- 1Laboratory of Animal Breeding & Husbandry, Department of Animal Science, Agricultural University of Athens, Athens, Greece
- 2Laboratory of Anatomy & Physiology of Farm Animals, Department of Animal Science, Agricultural University of Athens, Athens, Greece
- 3Laboratory of Nutritional Physiology & Feeding, Department of Animal Science, Agricultural University of Athens, Athens, Greece
Ovine mastitis is defined as the inflammation of the sheep udder, most commonly caused in response to intramammary infections. Based on the occurrence of clinical signs, mastitis is characterized as either clinical or subclinical (SCM). The impact of ovine SCM on the overall sustainability of dairy sheep farms has been substantially documented underpinning the significance of efficient diagnosis. Although SCM can be detected in cows, the performance and the validity of the methods used do not transfer in dairy sheep. This fact challenges the development of evidence-based ovine udder health management protocols and renders the detection and control of ovine mastitis rather problematic. Currently, cell culture-based models are being successfully used in biomedical studies and have also been effectively used in the case of bovine mastitis. The objective of the present study was to culture ovine primary mammary cells for the development of 2D and 3D cell culture-based models for the study of ovine mammary gland and to focus on the first stages of the intramammary infection by common mastitis-inducing pathogens. Cells were infected by E. coli and S. aureus mimicking the first stages of natural intramammary infections. The secreted proteins were subjected to mass-spectrometry resulting in the identification of a total of 79 distinct proteins. Among those, several had already been identified in healthy or mastitic milk, while others had not been previously detected for in the ovine mammary secretome. Our results suggest that the development of cell-based models for studying specific stages of intramammary infection has the potential to be beneficial for the udder health management in dairy sheep.
Introduction
Although bovine milk traditionally accounts for most of the world’s milk production, ovine milk holds a significant and steadily growing part of the production and the dairy market. The interest in ovine milk derives mostly from countries in Asia, Africa, and the southern Europe, where dairy sheep farming is of major importance. This is attributed mainly to historical/traditional and socioeconomic reasons, as well as to the distinct climate and territorial conditions of these areas that do not favor dairy cow farming. The limitation of low capital investment in the livestock sector of disadvantaged regions and the ability of sheep to utilize natural pasturelands for household milk production and consumption are also important in some countries (Libera et al., 2021). However, during the last decades, a remarkable intensification of dairy sheep farming has occurred in Europe and farming systems have evolved to meet the current consumer-driven demands for premium-quality, safe, healthy, and niche products in accordance to the modern health challenges and lifestyle perceptions. For example, in the context of increasing demands for animal-derived protein intake, sheep milk offers an alternative source for those with allergies to cow milk. It also fits well with organic farming (Mazinani and Rude, 2020) and has a decreased carbon footprint compared to other livestock sectors favoring environmentally friendly production.
Ovine mastitis is characterized by the inflammation of the udder in sheep and is usually caused by intramammary infections (Menzies and Ramanoon, 2001). The term subclinical mastitis (SCM) includes all these cases that although cannot be readily detected, due to the absence of clinical signs, they still affect both production and animal health status. Indeed, SCM decreases the milk yield, impairs the chemical and physical properties of the milk and its cheese yield capacity (Martí-De Olives et al., 2020) and is considered to negatively impact the overall sustainability of dairy sheep farms (Fthenakis and Jones, 1990; Ahmad et al., 1992; Murphy et al., 2018; Knuth et al., 2019). Furthermore, SCM associated with specific pathogens raises public health concerns as it increases the risk for milk-borne diseases. Moreover, it affects animal health status and increases the cost associated with: i) decreased milk production both quantitatively and qualitatively, ii) increased replacement rate due to unvoluntary culling of persistently affected animals, iii) specific preventive measures, veterinary services and treatment protocols iv) strict biosecurity measures, specialized equipment and labour-intensive practices to mitigate the risk of intramammary infections and SCM (Tvarožková et al., 2019).
It is obvious from the above that the prevention and control of SCM is of major importance for dairy sheep farming. However, research on SCM in sheep, a crucial step in understanding and managing SCM, using in vivo approaches, has several difficulties. One of the most important is the high cost of maintaining and working with animals, which is a factor that should be taken into particular consideration when planning experiments. Furthermore, the absence of a well-accepted diagnostic tool for ovine SCM can also be a challenge. The two most common tests for mastitis are the California Mastitis Test and the Somatic Cell Count of milk. Both of them are developed and established for dairy cows and although they have been used also in sheep, they may lead to inaccurate interpretation of udder health status (Libera et al., 2021). If the above tests are used for grouping animals, it is obvious that the establishment of groups with animals of similar characteristics and infection severity may have many difficulties. A different strategy for studying mastitis employs the infection of ewe’s udders with mastitis-inducing pathogens. This approach enables better-controlled conditions of infection, animal grouping and monitoring of the disease progression. However, it is an experimental approach that is not in line with animal welfare.
Two-dimensional (2D) and three-dimensional (3D) cell culture models (Duval et al., 2017) as well as more sophisticated organoids (Lou and Leung, 2018) are being successfully used for several years in human biomedical research for physiology and pathophysiology modelling. These models have the advantage of being quick, cost-efficient, and user-friendly when compared to in vivo experiments and they enable efficient control of the experimental conditions allowing appropriate modifications. There are of course limitations mainly associated with their inability to account for organ-organ interactions. However, complementary in vivo validation of model-derived results in a limited number of animals can overcome the aforementioned drawback while the rapid and continuous advances in the field make these models increasingly predictive and accurate.
In the last few years, mammary organoids derived from humans and rodents have been used in biomedical research contributing in the better understanding of mammogenesis, the physiological functions and pathophysiological responses (Mohan et al., 2021). Similar efforts have been made for the modelling of the bovine mammary gland, where both primary cells and cell lines have been utilized in 2D and 3D cultures for the study of mammogenesis and the secretion of milk proteins (Finot et al., 2021). Additionally, a few studies regarding 3D cultures of mammary gland are available in pigs and goats (Finot et al., 2021). On the contrary, to the best of our knowledge, modelling of the mammary gland of sheep using cell culture has not been attempted yet. Such species-specific tools could significantly facilitate the better understanding of the physiology of the ovine mammary gland. Furthermore, it could serve as the starting point for modelling mastitis in sheep resulting in the identification of markers with a diagnostic value.
Here we have made an attempt to model the ovine mammary gland by both 2D and 3D cell culture methods. Additionally, we infected these cell cultures with mastitis-induced pathogens to mimic the early stages of intramammary infection and we used mass-spectrometry in order to identify cell-secreted proteins potentially associated with this initial step of infection.
Materials and methods
Isolation and culture of ovine mammary cells
A tissue sample was excised from the mammary gland of a healthy four-year-old Chios ewe during late lactation. The procedure was carried out by a veterinary surgeon at the premises of the Agricultural University of Athens, under sedation and local anesthesia. Mammary gland tissue was collected via biopsy. All surgical procedures were performed under aseptic conditions and following standard protocols for the pain management post-operation. After obtaining the tissue sample, a part of the fresh tissue was placed in a sterile tube containing ice-cold Dulbecco’s Phosphate Buffer Saline (DPBS, Takara) and immediately transported to the laboratory. The tissue was adequately washed with DPBS containing penicillin/streptomycin and was cut into 1 mm3 pieces. Afterwards, these pieces were further processed for mechanical cell dissociation (Tissue grinder kit, Sigma Aldrich, Cat. # CD1-1KT), using a 100-mesh screen and according to the manufacturer’s instructions. Isolated mammary cells were washed twice in PBS and plated at a concentration of 1x106/ml into 100 mm plastic cell culture dishes (TC-treated, Sarstedt) for the 2D cultures. For the 3D cultures, cells were used to seed a PS scaffold according to the manufacturer’s instructions (3D Biotek 3D Insert™ PS scaffold, Sigma, cat. no. Z724181). Cultures were maintained at 37°C in a humidified incubator under 5% CO.
