- 1Institute of Animal Breeding and Husbandry, Christian-Albrechts-University, Kiel, Germany
- 2Institute for Animal Hygiene, Animal Welfare and Farm Animal Behavior, University of Veterinary Medicine Hannover, Foundation, Hanover, Germany
Understanding behavior is important in terms of welfare assessments to be able to evaluate possible changes in behavior among different husbandry systems. The present study applied principal component analysis (PCA) to reveal relationships between behavioral indicators to identify the main components of sows' behavior promoting feasibility of welfare assessments by providing possibilities for variable reduction and aggregation. The indicators of the Welfare Quality® protocol's principle to assess behavior were repeatedly applied by two observers on 13 farms in Northern Germany. This included Qualitative Behavior Assessments (QBA) to evaluate animals' body language using 20 pre-defined adjectives, assessments of social and exploratory behavior, stereotypies, and human–animal relationship tests. Two separate PCA were performed with respect to the QBA: (1) adjectives were included as independent variables and (2) adjectives were pre-aggregated using the calculation rules of the Welfare Quality® protocol for fattening pigs since a calculation for sows does not yet exist. In both analyses, two components described sows' behavior. Most variance was explained by the solution with adjectives as independent variables (51.0%). Other behavioral elements not captured as indicators by the protocol may still be important for all-inclusive welfare assessments as the required variance of 70% was not achieved in the analyses. Component loadings were used to determine components' labels as (1) “satisfaction of exploratory behavior” and (2) “social resting”. Both components reflected characteristics of sows' natural behavior and can subsequently be used for variable reduction but also for development of component scores for aggregation. As defined for PCA, component 1 explained more variance than component 2. PCA is useful to determine the main components of sows' behavior, which can be used to enhance feasibility of welfare assessments.
Introduction
Animal welfare is generally defined by both physical and mental health (Dawkins, 2004; Webster et al., 2004) and, following the five freedoms published by the Farm Animal Welfare Council (FAWC), animal welfare involves the freedom from hunger and thirst, from discomfort, from pain, injury, and disease, from fear and distress and the freedom to express normal behavior (Farm Animal Welfare Council, 1993). Based on FAWC's definition, the Five Domains model was developed to determine the influence of experiments or their use on animal welfare. The Five Domains are subdivided into “nutrition,” “environment,” “health,” “behavior,” and “mental state” (Mellor and Reid, 1994). Growing public demand for improved welfare of farm animals has resulted in the need to develop valid, reliable, and practicable systems for the assessment of animal welfare (Webster, 2005). The most representative example to fulfill this need is the Welfare Quality® system. The Welfare Quality® protocols were developed between 2004 and 2009 by a research collaboration as part of an EU project and are intended to enable the scientifically based, standardized and objective measurement of animal welfare (Blokhuis et al., 2013). Within the protocols, the multidimensionality of animal welfare described above is reflected in four main principles assessing feeding, housing, health, and behavior. Independent but complementary criteria were chosen for each of these principles, as can be seen in Figure 1. These are measured using mainly animal-based indicators (Botreau et al., 2007). The Welfare Quality® protocol's behavior principle assesses animals' motivated behavior or the expression of species-specific behavior, respectively. Modifications in the behavioral patterns often represent an animal's first reaction to an aversive or stressful environment. Behaviors that deviate, for example, in frequency from those shown when an animal is able to perform its natural behavior are called “abnormal behaviors” (Fraser and Broom, 1990).
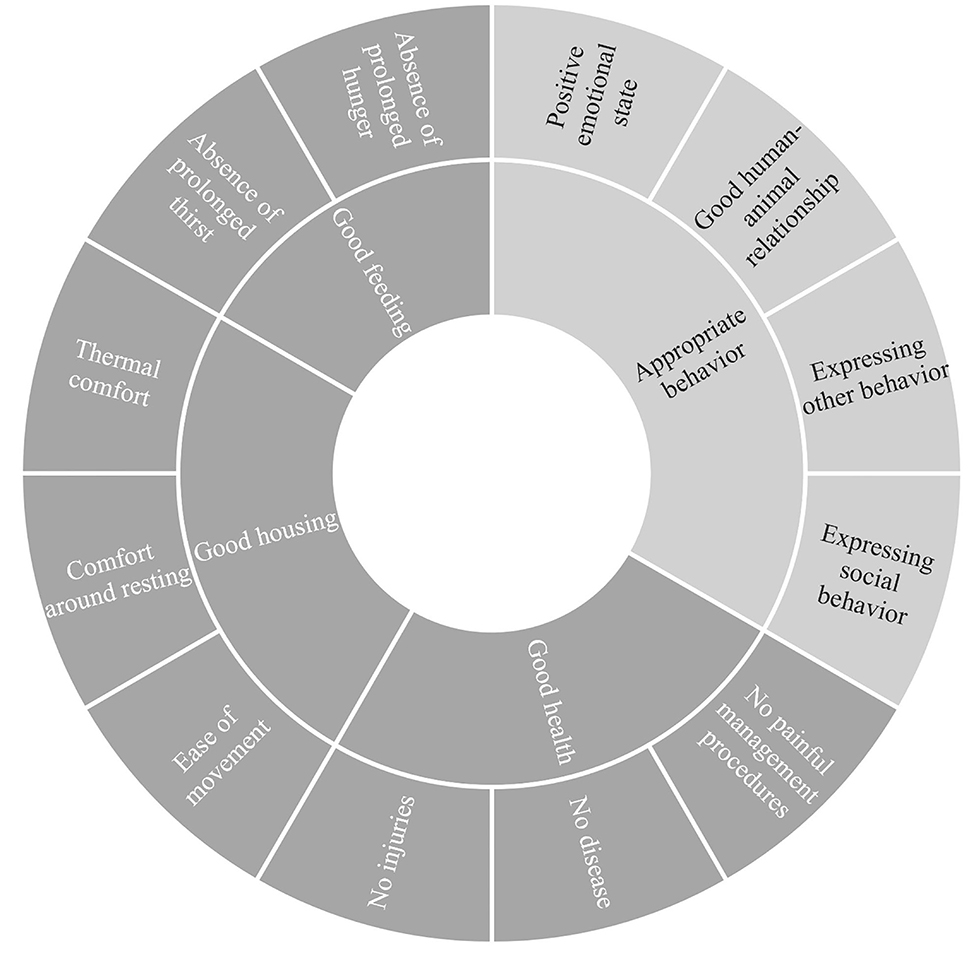
Figure 1. Overview of the four Welfare Quality® principles and its 12 criteria (modified after Blokhuis et al., 2013). The principle to assess behavior (“appropriate behavior”), which is the focus of the present study, is highlighted in light gray.
