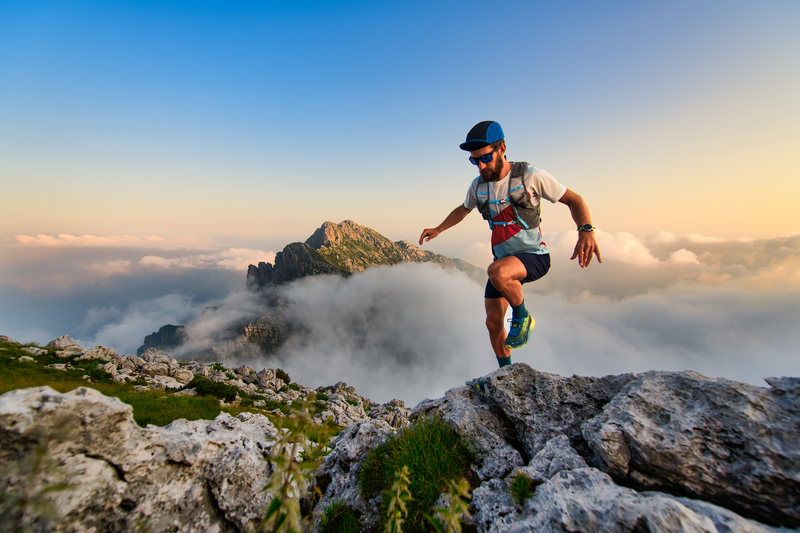
95% of researchers rate our articles as excellent or good
Learn more about the work of our research integrity team to safeguard the quality of each article we publish.
Find out more
EDITORIAL article
Front. Appl. Math. Stat. , 09 December 2024
Sec. Mathematical Biology
Volume 10 - 2024 | https://doi.org/10.3389/fams.2024.1534439
This article is part of the Research Topic Mathematical Modeling of Diseases at Population-Level and Cellular-Level View all 7 articles
Editorial on the Research Topic
Mathematical modeling of diseases at population-level and cellular-level
The growing global burden of communicable and non-communicable diseases necessitates innovative tools for understanding and managing health challenges. Mathematical modeling has emerged as a cornerstone in addressing these needs, offering deep insights into disease transmission, progression, and control. From guiding public health policies during pandemics to optimizing personalized treatment protocols, these models continue to shape the future of healthcare.
The COVID-19 pandemic highlighted the power of mathematical models in predicting outbreaks, evaluating intervention strategies, and informing public health responses. These models help policymakers simulate “what-if” scenarios, enabling data-driven decisions that save lives and optimize resource allocation. Beyond infectious diseases, chronic conditions such as cancer, diabetes, and cardiovascular diseases have also benefited from mathematical approaches, which estimate disease burden, evaluate cost-effectiveness of interventions, and inform prevention strategies.
Recent studies illustrate the diverse applications of mathematical modeling. For example, Zou et al. introduced a nomogram for predicting cardiovascular disease (CVD) risk in patients with erythrodermic psoriasis (EP). By leveraging clinical parameters, this study offered valuable insights into the relationship between psoriasis and cardiovascular health, enabling more effective patient care strategies. Similarly, Aga et al. developed a mathematical model to understand and control co-infections of pneumonia and COVID-19. Using numerical simulations and optimal control theory, they showed that combining treatment and prevention strategies is the most effective approach to minimize the impact of these diseases.
At the population level, Ogana et al. presented a deterministic model to analyze the effects of non-pharmaceutical interventions (NPIs) on COVID-19 transmission in Kenya. Their model revealed that NPIs reduced disease transmission by approximately 43.7% from baseline levels but showed a subsequent increase in transmission rates by 32% upon relaxation of measures. This comprehensive analysis emphasized the critical role of NPIs in managing infectious diseases. Alemneh et al. developed a cholera transmission model that included pathogen shedding and environmental dynamics, highlighting that combining vaccination, water quality improvements, and treatment effectively reduces cholera prevalence.