Culture and maintenance of primary cells
Primary cultures were maintained for the scope of the present study in order to avoid repeated freezing and thawing cycles and experiments were conducted using only primary cells that were not subjected in cryopreservation. However, stocks of isolated primary cells were subjected to several cycles of cryopreservation to test for their ability to propagate, adhere and form monolayers.
Mammary cells were maintained in Dulbecco’s modified Eagle’s medium (DMEM, Gibco) containing 10% fetal bovine serum (FBS, Biosera), penicillin-streptomycin (Biosera), sodium pyruvate (Biosera) and MEM Non-Essential Amino Acids (Biosera). The primary cell cultures were being passaged at circa 80% confluency. For the 2D cell cultures, the medium was replaced with fresh medium every two or three days until the cells formed a confluent monolayer. For the 3D cell cultures, PS scaffolds were used. Medium was replaced when deemed necessary for the enhancement of cells and the removal of non-adherent and dead cells.
Histology
Tissue sections were obtained from paraffin embedded, formalin fixed 3D scaffold cultures, with established presence of cells. Briefly, 3D scaffold cultures were placed in 4°C fixative solution (10 ml 37% formaldehyde in 90 ml PBS) overnight with gentle agitation, followed by a rinse in distilled H2O and submitted to microtome for glass slide preparation in 70% ethanol (in distilled H2O). Subsequently, glass slides were subjected to three changes of xylene for 2 min per change, followed by hydration as follows: i) two changes of 100% ethanol for 2 min per change, ii) 95% ethanol for 1 min, iii) 70% ethanol for 2 min. and thorough rinsing of the slides in running tap water at room temperature for 3 min. Slides were then stained in hematoxylin solution for 3 min (Mayer’s modified, Cat.No.: ab220365, AbCam), followed by placing the slides under running tap water at room temperature for 2 minutes. Slides were then stained in a working eosin Y solution for 2 min (Cat. No.: ab246823, AbCam). Slides were then dehydrated by dips in 95% ethanol and two changes of 100% ethanol for 1 min per change, followed by two changes in xylene for 1 min per change. and deparaffinized for 5 min at room temperature in xylene. Slides were gradually rehydrated in solutions of descending concentration of ethanol (100%, 80%, 70%, and 50% v/v) and finally in TBS (Tris-buffered saline) or PBS (Phosphate-buffered saline) solution. Finally, slides were counterstained with Hematoxylin, followed by mounting with glycerol and sealed with a cover slip.
Bacterial strains and growth conditions
Two bacterial strains associated with subclinical mastitis were used to infect the cell cultures, namely Staphylococcus aureus (S. aureus) (NCIMB 701499) and Escherichia coli (E. coli) (NCIMB 702070). Before each experiment, a fresh bacterial suspension was prepared from a cryopreserved stock culture. Both bacteria were cultured overnight at 37°C with agitation in 20 mL liquid Luria-Bertani (LB) (Tryptone 10 g/L, Yeast extract 5 g/L, and NaCl 10 g/L). Bacterial growth was monitored by measuring the optical density at 600 nm (OD600). When S. aureus and E. coli entered the logarithmic growth phase, the bacteria were used in experiments.
Infection with E. coli and S. aureus
Before the bacterial infection, primary cells were incubated under 5% CO2 at 37°C, in culture medium with decreasing quantity of FBS, for about two weeks until the day of the experiment. After removing FBS and antibiotics, cells were washed three times with DPBS. Subsequently, fresh bacterial cultures of E. coli and S. aureus in their logarithmic growth phase were added separately to the cultures diluted in DMEM in three different ratios to achieve three multiplicities of infection (MOI; defined as the ratio of bacteria to potential host cells). Thoroughly, confluent monolayers of mammary cells (3 × 106 cells/plate) were incubated with 3 × 106, 3 × 107, or 3 × 108 bacterial cells in order to achieve MOIs of 1, 10, or 100, respectively. The aim of three different MOIs, was to evaluate the ability of the bacteria to adhere and invade the mammary cells.
Adhesion and invasion assay
The experimental conditions for the assays were based on Roussel et al. (Roussel et al., 2017) with slight modifications. At 3 hours after infecting mammary cells with bacteria at several MOI, the supernatants were collected and the bacterial loads, representing bacteria free in the medium, were calculated after dilution of bacterial suspensions in sterile PBS and numeration on Tryptic Soy Agar (TSA, Sigma) plates. Then, the mammary cells were washed twice with DPBS to remove non-associated bacteria, lysed by addition of 0.2% Triton X-100 diluted in DPBS and incubated at 37°C for 10 min to release total associated bacteria (adherent and invasive). The bacterial loads, representing adherent and invasive bacteria (total associated bacteria), were determined after dilutions of bacterial suspensions in sterile PBS and numeration on TSA plates. In parallel, the gentamicin protection assay was performed in separate plates as follows for the quantification of invasive bacteria. Mammary cells were infected with bacteria as it was done in the quantification of total associated bacteria, with the following modification. After 3 hours post infection, cells were washed three times in DPBS and then incubated for 30 minutes with medium supplemented with 100 μg/ml gentamicin (Sigma–Aldrich) to kill any extracellular bacteria, so that invasion but not adherence was measured (Roussel et al., 2017). After 30 min, cells were washed with DPBS, lysed with 0.2% Triton X-100 diluted in DPBS and incubated at 37°C for 10 min. Lysates were enumerated on TSA plates. The invasion efficiency for each bacterial strain and for each MOI was defined as the ratio of invaded bacteria to the sum of bacteria in the supernatant and the total associated bacteria. Among the three MOIs, the one equal to 1 was chosen for the following analyses, because a) it was sufficient to enable bacteria to invade mammary cells, and b) exhibited the smallest invasion efficiency, which was considered as optimal approximation of the conditions in real farms where exposure to mastitis-inducing pathogens is not necessarily severe and acute. It should be noted that MOIs and invasion/adhesions assays were done solely on 2D cultures, as we find that for the case of 3D cultures it would be rather unnecessary to take into consideration all cells (included those of the inner layers) for estimating the above. However, we used the same number of bacteria for infecting both models.
Preparation of samples for proteomics analysis
After incubation for 3 h at 37°C, supernatants were centrifuged at 5,000×g for 3 min. Then, the supernatants were passed through 0.22 μm pore size filters and stored at -20°C for the subsequent analysis of proteins with mass spectrophotometry. In all experiments, S. aureus and E. coli-free cultures were used as control both for 2D and 3D cell culture model.