Even though the Welfare Quality® protocols are a representative example of an objective welfare assessment system, the protocols are commonly criticized for their lack of feasibility (Czycholl et al., 2016b; Friedrich et al., 2019b). In this context, the assessment of behavioral indicators in particular is said to be time-consuming (Rushen et al., 2012). However, Friedrich et al. (2020b) identified the assessment of stereotypies as an iceberg indicator for the assessment of welfare in sows. Iceberg indicators aggregate the information of several indicators in one indicator enhancing the feasibility of the assessment (Farm Animal Welfare Council, 2009). Consequently, the assessment of behavior is crucial for the assessment of welfare in sows based on the definitions of animal welfare (FAWC's five freedoms, Five Domains, Welfare Quality® principles).
In the present study, the behavioral indicators of the principle to assess behavior using the Welfare Quality® protocol for sows and piglets were applied to 13 farms and subsequently analyzed using principal component analysis (PCA). PCA is an investigative statistical method for identifying correlation structures among multiple variables and was introduced by Munsterhjelm et al. (2015) to aggregate the Welfare Quality® protocol's indicators to so-called main welfare issues. In doing so, no aggregation of behavioral indicators was performed (Munsterhjelm et al., 2015). Therefore, the present study is the first of its kind in which behavioral indicators were analyzed contextually.
The present study aimed at contributing to the feasibility of the assessment of behavior in terms of the Welfare Quality® protocol by identifying redundancies among the variables allowing the protocol to be subsequently shortened to enhance feasibility. Moreover, it also aimed at identifying the main components within the behavioral indicators of the principle to assess behavior using the Welfare Quality® protocol for sows and piglets. In comparison with the Welfare Quality® protocol, behavior is not sufficiently considered in other assessment systems for animal welfare (Friedrich et al., 2020a). It is therefore important to identify those behavioral indicators that are most important from a scientific point of view to assess behavior so that other assessment systems can be improved accordingly. Lastly, main components represent a first approach to developing an accessible overall score since there is no aggregation of the variables applied to sows in the Welfare Quality® protocol at present. In summary, the present study contributes to the further development of the Welfare Quality® protocol for sows and piglets by indicating which variables may be removed from the protocol to increase feasibility, and by providing a first approach for calculating an overall evaluation. Higher feasibility allows the protocol to be more widely applicable, e.g., for farmers, and overall evaluation may help to simplify the interpretation of results and allow easier comparisons between farms. The results can be consulted by other assessment systems for animal welfare as well. All this contributes to an increase in welfare in sows.
Materials and Methods
Data Collection
Behavioral data were collected by two observers on 13 farrowing farms in Northern Germany between September 2016 and April 2018 (Observer 1: female, aged 27, veterinarian; Observer 2: female, aged 25, student of agricultural sciences; both observers had experience in handling pigs and collecting data). One observer visited each farm five times (day 0, day 3, week 7, month 5, month 10). The monthly distribution of the visits on the farms during the data collection can be seen in Figure A of the Supplementary Material. Twenty random chosen visits were performed by the second observer. The second observer performed the evaluation on the same animals at the same time and under the same conditions but independently of the first observer. By spreading visits across all seasons and since animals rotated within the farms due to the production cycle or since there was a change in animals due to replacement, different animals were observed on each farm during each visit. Therefore, the resulting 85 farm visits were considered independent in the further analyses. The participating farms were selected to ensure an inter-farm variability as large as possible. The Chamber of Agriculture Schleswig Holstein helped in approaching the farms. However, the farms' participation was voluntary. The farms had different production types (conventional vs. organic), farm sizes (40–5,000 sows) and production rhythms (1-week−4-weeks rhythm) as can be seen in Table 1. All farms worked as a closed system.
The Welfare Quality® protocols are based on four main principles to assess feeding, housing, health, and behavior. This study aimed at identifying main components in the behavioral indicators and therefore focused on the principle to assess behavior in sows. Thus, for each farm visit, the observers applied the complete Welfare Quality® protocol for sows and piglets but only the behavioral indicators of the protocol's principle to assess behavior were of interest for the analyses. The observers were trained by experts of the Welfare Quality® consortium before starting data collection to ensure that the assessments complied with the protocol. The observers were re-trained and re-evaluated using pictures and videos midway through the data collection to prevent observer drift. The behavioral indicators at each farm visit included a Qualitative Behavior Assessment (QBA) that evaluated the positive affective state of the animals, an instantaneous scan sampling that measured social and exploratory behavior, the assessment of stereotypies and a human–animal relationship test. In short, the QBA comprised the evaluation of 20 adjectives (1: active; 2: relaxed; 3: fearful; 4: agitated; 5: calm; 6: content; 7: tense; 8: enjoying; 9: frustrated; 10: sociable; 11: bored; 12: playful; 13: positively occupied; 14: listless; 15: lively; 16: indifferent; 17: irritable; 18: aimless; 19: happy; 20: distressed). The expressive quality of the activities of the animals were observed within a given time of 20 min. Observation points were evenly spread across a farm to account for farm dynamics including rooms on different sides of the buildings and pens evenly distributed within a room, e.g., rooms from the north and the south side of a building and the first, one middle and the last pen in a room were assessed. At the end of the observation time of 20 min, the expression of all animals under observation was rated for each adjective on a visual analog scale from 0 (absent) to 125 mm (dominant) thereby summarizing the expressive quality of all animals' activities observed. Using five scans at 2 min intervals, the number of animals involved in positive and negative social behavior, discovering enrichment material, performing pen investigations or other active behaviors such as drinking, or walking was assessed in the instantaneous scan sampling. The assessment was limited to the gestation unit but different observation points were used to generate an overall picture of the sows' behavior. In addition, a random sample of sows in the gestation unit was observed for the presence of stereotypical behavior such as sham chewing or tongue rolling. A binary score (0 = absent, 1 = present) per animal was used for recording. Lastly, randomly sampled sows in the gestation unit were subjected to a human–animal relationship test. Here, a three-point scale (0 = no fear response, 1 = light fear response, 2 = strong fear response) was applied. A detailed description of the exact performance of these tests can be found in the protocol (Welfare Quality®, 2009). Moreover, further details on data collection/farm types are described in Friedrich et al. (2019a).
