- 1Bioeconomy and Environment, Natural Resources Institute Finland (Luke), Helsinki, Finland
- 2Marketing and Consumer Behaviour Group, Department of Social Science, Wageningen University, Wageningen, Netherlands
- 3Animal Welfare and Nutrition Service (SNiBA), Department of Animal and Food Science, Universitat Autònoma de Barcelona, Cerdanyola del Vallès, Spain
Within the European Union, there is no harmonization of farm animal welfare quality schemes for meat and dairy products. Instead, there are several industry-driven initiatives and voluntary schemes that seek to provide information on animal welfare for attentive consumers. This study had two aims. First, we quantified how selected industry-wide quality schemes cover the welfare of pigs and dairy cattle on farms by comparing the evaluation criteria selected by schemes with the animal-, resource- and management-based measures defined in the Welfare Quality protocol (WQ®). Second, we identified how these quality schemes use the data generated along the value chain (sensors, breeding, production, and health recordings) for animal welfare assessments. A total of 12 quality schemes, paying attention to animal welfare but not necessarily limited to welfare, were selected for the analysis. The schemes originated from eight European countries: Finland, Sweden, Denmark, Ireland, the Netherlands, Germany, Austria, and Spain. Among the studied quality schemes, we have identified 19 standards for certification: nine for dairy and 10 for pig production. Most of the analyzed standards were comprehensive in welfare assessment. In total, 15 out of 19 standards corresponded to WQ® in more than 70%. However, this high correspondence was obtained when allowing for different information sources (environment instead of animal) than defined in WQ®. Compared to WQ®, the investigated schemes were lagging in terms of the number of measures evaluated based on the animals, with only five standards, out of 19, using predominantly animal-based measures. The quality schemes mostly applied resource-based instead of animal-based measures while assessing good health and appropriate behavior. The utilization of data generated along the value chain by the quality schemes remains insignificant as only one quality scheme allowed the direct application of sensor technologies for providing information on animal welfare. Nevertheless, several schemes used data from farm recording systems, mostly on animal health. The quality schemes rely mostly on resource-based indicators taken during inspection visits, which reduce the relevance of the welfare assessment. Our results suggest that the quality schemes could be enhanced in terms of data collection by the broader utilization of data generated along the value chain.
Introduction
The European Union (EU) regulatory framework on animal welfare reflects the five freedoms and covers farming (Council of the European Union, 1998, 2008a,b), transport (Council of the European Union, 2005), and slaughter of animals (Council of the European Union, 2009). Yet, the EU does not regulate the market-based labeling of animal welfare-related product attributes explicitly, except to some extent for the organic and eggs production, as well as poultry meat. Therefore, only the generic EU regulations on product labeling apply to animal welfare claims made on the markets (van Wagenberg et al., 2012). However, the EU has initiated studies and a working group in the recently created Animal Welfare Platform of the European Commission to examine the options for creating an EU-wide animal welfare labeling scheme.
Recent years have seen the emergence of new policy initiatives for dairy and pig welfare (European Parliament, 2021). These include voluntary certification systems and private standards, hereafter called quality schemes, often introduced by non-governmental bodies such as food industry stakeholders or farmers' associations. The various quality schemes aim to build trust between stakeholders (e.g., between producers and their customers and, finally, end-consumers) by providing information on legal compliance and product differentiation concerning animal welfare-related attributes for meat and milk. This goal might be achieved using different criteria (standards for certification).
The methods used to assess animal welfare on the farm may vary in terms of the measures used (based on the animals, their handling, or their environment) and scoring systems (Main et al., 2003). Even though there is no one-size-fits-all solution for assessing animal welfare on farms (Main et al., 2003), various reports advocate for the increased use of animal- rather than resource- and management-based measures, as direct indicators of animal welfare (Welfare Quality®, 2009a; Panel EFSA on Animal Health Welfare, 2012; European Food Safety Authority, 2015). The shift toward animal-based welfare assessment has also been criticized for its impracticality, mainly due to the long time spent in conducting assessments and the challenges in delivering feedback to the farmers (Roe et al., 2011; European Food Safety Authority, 2015). Despite the above-mentioned difficulties in incorporating animal-based measures for assessment protocols, several quality schemes introduced such measures in the assessment procedures as they are considered the most valid indicators for welfare assessment (Lundmark et al., 2016; Lin et al., 2018; European Parliament, 2021).
Data-driven welfare assessment refers to the practice of basing welfare evaluation, whenever possible, on different data sources, namely sensors and breeding, production as well as health recordings, collected on farms or by processors. It may have benefits compared to the more traditional approaches to welfare assessment. In-person farm visits (e.g., inspections to check compliance with audit schemes) have been used for many years (e.g., Blokhuis et al., 2010). However, they only provide a snapshot of welfare, are time-consuming and costly, and may increase biosecurity risks on farms (Sørensen et al., 2007; Andreasen et al., 2014; Alarcón et al., 2021). One way to reduce the need for in-person assessment visits would be to exploit the data continuously collected on farms or by processors, through Precision Livestock Farming (PLF) tools or management software. Recent literature reviews and market analyses indicate that sensor technologies have a high potential in assessing animal welfare at the level of an individual animal (Buller et al., 2020; Gómez et al., 2021; Stygar et al., 2021). Routinely collected on-farm data have already been proven to be a useful source of welfare information in the dairy (e.g., Nyman et al., 2011; de Vries et al., 2014; Lutz et al., 2021) and pig (e.g., Stygar et al., 2020) sectors. Several studies suggest that recordings from slaughterhouses may provide useful information on animal wellbeing on farms (Vial, 2019).
Recently, the European Parliamentary Research Service (European Parliament, 2021) reported and compared animal welfare quality schemes across Europe. However, the utilization of routinely collected data along the value chain (e.g., farmers, processors) by different quality schemes was beyond the scope of the report. The same document pointed out the need for a more targeted comparison of quality schemes regarding their use of established animal welfare standards. In scientific literature, Welfare Quality® (WQ®) has been considered to be the most impactful assessment protocol in promoting the adoption of animal-based measures (Brscic et al., 2021). Despite some limitations of the WQ®, such as being time-consuming and lacking the clear aggregation of all indicators, it offers many advantages. In addition to the incorporation of animal-based measures, the advantages of WQ® are that it has been scientifically validated (Knierim and Winckler, 2009; Temple et al., 2011) and that it can be used for multiple farm animal species including pigs and dairy cattle. Also, WQ® is possibly the most scrutinized welfare assessment protocol (Czycholl et al., 2018; Tuyttens et al., 2021) and has been widely used as a reference protocol in scientific literature. However, the extent to which various quality schemes utilize the animal-, management- and resource-based measures defined by WQ® protocols has been seldom discussed (Heinola et al., 2021). Moreover, despite the cost of collecting data that verifies animal welfare for a quality scheme (Niemi et al., 2021), studies have paid little attention to the potential of using sensors and existing data recording systems to verify animal welfare in quality schemes.
The aim of this study was to compare the requirements set by animal welfare quality schemes with the WQ® scientific protocol and to quantify how industry-wide quality schemes (1) include animal-based indicators to assess the welfare of pigs and dairy cattle on farms and (2) utilize data generated along the value chain, especially on farms or at processing plants. The study objective was achieved by conducting a descriptive analysis of quantitative data gathered from certification standards. Hence, this study contributes to the literature on animal welfare assessment by describing the methods to assess animal welfare in the current quality schemes.
Materials and Methods
Criteria for Selecting the Quality Schemes
In this study, we have focused on industry-wide welfare quality schemes concerning pig and dairy production in EU countries. Industry-wide, in the context of this research, refers to systems that farmers can join voluntarily, irrespective of the processor they are affiliated to.
Quality assessment schemes that pay attention to animal welfare, but may not be limited to welfare only, were identified through desk research. Desk research was conducted between January 2020 and May 2020, using the Google search engine with the following search criteria: (dairy OR pork) (labeling scheme OR quality scheme) (animal welfare). The criteria were translated, and the search was performed in English, Spanish, Italian, Dutch, Danish, Polish, Finnish, and German languages. The search languages were selected based on the language coverage within the ClearFarm consortium. The translated terms are presented in Supplementary Table 1. The first five pages of the results from the Google search were scanned. The list of quality schemes identified was further checked and, where needed, supplemented based on the recently released report from the European Parliamentary Research Service (European Parliament, 2021) and other published scientific reports (Sørensen and Schrader, 2019; Heinola et al., 2021). One of the quality schemes identified did not have publicly available standards for certification and was therefore excluded from the analyses.
