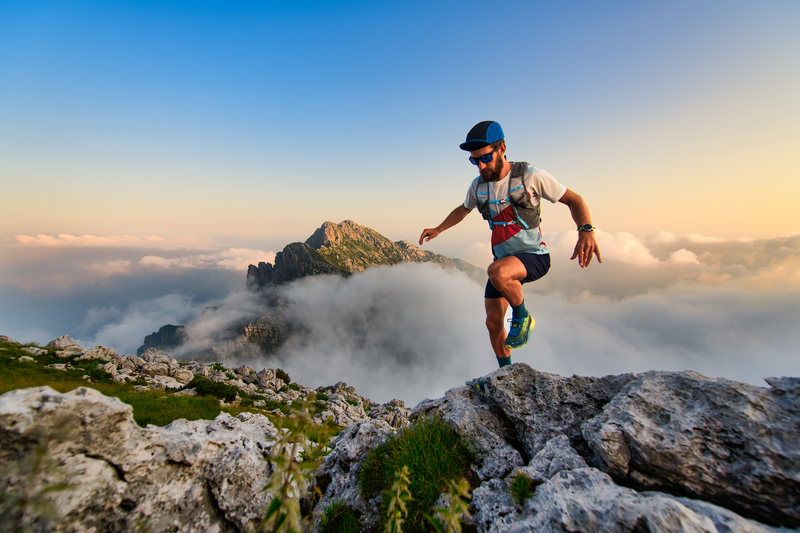
94% of researchers rate our articles as excellent or good
Learn more about the work of our research integrity team to safeguard the quality of each article we publish.
Find out more
HYPOTHESIS AND THEORY article
Front. Appl. Math. Stat. , 24 January 2023
Sec. Mathematical Biology
Volume 9 - 2023 | https://doi.org/10.3389/fams.2023.1046185
This article is part of the Research Topic Do individuals matter? - Individual-based versus population-based models applied to biology and health View all 5 articles
Ecology is usually very good in making descriptive explanations of what is observed, but is often unable to make predictions of the response of ecosystems to change. This has implications in a human-dominated world where a suite of anthropogenic stresses are threatening the resilience and functioning of ecosystems that sustain mankind through a range of critical regulating and supporting services. In ecosystems, cause-and-effect relationships are difficult to elucidate because of complex networks of negative and positive feedbacks. Therefore, being able to effectively predict when and where ecosystems could pass into different (and potentially unstable) new states is vitally important under rapid global change. Here, we argue that such better predictions may be reached if we focus on organisms instead of species, because organisms are the principal biotic agents in ecosystems that react directly on changes in their environment. Several studies show that changes in ecosystems may be accurately described as the result of changes in organisms and their interactions. Organism-based theories are available that are simple and derived from first principles, but allow many predictions. Of these we discuss Trait-based Ecology, Agent Based Models, and Maximum Entropy Theory of Ecology and show that together they form a logical sequence of approaches that allow organism-based studies of ecological communities. Combining and extending them makes it possible to predict the spatiotemporal distribution of groups of organisms in terms of how metabolic energy is distributed over areas, time, and resources. We expect that this “Organism-based Ecology” (OE) ultimately will improve our ability to predict ecosystem dynamics.
Natural ecosystems across the biosphere are increasingly being damaged or destroyed by a suite of anthropogenic processes, including deforestation, over-harvesting of plants and animals, agricultural intensification, pollution, invasive plants and animals, and climate change [1–6]. As a result, significant declines of biodiversity are being reported [7, 8]. The implications of global environmental changes and the loss of biodiversity for the structure of communities and the functioning of ecosystems are being widely discussed and debated [9, 10]. However, given that ecological responses are highly contingent, i.e., depending on location and time, ecology is often unable to accurately predict the response of ecosystems to change [11–21]. This is essential if we are to take remedial measures before critical tipping points are passed [22]. So, we not only need to know what will change, but also the mechanisms through which communities and ecosystems will change.
In the past, the amount of data that could be collected and analyzed has limited our ability to study ecosystems. After all, during one season, the properties of only a restricted number of organisms could be assessed in the field by one person, as could the population size of only a small number of species, or the state values of only a few abiotic factors. This is changing thanks to automatic data collection techniques and enlarged computer capacity ([15, 23, 24]; Appendix 1 in Supplementary material). We are now in the position that we can re-evaluate our need for data and develop new avenues of research. The ability of collecting more data and interpreting them with new technology will be helpful in many ways, but the question remains how to produce better predictions in ecology. We regard as “better predictions,” predictions of the empirical changes in ecosystems that are more accurate over larger spatial and longer time scales. We agree with Marquet et al. [25] that, although more and better data are important, they can only result in better predictions of ecosystems changes when they are analyzed based on well-established concepts and theories. In this paper, we focus on predictions of the biotic part of ecosystems, that is the community (see Table 1 for our definitions of concepts), and suggest that better predictions of community behavior may be reached when we take organisms as fundamental units rather than species. For organisms “efficient theories,” i.e., theories that are simple, parsimonious, derived from first principles, quantitative, and mathematical, with few inputs and many predictions, are available, which is not true for species [25]. We will (1) argue that a shift in main emphasis from species to organisms is theoretically justified, and (2) show that such a shift has great theoretical and practical prospects for producing better predictions of the changes in communities.
In ecological literature, a population is usually defined as the set of organisms that belong to the same species, a community as the set of species, and a meta-community as the set of communities [26]. So, the organism is the entity that defines a population, the species that of a community, and the community that of a meta-community. This step-wise approach, which we could call “Organism-Species-Community-based Ecology” (OSCE; Table 1), has long proven to be successful as a conceptual approach. OSCE has led to our present body of knowledge on populations, communities, and meta-communities and our ability to explain many ecological phenomena and patterns. But OSCE has not succeeded in reaching the better predictions of community and ecosystem dynamics we need.
A stronger emphasis on organisms may help alleviating this lack of predictability. Organisms are fundamentally different from populations and communities, because an organism is a living entity that has an organized structure, maintains homeostasis, and can act opportunistically. To do that, it collects information of its surroundings and its past successes and failures. Organisms react directly to each other and to their environment in order to increase the chance that they will survive or participate in the reproduction. In this teleonomic sense they are goal-oriented, which cannot be said for populations and communities [26]. Organisms are therefore the principal agents in both populations and communities [34–36, 38–41]. Or, as DeAngelis and Grimm [36] paraphrased Dobzhansky “Nothing makes sense in ecology except in the light of the individual.” This can also be recognized in the definitions of ecology that one can find in handbooks: they essentially all start by stating that ecology is the study of the interactions among organisms and their environment. Importantly, the definitions are never about species or communities, such as [42–45]. In light of these definitions, it is remarkable that so much of ecology is about species, and not organisms [46]. Even more so when one realizes that a species is a taxonomic unit, not an ecological one.