Data Processing and Statistical Analysis
Data processing and statistical analysis were performed using the statistical software SAS® 9.4 (SAS Institute Inc, 2008). All data were analyzed at farm visit level and therefore converted to percentage values. The analysis at farm visit level was conducted since the animals observed were part of a randomly chosen sample. In addition, different numbers of animals were observed in the QBA and the instantaneous scan sampling due to different group sizes on the different farms. For this, the mm scores of the QBA were divided by the total length of the scale (125 mm) to receive a comparable percentage value. The number of animals performing distinct behavior in the instantaneous scan sampling was divided by all animals under assessment showing active behavior to calculate the proportion of the total active behavior. The percentage of animals in a category during a farm visit was calculated for the assessment of stereotypies and the human–animal relationship test (e.g., farm 1, farm visit 1: sham chewing category 0: 90%; sham chewing category 1: 10%; sum = 100%). Further, the resulting variables were modified in such a way that the higher their value, the higher the animal welfare, otherwise the interpretation of opposite variables becomes difficult (O'Rourke and Hatcher, 2013). This means that in variables with more than one level of categorization as, for example, in the human–animal relationship test, only the category describing a high level of animal welfare (category 0, “no fear response”) was included in the analysis. Variables such as “frustrated” from the QBA were included in the analysis as 100—value of variable (“not frustrated”). At this point it should be noted that during the development of the Welfare Quality® protocols concerning the QBA, it was emphasized that not the different adjectives describe animal welfare but the pattern resulting from the combination of adjectives (Wemelsfelder and Millard, 2009). Therefore, the following PCA was carried out using two different approaches considering the QBA. First, the 20 adjectives were used as independent variables as just described, e.g., “tense,” “active”. Second, the QBA was pre-aggregated prior to the implementation of the PCA on sows' behavior. Therefore, the weights and calculation rules defined in the Welfare Quality® protocol for fattening pigs were applied since a calculation rule for sows does not yet exist (Welfare Quality®, 2009). The values obtained for each adjective were aggregated to a weighted sum applying the formula:
with Nk being the value recorded for a given adjective k in a farm visit and wk being the defined weights for a given adjective k. The weights can be consulted in the protocol itself (Welfare Quality®, 2009) or, for example, in Zhou et al. (2013). Lastly, a score for each farm visit was created from each weighted sum using I-spline function and the following rule: if weighted sum ≤ 0, then score = –(10 × weighted sum) – (1.25 × weighted sum2), if weighted sum > 0, then score = 50 + (11.667 × weighted sum) – (0.55556 × weighted sum2). For better understanding, the aggregated score of the QBA is still referred to as weighted sum in the following. Unlike the other values used in the PCA, the use of the calculation rules for fattening pigs did not result in a percentage value, but a weighted sum of the individual values of the adjectives for each farm visit; this was transformed to a score from 0 to 100. Consequently, the following PCA was applied to 30 variables when the QBA adjectives were used as independent variables. The number of variables was reduced to 11 when the QBA was pre-aggregated using weighted sums.
The dataset was subjected to PCA, which is used for the reduction of variables. PCA requires that several variables are correlated and measure the same construct, which is called redundancy among the variables. Because of this redundancy, it is assumed that the number of observed variables can be reduced to certain principal components, which continue to explain most of the variance in the dataset (O'Rourke and Hatcher, 2013).
The suitability of the dataset for PCA was first verified. A correlation matrix was calculated applying Spearman's rank correlation coefficient since the data were not normally distributed. The correlation matrix is included as Table A in the Supplementary Material. The minimal correlation of 0.30 was achieved for each variable (Hair et al., 1995). In addition, the Kaiser-Meyer-Olkin (KMO) measure of sampling adequacy (Kaiser, 1970; Kaiser and Rice, 1974) was calculated using the proc factor statement in SAS (in SAS: msa). The overall KMO measure achieved a value of 0.83 for the dataset using the QBA adjectives as independent variables and a value of 0.58 for the dataset when the QBA was pre-aggregated using weighted sums. In both cases, the values were larger than the required threshold of 0.50 (Hair et al., 1995) thereby confirming that the dataset was suitable for PCA.
PCA is conducted using a sequence of steps, which include somewhat subjective decisions. Therefore, the present study followed the steps presented by O'Rourke and Hatcher (2013) using the proc factor statement in SAS. Since the data were not normally distributed, the correlation matrix applying Spearman's rank correlation coefficient was the starting point for the analyses (input in SAS: type = corr). Prior communality estimates were set to ones (in SAS: priors = one) to create a PCA. The components were extracted by the principal axis method (in SAS: method = prin), which went along with a varimax (orthogonal) rotation (in SAS: rotate = varimax) resulting in uncorrelated components to ease the interpretation of the results. Each of the variables included in the PCA on sows' behavior received a loading between −1.00 and +1.00 on a component. Further analysis was performed again in accordance with O'Rourke and Hatcher (2013). Four criteria had to be fulfilled to determine a component as meaningful:
1. The eigenvalue, also known as Kaiser-Guttman rule (Kaiser, 1960, 1991), which displays the amount of variance mirrored by a given component, was >1.00.
2. In the scree test, which is the plot of eigenvalues associated with each component (Cattell, 1966), the component laid in front of the point of inflection.
3. The component accounted for at least 10% of variance in the dataset. In terms of cumulative variance, the extracted components accounted for at least 70% of the variance of the dataset.
Since it is known that the use of the Kaiser-Guttman rule and the scree plot may overestimate the number of components (Henson and Roberts, 2016), a parallel analysis to determine the number of components was also performed (Horn, 1965). Eigenvalues of random datasets based on the correlation matrix introduced above were calculated with 1,000 iterations and the medians of these simulated eigenvalues were compared to the actual eigenvalues. Components were retained if their actual eigenvalue was greater than the median of the simulated eigenvalue (Williams et al., 2010). Hence, if the actual eigenvalue was higher than the simulated eigenvalue, it can be assumed that the component was in fact underlying in the dataset and did not result from chance (Horn, 1965).
4. Lastly, the following interpretability criteria had to be met in the rotated factor pattern, which O'Rourke and Hatcher (2013) considered most important:
a) At least three variables with a significant loading belonged to a component. Literature claims that two variables are required for a component to be namable (Henson and Roberts, 2016).
b) O'Rourke and Hatcher (2013) suggested interpreting loadings as significant if they were greater than 0.40 or smaller than −0.40. However, due to the small sample size of the present study, loadings were only interpreted as significant if they were greater than 0.70 or smaller than −0.70 (Budaev, 2010).
c) The variables within a component shared the same construct. In contrast, variables of different components were based on different constructs.
d) The rotated factor pattern demonstrated “simple structure”, which means that variables that had high loadings on one component possessed loadings near zero on other components.
Finally, it was identified what the variables of a component had in common and an overall label was determined for the component (O'Rourke and Hatcher, 2013).
