Editorial: Data driven modeling in mathematical biology
- 1CHU Rennes, INSERM, LTSI–UMR 1099, Univ Rennes, Rennes, France
- 2Department of Cardiovascular Disease, KU Leuven, Leuven, Belgium
Introduction: Although observational studies of patients with left bundle branch block (LBBB) have shown a relation between strain morphologies and responses to cardiac resynchronization therapy (CRT), the evaluation of left ventricle (LV) dyssynchrony from echocardiography remains difficult. The objective of this article is to propose a patient-specific model-based approach to improve the analysis and interpretation of myocardial strain signals.
Methods: A system-level model of the cardiovascular system is proposed, integrating: (i) the cardiac electrical system, (ii) right and left atria, (iii) a multi-segment representation of the RVs and LVs, and (iv) the systemic and pulmonary circulations. After a sensitivity analysis step, model parameters were identified specifically for each patient. The proposed approach was evaluated on data obtained from 10 healthy subjects and 20 patients with LBBB with underlying ischemic (n = 10) and non-ischemic (n = 10) cardiomyopathies.
Results: A close match was observed between estimated and observed strain signals, with mean RMSE respectively equal to 5.04 ± 1.02% and 3.90 ± 1.40% in healthy and LBBB cases. The analysis of patient-specific identified parameters, based on bull's-eye representation, shows that strain morphologies are related to both electrical conduction delay, and heterogeneity of contractile levels within the myocardium.
Discussion: The model-based approach improve the interpretability echocardiography data by bringing additional information on the regional electrical and mechanical function of the LV. The analysis of model parameters show that septal motion and global strain morphologies are not only explained by electrical conduction delay but also by the heterogeneity of contractile levels within the myocardium. The proposed approach represents a step forward in the development of personalized LV models for the evaluation of LV dyssynchrony in the field of CRT.
1. Introduction
Left bundle branch block (LBBB) is a common electrocardiographic abnormality that causes intra- and interventricular conduction delay and leads to uncoordinated contraction of the ventricle, alterations in LV mechanical activity, and LV dysfunction [1]. Observational studies of patients with LBBB have shown a relation between strain curve morphologies, obtained by speckle-tracking echocardiography (STE), and responses to cardiac resynchronization therapy (CRT) [2–4]. However, the regional distribution patterns of dyssynchrony in LBBB are highly heterogeneous as it involves different septal and lateral walls [5, 6]. Moreover, strain morphologies could also be affected by mechanical dysfunctions, such as those observed in ischemia [7]. Therefore, the assessment of dyssynchrony patterns in LBBB appears particularly complex because strain morphologies reflect dynamics associated with both electrical conduction delays and mechanical cardiac activities. Previous studies have shown that only the mechanical dysfunction attributable to an electrical conduction delay can be corrected by CRT [8]. The possibility of using strain-derived data to disclose the complex interplay between electrical conduction delay and the specific mechanical substrate associated with LV dyssynchrony is particularly interesting and might have a pivotal role in the selection of CRT candidates.
In this context, model-based approaches may provide a better understanding of myocardial deformations observed in LBBB, since these approaches explicitly represent the underlying physiological mechanisms. Indeed, computational modeling appears as an efficient tool to integrate knowledge, concerning cardiac electrical activation, mechanical properties, and hemodynamic conditions, in the data processing. A variety of cardiac electro-mechanical models has been proposed in the literature, at many different levels of detail [9] and representing different physiological functions, including the cardiac electrical activity [10–12], the excitation–contraction coupling [13, 14], the mechanical activity [15], and the mechano-hydraulic coupling [16]. Most of the proposed cardiac models are based on the finite element (FE) method [17–24] for the simulation of cardiac mechanical activity including a 3D mesh geometry. Some of them include multimodality imaging [25] or used atlases [26] to reduce the computational cost. However, these models require high computational resources, and they are still difficult to personalize. Moreover, dynamic loading conditions and interventricular interactions are usually not taken into account in these models and their integration is possible only at the expense of an increasing amount of model complexity. Alternative approaches have been proposed to overcome this computational cost [27–30], by reducing drastically the patient anatomy representation with lower dimension models. These types of models allow for a better clinical translation [31] and incorporation of components such as heart hemodynamics within the entire circulation. Although these particular models' examples have been successfully used to propose keys to understand the CRT response with virtual or animal cohorts, efforts still have to be made in order to adapt these studies to non-invasive, patient-specific data.
In Le Rolle et al. [32], our team has proposed the first model-based approach for the analysis of tissue Doppler imaging (TDI). Model parameters for the LV were estimated by minimizing an error computed between strain signals synthesized by the computational model and strain signals obtained through TDI from several myocardial segments in a patient-specific approach. However, this model represents only the LV and does not integrate interactions with the right ventricle (RV) or systemic and pulmonary circulations. Moreover, the approach was not validated in the case of LV dyssynchrony. The objective of this article is to propose a model-based approach in order to reproduce myocardial strain curves specifically for each patient with LBBB and to analyze patient-specific parameters. Therefore, a novel method is proposed, based on the patient-specific identification of a multi-segment model of LVs and RVs coupled to atria, systemic, and pulmonary circulations [33, 34]. Recently, our team article has proposed an interpretation of the different patterns of LV contraction observed in different cases of LBBB, based on a manual evaluation of the model parameters of three patients with LBBB [35]. Interestingly, this model took into account not only the electrical activation delay of each segment but also the differences in regional contractility, which are known to largely contribute to strain morphology and global cardiac mechanics.
The article is organized as follows: Section 2 describes the clinical data, the computational model, and patient-specific adaptation; Section 3 includes the results concerning subjects with and without LBBB; and in Section 4 results are discussed.
2. Materials and methods
2.1. Experimental data
2.1.1. Study population
We prospectively included 10 healthy adults and 20 patients with LBBB, including ischemic (n =10) and non-ischemic (n =10) cardiomyopathies. Table 1 summarizes patients' clinical characteristics. The study was carried out in accordance with the principles outlined in the Declaration of Helsinki on research in human subjects and received specific ethical approval from the local Medical Ethical Committee. All patients signed a written informed consent before participating in the study protocol.
2.1.2. Echocardiography
All patients underwent a standard trans-thoracic echocardiography (TTE) using a Vivid S6, E7, or E9 ultrasound system (General Electric Healthcare, Horten, Norway). Images were recorded on a remote station for offline analysis by the dedicated software (EchoPAC PC, version BT 202, General Electric Healthcare, Horten, Norway). The experimental dataset includes the measured regional myocardial strain curves obtained by STE at transthoracic echocardiography in apical four-chamber, two-chamber, and three-chamber views. Excel files of these three longitudinal strain view analyses were exported for a dedicated analysis performed in python language. Strain curves references were fixed at the onset of the QRS complex.
2.1.3. Cardiac magnetic resonance image
For 10 patients with LBBB, the location of the scar was performed by cardiac magnetic resonance imaging (cMRI) and then confirmed by echocardiography. Before CRT implantation, cardiac magnetic resonance was performed on a 3-T clinical magnetic resonance system (Ingenia, Philips Medical Systems, Best, the Netherlands) with a 32-channel cardiovascular array coil. LGE images were acquired 10–15 min after intravenous administration of 0.2 mmol/kg of gadolinium (Gadoterate meglumine, Dotarem, Guerbet, Aulnay-sous-bois, France), using 2D breath-hold inversion-recovery and phase-sensitive inversion-recovery sequences in the short-axis plane (spoiled gradient-echo, slice thickness 8 mm, repetition time 6.1 ms, echo time 2.9 ms, flip angle 25°, inversion time adjusted to null normal myocardium, and typical breath-hold 11 s). The localization of the myocardial scar was performed by a trained radiologist and the regional LGE extent was semiquantitatively assessed on a per-segment basis [36].
2.2. Computational model
The model of the cardiovascular system (CVS) integrates four main sub-models: (1) cardiac electrical system, (2) right and left atria, (3) multi-segment representation of the right ventricles (RVs) and the left ventricles (LVs), and (4) systemic and pulmonary circulations. The combined model is characterized by 44 state variables and 551 parameters. It was implemented using the Multiformalism Modeling and Simulation Library (M2SL) [37, 38]. Equations and parameters can be found in the Supplementary material.
2.2.1. Cardiac electrical system
The proposed model of the cardiac electrical activity is based on a set of coupled automata, adapted from Le Rolle [32]. In order to perform comparisons between simulations and clinical data, the LV wall was divided into 16 segments according to the standardized segmentation of the AHA [39]. The base (Bas) and medium (Mid) layers are separated in six components as follows: anterior (Ant), anteroseptal (AntSep), inferoseptal (InfSep), inferior (Inf), inferolateral (InfLat), and anterolateral (AntLat) walls. The apex (Ap) layer is divided in to four components as follows: anterior, septal, inferior, and lateral. The RV wall is divided into three layers (base, medium, and apex) (Figure 1). The whole model consists of 26 automata representing the sinoatrial node (NSA), right and left atria (RA and LA), the atrioventricular node (NAV), an upper bundle of His (UH), bundle branches (RBB and LBB), three segments of RV, and 16 segments of the LV.