Organisms can react on changes in their direct biotic and abiotic environment by changes in their metabolism, physiology, appearance, and behavior. These changes can be measured by assessing the distribution of organisms in space and time, and/or by assessing their properties, i.e., their internal or external trait values. Importantly, these changes are individual and fast as compared to changes in populations or communities. For example, measurements of stable isotope ratios of water in tree xylem allowed detecting the impacts of salinization on diminishing the resilience of salinity-intolerant trees up to 25 years before the glycophytic trees were actually threatened with a regime shift to halophytic ones [47, 48]. This kind of early detection provides critical lead time and valuable information for planning mitigation against the adverse impacts of, in this case, sea level rise and climate change. Furthermore, the example shows that the time scale of an organism-based study may be quite different from that of a species-based study of the same system.
Therefore, we postulate that studying the reactions of organisms to environmental changes may be a fruitful strategy when aiming to predict the functioning of communities and meta-communities. We follow Gouveia et al. [30] in calling this “Organism-based Ecology” (OE). The above quote of DeAngelis and Grimm [36] points out that in much ecological literature “individual” seems to be equivalent to “organism.” The individual is usually regarded as the smallest indivisible entity, irrespective of it being a goal-oriented entity or not. We prefer to use “organism” because we like to stress that the organism is the largest biotic entity that shows goal-orientation [39, 40].
Because population biology is traditionally built on individual organisms as the basic research unit, most of traditional population ecology is OE, or rather it should be [38, 49]. But are community and meta-community studies also possible with organisms as basic units? In other words, can spatiotemporal patterns, which are now being explained with species and communities, also be explained at the organismal level? And, more importantly, can changes in communities and meta-communities also be predicted based on organisms? We think that the references we cited in the previous section have convincingly shown that better predictions can be expected, but that does not mean that we think that OE will always succeed. Much would already be gained if we could learn which community changes can be predicted and which not, and what would be the best way to do so. For that it is important that a consistent organism-based approach is chosen in all steps of a study, from the data collection and exploration, to the identification of potential mechanisms, and the statistical analysis of the data that results in predictions. A tentative procedure for that can be found in Figure 1, which combines three existing approaches that are all essential organism-based: Trait-based Ecology (TE), Agent-based Models (ABMs), and Maximum Entropy Theory of Ecology (METE).
When a community or meta-community is described as a set of organisms, our prediction task becomes essentially the estimation of the distribution in space and time of the future set of organism Ct+1 from the present set of organisms Ct and the expected changes in the state of the ecosystem. While Ct changes axiomatically into Ct+1 as result of birth, death, immigration, and emigration, the distribution of trait values over the organisms of Ct changes into that of Ct+1 because of selection (sensu Vellend [50]) and migration, including the stochastic effects of these processes. In essence, selection means that the organisms within a spatiotemporal set differ among themselves in their ability to survive and reproduce, and that the distribution of this ability changes from set Ct to future set Ct+1 as a consequence of changing biotic and abiotic factors [51].
For clarity, we constrain the discussion on the causes of changes in selection and migration, to two types: (1) as being the result of changing abiotic factors, and (2) as being the result of changing interactions between organisms. We describe how the exploration of data, the study of mechanisms, the development of statistical models, and the applications of these models may be different between OSCE and OE.
It is common knowledge that the composition of a community in terms of properties of organism may change due to the change of abiotic factors. A well-known example of an organismic change in a community as a result of human activity is the decrease of body size of fish due to fishery [52]. Another example is the change in the relative size of body appendages of endotherms, such as bills, ears, and tails, due to climate change. The change is thought to be related to body cooling and has been observed both among and within species [53]. Both these examples could be regarded as examples of Trait-based Ecology (TE), ecology that focuses on the effect of differences in trait values among organisms [31]. TE has already a rich history, both in plant and animal ecology (Appendix 1). The exploration of trait distribution in communities, independent of species, could improve our ability to predict the community's biomass yield and resource use, as Fontana et al. [54] did in their organism-based phytoplankton study.
To illustrate how an organism-based approach may improve predictions, we explore the changes in migratory birds in North America (Appendix 2 in Supplementary material). We chose this example because Weeks et al. [55] provided an easily accessible, consistently measured, and extensive dataset of trait values of individual organisms belonging to many different species and measured over many years. Such datasets are extremely rare. Using an organism-based approach, i.e., when taxonomic association of the birds was ignored, resulted in models that were reliable, while models with species information included were overfitted. We found that since 2000 the changes in body size differed from those before 2000, especially in hatchlings, and that wing length changed before 2000 only in the birds that are older than hatchlings (Figure 2), a pattern that could not be discovered with species information included in the analysis. Moreover, this illustrates that when organisms are grouped as done by Weeks et al., that is according to age, viz hatchlings vs. older birds, meaningful ecological information is gained. We propose to call these kinds of trait groups, such as hatchlings and older birds, “Operational Ecological Units” (OEU) and thus revitalize the term of Hendrickson and Ehrlich [57] under a slightly new definition: an OEU is here regarded as a set of organisms that are ecologically alike, i.e., they all have properties that lie in the same predefined trait value range for a predefined, limited set of traits (Table 1). An OEU differs from a population in that its members do not need to belong to the same taxon, nor is it necessary to include all individuals of a taxon. OEUs enable the study of the differences and interactions between functional, but taxon independent, groups of organisms.
Figure 2. Results of the analysis of the change in wing length of North American migration birds ignoring taxonomic information; the lines are the predicted changes in Wing length. Green lines are hatchlings, blue lines older birds. Predictions were made with linear models, lm() in R version 4.0.3 [56], with age, year, period and all their interactions as independent variables. A description of this analysis is given in Appendix 2. To draw the graphs, the variable Sex and random effect variable year were ignored, in comparison with the model in Table A2.2 in Appendix 2.
Organism- and species-based approaches differ in how they treat biotic interactions. Interactions among organisms are local, that is organisms interact only with other organisms in their direct surroundings [58]. They perceive information about other organisms which enables them to adapt their reaction [59, 60]. For example, individual leopards may change their activities in the presence of tigers, depending on their location within a nature conservation area [61]. As an example of autotrophs, Clark [62] observed that the coexistence of the large number of tree species in the southeastern United States could not be explained at the species level, because there were no mean differences in the characteristics of species relevant to competition. He asserted that it is necessary to look at competition as being local and between organisms. Calculation of competition coefficients at the level of species tends to wash out organism-level effects [63]. This was confirmed by Paine et al. [64] who concluded that accounting for within-species trait variation, enabled them to more properly consider the ecological interactions among individual trees and between individual trees and their environment. And Liu et al. [65] found that individual-level trait information of trees greatly refined their understanding of how traits link to community structure, dynamics, and assembly when considering the context in which the individual is found. In summary, Trait-based Ecology for exploring datasets of organisms while ignoring species provides a simple and attractive first step toward OE by including trait variation among organisms [66].