LC-MS/MS analysis
Samples were subjected to an in-solution tryptic digest using a modified version of the Single-Pot Solid-Phase-enhanced Sample Preparation (SP3) protocol (Moggridge et al., 2018). Samples were added to Sera-Mag Beads (Thermo Scientific, #4515-2105-050250, 6515-2105-050250) in 10 µl 15% formic acid and 30 µl of ethanol. Binding of proteins was achieved by shaking for 15 min at room temperature. SDS was removed by 4 subsequent washes with 200 µl of 70% ethanol. Proteins were digested overnight at room temperature with 0.4 µg of sequencing grade modified trypsin (Promega, #V5111) in 40 µl Hepes/NaOH, pH 8.4 in the presence of 1.25 mM TCEP and 5 mM chloroacetamide (Sigma-Aldrich, #C0267). Beads were separated, washed with 10 µl of an aqueous solution of 2% DMSO and the combined eluates were dried down. Peptides were reconstituted in 10 µl of H2O and reacted for 1 h at room temperature with 80 µg of TMT6plex (Thermo Scientific, #90061) (Werner et al., 2014) label reagent dissolved in 4 µl of acetonitrile. Excess TMT reagent was quenched by the addition of 4 µl of an aqueous 5% hydroxylamine solution (Sigma, 438227). Peptides were reconstituted in 0.1% formic acid, mixed to achieve a 1:1 ratio across all TMT-channels and purified by a reverse phase clean-up step (OASIS HLB 96-well µElution Plate, Waters #186001828BA). Peptides analyzed by LC-MS/MS on an Orbitrap Fusion Lumos mass spectrometer (Thermo Scentific). To this end, peptides were separated using an Ultimate 3000 nano RSLC system (Dionex) equipped with a trapping cartridge (Precolumn C18 PepMap100, 5 mm, 300 μm i.d., 5 μm, 100 Å) and an analytical column (Acclaim PepMap 100. 75 × 50 cm C18, 3 mm, 100 Å) connected to a nanospray-Flex ion source. The peptides were loaded onto the trap column at 30 µl per min using solvent A (0.1% formic acid) and eluted using a gradient from 2 to 80% Solvent B (0.1% formic acid in acetonitrile) over 90 min at 0.3 µl per min (all solvents were of LC-MS grade). The Orbitrap Fusion Lumos was operated in positive ion mode with a spray voltage of 2.4 kV and capillary temperature of 275°C. Full scan MS spectra with a mass range of 375–1500 m/z were acquired in profile mode using a resolution of 60,000 (maximum fill time of 50 ms or a maximum of 2e5 ions (AGC) and a RF lens setting of 30%. Fragmentation was triggered for 3 s cycle time for peptide like features with charge states of 2–7 on the MS scan (data-dependent acquisition). Precursors were isolated using the quadrupole with a window of 0.7 m/z and fragmented with a normalized collision energy of 36. Fragment mass spectra were acquired in profile mode and a resolution of 15,000 in profile mode. Maximum fill time was set to 54 ms. The dynamic exclusion was set to 60 s.
Acquired data were analyzed using IsobarQuant (Franken et al., 2015) and Mascot V2.4 (Matrix Science) using a reverse OvisOme database including common contaminants. The following modifications were taken into account: Carbamidomethyl (C, fixed), TMT10plex (K, fixed), Acetyl (N-term, variable), Oxidation (M, variable) and TMT10plex (N-term, variable). The mass error tolerance for full scan MS spectra was set to 10 ppm and for MS/MS spectra to 0.02 Da. A maximum of 2 missed cleavages were allowed. A minimum of 2 unique peptides with a peptide length of at least seven amino acids and a false discovery rate (FDR) below 10% were required on the peptide and protein level (Franken et al., 2015).
Data analysis
The raw output files of IsobarQuant (protein.txt – files) were processed using the R programming language (ISBN 3-900051-07-0). Only proteins that were quantified with at least two unique peptides were considered for the analysis. Seventy-nine proteins passed the quality control filters. Raw TMT reporter ion intensities (signal_sum columns) were first cleaned for batch effects using limma (Ritchie et al., 2015) and further normalized using vsn (variance stabilization normalization; Huber et al., 2002). Proteins were tested for differential expression using the limma package. The replicate information was added as a factor in the design matrix given as an argument to the ‘lmFit’ function of limma. A protein was annotated as a hit with an FDR below 5% and a fold-change of at least 100% and as a candidate with an FDR below 20% and a fold-change of at least 50%.
Results and discussion
Development of two in vitro models for intramammary infection
For the 2D model, the cells were propagated directly on cell culture dishes for up to 8 passages. The cells had a typical morphology of primary epithelial cells (Figure 1A) and a duplication time of around 5 days. For the 3D model, the primary cells were grown on a 3D according to the manufacturer’s instructions. Figures 1B–D, shows a cross section of the 3D culture stained with hematoxylin and eosin, where cells have colonized all spaces of the scaffold forming a 3D structure, recapitulating characteristics of connective tissue.
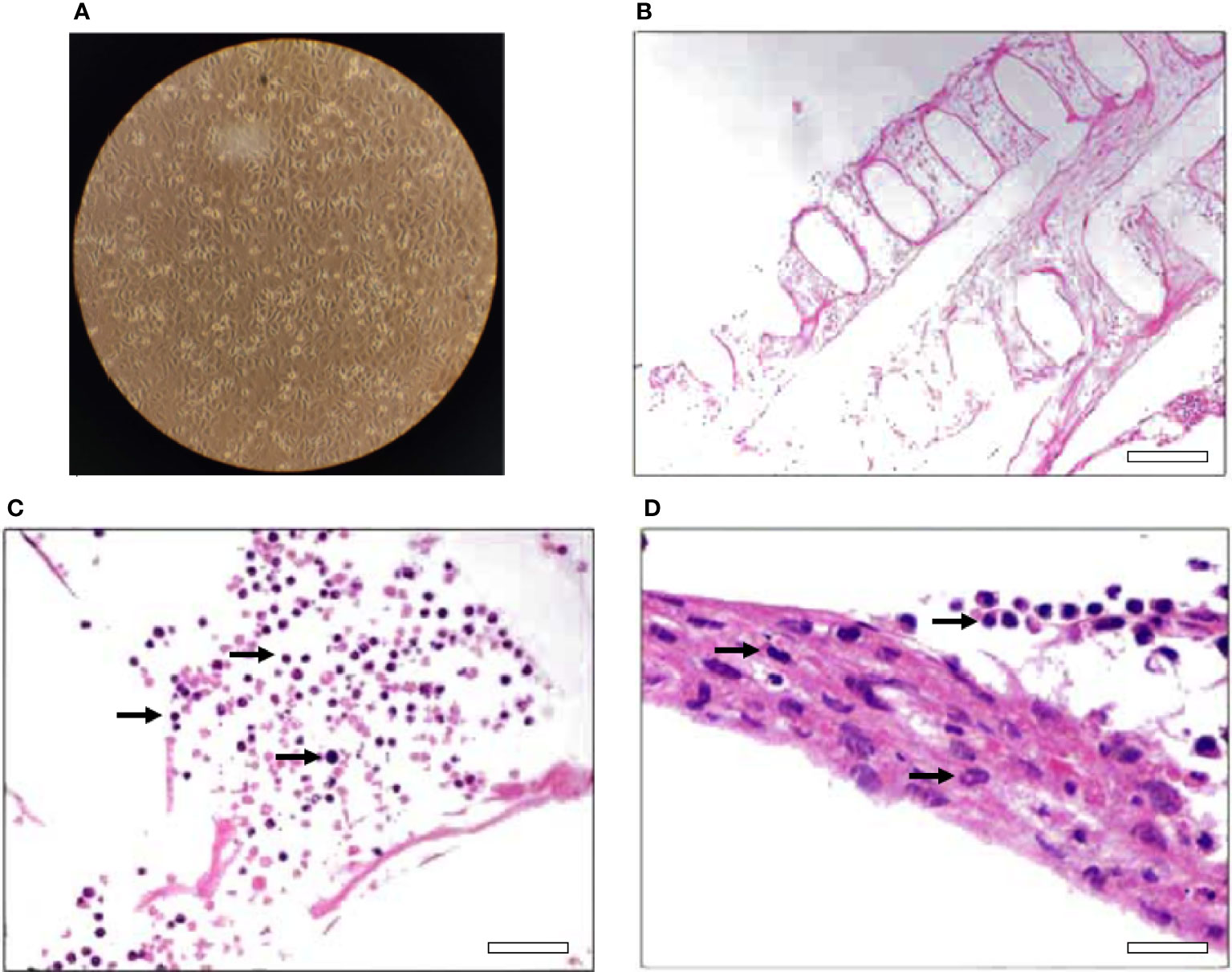
Figure 1 2D and 3D cell culture models for the ovine mammary tissue. (A) 2D culture of primary cells depicting characteristic monolayer formation of cells, (B) Hematoxylin-eosin-stained slice of the 3D culture of primary cells depicting the extracellular matrix, objective lens ×20, scale bar—100 μm and (C, D) separate sections of hematoxylin-eosin-stained slices of the 3D culture of the primary cells depicting the cells (pointed randomly with arrows), objective lens ×40, scale bars—50 and 25 μm respectively (D was further magnified 2X).