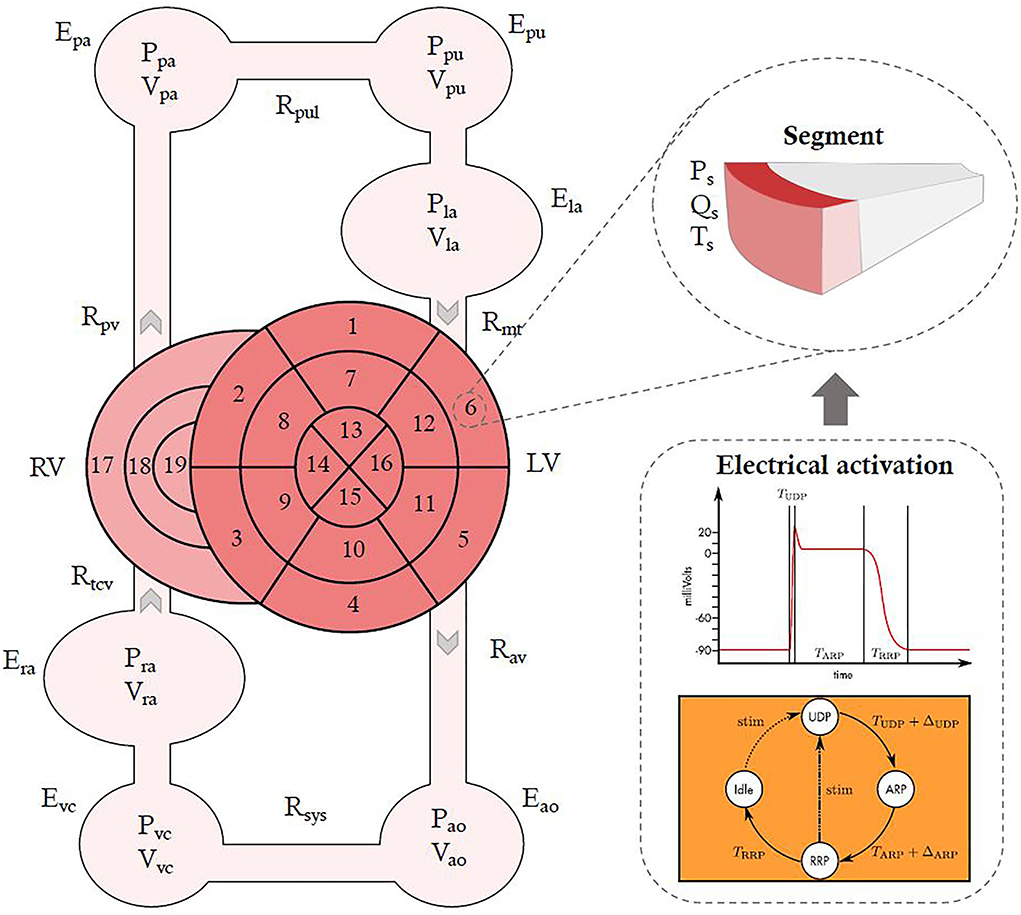
Figure 1. Closed-loop model of the cardiovascular system and representation of a myocardial segment. Vascular network: ao: aorta, vc: vena cava, pa: pulmonary artery, pu: pulmonary veins. Heart valves: mt: mitral, av: aortic, tc: tricuspide, pv: pulmonary. Left ventricle segmentation: 1: BasAnt; 2: BasAntSep; 3: BasInfSep; 4: BasInf; 5: BasInfLat; 6: BasAntLat; 7: MidAnt; 8: MidAntSep; 9: MidInfSep; 10: MidInf; 11: MidInfLat; 12: MidAntLat; 13: ApAnt; 14: ApSep; 15: ApInf; 16: ApLat. Right ventricle segmentation: 17: BasRV; 18: MidRV; 19: ApRV.
The distribution of the electrical activation between automata is represented in Figure 2. Each automaton represents the electrical activation state of a given myocardial tissue, covering the main electrophysiological activation periods: slow diastolic depolarization (SDD) or waiting period (Idle), upstroke depolarization (UDP), absolute refractory (ARP), and relative refractory (RRP) (Figure 2A). The transitions between states happen spontaneously at the end of the phase. After the UDP period, each automaton transmits a stimulus to its neighboring segments. Each automaton is fully connected (antegrade and retrograde connections) to its neighbors. The connections between automaton are illustrated in Figure 2B, where we can see that the excitation arrives from the LBB automaton and is propagated to the apex, through septal automata and the medium anterolateral automaton (segments numbered 2, 3, 8, 9, 12, 13, 14, and 16), then to the other segments in the function of each automaton's parameter values (TUDP, TARP, TRRP, and TSDD).
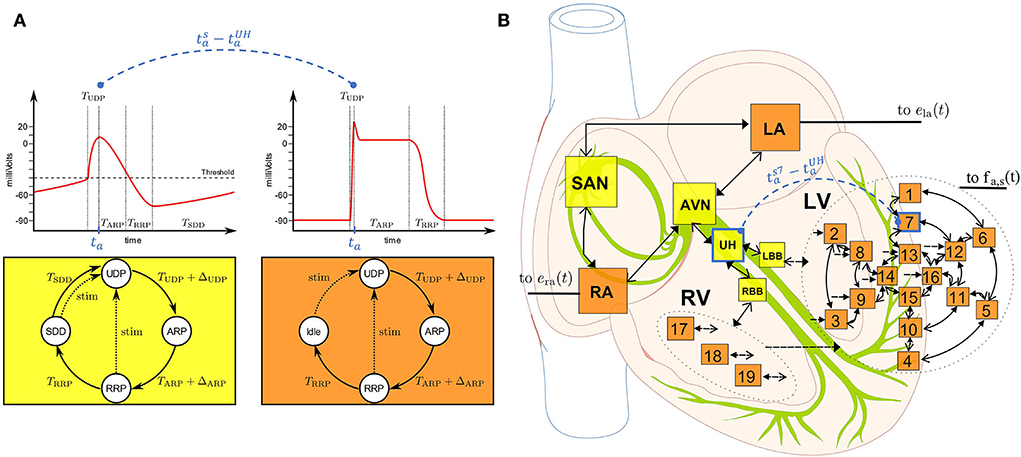
Figure 2. Cardiac electrical system model: (A) State diagram of the generalized automaton that represents nodal cells (yellow, left) and myocardial cells (orange, right) and diagrams showing the correspondence of the transition parameters with the myocardial action potential dynamics. (B) The whole heart is represented by 26 cellular automata and their sequence of electrical activation. In blue, a representation of the electrical activation delay for the seventh LV segment (s7).
The electrical activation delay associated with each ventricular segment could be defined by the time elapsed between the electrical activation of the UH automaton and the segmental one. An illustration of this electrical activation delay is proposed in Figure 2B for the seventh LV segment (s7). These delays of activation, accessible for each segment, will provide us with a representation of the dyssynchrony. UH automaton activation also corresponds to the initialization of the simulated strain curves.
2.2.2. Right and left atria
To account for the mechanical function of the atria, the right and left atrial pressures (Pra and Pla) are defined as linear functions of instantaneous volumes (Vra and Vla). These pressures are determined by their volumes intercept (Vra,d and Vla,d) and their elastances (Era and Ela), whose elastances represent the elastic properties of the atrial wall and are bounded by Ex,min and Ex,max :
where x ∈ {ra,la} and ex(t) is a Gaussian driving function that cycles between atrial diastole and systole:
where ta is the time elapsed since the atrial activation by the automata corresponding to the right and left atriums. Parameters Ax, Bx, and Cx could be used to control the rise and peak of the atrial systole.
2.2.3. Right and left ventricles
Each LV and RV automaton triggers an electro-mechanical driving function (EMDF) [40, 41], which represents in a simplified manner, the complex processes involved in the electro-mechanical coupling at the tissue level:
The onset of the cardiac cycle, denoted ts, is determined by the activation instant of the corresponding segment in the cardiac electrical model presented in the previous section. The first and second terms in Eq. 4 represent ventricle segment contraction and relaxation presented after an electrical activation, respectively. T is the heart period, α1, α2 are shape parameters, and n1, n2 control the steepness of the curve. These four parameters (α1, α2, n1, n2) are assumed positive. Amax is the maximum EMDF value, and s ∈ {Slv, Srv} with Slv = {BasAnt, BasAntSep, BasInfSep, BasInf, BasInfLat, BasAntLat, MidAnt, MidAntSep, MidInfSep, MidInf, MidInfLat, MidAntLat, ApAnt, ApSep, ApInf, ApLat} and Srv = {BasRV, MidRV, ApRV}.
Concerning each segment s, cardiac mechanical activity can be separated into active and passive components:
Passive myocardial tension depends on myocardial strain (εs = (ls − ls,ref)/ls,ref) according to Lumens et al. [42]:
where Kpass is a parameter related to passive stiffness that is comprised between 0 and 1, Tref,pass is the reference passive tension at εs = 1, ls and ls,ref are current and reference fiber lengths. Active myocardial tension is represented by a non-linear law inspired from Hunter et al. [43]:
where Kact is a parameter related to myofiber contractility, Tref,act is the reference active tension at εs = 1, and β, Fa are constants related to the muscle kinetic. The relation between pressure Ps and tension Ts in each segment is approximated by the Laplace law (Equation 8):
In Equation (8), θ is the mean angle of the muscular fibers. Rm and Rp are the radii of curvature in the meridian and parallel directions, while e is the mean wall thickness. As the ventricle was assumed to be an ellipsoid of revolution, Rp and Rm could be calculated analytically. Length variation is obtained by a power conservation: Ps · Qs = Fs · dls/dt. Where the force is Fs = Ts·Ss, Ss is the area of each segment. The hydraulic behavior of the blood volume in contact with the wall segment are represented by its inertial (Is) and resistive (Rs) effects:
with y ∈ {lv,rv} and Rs ∈ {Rmin,Rmax} according to the mitral valve opening. Ventricular flow is calculated taking into account the contribution of the flow of each one of the segments Qs,y and of the intra-ventricular cavity Qc,y:
where Py and Qy are, respectively, cavity center pressure and flow. Segments, associated with the septum, are treated separately since their pressure depends on the pressure gradient across the septal wall:
2.2.4. Systemic and pulmonary circulations
The arteries, veins, and capillaries of systemic and pulmonary circulations were included (Figure 1). The volume change, ΔV, of each compartment is computed from the integral of their respective net flow:
with z ∈ {lv, rv, la, ra, pa, pu, ao, vc}, and in and out ∈ { la, ra, pa, pu, ao, vc, sys, pul, art, veins}, while the flow, Q, is defined by the pressure gradient, ΔP, across chambers and a resistance, R:
R ∈ {Rpul, Rsys, Rart, Rveins, Rla, Rra, Rmt, Rav, Rtcv, Rpv}. Pressures on venous and arterial vessels are defined as an elastance dependent relationship:
Vd ∈ {Vd,lv, Vd,rv, Vd,la, Vd,ra, Vd,pa, Vd,pv, Vd,ao, Vd,art, Vd,vc, Vd,veins}, where E is the elastance and Vd refers to the dead volume. For example, these equations become as follows:
in the systemic part of the model (Figure 1 bottom). The same equations are applied all around the myocardial loop. The heart valves are modeled as perfect diodes.