This is not the place to go into the discussion on mechanisms vs. statistical models (see [67, 68]). We can agree that mechanisms belong to the realm of explaining, while at least some statistics belong to that of predicting. Although we propose OE in the hope of making better predictions, we think that predictions become more convincing when linked to sound knowledge of mechanisms. However, there is no shortage of potential mechanisms in ecology. On the contrary, Gaston and Blackburn [69] counted, for example, eight hypotheses for explaining the positive interspecific abundance-range size correlation, six for the interspecific abundance-body size relationship, and five for Rapoport's rule that states that species ranges are generally smaller at lower latitudes than at higher latitudes. What is needed is meticulous testing of alternative mechanisms [69]. For OE, an emphasis on mechanisms that concern individual organisms is needed. For that, “Agent-based Models” (ABMs; Appendix 1) are especially fit.
ABMs are tailored at simulating organism-based processes [33, 34, 36]. They make it possible to predict the effect of environmental changes on communities, and even ecosystems, by modeling organismal adaptations to local biotic and abiotic changes [34, 48, 70]. They are used for studying the effects of abiotic factors, the effects of changing biotic interactions, as well as for the effects of their combinations on the spatiotemporal distribution of organisms [34, 48]. But there seem to be different developments within the modeling within plant ecology and animal ecology.
In plant ecology, ABMs for population and community-level modeling have been developed largely independently from functional–structural plant models (FSPMs: models that describe the individual plant as build up out of modules, or metamers; Appendix 1). FSPMs and ABMs occupy a continuum of spatial and mechanistic detail. For example, plant growth rates are described by allometric equations that are individual-based in the LANDIS model [71]. In ABMs they may be described as whole-plant process, for example [72, 73], and in some FSPMs as multi-organ process models, for example [74]. Finally, at the mechanistically detailed end of the continuum in FSPMs, they are described as 3-D plant architecture.
In animal ecology, it has become possible to include pursuit and aversion behavior of organisms in ABMs by combining existing theories of foraging and avoidance of competition and danger, such as predation or traps [75–78]. Coexistence theory has improved with a more complete inclusion of behavioral plasticity, local heterogeneities, biotic interactions, and feedbacks [60]. Metabolic constraints of organisms may be added using the results of the Metabolic Theory of Ecology (MTE), as well as energy costs of growth, reproduction, movement, and landscape resistance [75, 79–82].
Giacomini et al. [83] describe the only study we know showing that an approach based on OEUs (called “super-individuals” in the study) instead of species could teach us much on the origin of phenotypical different groups of organisms within a community.
ABMs may contain large numbers of parameters, and the question has been raised concerning whether the uncertainty in these parameters may propagate, resulting in model outcomes with great uncertainty [84]. However, several studies have shown that this is hardly the case in applications [84–87]. A related problem is that ABMs tend to describe particular systems and produce detailed models [34]. The development of ABMs that are based on general theories and first principles—so that they can be applied for different kind of OEUs, under different sets of biotic and abiotic circumstances, and at different spatiotemporal scales—has been a real challenge. For that, protocols, such as Pattern Oriented Modeling [88], Overview, Design concepts, and Details [89], and Approximate Bayesian Computation [90], have been proposed, evaluated [34, 41, 89, 91–93], and at least for one population successfully tested [94]. All this, as well as recent developments in trait-based approaches, molecular technologies, and big data approaches in general (Appendix 1), will help to further minimize the uncertainty and increase the general applicability of ABMs.
When successful, simulations with ABMs meet the standards of pattern-oriented modeling. That is, they will agree reasonably well with at least a few empirical patterns in the system being modeled, so that it can be assumed that the underlying mechanisms are correctly represented in the model [34]. The parametrized mechanisms with which the ABMs were built can then be hypothesized to also be important for structuring real communities in space and time in general. So, successful applications of ABMs result in quantitative hypotheses of parameters of organism-based models that can be tested empirically.
A welcome advantage of OE is that it transforms communities and meta-communities from relatively “middle-number systems,” with a limited number of units (species or communities), into relatively “large-number systems,” with a large number of units (organisms), without the need to change the level of spatiotemporal scale [20, 95]. This will improve the applications of statistical models and their testing [20, 30].
The Maximum Entropy Theory of Ecology (METE) is a statistical tool to describe large-number communities as probability distribution models [37]. According to Marquet et al. [25] it is one of the examples of an efficient theory. As it is applied now, species numbers and species abundancies are an important part of METE, but models without species have already been described, viz the distribution of metabolic rates and biomasses across all individuals of a community. In theory, models that ignore species can be further developed [37]. OE could focus on a version of METE with OEUs instead of species. The core distribution of METE, the structure function Rs(n, ε | A0, S0, N0, E0), is the probability that, if a species is picked at random from the species list, then it has abundance n and if an individual organism is picked at random from the pool of individuals belonging to species of abundance n, its metabolic requirement is ε. This probability is constrained by the state variables of total area A0, total number of species S0, total number of organisms N0, and total metabolic energy rate E0 [37, 96, 97]. METE predicts universality regardless the taxonomic choices that define the assemblage of species [98], which means that one could replace the S0 by a set of OEUs, as long as organisms can unambiguously be assigned to an OEU. For OE the structure function can therefore be changed into Ro(n, ε | A0, O0, N0, E0), where O0 is the total number of OEUs, which would be the probability that if an OEU, such as body size class, is picked at random from the OEU list, such as the list of all body size classes, then it has abundance n, and if an individual organism is picked at random from the pool of organisms belonging to OEUs of abundance n, its metabolic requirement is ε. METE gives the prospect for predicting the structure of set Ct, i.e., the spatial distribution of a set of organisms, in terms of how metabolic energy is distributed over individuals, and how individuals are distributed over area, resources, and OEUs [99]. It could, for example, be used to describe the probability distribution of biomass over organisms of different feeding strategies, such as autotrophs, herbivores, predators, parasites, omnivores, and decomposers, within a given area under the assumption of a specific relationship between metabolic rate and biomass, so that B0 replaces E0 [37, 78]. The study of the effect of a factor on this distribution could then be described in terms of changes on the total number of OEUs O0, the total number of organisms N0, and the total biomass B0 within equal subareas A of A0. In Appendix 2 we give an example of that by applying METE on the dataset of North American migration birds of Weeks et al. [55]. Including the size of the individual birds, that is small (< 5 g) vs. large birds, as an independent variable in the analyses resulted in a better fitting model and thus in a better prediction and showed that only the hatchlings of large birds did not change in deviations of METE predictions in the period till 2000, and that only the small, older birds decreased after 2000 (Figure 3). Recently, Harte et al. [100] have proposed DynaMETE, an extension of METE that makes the structure function time dependent. Prior information, such as quantitative information on model parameters, may be included via a more general Bayesian framework as equation of states, that is as fixed ratios between the state variables [67, 101, 102].