At the cellular level, mathematical models provide insights into disease mechanisms. Chakraborty et al. explored within-host dynamics of Mycobacterium tuberculosis (Mtb), offering a detailed overview of how Mtb interacts with the immune system. Their findings underscored the need for more sophisticated models to address gaps in understanding Mtb dynamics, which could inform better treatment strategies. Ganusov highlighted the importance of carefully considering assumptions in biological models, providing a critical lens for interpreting findings and improving the reliability of these tools.
The integration of population- and cellular-level data marks an exciting frontier in disease modeling. While population-level models capture macroscopic trends such as transmission rates and intervention impacts, cellular-level models reveal the molecular and immunological mechanisms driving these trends. A dual-scale approach can bridge these perspectives, enhancing our understanding of diseases like HIV, cancer, and future pandemics. For example, models that concurrently track viral spread and immune responses could refine strategies for both prevention and treatment.
The evolution of mathematical modeling is closely tied to advancements in computational power, data availability, and artificial intelligence (AI). Machine learning techniques now enable real-time adaptation and optimization of models, making them invaluable during rapidly evolving scenarios like pandemics. These data-driven approaches complement traditional methods, opening new avenues for precision in disease management.
In this journal, we welcome research that spans a diverse range of topics, from the dynamics of infectious and chronic diseases to the qualitative behavior of complex systems. We encourage submissions that explore innovative modeling techniques, including deterministic, stochastic, and agent-based approaches. Particularly, we aim to foster studies that integrate multi-scale data, addressing both macroscopic epidemiological trends and microscopic cellular processes.
Mathematical modeling has become a cornerstone of modern disease management, offering comprehensive insights that inform policy, optimize treatment, and improve health outcomes. As computational and data-driven methodologies advance, the field holds immense promise for addressing the pressing challenges of global health. By integrating cutting-edge research and fostering interdisciplinary collaboration, we can unlock new strategies to combat diseases and enhance public health worldwide.
PD: Conceptualization, Data curation, Investigation, Methodology, Project administration, Supervision, Writing – original draft, Writing – review & editing. JM: Project administration, Supervision, Writing – original draft, Writing – review & editing.
The authors declare that the research was conducted in the absence of any commercial or financial relationships that could be construed as a potential conflict of interest.
All claims expressed in this article are solely those of the authors and do not necessarily represent those of their affiliated organizations, or those of the publisher, the editors and the reviewers. Any product that may be evaluated in this article, or claim that may be made by its manufacturer, is not guaranteed or endorsed by the publisher.
Keywords: mathematical modeling, SIR models, virus dynamics models, infectious diseases, rare diseases
Citation: Dubey P and Malinzi J (2024) Editorial: Mathematical modeling of diseases at population-level and cellular-level. Front. Appl. Math. Stat. 10:1534439. doi: 10.3389/fams.2024.1534439
Received: 25 November 2024; Accepted: 27 November 2024;
Published: 09 December 2024.
Edited and reviewed by: Raluca Eftimie, University of Franche-Comté, France
Copyright © 2024 Dubey and Malinzi. This is an open-access article distributed under the terms of the Creative Commons Attribution License (CC BY). The use, distribution or reproduction in other forums is permitted, provided the original author(s) and the copyright owner(s) are credited and that the original publication in this journal is cited, in accordance with accepted academic practice. No use, distribution or reproduction is permitted which does not comply with these terms.
*Correspondence: Preeti Dubey, cHJlZXRpdXAyOEBnbWFpbC5jb20=
Disclaimer: All claims expressed in this article are solely those of the authors and do not necessarily represent those of their affiliated organizations, or those of the publisher, the editors and the reviewers. Any product that may be evaluated in this article or claim that may be made by its manufacturer is not guaranteed or endorsed by the publisher.
Research integrity at Frontiers
Learn more about the work of our research integrity team to safeguard the quality of each article we publish.