2.2.5. Simulations of desynchronization strain patterns
Desynchronization strain patterns were obtained by modifying electrical activation delays of the LV segments (UDP) and the tissue contractility Kact. These parameter modifications could be done independently on each segment at the same time. As performed in Owashi et al. [35], model parameters were adjusted manually in order to reproduce characteristic strain patterns for a healthy subject and three patients with typical LBBB.
2.3. Patient-specific adaptation
2.3.1. Sensitivity analysis
The first step of patient-specific adaptation corresponds to the sensitivity analysis of the model in order to provide insight into the relation between parameters and outputs and to allow a characterization of the relative significance of each parameter. Using the Morris elementary effects method [44], the sensitivity of each parameter is estimated by repeated measurements of a simulation output Y with parameters X, while changing one parameter Xj at a time.
The analysis was applied to a total of 288 parameters with 18 parameters for each of the 16 segments: one from the electrical automaton and the 16 other one from the electro-mechanical coupling part of the segmental sub-model equivalent. The circulatory parameters were previously studied in Calvo et al. [33]. In the proposed article, our analysis is focused on the LV desynchrony and especially on the influence of electro-mechanical parameters on strain morphologies. In order to preserve computational costs, we have decided to include only parameters associated with the electro-mechanical activity of ventricles. For each parameter Xj, the range of possible values was selected from the nominal literature and previous work values ±30% [34, 45, 46], except for the electrical depolarization time parameter (UDP) that the range was defined between 2 and 150 ms. The resulting change in Y, compared to the simulation output using the initial values of X, is calculated by the elementary effect:
where Δ is a predefined variation, such as . The parameter p and the number of trajectories r were equal respectively to 6 and 30 in this study. EEj is calculated r times. Then, the mean (μ*) and standard deviation (σ) of these effects are computed to derive sensitivity information of each parameter j. In order to establish a global rank of importance among parameters, we calculated the Euclidean distance Dj in the μ* − σ plane, from the origin to each (, σj) point:
The analysis was performed with the following: , and , where and correspond, respectively, to the minimum value of strain and the corresponding time for each segment s. The mean and standard deviation values were calculated over the 16 strain signals.
2.3.2. Parameter identification
The second step of the patient-specific adaptation is the identification of a set of parameters selected from the sensitivity analysis. Figure 3 illustrates the parameters identification process.
For each healthy adult and patient with LBBB, an error function Jerror between simulation outputs and experimental strain curves was minimized in order to find patient-specific parameters:
where and are the myocardial strain signals obtained from available clinical data and simulated outputs, respectively. te corresponds to the time elapsed since the onset of the identification period and Tc is the duration of a cardiac cycle. To build this error function, experimental and simulated strain curves were synchronized on the onset of QRS of synthesized and clinical ECG. The error function Jerror was minimized using evolutionary algorithms (EA). These stochastic search methods are founded on theories of natural evolution, such as selection, crossover, and mutation [47]. In this study, a differential evolution (DE) algorithm [48] was applied to find the optimal set of parameters. In order to reduce the search space, values for parameters were bounded to the physiologically plausible intervals: IKact = [0;1], IKpass = [0;1], In1 = [0.5;2], In2 = [5;15], Iα1 = [0.2;0.6], Iα2 = [0.2;0.6], IUDP = [1;200]. These intervals were defined around parameter values used for the simulation of baseline conditions and are based on physiological knowledge of the electromechanical activities of the heart. DE was implemented with 200 individuals through 100 generations with crossover and mutation probabilities equals to 0.9 and 0.02 using the C++ library PAGMO (parallel global multiobjective framework for optimization) [49].
2.3.3. Solution unicity
In order to evaluate the robustness of our method, we repeated 10 times our identification process on five patients. Two patients of the healthy and LBBB ischemic population and one in the LBBB non-invasive population were randomly chosen for this evaluation. In fact, a different set of parameters could give similar simulated strain curves. The 10 obtained sets of parameters were analyzed to justify the solution unicity of the identification process by comparing the distribution of each parameter pi in its own value interval Ipi. For each parameter, the ratio of the standard deviation over its value interval length was calculated. Then, the average was calculated over the 16 LV segments and expressed as the percentage:
where , , , , ,, UDPs} and s ∈ {BasAnt, BasAntSep, BasInfSep, BasInf, BasInfLat, BasAntLat, MidAnt, MidAntSep, MidInfSep, MidInf, MidInfLat, MidAntLat, ApAnt, ApSep, ApInf, ApLat}.
2.3.4. Quantification of error between simulated and clinical data
In order to compare simulated and clinical strain curves, the root-mean-square error (RMSE) was calculated for each segment s :
A mean RMSE value, over the 16 segments, was calculated for each subject. Moreover, bull's-eye plot was used to describe mean RMSE values calculated for each segment over each population: patients with healthy, ischemic LBBB, and non-ischemic LBBB.
3. Results
3.1. Baseline simulations
Figure 4 illustrates a simulation results' example from the proposed computational model with a set of parameters determined in previous work and literature (the set of parameter values are included in Supplementary material). Ventricular, aortic, and atrial pressures as well as ventricular volume are presented on the left of the figure. Myocardial strain signals corresponding to the 16 LV segments are presented on the right of the figure. The results are presented for a healthy case. Systolic LV pressure is equal to 120 mmHg and the aortic pressure varies between 50 and 120 mmHg. The LV volume varies between 70 and 130 mL. The strain signals present similar morphologies between all the segments due to the mechanical synchronicity between them. Generally, simulation results agree with the physiological values and behaviors of a healthy subject.
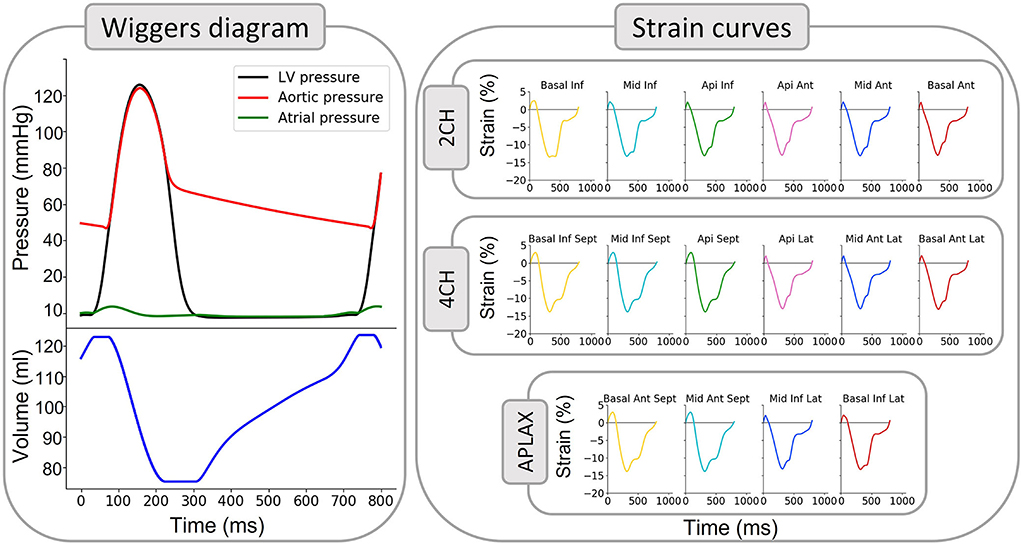
Figure 4. Model simulation example results in healthy conditions. Wigger diagram: left ventricle (black), aortic (red) and atrial pressure (green), and LV volume (blue). Strain curves: 16 LV segments strain signals for the three views (2CH, 4CH, and APLAX).
3.2. Simulations of desynchronization strain patterns by parameter variations
Figure 5 illustrates the simulated strain traces obtained in the septal and lateral walls for a healthy subject, LBBB with only electrical modification, LBBB with electrical modification and septal contractility reduction, LBBB with electrical modification and lateral contractility reduction. First, to induce an electrical modification, the electrical delay of all the LV segment were increased as well as the electrical delay of the LBBB. Then, the septal and lateral hypocontractility was respectively induce by a reduction of the active components of the LV septal and lateral segments: Kact.
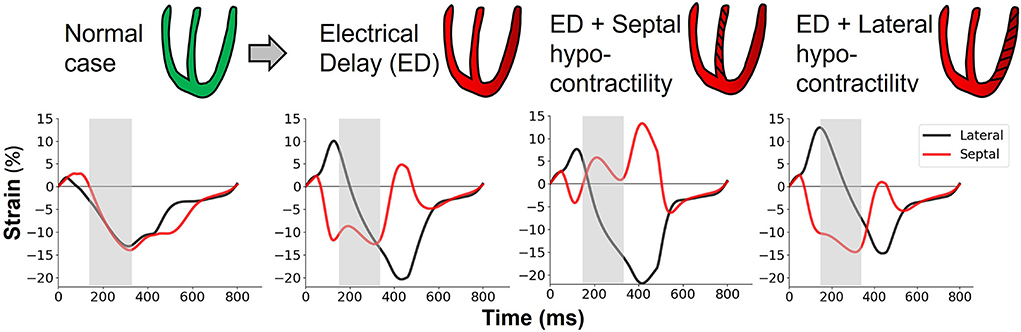
Figure 5. Simulated septal and lateral strain curves of a: healthy case, LBBB with only electrical modification, LBBB with electrical modification and septal contractility reduction, and LBBB with electrical modification and global contractility reduction. Gray background indicates the aortic valve opening to the closure period.