Figure 3. Results of the METE-analysis of the metabolic rate of North American migration birds. Lines are the predicted changes in (A) residuals of the METE predicted metabolic rate ignoring body size and (B) including the difference between small and large birds. Green lines are hatchlings, blue lines older birds, solid lines are large (≥5 g), and dashed lines small birds. Predictions were made with linear models, lm() in R version 4.0.3 [56], with age, year, period, and size [only in (B)], and all their interactions as independent variables. A description of this analysis is given in Appendix 2. To draw these graphs, the variable sex and random effect variable year were ignored, in comparison with the models in Table A2.4 in Appendix 2.
In the current METE, probability distributions of commonality are available [37], that can be transformed to local interactions of organisms of different OEUs the same way as we transformed the structure function. Deviations from such probability distributions may be used to study pursuit and aversion behavior of organisms of different OEUs. Also, link functions for networks of species [37, 103] could be transformed to link functions for OEUs. In general, a maximum entropy approach offers exciting perspectives to developing null-models for real-world networks [104, 105].
It may seem that the strictly statistical approach of METE is at odds with our previous statement that the organism is the agent of ecology. For METE, organisms behave like neutral particles, i.e., like particles that are all equivalent and have no differences in trait values, which is certainly not the case, as explained above. But application of METE can be defended because neutral behavior can be regarded as being an adequate proxy of the emergent behavior of a large set of organisms with different properties, that each react differently on many different local factors and on each other to achieve the common goal of sufficient fitness [37, 67, 101, 102]. METE gives unbiased probability distributions under a very limited number of constraints and unbiased collector's curves can be derived from these [37]. This makes METE also a sampling theory. When the organisms of an area are in considerable numbers affected the same way by a factor or when an interaction between organisms results in a distinct non-random pattern, the empirical collector's curve will show a deviation from the one predicted by METE's null-models. We could then start to explore mechanisms again, that is try to identify the property, factor, or biotic interaction that causes the deviation, of which we now know that it is crucial for understanding and predicting the community we are studying. Traditional statistical analyses may be used for that, although we also might need new statistical analyzing techniques to do so (Appendix 2). The search for crucial properties, factors, and interactions is facilitated by trait-based ABMs, which are well fit for organism-based identification of mechanistic factors. We think that this combination of search for organism-based mechanisms and predictions based on first principles forms the strength of OE.
Above we have described what we think it could mean to apply OE. We think that OE could start with combining existing ecological fields, such as TE, ABM, and METE (Figure 1). Because these existing fields are well-established in ecology, the development of each of them has resulted in a tradition in focus communities, sets of concepts, mathematical description, and research schools, for example [106]. One can expect that it will be a real challenge to bring them together in a consistent approach from collecting data to predicting ecosystem changes. But we think that the urgent need for improved nature conservation justifies great efforts to bring all our expertise together in the quest for predictability.
An important question still to be answered is whether OE can provide predictions that are needed for nature conservation in a changing world. After all, good examples of organism-based field studies of eukaryote communities are still rare (Table 2). Some of the theories for organisms discussed above provide such predictions. For example, the MTE allows for the correction of body sizes and metabolic rates for temperature changes. And the adaptive foraging theory of Beckerman et al. [110] enables the prediction of changes in food web complexity after the extinction of specific groups of organisms. But current theories usually assume steady-states and the results of simulations are to be described after some implementation time. Newman et al. [111] found that the METE is more robust in slowly changing or steady-state communities. Moreover, and in general, parameters of statistical models are often estimated from datasets collected in “natural” communities, that is communities that are in a relatively stable state, so that they may not be applicable for predicting short- and long-term effects of human impacts [59]. The adoption of OE could be used to shift the emphasis of METE to models for dynamic systems using DynaMETE that enables simulating what will happen to a community under stress [100].
The human fascination with and commitment to understanding species richness is so deep, that it often seems the reason why we are studying ecology in the first place [67]. Consequently, ecology is often motivated by and contributing to conservation biology that aims at finding solutions for the decrease of populations and the loss of species diversity. This made Vellend [50] regard species diversity as the first order property of a community that needs to be explained. But it may contribute to the reasons that our understanding of communities is limited, because the focus on species tends to distract from the actual ecological agents. We think that OE is well-equipped to reveal general ecological patterns, and such organism-based patterns have already been shown (Table 2). Being general, they will also be applicable to conservation of taxa [46]. So, OE will not hinder taxonomic-based applications. In fact, studies on the failures of OE to predict empirical patterns may increase our understanding of the role of taxonomic units in ecological processes, for example when Rs(n, ε) turns out to better predict communities than Ro(n, ε). OE will, therefore, enrich our fundamental understanding of ecology, including the processes that affect biodiversity [59, 63, 112, 113].
With the growing evidence that human activities are reducing biodiversity at an accelerating rate, it becomes vital to predict their impacts on the functioning of communities and ecosystems. Accurate ecological predictions are a vital tool for alerting policy-makers and land managers about future scenarios with profoundly important societal consequences [114]. Here, we have argued that better predictions of community dynamics may more effectively be achieved by focusing on organisms, rather than species, because organisms are the principal biological agents in ecosystems. Organism-based Ecology (OE) is a direct operationalization of the definition of ecology. We provided reasons to expect that it may result in urgently needed better predictions in ecology. Particularly, the properties and behavior of organisms in reaction to their environment and each other are key in the way forward to achieve a deeper understanding of ecological processes. Developments in combining the essential organism-based approaches of Trait-based Ecology (TE), Agent Based Models (ABMs), and Maximum Entropy Theory of Ecology (METE) open up new opportunities for studying communities. TE studies trait variation among organisms and can show correlations between the distribution of organismal trait values and biotic and abiotic environmental factors, ABMs can result in quantitative hypotheses of spatiotemporal patterns of Operational Ecological Units (OEUs) that can be tested empirically, and unbiased predictions of the distribution of OEUs in ecosystems can be done with the help of the statistical models of METE (Figure 1). We think ecology is ready to take up this challenge now and establishing OE in search for general patterns can be accomplished. We expect that this ultimately will improve our ability to predict ecosystem dynamics.
Publicly available datasets were analyzed in this study. This data can be found at: Dryad Digital Repository: https://doi.org/10.5061/dryad.8pk0p2nhw.
