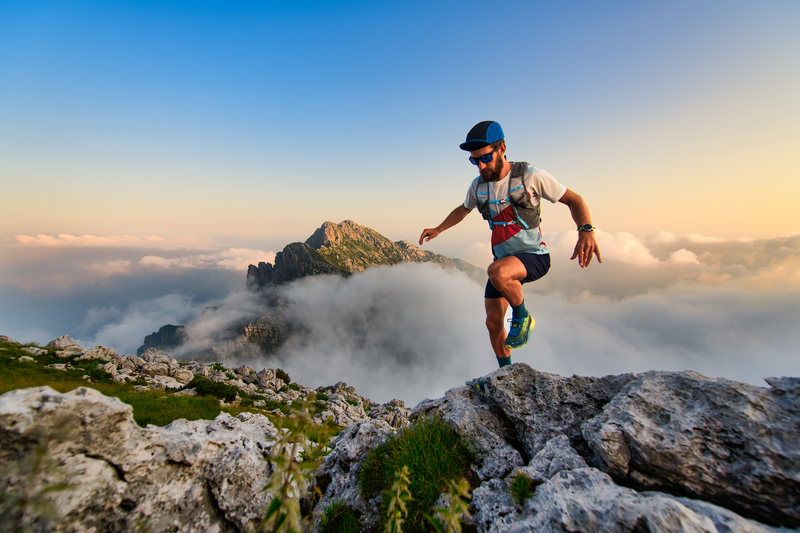
94% of researchers rate our articles as excellent or good
Learn more about the work of our research integrity team to safeguard the quality of each article we publish.
Find out more
ORIGINAL RESEARCH article
Front. Appl. Math. Stat. , 31 January 2022
Sec. Dynamical Systems
Volume 7 - 2021 | https://doi.org/10.3389/fams.2021.717523
This article is part of the Research Topic Modelling collective motion across scales View all 9 articles
Moving animal groups exhibit a range of fascinating behaviors. The standard explanation for how these groups form and function is that the individual animals interact via attraction, repulsion, and alignment, where alignment is proposed to drive the collective motion. However, it has been shown both experimentally and theoretically that alignment interactions are not required to induce group level alignment. In particular, via the use of self-propelled particle models it has been established that several other mechanisms induce group level alignment (aka polarization) in combination with attraction alone. However, no systematic comparison of these mechanisms among themselves, or with explicit alignment, has been presented and it remains unclear how, or even if, they can be distinguished at the collective level. Here, we introduce two previously unreported mechanisms, burst-and-glide and burst-and-stop, and show via simulation that they also induce polarization in combination with attraction alone. Then, we compare the polarization inducing characteristics of six mechanisms; asymmetric interactions, asynchrony, anticipation, burst-and-glide, burst-and stop, and explicit alignment. We show that the mechanisms induce polarization in different parts of the attraction parameter space, that the route to polarization from uniformly random initial conditions, as well as repolarization following strong perturbations, is markedly different among the mechanisms. In particular, we find that alignment based and non-alignment based mechanisms can be distinguished via their polarization and repolarization processes. These findings further challenge the current alignment based theory of collective motion and may contribute to a more versatile theory of collective motion across scales.
Animals moving together in flocks, schools, and herds are ubiquitous in nature. The standard explanation for how individuals in these groups coordinate to generate the group level behavior we observe is that they interact locally with nearby individuals via some combination of attraction, repulsion, and alignment interactions [1, 2]. More specifically, attraction allows individuals to aggregate, repulsion prevents collisions, and the alignment interaction where individuals align with the average heading of their neighbors is proposed to drive the collective motion [3].
Much of our understanding of how animals in moving groups coordinate their motion has come out of the study of self-propelled particle (spp) models [3]. In spp models a number of particles move and interact with nearby particles via a set of local interaction rules, for example, attraction, repulsion, and alignment [2, 4]. Models including these three interactions have been shown to generate the standard groups: mills, swarms, and aligned (or polarized or dynamic) groups [4, 5], and have been widely adopted in modeling collective motion in specific real animal groups (e.g., [6, 7]) and as base models for general theoretical investigations (e.g., [8, 9]). The capacity of these models to produce group level alignment via the explicit alignment interaction has been critical in modeling groups of animals that move collectively through the environment. From now on we refer to group level alignment as “polarization,” and aligned groups as “polarized groups,” to clearly distinguish these from the explicit alignment interaction.
Over the past decade experimental studies has failed to detect explicit alignment interactions between individuals despite observing polarized schooling [10, 11]. It has also been established that explicit alignment interactions between particles are not required to produce polarized groups in spp models [12–17]. In addition, calculating explicit alignment has been described as a computationally intensive process [15], and [11] argue that it is unlikely that real animals will measure and store the speed and heading of neighbors that are required to compute the explicit alignment. Furthermore, [17, 18] speculate that the inclusion of explicit alignment interactions in spp models might explain why these models tend to fail to produce disruptive phenomena that are ubiquitous in nature, for example, bistability and switching between group types in fish [19]. Combined this suggests that alternatives to explicit alignment for generating polarized groups is required to explain collective motion in some animal groups and to address a number of issues related to a theory of collective motion based on explicit alignment interactions.
