate downstream biological interactions with the ruminal microbiome. Previous studies have identified some impacts of E2 with bacterial communities, specifically noting 17β-estradiol degradation via the ruminal microbial communities (Isabelle et al., 2011). The interaction between the microbial communities and steroid hormones could influence shifts in the microbiome that leading to alterations in growth and metabolic activity.
The rumen microbiome has been studied in depth to demonstrate the importance of microbial communities on feed efficiency and gain in beef cattle (Myer et al., 2015). Previous studies have primarily focused on bacterial communities because of ease of technology and prevalence of bacterial communities in the gut. However, archaeal and protozoal communities also play important roles, namely in methane production and nitrogen cycling in the rumen which contribute to feed efficiency and growth (Hegarty et al., 2007; Li et al., 2017). Furthermore, investigations of metabolic activity are being performed to understand relationships between microbial communities and production-relevant phenotypes (Artegoitia et al., 2017; Clemmons et al., 2020; Foroutan et al., 2020). Elucidating the interaction between the rumen microbiome and metabolome can improve understanding of ruminal microbiome and host interactions.
With the increased demand for sustainably-produced beef, there is a critical need for an improvement in feed efficiency and growth performance in beef cattle. The presence of estrogenic hormone receptors on different tissues, namely the gastrointestinal tract, may indicate the influence of these growth-promoting hormones on the rumen microbial communities, indirectly or synergistically improving gain and potentially altering ruminal metabolic activity. The objective of this study was to examine the rumen microbial communities and their metabolic features impacted by two growth-promoting implant strategies that may be influencing feed efficiency and growth in beef cattle.
Methods and Materials
Animal Ethics Statement
The U.S. Meat Animal Research Center (MARC) Animal Care and Use Committee approved procedures utilized on animals in this study.
Experimental Design
Using a completely randomized design, germplasm evaluation composite breed steers 300-350 days of age from U.S. MARC (n = 336) were placed into feedlot pens. These steers consist of the 18 breeds in the U.S. with the highest registered animal numbers from breed association data. Steers were given ad libitum access to water and gradually adjusted to a feedlot diet consisting of 87% concentrate with vitamin and mineral supplements containing Rumensin and Tylan (Table 1). Unshrunk body weight was measured once a month throughout the entire study with an average initial body weights of 439.8 ± 43.1 kg.
A moderate or an aggressive implant strategy treatment was randomly assigned to steers and balanced across breed. The moderate implant strategy (n = 167) consisted of an initial implant of Revalor-IS (80 mg trenbolone acetate and 16 mg estradiol) followed by the re-implantation treatment of Revalor-S (120 mg trenbolone acetate and 24 mg estradiol), which is an all-around moderate implant strategy suggested by Merck Animal Health (Kenilworth, NJ, USA). The aggressive implant strategy (n = 169) consisted of an initial implant of Revalor-IS, followed by the re-implantation treatment of Revalor-200 (200 mg trenbolone acetate and 20 mg estradiol), per suggestions by Merck Animal Health. The initial implant was administered 60 days after adjustment to the high-concentrate diet, with the re-implantation of the treatment implants administered 90 days after the initial implant of Revalor-IS.
After 85 days after the terminal implant, 200 mL of rumen content was sampled from each animal via orogastric tubing. A ½-inch tube was connected to a vacuum flask and vacuum pumped to sample ruminal liquid content with fibrous particles. Tubes were discarded between animals to prevent cross contamination. Ruminal content from individual animals was separated into 50 mL conical tubes and snap-frozen in liquid nitrogen. Samples were then stored at -80°C until further analysis.
DNA Extraction, Amplification, and Purification of Ruminal Content
Rumen samples containing fiber and content were sent to the University of Tennessee, Knoxville, TN for DNA extraction and purification, following the rumen digesta extraction protocol previously described by (Yu and Morrison, 2004). Following extraction and purification, the resultant DNA was then used for 16S and 18S rRNA amplicon sequencing, undergoing polymerase chain reaction (PCR) library construction. Primers for DNA amplification are listed in Table 2. Amplicon libraries of the 16S rRNA gene for bacteria (V1-V3) were prepared as described (Kozich et al., 2013), using primers 27F (Stahl, 1991) and 519R (Lane et al., 1985). As used in (Abbas et al., 2020), each 20 μL PCR amplification reaction contained 0.5 μL Terra PCR Direct Polymerase Mix (0.625 Units), 7.5 μL nuclease-free sterile water, 10 μL 2X Terra PCR Direct Buffer, 1 μL indexed fusion primers (10 μM), and 1 μL DNA (20 to 70 ng). Thermocycling conditions were as follows: initial denaturation at 98°C for 3 minutes, 25 cycles at 98°C for 30 seconds, 55°C for 30 seconds, and 68°C for 45 seconds, and a final extension of 68°C for 4 minutes.
For archaeal communities, amplicon libraries of the 16S rRNA gene for archaea (V3-V4) were prepared using primers ARC344F_TS (Wemheuer et al., 2012) and ARCH806R_TS (Takai and Horikoshi, 2000), found in Table 2. Each 10 μL run contained 4.2 μL nuclease-free sterile water, 1.25 μL 10X Buffer, 0.65 μL 25 mM MgCl2, 0.2 μL 25 mM dNTPs, 0.5 μL FC1:TS1 primer (4 μM), 0.2 μL HotStar Taq (QIAGEN, Valencia, CA, USA), 1 μL of bacterial indexes (1.875 μM), 1 μL of methanogen primers (1 μM), and 1 μL of DNA (15 ng/μL). Thermocycler conditions were as follows: 95°C for 15 minutes, 8 cycles of 94°C for 1 minute, 55°C for 30 seconds, and 72°C for 45 seconds, 24 cycles of 94°C for 20 seconds, 62°C for 30 seconds, and 72°C for 30 seconds, and a final extension of 72°C for 5 minutes.
Amplicon libraries of the 18S rRNA gene for protozoa (V3-V4) were prepared using primers P-SSU-316F (Sylvester et al., 2004) and GIC758R (Ishaq and Wright, 2014) (Table 2). Each 10 μL reaction consisted of 3.2 μL nuclease-free sterile water, 1 μL 10X Buffer, 0.65 μL 25 mM MgCl2, 0.2 μL 25 mM dNTPs, 1 μL protozoal primers (0.5 μM), 0.5 μL FC1:TS1 primer (4 μM), 0.2 μL HotStar Taq (QIAGEN, Valencia, CA, USA), 1 μL barcode, and 1 μL DNA (15 ng/μL). Thermocycler conditions were as follows: 95°C for 15 minutes, 8 cycles of 94°C for 1 min, 50°C for 30 seconds, and 72°C for 45 seconds, and 24 cycles of 94°C for 20 seconds, 62°C for 30 seconds, and 72°C for 30 seconds, and a final extension of 72°C for 5 minutes.
The libraries were sequenced using the V2 500-cycle kit on an Illumina MiSeq (Illumina, San Diego, CA, USA).
Bioinformatic Analyses in R
The Illumina 2x250 fastq sequencing files for bacterial and archaeal communities were processed through an R pipeline as described by (Callahan et al., 2016b). Fastq Illumina files were entered into R and open-source R packages ‘phyloseq’ (McMurdie and Holmes, 2013) and ‘DADA2’ (Callahan et al., 2016a) were used to perform filtering, merging, and taxonomic assignment. Forward and reverse reads were trimmed based on a quality score of 25 or greater. The expected errors per read were set at two for forward reads and four for reverse reads, thus filtering out any data not meeting these criteria (Edgar and Flyvbjerg, 2015). Following trimming and filtering of reads, ‘DADA2’ was used to identify real variants and correct for amplicon errors using a naïve Bayesian classifier (Wang et al., 2007; Callahan et al., 2016a). From DADA2, amplicon sequence variants (ASVs) were generated, which can moderately increase genetic resolution in comparison to 97% operational taxonomic units (OTUs) (Callahan et al., 2017; Glassman and Martiny, 2018). Quality-filtered forward and reverse reads were then merged and chimeras were removed. Sequences underwent taxonomic assignment to the genus level using the SILVA v132 database (Quast et al., 2012). The chimeras, Cyanobacteria, and Eukaryota were removed from the data.
Protozoal fastq sequencing files were also processed through the same pipeline as described by (Callahan et al., 2016b) and utilized the same open-source R packages. Forward and reverse reads were trimmed based on quality score (Q ≥ 25). The expected errors per read were set at two for forward and five for reverse reads, thus, filtering out data not meeting these criteria. Samples with initially less than 10,000 input reads were removed. Then, DADA2 was used to identify ASVs, forward and reserve reads were merged, and chimeras were removed. Sequences underwent taxonomic assignment to the genus level using the SILVA v132 database, and singletons, Cyanobacteria, and any communities within the kingdom Bacteria were removed from the data.
Rumen Metabolite Extraction and Analysis
After removing from storage in a -80°C freezer, a 2 mL aliquot of rumen content was transferred to two sterile 2 mL microcentrifuge tubes and centrifuged at 6,000 x g for 15 minutes at 4°C. Supernatant was aspirated and combined from each tube using a 3 mL syringe and then filtered through a 0.22 μM syringe filter (MidSci, St. Louis, MO, USA) to a sterile 2 mL cryovial. Samples were stored at -20°C until analyzed. Samples were processed at the Biological and Small Molecule Mass Spectrometry Core (BSMMSC), University of Tennessee, Knoxville, TN (RRID: SCR_021368). Water soluble metabolites were extracted from 100 μL rumen content using 4:4:2 acetonitrile/methanol/water with 0.1 M formic acid as previously described by (Domingo-Almenara et al., 2019). Following extraction, the supernatant was dried under N2 then resuspended in 300 μL water. All solvents used were HPLC grade and purchased from Fisher Scientific (Hampton, NH, USA). Metabolites were identified using ultra high performance liquid chromatography high resolution mass spectrometry (UHPLC-HRMS) (Thermo Scientific, San Jose, CA, USA) with a previously validated untargeted metabolomics method by (Lu et al., 2010). Prior to mass analysis, reverse-phase (RP), ion-paring chromatography was used to separate metabolites based on polarity. A Synergi Hydro RP column (100 mm x 2.1 mm, 2.6 μm, 100 Å) and an UltiMate 3000 pump (Thermo Fisher) were used for chromatographic separation. An Exactive Plus Orbitrap MS (Thermo Fisher) was coupled to the UHPLC system and used for mass analysis.
Statistical Analyses
For bacterial, archaeal, and protozoal sequences, alpha- and beta-diversity metrics were analyzed in R. For alpha diversity, observed communities were calculated along with expected species (Chao1) as well as richness and diversity estimates were performed (Shannon). Alpha diversity was calculated individually for bacteria, archaea, and protozoa, and for each alpha diversity metric, differences between treatments were tested in SAS v9.4 (SAS Institute, Cary, NC, USA) using mixed model analysis of variance and Fisher’s least significant difference mean comparisons. Beta diversity was measured with a Bray-Curtis distance matrix producing a principal coordinates analysis (PCoA). Following, a PERMANOVA was conducted with 999 permutations to determine significance of the Bray-Curtis PCoA using ‘vegan’ in R (Oksanen et al., 2007). Differential abundances between treatments for bacterial, archaeal, and protozoal communities were individually calculated using R package ‘DESeq2’ (Anders and Huber, 2010; Love et al., 2014).