Results
Table 2 displays the minimum, maximum and median values recorded for the variables of the behavioral indicators of the 85 farm visits. The values of the adjectives of the QBA ranged from 0 to 100%. The occurrence of social behavior during the scan samplings was rare. Most animals that were not resting were sorted in the category “other active behavior” (e.g., walking). Sham chewing and tongue rolling were the stereotypies most frequently observed. The absence of fear responses again ranged from 0 to 100% so that in some farm visits all animals showed a fear response, in others none. Table 2 further includes the descriptive statistics for the pre-aggregated QBA using weighted sums.
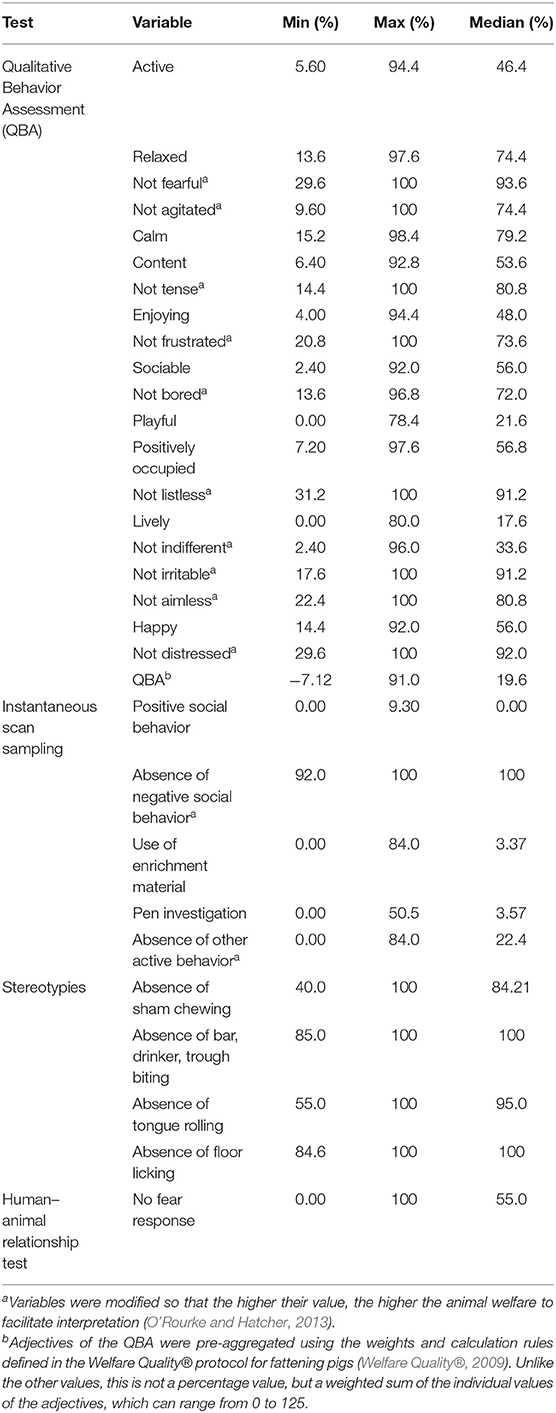
Table 2. Minimum, maximum and median values (%) of the variables included in the PCA on sows' behavior.
In the analysis of sows' behavior using the QBA adjectives as independent variables, seven components had an initial eigenvalue of greater than 1.00. The values ranged from 1.05 to 11.3. However, the scree test showed a point of inflection between components 4 and 5. Further, only the first three components accounted for more than or ~10% of variance. The parallel analysis confirmed these results. The actual and simulated eigenvalues can be examined in Table B of the Supplementary Material. In this regard, three components were used as the starting point of the PCA. The number of components was then reduced in order to obtain the best possible PCA for interpretation. The solution with three components did not present at least two significant loadings on each component, thus it did not comply with item 4a of the interpretability analysis explained above. In contrast, the solution using two components fulfilled item 4a of the interpretability analysis. The solution using two components showed variables of the same component measuring the same construct and variables loading on different components measuring different constructs and therefore complied with the “simple structure” as defined in item 4d of the interpretability analysis. The two components explained 51.0% of the dataset's variance (component 1: 37.6%, component 2: 13.5%). The whole PCA procedure was repeated with QBA aggregated applying weighted sums. Here, three components had an eigenvalue of greater than 1.00 (range 1.26–3.63) in the first analysis. These three components explained more than or ~10% of variance each. A point of flexion between components 3 and 4 in the scree test confirmed these findings. Parallel analysis suggested two components as underlying in the dataset beyond chance (Table B in Supplementary Material). Having a higher threshold of 0.70, the solution using two components did not completely fulfill item 4b of the above defined interpretability analysis. However, the loadings were close enough to 0.70 to be interpreted as significant. The remaining two components of this approach explained 48.3% of the dataset's variance (component 1: 33.0%, component 2: 15.3%). The variables' loadings of both approaches can be seen in Table 3.
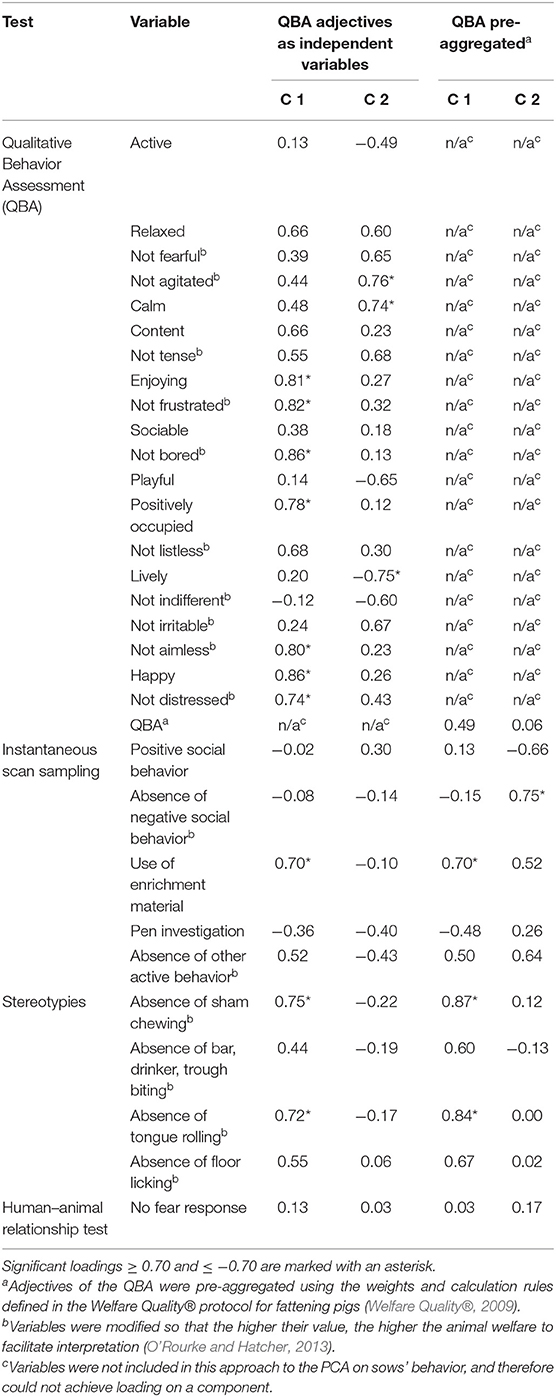
Table 3. Loadings on detected components 1 and 2 (C1, C2) of the variables of the behavioral indicators in the rotated factor pattern of the PCA on sows' behavior.