In the case of LBBB with only electrical modification, simulations present a typical septo-to-lateral activation pattern. In this case, the pre-ejection contraction of the septal wall is followed by an immediate re-lengthening of the wall, which induces a septal rebound stretch. In the septal hypocontractility case, the rebound stretch effect increases. The lateral hypocontractility case is characterized by a modification of the LV activation pattern and is associated with a significant reduction in lateral wall strain and a diminution of the septal rebound stretch. The simulations could be related to Aalen et al. [6] experimental results, where LBBB was induced in dogs with or without LV scar and previous results of our team [35].
3.3. Sensitivity analysis
Figure 6 shows a Morris scheme where 100 of the most influential parameters are plotted in the μ* − σ plane based on the Dj index (full lists of the sensitivity analysis results with all the parameters are included in Supplementary material). This representation highlights the parameters with negligible (in the lower left-hand corner), the linear without interaction (at the bottom right), and non-linear or interaction (on the top right) impact on Y. Parameters α2 and n1 present a great recurrence impact on the sensitivity of the evaluated outputs Y. In fact, as α1 and n2, they are involved in the electro-mechanical coupling at the tissue level (Equation 4) which cause modifications in mechanical contraction and, consequently, in the deformation of the LV segments. These parameters appear especially important for lateral and septal segments.
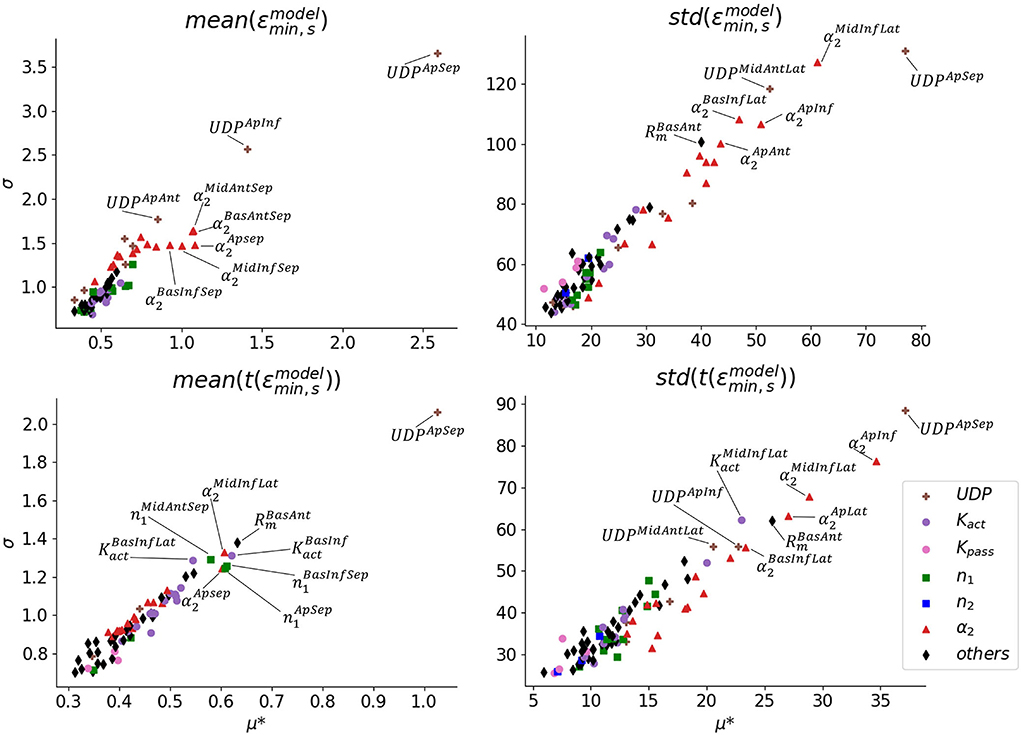
Figure 6. Most influential parameters on (i) the average of the minimum peaks over all segments (top, left), (ii) the standard deviation of the minimum peaks over all segments (top, right), (iii) the average time associated with each minimum peak over all segments (bottom, left), and (iv) the standard deviation of the time associated with each minimum peak over all segments (bottom, right); according to Morris sensitivity results. Only the first 100 parameters according to their distance Dj are plotted in the μ* − σ plane.
UDP, related to the electrical depolarization time, is also one of the most influential parameters. UDP is the time of the upstroke depolarization, it drives the activation of the neighbor's automata and affects the ta and ts value of the Equations (3, 4). ta is the time elapsed since the beginning of the activation and ts is initialized by the activation of the neighbors so directly impact by the UDP times of the previous automata. If we look closer at the sensitivity analysis, we can notice that the UDP related to the apical segments has the highest influence on the mean and standard deviation of the minimum strain value as well as the corresponding time. This could be explained by the electrical path. Indeed, the electrical and mechanical activities are closely related therefore, the deformation of a segment is highly dependent on the occurrence of electrical depolarization. Kact and Kpass, respectively related to the active and passive components of the cardiac muscle, show also high sensitivity.
Results from the sensitivity analysis were used to select the seven most significant model parameters to be identified for each segment: parameters related to the EMDF (n1, n2, α1, α2), the active (Kact) and passive (Kpass) components of the cardiac muscle and the electrical depolarization time (UDP). The electrical depolarization time of the left bundle branch (UDPLBB) was also added to the parameter identification list.
3.4. Patient-specific simulations
3.4.1. Segmental strain curves
Myocardial strain curves of the 16 LV segments acquired by experimental measurements and patient-specific simulations are presented in one representative healthy subject (Figure 7), an anterior ischemic (Figure 8) and a non-ischemic (Figure 9) patient with LBBB (all results are included in Supplementary material). For both healthy and LBBB cases, a good agreement was observed between clinical and simulated strain signals. The RMSE errors are similar through the 16 strain curves for each patient in both patient with LBBB types. Concerning healthy cases, the strain curves present similar morphologies in all the segments due to the synchronization in all LV regions when the myocardium contracts, but we can notice some difficulties to well fit the basal anterior and lateral strain in some healthy patients. The Figure 10 presents this RMSE average by regions for the three groups (the same RMSE bull's-eye representation is included in Supplementary material for each patient). The mean RMSE between estimated and observed strain signals in the healthy adults was equal to 5.04 ± 1.02 (Table 2).
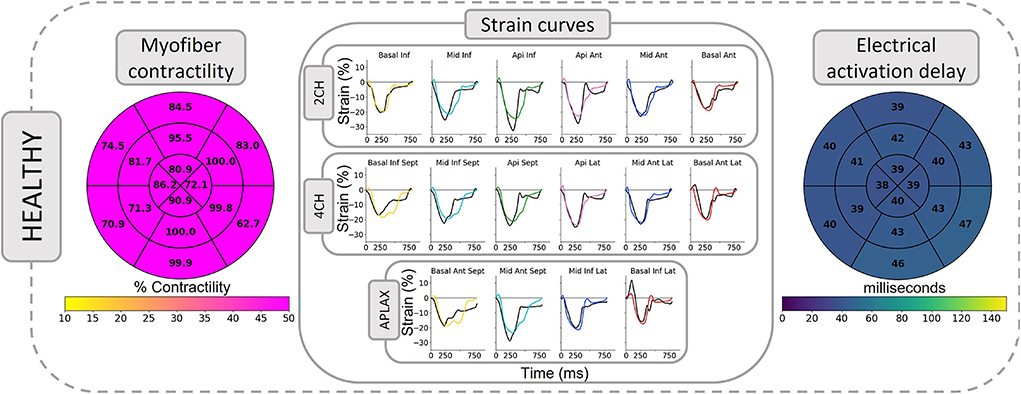
Figure 7. Patient-specific simulation results for a healthy subject. Experimental (black) and simulated (colored) strain curves corresponding to the 16 LV segments. Bull's-eye representations of segmental electrical activation delay and segmental myofiber contractility. Color scale at the contractility bull's-eye plot is set between 10 and 50% to highlight the segments with low contractility.
