- 1Department of Mathematics, College of Science, University of Baghdad, Baghdad, Iraq
- 2Department of Mathematics and Faculty of Medicine, The University of Ottawa, Ottawa, ON, Canada
- 3School of Mathematical Science, The University of Nottingham Ningbo China, Ningbo, China
We propose an intraguild predation ecological system consisting of a tri-trophic food web with a fear response for the basal prey and a Lotka–Volterra functional response for predation by both a specialist predator (intraguild prey) and a generalist predator (intraguild predator), which we call the superpredator. We prove the positivity, existence, uniqueness, and boundedness of solutions, determine all equilibrium points, prove global stability, determine local bifurcations, and illustrate our results with numerical simulations. An unexpected outcome of the prey's fear of its specialist predator is the potential eradication of the superpredator.
Introduction
A functioning ecosystem depends on the framework of food webs that it supports [1]. Food webs involve many types of predator–prey interactions, which are fundamental interactions for sustaining species [2]. Interaction between the prey and the predator can be affected by factors such as refuge, disease, stage structure, competition, and fear [3–7]. Intraguild predation (IGP), on the other hand, is described as predator–prey interactions among consumers who may be fighting for limited resources. In natural communities, there is a growing body of literature emphasizing the relevance of intraguild predation [8, 9]. Three species are involved in the simplest intraguild predation model: a superpredator (IG predator), a specialist predator (IG prey), and a basal prey. Bai et al. recently suggested a three-species IGP food web model with the IG predator, IG prey, and basal prey, in which the basal prey grows logistically with a large Allee effect [10]. They looked into the model's local and global dynamics, focusing on the impact of the Allee effect and discovered that the intraguild predation food web model has rich and complicated dynamic behavior and that a large Allee effect in the basal prey raises the danger of extinction for not only the basal prey but also the IG prey or/and IG predator.
Many predators in food webs are superpredators, who may not restrict their diets to a specific prey species but feed also on other predators [11]. Therefore, superpredators are expected to compete not only with other predators for food and space but in many cases also through intraguild predation [5, 12, 13]. Predators induce indirect effects such as fear in prey that can change the prey's behavior [14]. Fear takes the form of sustained psychological stress on the prey, as prey species are always wary of possible attack [15]. Suraci et al. experimentally showed that fear of large carnivores reduces mesocarnivore foraging, which benefits the mesocarnivore's prey [16].
A handful of mathematical models have studied the effect of fear on food webs. Panday et al. investigated the impact of fear in a tri-trophic food chain model, with prey fear in response to both predators [15], from the middle predator to the generalist predator [17] and with delays [18]. Cong et al. introduced a fear-adjusted birth rate to a three-species food web [19]. Hossain et al. limited the growth of the prey due to fear in an intraguild predation model [20]. Mukerjee incorporated interspecific competition and fear affecting the death rate of the prey [21]. Ibrahim et al. limited prey growth due to fear of the generalist predator and showed that fear could have a stabilizing effect on the system [22]. Mondal et al. showed that the prey's fear of predators was responsible for the increase in intraspecific competition among the prey species [23]. Roy et al. showed that fear could play a destablizing role if it caused a reduction in the birth rate of susceptible prey, whereas the levels of fear responsible for the increase in the intraspecies competition of susceptible prey and eradication of the disease prevalence could stabilize an otherwise unstable system [24]. Maity et al. considered time-varying fear effects, showing that periodic solutions could arise [25]. Hossain et al. showed that perceived fear of predators could reduce the prey birth rate [26]. Tiwari et al. showed that seasonal variations in the level of prey fear generated higher-order periodic solutions [27].
Here, we examine the impact of fear on the dynamical behavior of an IGP food web system in which the prey responds with fear to the specialist predator but not the superpredator; because the superpredator has alternative food sources, it will be less dangerous to the basal prey compared to the IG prey. To the best of our knowledge, this is the first model to examine this effect.
Food-web model formulation
Our food web consists of a prey at the first level, a specialist predator at the second level, and a superpredator at the third level. Let x(t), y(t), and z(t) be the population densities at time t for the prey, specialist predator, and superpredator, respectively. The prey grows logistically in the absence of predators, while it has a fear property of predation in the presence of the specialist predator. Hence, the intrinsic growth rate of prey becomes , which is a monotonic decreasing function of both k and y, where k represents the fear rate [28]. The food transport attack rates are given by the parameters a1, a2, and a3, with conversion rates e1, e2, and e3. Finally, the predators face natural death rates d1 and d2 for the specialist predator and superpredator, respectively. The dynamics of the food web with fear can be represented mathematically by the following set of differential equations:
Initial conditions satisfy x(t) ≥ 0, y(t) ≥ 0, and z(t) ≥ 0, and all parameters are assumed to be positive.
The interaction functions fi (i = 1, 2, 3) are continuous and have continuous partial derivatives and are thus Lipschitzian, so system (1) has a unique solution. Furthermore, for any initial condition in , the solution of system (1) is positive and uniformly bounded as shown in the following theorem. Hence, system (1) will be a dissipative system.
Theorem (1): The domain of system (1), , is positively invariant, and all solutions of system (1) starting in are uniformly bounded.
Proof. Let x(t), y(t), and z(t) be any solution of system (1). Since the solution (x(t), y(t), z(t)) of the system (1) with initial condition in exists and is unique on [0 , δ), where 0 < δ ≤ +∞, we have:
From the first equation of system (1):
Then, it is easy to verify that for all t.