To visualize raw UHPLC-HRMS data and identify rumen metabolites, an open source metabolomics software package with a grouping algorithm and peak alignment feature, Metabolomic Analysis and Visualization Engine (MAVEN), was used. Metabolites were identified in MAVEN based on the exact mass and retention time of each metabolite (Clasquin et al., 2012). For all 115 identified metabolites, area under the curve was integrated and these data were used for further analysis. Data from MAVEN were processed and analyzed in MetaboAnalyst 4.0 (Chong et al., 2018), with significant pathways identified using a global test with relative-betweenness centrality topology analysis with the reference library of Escherichia coli K-12 MG1655 (Kanehisa et al., 2013). Rumen metabolites were imported into SAS v9.4 (SAS Institute, Cary, NC, USA) and normality was assessed using the PROC UNIVARIATE procedure on raw, untransformed data. Normally distributed data were identified using Shapiro-Wilks (W) score of ≥ 0.85 as well as visual assessment of histograms and residuals. Non-normal data (W < 0.85) were then log-transformed and analyzed with a mixed model analysis of variance (ANOVA) to compare between moderate and aggressive implant strategies with a covariate of initial body weight. To address multiple testing, a Fisher’s-protected LSD was used. Significance was determined using P = 0.05.
To understand associations between low abundance metabolites and implant treatment, binary variables were created to indicate the presence of absence of low abundance metabolites in each sample. The frequency procedure (PROC FREQ; SAS v9.4) was used to compare the presence or absence of these metabolites by each treatment using Chi Square analyses (SAS Institute, Cary, NC, USA). When low-abundance metabolites were found significantly different between moderate and aggressive implant strategies, least significant differences were used to differentiate between the ADG of moderate and aggressive implant strategies. Significance was determined using P = 0.05.
Results
Steer Performance
Steers within the aggressive implant strategy had significantly greater ADG (1.72 ± 0.02 kg) compared to steers within the moderate implant strategy (1.66 ± 0.02 kg; P < 0.02).
Ruminal Bacterial Communities
Within ruminal bacterial communities, the SILVA v138 database identified 18,381 taxa within 6 taxonomic ranks. Chimeras were identified to represent 2% of sequences after filtering. Following taxonomic assignment, alpha- and beta-diversity metrics were measured to identify richness and diversity. Observed species richness of bacterial communities in the rumen was determined from samples, then estimated richness of communities was calculated for the Chao1 metric, and samples were analyzed for species richness and diversity using Shannon diversity indices (Figure 1). Richness and evenness of species (Shannon) was different between the moderate and aggressive implant strategies (P < 0.01), however Chao1 and observed species were not (P > 0.05). A PCoA identified any dissimilarity among clustered samples, using the Bray-Curtis distance method (Figure 2). Beta diversity was analyzed with a PERMANOVA for the Bray-Curtis PCoA with no dissimilarity found between treatments (P > 0.05).
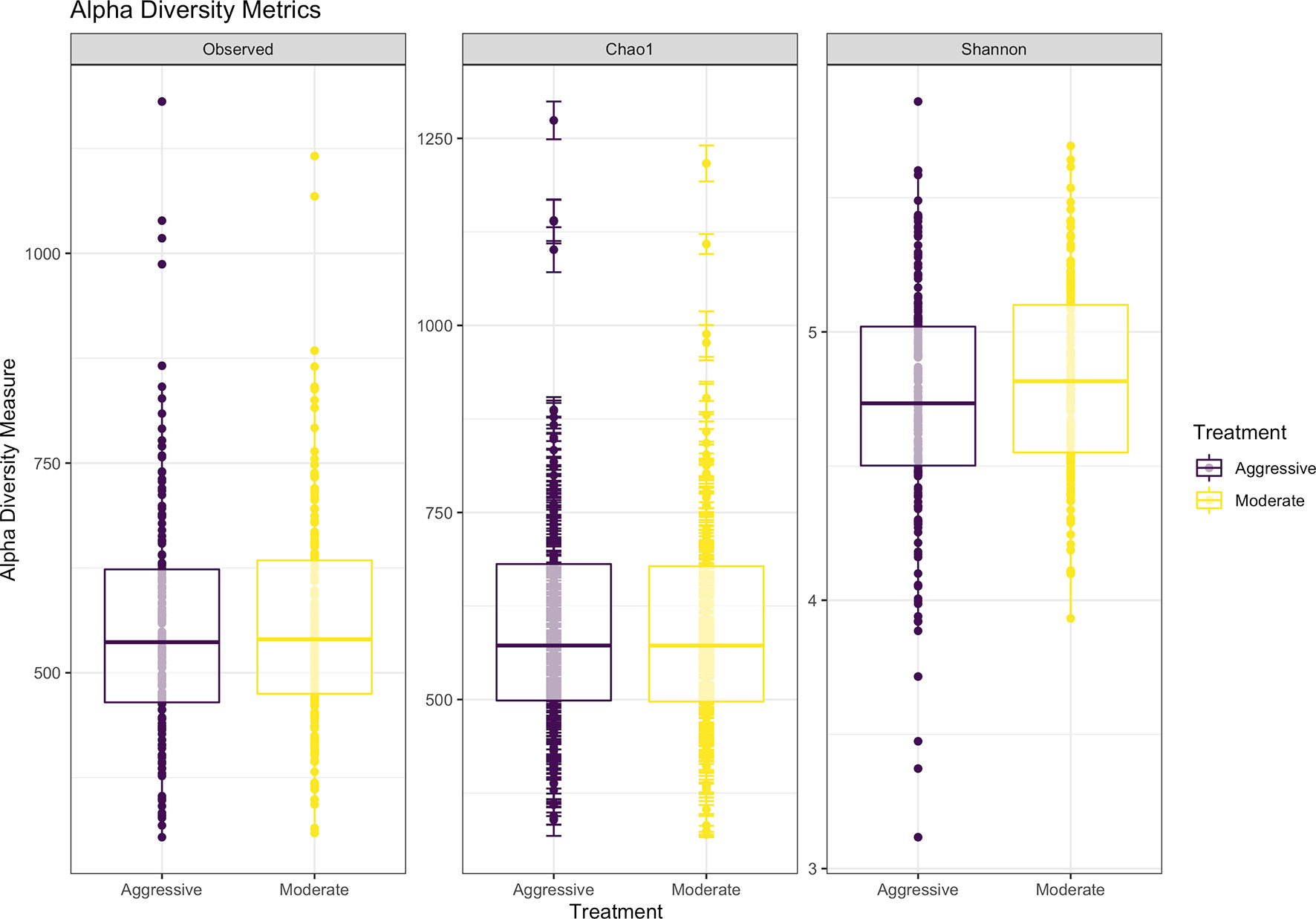
Figure 1 Alpha-diversity metrics for bacterial communities with observed abundances of communities, expected abundances (Chao1), and richness and diversity measured with Shannon diversity index. Aggressive treatments are represented in purple and moderate treatments are represented in yellow. Significant differences were only noted in the Shannon diversity index between treatments (P < 0.05).
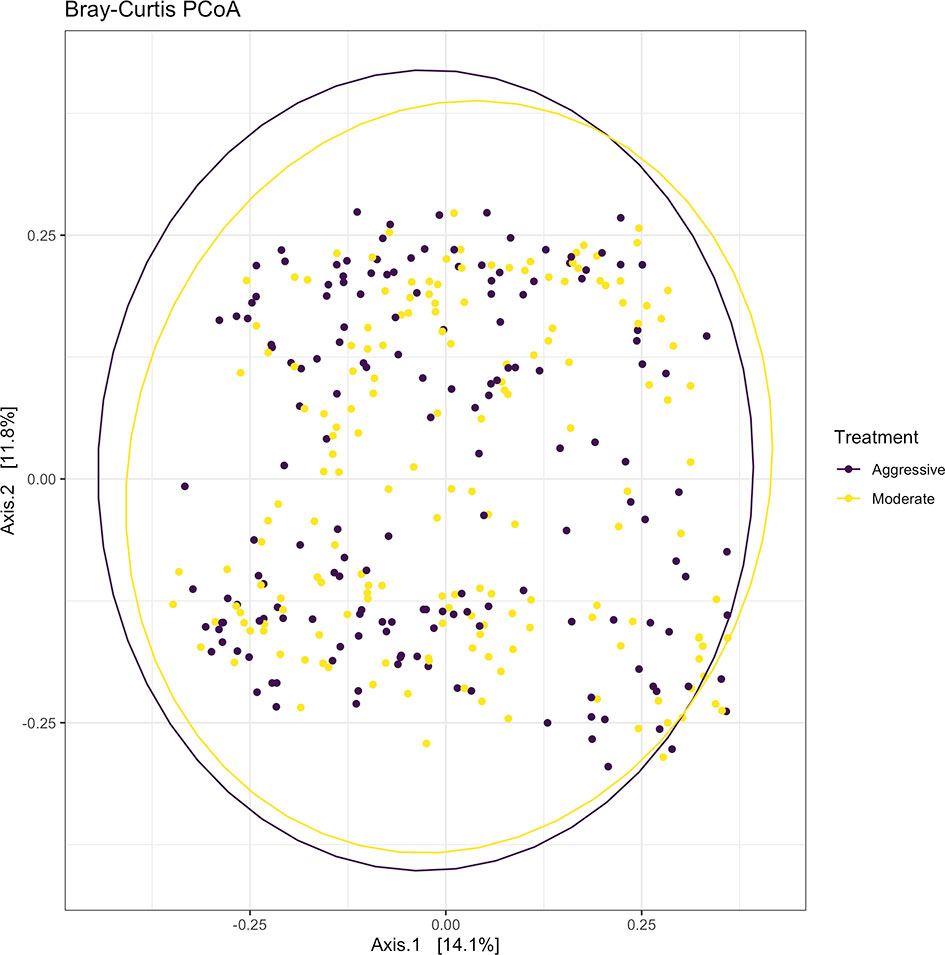
Figure 2 Beta-diversity measurements for bacterial communities using Bray-Curtis distances to create a PCoA. Aggressive treatments are represented in purple and moderate treatments are represented in yellow. Ellipses represent 95% confidence intervals around means for aggressive and moderate implant strategies. Significant overlap between communities reveals no differences between moderate or aggressive treatments.
There were no differences in relative abundance of any bacterial taxa between treatments. The majority of bacterial phyla identified in rumen content were Bacteroidota (52.79%) and Firmicutes (36.48%), as shown in Figure 3. The Proteobacteria accounted for 9.28% of reads. Many phyla represented less than 1% of relative abundance. Top 10 bacterial phyla present in rumen of cattle within both treatments are presented in Figure 3. Identified bacterial genera (Figure 4) consisted primarily of Prevotella, making up 38.28% of samples, but also Succininivibrionaceae UCG-001 (8.50%), Dialister (7.39%), Mitsuokella (2.42%), and others under 2% abundance. Presence of other genera were less than 1% of relative abundances in rumen fluid.