After the 2D and 3D cell cultures were established, both cultures were subjected to infection by two different mastitis-inducing pathogens, Escherichia coli and Staphylococcus aureus, for mimicking intramammary infections leading to mastitis. These two pathogens were chosen because a) they are commonly related with mastitis in dairy animals (Contreras and Rodríguez, 2011; Arteche-Villasol et al., 2022) and b) they show differences in clinical manifestations which may have a basis on different mechanisms of infection establishment (Persson-Waller et al., 1997; Lammers et al., 2001; Bannerman et al., 2004). Subclinical and clinical mastitis can be caused by rather the same species of bacterial pathogens and thus in order to mimic the early steps of intramammary infection that could lead to SCM in the subsequent experiments, the ratio of bacteria to mammary cells that was chosen was the lowest one that could result in bacterial invasion, as described in materials and methods.
Identification of secreted proteins following in vitro infection
After the establishment of the two ovine mammary gland models and the optimization of the step regarding the infection with mastitis-inducing pathogens, the cultures were employed for the identification of secreted proteins by mass-spectrometry. Thus, for both models, cell cultures were mock-treated or infected with either E. coli or S. aureus, incubated for 3 hours at 37°C and the secreted to the medium proteins were collected and analyzed by mass-spectrometry. The proteomics analysis, after filtering the peptides as described in the “materials and methods”, revealed 79 proteins secreted by the bovine cultured cells.
From an overrepresentation analysis of the GOCC terms on the 79 identified proteins, it was found that some of the most significant terms are related with secreted proteins, like “extracellular region” and “external encapsulating structure” (Figure 2A). These secretion-related terms validate the applicability of our strategy to study the ovine mammary secretome. Furthermore, the enrichment of such terms indicates a reduced possibility that the identified proteins originate from unwanted sources, e.g., cytosolic proteins leakage because of cellular degradation. When an overrepresentation analysis for Reactome terms was done, among the top term hits, several hits related to “Immune system” appeared (Figure 2B). This indicates that the two cell cultures models responded to the infection by pathogen cultures applied in our study.
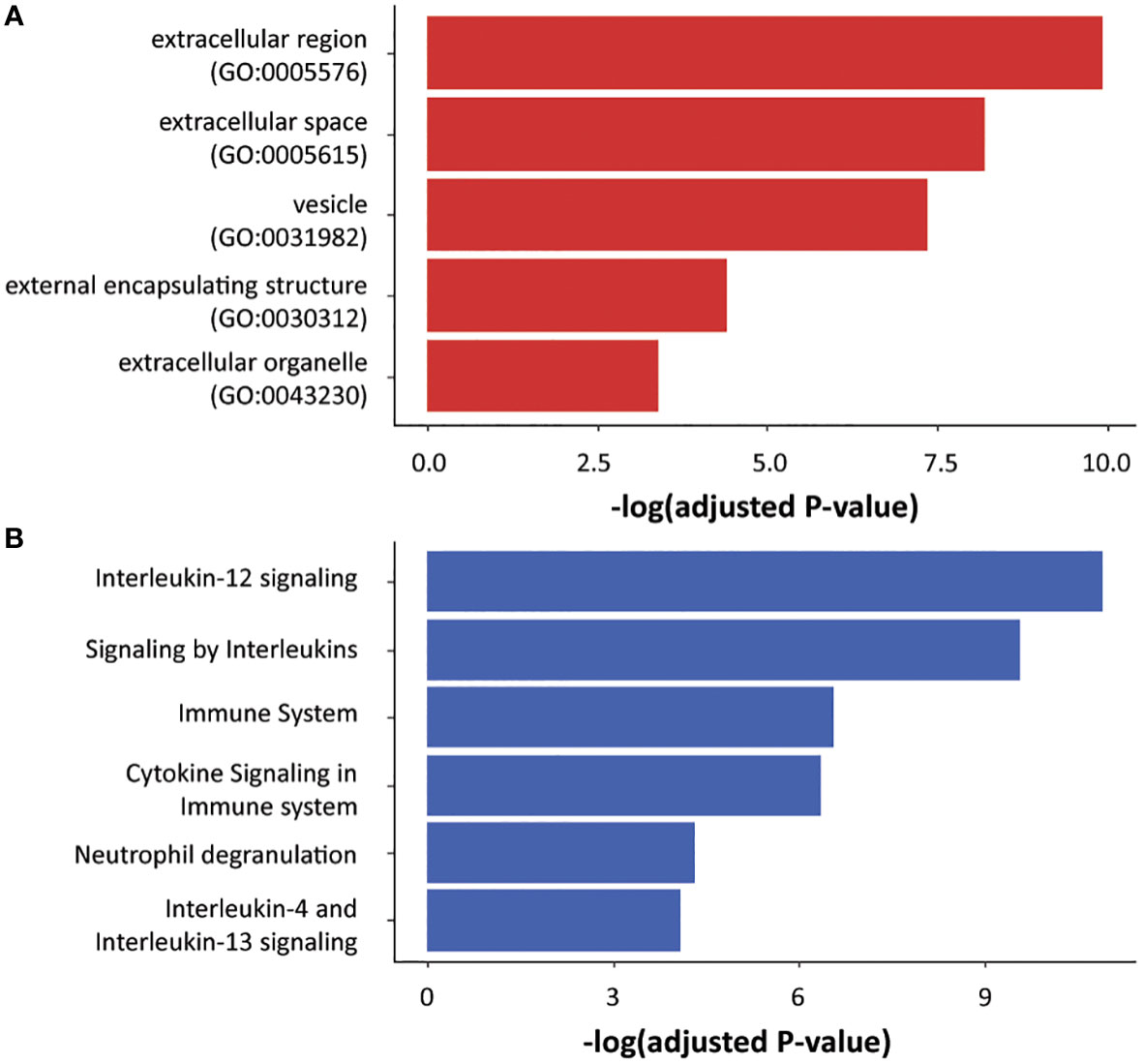
Figure 2 Ontology terms enrichment for the identified proteins. The 79 proteins identified by mass-spectrometry were analyzed for overrepresented GOCC (A) and Reactome (B) terms. The significant terms that are associated with secretion and immune system are shown.
To further test the validity of the two models, the identified proteins list was compared with three lists found in the literature which have resulted by proteomics analyses in milk. In these studies proteomic analysis of colostrum or milk from healthy animals or milk from animals with mastitis was performed (Addis et al., 2013; Scumaci et al., 2015; Cunsolo et al., 2017). Almost two thirds of the identified proteins in the present study were also identified as secreted proteins in ovine milk in the abovementioned studies, supporting our cell culture approach for modelling the secreting function of the ovine udder.