Let = x + y + z. Then, since ei ∈ (0, 1); i = 1, 2, 3, we have
,
where M = min{1, d1, d2}. Solving the following linear differential inequality
we obtain that, as t → ∞,
Existence of equilibrium points
System (1) has at most five nonnegative biologically feasible equilibrium points. The trivial equilibrium E0 = (0, 0, 0) always exists. The axial equilibrium E1 = (1, 0, 0) always exists on the boundary of the first octant. The specialist-free equilibrium point, , where
exists provided that
The superpredator-free equilibrium point, , where
exists, provided that
There is also an interior equilibrium point, , where
with x* a positive root of the following second-order polynomial equation:
where
Therefore, there is a unique interior equilibrium point in the interior of provided that the following conditions hold:
Persistence
Next, we determine the requirements that ensure persistence in the system (1). Because the types of attractors available in the boundary planes affect the creation of our IGP food web model's persistence conditions, an analysis of the dynamics in the boundary planes of the IGP food web system is conducted. System (1) has two subsystems: the first occurs in the absence of the superpredator (IG predator) and the second occurs in the absence of the specialist predator (IG prey). The first subsystem is
The second subsystem can be written as follows:
Subsystem (5) has a unique positive equilibrium point in the interior of xy-plane given by , while subsystem (6) has a unique positive equilibrium point in the interior of xz-plane given by . The interior equilibrium point of subsystem (5) corresponds to system (1)'s superpredator-free equilibrium point; similarly, the interior equilibrium point of subsystem (6) corresponds to system (1)'s specialist-predator-free equilibrium point. The dynamics around these equilibrium points can be described in the following theorem.
Theorem (2): There are no periodic dynamics in the interior of xy-plane or the xz-plane.
Proof. Define a continuously differential function . Then, we have:
Clearly, Δ has the same sign and does not equal zero almost everywhere in a simply connected region of the xy-plane. By the Dulac–Bendixson criterion, system (1) has no periodic solutions lying entirely in the interior of xy−plane. The second part follows by using the Dulac function .
Theorem (3): System (1) is uniformly persistent in the interior of provided that
Proof. Consider the function , where bi, i = 1, 2, 3, are positive constants and U(x, y, z) is a C1 nonnegative function in the interior of . Hence, we have
,
with
Therefore,
Since there are no periodic solutions in the boundary planes of the system (1), it follows that system (1) is uniformly persistent provided that Ψ(Ei) > 0 for each i = 1, 2, 3. We have
Consequently, conditions (7a)–(7d) satisfy Ψ(Ei) > 0 for each i = 1, 2, 3.
Global stability analysis
Here, we use Lyapunov functions to investigate the global stability of equilibria.
Theorem (4): The axial equilibrium point E1 = (1, 0, 0) is globally asymptotically stable provided that the following sufficient condition holds:
Proof. Consider the following positive-definite, real-valued function around E1:
Then, we have:
Then, by choosing c1 = e1, c2 = 1 and , we obtain
Clearly, under condition (8), is negative definite. Moreover, since V1 is radially unbounded, the axial equilibrium point E1 = (1, 0, 0) is globally asymptotically stable.
Theorem (5): The specialist-free equilibrium point is globally asymptotically stable provided that the following sufficient conditions hold:
Proof. Consider the following positive-definite, real-valued function around E2:
Then, we have:
Then, by choosing c1 = 1, and , we have:
Obviously, under conditions (9a)–(9b), is negative definite. Moreover, since V2 is radially unbounded, the specialist-free equilibrium point is globally asymptotically stable.
Theorem (6): The superpredator-free equilibrium point is globally asymptotically stable, provided that, in addition to condition (9b), the following sufficient conditions hold:
Proof. Consider the following positive-definite, real-valued function around E3:
Then, we have
where R = (1 + ky) and . Then, by choosing c1 = 1, and , we obtain:
Under conditions (10a)–(10b), is negative definite. Since V3 is radially unbounded, the superpredator-free equilibrium point is globally asymptotically stable.
Theorem (7): The coexistence equilibrium point is globally asymptotically stable provided that, in addition to condition (9b), the following sufficient condition holds:
Proof. Consider the following positive-definite, real-valued function around E4:
Then, we have:
where R* = 1 + ky*. Then, by choosing c1 = e2, c2 = e3, and c3 = 1, we have
Then, under condition (11) with (9b), is negative definite. Since V4 is radially unbounded, the coexistence equilibrium point is globally asymptotically stable.
Bifurcation analysis
In this section, we investigate the effect of varying parameter values on the dynamics of the system (1) using local bifurcation analysis and the Sotomayor theorem [29]. First, we rewrite system (1) in the vector form as follows:
The second derivative of F with respect to X can be written as:
where is a general vector.
Theorem (8): Assume that . Then system (1) undergoes a transcritical bifurcation at the axial equilibrium point E1, but neither a saddle node nor a pitchfork bifurcation can occur if
Proof. The Jacobian matrix of system (1) at the axial equilibrium point E1 with can be written as:
J1 has eigenvalues , under condition (13), and . Hence, the necessary but not sufficient condition for a bifurcation is satisfied, and E1 is a non-hyperbolic point.
Let be the eigenvector of J1 corresponding to the eigenvalue . Straightforward computation gives , where v13 represents any nonzero real number and .
Let be the eigenvector of corresponding to the eigenvalue . Direct calculation shows that , where ψ13 is any nonzero real number. Because , we obtain that , which yields:
By Sotomayor's theorem, system (1) at E1 with does not experience a saddle-node bifurcation. Moreover, we have
where DFd2 represents the derivative of Fd2 with respect to X. Applying equation (12) at with the eigenvector Φ1, we obtain that
By Sotomayor's theorem, system (1) near the equilibrium point E1 with undergoes a transcritical bifurcation, but a pitchfork bifurcation cannot occur.
Theorem (9): System (1) at the specialist-free equilibrium point E2 undergoes a transcritical bifurcation when , but neither a saddle-node nor a pitchfork bifurcation can occur, provided
Proof. The Jacobian matrix of system (1) at the specialist-free equilibrium point E2 with takes the form:
J2 has eigenvalues
while ; hence, the necessary but not sufficient condition for a bifurcation is satisfied, and E2 is a non-hyperbolic point.
Let be the eigenvector of J2 corresponding to the zero eigenvalue. Straightforward computation gives , where v22 represents any nonzero real number, with
Let be the eigenvector of corresponding to the zero eigenvalue. Direct calculation shows that , where ψ22 is any nonzero real number. Because , we obtain that , which yields
Thus, by Sotomayor's theorem, system (1) at E2 with does not experience a saddle-node bifurcation. Moreover, we have:
where DFd1 represents the derivative of Fd1 with respect to X. Using equation (12) at with the eigenvector Φ2, we obtain that
It is easy to verify that due to condition (14). Hence, by Sotomayor's theorem, system (1) near the equilibrium point E2 with undergoes a transcritical bifurcation but a pitchfork bifurcation cannot occur.