CM and GS conceived the ideas. CM performed the statistical analyses and wrote the drafts. DD, JH, WM, and PB added to the ideas, improved and sharpened them, contributed critically to the drafts, and gave final approval for publication. All authors contributed to the article and approved the submitted version.
DD was supported by Greater Everglades Priority Ecosystem Science program.
We would like to thank James McAllister and Ellen Cieraad for the stimulating discussions and the reviewers for their helpful comments. Any use of trade, firm, or product names is for descriptive purposes only and does not imply endorsement by the U.S. Government.
The authors declare that the research was conducted in the absence of any commercial or financial relationships that could be construed as a potential conflict of interest.
All claims expressed in this article are solely those of the authors and do not necessarily represent those of their affiliated organizations, or those of the publisher, the editors and the reviewers. Any product that may be evaluated in this article, or claim that may be made by its manufacturer, is not guaranteed or endorsed by the publisher.
The Supplementary Material for this article can be found online at: https://www.frontiersin.org/articles/10.3389/fams.2023.1046185/full#supplementary-material
1. Crutzen PJ. The “anthropocene”. In: Earth System Science in the Anthropocene. Berlin: Springer Verlag (2006). p. 13–8. doi: 10.1007/3-540-26590-2_3
2. Salafsky N, Salzer D, Statterfield AJ, Hilton-Taylor C, Neugarten R, Butchart SHM et al. A standard lexicon for biodiversity conservation: Unified classifications of threats and actions. Conserv Biol. (2008) 22:897–911. doi: 10.1111/j.1523-1739.2008.00937.x
3. Stoate C, Báldi A, Beja P, Boatman ND, Herzon I, de Snoo GR et al. Ecological impacts of early 21st century agricultural change in Europe. A review. J Environ Manag. (2009) 91:22–46. doi: 10.1016/j.jenvman.2009.07.005
4. Morris RJ. Anthropogenic impacts on tropical forest biodiversity: A network structure and ecosystem functioning perspective. Proc R Soc B. (2010) 365:3709–18. doi: 10.1098/rstb.2010.0273
5. Ellis EC. Anthropogenic transformation of the terrestrial biosphere. Proc R Soc B. (2011) 369:1010–35. doi: 10.1098/rsta.2010.0331
6. Lewis SL, Maslin MA. Defining the anthropocene. Nature. (2015) 519:171–80. doi: 10.1038/nature14258
7. Dirzo R, Young HS, Galetti M, Ceballos G, Isaac NJB, Collen B. Defaunation in the anthropocene. Science. (2014) 345:401–6. doi: 10.1126/science.1251817
8. Ceballos G, Ehrlich PR, Barnosky AD, García A, Pringle RM, Palmer TM. Accelerated modern human–induced species losses: Entering the sixth mass extinction. Sci Adv. (2015) 1:e1400253. doi: 10.1126/sciadv.1400253
9. Srivastava DS, Vellend M. Biodiversity-ecosystem function research: Is it relevant to conservation? Annu Rev Ecol Evol Syst. (2005) 36:267–94. doi: 10.1146/annurev.ecolsys.36.102003.152636
10. Eisenhauer N, Barnes AD, Cesarz S, Craven D, Ferlian O, Gottschall F et al. Biodiversity–ecosystem function experiments reveal the mechanisms underlying the consequences of biodiversity change in real world ecosystems. J Veget Sci. (2016) 27:1061–70. doi: 10.1111/jvs.12435
13. Coreau A, Pinay G, Thompson JD, Cheptou PO, Mermet L. The rise of research on futures in ecology: Rebalancing scenarios and predictions. Ecol Lett. (2009) 12:1277–86. doi: 10.1111/j.1461-0248.2009.01392.x
14. Bellard C, Bertelsmeier C, Leadley P, Thuiller W, Courchamp F. Impacts of climate change on the future of biodiversity. Ecol Lett. (2012) 15:365–77. doi: 10.1111/j.1461-0248.2011.01736.x
15. Evans MR, Bithell M, Cornell SJ, Dall SRX, Díaz S, Emmott S et al. Predictive systems ecology. Proc R Soc B. (2013) 280:20131452. doi: 10.1098/rspb.2013.1452
16. Thuiller W, Lavergne S, Roquet C, Boulangeat I, Lafourcade B, Araujo MB, et al. Road map for integrating eco-evolutionary processes into biodiversity models. Ecol Lett. (2013) 16:94–105. doi: 10.1111/ele.12104
17. Harfoot M, Tittensor DP, Newbold T, McInerny G, Smith MJ, Scharlemann JPW. Integrated assessment models for ecologists: The present and the future. Glob Ecol Biogeogr. (2014) 23:124–43. doi: 10.1111/geb.12100
18. Mouquet N, Lagadeuc Y, Devictor V, Doyen L, Duputié A, Eveillard D, et al. Predictive ecology in a changing world. J Appl Ecol. (2015) 52:1293–310. doi: 10.1111/1365-2664.12482
19. Green SJ, Brookson CB, Hardy NA, Crowder LB. Trait-based approaches to global change ecology: Moving from description to prediction. Proc R Soc B. (2022) 289:20220071. doi: 10.1098/rspb.2022.0071
20. Maurer BA. Untangling Ecological Complexity. The Macroscopic Perspective. Chicago, IL: The University of Chicago Press (1999).
21. Evans MR, Grimm V, Johst K, Knuuttila T, de Langhe R, Lessells CM, et al. Do simple models lead to generality in ecology? Tree. (2013) 28:578–83. doi: 10.1016/j.tree.2013.05.022
22. Dakos V, Matthews B, Hendry A, Levine J, Loeuille N, Norberg J et al. Ecosystem tipping points in an evolving world. Nat Ecol Evol. (2019) 3:355–62. doi: 10.1038/s41559-019-0797-2
23. Simberloff D. Community ecology: Is it time to move on? Am Nat. (2004) 163:787–99. doi: 10.1086/420777
24. Reichstein M, Camps-Valls G, Stevens B, Jung M, Denzler J, Carvalhais N, et al. Deep learning and process understanding for data-driven Earth system science. Nature. (2019) 566:195–204. doi: 10.1038/s41586-019-0912-1
25. Marquet PA, Allen AP, Brown JH, Dunne JA, Enquist BJ, Gillooly JF, et al. On theory in ecology. Bioscience. (2014) 64:701–10. doi: 10.1093/biosci/biu098
26. Allen TFH, Hoekstra TW. Toward a Unified Ecology. 2nd Ed. New York, NY: Columbia University Press (2015). doi: 10.7312/alle06918
27. Thompson NS. The misappropriation of teleonomy. Perspect Ethol. (1987) 7:259–74. doi: 10.1007/978-1-4613-1815-6_10
29. Blew RD. On the definition of ecosystem. Bullet Ecol Soc Am. (1996) 1996:171–3. doi: 10.2307/20168067
30. Gouveia SF, Rubalcaba JG, Soukhovolsky V, Tarasova O, Barbosa AM, Real R. Ecophysics reload exploring applications of theoretical physics in macroecology. Ecol Modell. (2020) 424:109032. doi: 10.1016/j.ecolmodel.2020.109032
31. Bolnick DI, Amarasekare P, Araújo MS, Bürger R, Levine JM, Novak M, et al. Why intraspecific trait variation matters in community ecology. Tree. (2011) 26:183–92. doi: 10.1016/j.tree.2011.01.009
32. Garnier E, Navas M-L, Grigulis K. Plant Functional Diversity. Organism Traits, Community Structure, and Ecosystem Properties. Oxford: Oxford University Press (2016).