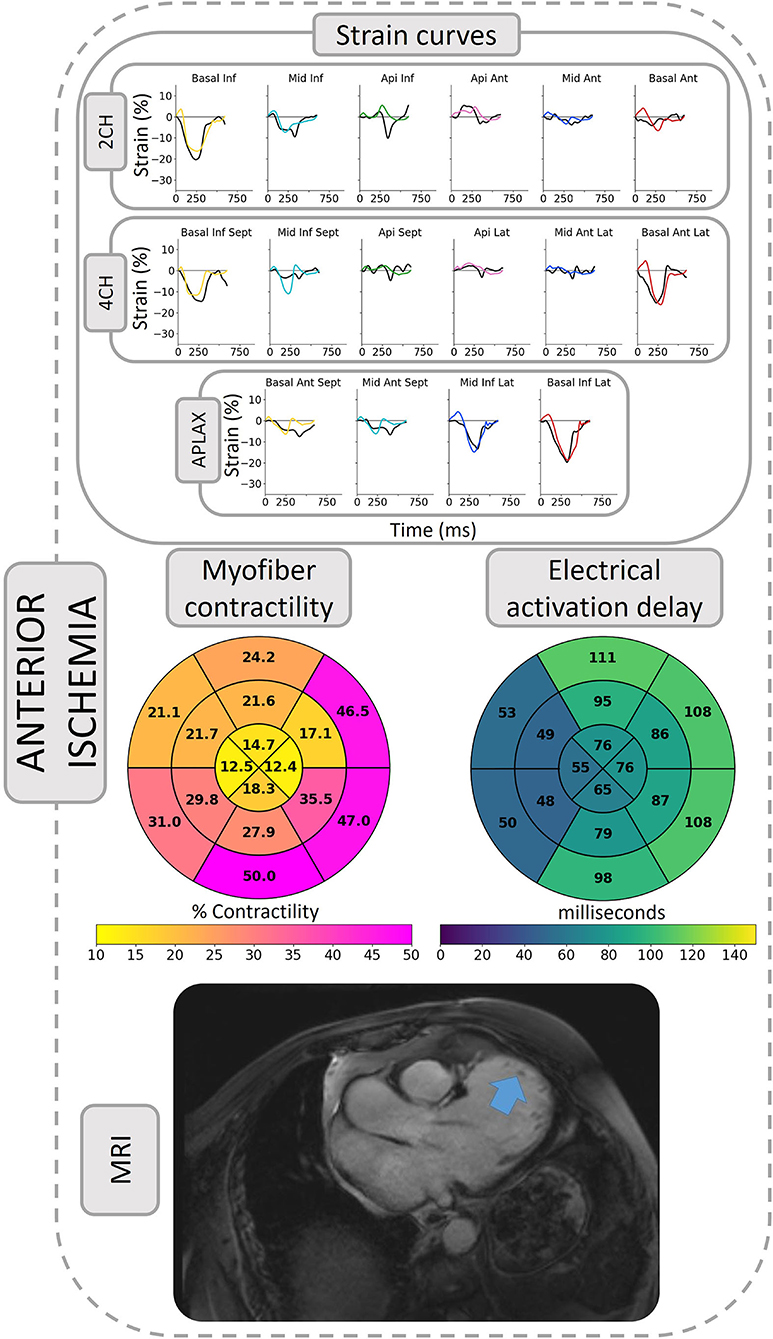
Figure 8. Patient-specific simulation results for an patient LBBB with an anterior ischemia. Experimental (black) and simulated (colored) strain curves corresponding to the 16 LV segments. Bull's-eye representations of segmental electrical activation delay and segmental myofiber contractility obtained by patient-specific simulations. The ischemia is localized on the cMRI (blue arrow).
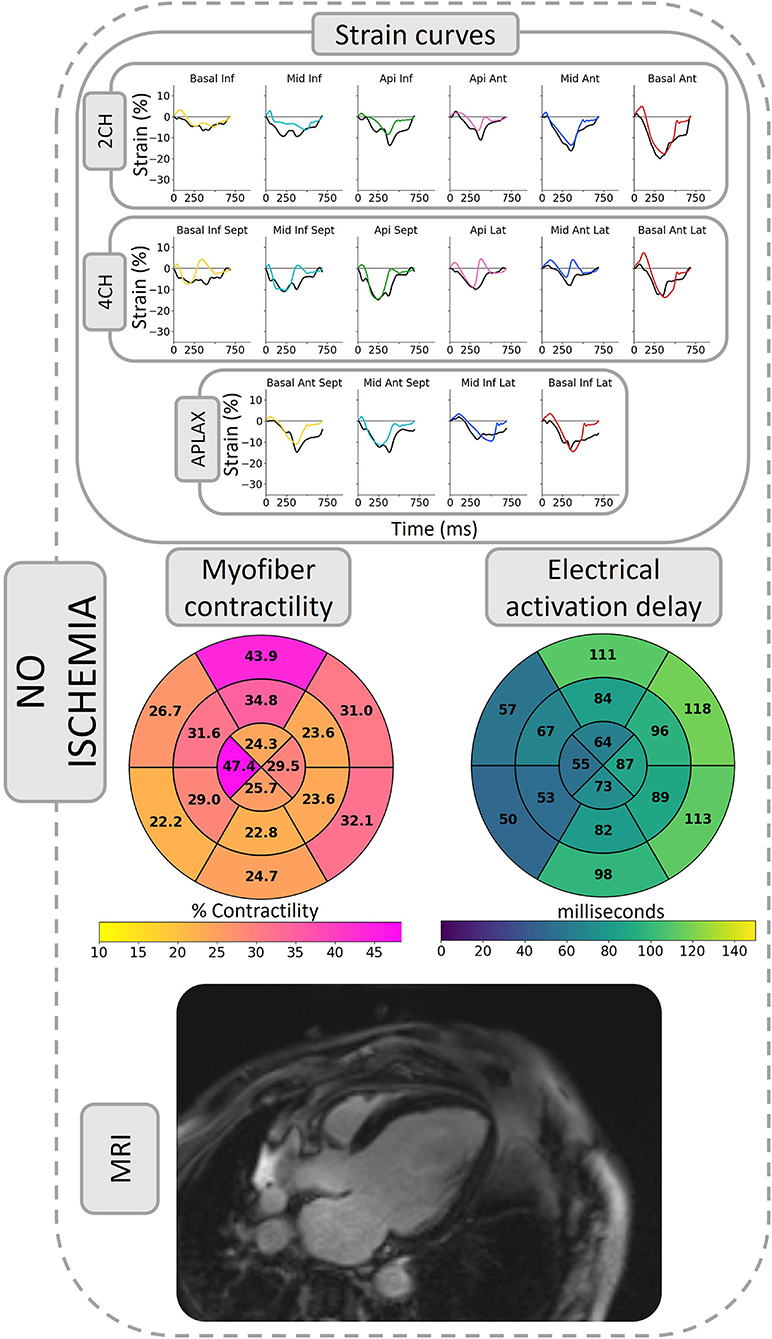
Figure 9. Patient-specific simulation results for a patient with non-ischemic LBBB. Experimental (black) and simulated (colored) strain curves corresponding to the 16 LV segments. Bull's-eye representations of segmental electrical activation delay and segmental myofiber contractility. No ischemia is localized on the cMRI.
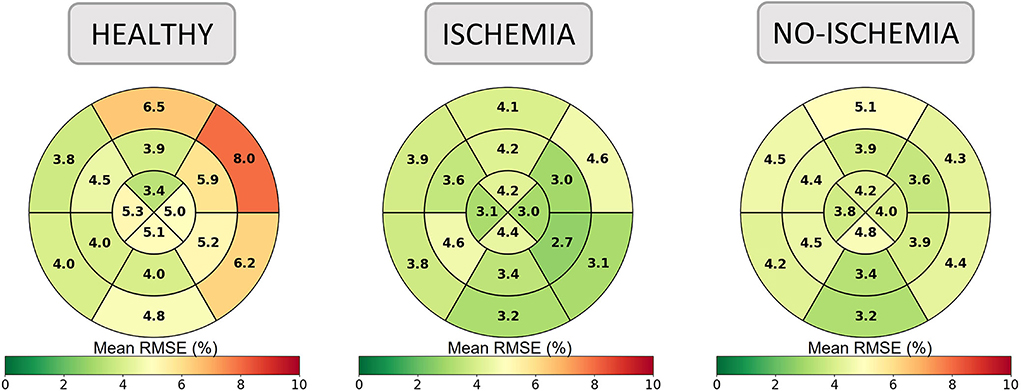
Figure 10. Mean RMSE by region for the three groups of patients: healthy and LBBB ischemia, LBBB no ischemia.
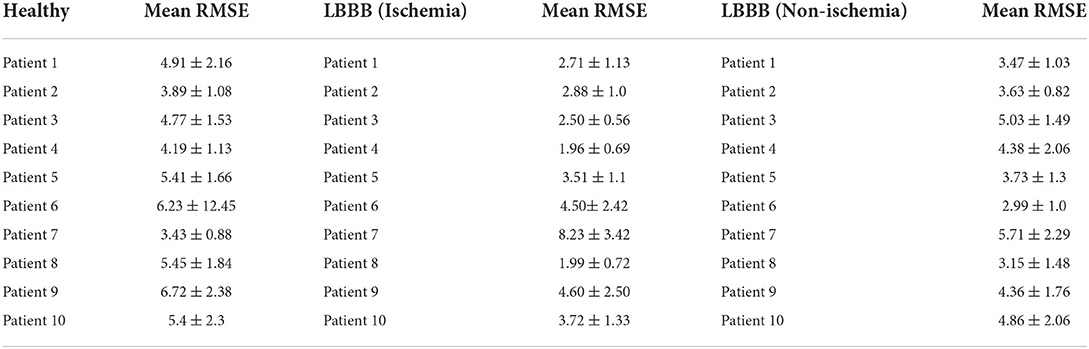
Table 2. Mean RMSE between the 16 experimental and simulated LV strain curves of the study population.
In LBBB cases, the mean RMSE was equal to 3.90 ± 1.40% (Table 2). In these cases, the strain curves obtained in patients with LBBB present dissimilar morphologies between the different segments. Particularly, the septum and the lateral wall segments of the ventricle present opposite curves, where the shortening of septal segments occurs at the same time as in the lengthening of lateral segments.
3.4.2. Bull's-eye representations
From patient-specific simulations, segmental electrical activation delay and the percentage of myofiber contractility (Kact) were represented on bull's-eye plots in Figures 7–9, for three representative cases : (1) Healthy adult, (2) patient with LBBB with LV anterior ischemia, and (3) patient with non-ischemic LBBB.
In LBBB cases, electrical activation bull's eye shows a significant electrical activation delay between the lateral and the septal wall of the LV; while in the healthy case, all LV segments are activated almost synchronously. Furthermore, the LBBB patient with LV anterior ischemia presented reduced contractility in anterior segments of the bull's-eye representation (Figure 8).