Theorem (10): At the superpredator-free equilibrium point E3, system (1) undergoes a transcritical bifurcation when , but neither a saddle-node nor a pitchfork bifurcation can occur, provided
Proof. The Jacobian matrix of system (1) at the superpredator-free equilibrium point E3 with is
J3 has eigenvalues
while ; hence, the necessary but not sufficient condition for bifurcation is satisfied, and E3 is a non-hyperbolic point.
Let be the eigenvector of J3 corresponding to the zero eigenvalue. Straightforward computation gives , where v33 represents any nonzero real number, while
Let be the eigenvector of corresponding to the zero eigenvalue. Direct calculation shows that , where ψ33 is any nonzero real number. Because , we obtain , which yields
Thus, by Sotomayor's theorem, system (1) at E3 with does not experience a saddle-node bifurcation. Moreover, we have:
where DFd2 represents the derivative of Fd2 with respect to X. By using equation (12) at with the eigenvector Φ3, we obtain that:
Straightforward computation shows that due to condition (15). Thus, by Sotomayor's theorem, system (1) near the equilibrium point E3 with undergoes a transcritical bifurcation, but a pitchfork bifurcation cannot occur.
Theorem (11): System (1) undergoes a saddle-node bifurcation at the coexistence equilibrium point E4, but neither a transcritical nor a pitchfork bifurcation can occur when e3 passes through the value , provided the following conditions hold:
Proof. Direct computation shows that system (1) at the coexistence equilibrium point and has Jacobian matrix
Straightforward computation shows that |J4| = 0, under condition (16a). Hence, J4 has two eigenvalues with negative real parts, and the third one is λ* = 0. It follows that E4 becomes a non-hyperbolic equilibrium point.
Let be the eigenvector of J4 corresponding to the eigenvalue λ* = 0. Straightforward computation gives , where v41 represents any nonzero real number, and .
Let be the eigenvector of corresponding to the eigenvalue λ* = 0. Direct calculation shows that , where ψ33 is any nonzero real number, with and .
Since , we have , which yields
By Sotomayor's theorem, transcritical and pitchfork bifurcations cannot occur while the first condition of the saddle-node bifurcation is satisfied. Moreover, from equation (12) with and Φ4, we obtain that:
Hence,
Further computation shows that
Using condition (16b), we have . Hence, system (1) has a saddle-node bifurcation at E4 when .
Theorem (12): System (2) undergoes a Hopf bifurcation around E4 when , if and only if the following conditions hold:
where
with and,
Proof. Consider the Jacobian matrix J4 given in Theorem (11), where . It is simple to determine that the characteristic equation is
Obviously, condition (17a) guarantees that C > 0. A straightforward computation shows that AB = C when . Hence, the characteristic equation (18) becomes
Consequently, we obtain that λ1 = −A and . Therefore, the Jacobian matrix has one negative real eigenvalue and two pure imaginary complex conjugates when . As a result, the first criterion for having a Hopf bifurcation is met.
Moreover, where e3 belongs to the neighborhood of , the eigenvalues become λ2,3 = δ1(e3) ± iδ2(e3).
Next, we substitute δ1(e3) + iδ2(e3) into equation (18) and take the derivative of the resulting equation with respect to e3. Equating their real and imaginary parts, we obtain that
where
Solving system (20) for gives
The result follows if and only if or, equivalently, H1(e3)H3(e3) + H2(e3)H4(e3) ≠ 0 when . Note that we have the following:
Consequently,
Condition (17b) ensures that .
Hence, the system has a Hopf bifurcation because under the conditions (17a)–(17b).
Numerical simulations
To illustrate the global dynamics of the system and confirm our analytical findings, we numerically simulated a hypothetical set of parameter values. Consider the following set of parameters:
Using these data, system (1) asymptotically approaches the coexistence equilibrium point E4 = (0.5, 0.2, 0.43), as shown in Figure 1.
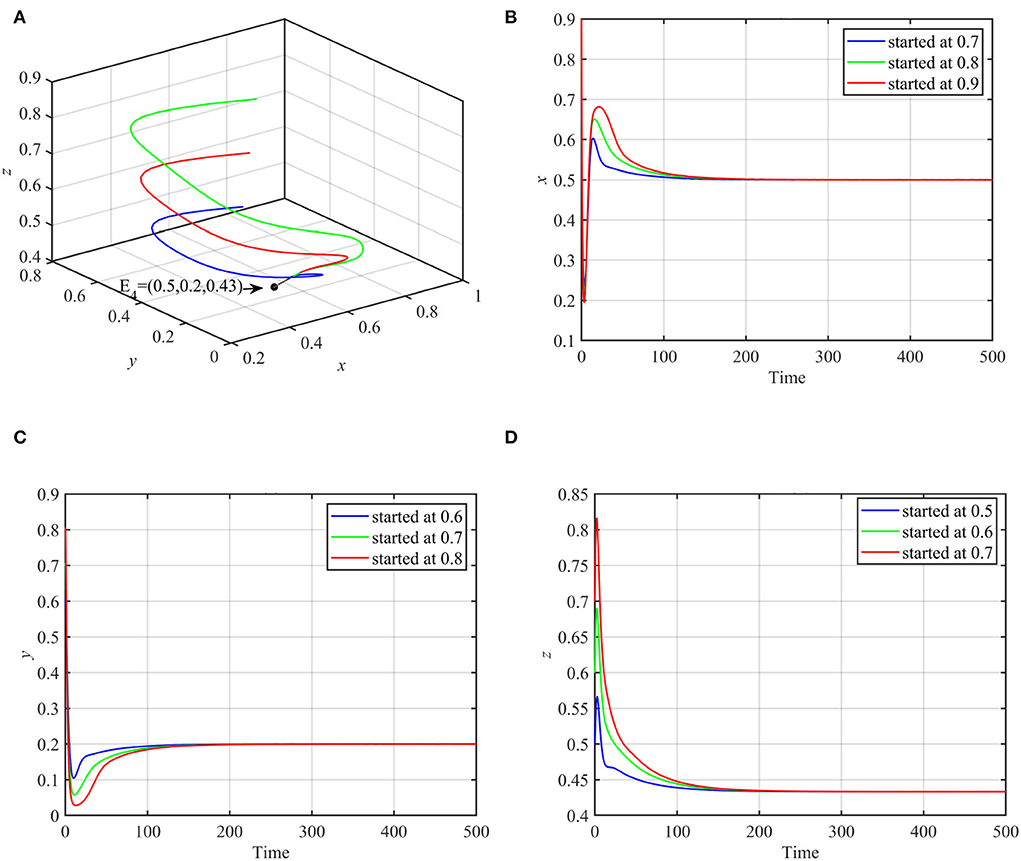
Figure 1. Globally asymptotically stable coexistence equilibrium point using data (17) and multiple initial conditions. (A) 3D-Phase portrait of the system (1). (B) Time series for trajectories of x. (C) Time series for trajectories of y. (D) Time series for trajectories of z.