Altogether 12 quality schemes from eight European countries fulfilled the selection criteria. The schemes originated from Finland (Laatuvastuu), Sweden (KRAV), Denmark (Bedre Dyrevelferd, Anbefalet af Dyrenes Beskyttelse), Ireland (Origin Green), the Netherlands (Beter Leven, Meadow Milk), Germany (Initiative für mehr Tierwohl, Für Mehr Tierschutz), Austria (AMA-Gütesiegel), and Spain (Welfair, IAWS) were analyzed.
Assessment of Similarity Between WQ® and Standards Used by Quality Schemes
The Welfare Quality® protocol (WQ®) is a scientifically rigorous animal welfare assessment protocol for cattle (Welfare Quality®, 2009a) and pigs (Welfare Quality®, 2009b) developed by an EU-funded research project (Welfare Quality project, Grant Agreement 506508). Four animal welfare principles (good housing, good feeding, good health, and appropriate behavior) are further divided into 12 criteria (1. Absence of prolonged hunger, 2. Absence of prolonged thirst, 3. Comfort around resting, 4. Thermal comfort, 5. Ease of movement, 6. Absence of injuries, 7. Absence of disease, 8. Absence of pain by management procedures, 9. Expression of social behaviors, 10. Expression of other behaviors, 11. Good human-animal relationship, and 12. Positive emotional state). Regarding the specific types of measures, WQ® bases its assessment on indicators that can be observed on animals predominantly, however, in cases where animal-based indicators are not sensitive or reliable enough, resource and/or management-based measures have also been defined (Welfare Quality®, 2009a). In the dairy WQ®, around 68% of all measures are animal-based. For the pig WQ® (the combined measures for fattening pigs, sows, and piglets), the share of animal-based indicators is even higher, reaching 76%. For both pig and dairy protocols, good feeding is mostly based on resources or management measures. Good health, housing and appropriate behavior, by contrast, are largely based on animal-based measures. For some measures (e.g., mortality rate), WQ® allows the use of farm records.
In this study, the WQ® was used as a reference to compare how the reviewed assessment schemes address different aspects of dairy and pig welfare on farms. An Excel file with the list of welfare principles, criteria, and measures for growing pigs, sows, piglets, and dairy cows assessed on farms or at slaughter (when used to monitor the welfare on the farms) was prepared (Supplementary Tables 2, 3 for dairy and pig schemes, respectively). For each quality scheme, the standards for certification were identified (Table 1). In case the English version of a standard was not available, the Google Translate tool was used for translations. The standards were scanned in search of measures evaluating animal welfare. The measures identified were categorized according to their type (animal-, resource-, or management-based) and assigned to the appropriate principle, criteria, and measures defined in WQ®. Measures that could not be assigned to any of the measures defined in WQ® were omitted.
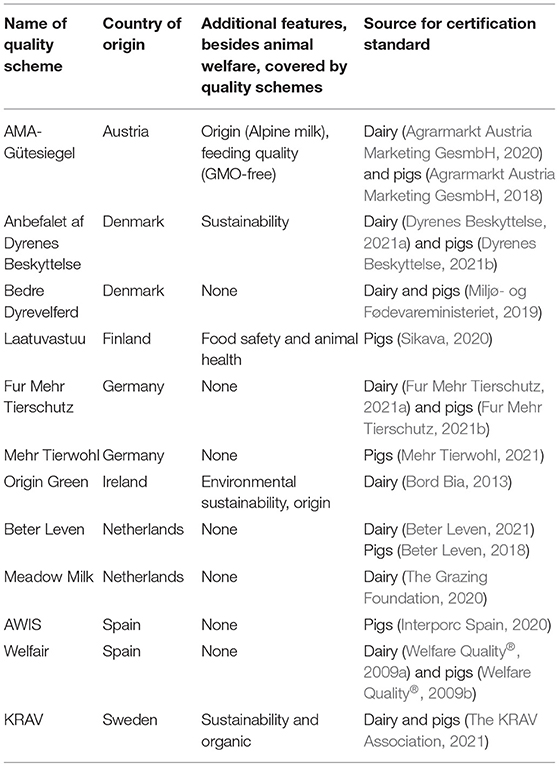
Table 1. Name, origin, additional features, and source of information concerning certification standards for analyzed quality schemes.
The correspondence of the welfare measures of the schemes to the WQ was calculated (as a percentage). Measures with the same source of information (animal, their handling/management, or their environment) as defined in WQ® were assigned direct correspondence. For example, direct correspondence was assigned when a quality scheme was used, similarly to WQ®, an animal-based measure to indicate prolonged hunger or a resource-based measure to indicate prolonged thirst. Direct correspondence was assigned irrespective of the method of the measurement applied. For instance, even though the evaluation of the absence of prolonged hunger was based on a different scale for body condition scoring than the measure defined by WQ®, it was classified as direct correspondence. Indirect correspondence was assigned to the measures that used a different source of information than those defined in WQ® (e.g., environment instead of animal). An example of indirect correspondence is that a quality scheme used a resource-based measure for evaluating feed quality or the access to roughage to assess the absence of prolonged hunger. Hence, in the context of this study, the “correspondence” means that the quality scheme used similar, but not necessarily identical, measures to assess animal welfare as WQ®. Finally, the shares of direct and indirect correspondence for animal-, resource-, and management-based types were calculated.
Because most schemes were initiated and operated by the private sector (European Parliament, 2021), to avoid using our results for commercial purposes, we decided to present them in an anonymized format. Standards for certifications defined by quality schemes were assigned to a number from 1 to 19. Data on the correspondence of the assigned numbers with the quality schemes are available upon request.
The Use of Different Data Sources for Animal Welfare Assessment
The documents describing the criteria for certifications were used to identify different data sources currently used in animal welfare assessment by quality schemes. In our analysis, we looked for sensors and breeding, production as well as health recordings, collected on farms or by processors. The following sources of data were considered: sensors that provide animal-based (e.g., milking robots), resource-based, or management-based (e.g., ambient monitoring sensors) information on farms, breeding data (e.g., test milking), self-reporting registries (e.g., mortality recordings, veterinary treatments) welfare self-assessment apps, pre- and post-mortem inspections at slaughter, as well as data from dairy companies on milk quality. For the data source, the inclusion criterion was that the information obtained from a particular data source had to be used for actual evaluation (a threshold was defined). For example, in the case of official registrations of health care and veterinary treatments, the scheme should provide a method for measuring compliance (e.g., numbers of treatment registrations, and the use of antibiotics below a specified target). The search also involved data sources that are not yet used for evaluation but are already included in the standards and planned to be incorporated in evaluation with a known date of applicability.
Results
Among the 12 quality schemes analyzed, seven included protocols for both pig and dairy production, while the remaining five were limited to either pigs or dairy. In total, we have identified 19 standards for certification, nine for dairy and 10 for pig production. One of the quality schemes analyzed had adopted WQ® for both dairy and pig production while others had developed their criteria for evaluation. The evaluation criteria, with relevance to WQ®, for each certification standard, are presented in Supplementary Table 2 for dairy and Supplementary Table 3 for pigs.
Quality Schemes vs. WQ®
The compliance of the quality schemes analyzed to the standards for certification in WQ® is presented in Figure 1. Of the 19 standards analyzed, only six directly complied with WQ® in more than 50% of the measures (three dairy protocols and three pig protocols). However, the majority of the schemes analyzed indirectly address the welfare principles included in the WQ® protocol by using a different source of information for a particular measure (e.g., space requirements for comfort around resting). The indirect correspondence was based on replacing information collected from animals with data gathered from their handling/management or their environment. When considering both direct and indirect correspondence, most schemes were comprehensive in animal welfare assessment. As an indicator of this correspondence, 15 out of 19 standards agreed with WQ® in more than 70%.