Using the QBA adjectives as independent variables, component 1 was characterized by positive loadings of the variables absence of sham chewing, absence of tongue rolling and use of enrichment material. Adjectives such as “enjoying” or “not frustrated” loaded positively on component 1 as well. Component 2 contained positive loadings of the adjectives “not agitated”, “calm” and “not lively”. The results remained similar when the QBA was pre-aggregated to a weighted sum. Here, component 1 was characterized by positive loadings of the variables applied to assess stereotypies and the use of enrichment material. Component 2 was described by negative loadings of positive social behavior and positive loadings of absence of negative social behavior and absence of other active behavior. To better illustrate these relationships, the component loadings achieved by the variables of the behavioral indicators in the rotated factor pattern of the PCA on sows' behavior are shown in graphical form in Figure 2.
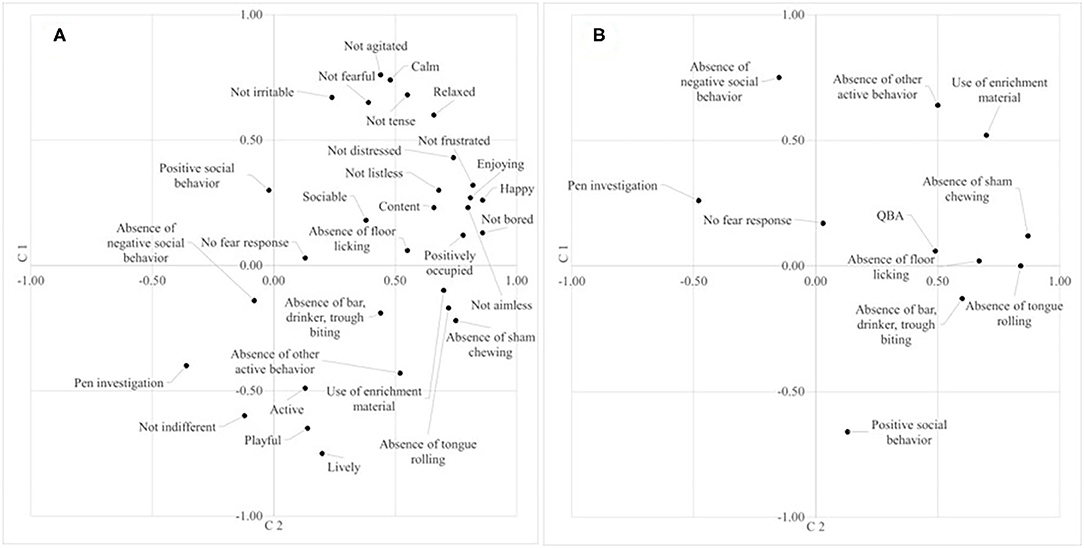
Figure 2. Loadings on detected components 1 and 2 (C1, C2) of the variables of the behavioral indicators in the rotated factor pattern of the PCA on sows' behavior, compromising (A) solution with adjectives of the Qualitative Behavior Assessment (QBA) as independent variables, (B) solution with adjectives of the QBA pre-aggregated using the weights and calculation rules defined in the Welfare Quality® protocol for fattening pigs (Welfare Quality®, 2009).
Discussion
In their study on main welfare issues, Munsterhjelm et al. (2015) indicated that when determining principal components, the biological plausibility of the constructs according to scientific knowledge, but also common sense, should be taken into account. Hence, the results of the present study are discussed in comparison to current literature to ensure the plausibility of the components.
Two principal components could be revealed when the adjectives of the QBA were used as independent variables. These two components accounted for 51.0% of the total variance. Component 1 included positive loadings of the absence of sham chewing, the absence of tongue rolling and the use of enrichment material. Further, it was associated with positive loadings of adjectives such as “enjoying” or “not frustrated”. Component 1 describes the use of enrichment material while not performing “abnormal behavior” such as stereotypies and is further connected to adjectives describing a positive animals' mood such as “enjoying” or “not frustrated”. Stereotypies are defined as repeated, unaltered, and non-functional behaviors and can be associated with stress and compromised welfare (Mason, 1991). Therefore, the absence of stereotypies was added to the analysis which aimed at presenting indicators of high animal welfare.
Pigs have a strong urge to explore and spend a large proportion of the day looking for food, i.e., rooting, in nature (Bracke and Hopster, 2006). Therefore, access to appropriate enrichment material is important. In rearing pigs, a direct link between the use of enrichment material and the absence of stereotypies has been demonstrated (Casal-Plana et al., 2017). This is also reflected in component 1 of the present study. The presence of enrichment material encourages the animals to follow their natural exploratory behaviors, which is necessary to ensure animal welfare in pigs (Studnitz et al., 2007). Having in mind the natural motivation of pigs to explore, it seems likely that the use of enrichment material is accompanied by body language signals such as “enjoying” or “not bored”. Similar to the results of the present study, studies in fattening pigs have identified a component containing the adjectives “happy” and “positively occupied” (Mullan et al., 2011; Temple et al., 2011, 2013; Munsterhjelm et al., 2015).
Component 2 was characterized by positive loadings of the adjectives “not agitated”, “calm” and “not lively”. Thus, component 2 is characterized by a low state of arousal. In their natural environment, pigs are highly social animals and form stable groups (Stolba and Wood-Gush, 1989). Positive interactions have been proven to result in physiological processes that are perceived as beneficial (Panksepp and Burgdorf, 2006). Moreover, positive social behavior reduces the effect of stressful impacts, so-called “social buffering” (Kikusui et al., 2006), which has been identified in for instance sheep (Porter et al., 1995) and cattle (Mounier et al., 2006). Copado et al. (2004) found that non-agonistic interactions between pigs occurred especially in inactive or resting animals. This is consistent with the finding that pigs come together in a large group especially for resting (Rodríguez-Estévez et al., 2010). In sum, the components could be labeled as (1) “satisfaction of exploratory behavior” and (2) “social resting” and are plausible from a biological point of view. In this context, it is important to note that the first component always explains the largest proportion of the variance in the dataset according to the definition of PCA (O'Rourke and Hatcher, 2013).