A number of specific mechanisms that can induce polarized collective motion from non-alignment interactions are known [12–17]. In particular, it is known that asymmetric interactions [13], anticipation [17], and asynchrony [16] induce polarization in combination with attraction. Asymmetric interactions, via blind zones, have been extensively studied in spp models [5, 15, 20–22]. In these models particles that are in a blind zone behind a particle relative to its direction of travel do not contribute to some, or all, of the interactions between the particles, resulting in asymmetric interactions. Given that many animals have restricted fields of vision [23, 24] and/or interact more strongly with nearby individuals in certain directions than others [25] asymmetric interactions represent a biologically plausible alternative to explicit alignment for explaining collective motion in some groups. Anticipation, where individuals use the future anticipated positions and headings of other individuals, rather than their current positions and headings to update their own headings is used by a number of animals [26–28], including humans moving in crowds [29–31]. This type of anticipation has been included in spp models that contain explicit alignment interactions [32, 33] and models that do not include them [17, 34]. In alignment based models anticipation has been reported to inhibit polarization and promote milling and swarming [32, 33], whereas in attraction based models anticipation has been shown to induce polarized collective motion [17]. In most spp models particles update their headings and positions synchronously, i.e., all particles update at exactly the same time, however, given stochasticity and other factors it is likely that individual animals in a moving group update at different times, in an asynchronous fashion [16]. A number of studies have investigated asynchronous updating in spp models [16, 35–37], in particular, [16] has established that sequential random asynchrony in update in combination with attraction induces polarization. However, sequential random asynchrony, where individuals update in a random sequential order on each time step, is unlikely to occur in real animal groups, but other types of asynchronous intermittent locomotion has been observed in animals across taxa [38]. In particular, the burst-and-glide and burst-and-stop type dynamics observed in fish [39–41], mammals [39, 42], birds [43], and insects [44] would be a more biologically plausible type of asynchrony. However, at present no study is available that has established that this type of asynchrony has similar polarization inducing capabilities, in combination with attraction, or other interactions.
Attraction is a fundamental biologically plausible interaction operating in animal groups [1, 3], and a component of many spp models [2, 13], but at present its perceived role is often limited to explain aggregation [3]. However, given that alternatives to explicit alignment are needed to explain collective motion in some animal groups, and to address issues related to the current alignment based theory of collective motion, and the recent discoveries of several mechanisms that induce polarization in combination with attraction, its role in the context of collective motion might require revision. Unfortunately, the information relating to the polarization inducing capacity of attraction is scattered throughout the literature and no direct comparison of the discovered polarization inducing mechanisms among themselves, or with explicit alignment is available, so how they differ with respect to the dynamics induced is largely unknown. Therefore, beyond biological plausibility arguments it remains unclear how the available polarization inducing mechanisms can help advance our understanding of collective motion in moving animal groups.
Here, we present a comparison of a number of known polarization inducing mechanisms in combination with attraction: explicit alignment [45, 46], asynchrony [16], anticipation [17], and asymmetric interactions (via a blind zone) [13]. We also introduce two previously unreported polarization inducing mechanisms in combination with attraction, burst-and-glide and burst-and-stop update, and add these, as well as explicit alignment alone, to our comparison.
We use the synchronous local attraction model (LAM) [16], known to not produce polarized groups, as our base model and add the polarization inducing mechanisms to it to allow for a direct comparison of their polarization inducing capabilities. We start by summarizing this model and then describe how it was adapted for each of the polarization inducing mechanisms. We consider eight models in total and label them (I)–(VIII). We note that all of these, except (V) and (VI), have been previously described in the literature and we provide the main references in the description of each. Models (I)–(IV) are identical to those presented in the references, but for (VII)–(VIII) we have only included the main interactions from the listed sources into the common framework of (I)–(IV) to facilitate focused comparison of the effects of the mechanisms themselves. Throughout this manuscript we use “hat” notation for normalized vectors (e.g., ), and “bar” notation for non-normalized vectors (e.g., ).
I. Synchronous LAM [16] This is a self-propelled particle model in which N particles move at constant speed δ in two dimensions and interact via local attraction only (see Figure 1A). On every time step, each particle calculates the position of the local center of mass (LCM) of all particles within a distance of R from it (its neighbors). The new heading of particle i () is a linear combination of the normalized direction toward the local center of mass () and its normalized current heading ()
The parameter c specifies the relative strength of attraction to the LCM when the relative tendency to proceed with the current heading is 1. Once all particles have calculated their new headings based on the current positions of their neighbors, all particles are simultaneously moved a distance of δ in the direction specified by .