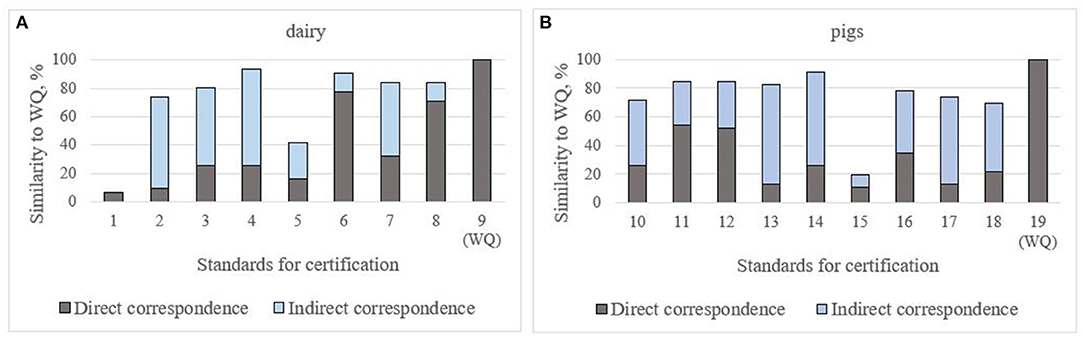
Figure 1. Similarity to WQ® expressed as direct and indirect correspondence in dairy (A) and pig (B) standards for certification. The direct correspondence was assigned to measures with the same source of information (animal, handling, or environment) as defined in WQ®. On the contrary, the indirect correspondence was assigned to those measures with different sources of information (e.g., environment instead of animal) as defined in WQ®. Standards 9 and 19 were based fully on WQ®. Standards listed in Table 1 were assigned to numbers 1–19.
Figure 2 illustrates the direct correspondence to WQ® for three types of measures (animal-, resource-, and management-based) in the dairy and pig standards studied. The results indicate that only three dairy and two pig standards had an elevated number of animal-based measures (equal to or above 50% in agreement with the animal-based measures defined in WQ®). Contrary to the animal-based measures, resource and management practices were well-represented across different standards. At least 50% agreement with both resource- and management-based measures defined in WQ® was found in 14 out of the 19 standards analyzed.
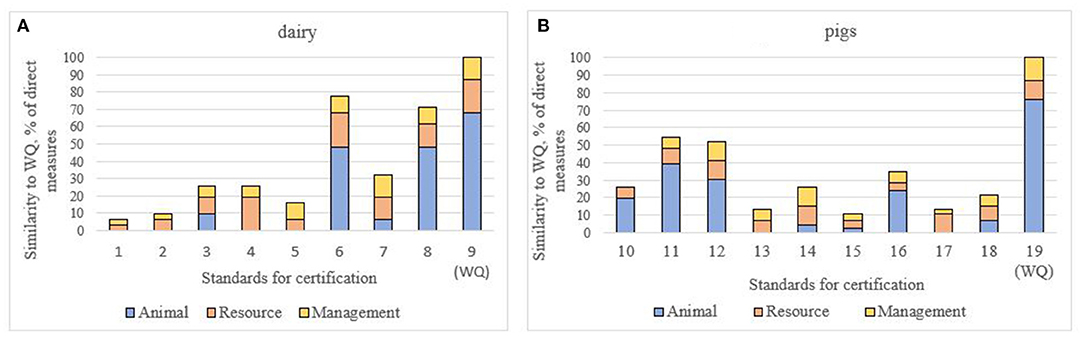
Figure 2. The direct correspondence to WQ® according to the type (animal-, resource-, management-based) in dairy (A) and pigs (B) standards for certification. The direct correspondence was assigned to measures with the same source of information (animal, their handling, or their environment) as defined in WQ®. Standards 9 and 19 correspond to one certification scheme that was based fully on WQ® and represent the distribution of animal-, resource- and management-based measures in WQ® for dairy cows and pigs. Standards listed in Table 1 were assigned to numbers 1–19.
Regarding the adherence to welfare principles, 18 out of 19 standards used at least one measure to assess good feeding and health. All (19/19) of the standards studied used at least one measure to assess good housing and appropriate behavior (Table 2). According to our analyses, good housing, health, and feeding had good coverage in the schemes analyzed. For good housing, in total, 18 out of 19 schemes had at least half of the measures indirectly or directly compliant with WQ®. For good health and feeding, the number of standards agreeing with a minimum of 50% of the measures defined by WQ® reached 16 and 13, respectively. The lowest correspondence was obtained for appropriate behavior, with only eight (out of 19) standards agreeing directly or indirectly with at least 50% of the measures defined in WQ® (Table 2).
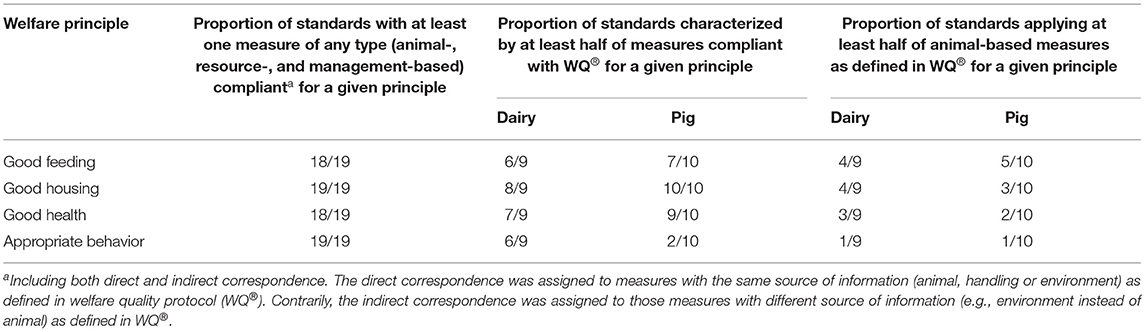
Table 2. The coverage of welfare principles (good feeding, good housing, good health, and appropriate behavior) by analyzed schemes.
Regarding the adherence to the good feeding principle, nine out of the 19 standards studied implemented animal-based measures (i.e., body condition) to evaluate the absence of prolonged hunger. The schemes relying on the resources to assess good feeding mostly verified access to feed (e.g., roughage), feed quality, or the condition of feeding equipment.
For good housing, the number of standards applying at least 50% of animal-based measures, as defined in WQ®, was slightly lower than for the good feeding principle (seven out of 19 standards). Dairy quality schemes that had developed their assessment protocols included resource-based (space requirements, type of bedding) instead of animal-based measures (e.g., the time needed to lie down) to describe the potential issues with space and stocking density in dairy cattle. Regarding pig schemes, thermal comfort and comfort around resting were mostly assessed using resources (type of bedding material, ventilation, and climate check).
The lowest share of animal-based measures was observed for good health and appropriate behavior. According to our analyses, only five and two standards applied at least 50% of animal-based measures as defined in WQ® for the evaluation of good health and appropriate behavior, respectively. Good health assessment, in most assessment protocols, was based on a few selected animal-based measures (e.g., milk somatic cell count, and tail biting) and supplemented with different resource-based measures (e.g., health plans designed together with veterinarians, antimicrobial monitoring). Regarding appropriate behavior, the schemes studied mentioned social behavior (negative and positive), exploratory behavior, and fear of humans evaluated on an animal level as part of the welfare evaluation.
The Use of Welfare-Relevant Data Generated on Farm or During Processing
Regarding the data sources, the direct application of sensor technologies and meters (for the animal-, resource- or management-based monitoring) was identified in one of the assessment protocols. The protocol allowed the application of accelerometer-based devices of three companies (DeLaval, Lely, and GEA) to track cow grazing time. However, we were unable to identify any further direct application of sensor technologies systematically generating data for the quality schemes we have studied.
Nevertheless, dairy quality schemes could indirectly utilize data generated through automatic milking systems (AMS), processed by official milk recording schemes. The example of the AMS application in official milk records includes an estimation of daily milk yields (ICAR, 2017). Several quality schemes mentioned the possibility to obtain milk production data from institutions carrying out official milk recordings, such as Swedish Kokontrollen®, Irish Cattle Breeding Federation, or LKV Austria, but the degree to which the quality schemes utilized the AMS-generated data for welfare assessment remains unknown.
The quality schemes used the breeding, production, and health recordings generated within the value chain to varying degrees. In total, 13 standards allowed for the gathering of data on at least one welfare measure from breeding, production, or health recordings. Regarding dairy standards, data from breeding, production, or health monitoring collected by third parties (the Dutch KoeMonitor,1 the Swedish Signaler Djurvälfärd,2 Irish Cattle Breeding Federation, and dairy health improvement associations LKV Austria) were listed as sources of relevant key performance parameters (mortality rates, milk production data, and/or prevalence of health problems such as mastitis or ketosis). Regarding pig welfare assessment, the identified data sources originated from farm management systems (mortality rates), health recordings (antimicrobial monitoring database), and slaughter meat inspection records (e.g., pneumonia or lesions on the body). A total of nine out of 10 standards for pig welfare evaluation used information from meat inspections at the slaughterhouse. For health records, four standards for certification (one dairy and three pigs) used treatment records to verify compliance with the target value (e.g., number of treatments).