The 2D and 3D models were tested for any bias in terms of the identified proteins and protein abundances. First, it was assessed whether the two models demonstrate protein-specific performance. In Figure 3A the signal of the 79 proteins for both models is plotted; all of the 79 proteins were identified and most of them were comparably quantified by both models. Next it was checked whether the two models show any systematic bias for protein abundancy. In Figure 3B the ratio of the signal between the two models was plotted against the total signal for all identified proteins. As it is shown in the figure, both models are proper for identifying a wide range from less abundant to more abundant proteins. Finally, the efficacy of the two models was checked in terms of identifying secreted proteins under physiological or pathological conditions. Thus, the up-regulated proteins in the 2D and the up-regulated in the 3D models, as shown in Figure 3B, were checked for their presence in proteomics studies implying milk of healthy animals (Cunsolo et al., 2017; Zhang et al., 2020) or animals with mastitis (Addis et al., 2013; Chiaradia et al., 2013). From the data shown in Figure 3C, it is suggested that proteins identified by the 2D model match up better to proteins found to be secreted in mastitic milk, while proteins identified by the 3D model to proteins found to be present in milk from healthy ewes. This result may reflect the fact that the MOI was optimized based on the 2D culture and thus may not be optimal for the 3D culture conditions. It should be noted that what is shown in Figure 3C is rather a first observation, while extra validating experiments are needed for safe conclusions.
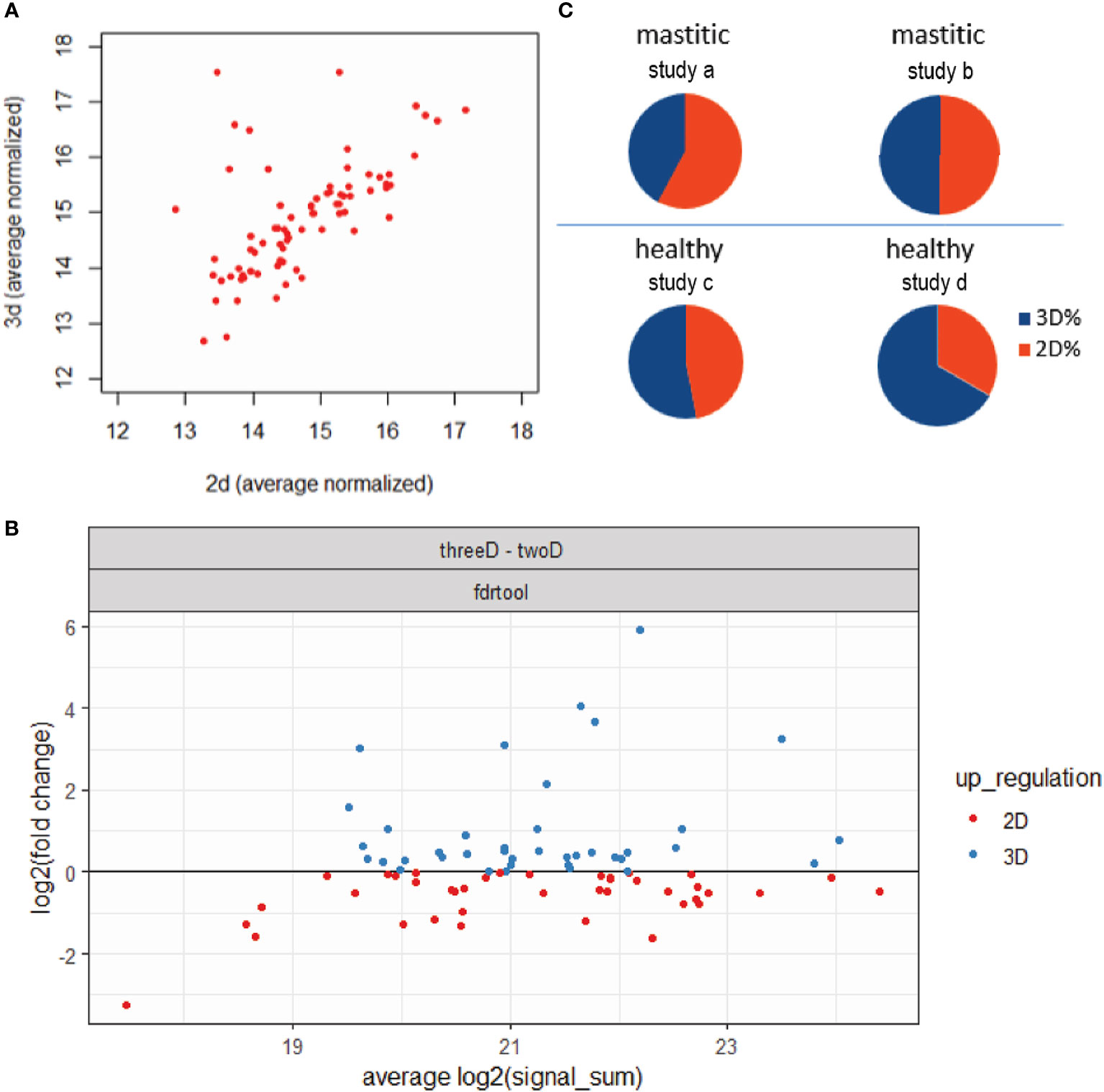
Figure 3 Comparison of the proteins identified by the 2D and 3D models. (A) Normalized signal for the 79 identified proteins for both models. (B) Fold-change of the signal of the 79 identified proteins between the two models against the total signal of each protein. With red are shown the proteins with higher signal in the 2D model and with blue the proteins with higher signal in the 3D model. (C) Ratios of common proteins between 2D and 3D overrepresented proteins and two studies regarding healthy milk from (Cunsolo et al., 2017;study a) and (Zhang et al., 2020;study b) or two studies regarding mastitic milk from (Addis et al., 2013; study c) and (Chiaradia et al., 2013; study d).
Identification of potential markers related to early intramammary infection
Since one of the most interesting outcomes of modelling mastitis would be the identification of potential mastitis markers, an analysis followed in order to identify significantly overexpressed proteins in the conditions mimicking the early stages of infection. Specifically, the experimental conditions were grouped into three categories: non-infected (or control), E. coli-infected (or E. Coli) and S. aureus-infected (or S. aureus). Following the analysis pipeline described in materials and methods adjusted to the identification of the overrepresented secreted proteins in the “E. Coli” and “S. Aureus” over the “control”, several proteins were found to be significant for “E. coli” but none was found to be significant for “S. Aureus” (Figure 4). The overrepresented proteins were characterized as “hit” or “candidate” according to what is described in materials and methods section.
Heat Shock Protein 90 Alpha Family Class B Member 1 (HSP90AB1) was one of the hit proteins. This is a ubiquitous protein with chaperone activity and given the great number of proteins it binds to, HSP90AB1 is considered to have a universal impact on a cell’s biological processes (Haase and Fitze, 2016). The identification of HSP90AB1 using the methods developed here is feasible as it has been found among the secreted proteins that are components of extracellular vesicles (Hoshino et al., 2020). Furthermore, HSP90AB1 has been identified as a component protein in the whey of several species besides sheep, (Johnston et al., 2018; Zhang et al., 2019) and has been reported among the identified proteins in proteomics studies of ovine milk (Addis et al., 2013; Pisanu et al., 2015; Zhang et al., 2020). Regarding its levels in mastitic conditions, there are two studies that report its transcription in bovine mammary gland to be upregulated after infection by E. coli (Chen et al., 2015), and a proteomics study that reports it to be elevated in milk from goats with mastitis (Pisanu et al., 2020b). Taken together, the above support that HSP90AB1 is a good candidate marker for mastitis.
LIM and SH3 Protein 1 (LASP1) was also identified as a hit protein. LASP1 was originally identified in human breast cancer and is implicated in several cellular processes with cell signaling being among them (Butt and Raman, 2018). Given that LASP1 is associated with secreted vesicles (Butt and Raman, 2018), it is expected to be identified with the current methodology. Indeed, it has also been identified before in proteomic analysis of ovine milk fat globules (Addis et al., 2013). To the best of our knowledge, there are no data associating LASP1 with mastitis infection and this renders the protein as an interesting novel candidate marker for mastitis.