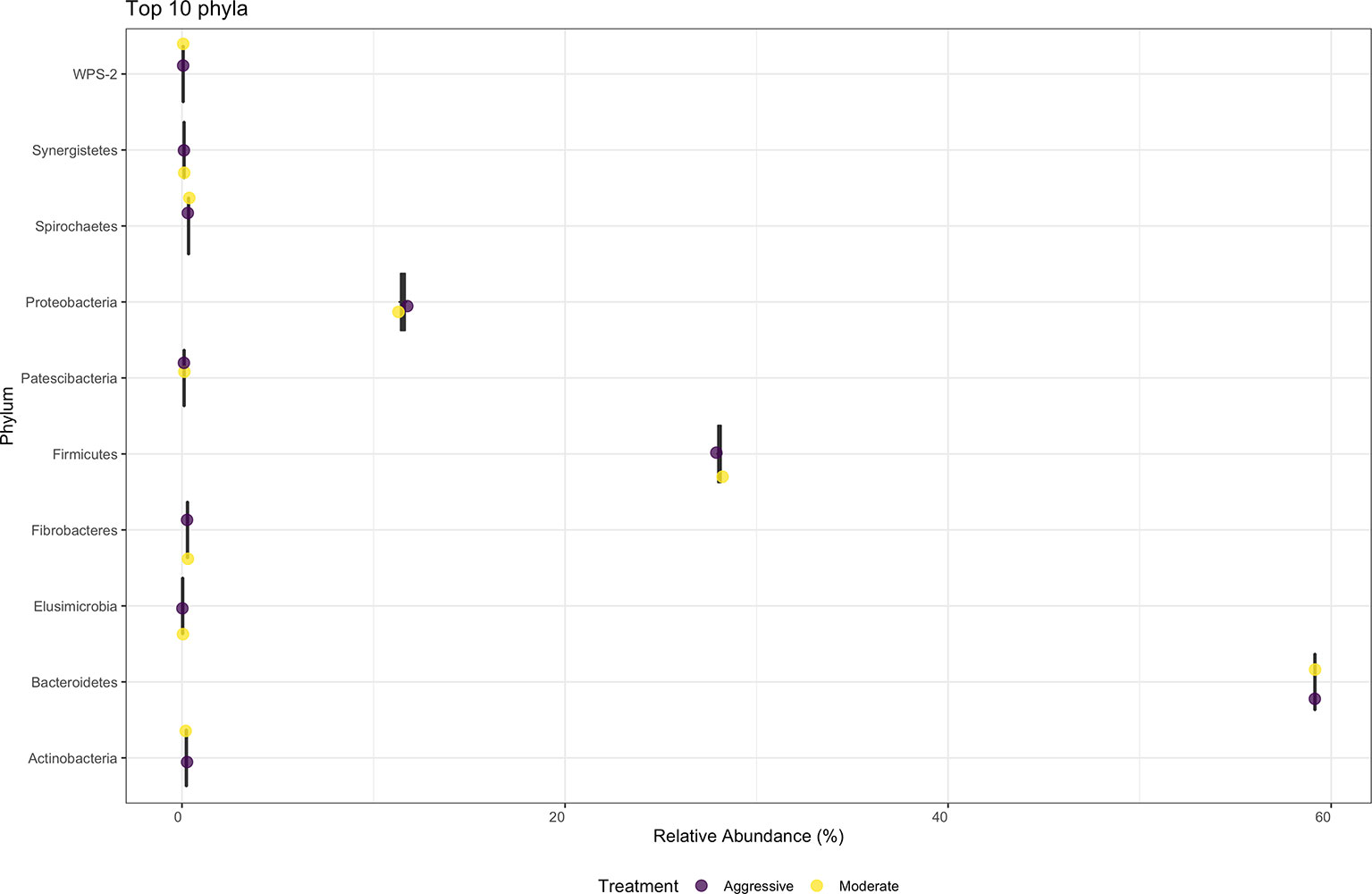
Figure 3 Average relative abundance of the top ten phylum-level bacterial communities classified in ruminal fluid between aggressive or moderate implant strategies. Mean relative abundance of bacterial communities associated with the aggressive implant strategy are represented in purple and moderate implant strategy in yellow.
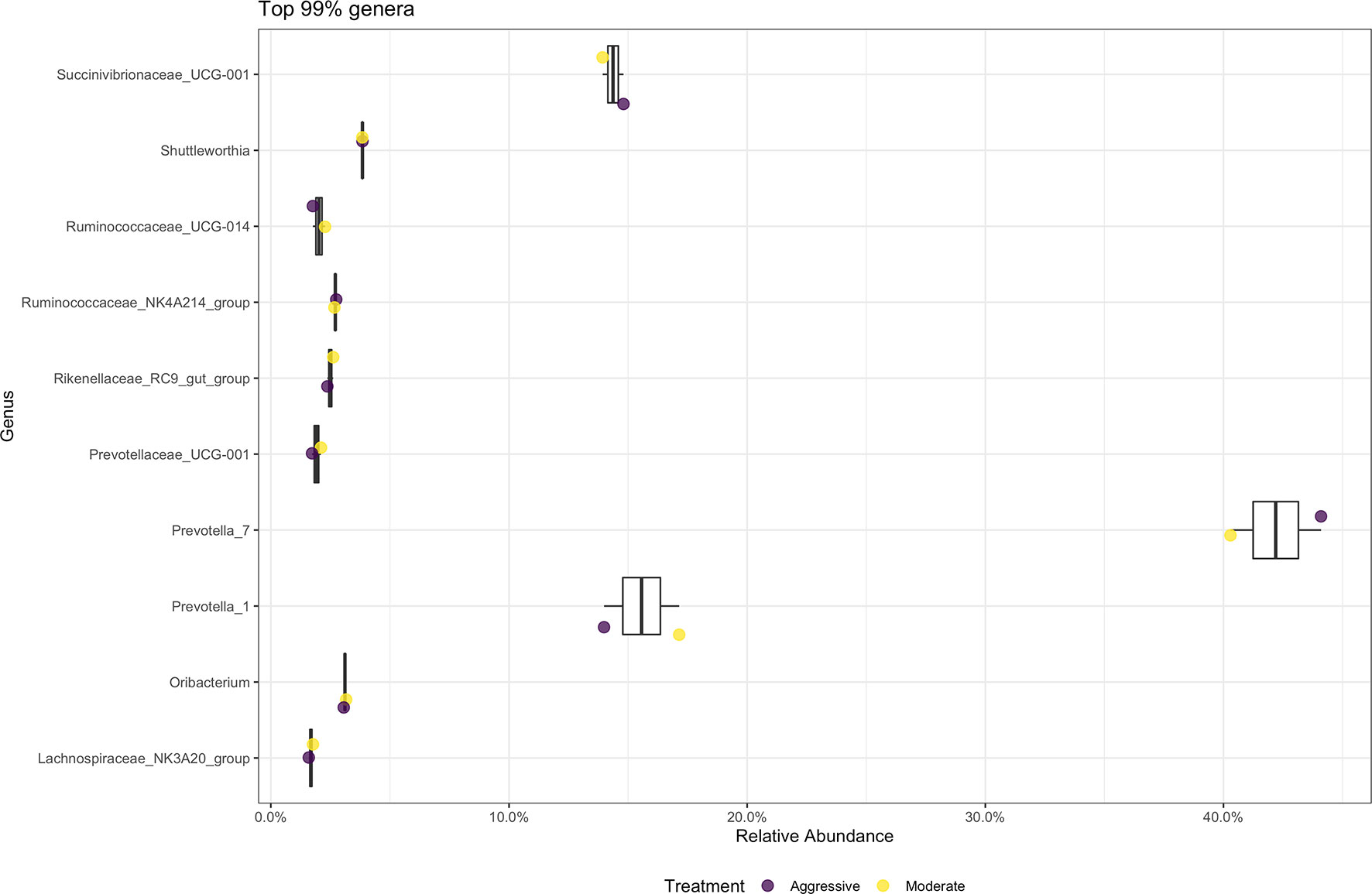
Figure 4 Average relative abundance of the top 99% genus-level bacterial communities classified in ruminal fluid between aggressive or moderate implant strategies. Mean relative abundance of bacterial communities associated with the aggressive implant strategy are represented in purple and moderate implant strategy in yellow. Relative abundance was calculated by reads of taxon/total reads in a sample to compare between treatments.
Ruminal Archaeal Communities
For archaeal communities, the SILVA v138 database identified 858 taxa within 6 taxonomic ranks. Chimeras were identified to represent 23.19% of sequences after filtering. Alpha-diversity metrics of observed archaeal abundances (Observed), expected species abundance (Chao1), and richness and evenness estimates (Shannon) were calculated and not different between treatments (P > 0.05; Figure 5). Beta diversity, measured through a Bray-Curtis PCoA, visually demonstrated similarities between treatment groups and the PERMANOVA performed showed no differences between the moderate and aggressive implant strategies (P > 0.05; Figure 6).
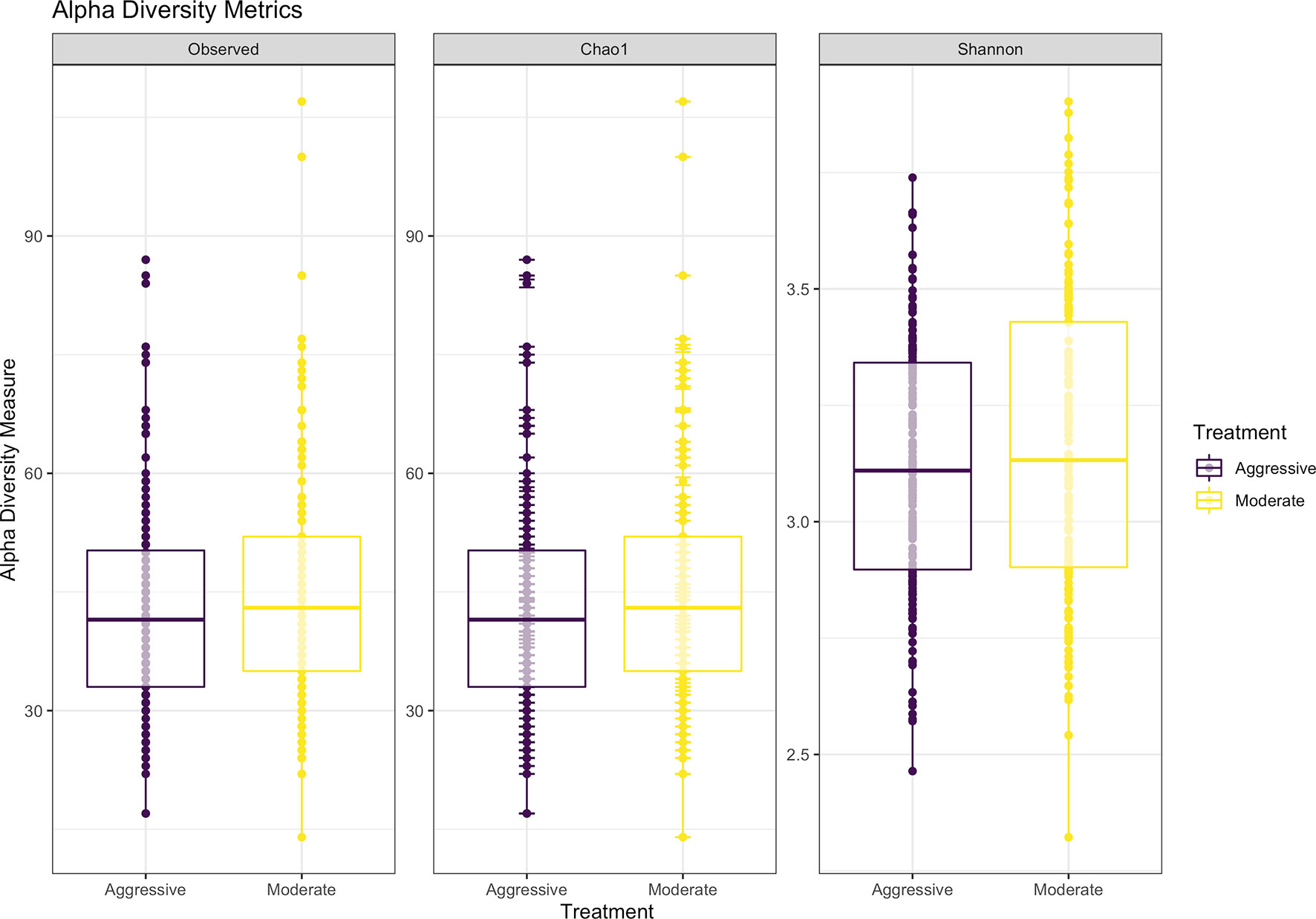
Figure 5 Alpha-diversity metrics for archaeal communities with observed abundances of communities, expected abundances (Chao1), and richness and diversity measured with Shannon diversity index. Aggressive treatments are represented in purple and moderate treatments are represented in yellow. There were no significant differences between alpha-diversity metrics between moderate and aggressive implant strategies.