Discussion
This study considered the question: How far are we from a data-driven and animal-based welfare assessment? To answer this question, we identified and analyzed 12 industry-wide quality schemes, seven assessing welfare for both dairy and pig production, three focusing on pig, and two on dairy production. In total, 19 standards for certification were examined, and the requirements set in each standard were compared with the animal-, management- and resource-based measures defined by a scientifically rigorous welfare assessment protocol (WQ®). Information on the utilization of data generated along the value chain (e.g., sensor data, farm registration, and slaughterhouse data) was systematically collected. The quality schemes we studied showed variability in the animal welfare requirements they evaluated. Most of the quality schemes analyzed in this study were comprehensive in animal welfare assessment, covering all welfare principles: good feeding, good housing, good health, and appropriate behavior. However, we also found a few quality schemes that considered selected measures (e.g., pasture time, intact tails, and space allowance) rather than employing a comprehensive welfare assessment protocol.
To date, several reports and scientific publications have analyzed the coverage (European Food Safety Authority, 2015; Sørensen and Schrader, 2019; European Parliament, 2021; Heinola et al., 2021), comparison to legislative requirements (Annen et al., 2011; Vogeler, 2019; Heinola et al., 2021), and transparency (More et al., 2021) of animal welfare quality schemes. However, to the authors' knowledge, none of these studies has used WQ® as a reference for the analysis of welfare standards. The Welfare Quality protocol was developed by experts on animal welfare throughout Europe and elsewhere, and it is considered a valid and comprehensive protocol for assessing animal welfare that is also independent of any market organization. The comparison of our results with the previously published analyses might be altered by year-to-year changes in the requirements set by the schemes, different inclusion criteria for the schemes as well as the emergence of new initiatives and quality schemes. Therefore, the discussion of our results will be limited to the current coverage of welfare issues by the different quality schemes, their strategies for data utilization, and the potential benefits of a data-driven animal-based welfare assessment that would be relevant to consumers, farmers, as well as for farm animals.
Welfare Coverage of the Quality Schemes
The starting point for our analyses was that animal welfare is measurable and that there is an established protocol that provides an approximate value for the welfare of dairy cattle and pigs. WQ®, at the time of being developed, was primarily considered a standardized research protocol, however, it was also envisioned as a potential tool for assessing compliance with welfare legislation, EU standards for welfare labeling as well as a farm management tool (Blokhuis et al., 2010). More than 10 years after being published (Blokhuis et al., 2010), WQ® certainly has had an impact on farm animal welfare assessment, with increased numbers of animal-based measures being referred to in the scientific literature (Brscic et al., 2021). Based on our results, WQ® has also influenced the assessment protocols of several quality schemes, with one quality scheme fully implementing the WQ® protocol for welfare assessment. However, WQ® or any other inventory of measures, has so far not become an official welfare quality assurance system at the EU level. There could be several reasons for the only partial success of WQ® as a welfare labeling initiative. Probably the most notorious is the lack of transparency related to scoring aggregation algorithms and the high workload associated with the assessment (Tuyttens et al., 2021).
Considering both direct and indirect correspondence, 15 out of 19 standards were in line with WQ® in more than 70% of the measures. Therefore, the standards used by most of the quality schemes build quite a comprehensive picture of animal welfare, like WQ®, providing information both on compliance with legal requirements and on consumer-desired welfare attributes which facilitate product differentiation. To provide information on compliance with the minimum legal requirements, 18 out of 19 standards used measures to assess the absence of prolonged thirst and hunger also required by the Council Directive 98/58/EC, which sets the welfare standards of all farmed animal species in the EU (Council of the European Union, 1998). Furthermore, all the pig welfare protocols analyzed, by assessing either the animals or their environment, evaluated the provision of enrichment material for pigs required by the EU Council Directive 2008/120/EC (Council of the European Union, 2008a) and Commission Recommendation (EU) 2016/336 of 8 March 2016 (The European Commision, 2016), both regulating pigs' welfare. Regarding the compliance with animal welfare characteristics that exceed the current minimum legal requirements and are desired by consumers (e.g., outdoor access), the quality schemes varied in the number of measures they evaluated. The tendencies in product differentiation are noticeable, shifting from the specific aspects of welfare (standards 1, 5, and 15) toward a more holistic evaluation of the state of welfare (other schemes). The holistic evaluation covering all four welfare principles (good feeding, good housing, good health, and appropriate behavior), was, however, obtained by using many different types of measures that were mostly resource-based. An obvious consequence of this diversification at the EU level is the lack of clarity for consumers to understand which labeled products have the highest welfare standard and/or better reveal the welfare of farm animals.
In this study, we have analyzed publicly available standards of certification. However, measurements used during audits are generally more complex than indicated in the regulation paragraphs (Lundmark et al., 2016). Therefore, the results obtained concerning welfare coverage should be confirmed in future studies using more precise information from assessment protocols.
The New Data Era—The Current and Potential Utilization
According to our results, quality schemes are, to a great extent, assessing animal welfare by following environmental factors (inputs, such as a type of bedding) rather than an animal-based response to those factors (outcomes, such as lameness or cleanliness). This result is expected when taking into account that quality schemes are assessing welfare by performing regular (mostly annual) farm visits (Heinola et al., 2021). In-person evaluations favor resource- or management-based measures because they are expected to require a shorter time for farm visits, which reduces the cost of the assessment. Furthermore, a resource-based evaluation is less prone to bias and interference compared to an animal-based assessment because resources can often be measured without the challenges associated with the interpretation of animal welfare. Contrarily, collecting data on animal-based measures by evaluators remains challenging. For example, the experience of the assessor might influence an animal-based evaluation and affect the robustness of the assessment (Bokkers et al., 2012; Czycholl et al., 2017). Also, the time required to observe a sufficient number of animals in person can be substantial (Knierim and Winckler, 2009), however, usually in a limited observation window (a certain part of a day), which may affect scoring (Gutmann et al., 2015). Solutions for increasing the use of animal-based measures and facilitating real-time welfare assessment would replace subjective in-person evaluations, potentially resulting in more objective data-based evaluations. Farms and processors are already collecting a vast amount of data in an automated manner, for example, data on milk yield and composition, including indicators of subclinical mastitis, is collected in a centralized manner from 30 to 93% of the cows in selected central European countries (Bucek et al., 2014), and ~70–97% of the cows in the Nordic countries (Wolff et al., 2012). Also, the EU legislation imposes on all suppliers an obligation of bulk tank somatic cell count testing for raw milk (More et al., 2013), as well as meat inspection before and after slaughter for animals sent to the slaughterhouse (Alban et al., 2011). The adoption of on-farm sensor systems for monitoring animal health, fertility, and productivity may also provide relevant information to assess animal welfare. For instance, in Italy and the Netherlands, over 40% of medium or large dairy farms (>50 cows) use sensor technology (Steeneveld and Hogeveen, 2015; Lora et al., 2020). In the Nordic countries, this percentage is even higher, reaching ~70% of larger farms in Finland (Utriainen et al., 2019). The possibility to utilize the above-mentioned and other readily collected data would benefit quality schemes because it could reduce the workload and costs of verification of conformity and increase the transparency of labels.
The increasing digitalization of the livestock sector offers promising developments for information policy, such as product labeling, which has led to proposals basing the livestock certification system on real-time measurements and considering animal behavior as a criterion for quality labeling (Council on Animal Affairs, 2019). However, our analyses of the existing quality schemes indicated that the utilization of data generated along the value chain remains insignificant and that industry-wide schemes are still far from providing real-time and animal-based welfare assessments. We were unable to identify schemes that systematically integrate data from multiple sources (from sensors and breeding, production as well as health recordings). Reasons for such an underutilization of data are related to, among others, big data characteristics (volume, velocity, variety, and veracity), issues related to data ownership, and compliance with data protection regulations (Bahlo et al., 2019; Klerkx et al., 2019; Osinga et al., 2022). Other reasons for the deficiencies in the utilization of the existing data include the unavailability of welfare classification algorithms based on automatically-collected data. The recent market reviews of PLF technologies indicated that farmers have access to various sensors tracking dairy and pig behavior and productivity (Gómez et al., 2021; Stygar et al., 2021). However, none of the solutions currently available on the market provide an overall welfare assessment. Moreover, the currently available PLF technologies have a low potential for assessing appropriate behavior (Stygar et al., 2021). Therefore, replacing manually collected resource-based measures, such as the availability of enrichment material with automatic animal-based evaluation provided with sensor technologies seems difficult in commercial farm settings. While several models for detecting health issues with sensor data exist, their predictive performance remains a challenge (Steensels et al., 2017). Recent research has nevertheless shown the potential of sensor data to estimate complex traits and predict the resilience rank of cows on a farm accurately (Adriaens et al., 2021).