3.4.3. Unicity evaluation
The ratio of the mean standard deviation over the interval length of each type of parameter is presented in Table 3. The result of the repeated identification shows that the parameter values are gathered in the same part of the possible values of the interval. In fact, for all the parameters this means the standard deviation is between 0.34 and 16.32% of their respective interval. Especially for the electrical parameter UDPLBB, the ratio is less than 0.6%.

Table 3. The mean ratio of the standard deviation over interval length for 10 identification repetitions over five patients (two LBBB with ischemia, one LBBB without ischemia, and two healthy).
4. Discussion
This article presents a novel model-based approach that yields simulations of patient-specific strain curves in several LV regions for healthy adults and patients diagnosed with LBBB. The main contributions of this study are as follows: (i) the proposal of an integrated model of the CVS coupled to multi-segment representations of ventricles (ii) the sensitivity analysis of model parameters on myocardial strains, (iii) the identification of model parameters to reproduce myocardial strain curves specifically for each patient, and (iv) the analysis of patient-specific identified parameters.
The proposed CVS model is based on a functional integration of interacting physiological systems that takes into account the electro-mechanical coupling, the interventricular interaction, and a simplified representation of systemic and pulmonary circulations. The model includes the main cardiac properties required to tackle the problem under study, like the Frank–Starling law and the influence of preload and afterload. Results illustrate the model ability to simulate jointly the hemodynamic variables and myocardial deformations. Strain curves notably reflected typical characteristics associated with each phase of the cardiac cycle. In order to personalize models to patient-specific data, a large number of simulations should be performed. In opposition to FEM representations [17], the proposed model requires limited computational resources, as the simulation of one cardiac cycle (1,000 ms of simulation) takes approximately 0.5 s (Processor : 2,2 GHz Intel Core i7). The low computational cost is of primary importance to use cardiac modeling in clinical practice and to adapt models to each patient.
The first step of patient-specific adaptation is the sensitivity analysis of model parameters, which highlights (i) the close relationship between cardiac electrical and mechanical systems and (ii) the importance of active and passive properties of the myocardium during cardiac contraction. The analysis results show that the electric stimulation timing between the different segment has a great importance in the variability between the strain curves. The sensitivity analysis also highlights the importance of parameters related to myocardial mechanical properties. In fact, a close relationship exists between excitation and contraction since a synchronous ventricular activation is a prerequisite for an adequate LV function, whereas the electrical activation delay between opposite LV walls might lead to dyssynchronous ventricular contraction and LV failure [50]. Nevertheless, it has been shown that typical myocardial strain morphologies in LBBB could be modified by the presence of scar and low regional LV contractility (Figure 5 illustrates this point, by presenting different pattern though the independent modulation of parameters related to the electrical behaviors and to the tissues contractility quality). Moreover, the parameters of the septal and lateral part of the LV present the highest influence on the strain curves dyssynchrony. This is particularly interesting knowing the recent study on the importance of the septal variability in the contribution of the LV reverse modeling [6]. In this context, the ability of the model to disclose the relationship between electrical activation delay and LV contractility has pivotal importance because it might ease the identification of myocardial substrates that more prone to be associated with CRT response.
In the second step of patient-specific adaptation, EA were used to identify the most influential parameters in each patient. The error function was minimized based on experimental and simulated strain curves previously synchronized on the onset of QRS of synthesized and experimental ECG. Patient-specific simulations have shown satisfactory results since we observed a good agreement between simulated and experimental myocardial strain curves given the reproducibility of strain signals [51, 52]. For healthy cases, morphologies of the myocardial strain curves were similar in all segments due to the synchronous contraction of the entire LV [36]. Associated bull's eyes show normal electrical activation times and elevated contractile levels.
In most patients with non-ischemic LBBB, the early activation of the LV septum, followed by the delayed activations of the LV wall [53, 54], causes a typical myocardial strain pattern. This pattern is characterized by an early marked shortening of the septum in the pre-ejection phase, known as “septal flash” [55] followed by an immediate re-lengthening of the septum, the “septal rebound stretch.” Both the septal flash and septal rebound are known to be predictors of CRT response [4, 56].
In ischemic patients, the typical activation pattern induced by LBBB can be disrupted by the association of electrical delay and inhomogeneous LV contractility. In patients with LBBB and lateral scar, hypocontractile regions are localized in the lateral wall. In this case, deformation patterns are highly modified because the local impairment of contractility in the lateral wall caused the loss of the rebound stretch in the septum [6]. On the contrary, the presence of an anterior scar was associated with a reduced contractility of the corresponding myocardial segments and had less impact on septal deformation [6]. The strength of our model was, therefore, to reproduce the “atypical” strain patterns observed in patients with LBBB and ischemic cardiomyopathy thought the correct localization of the hypocontractile segments, which correspond to areas of myocardial scar identified by a clinician based on cMRI. Although the scare localizations, observed with cMRI, are not provided on a per-segment basis, it was possible to make a correspondence between low contractility segments and scars regions. Segments associated with low contractility corresponds to the areas of myocardial scar observed with cMRI.
There are several important consequences of our findings. First, the results of our model-based approach underscore that septal motion and global strain morphologies are not only explained by electrical conduction delay but also by the heterogeneity of contractile levels within the myocardium and suggest that the evaluation of LV dyssynchrony should take into account both electrical delay and regional mechanical function. Second, the application of a model-based approach could bring additional information on the regional electrical and mechanical function of the LV from the simple analysis of echocardiography data. This is particularly important because it can help to disclose the intrinsic complexity of LV mechanics in CRT candidates, and represents a step forward in the development of personalized LV modeling in the field of CRT. Third, one of the main strengths of the approach was to perform a parameter identification process for the patient-specific estimations of the segmental strain curves. In order to build the cost function, experimental and simulated strain curves were synchronized on QRS peaks of synthesized and experimental ECG. Model parameters were identified from the myocardial strain curves of the 16 LV segments acquired by STE. For both healthy and LBBB cases, a good agreement was observed between measured and estimated strain signals.
These results bode well in our model capacity to reproduce clinical measurements and could be promising in the LV function analysis for an individual patient and eventually in the prediction of optimal treatments.
Although several studies have successfully used computational models of the CVS to understand myocardial deformation patterns [20, 57, 58], or investigate the best CRT pacing location [20, 21, 59] our approach provides interesting advantages and originalities. The multi-segment model of the LV allows not only the analysis of the deformation curves of the septal and lateral walls but also the strain signals of all the ventricular regions. Therefore, the proposed model resolution was adapted to the standardized segmentation of the AHA, keeping a similar abstraction level as clinicians for the analysis of strain signals. It also uses data from 2D STE, highly accessible in clinical routine with well-known strengths and limitations. Moreover, our approach applies a parameter identification process, providing customized models specifically for each patient and allowing the recognition of hypocontractile areas that could be associated with the presence of fibrosis.
5. Limitations
The proposed model-based approach presents some limitations that should be mentioned. Several hypotheses were made in order to propose tissue-level representations of ventricles: (i) the ventricular torsion was neglected, (ii) the mechanical continuity between myocardial segments was not always assured because ventricles are represented by a set of sub-pumps controlled by a coordinated electrical activity and coupled in the hydraulic domain, (iii) only mean myocardial fiber orientation was considered, and (iv) electro-mechanical coupling was approximated by an analytic expression.
Despite these hypotheses, the model definition is in accordance with the problem under study and appears to be a useful tool to assist the in interpretation of strain data. Moreover, in order to reduce computational costs, only a small sample of variables was selected for parameter identification. The heterogeneity in parameters is mainly explained by pathological characteristics of LBBB patients (ischemic tissues, alterations of cardiac electrical pathways,…). Parameter heterogeneities are also reflected in strain values and morphologies that could be observed for myocardial segments of the same patient. These parameters may also have absorbed changes in other fixed parameters. For instance, septal segment parameters may have been affected by RV variations. Thus, a wider range of parameters could be included in the future. Finally, this study is based on a small population of patients with LBBB, an extension of our simulation on a larger clinical database and simulation repetitions should give us a better estimation of the reproducibility and the robustness of the method.
Moreover, as the results show, the surface of the hypocontractile regions seems overestimated. That suggests a diffusion of the tissue quality in the parameters identification process. In the same way, a mismatch still exists between the experimental and simulated curves. Some efforts must still be made to reduce it, but the simplifications chosen in the model definition, as well as the reduce number of parameters used in the patient-specific identification, explain it. Future work must be dedicated on a precise validation of identified parameters that are not validated yet, due to clinical difficulties to measure it on such a population.
Nevertheless, this work proposes patient-specific simulations of strain curves in the case of LBBB in association with ischemia and the proposed approach is a step forward toward the integration of computational models in the patient selection process before CRT procedures. Future work will be dedicated to evaluate the proposed model-based indices, in wider multi-parametric approach [60], for the prediction of CRT response.
6. Conclusion
In this work, we propose a novel model-based approach for the analysis of myocardial strains in patients with LBBB. The global method is based on (i) a physiological model of the CVS that integrates the electrical, mechanical, and hydraulic processes leading to ventricular contraction and (ii) a parameter identification procedure for patient-specific simulations. The proposed model-based approach was evaluated with echocardiography data from 10 healthy individuals and 20 patients with LBBB. Results show a close match between experimental and simulated strain curves in all the cases. Furthermore, the approach is able to reproduce electrical activation delay and segmental myofiber contractility properly.
More extensive evaluations including a greater population of patients, as well as the analysis on a wider multi-parametric approach, should be performed in the future. Nevertheless, this article presents a first work toward the evaluation of myocardial strain signals and the assessment of certain echo-based parameters by patient-specific simulations based on computational models. The proposed personalized approach represents a promising tool for the LV mechanical dyssynchrony understanding and CRT responders identification.
Data availability statement
The original contributions presented in the study are included in the article/Supplementary material, further inquiries can be directed to the corresponding author.