One of the Welfare Quality® protocol's behavioral indicators, the human–animal relationship test, did not significantly load on the components detected but only achieved loadings of 0.13 and 0.03, respectively. Hence, taking into account the fact that the Welfare Quality® protocols have been criticized for their lack of feasibility (Friedrich et al., 2019b), it may be possible to reduce the Welfare Quality® protocol for sows and piglets by eliminating this indicator.
As explained above, not necessarily the adjectives of the QBA themselves, but their pattern is intended to describe the behavior and body language of the animals (Wemelsfelder and Millard, 2009). Therefore, the QBA was pre-aggregated to weighted sums following the calculation rules for fattening pigs (Welfare Quality®, 2009). The corresponding weighted sums were used for the subsequent PCA on sows' behavior instead of the QBA adjectives. As a result, the variance explained slightly decreased to 48.3%. Literature has claimed that the resulting principal components should be able to explain between 70 and 80% of the total variance even though these values have been discussed as subjective and arbitrary (O'Rourke and Hatcher, 2013). Nevertheless, variance did not achieve these values in the present study. Thus, taking into account the small sample size, further studies are necessary to confirm the results of the present study. However, it is also possible that the behavioral indicators of the Welfare Quality® protocol do not cover all aspects of sows' behavior, resulting in only a fraction of the variance being explained. For example, Krugmann et al. (2019) mentioned other indicators (e.g., play behavior, body language signals) that can be used to indicate an influence on the animals' positive affective state. This was measured by the QBA in the present study.
The solution with the pre-aggregated QBA was comparable to the solution outlined above. Again, the two components could be labeled as (1) “satisfaction of exploratory behavior” and (2) “social resting”. In contrast to the solution using the adjectives of the QBA as independent variables, component 2 of the solution with pre-aggregated QBA included negative loadings of positive social behavior and positive loadings of the absence of negative social behavior and the absence of other active behavior, thus, non-active social behavior.
The pre-aggregated QBA did not reach the threshold for significant loadings with a loading of 0.49 on the first component. This seems reasonable since aggregating the QBA it measures whether the welfare on a farm is high, i.e., whether an animal is satisfying its natural exploring behavior and has high welfare, measured by both components detected. Still, an anthropocentric assessment of emotions in animals cannot be ruled out: the results of the present study indicate that one observer scored an animal using enrichment material and not performing abnormal behavior as content while being free from boredom. The validity of the QBA has already been questioned in pigs (Czycholl et al., 2017; Friedrich et al., 2019a, 2020c). Further, subjectivity of the QBA has also been addressed in other studies (Wemelsfelder et al., 2009, Bokkers et al., 2012, Tuyttens et al., 2014). Hence, given the potential subjectivity of the QBA and the discussed lack of feasibility (Friedrich et al., 2019b), it may be possible to reduce the Welfare Quality® protocol for sows and piglets by the QBA.
In conclusion, the two components extracted were able to mirror behavioral patterns that are performed in a natural environment and are beneficial to the animals (Bracke and Hopster, 2006). Using the components found, it would subsequently be possible to calculate component scores (O'Rourke and Hatcher, 2013). There is currently only one aggregation for fattening pigs and none for sows in the Welfare Quality® protocol for pigs (Welfare Quality®, 2009). The data could be aggregated via component scores in order to enable a feasible comparison for instance between farms or to be used for labeling purposes.
In addition, the present study highlighted the importance of direct observations of behavior using instantaneous scan sampling. These observations were able to identify two important components of sows' behavior, exploratory behavior and social interactions. Direct behavioral observations using instantaneous scan sampling have proven to be feasible and reliable in studies on both fattening pigs and sows (Czycholl et al., 2016a,b; Friedrich et al., 2019a, 2020c). Their use is therefore highly recommended for the objective assessment of animal welfare in pigs in general and in sows in particular.
The present study's data collection was carried out on 13 farms. Repeated farm visits to each farm were considered independent since animals rotated within the farm due to the production cycle or because animals left or entered the farm due to replacement. The resulting 85 farm visits could be seen as only a small sample size. However, the tests for suitability of the dataset performed beforehand (Spearman's rank correlation coefficient, KMO measure) and the verification of the number of components using parallel analysis confirmed the validity of the analysis. Moreover, significant components could be obtained even with a small dataset.
The farms participating in the present study were selected with the aim of maximizing inter-farm variability. Nevertheless, it cannot be ruled out that none of the farms exhibited animals performing “appropriate behavior” as classified by Welfare Quality®. On the other hand, it is possible that the farms included were a sample of farms with higher welfare since participation was voluntary. However, as there is no reliable gold standard to measure the latent variable “appropriate behavior”, the present study is a starting point to describe on-farm behavior in sows.
Conclusion
The present study emphasized the importance of objective behavioral indicators to assess welfare in sows. In this context, instantaneous scan sampling is particularly noteworthy. On the other hand, the importance of the variables QBA and the human–animal relationship test are questionable, especially in line with feasibility. However, as not all variance could be explained, it can be hypothesized that some important behavioral aspects are not captured by the Welfare Quality® protocol at present. The results of the present study can be used to improve the feasibility of the protocol assessment since PCA contributes to a reduction in variables but can also be applied for the calculation of component scores to develop an aggregation of the variables. This in turn may increase comparability between farms and thus contribute to animal welfare on-farm.
Data Availability Statement
The datasets presented in this article are not readily available because data are only available after finishing all studies. Requests to access the datasets should be directed to bGZyaWVkcmljaEB0aWVyenVjaHQudW5pLWtpZWwuZGU=.
Ethics Statement
Ethical review and approval was not required for the animal study because the animals were farm animals that were only observed and not handled in any way. All animals were kept according to EU and national law. The observations of the Welfare Quality protocol were developed with the aim to keep animal disturbance as well as disturbance of routine works on the farm to an absolute minimum. No pain, suffering or injury was inflicted on the animals during the study.
Author Contributions
LF, JK, and IC: conceptualization, investigation, and methodology. LF: data curation, visualization, and writing—original draft. LF and IC: formal analysis and software. JK and IC: funding acquisition and resources. IC: project administration. JK, NK, and IC: supervision. JK and NK: validation. LF, JK, NK, and IC: writing—review and editing. All authors contributed to the article and approved the submitted version.
Funding
This work was financially supported by the H.W. Schaumann Foundation. Further, we acknowledge financial support by DFG within the funding programme Open Access Publizieren.
Conflict of Interest
The authors declare that the research was conducted in the absence of any commercial or financial relationships that could be construed as a potential conflict of interest.