Previous studies indicated that business models using farm advisers to interpret the information may enhance the value of information from PLF (Rojo-Gimeno et al., 2019). A few examples of data use for welfare assessment identified in this study seem to indicate that farm advisers might play a special role in strengthening the utilization of data for welfare assessment. It seems that the key element for data integration for animal welfare assessment is the cooperation between actors holding the data (e.g., farmers associations, farm advisers, and technology providers) and supply chain stakeholders such as private labeling initiatives. Farm adviser organizations could contribute to distributing the benefits from the use of digital data (Ayre et al., 2019) between consumers, farmers, and ultimately farm animals.
Implications of Data-Driven Animal Assessment for Consumers
Earlier, consumer study results indicated that consumers have high expectations that the integration of PLF technology in herd management would lead to improvements in the health and welfare of farm animals and the environment (Krampe et al., 2021). Nevertheless, consumers also fear that the integration of technology-driven approaches will lead to more industrialization, digitalization, and robotization, which is in contradiction to the pre-existing images they have of livestock farming. Since consumers tend to prefer natural approaches to managing animal production (Clark et al., 2019), value-based production concepts using state-of-the-art technology may be the less preferred options for obtaining animal welfare information. Consumers also believe that data systems are vulnerable to misuse, which have an impact on the trust consumers associate with assessment schemes and consequently the labeled products. These aspects must be acknowledged when developing data-driven approaches to animal welfare assessment.
As indicated before, resource-based measures are an easy solution for labeling, but less informative of the real welfare of farm animals. Animal-based assessment is preferable as it is more reliable but would be more challenging to convert into something marketable to be presented on the package, leaflet, or an innovative mobile application. Therefore, the introduction of data-driven, animal-based welfare assessment requires new business models and intelligent communication solutions, where the consumer can inter alia “visit” farms in a digital context, while only receiving individualized information on the desired features generated from different data sources. Using sensor and registry data, consumers could be informed on, for example, how many hours animals were outside, how they were interacting with each other (playing, suckling, etc.) and their current health status. Data-driven solutions for animal welfare might also play an important role in increasing food production transparency, which is a major concern of quality assurance programs (More et al., 2021). The harmonization of consumer needs and technological possibilities, therefore, play a pivotal role in the integration or development of labeling strategies. The demands of consumers and other supply chain actors should be at the core of any (re)developmental process of communication policies that are built on state-of-the-art technologies. Since the consumers' level of information is determined to a large degree by specific product labeling at the retail level (Faucitano et al., 2017), there should be more industrial or institutional leadership to introduce PLF as the source of data and subsequently information. Finally, the utilization of data-driven assessment schemes might help companies, governments, and non-governmental organizations to define objective standards that can be used to inform consumers in a harmonized way.
Implications of Data-Driven Animal-Based Assessment for Farmers and Animals
Farms with major animal welfare problems will also lag in terms of production performance (Frondelius et al., 2020). Therefore, farmers have an economic interest in maintaining high standards for animal welfare. So far, dedicated tools for automatically-collected data on cow behavior and milk production have been successfully used in providing farmers with precise and real-time information on how small changes in herd management, for instance, on feeding (Stygar et al., 2017) and housing practices (Czubernat et al., 2020), influence the production results and consequently economic profit. Therefore, data-driven animal-based welfare assessment can facilitate improvements and allow farmers to adjust their strategies according to animal productivity. The utilization of animal-based measures and continuously recorded digital data could refine welfare evaluation so that herd management can be improved. This may represent a significant change in welfare assessment for certification purposes, as continuous monitoring will allow certifying of products based on their day-to-day performance, instead of single visits once a year. However, even though the PLF assessment brings important benefits for the transparency and refinement of animal farming, some voices have raised concerns that the application of PLF for farm management may lead to farmer deskilling and loss of autonomy (Cornou, 2009; Schillings et al., 2021), whereas, as mentioned, consumers are worried about robotization concerning animal care (Krampe et al., 2021). These concerns are, to some degree, related to the novelty of digitalization in animal farming, so they might disappear in the future, but they should not be ignored. They can be addressed by informing stakeholders (such as producers and consumers) about the benefits (and the risks) of using new technologies on the farms. For instance, Schillings et al. (2021) list potential solutions for the ethical implications of PLF utilization, namely developing technologies in consultations with multiple stakeholders and advisory support for farmers.
Animal welfare can be improved by market initiatives, however, the improvement requires a range of favorable conditions, such as an appealing narrative, tying up animal welfare with attributes related to human health, good product availability, labeling, and a low-price premium (Heerwagen et al., 2013). To strengthen the application of data-driven welfare assessment in the markets, we need to be more knowledgeable about the socioeconomic implications of technology use in animal production. For instance, on how the application of sensor technologies could affect the economic results and animal welfare on a farm, or how farmers/consumers can utilize data from different sources in making informative decisions in herd management/purchase.
Perspectives
Animal welfare could be evaluated using different methods (Czycholl et al., 2015), and the selection of a suitable protocol/method depends on the purpose for which it is used [e.g., checking compliance with legislation, farm management tools (Berg and Hedman, 2020)]. Our intention was not to study the question: “Which certification standard is best suited for the welfare evaluation of farm animals?” but our approach could serve as a starting point for future analyses to identify recurring trends in quality schemes in the EU. As the range of measures shows, striving for the development of improved animal welfare protocols requires multidisciplinary approaches, the involvement of different stakeholders, and also the consideration of (national and EU-wide) animal welfare legislation, which is anchored in the respective policies of the member states. To identify improved solutions for welfare assessment in quality schemes, welfare protocols should be analyzed by considering the perspectives of multiple stakeholders: the farm animals', business actors', and consumers'. Even though animals cannot provide their subjective evaluation of wellbeing, their coping abilities in challenging conditions can be assessed by measuring the endocrine response (Tilbrook and Ralph, 2018) or changes in behavior (Llonch et al., 2020). Therefore, future efforts should focus on studying the correlation between the biological and behavioral responses to breeding conditions (expressed as different levels of hormones or activity) with measures that are defined in welfare assessment protocols, and integrate those that are more sensitive to animal welfare, so it can be better monitored. Moreover, since welfare protocols should also provide information that is aligned with consumer preferences (confirming or not that the product has desirable qualities), future research should consider the comparison of farm rankings obtained based on objective welfare assessment protocols and subjective consumer/welfare expert rankings.
Conclusion
How far are we from data-driven and animal-based welfare assessment? The results of our analysis suggest that there is still a long way to systematically integrate data-driven animal-based welfare assessments across the EU member states in a harmonized manner. Currently, only 26% of the assessment protocols use animal-based measures as the main source of information about welfare. Most protocols still rely on environmental (housing conditions) or animal handling (management procedures) measures to provide consumers with information on animal welfare. The practice of basing welfare evaluation on different data sources originating from sensors and breeding, production as well as health recordings, is not widely adopted. Only one out of 19 standards included the option of using data from sensor technologies. Even though 13 analyzed standards allowed obtaining information on welfare from breeding, production, or health records generated within the value chain, data-driven information was limited to a few selected measures. Multidisciplinary research can help to implement innovative technologies and algorithms for welfare assessment. More efficient communication regarding animal welfare could be achieved by involving the main stakeholders (farmers, business actors, and consumers) in the development of new animal welfare labeling schemes.
Data Availability Statement
The original contributions presented in the study are included in the article/Supplementary Material, further inquiries can be directed to the corresponding author.
Author Contributions
AS and JN developed the study concept. AS, CK, PL, and JN conducted quality schemes search. AS was responsible for assigning welfare criteria from analyzed schemes and wrote the paper with inputs from CK, PL, and JN. All authors approved the submitted version.
Funding
This study was conducted within the ClearFarm Project aiming to co-design, develop, and validate a software platform powered by PLF Technologies to provide animal welfare information. This project has received funding from the European Union's Horizon 2020 research and innovation program under grant agreement no. 862919.
Conflict of Interest
The authors declare that the research was conducted in the absence of any commercial or financial relationships that could be construed as a potential conflict of interest.