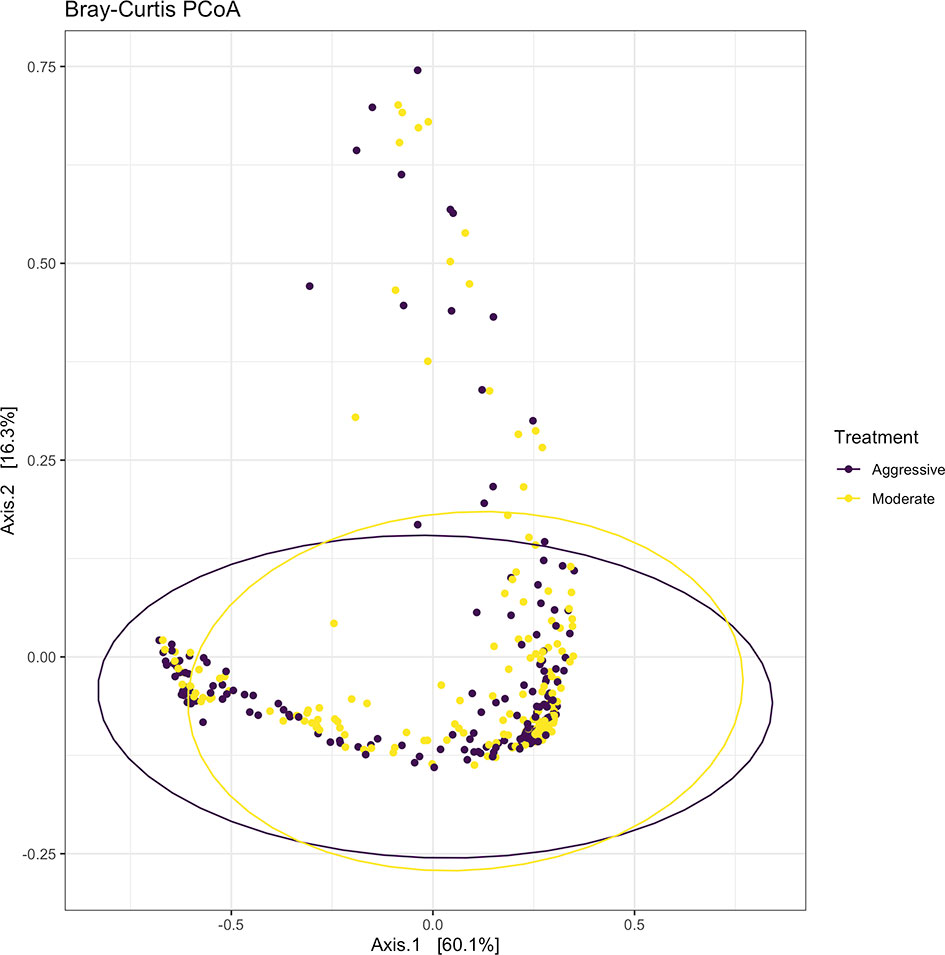
Figure 6 Beta-diversity measurements for archaeal communities using Bray-Curtis distances to create a PCoA. Aggressive treatments are represented in purple and moderate treatments are represented in yellow. Ellipses represent 95% confidence intervals around means for aggressive and moderate implant strategies. Significant overlap between communities reveals no differences between moderate or aggressive treatments.
There were no differences between the moderate and aggressive implant strategies at any taxonomic level after performing analyses in DESeq2. The majority of archaea at the phylum level were Eurarchaeota, making up 99% of samples. At the genus level (Figure 7), the majority of archaeal communities identified were Methanobrevibacter (91.75%). Minor archaeal communities consisted of the genera Methanosphaera (3.81%) and Candidatus methanomethylophilus (2.82%).
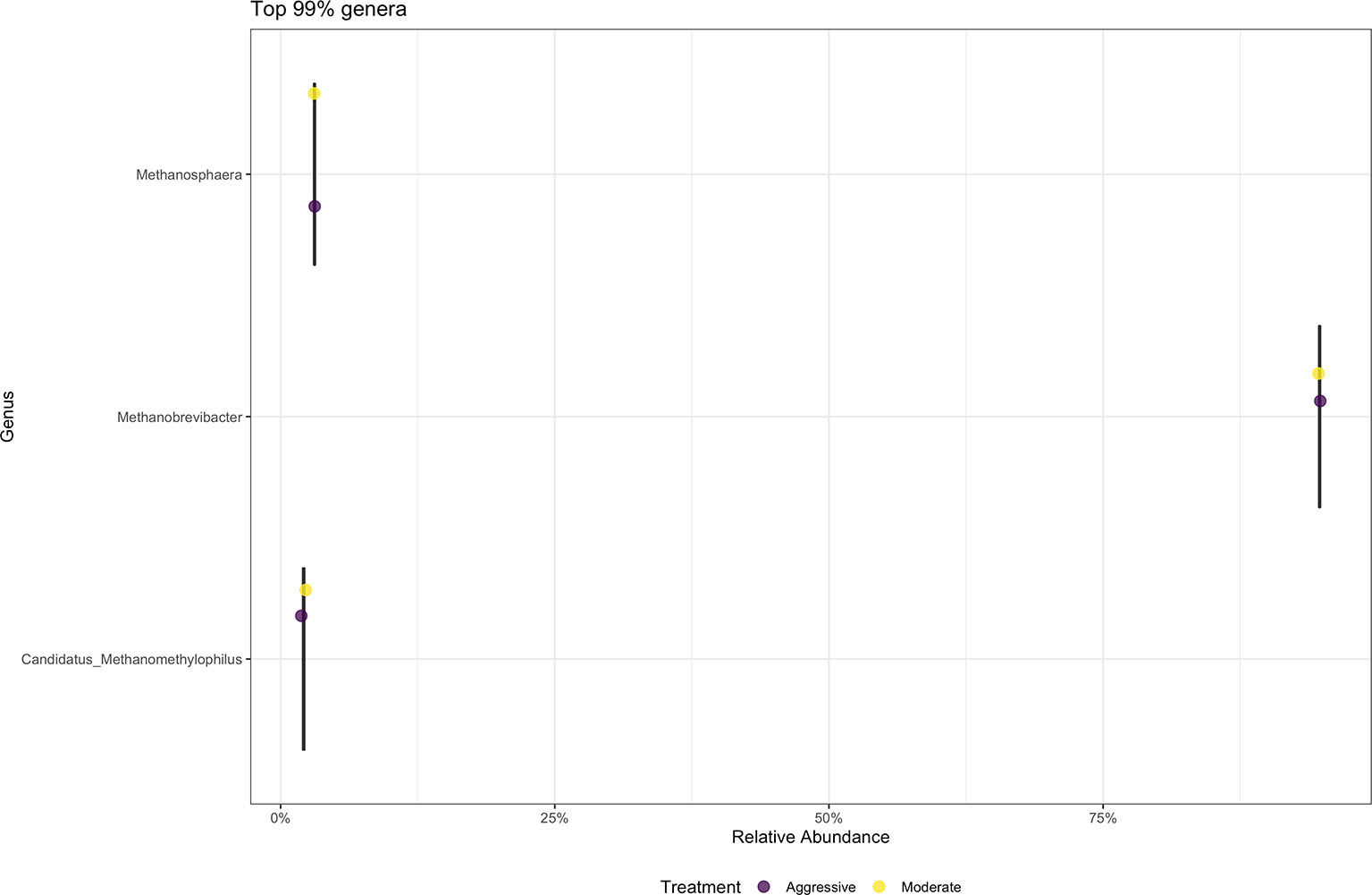
Figure 7 Average relative abundance of the top 99% genus-level archaeal communities classified in ruminal fluid between aggressive and moderate implant strategies. Mean relative abundance of archaeal communities associated with the aggressive implant strategy are represented in purple and moderate implant strategy in yellow.
Ruminal Protozoal Communities
After filtering and processing in R for protozoal communities, the SILVA v132 database identified 220 taxa within 6 taxonomic ranks. Chimeras were identified to represent 4.9% of sequences after filtering. Out of the 336 samples, 128 protozoal fastq files did not meet filtering standards and were removed from data, resulting in 208 final samples for the protozoal communities (moderate = 109, aggressive = 99). After taxonomic assignment, 220 taxa were identified. Alpha diversity was measured for observed abundance, estimated abundance (Chao1), and species evenness and richness (Shannon) (Figure 8) and no differences were found between treatments (P > 0.05). Beta diversity was measured with a Bray-Curtis PCoA (Figure 9) and after testing with a PERMANOVA, was not significant between the moderate or aggressive strategies (P > 0.05).
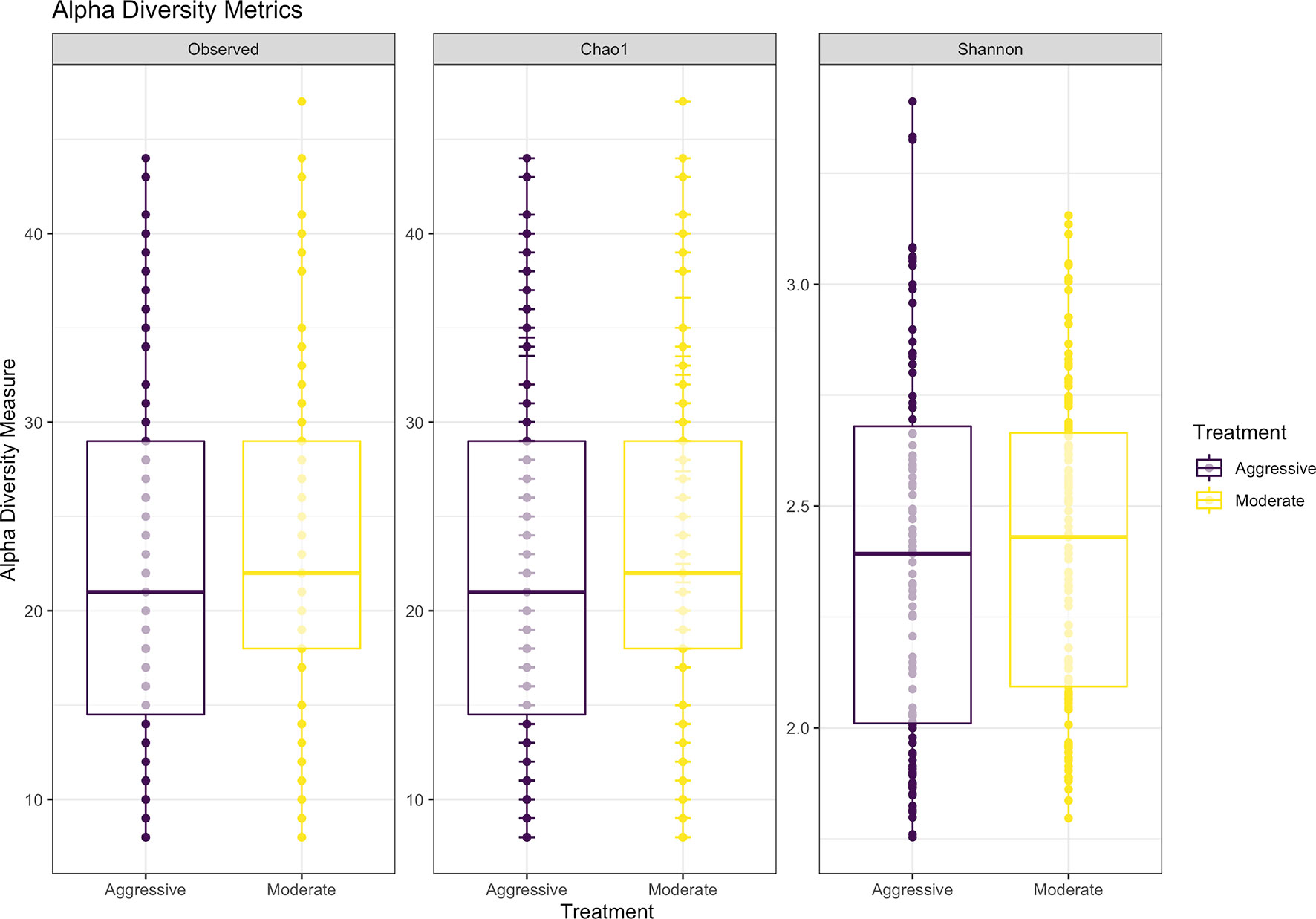
Figure 8 Alpha-diversity metrics for protozoal communities with observed abundances of communities, expected abundances (Chao1), and richness and diversity measured with Shannon diversity index. There were no significant differences between alpha-diversity metrics between moderate and aggressive implant strategies. Aggressive treatments are represented in purple and moderate treatments are represented in yellow.