Among the identified proteins that were characterized as candidates, CFL1 (Cofilin 1) is a protein that binds to actin and has a crucial role in actin-based cell motility as well as in a number of other processes, including stress response (Kanellos and Frame, 2016). Given its roles at the cell periphery (Kanellos and Frame, 2016), CFL1 is expected to be present in the secretome of a body fluid, like milk. It has already been suggested as a cancer marker in other kinds of body fluid samples (sputum) (Rangel et al., 2018). Furthermore, CFL1 has been identified by mass-spectrometry analysis in several studies in both ovine milk and colostrum (Addis et al., 2013; Pisanu et al., 2015; Scumaci et al., 2015; Cunsolo et al., 2017; Zhang et al., 2020) and has been reported to be increased in several proteomic investigations of mastitis in cattle (De Almeida, 2018).
One more “candidate” hit is W5NR06, which corresponds to the ovine predicted Histone 2B (H2B). The extracellular histones have been suggested as markers of pathological situations and diseases, including inflammatory diseases (Chen et al., 2014). H2B has been suggested as a mastitis marker in milk (Pisanu et al., 2015) and has been found upregulated in proteomics studies in milk of ewes with subclinical mastitis (Chiaradia et al., 2013). Furthermore, H2B has been found upregulated in a proteomics study on milk from goats with mastitis (Pisanu et al., 2020a), while histones in general are found upregulated in bovine mastitic milk in several studies (De Almeida, 2018).
PLS3 (Plastin 3), which was also found among candidates, is implicated in cross-linking of actin filaments into bundles. Besides this, PLS3 has been reported to take part in several other processes, one of them regarding the cell’s reaction upon the entrance of pathogens (Wolff et al., 2021). PLS3 has also been found upregulated in proteomics studies on milk from both sheep (Addis et al., 2013) and goats with intramammary bacterial infections (Pisanu et al., 2020a).
Another candidate hit was EEF1G (Elongation factor 1 G), which is involved in the translation of proteins. It is also a protein which is expected to be found with the strategy followed here since it has been identified by proteomics analysis in milk of sheep (Addis et al., 2013; Pisanu et al., 2015; Cunsolo et al., 2017; Zhang et al., 2020) and colostrum and milk of goats (Sun et al., 2020).
The last candidate protein was W5P9L9 or HNRNPK (Heterogeneous nuclear ribonucleoprotein K) a DNA/RNA binding protein known for its role in transcription and maturation of RNAs. It also takes part in other processes like translation and cell signaling (Sun et al., 2020). HNRNPK has been identified by proteomics as a protein of ovine milk samples (Addis et al., 2013) and, furthermore, has been found upregulated in mastitic ovine milk (Pisanu et al., 2015).
As already mentioned, the models developed here did not result in any significantly increased proteins in the secretome of the cells infected with S. aureus (Figure 4). However, almost all proteins that are significantly up- or down-regulated in the secretome of the cells infected with E. coli, show a tendency in change of their expression levels in the same direction in the S. aureus infected cell secretome (Figure 5). It is possible that the approaches presented here and under the specific experimental conditions that were used, do not discriminate between pathogens but rather mimic the response of the mammary tissue to pathogens. In this context, the lack of significance in the S. aureus model may just reflect a low pathogens:mammary cells ratio that could possibly be insufficient to trigger statistically significant changes under the conditions used here. Of course, the hypothesis that the absence of any significant hits only for S. aureus could reflect between-pathogens differences regarding the establishment of infection and the responses that they trigger cannot be rejected (Persson-Waller et al., 1997; Lammers et al., 2001; Bannerman et al., 2004).
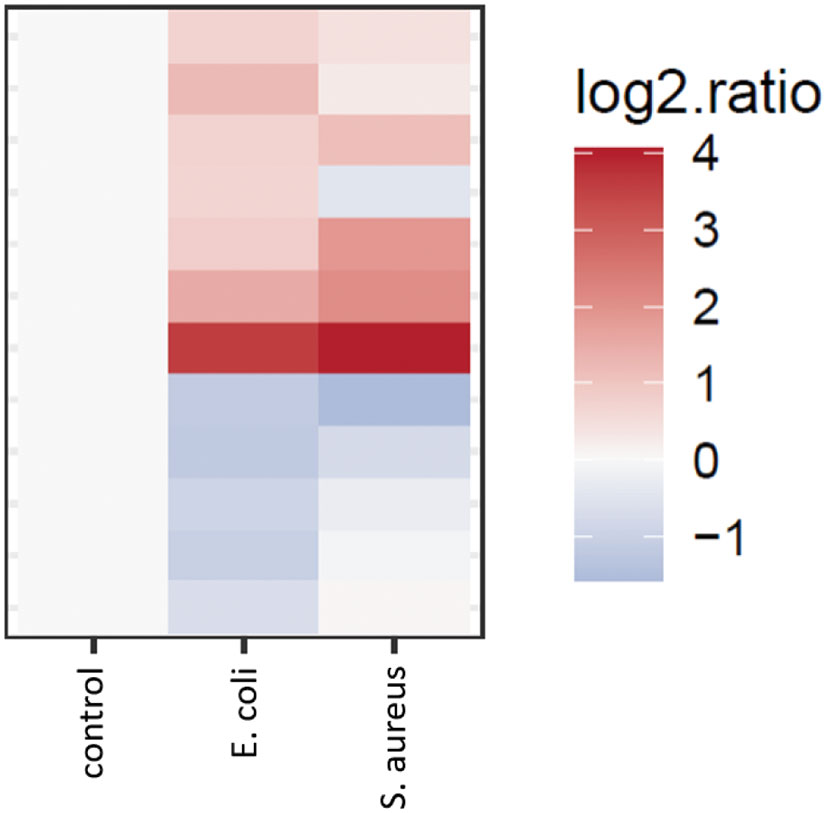
Figure 5 Heatmap of the signal of proteins over- and under-represented in the E. coli condition versus control, shown for both “E. coli vs control” and “S. aureus vs control” comparisons.
In total, from the seven candidate markers presented here, only 3 have already been documented to be upregulated in mastitic milk in other proteomics-based published studies. One could inquire as to the reasons that the relatively short list of candidate markers that resulted from the current procedure is not totally included in the longer already published lists. This partial overlap could be considered as an interesting advantage of our procedure since it: a) validates the results of our approach and b) makes possible the detection of some novel mastitis-related proteins. Concerning the possible reasons driven the partial overlap, two major differences should be pointed out between our approach and the approaches that have been followed in the literature. First, in the present study an LC-MS/MS was followed in the whole secretome, while in the already published studies only a fraction of the milk (Pisanu et al., 2015) or a set of proteins after 2D electrophoresis (Addis et al., 2013; Chiaradia et al., 2013; Katsafadou et al., 2019) were analyzed. Secondly, in our approach the “noise” or confounding effect of the proteins that are found abundant in milk (even in its fractions) is totally removed, leaving space for other, less abundant proteins to be identified. Lastly it should be emphasized that our approach focuses on the first stages of intramammary infection.