Next, we varied specific parameters to understand the effect of that parameter on the dynamical behavior of the system. For the values of the parameter r satisfying r ≥ 1.84 and other parameters as in equation (17), system (1) approaches the specialist-free equilibrium point, as shown in Figure 2A for the typical value r = 2. For r ≤ 0.76 and other parameters as in equation (17), system (1) asymptotically approaches the superpredator-free equilibrium point, as shown in Figure 2B.
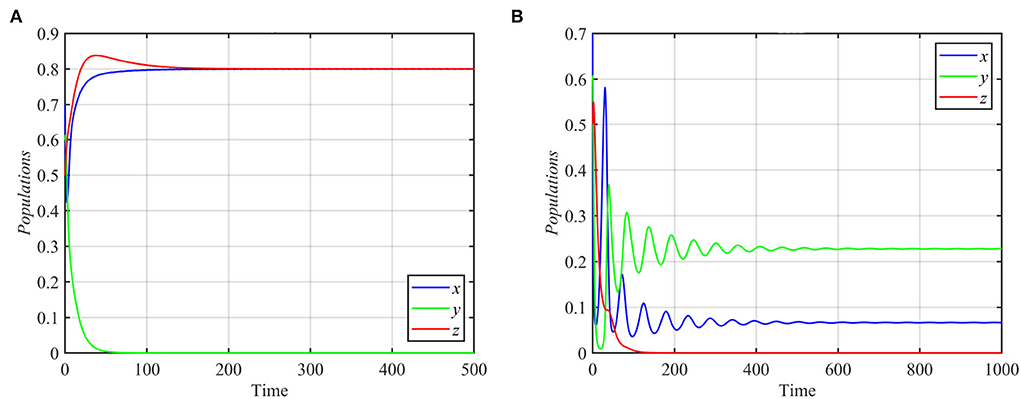
Figure 2. Trajectories of system (1) for different values of r, with other parameters as in equation (17). (A) Time series for trajectories of x, y, and z for r = 2, which approach E2 = (0.8, 0, 0.8). (B) Time series for trajectories of x, y, and z for r = 0.3, which approach E3 = (0.06, 0.22, 0).
For different values of the fear rate k with the rest of the data as given in equation (17), system (1) is solved numerically and illustrated in Figure 3.
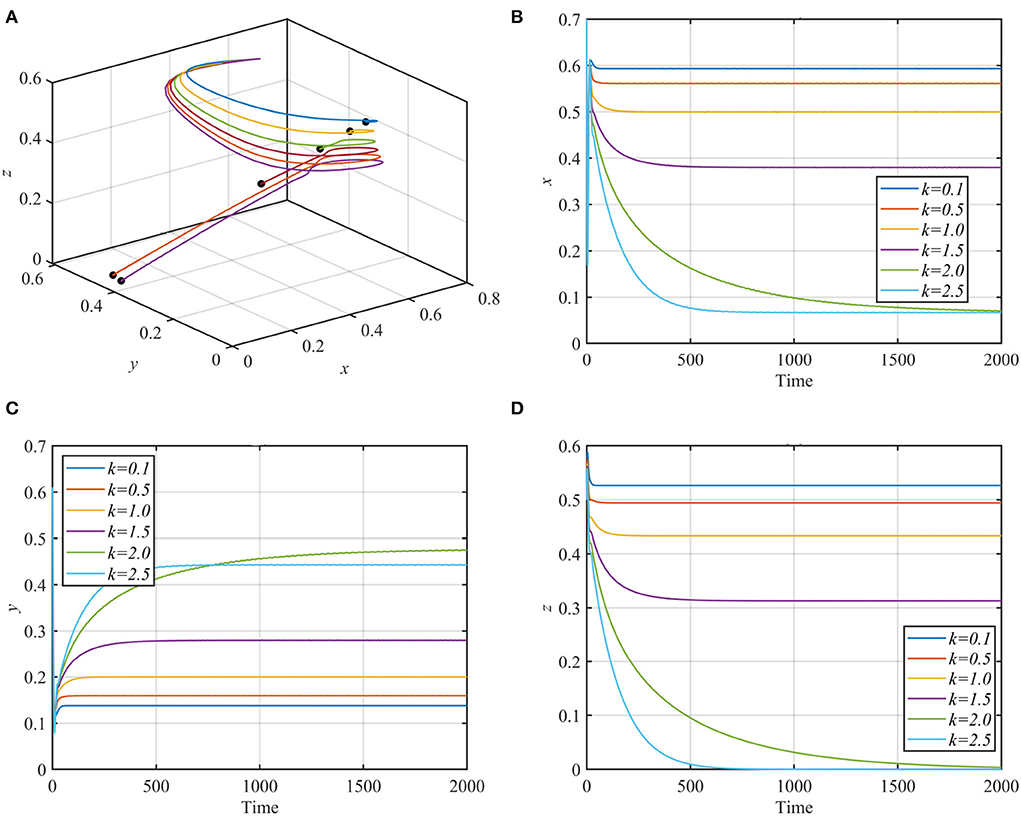
Figure 3. Trajectories of system (1) using data given in equation (17) with different values of k. (A) 3D-Phase portrait of system (1) for different values of k. (B) Time series for the trajectory of x, in which x decreases but remains positive as k increases. (C) Time series for the trajectory of y, in which y increases as k increases. (D) Time series for the trajectory of z, in which z decreases as k increases and approaches zero for k > 2.