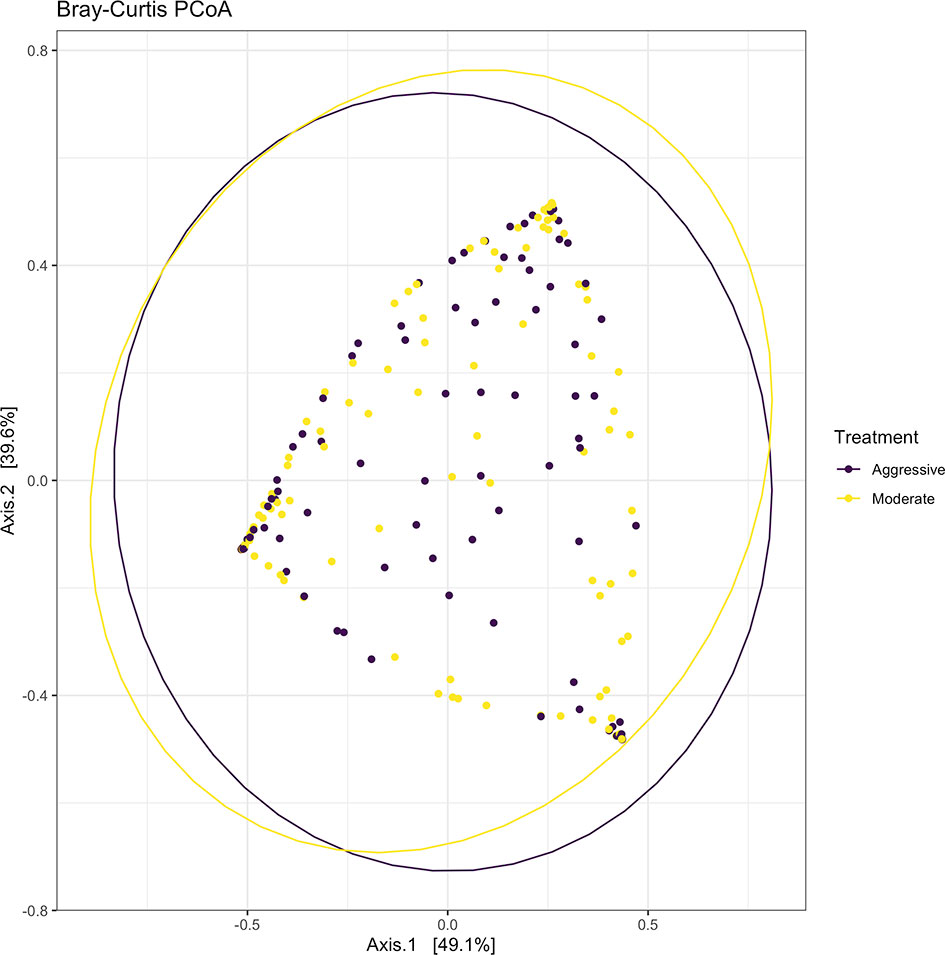
Figure 9 Beta-diversity measurements for protozoal communities using Bray-Curtis distances to create a PCoA. Aggressive treatments are represented in purple and moderate treatments are represented in yellow. Ellipses represent 95% confidence intervals around means for aggressive and moderate implant strategies. Significant overlap between communities reveals no differences between moderate or aggressive treatments.
At the phylum level, SAR was the most abundant category, making up 99% of samples. Top 10 phyla relative abundance are shown in Figure 10. At the genus level (Figure 10), the majority of protozoal communities identified were Entodinium (58.86%). Minor protozoal communities consisted of Ophryoscolex (6.8%), Isotricha (3.2%) and Polyplastron (1.6%). There was a difference between the relative abundance of Isotricha between the moderate (3.0% ± 0.03) and aggressive (3.3% ± 0.03) implant strategies (P < 0.05).
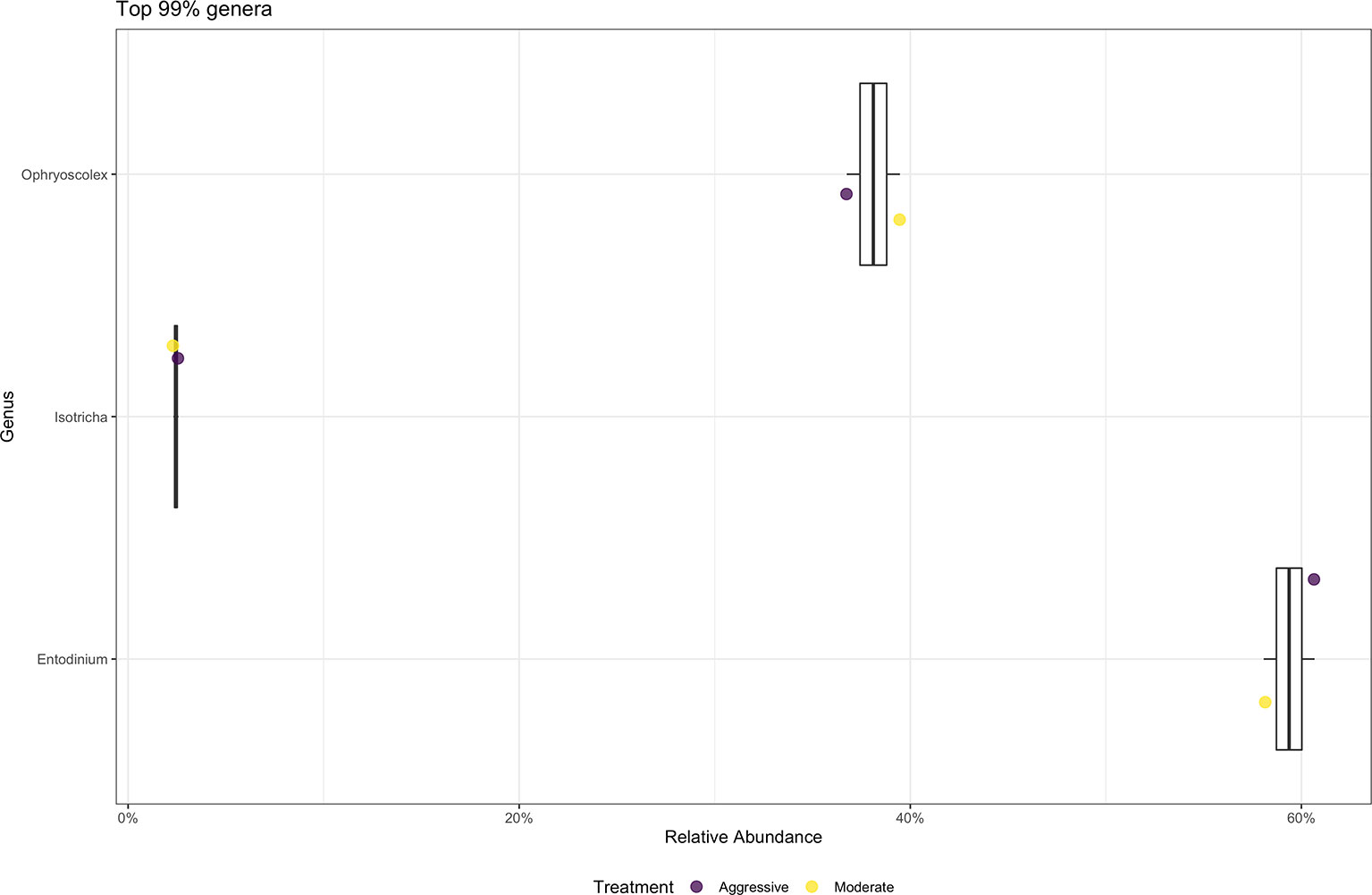
Figure 10 Average relative abundance of the top 99% genus-level protozoal communities classified in ruminal fluid between aggressive and moderate implant strategies. Mean relative abundance of protozoal communities associated with the aggressive implant strategy are represented in purple and moderate implant strategy in yellow.
Rumen Metabolite Data
After untargeted metabolomics were performed on rumen samples, 115 unique metabolites were identified. Partial least-squares discriminant analysis of the 115 metabolites demonstrated similarity in the metabolic profile of the moderate and aggressive implant strategies (Figure 11). Between the moderate and aggressive implant strategies, two metabolites were found to be different (Table 3). In the moderate treatment group, N-acetyllysine (P ≤ 0.03) and N-acetylornithine (P ≤ 0.04) were greater than the aggressive treatment. The covariate of initial body weight was not significant in any model (P > 0.05). From analysis in MetaboAnalyst 4.0, these metabolites are most similarly correlated to arginine synthesis in metabolic pathways (P < 0.05).
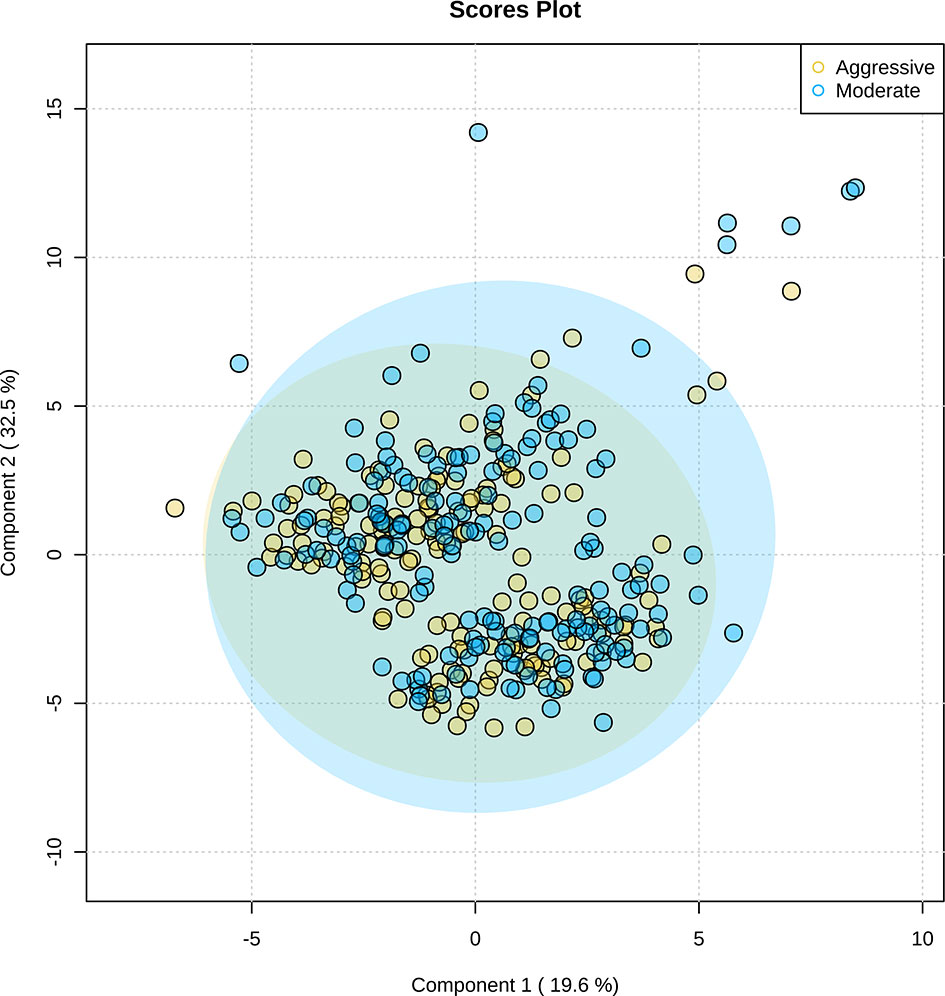
Figure 11 Partial least-squares discriminant analysis of 115 rumen fluid metabolites identified through untargeted metabolomics. The aggressive implant strategy is represented in yellow and the moderate implant strategy is represented in blue. Significant overlap of metabolites present in moderate and aggressive implant strategies illustrates similarity of rumen fluid metabolic profiles. Shaded regions represent a 95% confidence interval around the metabolites per treatment strategy.