Conclusion
Two versions of an in vitro approach were tested for modeling the early stages of intramammary infection by mastitis-inducing pathogens in sheep. Both approaches enabled the identification by MS of several secreted proteins, rendering them as basis for the development of more efficient and effective models. The two models enabled the identification of a considerable fraction of already known protein components of milk produced under physiological or pathological (i.e., mastitic) conditions. The main advantage of the methodology presented here compared to in vivo approaches is the compliance with the welfare principles of farm animals, since infecting ewes with mastitis-inducing pathogens is avoided. Furthermore, the presented approaches are easier, quicker, and cheaper without needing access and handling of milking animals. We also show that the in vitro strategies described here enabled the identification of a set of 7 proteins related to early stages of intramammary infection, with 3 of them having already been described in the literature as upregulated proteins in mastitic milk and the rest 4 being novel protein hits. This is an interesting outcome towards finding novel mastitis markers that could enable the development of tests used directly on ovine milk by non-specialized staff. Advantages of our approach specifically linked with the identification of new mastitis markers include a) the absence of the abundant proteins of milk, that allows the identification of less abundant proteins without the need of the demanding and costly mass-spectrometry technology and b) its potential to be applied for other pathogens, other stages of infection or other types of cells present in the mammary gland. To sum up, here we presented a promising approach to model the ovine mammary gland, focusing on the early step of infection, which can be further improved towards the development of more sophisticated in vitro models.
Data availability statement
The data presented in the study are deposited in the ProteomeXchange Consortium via the PRIDE partner repository, accession number PXD036985.
Ethics statement
All experimental procedures were approved by the Ethics Research Committee of the Agricultural University of Athens, Greece (34/05.07.2019) and performed in accordance with the animal welfare and ethics guidelines.
Author contributions
GT, DV and IPo conceived the original idea. GT, ED, DV and IPa conceived and planned the experiments. AIG and AK isolated the mammary tissue that was used in experiments. DV and ED performed the cell culture of ovine mammary cells. ED and IPa performed the bacterial invasion assays. GCS contributed the in silico analysis of proteomics data. ED, DV and IPa wrote the manuscript. GT supervised the project and edited the final version of the manuscript. IPo helped supervise the project. All authors provided critical feedback and helped shape the research, analysis and manuscript. All authors contributed to the article and approved the submitted version.
Funding
This research was co-financed by Greece and the European Union (European Social Fund-ESF) through the operational Programme ‘Human Resources Development, Education and Lifelong Learning 2014-2020’, project code MIS 5048811.
Acknowledgments
We acknowledge Per Haberkant and Frank Stein from the proteomics core facility at the EMBL in Heidelberg for measuring our samples and helping with the data analysis and Prof. Theofilos Poutahidis from the Aristotle University of Thessaloniki for Histology methods and analysis.
Conflict of interest
The authors declare that the research was conducted in the absence of any commercial or financial relationships that could be construed as a potential conflict of interest.
Publisher’s note
All claims expressed in this article are solely those of the authors and do not necessarily represent those of their affiliated organizations, or those of the publisher, the editors and the reviewers. Any product that may be evaluated in this article, or claim that may be made by its manufacturer, is not guaranteed or endorsed by the publisher.
References
Addis M. F., Pisanu S., Marogna G., Cubeddu T., Pagnozzi D., Cacciotto C., et al. (2013). Production and release of antimicrobial and immune defense proteins by mammary epithelial cells following streptococcus uberis infection of sheep. Infect. Immun. 81, 3182–3197. doi: 10.1128/IAI.00291-13
Ahmad G., Timms L. L., Morrical D. G., Brackelsberg P. O. (1992). Dynamics and significance of ovine subclinical intramammary infections and their effects on lamb performance. Sheep Res. J. 8, 25–29.
Arteche-Villasol N., Fernández M., Gutiérrez-Expósito D., Pérez V. (2022). Pathology of the mammary gland in sheep and goats. J. Comp. Pathol. 193, 37–49. doi: 10.1016/j.jcpa.2022.02.007
Bannerman D. D., Paape M. J., Lee J. W., Zhao X., Hope J. C., Rainard P. (2004). Escherichia coli and staphylococcus aureus elicit differential innate immune responses following intramammary infection. Clin. Diagn. Lab. Immunol. 11, 463–472. doi: 10.1128/CDLI.11.3.463-472.2004
Butt E., Raman D. (2018). New frontiers for the cytoskeletal protein LASP1. Front. Oncol. 8. doi: 10.3389/fonc.2018.00391
Chen X., Cheng Z., Zhang S., Werling D., Wathes D. C. (2015). Combining genome wide association studies and differential gene expression data analyses identifies candidate genes affecting mastitis caused by two different pathogens in the dairy cow. Open J. Anim. Sci. 05, 358–393. doi: 10.4236/ojas.2015.54040
Chen R., Kang R., Fan X. G., Tang D. (2014). Release and activity of histone in diseases. Cell Death Dis. 5, 1–9. doi: 10.1038/cddis.2014.337
Chiaradia E., Valiani A., Tartaglia M., Scoppetta F., Renzone G., Arena S., et al. (2013). Ovine subclinical mastitis: Proteomic analysis of whey and milk fat globules unveils putative diagnostic biomarkers in milk. J. Proteomics 83, 144–159. doi: 10.1016/j.jprot.2013.03.017
Contreras G. A., Rodríguez J. M. (2011). Mastitis: Comparative etiology and epidemiology. J. Mammary Gland Biol. Neoplasia 16, 339–356. doi: 10.1007/s10911-011-9234-0
Cunsolo V., Fasoli E., Di Francesco A., Saletti R., Muccilli V., Gallina S., et al. (2017). Polyphemus, Odysseus and the ovine milk proteome. J. Proteomics 152, 58–74. doi: 10.1016/j.jprot.2016.10.007
De Almeida A. M. (2018). Proteomics in domestic animals: from farm to systems biology (Cham, Switzerland: Springer International Publishing AG). doi: 10.1007/978-3-319-69682-9
Duval K., Grover H., Han L. H., Mou Y., Pegoraro A. F., Fredberg J., et al. (2022). Modeling physiological events in 2D vs 3D cell culture. Physiology (Bethesda) 32, 266–277. doi: 10.1152/physiol.00036.2016
Finot L., Chanat E., Dessauge F. (2021). Mammary gland 3D cell culture systems in farm animals. Vet. Res. 52, 1–10. doi: 10.1186/s13567-021-00947-5
Franken H., Mathieson T., Childs D., Sweetman G. M. A., Werner T., Tögel I., et al. (2015). Thermal proteome profiling for unbiased identification of direct and indirect drug targets using multiplexed quantitative mass spectrometry. Nat. Protoc. 10, 1567–1593. doi: 10.1038/nprot.2015.101
Fthenakis G. C., Jones J. E. T. (1990). The effect of experimentally induced subclinical mastitis on milk yield of ewes and on the growth of lambs. Br. Vet. J. 146, 43–49. doi: 10.1016/0007-1935(90)90075-E
Haase M., Fitze G. (2016). HSP90AB1: Helping the good and the bad. Gene 575, 171–186. doi: 10.1016/j.gene.2015.08.063