Publisher's Note
All claims expressed in this article are solely those of the authors and do not necessarily represent those of their affiliated organizations, or those of the publisher, the editors and the reviewers. Any product that may be evaluated in this article, or claim that may be made by its manufacturer, is not guaranteed or endorsed by the publisher.
Supplementary Material
The Supplementary Material for this article can be found online at: https://www.frontiersin.org/articles/10.3389/fanim.2021.728608/full#supplementary-material
References
Blokhuis, H. J., Miele, M., Veissier, I., and Jones, B, . (eds) (2013). Improving farm Animal Welfare: Science and Society Working Together: the Welfare Quality Approach. Wageningen, The Netherlands: Wageningen Academic Publishers. doi: 10.3920/978-90-8686-770-7
Bokkers, E. A. M., Vries, M., de Antonissen, I. C. M. A., and de Boer, I. J. M. (2012). Inter- and intra-observer reliability of experienced and inexperienced observers for the qualitative behaviour assessment in dairy cattle. Anim. Welf. 21, 307–318. doi: 10.7120/09627286.21.3.307
Botreau, R., Veissier, I., Butterworth, A., Bracke, M. B. M., and Keeling, L. J. (2007). Definition of criteria for overall assessment of animal welfare. Anim. Welf. 16, 225–228.
Bracke, M. B. M., and Hopster, H. (2006). Assessing the importance of natural behavior for animal welfare. J. Agric. Environ. Ethics 19, 77–89. doi: 10.1007/s10806-005-4493-7
Budaev, S. V. (2010). Using principal components and factor analysis in animal behaviour research: caveats and guidelines. Ethology 116, 472–480. doi: 10.1111/j.1439-0310.2010.01758.x
Casal-Plana, N., Manteca, X., Dalmau, A., and Fàbrega, E. (2017). Influence of enrichment material and herbal compounds in the behaviour and performance of growing pigs. Appl. Anim. Behav. Sci. 195, 38–43. doi: 10.1016/j.applanim.2017.06.002
Cattell, R. B. (1966). The scree test for the number of factors. Multivar. Behav. Res. 1, 245–276. doi: 10.1207/s15327906mbr0102_10
Copado, F., Aluja, A. S., de Mayagoitia, L., and Galindo, F. (2004). The behaviour of free ranging pigs in the Mexican tropics and its relationships with human faeces consumption. Appl. Anim. Behav. Sci. 88, 243–252. doi: 10.1016/j.applanim.2004.03.013
Czycholl, I., Grosse Beilage, E., Henning, C., and Krieter, J. (2017). Reliability of the qualitative behavior assessment as included in the “Welfare Quality Assessment protocol for growing pigs.” J. Anim. Sci. 95, 3445–3454. doi: 10.2527/jas2017.1525
Czycholl, I., Kniese, C., Büttner, K., Grosse Beilage, E., Schrader, L., and Krieter, J. (2016a). Interobserver reliability of the ‘Welfare Quality® Animal Welfare Assessment Protocol for Growing Pigs'. SpringerPlus 5:1114. doi: 10.1186/s40064-016-2785-1
Czycholl, I., Kniese, C., Büttner, K., Grosse Beilage, E., Schrader, L., and Krieter, J. (2016b). Test–retest reliability of the Welfare Quality® animal welfare assessment protocol for growing pigs. Anim. Welf. 25, 447–459. doi: 10.7120/09627286.25.4.447
Farm Animal Welfare Council (1993). Second Report on the Priorities for Research and Development in Farm Animal Welfare. Tolworth (now DEFRA, London), United Kingdom: Ministry of Agriculture, Fisheries and Food.
Farm Animal Welfare Council (2009). Farm Animal Welfare in Great Britain: Past, Present and Future. Tolworth (now DEFRA, London), United Kingdom: Ministry of Agriculture, Fisheries and Food.
Fraser, A. F., and Broom, D. M. (1990). Farm Animal Behaviour and Welfare. Wallingford, United Kingdom: CAB International.
Friedrich, L., Krieter, J., Kemper, N., and Czycholl, I. (2019a). Test–retest reliability of the ‘welfare quality® animal welfare assessment protocol for sows and piglets'. Part 1. Assessment of the welfare principle of “appropriate behavior.” Animals 9:398. doi: 10.3390/ani9070398
Friedrich, L., Krieter, J., Kemper, N., and Czycholl, I. (2019b). Test–retest reliability of the Welfare Quality Assessment protocol for pigs applied to sows and piglets. Part 2. Assessment of the principles good feeding, good housing, and good health. J. Anim. Sci. 97, 1143–1157. doi: 10.1093/jas/skz018
Friedrich, L., Krieter, J., Kemper, N., and Czycholl, I. (2020a). Animal welfare assessment in sows and piglets—introduction of a new german protocol for farm's self-inspection and of new animal-based indicators for piglets. Agriculture 10:506. doi: 10.3390/agriculture10110506
Friedrich, L., Krieter, J., Kemper, N., and Czycholl, I. (2020b). Iceberg indicators for sow and piglet welfare. Sustainability 12:8967. doi: 10.3390/su12218967
Friedrich, L., Krieter, J., Kemper, N., and Czycholl, I. (2020c). Interobserver reliability of measures of the “Welfare Quality® animal welfare assessment protocol for sows and piglets.” Anim. Welf. 29, 323–337. doi: 10.7120/09627286.29.3.323
Hair, J., Anderson, R., Tatham, R., and Black, W. (1995). Multivariate Data Analysis. Simon and Schuster Company: Englewood Cliffs, NJ.