33. DeAngelis DL, Diaz SG. Decision-making in Agent-Based Modeling: A current review and future prospectus. Front Ecol Evol. (2019) 6:237. doi: 10.3389/fevo.2018.00237
34. Grimm V, Railsback SF. Individual-Based Modelling and Ecology. Princeton, NJ: Princeton University Press (2005). doi: 10.1515/9781400850624
35. DeAngelis DL, Mooij WM. Individual-based models in ecology and evolutionary processes. Annu Rev Ecol Evol Syst. (2005) 36:147–68. doi: 10.1146/annurev.ecolsys.36.102003.152644
36. DeAngelis DL, Grimm V. Individual-based models in ecology after four decades. F1000Prime Rep. (2014) 6:39. doi: 10.12703/P6-39
38. Łomnicki A. Population Ecology of Individuals. Princeton, NJ: Princeton University Press (1988).
39. Mahner M, Bunge M. Foundations of Biophilosophy. Heidelberg: Springer (1997). doi: 10.1007/978-3-662-03368-5
40. Jagers OP, Akkerhuis GAJM. Towards a hierarchical definition of life, the organism and death. Found Sci. (2010) 15:245–62. doi: 10.1007/s10699-010-9177-8
41. Grimm V, Ayllón D, Railsback SF. Next-generation Individual-Based Models integrate biodiversity and ecosystems: Yes we can, and yes we must. Ecosystems. (2017) 20:229–36. doi: 10.1007/s10021-016-0071-2
43. Krebs CJ. Ecology: The Experimental Analysis of Distribution and Abundance. 2nd Ed. New York, NY: Harper and Row Publishers (1978).
45. Begon M, Townsend CR, Harper JL. Ecology. From Individuals to Ecosystems. 4th Ed. Hoboken, NJ: Blackwell Publishing (2005).
46. Johnston ASA, Boyd RJ, Watson JW, Paul A, Evans LC, Gardner EL, et al. Predicting population responses to environmental change from organismal level mechanisms: Towards a standardized mechanistic approach. Proc R Soc B. (2019) 286:20191916. doi: 10.1098/rspb.2019.1916
47. Zhai L, Jiang J, DeAngelis DL, da Silveira Lobo Sternberg L. Prediction of plant vulnerability to salinity increase in a coastal ecosystem by stable isotope composition (δ18O) of plant stem water: A model study. Ecosystems. (2016) 19:32–49. doi: 10.1007/s10021-015-9916-3
48. Subedi SC, Sternberg L, DeAngelis DL, Ross MS, Ogurcak DE. Using carbon isotope ratios to verify predictions of a model simulating the interaction between coastal plant communities and their effect on ground water salinity. Ecosystems. (2020) 23:570–85. doi: 10.1007/s10021-019-00423-4
49. Vahl WK. Interference Competition Among Foraging Waders. (Dissertation), University of Groningen, Groningen, Netherlands (2006).
50. Vellend M. The Theory of Ecological Communities. Monographs in Population Biology. Princeton, NJ: Princeton University Press (2016). p. 57.
51. Okasha S. Evolution and the Levels of Selection. Oxford: Oxford University Press (2006). doi: 10.1093/acprof:oso/9780199267972.001.0001
52. Heino M, Díaz Pauli B, Dieckmann U. Fisheries-induced evolution. Annu Rev Ecol Evol Syst. (2015) 46:461–8. doi: 10.1146/annurev-ecolsys-112414-054339
53. Ryding S, Klaassen M, Tattersall GJ, Gardner JL, Symonds MRE. Shape-shifting: Changing animal morphologies as a response to climatic warming. Tree. (2021) 7:6. doi: 10.1016/j.tree.2021.07.006
54. Fontana S, Thomas MK, Moldoveanu M, Spaak P, Pomati F. Individual-level trait diversity predicts phytoplankton community properties better than species richness or evenness. ISME J. (2018) 12:356–66. doi: 10.1038/ismej.2017.160
55. Weeks BC, Willard DE, Zimova M, Ellis AA, Witynski ML, Hennen M, et al. Shared morphological consequences of global warming in North American migratory birds. Ecol Lett. (2020) 23:316–25. doi: 10.1111/ele.13434
56. R Core Team. R: A Language and Environment for Statistical Computing. Vienna: R Foundation for Statistical Computing (2020).
57. Hendrickson JA, Ehrlich PR. An expanded concept of “Species diversity”. Notulae Naturae. (1971) 439:1–6.
58. Huston M, DeAngelis DL, Post W. New computer models unify ecological theory. Bioscience. (1988) 38:682–91. doi: 10.2307/1310870
59. Berger U, Piou P, Schiffers K, Grimm V. Competition among plants: Concepts, individual-based modelling approaches, and a proposal for a future research strategy. Perspect Plant Ecol Evol Syst. (2008) 9:121–35. doi: 10.1016/j.ppees.2007.11.002
60. Jeltsch F, Grimm V, Reeg J, Schlägel UE. Give chance a chance: From coexistence to coviability in biodiversity theory. Ecosphere. (2021) 10:e02700. doi: 10.1002/ecs2.2700
61. Upadhyaya SK, Lamichhane BR, Musters CJM, Subedi N, de Snoo GR, Thapa P, et al. Activity patterns of co-existing tigers and leopards. In: SK Upadhyaya , editors, Human-wildlife Interactions in the Western Terai of Nepal. An analysis of factors influencing conflicts between sympatric tigers (Panthera tigris tigris) and leopards (Panthera pardus fusca) and local communities around Bardia National Park, Nepal. (Dissertation), Leiden University, Leiden, Netherlands (2019). p. 27–42.