As shown in Figure 2, the superpredator decreases as k increases, with extinction for k > 2. We also varied the parameter a1 with other parameters still fixed as in equation (17). For the range a1 ≥ 1.3, system (1) asymptotically approaches the superpredator-free equilibrium, as shown in Figure 4A for the typical value a1 = 1.75. Conversely, system (1) approaches asymptotically to the specialist-free equilibrium point, as shown in Figure 4B for the typical value a1 = 0.4.
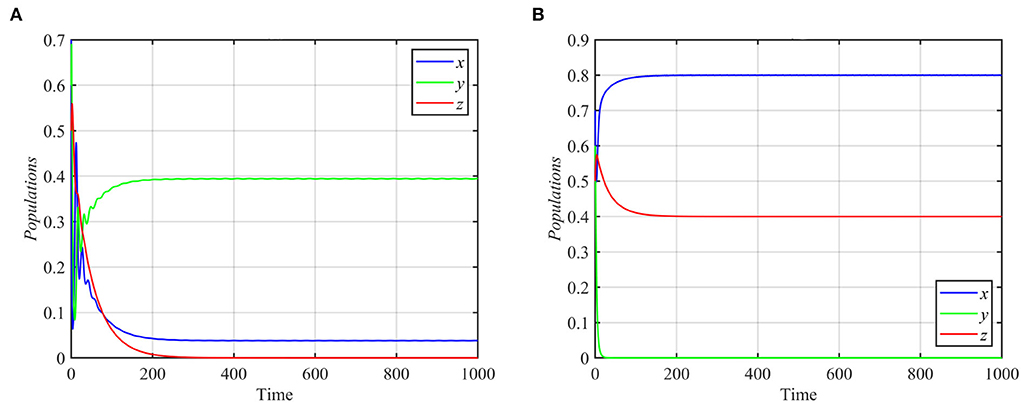
Figure 4. Trajectories of system (1) for different values of a1 and other parameters as in equation (17). (A) Time series for trajectories of x, y, and z for a1 = 1.75, which approach E3 = (0.03, 0.39, 0). (B) Time series for trajectories of x, y, and z for a1 = 0.4, which approach E2 = (0.8, 0, 0.4).
System (1) still asymptotically approaches the coexistence equilibrium point for the data given in equation (17), when e1 or e3 increases or when e2 decreases. However, for e1 ≤ 0.44, system (1) asymptotically approaches the specialist-free equilibrium point E2 = (0.8, 0, 0.4), as shown in Figure 4B. For e2 ≥ 0.3 or e3 ≤ 0.2 and the other parameters as in equation (17), the trajectories of system (1) asymptotically approach the specialist-free equilibrium point or the superpredator-free equilibrium point as shown in Figure 5.
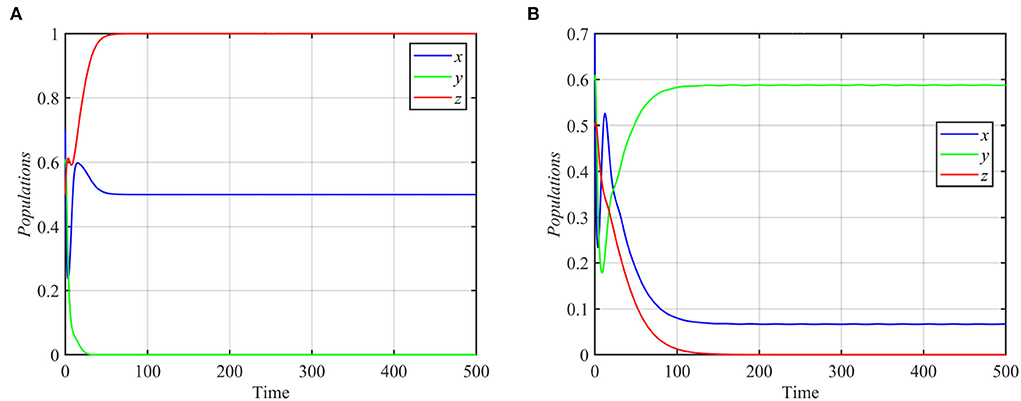
Figure 5. Trajectories of system (1) for different values of e2 and e3, with other parameters as in equation (17). (A) Time series for trajectories of x, y, and z for e2 = 0.4, which approach E2 = (0.5, 0, 1). (B) Time series for trajectories of x, y, and z for e3 = 0.1, which approach E3 = (0.06, 0.58, 0).
Further investigation for the effect of varying parameters a2 and a3 while keeping the rest of the parameters as in equation (17) shows that the parameter a2 has similar effects as the parameter e2 with a bifurcation point at a2 = 0.63. However, parameter a3 has similar effects as parameter r, with two bifurcation points: a3 = 1.38 and a3 = 0.62.
Finally, for d1 ≥ 0.31 and the rest of the parameters as in equation (17), the trajectories of system (1) asymptotically approach the specialist-free equilibrium point, as shown in Figure 6A for the typical value d1 = 0.4. However, system (1) approaches the coexistence equilibrium point otherwise. On the other hand, for d2 ≥ 0.12 or d2 ≤ 0.08 and the other parameters as in equation (17), trajectories of system (1) asymptotically approach either the superpredator-free equilibrium point or the specialist-free equilibrium point, as shown in Figures 6B,C for the typical values d2 = 0.15 and d2 = 0.08, respectively.