Discussion
Improving the efficiency of weight gain and growth performance in beef cattle is one way to contribute to a reliable food source to feed a growing population. Growth-promoting implant strategies remain one of the most effective ways to improve gain in beef cattle, increasing ADG up to 30% and feed efficiency by 15% (Preston, 1999). The use of aggressive implant strategies is thought to improve ADG beyond the capabilities of moderate implant strategies due to increased concentrations of androgenic and estrogenic compounds and altered ratio of the hormones.
The use of growth-promoting hormones in management strategies may be influencing other factors that impact weight gain and feed efficiency in beef cattle, such as the rumen microbiome. The rumen microbiome has been previously identified to impact feed efficiency in beef cattle through the conversion of nutrients to energy for the host (Mizrahi, 2011; Myer et al., 2015). Further, fermentative products of the rumen microbial communities, particularly volatile fatty acids, supply energy to the host and play a role in metabolic pathways (Anantasook et al., 2013). While one alpha diversity measurement for bacterial communities did differ, the difference is likely not biologically relevant as no microbial communities differed between treatments. This is further supported by little differences seen in the other diversity metrics calculated when assessing the archaeal and protozoal communities between treatments.
The few differences in the rumen microbiome and metabolome may be explained by all steers being fed the same diet. While these implant strategies are identified as moderate and aggressive, there was overall little biological differences in ADG, likely because the cattle were tubed for rumen fluid at equal days on feed, irrespective of 12th rib fat thickness. It is likely the influence of the composite breed type of the cattle would allow them to reach maturity at different times and were on varying places of the growth curve at the time of tubing for rumen fluid. A previous study conducted demonstrated a similar core rumen microbiome composition across animals in different climates and farming practices, with the major driving factor being diet (Henderson et al., 2015). Therefore, if the hormones present in the implant strategy are not influencing the rumen microbiome, animals receiving the same diet within the same environment would have rumen microbial profiles that are very similar.
Previous research has not identified estrogenic and androgenic receptors on the rumen epithelium of cattle with a fully developed rumen, potentially signifying the lack of a mechanism for these hormones to enter the rumen environment. While estrogen-like receptor-α, highly similar in sequence similarity to estrogen receptors (Giguère et al., 1988), has been researched on the rumen epithelium in calves 14-42 days of age (Connor et al., 2014), these studies have not identified other binding sites for estrogenic and androgenic compounds found in growth-promoting implants. Further, the estrogen-related receptors have not been identified to bind to estrogens or related steroid hormones, but function to increase transcription of genes in energy metabolism through an unknown endogenous ligand (Schreiber et al., 2003). Future studies should assess receptors present on rumen tissue that interact with growth-promoting hormones and the rumen environment.
The presence of estrogenic receptors on gastrointestinal tissues has been identified in human studies, prompting further research into the gut-brain axis involving the activity of estrogens. Decreases in the diversity in the gut microbiome in humans have been linked to a reduction of β-glucuronidase, which functions to deconjugate estrogen and phytoestrogens into their active and circulating forms (Baker et al., 2017). If these estrogenic receptors can be identified on rumen tissue, there is potential for additional circulating estrogen to spur additional production of GH and IGF-1 to increase muscle tissue growth through binding sites present on muscle tissue.
In bovines, androgenic compounds have been demonstrated to have direct and indirect effects on muscle and growth through binding directly to receptors in muscle tissues (Sauerwein and Meyer, 1989), while indirectly spurring production of GH and IGF-I (Grigsby and Trenkle, 1986). Bovine skeletal muscle directly interacts with free androgens that are introduced via implanting strategies through androgen receptors, which in addition to estrogen, can cause additive growth in muscle tissue (Snochowski et al., 1981). While GH and IGF-I play an important role in muscle development, they have not been associated with rumen functionality. Research is still needed to identify potential receptors in the rumen in order to draw conclusions.
While the protozoal genus Isotricha was found in greater presence in the moderate implant group, no other microbial community was found significantly different between treatments. Interestingly, past studies have examined the defaunation of protozoal communities to understand their impact on the rumen microbiome (Newbold et al., 2015). The protozoal communities present in the rumen often outweigh their positive interaction with digestion of fiber and starch particles (Mendoza et al., 1993; Takenaka et al., 2004) by decreasing efficient N utilization in the rumen, thus decreasing energy provided to the host. Further, ruminal protozoa function to phagocytize some bacterial communities, which decreases availability of microbial protein (Ushida et al., 1990). When investigating impacts of saponins on methane production and the composition of the rumen microbiome, Isotricha were found in greater abundance in animals with higher CH4 production (Tan et al., 2020). Generally, a higher methane production is associated with decreased feed efficiency in cattle (Hegarty et al., 2007), which may be indicative of rumen microbial composition (McGovern et al., 2020). This association may indicate that the steers within the moderate implant strategy are not using energy as efficiently as those in the aggressive implant strategy, which may play a role in altering gain. Despite the interesting functionality behind host energy supply, protein metabolism, and methane production from protozoal communities, the Isotricha populations cannot be directly associated with differences in the metabolome or gain within this study and influences can only be speculated.
Out of the 115 metabolites identified in rumen fluid, only two were significantly different between the moderate and aggressive implant strategies. While there were significant differences in metabolites, it is important to note that there were no significant differences between bacterial and archaeal communities in the rumen microbiome. Thus, the differences in metabolites likely does not stem from rumen bacterial or archaeal composition, although the metabolic activity of these communities may have been altered. With the stability of the bacterial and archaeal communities and only one protozoal genus differing between the moderate and aggressive implant strategy, significant changes in metabolite concentrations could be due to host metabolic activity rather than any microbial activity or fermentation. N-acetyllysine is an acetyl-derivative of lysine, an essential amino acid that is critical for growth in beef cattle. Interestingly, lysine functions as one of the most limiting amino acids in growing cattle (Richardson and Hatfield, 1978), as it contributes greatly to maximizing lean tissue deposition (Friesen et al., 1994). The acetylation of lysine may explain why the moderate implant treatment resulted in lower ADG, as less lysine may be contributing towards muscle growth. However, this is likely not the sole explanation for the decrease in ADG in the moderate implant treatment group, as amounts of androgenic and estrogenic hormones directly influence skeletal muscle by stimulating GH and IGF-I to bind to muscle, causing proliferation of muscle satellite cells and resulting in additional growth (Froesch and Zapf, 1985). Based on this, the use of growth-promoting implants appears unlikely to influence the rumen metabolome, rather directly acting on muscle tissue to impact ADG.
While previous studies have analyzed rumen metabolites (Artegoitia et al., 2017; Clemmons et al., 2020), the pathways and activity of many of these metabolites play multiple functions throughout the rumen and host metabolism, and thus can be difficult to identify true functionality. In addition, other studies have concluded that the origin of the metabolites can be difficult to trace, with half of the rumen metabolites coming from bovine origin and the remaining from microbial origin (Saleem et al., 2013). Furthermore, previous studies have also found a wide variation among the abundance of metabolites, leading to belief that more research is required to be able to associate rumen metabolites with diet and feed efficiency (Saleem et al., 2013). While the presence of N-acetyllysine may be due to shifts in protozoal communities, other metabolites such as N-acetylornithine could be due to differential absorption across the rumen epithelium. The lack of differences in ruminal bacterial and archaeal communities may demonstrate that the metabolites found in this study are of host origin. Notably, there are few studies involving N-acetylornithine and its metabolic effect on beef cattle, making associations more difficult. Previous studies have suggested the relationship of N-acetylornithine with enzymatic activity for arginine and lysine biosynthesis (Ledwidge and Blanchard, 1999), may be contributing towards protein use in cattle.
Previous studies analyzing how shifts in protozoal communities influence rumen metabolites have commonly identified purine derivatives due to the effects of purine derivatives on microbial protein synthesis (Chen and Gomes, 1992). Interestingly, the metabolites identified in this study that could be related to significant shifts in protozoal communities were not identified in other studies. Purine derivatives, such as allantoic acid and hypoxanthine, were identified (Chen and Gomes, 1992); however, they did not significantly differ between treatments. In addition, metabolites that have been previously identified to be influenced by protozoa in lambs such as aminoadipate, cholate, and pantothenate did not significantly differ in this study (Morgavi et al., 2015). Aminoadipic acid has been identified to be influenced by protozoal communities, and plays an important role in lysine metabolism. While aminoadipic acid was not different between treatments, N-acetyllysine also affects presence of lysine in biological fluids and was found different between treatments.
Understanding the relationship of microbial communities with host phenotypes for feed efficiency and growth may allow for better identification of efficient beef cattle. The topic of growth-promoting implants has been well-reviewed, identifying that implants greatly improve ADG in beef cattle and making implants one of the most effective management strategies in increasing growth (Duckett et al., 1996; Preston, 1999). Additionally, studies have identified the importance of the rumen microbiome in nutrient conversion and overall feed efficiency (Myer et al., 2015; Auffret et al., 2020). With rumen microbial communities producing fermentative products and metabolites that may be linked to phenotypic traits, metabolites should be further characterized in the rumen.
Conclusion
There were no differences in the ruminal bacterial and archaeal community abundance between the moderate and aggressive implant strategies. Between a moderate and aggressive implant strategy, only one protozoal genus differed, potentially indicating that the growth-promoting hormones included in implants have minimal influence on the rumen. While no differences were identified between bacterial or archaeal communities, this study corroborated previously determined differences in ADG due to implant strategy, while finding one ruminal protozoal community that differed in steers given a moderate or aggressive implant strategy. Future directions with this study could involve multiple brands of implant strategies as well as including non-implanted cattle to determine more ruminal and metabolic differences.
Data Availability Statement
The datasets generated and analyzed for this study can be found in the Sequence Read Archive (PRJNA811160) [https://www.ncbi.nlm.nih.gov/bioproject/PRJNA811160/].
Ethics Statement
The animal study was reviewed and approved by the U.S. Meat Animal Research Center (MARC) Animal Care and Use Committee, USDA-ARS-U.S. Meat Animal Research Center, Clay Center, NE.
Author Contributions
JW, KH, AL-P, HF, LK, and PM contributed to conception and design of the study. MH performed all sample and statistical analyses. MH conducted data analyses and CC conducted metabolomics analyses. MH, PM, KM, and LS aided in the interpretation of results. MH wrote the first draft of the manuscript. CC, SC, AL-P, LS wrote sections of the manuscript. All authors contributed to manuscript revision, read, and approved the submitted version.
Funding
This study was supported by the USDA-NIFA Hatch/Multistate Project W4177 - TEN00524 - Enhancing the Competitiveness and Value of U.S. Beef; Accession Number: 1016984.
Author Disclaimer
Mention of a trade name, proprietary product, or specific equipment does not constitute a guarantee or warranty by the USDA and does not imply approval to the exclusion of other products that may be suitable. USDA is an equal opportunity provider and employer.