62. Clark JS. Individuals and the variation needed for high species diversity in forest trees. Science. (2010) 327:1129–32. doi: 10.1126/science.1183506
63. Clark JS, Bell DM, Hersh MH, Kwit MC, Moran E, Salk C, et al. Individual-scale variation, species-scale differences: Inference needed to understand diversity. Ecol Lett. (2011) 14:1273–87. doi: 10.1111/j.1461-0248.2011.01685.x
64. Paine CET, Baraloto C, Chave J, Hérault B. Functional traits of individual trees reveal ecological constraints on community assembly in tropical rain forests. Oikos. (2011) 120:720–7. doi: 10.1111/j.1600-0706.2010.19110.x
65. Liu X, Swenson NG, Lin D, Mi X, Umaña MN, Schmid B, et al. Linking individual-level functional traits to tree growth in a subtropical forest. Ecology. (2016) 97:2396–405. doi: 10.1002/ecy.1445
66. Yang J, Cao M, Swenson NG. Why functional traits do not predict tree demographic rates. Tree. (2018) 33:326–36. doi: 10.1016/j.tree.2018.03.003
68. McGill BJ, Nekola JC. Mechanisms in macroecology: AWOL or purloined letter? Towards a pragmatic view of mechanism. Oikos. (2010) 119:591–603. doi: 10.1111/j.1600-0706.2009.17771.x
69. Gaston KJ, Blackburn TM. A critique for macroecology. Oikos. (1999) 84:353–68. doi: 10.2307/3546417
70. Peters R, Lovelock C, López-Portillo J, Bathmann J, Wimmler MC, Jiang J, et al. Partial canopy loss of mangrove trees: Mitigating water scarcity by physical adaptation and feedback on porewater salinity. Estuar Coast Shelf Sci. (2021) 248:106797. doi: 10.1016/j.ecss.2020.106797
71. Mladenoff DJ. LANDIS and forest landscape models. Ecol Modell. (2004) 180:7–19. doi: 10.1016/j.ecolmodel.2004.03.016
72. Maréchaux I, Chave J. An individual-based forest model to jointly simulate carbon and tree diversity in Amazonia: Description and applications. Ecol Monogr. (2017) 87:632–64. doi: 10.1002/ecm.1271
73. Bathmann J, Peters R, Naumov D, Fischer F, Berger U, Walther M. The MANgrove-GroundwAter feedback model (MANGA) – Describing belowground competition based on first principles. Ecol Modell. (2020) 420:108973. doi: 10.1016/j.ecolmodel.2020.108973
74. Pretzsch H, Grote R, Reineking B, Rötzer T, Seifert S. Models for forest ecosystem management: A European perspective. Ann Bot. (2008) 101:1065–87. doi: 10.1093/aob/mcm246
75. Gallagher AJ, Creel S, Wilson RP, Cooke SJ. Energy landscapes and the landscape of fear. Tree. (2017) 32:88–96. doi: 10.1016/j.tree.2016.10.010
76. Teckentrup L, Grimm V, Kramer-Schadt S, Jeltsch F. Community consequences of foraging under fear. Ecol Modell. (2018) 383:80–90. doi: 10.1016/j.ecolmodel.2018.05.015
77. Hein AM, Altshuler DL, Cade DE, Liao JC, Martin BT, Taylor GK. An algorithmic approach to natural behavior. Curr Biol. (2020) 30:R663–75. doi: 10.1016/j.cub.2020.04.018
78. Vattiato G, Plank MJ, James A, Binny RN. Individual heterogeneity affects the outcome of small mammal pest eradication. Theoret Ecol. (2021) 14:219–31. doi: 10.1007/s12080-020-00491-6
79. Brown JH, Gilloolly JF, Allen AP, Savage VM, West GB. Toward a metabolic theory of ecology. Ecology. (2004) 85:1771–89. doi: 10.1890/03-9000
80. Buchmann CM, Schurr FM, Nathan R, Jeltsch F. An allometric model of home range formation explains the structuring of animal communities exploiting heterogeneous resources. Oikos. (2011) 120:106–18. doi: 10.1111/j.1600-0706.2010.18556.x
81. Graves T, Chandler RB, Royle JA, Beier P, Kendall KC. Estimating landscape resistance to dispersal. Landsc Ecol. (2014) 29:1201–12. doi: 10.1007/s10980-014-0056-5
82. Zhou J, Yu K, Lin G, Wang Z. Variance in tree growth rates provides a key link for completing the theory of forest size structure formation. J Theor Biol. (2021) 529:110857. doi: 10.1016/j.jtbi.2021.110857
83. Giacomini HC, DeAngelis DL, Trexler JC. Petrere Jr M. Trait contributions to fish community assembly emerge from trophic interactions in an individual-based model. Ecol Model. (2013) 251:32–43. doi: 10.1016/j.ecolmodel.2012.12.003
84. Mooij WM, DeAngelis DL. Error propagation in spatially explicit population models: A reassessment. Biol Conserv. (1999) 13:30–3. doi: 10.1046/j.1523-1739.1999.98153.x
85. Deutschman DH, Levin SA, Pacala SW. Error propagation in a forest succession model: The role of fine-scale heterogeneity in light. Ecology. (1999) 80:1927–43. doi: 10.2307/176669
86. Dubé P, Fortin MJ, Canham CD, Marceau DJ. Quantifying gap dynamics at the patch mosaic level using a spatially-explicit model of a northern hardwood forest ecosystem. Ecol Modell. (2001) 142:39–60. doi: 10.1016/S0304-3800(01)00238-1
87. Railsback SF, Harvey BC. Individual-Based Model Formulation for Cutthroat Trout, Little Jones Creek, California. General Technical Report No PSW-GTR-182. Albany, NY: Forest Service, United States Department of Agriculture (2001).