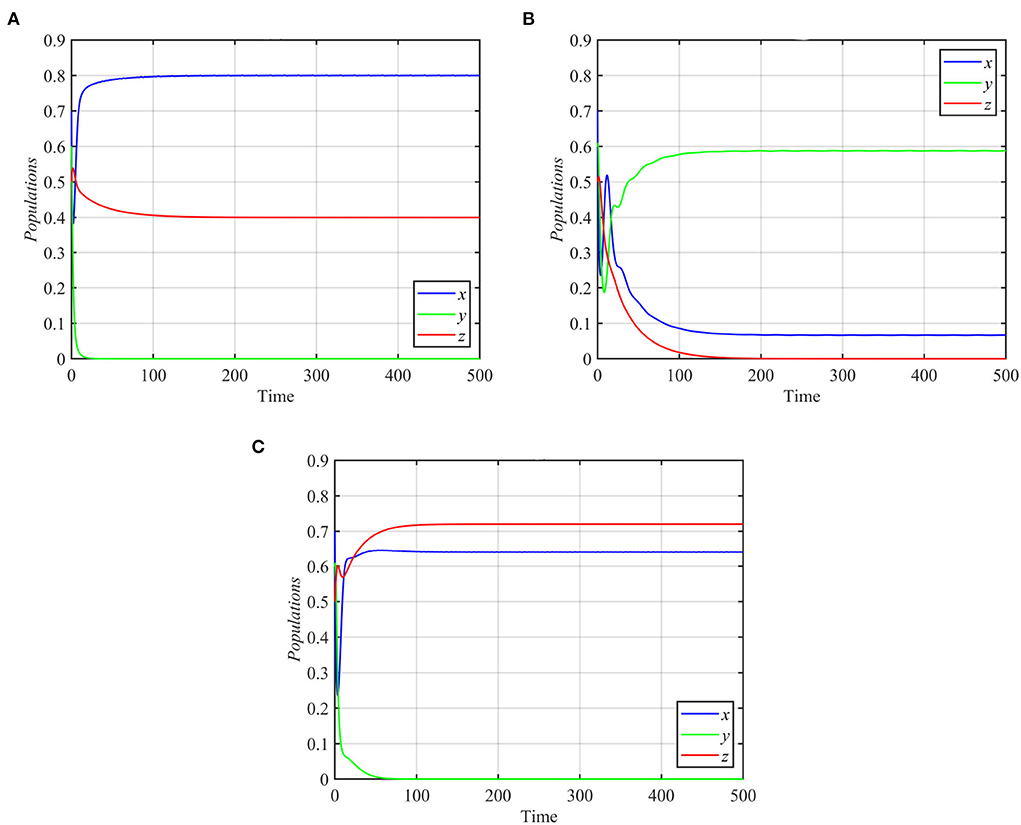
Figure 6. Trajectories of system (1) for different values of d1 and d2, with other parameters as in equation (17). (A) Time series for trajectories of x, y, and z for d1 = 0.4, which approach E2 = (0.8, 0, 0.4). (B) Time series for trajectories of x, y, and z for d2 = 0.15, which approach E3 = (0.06, 0.58, 0). (C) Time series for trajectories of x, y, and z for d2 = 0.08, which approach E2 = (0.64, 0, 0.72).
Varying parameters d1 and d2 together so that they satisfy conditions (7a) and (7b) while keeping other parameters as in equation (17) makes the trajectories of system (1) asymptotically approach the axial equilibrium point E1 = (1, 0, 0) as shown in Figure 7.
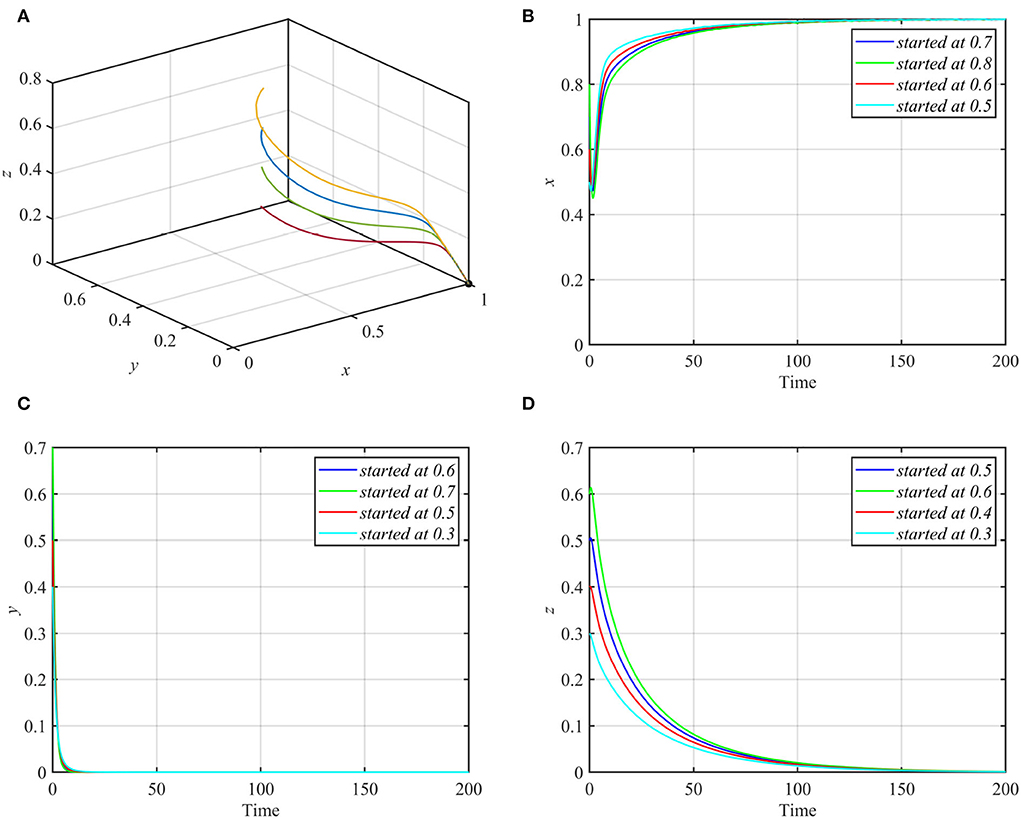
Figure 7. Globally asymptotically stable axial equilibrium point for d1 = 0.8, d2 = 0.15 and other parameters as in equation (17). (A) 3D-Phase portrait. (B) Time series for trajectories of x. (C) Time series for trajectories of y. (D) Time series for trajectories of z.