Ethics statement
The studies involving human participants were reviewed and approved by ethical approval from of the Local Medical Ethics Committee (Person Protection Committee West VCPP Ouest V). The patients/participants provided their written informed consent to participate in this study.
Author contributions
MT, KO, AIH, and VL conceived and designed the study. EG, JD, AH, and ED performed the clinical measurements and analyzed the experimental data and study population. KO, VL, and AIH developed the model. MT and KO run the in silico experiments and analyze. KO, MT, EG, AIH, and VL interpreted the results. MT, KO, and VL prepared the figures and drafted the manuscript. All the authors revised, read, and approved the final version of the manuscript.
Funding
This work was supported by the French National Research Agency (ANR) (ANR-16-CE19- 0008-01) (project MAESTRo) and the French Brittany council (ADvICE project).
Conflict of interest
The authors declare that the research was conducted in the absence of any commercial or financial relationships that could be construed as a potential conflict of interest.
Publisher's note
All claims expressed in this article are solely those of the authors and do not necessarily represent those of their affiliated organizations, or those of the publisher, the editors and the reviewers. Any product that may be evaluated in this article, or claim that may be made by its manufacturer, is not guaranteed or endorsed by the publisher.
Supplementary material
The Supplementary Material for this article can be found online at: https://www.frontiersin.org/articles/10.3389/fams.2022.833003/full#supplementary-material
References
1. Grines CL, Bashore TM, Boudoulas H, Olson S, Shafer P, Wooley CF. Functional abnormalities in isolated left bundle branch block. The effect of interventricular asynchrony. Circulation. (1989) 79:845–53. doi: 10.1161/01.CIR.79.4.845
2. Risum N, Tayal B, Hansen TF, Bruun NE, Jensen MT, Lauridsen TK, et al. Identification of typical left bundle branch block contraction by strain echocardiography is additive to electrocardiography in prediction of long-term outcome after cardiac resynchronization therapy. J Am Coll Cardiol. (2015) 66:631–41. doi: 10.1016/j.jacc.2015.06.020
3. De Boeck BWL, Teske AJ, Meine M, Leenders GE, Cramer MJ, Prinzen FW, et al. Septal rebound stretch reflects the functional substrate to cardiac resynchronization therapy and predicts volumetric and neurohormonal response. Eur J Heart Fail. (2009) 11:863–71. doi: 10.1093/eurjhf/hfp107
4. Stankovic I, Prinz C, Ciarka A, Daraban AM, Kotrc M, Aarones M, et al. Relationship of visually assessed apical rocking and septal flash to response and long-term survival following cardiac resynchronization therapy (PREDICT-CRT). Eur Heart J Cardiovasc Imaging. (2016) 17:262–9. doi: 10.1093/ehjci/jev288
5. Rao HB, Krishnaswami R, Kalavakolanu S, Calambur N. Ventricular dyssynchrony patterns in left bundle branch block, with and without heart failure. Indian Pacing Electrophysiol J. (2010) 10:115–21.
6. Aalen JM, Remme EW, Larsen CK, Andersen OS, Krogh M, Duchenne J, et al. Mechanism of abnormal septal motion in left bundle branch block: role of left ventricular wall interactions and myocardial scar. JACC Cardiovasc Imaging. (2019) 12:2402–13. doi: 10.1016/j.jcmg.2018.11.030
7. Hoit Brian D. Strain and strain rate echocardiography and coronary artery disease. Circulation. (2011) 4:179–90. doi: 10.1161/CIRCIMAGING.110.959817
8. Lumens Joost, Tayal Bhupendar, Walmsley John, Delgado-Montero Antonia, Huntjens Peter R, Schwartzman David, et al. Differentiating electromechanical from non–electrical substrates of mechanical discoordination to identify responders to cardiac resynchronization therapy. Circulation. (2015) 8:e003744. doi: 10.1161/CIRCIMAGING.115.003744
9. Trayanova NA, Rice JJ. Cardiac electromechanical models: from cell to organ. Front Physiol. (2011) 2:43. doi: 10.3389/fphys.2011.00043
10. Tusscher KHWJ, Noble D, Noble PJ, Panfilov AV. A model for human ventricular tissue. Am J Physiol Hear Circ Physiol. (2003) 286:1573–89. doi: 10.1152/ajpheart.00794.2003
11. Bartolucci C, Passini E, Hyttinen J, Paci M, Severi S. Simulation of the effects of extracellular calcium changes leads to a novel computational model of human ventricular action potential with a revised calcium handling. Front Physiol. (2020) 11:314. doi: 10.3389/fphys.2020.00314
12. O'Hara T, Virág L, Varró A, Rudy Y. Simulation of the undiseased human cardiac ventricular action potential: model formulation and experimental validation. PLoS Comput Biol. (2011) 7:e1002061. doi: 10.1371/journal.pcbi.1002061
13. Cortassa S, Aon MA, O'Rourke B, Jacques R, Tseng HJ, Marbán E, et al. A computational model integrating electrophysiology, contraction, and mitochondrial bioenergetics in the ventricular myocyte. Biophys J. (2006) 91:1564–89. doi: 10.1529/biophysj.105.076174
14. Dupuis LJ, Lumens J, Arts T, Delhaas T. Mechano-chemical interactions in cardiac sarcomere contraction: a computational modeling study. PLoS Comput Biol. (2016) 12:e1005126. doi: 10.1371/journal.pcbi.1005126
15. Regazzoni F, Dedé L, Quarteroni A. Biophysically detailed mathematical models of multiscale cardiac active mechanics. PLoS Comput Biol. (2020) 16:e1008294. doi: 10.1371/journal.pcbi.1008294
16. Pironet A, Desaive T, Kosta S, Lucas A, Paeme S, Collet A, et al. A multi-scale cardiovascular system model can account for the load-dependence of the end-systolic pressure-volume relationship. Biomed Eng Online. (2013) 12:8. doi: 10.1186/1475-925X-12-8
17. Rolle VL, Galli E, Danan D, Houari KE, Hubert A, Donal E, et al. Sensitivity analysis of a left ventricle model in the context of intraventricular dyssynchrony. Acta Biotheor. (2019) 68:45–59. doi: 10.1007/s10441-019-09362-y
18. Sack KL, Dabiri Y, Franz T, Solomon SD, Burkhoff D, Guccione JM. Investigating the role of interventricular interdependence in development of right heart dysfunction during LVAD support: a patient-specific methods-based approach. Front Physiol. (2018) 9:520. doi: 10.3389/fphys.2018.00520
19. Park JIK, Heikhmakhtiar AK, Kim CH, Kim YS, Choi SW, Song KS, et al. The effect of heart failure and left ventricular assist device treatment on right ventricular mechanics: a computational study. Biomed Eng Online. (2018) 17:62. doi: 10.1186/s12938-018-0498-0
20. Okada Ji, Washio T, Nakagawa M, Watanabe M, Kadooka Y, Kariya T, et al. Multi-scale, tailor-made heart simulation can predict the effect of cardiac resynchronization therapy. J Mol Cell Cardiol. (2017) 108:17–23. doi: 10.1016/j.yjmcc.2017.05.006
21. Crozier A, Blazevic B, Lamata P, Plank G, Ginks M, Duckett S, et al. The relative role of patient physiology and device optimisation in cardiac resynchronisation therapy: a computational modelling study. J Mol Cell Cardiol. (2016) 96:93–100. doi: 10.1016/j.yjmcc.2015.10.026
22. Niederer SA, Lamata P, Plank G, Chinchapatnam P, Ginks M, Rhode K, et al. Analyses of the redistribution of work following cardiac resynchronisation therapy in a patient specific model. PLoS ONE. (2012) 7:e43504. doi: 10.1371/journal.pone.0043504
23. Aguado-sierra J, Krishnamurthy A, Villongco C, Chuang J, Howard E, Gonzales MJ, et al. Patient-specific modeling of dyssynchronous heart failure : a case study. Prog Biophys Mol Biol. (2011) 107:147–55. doi: 10.1016/j.pbiomolbio.2011.06.014
24. Constantino J, Hu Y, Trayanova NA. A computational approach to understanding the cardiac electromechanical activation sequence in the normal and failing heart, with translation to the clinical practice of CRT. Prog Biophys Mol Biol. (2012) 110:372–9. doi: 10.1016/j.pbiomolbio.2012.07.009
25. Pezzuto S, Prinzen FW, Potse M, Maffessanti F, Regoli F, Caputo ML, et al. Reconstruction of three-dimensional biventricular activation based on the 12-lead electrocardiogram via patient-specific modelling. Europace. (2021) 23:640–7. doi: 10.1093/europace/euaa330
26. Vincent KP, Forsch N, Govil S, Joblon JM, Omens JH, Perry JC, et al. Atlas-based methods for efficient characterization of patient-specific ventricular activation patterns. Europace. (2021) 23:I88–95. doi: 10.1093/europace/euaa397
27. Lumens J, Leenders GE, Cramer MJ, De Boeck BWL, Doevendans PA, Prinzen FW, et al. Mechanistic evaluation of echocardiographic dyssynchrony indices: patient data combined with multiscale computer simulations. Circulat Cardiovasc Imaging. (2012) 5:491–9. doi: 10.1161/CIRCIMAGING.112.973446
28. Willemen E, Schreurs R, Huntjens PR, Strik M, Plank G, Vigmond E, et al. The left and right ventricles respond differently to variation of pacing delays in cardiac resynchronization therapy: a combined experimental- computational approach. Front Physiol. (2019) 10:17. doi: 10.3389/fphys.2019.00017