Conflict of Interest
The authors declare that the research was conducted in the absence of any commercial or financial relationships that could be construed as a potential conflict of interest.
Publisher’s Note
All claims expressed in this article are solely those of the authors and do not necessarily represent those of their affiliated organizations, or those of the publisher, the editors and the reviewers. Any product that may be evaluated in this article, or claim that may be made by its manufacturer, is not guaranteed or endorsed by the publisher.
Acknowledgments
Thank you to Emily Melchior and Brooke Clemmons for laboratory assistance.
Supplementary Material
The Supplementary Material for this article can be found online at: https://www.frontiersin.org/articles/10.3389/fanim.2022.889817/full#supplementary-material
Supplementary File 1 | Genus-level abundances for ruminal bacterial communities.
Supplementary File 2 | Genus-level abundances for ruminal archaeal communities.
Supplementary File 3 | Genus-level abundances for ruminal protozoal communities.
Supplementary File 4 | Raw data for rumen metabolomics.
References
Abbas W., Keel B. N., Kachman S. D., Fernando S. C., Wells J. E., Hales K. E., et al. (2020). Rumen Epithelial Transcriptome and Microbiome Profiles of Rumen Epithelium and Contents of Beef Cattle With and Without Liver Abscesses. J. Anim. Sci. 98 (12), 1–13. doi: 10.1093/jas/skaa359
Anantasook N., Wanapat M., Cherdthong A., Gunun P. (2013). Changes of Microbial Population in the Rumen of Dairy Steers as Influenced by Plant Containing Tannins and Saponins and Roughage to Concentrate Ratio. Asian-Australasian J. Anim. Sci. 26 (11), 1583–1591. doi: 10.5713/ajas.2013.13182
Anders S., Huber W. (2010). Differential Expression Analysis for Sequence Count Data. Nat. Precedings, 10. doi: 10.1038/npre.2010.4282.2
Arjmandi B. H., Salih M. A., Herbert D. C., Sims S. H., Kalu D. N. (1993). Evidence for Estrogen Receptor-Linked Calcium Transport in the Intestine. Bone Miner. 21 (1), 63–74. doi: 10.1016/S0169-6009(08)80121-3
Artegoitia V. M., Foote A. P., Lewis R. M., Freetly H. C. (2017). Rumen Fluid Metabolomics Analysis Associated With Feed Efficiency on Crossbred Steers. Sci. Rep. 7 (1), 2864. doi: 10.1038/s41598-017-02856-0
Auffret M. D., Stewart R. D., Dewhurst R. J., Duthie C.-A., Watson M., Roehe R. (2020). Identification of Microbial Genetic Capacities and Potential Mechanisms Within the Rumen Microbiome Explaining Differences in Beef Cattle Feed Efficiency. Front. Microbiol. 11. doi: 10.3389/fmicb.2020.01229
Baker J. M., Al-Nakkash L., Herbst-Kralovetz M. M. (2017). Estrogen–gut Microbiome Axis: Physiological and Clinical Implications. Maturitas 103, 45–53. doi: 10.1016/j.maturitas.2017.06.025
Callahan B. J., McMurdie P. J., Holmes S. P. (2017). Exact Sequence Variants Should Replace Operational Taxonomic Units in Marker-Gene Data Analysis. ISME J. 11 (12), 2639–2643. doi: 10.1038/ismej.2017.119
Callahan B. J., McMurdie P. J., Rosen M. J., Han A. W., Johnson A. J. A., Holmes S. P. (2016a). DADA2: High-Resolution Sample Inference From Illumina Amplicon Data. Nat. Methods 13 (7), 581–583. doi: 10.1038/nmeth.3869
Callahan B. J., Sankaran K., Fukuyama J. A., McMurdie P. J., Holmes S. P. (2016b). Bioconductor Workflow for Microbiome Data Analysis: From Raw Reads to Community Analyses. F1000Research 5, 1492–1492. doi: 10.12688/f1000research.8986.2
Chen X. B., Gomes M. (1992). Estimation of Microbial Protein Supply to Sheep and Cattle Based on Urinary Excretion of Purine Derivatives: An Overview of the Technical Details. Rowett Research Institure. 1–21.
Chong J., Soufan O., Li C., Caraus I., Li S., Bourque G., et al. (2018). MetaboAnalyst 4.0: Towards More Transparent and Integrative Metabolomics Analysis. Nucleic Acids Res. 46 (W1), W486–W494. doi: 10.1093/nar/gky310
Clasquin M. F., Melamud E., Rabinowitz J. D. (2012). LC-MS Data Processing With MAVEN: A Metabolomic Analysis and Visualization Engine. Curr. Protoc. Bioinf. 37 (1), 14.11.11–14.11.23. doi: 10.1002/0471250953.bi1411s37
Clemmons B. A., Powers J. B., Campagna S. R., Seay T. B., Embree M. M., Myer P. R. (2020). Rumen Fluid Metabolomics of Beef Steers Differing in Feed Efficiency. Metabolomics 16 (2), 23. doi: 10.1007/s11306-020-1643-x
Connor E. E., Baldwin R. L., Walker M. P., Ellis S. E., Li C., Kahl S., et al. (2014). Transcriptional Regulators Transforming Growth Factor-β1 and Estrogen-Related Receptor-α Identified as Putative Mediators of Calf Rumen Epithelial Tissue Development and Function During Weaning1. J. Dairy Sci. 97 (7), 4193–4207. doi: 10.3168/jds.2013-7471
Domingo-Almenara X., Montenegro-Burke J. R., Guijas C., Majumder E. L. W., Benton H. P., Siuzdak G. (2019). Autonomous METLIN-Guided In-Source Fragment Annotation for Untargeted Metabolomics. Anal. Chem. 91 (5), 3246–3253. doi: 10.1021/acs.analchem.8b03126
Duckett S. K., Wagner D. G., Owens F. N., Dolezal H. G., Gill D. R. (1996). Effects of Estrogenic and Androgenic Implants on Performance, Carcass Traits, and Meat Tenderness in Feedlot Steers: A Review11Approved for Publication by the Director, Oklahoma Agric. Exp. Sta. Prof. Anim. Sci. 12 (4), 205–214. doi: 10.15232/S1080-7446(15)32526-2
Edgar R. C., Flyvbjerg H. (2015). Error Filtering, Pair Assembly and Error Correction for Next-Generation Sequencing Reads. Bioinformatics 31 (21), 3476–3482. doi: 10.1093/bioinformatics/btv401
Elgendy R., Giantin M., Montesissa C., Dacasto M. (2016). The Transcriptome of Muscle and Liver is Responding Differently to a Combined Trenbolone Acetate and Estradiol Implant in Cattle. Steroids 106, 1–8. doi: 10.1016/j.steroids.2015.11.002
Foroutan A., Fitzsimmons C., Mandal R., Berjanskii M. V., Wishart D. S. (2020). Serum Metabolite Biomarkers for Predicting Residual Feed Intake (RFI) of Young Angus Bulls. Metabolites 10 (12), 491. doi: 10.3390/metabo10120491
Friesen K. G., Nelssen J. L., Unruh J. A., Goodband R. D., Tokach M. D. (1994). Effects of the Interrelationship Between Genotype, Sex, and Dietary Lysine on Growth Performance and Carcass Composition in Finishing Pigs Fed to Either 104 or 127 Kilograms2. J. Anim. Sci. 72 (4), 946–954. doi: 10.2527/1994.724946x
Froesch E. R., Zapf J. (1985). Insulin-Like Growth Factors and Insulin: Comparative Aspects. Diabetologia 28 (8), 485–493. doi: 10.1007/BF00281982
Giguère V., Yang N., Segui P., Evans R. M. J. N. (1988). Identification of a New Class of Steroid Hormone Receptors. Nature 331, 6151, 91–94. doi: 10.1038/331091a0
Glassman S. I., Martiny J. B. H. (2018). Broadscale Ecological Patterns Are Robust to Use of Exact Sequence Variants Versus Operational Taxonomic Units. mSphere 3, 4, e00148–e00118. doi: 10.1128/mSphere.00148-18
Grigsby M. E., Trenkle A. (1986). Plasma Growth Hormone, Insulin, Glucocorticoids and Thyroid Hormones in Large, Medium and Small Breeds of Steers With and Without an Estradiol Implant. Domest. Anim. Endocrinol. 3 (4), 261–267. doi: 10.1016/0739-7240(86)90024-X
Hayden J. M., Bergen W. G., Merkel R. A. (1992). Skeletal Muscle Protein Metabolism and Serum Growth Hormone, Insulin, and Cortisol Concentrations in Growing Steers Implanted With Estradiol-17β, Trenbolone Acetate, or Estradiol-17β Plus Trenbolone Acetate2. J. Anim. Sci. 70 (7), 2109–2119. doi: 10.2527/1992.7072109
Hegarty R. S., Goopy J. P., Herd R. M., McCorkell B. (2007). Cattle Selected for Lower Residual Feed Intake Have Reduced Daily Methane Production1, 2. J. Anim. Sci. 85 (6), 1479–1486. doi: 10.2527/jas.2006-236
Henderson G., Cox F., Ganesh S., Jonker A., Young W., Abecia L., et al. (2015). Rumen Microbial Community Composition Varies With Diet and Host, But a Core Microbiome is Found Across a Wide Geographical Range. Sci. Rep. 5 (1), 14567. doi: 10.1038/srep14567
Hunt D. W., Henricks D. M., Skelley G. C., Grimes L. W. (1991). Use of Trenbolone Acetate and Estradiol in Intact and Castrate Male Cattle: Effects on Growth, Serum Hormones, and Carcass Characteristics2. J. Anim. Sci. 69 (6), 2452–2462. doi: 10.2527/1991.6962452x
Isabelle M., Villemur R., Juteau P., Lépine F. (2011). Isolation of Estrogen-Degrading Bacteria From an Activated Sludge Bioreactor Treating Swine Waste, Including a Strain That Converts Estrone to β-Estradiol. Can. J. Microbiol. 57 (7), 559–568. doi: 10.1139/w11-051%M21770814
Ishaq S. L., Wright A.-D. G. (2014). Design and Validation of Four New Primers for Next-Generation Sequencing To Target the 18S rRNA Genes of Gastrointestinal Ciliate Protozoa. Appl. Environ. Microbiol. 80, 17, 5515–5521. doi: 10.1128/AEM.01644-14
Johnson B. J., Anderson P. T., Meiske J. C., Dayton W. R. (1996a). Effect of a Combined Trenbolone Acetate and Estradiol Implant on Feedlot Performance, Carcass Characteristics, and Carcass Composition of Feedlot Steers. J. Anim. Sci. 74 (2), 363–371. doi: 10.2527/1996.742363x
Johnson B. J., Hathaway M. R., Anderson P. T., Meiske J. C., Dayton W. R. (1996b). Stimulation of Circulating Insulin-Like Growth Factor I (IGF-I) and Insulin-Like Growth Factor Binding Proteins (IGFBP) Due to Administration of a Combined Trenbolone Acetate and Estradiol Implant in Feedlot Cattle. J. Anim. Sci. 74 (2), 372–379. doi: 10.2527/1996.742372x%JJournalofAnimalScience
Kanehisa M., Goto S., Sato Y., Kawashima M., Furumichi M., Tanabe M. (2013). Data, Information, Knowledge and Principle: Back to Metabolism in KEGG. Nucleic Acids Res. 42 (D1), D199–D205. doi: 10.1093/nar/gkt1076%JNucleicAcidsResearch