Hoshino A., Kim H. S., Bojmar L., Gyan K. E., Cioffi M., Hernandez J., et al. (2020). Extracellular vesicle and particle biomarkers define multiple human cancers. Cell 182, 1044–1061.e18. doi: 10.1016/j.cell.2020.07.009
Huber W., Von Heydebreck A., Sültmann H., Poustka A., Vingron M. (2002). Variance stabilization applied to microarray data calibration and to the quantification of differential expression. Bioinformatics 18, S96–S104. doi: 10.1093/bioinformatics/18.suppl_1.S96
Johnston D., Malo Estepa I., Ebhardt H. A., Crowe M. A., Diskin M. G. (2018). Differences in the bovine milk whey proteome between early pregnancy and the estrous cycle. Theriogenology 114, 301–307. doi: 10.1016/j.theriogenology.2018.04.008
Kanellos G., Frame M. C. (2016). Cellular functions of the ADF/cofilin family at a glance. J. Cell Sci. 129, 3211–3218. doi: 10.1242/jcs.187849
Katsafadou A. I., Tsangaris G. T., Anagnostopoulos A. K., Billinis C., Barbagianni M. S., Vasileiou N. G. C., et al. (2019). Differential quantitative proteomics study of experimental mannheimia haemolytica mastitis in sheep. J. Proteomics 205, 103393. doi: 10.1016/j.jprot.2019.103393
Knuth R. M., Stewart W. C., Taylor J. B., Yeoman C. J., Bisha B., Page C. M., et al. (2019). Subclinical mastitis in sheep: etiology and association with milk somatic cell count and ewe productivity in three research flocks in the Western united states. Transl. Anim. Sci. 3, 1739–1743. doi: 10.1093/TAS/TXZ078
Lammers A., Van Vorstenbosch C. J., Erkens J. H. F., Smith H. E. (2001). The major bovine mastitis pathogens have different cell tropisms in cultures of bovine mammary gland cells. Vet. Microbiol. 80, 255–265. doi: 10.1016/S0378-1135(01)00305-4
Libera K., Konieczny K., Grabska J., Smulski S., Szczerbal I., Szumacher-Strabel M., et al. (2021). Potential novel biomarkers for mastitis diagnosis in sheep. Animals 11, 1–15. doi: 10.3390/ani11102783
Lou Y. R., Leung A. W. (2018). Next generation organoids for biomedical research and applications. Biotechnol. Adv. 36, 132–149. doi: 10.1016/j.biotechadv.2017.10.005
Martí-De Olives A., Peris C., Molina M. P. (2020). Effect of subclinical mastitis on the yield and cheese-making properties of ewe’s milk. Small Rumin. Res. 184, 106044. doi: 10.1016/j.smallrumres.2019.106044
Mazinani M., Rude B. (2020). Population, world production and quality of sheep and goat products. Am. J. Anim. Vet. Sci. 15, 291–299. doi: 10.3844/ajavsp.2020.291.299
Menzies P. I., Ramanoon ,. S. Z. (2001). Mastitis of sheep and goats. Vet. Clin. North Am. Food Anim. Pract. 17, 333–358. doi: 10.1016/S0749-0720(15)30032-3
Moggridge S., Sorensen P. H., Morin G. B., Hughes C. S. (2018). Extending the compatibility of the SP3 paramagnetic bead processing approach for proteomics. J. Proteome Res. 17, 1730–1740. doi: 10.1021/acs.jproteome.7b00913
Mohan S. C., Lee T. Y., Giuliano A. E., Cui X. (2021). Current status of breast organoid models. Front. Bioeng. Biotechnol. 9. doi: 10.3389/fbioe.2021.745943
Murphy T. W., Stewart W. C., Taylor J. B. (2018). Factors affecting ewe somatic cell count and its relationship with lamb weaning weight in extensively managed flocks. Transl. Anim. Sci. 2, S159–S162. doi: 10.1093/TAS/TXY031
Persson-Waller K., Colditz I. G., Seow H. F. (1997). Accumulation of leucocytes and cytokines in the lactating ovine udder during mastitis due to staphylococcus aureus and escherichia coli. Res. Vet. Sci. 62, 63–66. doi: 10.1016/S0034-5288(97)90182-X
Pisanu S., Cacciotto C., Pagnozzi D., Uzzau S., Pollera C., Penati M., et al. (2020a). Impact of staphylococcus aureus infection on the late lactation goat milk proteome: New perspectives for monitoring and understanding mastitis in dairy goats. J. Proteomics 221, 103763. doi: 10.1016/j.jprot.2020.103763
Pisanu S., Cacciotto C., Pagnozzi D., Uzzau S., Pollera C., Penati M., et al. (2020b). Proteomic datasets of uninfected and staphylococcus aureus-infected goat milk. Data Br. 30, 2–5. doi: 10.1016/j.dib.2020.105665
Pisanu S., Cubeddu T., Pagnozzi D., Rocca S., Cacciotto C., Alberti A., et al. (2015). Neutrophil extracellular traps in sheep mastitis. Vet. Res. 46, 59. doi: 10.1186/s13567-015-0196-x
Rangel M. P., Antonangelo L., Acencio M. M. P., Faria C. S., de Sá V. K., Leão P. S., et al. (2018). Detection of sputum cofilin-1 as indicator of malignancy. Braz. J. Med. Biol. Res. 51, 1–6. doi: 10.1590/1414-431x20187138
Ritchie M. E., Phipson B., Wu D., Hu Y., Law C. W., Shi W., et al. (2015). Limma powers differential expression analyses for RNA-sequencing and microarray studies. Nucleic Acids Res. 43, e47. doi: 10.1093/nar/gkv007
Roussel P., Porcherie A., Répérant-Ferter M., Cunha P., Gitton C., Rainard P., et al. (2017). Escherichia coli mastitis strains: In vitro phenotypes and severity of infection in vivo. PloS One 12, 1–20. doi: 10.1371/journal.pone.0178285
Scumaci D., Trimboli F., Dell’Aquila L., Concolino A., Pappaianni G., Tammè L., et al. (2015). Proteomics-driven analysis of ovine whey colostrum. PloS One 10, e0117433. doi: 10.1371/journal.pone.0117433
Sun Y., Wang C., Sun X., Jiang S., Guo M. (2020). Characterization of the milk fat globule membrane proteome in colostrum and mature milk of xinong saanen goats. J. Dairy Sci. 103, 3017–3024. doi: 10.3168/jds.2019-17739
Tvarožková K., Tančin V., Holko I., Uhrinčať M., Mačuhová L. (2019). Mastitis in ewes: Somatic cell counts, pathogens and antibiotic resistance. J. Microbiol. Biotechnol. Food Sci. 9, 661–670. doi: 10.15414/jmbfs.2019/20.9.3.661-670
Werner T., Sweetman G., Savitski M. F., Mathieson T., Bantscheff M., Savitski M. M. (2014). Ion coalescence of neutron encoded TMT 10-plex reporter ions. Anal. Chem. 86, 3594–3601. doi: 10.1021/ac500140s
Wolff L., Strathmann E. A., Müller I., Mählich D., Veltman C., Niehoff A., et al. (2021). Plastin 3 in health and disease: a matter of balance. Cell. Mol. Life Sci. 78, 5275–5301. doi: 10.1007/s00018-021-03843-5
Zhang X., Li F., Qin F., Li W., Yue X. (2020). Exploration of ovine milk whey proteome during postnatal development using an iTRAQ approach. PeerJ 8, 1–19. doi: 10.7717/peerj.10105
Keywords: cell culture models, ovine, in vitro infection, proteomics, intramammary infections
Citation: Dalaka E, Vassilakos D, Stefos GC, Kalogianni AI, Palamidi I, Gelasakis AI, Politis I and Theodorou G (2022) Utilization of 2D and 3D cell cultures for the modelling of intramammary infection in sheep. Front. Anim. Sci. 3:1015982. doi: 10.3389/fanim.2022.1015982
Received: 10 August 2022; Accepted: 25 October 2022;
Published: 11 November 2022.
Edited by:
Erin E. Connor, University of Delaware, United StatesReviewed by:
Kiran Ambatipudi, Indian Institute of Technology Roorkee, IndiaTheodore Elsasser, Independent Consultant, Berwyn Heights, United States
Copyright © 2022 Dalaka, Vassilakos, Stefos, Kalogianni, Palamidi, Gelasakis, Politis and Theodorou. This is an open-access article distributed under the terms of the Creative Commons Attribution License (CC BY). The use, distribution or reproduction in other forums is permitted, provided the original author(s) and the copyright owner(s) are credited and that the original publication in this journal is cited, in accordance with accepted academic practice. No use, distribution or reproduction is permitted which does not comply with these terms.
*Correspondence: Georgios Theodorou, Z3RoZW9kQGF1YS5ncg==