29. van Everdingen WM, Walmsley J, Cramer MJ, van Hagen I, De Boeck BWL, Meine M, et al. Echocardiographic prediction of cardiac resynchronization therapy response requires analysis of both mechanical dyssynchrony and right ventricular function: a combined analysis of patient data and computer simulations. J Am Soc Echocardiogr. (2017) 30:1012–20.e2. doi: 10.1016/j.echo.2017.06.004
30. Leenders GE, Lumens J, Cramer MJ, De Boeck BWL, Doevendans PA, Delhaas T, et al. Septal deformation patterns delineate mechanical dyssynchrony and regional differences in contractility: analysis of patient data using a computer model. Circulat Heart Failure. (2012) 5:87–96. doi: 10.1161/CIRCHEARTFAILURE.111.962704
31. Niederer SA, Lumens J, Trayanova NA. Computational models in cardiology. Nat Rev Cardiol. (2019) 16:100–11. doi: 10.1038/s41569-018-0104-y
32. Le Rolle V, Hernández AI, Richard PY, Donal E, Carrault G. Model-based analysis of myocardial strain data acquired by tissue Doppler imaging. Artif Intell Med. (2008) 44:201–19. doi: 10.1016/j.artmed.2008.06.001
33. Calvo M, Le Rolle V, Romero D, Béhar N, Gomis P, Mabo P, et al. Model-based analysis of the autonomic response to head-up tilt testing in Brugada syndrome. Comput Biol Med. (2018) 103:82–92. doi: 10.1016/j.compbiomed.2018.10.007
34. Owashi KP, Hubert A, Galli E, Donal E, Hernández AI, Le Rolle V. Model-based estimation of left ventricular pressure and myocardial work in aortic stenosis. PLoS ONE. (2020) 15:e0229609. doi: 10.1371/journal.pone.0229609
35. Owashi K, Taconné M, Courtial N, Simon A, Garreau M, Hernandez A, et al. Desynchronization strain patterns and contractility in left bundle branch block through computer model simulation. J Cardiovasc Dev Dis. (2022) 9:53. doi: 10.3390/jcdd9020053
36. Lang RM, Badano LP, Mor-Avi V, Afilalo J, Armstrong A, Ernande L, et al. Recommendations for cardiac chamber quantification by echocardiography in adults: an update from the american society of echocardiography and the european association of cardiovascular imaging. J Am Soc Echocardiogr. (2015) 28:1–39.e14. doi: 10.1016/j.echo.2014.10.003
37. Hernández AI, Le Rolle V, Defontaine A, Carrault G. A multiformalism and multiresolution modelling environment: application to the cardiovascular system and its regulation. Philos Trans R Soc A Math Phys Eng Sci. (2009) 367:4923–40. doi: 10.1098/rsta.2009.0163
38. Hernández AI, Le Rolle V, Ojeda D, Baconnier P, Fontecave-Jallon J, Guillaud F, et al. Integration of detailed modules in a core model of body fluid homeostasis and blood pressure regulation. Prog Biophys Mol Biol. (2011) 107:169–82. doi: 10.1016/j.pbiomolbio.2011.06.008
39. Cerqueira MD, Weissman NJ, Dilsizian V, Jacobs AK, Kaul S, Laskey WK, et al. Standardized myocardial segmentation and nomenclature for tomographic imaging of the heart. Circulation. (2002) 105:539–42. doi: 10.1161/hc0402.102975
40. Stergiopulos N, Meister JJ, Westerhof N. Determinants of stroke volume and systolic and diastolic aortic pressure. Am J Physiol. (1996) 270:H2050–9. doi: 10.1152/ajpheart.1996.270.6.H2050
41. Le Rolle V, Carrault G, Richard PY, Pibarot P, Durand LG, Hernández AI. A tissue-level electromechanical model of the left ventricle: application to the analysis of intraventricular pressure. Acta Biotheor. (2009) 57:457. doi: 10.1007/s10441-009-9092-y
42. Lumens J, Delhaas T, Kirn B, Arts T. Three-wall segment (TriSeg) model describing mechanics and hemodynamics of ventricular interaction. Ann Biomed Eng. (2009) 37:2234–55. doi: 10.1007/s10439-009-9774-2
43. Hunter PJ, Mcculloch AD, Keurs HEDJ. Modelling the mechanical properties of cardiac muscle. Model Mech Prop Card muscle. (1998) 21:15.
44. Morris MD. Factorial sampling plans for preliminary computational experiments. Technometrics. (1991) 33:161–74. doi: 10.1080/00401706.1991.10484804
45. Guerrero G, Rolle VL, Hernandez A. Sensitivity analysis of a cardiorespiratory model for the study of sleep apnea. Comput Cardiol. (2010). 2018-Septe:18–21. doi: 10.22489/CinC.2018.357
46. Ojeda D, Le Rolle V, Harmouche M, Drochon A, Corbineau H, Verhoye JP, et al. Sensitivity analysis and parameter estimation of a coronary circulation model for triple-vessel disease. IEEE Trans Biomed Eng. (2014) 61:1208–19. doi: 10.1109/TBME.2013.2296971
47. Goldberg DE, Holland JH. Genetic algorithms and machine learning. Mach Learn. (1988) 3:95–9. doi: 10.1023/A:1022602019183
48. Storn R, Price K. Differential evolution — a simple and efficient heuristic for global optimization over continuous spaces. J Glob Optim. (1997) 11:341–59. doi: 10.1023/A:1008202821328
49. Biscani F, Izzo D. A parallel global multiobjective framework for optimization: pagmo. J Open Source Softw. (2020) 5:2338. doi: 10.21105/joss.02338
50. Nguyên UC, Verzaal NJ, van Nieuwenhoven FA, Vernooy K, Prinzen FW. Pathobiology of cardiac dyssynchrony and resynchronization therapy. Europace. (2018) 20:1898–909. doi: 10.1093/europace/euy035
51. Cheng S, Larson MG, McCabe EL, Osypiuk E, Lehman BT, Stanchev P, et al. Reproducibility of speckle-tracking-based strain measures of left ventricular function in a community-based study. J Am Soc Echocardiogr. (2013) 26:1258–66.e2. doi: 10.1016/j.echo.2013.07.002
52. Armstrong AC, Ricketts EP, Cox C, Adler P, Arynchyn A, Liu K, et al. Quality control and reproducibility in M-mode, two-dimensional, and speckle tracking echocardiography acquisition and analysis: the CARDIA study, year 25 examination experience. Echocardiography. (2015) 32:1233–40. doi: 10.1111/echo.12832
53. De Boeck BW, Kirn B, Teske AJ, Hummeling RW, Doevendans PA, Cramer MJ, et al. Three-dimensional mapping of mechanical activation patterns, contractile dyssynchrony and dyscoordination by two-dimensional strain echocardiography: rationale and design of a novel software toolbox. Cardiovasc Ultrasound. (2008) 6:22. doi: 10.1186/1476-7120-6-22
54. Gjesdal O, Remme EW, Opdahl A, Skulstad H, Russell K, Kongsgaard E, et al. Mechanisms of abnormal systolic motion of the interventricular septum during left bundle-branch block. Circulat Cardiovasc Imaging. (2011) 4:264–73. doi: 10.1161/CIRCIMAGING.110.961417
55. Parsai C, Bijnens B, Sutherland GR, Baltabaeva A, Claus P, Marciniak M, et al. Toward understanding response to cardiac resynchronization therapy: left ventricular dyssynchrony is only one of multiple mechanisms. Eur Heart J. (2009) 30:940–9. doi: 10.1093/eurheartj/ehn481
56. Doltra A, Bijnens B, Tolosana JM, Borrás R, Khatib M, Penela D, et al. Mechanical abnormalities detected with conventional echocardiography are associated with response and midterm survival in CRT. JACC Cardiovasc Imaging. (2014) 7:969–79. doi: 10.1016/j.jcmg.2014.03.022
57. Walmsley J, Arts T, Derval N, Bordachar P, Cochet H, Ploux S, et al. Fast Simulation of Mechanical Heterogeneity in the Electrically Asynchronous Heart Using the MultiPatch Module. PLoS Comput Biol. (2015) 11:e1004284. doi: 10.1371/journal.pcbi.1004284
58. Albatat M, Arevalo H, Bergsland J, Strøm V, Balasingham I, Odland HH. Optimal pacing sites in cardiac resynchronization by left ventricular activation front analysis. Comput Biol Med. (2021) 128:104159. doi: 10.1016/j.compbiomed.2020.104159
59. Pluijmert M, Bovendeerd PHM, Lumens J, Vernooy K, Prinzen FW, Delhaas T. New insights from a computational model on the relation between pacing site and CRT response. Europace. (2016) 18:iv94–iv103. doi: 10.1093/europace/euw355
Keywords: computational model, echocardiography, parameter identification, sensitivity analysis, left bundle branch block (LBBB)
Citation: Taconné M, Owashi KP, Galli E, Duchenne J, Hubert A, Donal E, Hernàndez AI and Le Rolle V (2022) Model-based analysis of myocardial strains in left bundle branch block. Front. Appl. Math. Stat. 8:833003. doi: 10.3389/fams.2022.833003
Received: 10 December 2021; Accepted: 25 October 2022;
Published: 17 November 2022.
Edited by:
Jacques Demongeot, Universitá Grenoble Alpes, FranceReviewed by:
Kevin P. Vincent, Alphatec Spine, Inc, United StatesAnnamaria Porreca, University of Studies G. d'Annunzio Chieti and Pescara, Italy
Copyright © 2022 Taconné, Owashi, Galli, Duchenne, Hubert, Donal, Hernàndez and Le Rolle. This is an open-access article distributed under the terms of the Creative Commons Attribution License (CC BY). The use, distribution or reproduction in other forums is permitted, provided the original author(s) and the copyright owner(s) are credited and that the original publication in this journal is cited, in accordance with accepted academic practice. No use, distribution or reproduction is permitted which does not comply with these terms.
*Correspondence: Alfredo I. Hernàndez, alfredo.hernandez@univ-rennes1.fr
†These authors have contributed equally to this work and share first authorship
‡These authors have contributed equally to this work and share last authorship