Kozich J. J., Westcott S. L., Baxter N. T., Highlander S. K., Schloss P. D. (2013). Development of a Dual-Index Sequencing Strategy and Curation Pipeline for Analyzing Amplicon Sequence Data on the MiSeq Illumina Sequencing Platform. Appl. Environ. Microbiol. 79, 17, 5112–5120. doi: 10.1128/AEM.01043-13
Lane D. J., Pace B., Olsen G. J., Stahl D. A., Sogin M. L., Pace N. R. (1985). Rapid Determination of 16S Ribosomal RNA Sequences for Phylogenetic Analyses. Proc. Natl. Acad. Sci. U. S. A. 82 (20), 6955–6959. doi: 10.1073/pnas.82.20.6955
Ledwidge R., Blanchard J. S. (1999). The Dual Biosynthetic Capability of N-Acetylornithine Aminotransferase in Arginine and Lysine Biosynthesis. Biochemistry 38 (10), 3019–3024. doi: 10.1021/bi982574a
Lee C. Y., Henricks D. M., Skelley G. C., Grimes L. W. (1990). Growth and Hormonal Response of Intact and Castrate Male Cattle to Trenbolone Acetate and Estradiol2. J. Anim. Sci. 68 (9), 2682–2689. doi: 10.2527/1990.6892682x
Li F., Guan L. L., McBain A. J. (2017). Metatranscriptomic Profiling Reveals Linkages Between the Active Rumen Microbiome and Feed Efficiency in Beef Cattle. Appl. Environ. Microbiol. 83 (9), e00061–e00017. doi: 10.1128/AEM.00061-17
Love M. I., Huber W., Anders S. (2014). Moderated Estimation of Fold Change and Dispersion for RNA-Seq Data With Deseq2. Genome Biol. 15 (12), 550. doi: 10.1186/s13059-014-0550-8
Lu W., Clasquin M. F., Melamud E., Amador-Noguez D., Caudy A. A., Rabinowitz J. D. (2010). Metabolomic Analysis via Reversed-Phase Ion-Pairing Liquid Chromatography Coupled to a Stand Alone Orbitrap Mass Spectrometer. Anal. Chem. 82 (8), 3212–3221. doi: 10.1021/ac902837x
McGovern E., McGee M., Byrne C. J., Kenny D. A., Kelly A. K., Waters S. M. (2020). Investigation Into the Effect of Divergent Feed Efficiency Phenotype on the Bovine Rumen Microbiota Across Diet and Breed. Sci. Rep. 10 (1), 15317. doi: 10.1038/s41598-020-71458-0
McMurdie P. J., Holmes S. (2013). Phyloseq: An R Package for Reproducible Interactive Analysis and Graphics of Microbiome Census Data. PloS One 8 (4), e61217. doi: 10.1371/journal.pone.0061217
Mendoza G. D., Britton R. A., Stock R. A. (1993). Influence of Ruminal Protozoa on Site and Extent of Starch Digestion and Ruminal Fermentation. J. Anim. Sci. 71 (6), 1572–1578. doi: 10.2527/1993.7161572x
Mizrahi I. (2011). “The Role of the Rumen Microbiota in Determining the Feed Efficiency of Dairy Cows”, in Beneficial Microorganisms in Multicellular Life Forms. Eds. Rosenberg E., Gophna U. (Berlin, Heidelberg: Springer Berlin Heidelberg), 203–210. doi: 10.1007/978-3-642-21680-0_14
Morgavi D. P., Rathahao-Paris E., Popova M., Boccard J., Nielsen K. F., Boudra H. (2015). Rumen Microbial Communities Influence Metabolic Phenotypes in Lambs. Front. Microbiol. 6 6(1060). doi: 10.3389/fmicb.2015.01060
Myer P. R., Smith T. P. L., Wells J. E., Kuehn L. A., Freetly H. C. (2015). Rumen Microbiome From Steers Differing in Feed Efficiency. PloS One 10 (6), e0129174–e0129174. doi: 10.1371/journal.pone.0129174
Newbold C. J., de la Fuente G., Belanche A., Ramos-Morales E., McEwan N. R. (2015). The Role of Ciliate Protozoa in the Rumen. Frontiers in Microbiology 6, 6(1313). doi: 10.3389/fmicb.2015.01313
Ohnoutka C. A., Bondurant R. G., Boyd B. M., Hilscher F. H., Nuttelman B. L., Crawford G. I., et al. (2021). Evaluation of Coated Steroidal Combination Implants on Feedlot Performance and Carcass Characteristics of Beef Heifers Fed for Constant or Varying Days on Feed. Appl. Anim. Sci. 37 (1), 41–51. doi: 10.15232/aas.2020-02013
Oksanen J., Kindt R., Legendre P., O’Hara B., Stevens M. H. H., Oksanen M. J., et al. (2007). The Vegan Package. Community Ecology Package. 10, 631-637, 719.
Parr S. L. (2020) "Anabolic Implant Strategies in Beef Production", in: American Association of Bovine Practitioners Proceedings of the Annual Conference) 20–24. doi: 10.21423/aabppro20207957
Perry T. C., Fox D. G., Beermann D. H. (1991). Effect of an Implant of Trenbolone Acetate and Estradiol on Growth, Feed Efficiency, and Carcass Composition of Holstein and Beef Steers1. J. Anim. Sci. 69 (12), 4696–4702. doi: 10.2527/1991.69124696x
Pfaffl M. W., Lange I. G., Meyer H. H. D. (2003). The Gastrointestinal Tract as Target of Steroid Hormone Action: Quantification of Steroid Receptor mRNA Expression (AR, Erα, Erβ and PR) in 10 Bovine Gastrointestinal Tract Compartments by Kinetic RT-PCR. J. Steroid Biochem. Mol. Biol. 84 (2), 159–166. doi: 10.1016/S0960-0760(03)00025-6
Preston R. L. (1999). Hormone Containing Growth Promoting Implants in Farmed Livestock. Adv. Drug Deliv. Rev. 38 (2), 123–138. doi: 10.1016/S0169-409X(99)00012-5
Quast C., Pruesse E., Yilmaz P., Gerken J., Schweer T., Yarza P., et al. (2012). SILVA Ribosomal RNA Gene Database Project: Improved Data Processing and Web-Based Tools. Nucleic Acids Res. 41 (D1), D590–D596. doi: 10.1093/nar/gks1219
Richardson C. R., Hatfield E. E. (1978). The Limiting Amino Acids in Growing Cattle. J. Anim. Sci. 46 (3), 740–745. doi: 10.2527/jas1978.463740x
Saleem F., Bouatra S., Guo A. C., Psychogios N., Mandal R., Dunn S. M., et al. (2013). The Bovine Ruminal Fluid Metabolome. Metabolomics 9 (2), 360–378. doi: 10.1007/s11306-012-0458-9
Sauerwein H., Meyer H. H. D. (1989). Androgen and Estrogen Receptors in Bovine Skeletal Muscle: Relation to Steroid-Induced Allometric Muscle Growth. J. Anim. Sci. 67 (1), 206–212. doi: 10.2527/jas1989.671206x
Sauerwein H., Pfaffl M., Hagen-Mann K., Malucelli A., Meyer H. (1995). Expression of Estrogen and Androgen Receptor in the Bovine Gastrointestinal Tract. Journal of Biological Chemistry. 102 (4), 164–168.
Schreiber S. N., Knutti D., Brogli K., Uhlmann T., Kralli A. (2003). The Transcriptional Coactivator PGC-1 Regulates the Expression and Activity of the Orphan Nuclear Receptor Estrogen-Related Receptor α (Errα). J. Biol. Chem. 278 (11), 9013–9018. doi: 10.1074/jbc.M212923200
Smith Z. K., Thompson A. J., Hutcheson J. P., Nichols W. T., Johnson B. J. (2018). Evaluation of Coated Steroidal Implants Containing Trenbolone Acetate and Estradiol-17β on Live Performance, Carcass Traits, and Sera Metabolites in Finishing Steers. J. Anim. Sci. 96 (5), 1704–1723. doi: 10.1093/jas/sky095
Snochowski M., Lundström K., Dahlberg E., Petersson H., Edqvist L. E. (1981). Androgen and Glucocorticoid Receptors in Porcine Skeletal Muscle1. J. Anim. Sci. 53 (1), 80–90. doi: 10.2527/jas1981.53180x
Stahl D. A. (1991). Nucleic Acid Techniques in Bacterial Systematics. Eds. Stackebrandt E., Goodfellow M. Dev. Appl. Nucleic Acid Probes, 205–248. Wiley.
Sylvester J. T., Karnati S. K. R., Yu Z., Morrison M., Firkins J. L. (2004). Development of an Assay to Quantify Rumen Ciliate Protozoal Biomass in Cows Using Real-Time PCR. J. Nutr. 134 (12), 3378–3384. doi: 10.1093/jn/134.12.3378
Takai K., Horikoshi K. (2000). Rapid Detection and Quantification of Members of the Archaeal Community by Quantitative PCR Using Fluorogenic Probes. Appl. Environ. Microbiol. 66 (11), 5066–5072. doi: 10.1128/AEM.66.11.5066-5072.2000
Takenaka A., Tajima K., Mitsumori M., Kajikawa H. (2004). Fiber Digestion by Rumen Ciliate Protozoa. Microbes Environ. 19 (3), 203–210. doi: 10.1264/jsme2.19.203
Tan C., Ramírez-Restrepo C. A., Shah A. M., Hu R., Bell M., Wang Z., et al. (2020). The Community Structure and Microbial Linkage of Rumen Protozoa and Methanogens in Response to the Addition of Tea Seed Saponins in the Diet of Beef Cattle. J. Anim. Sci. Biotechnol. 11 (1), 80. doi: 10.1186/s40104-020-00491-w
Ushida K., Kayouli C., De Smet S., Jouany J. P. (1990). Effect of Defaunation on Protein and Fibre Digestion in Sheep Fed on Ammonia-Treated Straw-Based Diets With or Without Maize. Br. J. Nutr. 64 (3), 765–775. doi: 10.1079/BJN19900078
Wang Q., Garrity G. M., Tiedje J. M., Cole J. R. (2007). Naïve Bayesian Classifier for Rapid Assignment of rRNA Sequences Into the New Bacterial Taxonomy. Appl. Environ. Microbiol. 73 (16), 5261–5267. doi: 10.1128/AEM.00062-07
Wemheuer B., Wemheuer F., Daniel R. (2012). RNA-Based Assessment of Diversity and Composition of Active Archaeal Communities in the German Bight. Archaea 2012, 695826. doi: 10.1155/2012/695826
Keywords: implants, microbiome, rumen, metabolomics, cattle
Citation: Henniger MT, Wells JE, Hales KE, Lindholm-Perry AK, Freetly HC, Kuehn LA, Schneider LG, McLean KJ, Campagna SR, Christopher CJ and Myer PR (2022) Effects of a Moderate or Aggressive Implant Strategy on the Rumen Microbiome and Metabolome in Steers. Front. Anim. Sci. 3:889817. doi: 10.3389/fanim.2022.889817
Received: 04 March 2022; Accepted: 02 May 2022;
Published: 31 May 2022.
Edited by:
Edward Narayan, The University of Queensland, AustraliaReviewed by:
Anusorn Cherdthong, Khon Kaen University, ThailandAndres Alfredo Pech-Cervantes, Fort Valley State University, United States
Copyright © 2022 Henniger, Wells, Hales, Lindholm-Perry, Freetly, Kuehn, Schneider, McLean, Campagna, Christopher and Myer. This is an open-access article distributed under the terms of the Creative Commons Attribution License (CC BY). The use, distribution or reproduction in other forums is permitted, provided the original author(s) and the copyright owner(s) are credited and that the original publication in this journal is cited, in accordance with accepted academic practice. No use, distribution or reproduction is permitted which does not comply with these terms.
*Correspondence: Phillip R. Myer, cG15ZXJAdXRrLmVkdQ==
†Present address: Kristin E. Hales, Texas Tech University, Department of Animal and Food Sciences, Lubbock, TX, United States