Publisher's Note
All claims expressed in this article are solely those of the authors and do not necessarily represent those of their affiliated organizations, or those of the publisher, the editors and the reviewers. Any product that may be evaluated in this article, or claim that may be made by its manufacturer, is not guaranteed or endorsed by the publisher.
Supplementary Material
The Supplementary Material for this article can be found online at: https://www.frontiersin.org/articles/10.3389/fanim.2022.874260/full#supplementary-material
Footnotes
1. ^https://www.koemonitor.nl/en/.
2. ^https://www.vxa.se/radgivning-och-kurser/analysera-nulaget/analysera-djurhalsan/signaler-djurvalfard/.
References
Adriaens, I., Brulle, I., van den D'Anvers, L., Statham, J. M. E., Geerinckx, K., Vliegher, S. D., et al. (2021). Milk losses and dynamics during perturbations in dairy cows differ with parity and lactation stage. J. Dairy Sci. 104, 405–418. doi: 10.3168/jds.2020-19195
Agrarmarkt Austria Marketing GesmbH (2018). Schweinehaltung. Available online at: https://amainfo.at/fileadmin/user_upload/B2B/Documents/LW_Schwein/AMA-G%C3%BCtesiegel_Richtlinie_Schweinehaltung.pdf (accessed October 25, 2021).
Agrarmarkt Austria Marketing GesmbH (2020). Haltung Von Kühen. Available online at: https://amainfo.at/fileadmin/user_upload/B2B/Documents/LW_K%C3%BChe/AMA-G%C3%BCtesiegel_Richtlinie_Haltung_von_K%C3%BChen.pdf (accessed October 22, 2021).
Alarcón, L. V., Allepuz, A., and Mateu, E. (2021). Biosecurity in pig farms: a review. Porc. Health Manag. 7:5. doi: 10.1186/s40813-020-00181-z
Alban, L., Steenberg, B., Stephensen, F. T., Olsen, A., and Petersen, J. V. (2011). Overview on current practices of meat inspection in the EU. EFS 3:8. doi: 10.2903/sp.efsa.2011.EN-190
Andreasen, S., Sandøe, P., and Forkman, B. (2014). Can animal-based welfare assessment be simplified? A comparison of the Welfare Quality extregistered protocol for dairy cattle and the simpler and less timeconsuming protocol developed by the Danish Cattle Federation. Anim. Welfare 23, 81–94. doi: 10.7120/09627286.23.1.081
Annen, D. N., Wieck, C., and Kempen, M. (2011). Evaluation of minimum animal welfare conditions in national standards and farm certification schemes for pig fattening. Acta Agri. Scand. Sect. A 61, 40–54. doi: 10.1080/09064702.2010.544753
Ayre, M., Mc Collum, V., Waters, W., Samson, P., Curro, A., Nettle, R., et al. (2019). Supporting and practising digital innovation with advisers in smart farming. Wageningen J. Life Sci. 90:100302. doi: 10.1016/j.njas.2019.05.001
Bahlo, C., Dahlhaus, P., Thompson, H., and Trotter, M. (2019). The role of interoperable data standards in precision livestock farming in extensive livestock systems: a review. Comput. Electr. Agri. 156, 459–466. doi: 10.1016/j.compag.2018.12.007
Berg, C., and Hedman, F. L. (2020). Compliance with animal welfare regulations: drivers and consequences. CAB Rev. 15:25. doi: 10.1079/PAVSNNR202015025
Beter Leven (2018). Criteria for Evaluation - Pork. Available online at: https://beterleven.dierenbescherming.nl/zakelijk/en/participate-2/company-type/livestock-farms/pigs/ (accessed October 21, 2021).
Beter Leven (2021). Criterial for Evaluation - Dairy. Available online at: https://beterleven.dierenbescherming.nl/zakelijk/en/participate-2/company-type/livestock-farms/dairy-cattle/ (accessed October 21, 2021).
Blokhuis, H. J., Veissier, I., Miele, M., and Jones, B. (2010). The Welfare Quality® project and beyond: safeguarding farm animal well-being. Acta Agri. Scand. Sect. A 60, 129–140. doi: 10.1080/09064702.2010.523480
Bokkers, E., Vries, A., de Antonissen, M., and de Boer, I. (2012). Inter- and intra-observer reliability of experienced and inexperienced observers for the Qualitative Behaviour Assessment in dairy cattle. Anim. Welf. 21, 307–318. doi: 10.7120/09627286.21.3.307
Bord Bia (2013). Sustainable Dairy Assurance Scheme Producer Standard. Available online at: https://www.bordbia.ie/globalassets/bordbia.ie/farmers–growers/farmers/qas/document-libraries/sdas-pdfs/sdas-producertandard.pdf (accessed October 22, 2021).
Brscic, M., Contiero, B., Magrin, L., Riuzzi, G., and Gottardo, F. (2021). The use of the general animal-based measures codified terms in the scientific literature on farm animal welfare. Front. Vet. Sci. 8:634498. doi: 10.3389/fvets.2021.634498
Bucek, P., Zottl, K., Onken, F., Klopčic, M., Radzio, D., Mészarós, G., et al. (2014). Practical Aspects in Milk Recording in Central and Eastern Europe and Its Effect on the Guidelines. Available online at: https://www.icar.org/index.php/icar-meetings-news/berlin-2014-presentations-pictures-and-inewsletter/ (accessed January 12, 2022).
Buller, H., Blokhuis, H., Lokhorst, K., Silberberg, M., and Veissier, I. (2020). Animal welfare management in a digital world. Animals 10:1779. doi: 10.3390/ani10101779
Clark, B., Panzone, L. A., Stewart, G. B., Kyriazakis, I., Niemi, J. K., Latvala, T., et al. (2019). Consumer attitudes towards production diseases in intensive production systems. PLoS ONE 14:e0210432. doi: 10.1371/journal.pone.0210432
Cornou, C.. (2009). Automation systems for farm animals: potential impacts on the human—animal relationship and on animal welfare. Anthrozoös 22, 213–220. doi: 10.2752/175303709X457568
Council of the European Union (1998). Council Directive 98/58/EC of 20 July 1998 Concerning the Protection of Animals Kept for Farming Purposes. Available online at: https://eur-lex.europa.eu/legal-content/EN/TXT/?uri=celex%3A31998L0058 (accessed December 28, 2021).
Council of the European Union (2005). Council Regulation (EC) No 1/2005 of 22 December 2004 on the Protection of Animals During Transport and Related Operations and Amending Directives 64/432/EEC and 93/119/EC and Regulation (EC) No 1255/97. Available online at: https://eur-lex.europa.eu/legal-content/en/ALL/?uri=CELEX%3A32005R0001 (accessed December 28, 2021).
Council of the European Union (2008a). Council Directive 2008/120/EC of 18 December 2008 Laying Down Minimum Standards for the Protection of Pigs. Available online at: https://eur-lex.europa.eu/legal-content/EN/ALL/?uri=CELEX%3A32008L0120 (accessed December 28, 2021).
Council of the European Union (2008b). Council Directive 2008/119/EC of 18 December 2008 Laying Down Minimum Standards for the Protection of Calves. Available online at: https://eur-lex.europa.eu/legal-content/EN/ALL/?uri=CELEX%3A32008L0119 (accessed December 28, 2021).
Council of the European Union (2009). Council Regulation (EC) No 1099/2009 of 24 September 2009 on the Protection of Animals at the Time of Killing. Available online at: https://eur-lex.europa.eu/legal-content/EN/TXT/?uri=celex%3A32009R1099 (accessed December 28, 2021).
Council on Animal Affairs (2019). Digitisation of the Livestock Farming Sector. Available online at: https://english.rda.nl/publications/publications/2020/02/13/digitisation-of-the-livestock-farming-sector (accessed January 4, 2020).