Discussion
Classical intraguild predation food web models have been extensively studied, but relatively little work has been done on the effect of fear on the prey. To the best of our knowledge, this is the first model considering prey fear of only the specialist predator. We determined the equilibria and global stability properties of the model, found bifurcations, and illustrated the theoretical behavior with numerical simulations.
Many previous studies have found that three-species intraguild predation models, in which a superpredator (IG predator) both attacks and competes with a specialist predator (IG prey), are often unstable, either because one consumer is excluded or because long feedback loops produce sustained oscillations [30]. Despite this, many natural IGP systems continue to thrive. Many empirical intraguild predation systems are entrenched in communities with alternative prey species, and standard models of intraguild predation simplify actual systems in significant ways that could affect persistence. Holt and Huxel [30] presented results of theoretical explorations of how alternative prey can influence the persistence and stability of a focal intraguild predation interaction. They reviewed the key conclusions of standard three-species IGP theory and then presented results of theoretical explorations of how alternative prey can influence the persistence and stability of a focal intraguild predation interaction. Conversely, Bai et al. [10] indicated that the intraguild predation food web model has rich and complicated dynamic behavior, and a large Allee effect in the basal prey raises the extinction risk of not only the basal prey but also the IG prey or/and IG predator.
Hossain et al. investigated fear in an intraguild predation model, in which the growth rate of a specialist predator (IG prey) is reduced due to the cost of fear of a superpredator (IG predator), and the growth rate of a basal prey is suppressed due to the cost of fear of both the IG prey and the IG predator [20]. In a three-species food chain system, they found that omnivory can cause chaos in the absence of fear. Fear, on the other hand, can help to keep the chaos under control. They also discovered that the system exhibits bistability between the IG prey-free and IG predator-free equilibrium, as well as bistability between IG prey-free and interior equilibria. Furthermore, they showed that the system can display numerous stable limit cycles for a given set of parameter values.
However, in our study, instilling the fear of a specialist predator (IG prey) in the presence of a superpredator (IG predator) had the unintended consequence of eradicating the superpredator. This demonstrates the often-surprising complexity of food-chain dynamics and has not been observed in previous articles dedicated to the study of fear [23–27]. In contrast, if the prey's intrinsic growth rate is sufficiently high, the IG prey can be eradicated, while the IG predator can be removed if the prey's intrinsic growth rate is sufficiently low. Coexistence or the eradication of both predators are other possibilities. As a result, system (1) has rich dynamical behavior, is sensitive to parameter changes, and at least one bifurcation point exists for each of the parameters. This result is comparable to decreasing the productivity of a resource (comparable to a higher fear effect in the prey in this manuscript) resulting in a reduction in the density of the highest trophic level [31].
The most important parameters that influenced the outcome were, in order, the growth rate r, the fear effect k, predation upon the prey by the specialist predator a1, the conversion factor between the prey and the superpredator e2, the conversion factor between the specialist predator and the superpredator e3, the death rate of the specialist predator d1, and the natural death rate of the superpredator d2.
Our model contains several limitations, which should be acknowledged. We only looked at the prey's fear of the specialized predator, not of the superpredator, and we ignored the specialist predator's fear of the superpredator. Our interpretation of such a case is that the pressure of the superpredator on the basal prey is much lower than that of a specialist predator due to the existence of alternative food sources. Mass-action kinetics and a conversion factor are used to model each predator's attack rates, which is a simplification of the genuine dynamics. Finally, the predators were completely reliant on a single food source, which is not always the case.
As a result, the dynamics of a food web in which the prey is afraid of one predator but not the other can produce unexpected results. In the absence of other fear effects, future research will consider the prey's fear of the superpredator but not the specialized predator; the specialist predator's fear of the superpredator could also be incorporated.
Data availability statement
The original contributions presented in the study are included in the article/supplementary files, further inquiries can be directed to the corresponding author.
Author contributions
NF developed the model, wrote the initial draft, conducted analysis, and performed numerical simulations. RN conducted analysis and performed numerical simulations. MH designed the study. SS? rewrote and edited and manuscript. All authors contributed to the article and approved the submitted version.
Funding
SS? is supported by an NSERC Discovery Grant. For citation purposes, please note that the question mark is part of her name.
Conflict of interest
The authors declare that the research was conducted in the absence of any commercial or financial relationships that could be construed as a potential conflict of interest.
Publisher's note
All claims expressed in this article are solely those of the authors and do not necessarily represent those of their affiliated organizations, or those of the publisher, the editors and the reviewers. Any product that may be evaluated in this article, or claim that may be made by its manufacturer, is not guaranteed or endorsed by the publisher.