Henson, R. K., and Roberts, J. K. (2016). Use of exploratory factor analysis in published research. Educ. Psychol. Meas. 66, 393–416. doi: 10.1177/0013164405282485
Horn, J. L. (1965). A rationale and test for the number of factors in factor analysis. Psychometrika 30, 179–185. doi: 10.1007/BF02289447
Kaiser, H. F. (1960). The application of electronic computers to factor analysis. Educ. Psychol. Meas. 20, 141–151. doi: 10.1177/001316446002000116
Kaiser, H. F. (1970). A second generation little jiffy. Psychometrika 35, 401–415. doi: 10.1007/BF02291817
Kaiser, H. F. (1991). Coefficient alpha for a principal component and the Kaiser-Guttman rule. Psychol. Rep. 68, 855–858. doi: 10.2466/pr0.1991.68.3.855
Kaiser, H. F., and Rice, J. (1974). Little jiffy, mark IV. Educ. Psychol. Meas. 34, 111–117. doi: 10.1177/001316447403400115
Kikusui, T., Winslow, J. T., and Mori, Y. (2006). Social buffering: relief from stress and anxiety. Philos. Trans. R. Soc. Lond. B. Biol. Sci. 361, 2215–2228. doi: 10.1098/rstb.2006.1941
Krugmann, K., Warnken, F., Krieter, J., and Czycholl, I. (2019). Are behavioral tests capable of measuring positive affective states in growing pigs? Animals 9:274. doi: 10.3390/ani9050274
Mason, G. J. (1991). Stereotypies: a critical review. Anim. Behav. 41, 1015–1037. doi: 10.1016/S0003-3472(05)80640-2
Mellor, D. J., and Reid, C. S. W. (1994). “Concepts of animal well-being and predicting the impact of procedures on experimental animals,” in Improving the Well-being of Animals in the Research Environment, eds R. Baker, G. Jenkin and D. J. Mellor (Glen Osmond, SA: Australian and New Zealand Council for the Care of Animals in Research and Teaching)
Mounier, L., Veissier, I., Andanson, S., Delval, E., and Boissy, A. (2006). Mixing at the beginning of fattening moderates social buffering in beef bulls. Appl. Anim. Behav. Sci. 96, 185–200. doi: 10.1016/j.applanim.2005.06.015
Mullan, S., Edwards, S. A., Butterworth, A., Whay, H. R., and Main, D. C. J. (2011). Inter-observer reliability testing of pig welfare outcome measures proposed for inclusion within farm assurance schemes. Vet. J. 190, 100–109. doi: 10.1016/j.tvjl.2011.01.012
Munsterhjelm, C., Heinonen, M., and Valros, A. (2015). Application of the Welfare Quality® animal welfare assessment system in Finnish pig production, part I: Identification of principal components. Anim. Welf. 24, 151–160. doi: 10.7120/09627286.24.2.151
O'Rourke, N., and Hatcher, L. (2013). Factor Analysis and Structural Equation Modeling. Cary: SAS Institute.
Panksepp, J., and Burgdorf, J. (2006). The neurobiology of positive emotions. Neurosci. Biobehav. Rev. 30, 173–187. doi: 10.1016/j.neubiorev.2005.06.001
Porter, R. H., Nowak, R., and Orgeur, P. (1995). Influence of a conspecific agemate on distress bleating by lambs. Appl. Anim. Behav. Sci. 45, 239–244. doi: 10.1016/0168-1591(95)00630-B
Rodríguez-Estévez, V., Sánchez-Rodríguez, M., Gómez-Castro, A. G., and Edwards, S. A. (2010). Group sizes and resting locations of free range pigs when grazing in a natural environment. Appl. Anim. Behav. Sci. 127, 28–36. doi: 10.1016/j.applanim.2010.08.010
Rushen, J., Chapinal, N., and de Am Passill, é (2012). Automated monitoring of behavioural-based animal welfare indicators. Anim. Welf. 21, 339–350. doi: 10.7120/09627286.21.3.339
Stolba, A., and Wood-Gush, D. G. M. (1989). The behaviour of pigs in a semi-natural environment. Anim. Sci. 48, 419–425. doi: 10.1017/S0003356100040411
Studnitz, M., Jensen, M. B., and Pedersen, L. J. (2007). Why do pigs root and in what will they root? A review on the exploratory behaviour of pigs in relation to environmental enrichment. Appl. Anim. Behav. Sci. 107, 183–197. doi: 10.1016/j.applanim.2006.11.013
Temple, D., Manteca, X., Dalmau, A., and Velarde, A. (2013). Assessment of test–retest reliability of animal-based measures on growing pig farms. Livest. Sci. 151, 35–45. doi: 10.1016/j.livsci.2012.10.012
Temple, D., Manteca, X., Velarde, A., and Dalmau, A. (2011). Assessment of animal welfare through behavioural parameters in Iberian pigs in intensive and extensive conditions. Appl. Anim. Behav. Sci. 131, 29–39. doi: 10.1016/j.applanim.2011.01.013
Tuyttens, F. A. M., Graaf, S., de Heerkens, J. L. T., Jacobs, L., Nalon, E., Ott, S., et al. (2014). Observer bias in animal behaviour research: can we believe what we score, if we score what we believe? Anim. Behav. 90, 273–280. doi: 10.1016/j.anbehav.2014.02.007
Webster, A. J. F., Main, D. C. J., and Whay, H. R. (2004). Welfare assessment: indices from clinical observation. Anim. Welf. 13, 93–98.
Webster, J. (2005). The assessment and implementation of animal welfare: theory into practice. Rev. Sci. Tech. 24, 723–734. doi: 10.20506/rst.24.2.1602
Welfare Quality® (2009). Welfare Quality®: Assessment Protocol for Pigs (Sows and Piglets, Growing and Finishing Pigs). Lelystad, The Netherlands: Welfare Quality® Consortium.
Wemelsfelder, F., and Millard, F. (2009). “Qualitative behaviour assessment,” in Welfare Quality Reports: Assessment of Animal Welfare Measures for Sows, Piglets and Fattening Pigs, eds. B. Forkman and L. J. Keeling (Cardiff: Cardiff University), 215–224.
Wemelsfelder, F., Nevison, I., and Lawrence, A. B. (2009). The effect of perceived environmental background on qualitative assessments of pig behaviour. Anim. Behav. 78, 477–484. doi: 10.1016/j.anbehav.2009.06.005
Williams, B., Onsman, A., and Brown, T. (2010). Exploratory factor analysis: a five-step guide for novices. J. Emerg. Primary Health Care 8:93. doi: 10.33151/ajp.8.3.93
Keywords: animal welfare, pigs, principal component analysis, aggregation, feasibility
Citation: Friedrich L, Krieter J, Kemper N and Czycholl I (2021) Application of Principal Component Analysis of Sows' Behavioral Indicators of the Welfare Quality® Protocol to Determine Main Components of Behavior. Front. Anim. Sci. 2:728608. doi: 10.3389/fanim.2021.728608
Received: 21 June 2021; Accepted: 23 August 2021;
Published: 13 September 2021.
Edited by:
Maria José Hötzel, Federal University of Santa Catarina, BrazilReviewed by:
Mateus Paranhos Da Costa, São Paulo State University, BrazilHayley Randle, Charles Sturt University, Australia
Copyright © 2021 Friedrich, Krieter, Kemper and Czycholl. This is an open-access article distributed under the terms of the Creative Commons Attribution License (CC BY). The use, distribution or reproduction in other forums is permitted, provided the original author(s) and the copyright owner(s) are credited and that the original publication in this journal is cited, in accordance with accepted academic practice. No use, distribution or reproduction is permitted which does not comply with these terms.
*Correspondence: Lena Friedrich, bGZyaWVkcmljaEB0aWVyenVjaHQudW5pLWtpZWwuZGU=