88. Grimm V, Revilla E, Berger U, Jeltsch F, Mooij WM, Railsback SF, et al. Pattern-oriented modeling of agent-based complex systems: Lessons from ecology. Science. (2005) 310:987–91. doi: 10.1126/science.1116681
89. Grimm V, Berger U, DeAngelis DL, Polhill JG, Giske J, Railsback SF. The ODD protocol: A review and first update. Ecol Modell. (2010) 221:2760–8. doi: 10.1016/j.ecolmodel.2010.08.019
90. Van der Vaart E, Johnston AS, Sibly RM. Predicting how many animals will be where: How to build, calibrate and evaluate individual-based models. Ecol Modell. (2016) 326:113–23. doi: 10.1016/j.ecolmodel.2015.08.012
91. Grimm V, Berger U, Bastiansen F, Eliassen S, Ginot V, Giske J et al. A standard protocol for describing individual-based and agent-based models. Ecol Modell. (2006) 198:115–26. doi: 10.1016/j.ecolmodel.2006.04.023
92. Uchmański J, Kowalczyk K, Ogrodowczyk P. Evolution of theoretical ecology in last decades: Why did individual-based modelling emerge. Ecol Quest. (2008) 10:13–8. doi: 10.12775/v10090-009-0002-3
93. Stillman RA, Railsback SF, Giske J, Berger U, Grimm V. Making predictions in a changing world: The benefits of Individual-Based Ecology. Bioscience. (2015) 65:140–50. doi: 10.1093/biosci/biu192
94. Martin BT, Jager T, Nisbet RM, Preuss TG, Grimm V. Predicting population dynamics from the properties of individuals: A cross-level test of Dynamic Energy Budget theory. Am Nat. (2013) 181:506–19. doi: 10.1086/669904
95. Sutherland WJ, Freckleton RP, Godfray HCJ, Beissinger SR, Benton T, Cameron DD, et al. Identification of 100 fundamental ecological questions. J Ecol. (2013) 101:58–67. doi: 10.1111/1365-2745.12025
96. Fravetti M. Maximum entropy theory of ecology: A reply to harte. Entropy. (2018) 20:308. doi: 10.3390/e20050308
97. Brummer AB, Newman EA. Derivations of the core functions of the maximum entropy theory of ecology. Entropy. (2019) 21:712. doi: 10.3390/e21070712
98. Harte J, Kitzes J, Newman EA, Rominger AJ. Taxon categories and the universal species-area relationship (a comment on Šizling et al. “Between Geometry and Biology: The Problem of Universality of the Species-Area Relationship”). Am Natural. (2013) 181:282–7. doi: 10.1086/668821
99. Harte J, Newman EA. Maximum information entropy: A foundation for ecological theory. Tree. (2014) 29:384–9. doi: 10.1016/j.tree.2014.04.009
100. Harte J, Umemura K, Brush M. DynaMETE: A hybrid MaxEnt-plus-mechanism theory of dynamic macroecology. Ecol Lett. (2021) 24:935–49. doi: 10.1111/ele.13714
101. Dewar RC, Porté A. Statistical mechanics unifies different ecological patterns. J Theoret Biol. (2008) 251:389–403. doi: 10.1016/j.jtbi.2007.12.007
102. Harte J, Brush M, Newman EA, Umemura K. An equation of state unifies diversity, productivity, abundance and biomass. Commun Biol. (2022) 5:874. doi: 10.1038/s42003-022-03817-8
103. Williams RJ. Simple MaxEnt models explain food web degree distributions. Theoret Ecol. (2010) 3:45–52. doi: 10.1007/s12080-009-0052-6
104. Cimini G, Squartini T, Saracco F, Garlaschelli D, Gabrielli A, Caldarelli G. The statistical physics of real-world networks. Nat. Rev. Phys. (2019) 1:58–71. doi: 10.1038/s42254-018-0002-6
105. Banville F, Gravel D, Poisot T. What constrains food webs? A maximum entropy ramework for predicting their structure with minimal biases. arXiv:2210.03190v1. (2022). doi: 10.48550/arXiv.2210.03190
106. Wilcox KR, Komatsu KJ, Avolio ML. Improving collaborations between empiricists and modelers to advance grassland community dynamics in ecosystem models. N Phytolog. (2020) 228:1467–71. doi: 10.1111/nph.16900
107. Bodelier PLE, Meima-Franke M, Hordijk CA, Steenbergh AK, Hefting MM, Bodrossy L, et al. Microbial minorities modulate methane consumption through niche partitioning. ISME J. (2013) 7:2214–28. doi: 10.1038/ismej.2013.99
108. Musters CJM, Evans TR, Wiggers JMR, van ‘t-Zelfde M, de Snoo GR. Distribution of flying insects across landscapes with intensive agriculture in temperate areas. Ecol. Indic. (2021) 129:107889. doi: 10.1016/j.ecolind.2021.107889
109. Musters CJM, Wiggers JMR, de Snoo GR. Distribution of ground-dwelling arthropods across landscapes with intensive agriculture in temperate areas. Ecol Indic. (2022) 140:109042. doi: 10.1016/j.ecolind.2022.109042
110. Beckerman AP, Petchey OL, Warren PH. Foraging biology predicts food web complexity. Proc Natl Acad Sci USA. (2006) 37:13745–9. doi: 10.1073/pnas.0603039103
111. Newman EA, Wilber MQ, Kopper KE, Moritz MA, Falk DA, McKenzie D, et al. Disturbance macroecology: A comparative study of community structure metrics in a high-severity disturbance regime. Ecosphere. (2020) 11:e03022. doi: 10.1002/ecs2.3022
112. Buchmann CM, Schurr FM, Nathan R, Jeltsch F. Habitat loss and fragmentation affecting mammal and bird communities - The role of interspecific competition and individual space use. Ecol Inform. (2013) 14:90–8. doi: 10.1016/j.ecoinf.2012.11.015
113. McLean M, Stuart-Smith RD, Villéger S, Auber A, Edgar GJ, MacNeil MA, et al. Trait similarity in reef fish faunas across the world's oceans. Proc Natl Acad Sci USA. (2021) 12:e2012318118. doi: 10.1073/pnas.2012318118
Keywords: community ecology, organism-based, Operational Ecological Unit, Trait-based Ecology, Agent-based Models, Maximum Entropy Theory of Ecology
Citation: Musters CJM, DeAngelis DL, Harvey JA, Mooij WM, van Bodegom PM and de Snoo GR (2023) Enhancing the predictability of ecology in a changing world: A call for an organism-based approach. Front. Appl. Math. Stat. 9:1046185. doi: 10.3389/fams.2023.1046185
Received: 16 September 2022; Accepted: 06 January 2023;
Published: 24 January 2023.
Edited by:
Ivo Siekmann, Liverpool John Moores University, United KingdomReviewed by:
Volker Grimm, Helmholtz Association of German Research Centres (HZ), GermanyCopyright © 2023 Musters, DeAngelis, Harvey, Mooij, van Bodegom and de Snoo. This is an open-access article distributed under the terms of the Creative Commons Attribution License (CC BY). The use, distribution or reproduction in other forums is permitted, provided the original author(s) and the copyright owner(s) are credited and that the original publication in this journal is cited, in accordance with accepted academic practice. No use, distribution or reproduction is permitted which does not comply with these terms.
*Correspondence: C. J. M. Musters, bXVzdGVyc0BjbWwubGVpZGVudW5pdi5ubA==
Disclaimer: All claims expressed in this article are solely those of the authors and do not necessarily represent those of their affiliated organizations, or those of the publisher, the editors and the reviewers. Any product that may be evaluated in this article or claim that may be made by its manufacturer is not guaranteed or endorsed by the publisher.
Research integrity at Frontiers
Learn more about the work of our research integrity team to safeguard the quality of each article we publish.