References
1. Thompson RM, Brose U, Dunne JA, Hall RO, Hladyz S, Kitching RL, et al. Food webs: reconciling the structure and function of biodiversity. Trends Ecol Evolut. (2012) 27:689–697. doi: 10.1016/j.tree.2012.08.005
2. Allesina S, Tang S. Stability criteria for complex ecosystems. Nature. (2012) 483:205–08. doi: 10.1038/nature10832
3. Aziz-Alaoui MA. Study of a leslie gower type tri-trophic population model. Chaos Solitons Fractals. (2002) 14:1275–1293. doi: 10.1016/S0960-0779(02)00079-6
4. Gakkhar S, Singh B, Naji RK. Dynamical behavior of two predators competing over a single prey. BioSystem. (2007) 90:808–817. doi: 10.1016/j.biosystems.2007.04.003
5. Gakkhar S, Naji RK. On a food web consisting of a specialist and generalist predator. J Biol Syst. (2003) 11:365–376. doi: 10.1142/S0218339003000956
6. Gakkhar S, Naji RK. Order and chaos in a food web consisting of a predator and two independent preys. Commun Nonlinear Sci Numer Simulat. (2005) 10:105–120. doi: 10.1016/S1007-5704(03)00120-5
7. Klebanoff A, Hastings A. Chaos in one predator two prey model: general results from bifurcation theory. Math. Biosciences. (1994) 122:221–223. doi: 10.1016/0025-5564(94)90059-0
8. Holt RD, Polis GA. A theoretical framework for intraguild predation. Am Natur. (1997) 149:745–764. doi: 10.1086/286018
9. Tanabe K, Namba T. Omnivory creates chaos in simple food web models. Ecology. (2005) 86:3411–3414. doi: 10.1890/05-0720
10. Bai YK, Ruan S, Wang L. Dynamics of an intraguild predation food web model with strong Allee effect in the basal prey. Nonlinear Anal Real World Appl. (2021) 58:103206. doi: 10.1016/j.nonrwa.2020.103206
11. Sabelis MW. Predatory arthropods. In: Natural enemies, the population biology of predators, parasites and diseases, eds M. J. Crawley (Oxford: Blackwell Scientific) (1992). p. 225–264. doi: 10.1002/9781444314076.ch10
12. Crowder DW, Snyder WE. Eating their way to the top? Mechanisms underlying the 456 success of invasive generalist predators. Biol Invas. (2010) 12:2857–2876. doi: 10.1007/s10530-010-9733-8
13. Schreiber SJ. Generalist and specialist predators that mediate permanence in ecological communities. J. Math. Biol. (1997) 36:133–148 doi: 10.1007/s002850050094
14. Lima S, Dill LM. Behavioral decisions made under the risk of predation: a review and prospectus. Can. J. Zool. (1990) 68:619–640. doi: 10.1139/z90-092
15. Panday P, Pal N, Samanta S, Chattopadhyay J. Stability and bifurcation analysis of a three-species food chain model with fear. Int J Bifurcation Chaos. (2018) 28:1850009. doi: 10.1142/S0218127418500098
16. Clinchy LMDi, Roberts D, Zanette LY. Fear of large carnivores causes a trophic cascade. Nat Commun. (2016) 7:10698. doi: 10.1038/ncomms10698
17. Panday NP, Samanta S, Chattopadhyay J. A three species food chain model with fear induced trophic cascade. Int J Appl Comput Math. (2019) 5:1–26. doi: 10.1007/s40819-019-0688-x
18. Panday P, Samanta S, Pal N, Chattopadhyay J. Delay induced multiple stability switch and chaos in a predator–prey model with fear effect. Math Comput Simulat. (2020) 172:134–58. doi: 10.1016/j.matcom.2019.12.015
19. Cong MF, Zou X. Dynamics of a three-species food chain model with fear effect. Commun Nonlinear Sci Numer Simulat. (2021) 99:105809. doi: 10.1016/j.cnsns.2021.105809
20. Hossain NP, Samanta S, Chattopadhyay J. Fear induced stabilization in an intraguild predation model international. J Bifurcation Chaos. (2020) 30:2050053. doi: 10.1142/S0218127420500534
21. Mukherjee D. Role of fear in predator–prey system with intraspecific competition. Math Comput Simulat. (2020) 177: 263–275. doi: 10.1016/j.matcom.2020.04.025
22. Ibrahim HA, Naji RK. Chaos in beddington–deangelis food chain model with fear. J Phys Conf Ser. (2020) 1591:012082. doi: 10.1088/1742-6596/1591/1/012082
23. Mondal B, Roy S, Ghosh U, Tiwari PK. A systematic study of autonomous and nonautonomous predator–prey models for the combined effects of fear, refuge, cooperation and harvesting. Eur Phys J Plus. (2022) 137:724. doi: 10.1140/epjp/s13360-022-02915-0
24. Roy S, Tiwari PK, Nayak H, Martcheva M. Effects of fear, refuge and hunting cooperation in a seasonally forced eco-epidemic model with selective predation. Eur Phys J Plus. (2022) 137:1–31. doi: 10.1140/epjp/s13360-022-02751-2
25. Maity PKTi, Pal S. An ecoepidemic seasonally forced model for the combined effects of fear, additional foods and selective predation. J Biol Syst. (2022) 12:1–37. doi: 10.1142/S0218339022500103
26. Pal PKTi, Pal N. Bifurcations, chaos, and multistability in a nonautonomous predator–prey model with fear Chaos: an interdisciplinary. J Nonlinear Sci. (2021) 31:123–34. doi: 10.1063/5.0067046
27. Tiwari PK, Verma M, Pal S, Kang Y, Misra AK. A delay nonautonomous predator–prey model for the effects of fear, refuge and hunting cooperation. J Biol Syst. (2021) 29:927–69. doi: 10.1142/S0218339021500236
28. Wang X, Zanette L, Zou X. Modelling the fear effect in predator–prey interactions. J Math Biol. (2016) 73:1179–1204. doi: 10.1007/s00285-016-0989-1
29. Perko L. Differential Equations and Dynamical Systems, Volume 7. New York: Springer (1996). doi: 10.1007/978-1-4684-0249-0
30. Holt RD, Huxel GR. Alternative prey and the dynamics of intraguild predation: theoretical perspectives. Ecology. (2007) 88:2706–2712. doi: 10.1890/06-1525.1
Keywords: intraguild predation, food web, fear effect, stability, specialist predator
Citation: Fakhry NH, Naji RK, Smith? SR and Haque M (2022) Prey fear of a specialist predator in a tri-trophic food web can eliminate the superpredator. Front. Appl. Math. Stat. 8:963991. doi: 10.3389/fams.2022.963991
Received: 08 June 2022; Accepted: 05 October 2022;
Published: 03 November 2022.
Edited by:
Aurelio A. de los Reyes V, University of the Philippines Diliman, PhilippinesReviewed by:
Pankaj Tiwari, University of Kalyani, IndiaAbdelalim A. Elsadany, Suez Canal University, Egypt
Copyright © 2022 Fakhry, Naji, Smith? and Haque. This is an open-access article distributed under the terms of the Creative Commons Attribution License (CC BY). The use, distribution or reproduction in other forums is permitted, provided the original author(s) and the copyright owner(s) are credited and that the original publication in this journal is cited, in accordance with accepted academic practice. No use, distribution or reproduction is permitted which does not comply with these terms.
*Correspondence: Mainul Haque, Mainul.Haque@nottingham.edu.cn