Czubernat, A., Makulska, J., Krogh, M., and Stygar, A. (2020). The use of evolutionary operations to assess the intervention effect on the behaviour of dairy cows. Roczniki Naukowe Polskiego Towarzystwa Zootechnicznego 16, 59–68. doi: 10.5604/01.3001.0014.6073
Czycholl, I., Beilage, E. G., Henning, C., and Krieter, J. (2017). Reliability of the qualitative behavior assessment as included in the Welfare Quality Assessment protocol for growing pigs. J. Anim. Sci. 95, 3445–3454. doi: 10.2527/jas.2017.1525
Czycholl, I., Büttner, K., Grosse Beilage, E., and Krieter, J. (2015). Review of the assessment of animal welfare with special emphasis on the Welfare Quality® animal welfare assessment protocol for growing pigs. Arch. Anim. Breed 58, 237–249. doi: 10.5194/aab-58-237-2015
Czycholl, I., Kniese, C., Casey-Schrader, L., and Krieter, J. (2018). How reliable is the multi-criteria evaluation system of the Welfare Quality® protocol for growing pigs? Anim. Welf. 27, 147–156. doi: 10.7120/09627286.27.2.147
de Vries, M., Bokkers, E. A. M., van Schaik, G., Engel, B., Dijkstra, T., de Boer, I. J. M., et al. (2014). Exploring the value of routinely collected herd data for estimating dairy cattle welfare. J. Dairy Sci. 97, 715–730. doi: 10.3168/jds.2013-6585
Dyrenes Beskyttelse (2021a). Specifikation - MALKEKVÆG. Available online at: https://www.dyrenesbeskyttelse.dk/sites/dyrenesbeskyttelse.dk/files/M%C3%A6rkekrav/M%C3%A6rkekrav%20malkekv%C3%A6g_0.pdf (accessed October 14, 2021).
Dyrenes Beskyttelse (2021b). Specifikation- Svin. Available online at: https://www.dyrenesbeskyttelse.dk/sites/dyrenesbeskyttelse.dk/files/M%C3%A6rkekrav/M%C3%A6rkekrav%20svin%2026.0.pdf (accessed October 14, 2021).
European Food Safety Authority (2015). The use of animal-based measures to assess animal welfare in EU – state of the art of 10 years of activities and analysis of gaps. EFS3 12:884. doi: 10.2903/sp.efsa.2015.EN-884
European Parliament (2021). Directorate General for Parliamentary Research Services. Animal Welfare on the Farm, Ex-post Evaluation of the EU Legislation: Prospects for Animal Welfare Labeling at EU Level : European Implementation Assessment. LU: Publications Office. Available online at: https://data.europa.eu/doi/10.2861/23838 (accessed October 8, 2021).
Faucitano, L., Martelli, G., Nannoni, E., and Widowski, T. (2017). “Fundamentals of animal welfare in meat animals and consumer attitudes to animal welfare,” in New Aspects of Meat Quality. (Amsterdam: Elsevier), 537–568. doi: 10.1016/B978-0-08-100593-4.00021-7
Frondelius, L., Jauhiainen, L., Niskanen, O., Mughal, M., and Sairanen, A. (2020). Can on-farm animal welfare explain relative production differences between dairy herds? Anim. Welf. 29, 449–461. doi: 10.7120/09627286.29.4.449
Fur Mehr Tierschutz (2021a). Kriterienkatalog für die Haltung und Behandlung von Milchkuhen. Available online at: https://www.tierschutzlabel.info/fileadmin/users/redakteur/redakteur_upload/Milchkuehe/RL_Milchkuehe_2021.pdf (accessed October 21, 2021).
Fur Mehr Tierschutz (2021b). Kriterienkatalog für die Haltung und Behandlung von Mastschweinen. Available online at: https://www.tierschutzlabel.info/fileadmin/users/redakteur/redakteur_upload/Mastschweine/RL_Mastschweine_2021.1.pdf (accessed October 21, 2021).
Gómez, Y., Stygar, A. H., Boumans, I. J. M. M., Bokkers, E. A. M., Pedersen, L. J., Niemi, J. K., et al. (2021). A systematic review on validated precision livestock farming technologies for pig production and its potential to assess animal welfare. Front. Vet. Sci. 8:660565. doi: 10.3389/fvets.2021.660565
Gutmann, A., Schwed, B., Tremetsberger, L., and Winckler, C. (2015). Intra-day variation of Qualitative Behaviour Assessment outcomes in dairy cattle. Anim. Welf. 24, 319–326. doi: 10.7120/09627286.24.3.319
Heerwagen, L., Christensen, T., and Sandøe, P. (2013). The prospect of market-driven improvements in animal welfare: lessons from the case of grass milk in Denmark. Animals 3, 499–512. doi: 10.3390/ani3020499
Heinola, K., Kauppinen, T., Niemi, J. K., Wallenius, E., and Raussi, S. (2021). Comparison of 12 different animal welfare labeling schemes in the pig sector. Animals 11:2430. doi: 10.3390/ani11082430
ICAR (2017). Procedure 1 of Section 2 of ICAR Guidelines - Computing 24-h Yields. Available online at: https://www.icar.org/Guidelines/02-Procedure-1-Computing-24-Hour-Yield.pdf (accessed December 17, 2021).
Interporc Spain (2020). Animal Welfare And Biosafety Technical Regulation “Interporc Animal Welfare Spain” IAWS. Available online at: https://www.bienestaranimalcertificado.com/animal-welfare-certified/how-is-it-obtained/ (accessed October 25, 2021).
Klerkx, L., Jakku, E., and Labarthe, P. (2019). A review of social science on digital agriculture, smart farming and agriculture. New contributions and a future research agenda. Wageningen J. Life Sci. 90:100315. doi: 10.1016/j.njas.2019.100315
Knierim, U., and Winckler, C. (2009). On-farm welfare assessment in cattle: validity, reliability and feasibility issues and future perspectives with special regard to the Welfare Quality extregistered approach. Anim. Welf. 18, 451–458. Available online at: https://www.ingentaconnect.com/contentone/ufaw/aw/2009/00000018/00000004/art00016 (accessed December 29, 2021).
Krampe, C., Serratosa, J., Niemi, J. K., and Ingenbleek, P. T. M. (2021). Consumer perceptions of precision livestock farming—a qualitative study in three European Countries. Animals 11:1221. doi: 10.3390/ani11051221
Lin, Y.-C., Mullan, S., and Main, D. C. J. (2018). Use of welfare outcome information in three types of dairy farm inspection reports. Asian-Australas J. Anim. Sci. 31, 1525–1534. doi: 10.5713/ajas.17.0851
Llonch, P., Hoffmann, G., Bodas, R., Mirbach, D., Verwer, C., and Haskell, M. J. (2020). Opinion paper: measuring livestock robustness and resilience: are we on the right track? Animal 14, 667–669. doi: 10.1017/S1751731119003306
Lora, I., Gottardo, F., Contiero, B., Zidi, A., Magrin, L., Cassandro, M., et al. (2020). A survey on sensor systems used in Italian dairy farms and comparison between performances of similar herds equipped or not equipped with sensors. J. Dairy Sci. 103, 10264–10272. doi: 10.3168/jds.2019-17973
Lundmark, F., Röcklinsberg, H., Wahlberg, B., and Berg, C. (2016). Content and structure of Swedish animal welfare legislation and private standards for dairy cattle. Acta Agri. Scand. Sect. A 66, 35–42. doi: 10.1080/09064702.2016.1198417
Lutz, B., Zwygart, S., Rufener, C., Burla, J.-B., Thomann, B., et al. (2021). Data-based variables used as indicators of dairy cow welfare at farm level: a review. Animals 11:3458. doi: 10.3390/ani11123458
Main, D. C. J., Kent, J. P., Wemelsfelder, F., Ofner, E., and Tuyttens, F. A. M. (2003). Applications for methods of on-farm welfare assessment. Anim. Welf. 12, 523–528. Available online at: http://www.ingentaconnect.com/content/ufaw/aw/2003/00000012/00000004/art00011 (accessed October 14, 2021).
Mehr Tierwohl (2021). Catalog of Criteria for Sow, Piglet and Pig Fattening. Available online at: https://initiative-tierwohl.de/tierhalter/downloads-ab-2021/ (accessed November 29, 2021).
Miljø- og Fødevareministeriet (2019). Bekendtgørelse om frivillig dyrevelfærdsmærkningsordning. Available online at: https://www.retsinformation.dk/eli/lta/2019/1441 (accessed October 20, 2021).
More, S. J., Clegg, T. A., Lynch, P. J., and O'Grady, L. (2013). The effect of somatic cell count data adjustment and interpretation, as outlined in European Union legislation, on herd eligibility to supply raw milk for processing of dairy products. J. Dairy Sci. 96, 3671–3681. doi: 10.3168/jds.2012-6182
More, S. J., Marchewka, J., Hanlon, A., Balzani, A., and Boyle, L. (2021). An evaluation of four private animal health and welfare standards and associated quality assurance programmes for dairy cow production. Food Policy 105:102169. doi: 10.1016/j.foodpol.2021.102169
Niemi, J. K., Heinola, K., Yrjölä, T., Väre, M., Kauppinen, T., Rausi, S., et al. (2021). Eläinten hyvinvointimerkintä suomalaisen kotieläintuotannon laadun ja kilpailukyvyn edistäjänä. Luonnonvara- ja biotalouden tutkimus 2021, 1–212. Available online at: http://urn.fi/URN:ISBN:978-952-380-279-7 (accessed January 31, 2022).
Nyman, A.-K., Lindberg, A., and Sandgren, C. H. (2011). Can pre-collected register data be used to identify dairy herds with good cattle welfare? Acta Vet. Scand. 53:S8. doi: 10.1186/1751-0147-53-S1-S8
Osinga, S. A., Paudel, D., Mouzakitis, S. A., and Athanasiadis, I. N. (2022). Big data in agriculture: between opportunity and solution. Agri. Syst. 195:103298. doi: 10.1016/j.agsy.2021.103298
Panel EFSA on Animal Health and Welfare (2012). Statement on the use of animal-based measures to assess the welfare of animals. EFS 2:10. doi: 10.2903/j.efsa.2012.2767
Roe, E., Buller, H., and Bull, J. (2011). The performance of farm animal assessment. UK Anim. Welf. 20, 69–78. https://www.ingentaconnect.com/contentone/ufaw/aw/2011/00000020/00000001/art00009 (accessed January 31, 2022).
Rojo-Gimeno, C., van der Voort, M., Niemi, J. K., Lauwers, L., Kristensen, A. R., and Wauters, E. (2019). Assessment of the value of information of precision livestock farming: a conceptual framework. Wageningen J. Life Sci. 90:100311. doi: 10.1016/j.njas.2019.100311
Schillings, J., Bennett, R., and Rose, D. C. (2021). Animal welfare and other ethical implications of Precision Livestock Farming technology. CABI Agri. Biosci. 2:17. doi: 10.1186/s43170-021-00037-8
Sikava (2020). Terveydenhuoltokäyntilomakkeen täyttöohje 12.6.2018 alkaen. Available online at: https://www.sikava.fi/PublicContent/Instructions (accessed October 12, 2021).
Sørensen, J., Rousing, T., Møller, S., Bonde, M., and Hegelund, L. (2007). On-farm welfare assessment systems: what are the recording costs? Anim. Welf. 16, 237–239. Available online at: https://www.ingentaconnect.com/contentone/ufaw/aw/2007/00000016/00000002/art00033 (accessed January 31, 2022).
Sørensen, J. T., and Schrader, L. (2019). Labelling as a tool for improving animal welfare—the pig case. Agriculture 9:123. doi: 10.3390/agriculture9060123
Steeneveld, W., and Hogeveen, H. (2015). Characterization of Dutch dairy farms using sensor systems for cow management. J. Dairy Sci. 98, 709–717. doi: 10.3168/jds.2014-8595
Steensels, M., Maltz, E., Bahr, C., Berckmans, D., Antler, A., and Halachmi, I. (2017). Towards practical application of sensors for monitoring animal health: the effect of post-calving health problems on rumination duration, activity and milk yield. J. Dairy Res. 84, 132–138. doi: 10.1017/S0022029917000176
Stygar, A. H., Chantziaras, I., Toppari, I., Maes, D., and Niemi, J. K. (2020). High biosecurity and welfare standards in fattening pig farms are associated with reduced antimicrobial use. Animal 2020, 1–9. doi: 10.1017/S1751731120000828
Stygar, A. H., Gómez, Y., Berteselli, G. V., Dalla Costa, E., Canali, E., Niemi, J. K., et al. (2021). A systematic review on commercially available and validated sensor technologies for welfare assessment of dairy cattle. Front. Vet. Sci. 8:177. doi: 10.3389/fvets.2021.634338
Stygar, A. H., Krogh, M. A., Kristensen, T., Østergaard, S., and Kristensen, A. R. (2017). Multivariate dynamic linear models for estimating the effect of experimental interventions in an evolutionary operations setup in dairy herds. J. Dairy Sci. 100, 5758–5773. doi: 10.3168/jds.2016-12251
Temple, D., Dalmau, A., Ruiz, A., de la Torre, J. L., Manteca, X., and Velarde, A. (2011). Application of the Welfare Quality® protocol to assess growing pigs kept under intensive conditions in Spain. J. Vet. Behav. 6, 138–149. doi: 10.1016/j.jveb.2010.10.003
The European Commision (2016). Commission Recommendation (EU) 2016/336 of 8 March 2016 on the Application of Council Directive 2008/120/EC Laying Down Minimum Standards for the Protection of Pigs as Regards Measures to Reduce the Need for Tail-Docking. Available online at: https://eur-lex.europa.eu/eli/reco/2016/336/oj (accessed January 13, 2022).
The Grazing Foundation (2020). Criteria for Evaluation - Meadow Milk. Available online at: https://www.weidemelk.nl/en/conditions.html (accessed October 21, 2021).
The KRAV Association (2021). Standards for KRAV-Certified Production - 2021 Edition. Available online at: https://www.krav.se/en/standards/download-krav-standards/ (accessed October 25, 2021).
Tilbrook, A. J., and Ralph, C. R. (2018). Hormones, stress and the welfare of animals. Anim. Prod. Sci. 58:408. doi: 10.1071/AN16808
Tuyttens, F. A. M., de Graaf, S., Andreasen, S. N., de Boyer des Roches, A., van Eerdenburg, F. J. C. M., Haskell, M. J., et al. (2021). Using expert elicitation to abridge the Welfare Quality® protocol for monitoring the most adverse dairy cattle welfare impairments. Front. Vet. Sci. 8:634470. doi: 10.3389/fvets.2021.634470
Utriainen, M., Pastell, M., Rinne, M., Kajava, S., and Myllymäki, H. (2019). “Sensor technologies in dairy farms in Finland,” in Precision Livestock Farming, eds B. O'Brien, D. Hennessy, L. Shalloo (Cork: 9th European Conference on Precision Livestock Farming), 98–104.
van Wagenberg, C. P. A., Brouwer, F. M., Hoste, R., and Rau, M. L. (2012). Comparative Analysis of EU Standards in Food Safety, Environment, Animal Welfare and Other Non-Trade Concerns With Some Selected Countries. Available online at: https://www.europarl.europa.eu/RegData/etudes/etudes/join/2012/474542/IPOL-AGRI_ET(2012)474542_EN.pdf (accessed December 28, 2021).
Vial, F.. (2019). Editorial: slaughterhouses as sources of data for animal health intelligence. Front. Vet. Sci. 5:332. doi: 10.3389/fvets.2018.00332
Vogeler, C. S.. (2019). Market-based governance in farm animal welfare—a comparative analysis of public and private policies in Germany and France. Animals 9:267. doi: 10.3390/ani9050267
Welfare Quality® (2009a). Welfare Quality® Assessment Protocol for Cattle. Lelystad: Welfare Quality®Consortium. Available online at: http://www.welfarequalitynetwork.net/media/1088/cattle_protocol_without_veal_calves.pdf (accessed September 18, 2020).
Welfare Quality® (2009b). Welfare Quality® Assessment Protocol for Pigs. Lelystad: Welfare Quality®Consortium. Available online at: http://www.welfarequalitynetwork.net/media/1018/pig_protocol.pdf (accessed August 23, 2021).
Keywords: cow, pig, labeling, PLF (precision livestock farming), animal-based measure
Citation: Stygar AH, Krampe C, Llonch P and Niemi JK (2022) How Far Are We From Data-Driven and Animal-Based Welfare Assessment? A Critical Analysis of European Quality Schemes. Front. Anim. Sci. 3:874260. doi: 10.3389/fanim.2022.874260
Received: 11 February 2022; Accepted: 07 April 2022;
Published: 06 May 2022.
Edited by:
Mara Miele, Cardiff University, United KingdomReviewed by:
Anders Ringgaard Kristensen, University of Copenhagen, DenmarkFrida Lundmark Hedman, Swedish University of Agricultural Sciences, Sweden
Copyright © 2022 Stygar, Krampe, Llonch and Niemi. This is an open-access article distributed under the terms of the Creative Commons Attribution License (CC BY). The use, distribution or reproduction in other forums is permitted, provided the original author(s) and the copyright owner(s) are credited and that the original publication in this journal is cited, in accordance with accepted academic practice. No use, distribution or reproduction is permitted which does not comply with these terms.
*Correspondence: Anna H. Stygar, YW5uYS5zdHlnYXImI3gwMDA0MDtsdWtlLmZp