Figure 1. The interactions in the models. In each model a focal particle is located at position at the center of the interaction zone with radius R and the black filled circles represents the (potential) neighbors. In the preceding timestep the focal particle moved a distance of δ from its previous position and its current heading is . (A) The local attraction model (LAM). The focal particle calculates the position of the local center of mass of its neighbors () and sets up the normalized vector pointing towards it. This describes the interactions in models (I,III,V,VI) and these differ only in how the update is executed. (B) LAM with blind zone (II). Same as in (A) except that any neighbors that are located in the blind zone specified by the angle β are not included in the and calculations. Here, there are five particles within the interaction range R in the figure, but one of them is in the blind zone and therefore excluded from the LCM calculation. (C) LAM with anticipation (IV). Here, the focal particle calculates the anticipated local center of mass () and the anticipated attraction vector using the anticipated future positions of its neighbors (open circles) rather than their current positions (black filled circles). The future anticipated position of neighbor j, currently at position , is , that is, the position that neighbor would be at if it continued with its current heading at its current speed δ for a time τ (the anticipation time). (D) LAM with explicit alignment (VII). Here, the focal particle calculates the local center of mass and set up the vector like in (A), but it also sets up a normalized vector which is the average heading of its neighbors. (E) Explicit alignment only (VIII). Here, the focal particle only sets up the vector, there is no attraction. Figure (A) is from [16] (CC-BY Strömbom), Figure (C) is from [17] (CC-BY Strömbom), and (B,D,E) have been adapted from these.
II. Synchronous LAM with a blind zone ([16] with blind zone from [13])
Identical to (I) except that a particle does not interact with other particles in a blind zone defined by the angle β behind it relative to its direction of travel (see Figure 1B).
III. Sequential random asynchronous LAM ([16])
Identical to (I) except that particles update their headings and move in random sequential order on every timestep.
IV. Positional anticipation in the synchronous LAM ([17])Identical to (I) except that instead of using the actual local center of mass of the neighbors for the heading update each particle uses the anticipated local center of mass of the neighbors instead (see Figure 1C).
V. Burst-and-glide asynchrony LAM
Identical to (I) except that instead of interacting on every timestep the particles only update their headings at random “burst” times T defined by Tj+1 = Tj+γ where γ is a glide time (time between bursts) drawn from a Weibull distribution Γ = Γ(κ, λ), where κ is shape parameter and λ is the scale parameter. The choice of the Weibull distribution is motivated by empiricial findings [41]. If a particle is scheduled to update its heading on timestep t it will update it according to Equation (1) and move a distance of δ in this direction (see Figure 1A), and for the subsequent timesteps until its next burst time Tj+1 the particle proceed with unchanged heading but exponentially decreasing speed (See Appendix A for more details).
VI. Burst-and-stop asynchrony LAM
Identical to (V) except that the particles only move when they are updating their headings, and on these timesteps they move a distance of δ. For timesteps between updates the particles remain stationary.
VII. LAM with explicit alignment (Interactions similar to [46, 47])
Identical to (I) except that an explicit alignment term has been added to Equation (1) resulting in
where
with Nn representing the number of neighbors of particle i and the normalized current heading of neighbor j (see Figure 1D).
VIII. Explicit alignment only. (Interaction from [45])
Identical to (VII) but without the attraction term cĈ. Here, particles only align with the average heading of their neighbors without any attraction (see Figure 1E).
Various aspects of the polarization behavior of (I)–(IV) and (VII)–(VIII) have already been described in the literature [13, 16, 17, 45, 46]. Here, we follow the simulation protocol employed to analyze (I,III,IV) in [16, 17] to compare and contrast the polarization inducing capacity of (II)–(VII). In particular, to illustrate over which attraction strengths c they induce polarization and where they do not. For the previously unpublished models (V) and (VI) we also collect particle trajectories and snapshots of the groups produced in simulations.
We use the standard polarization, or alignment, measure [45] to analyze all simulations. This measure is defined as follows. If N is the number of particles in the simulation and is the normalized current heading of particle i at time t then the polarization α is defined by
If all particles move in the same direction α = 1, and if all particle heading vectors cancel out α = 0. Polarized groups, by definition, have large α values and mills and swarms have low α values [16].
Following [16, 17] we ran 100 simulations for each value of c, from 0 to 2 in increments of 0.1, and measured the polarization over the last 50 timesteps of each simulation and the mean was returned. The total simulation time for each model was chosen so that full polarization would occur in each simulation, and the number of time steps used for each model was (I,II) 8,000, (III) 15,000, (IV) 5,000, (V) 40,000, (VI) 20,000, and (VII) 2,000. Plots showing the distribution of polarization values for each c in each model were then created to isolate regions of the c parameter space where polarized groups form for each model (I)–(VII). More specifically, we partitioned the range of possible polarization values, i.e., 0 to 1, into intervals of length 1/50, and then counted the number of polarization values that fell in each interval for a specific c. Then we divided each interval count for the specific c with the total number of simulations (100) to obtain the distribution (See [16] for more details on this type of plot). All models and all simulations shared the same basic parameters used in [13, 16, 17], namely, N = 100, R = 4, δ = 0.5, and for our comparison here we chose the auxiliary model parameters for the different models as follows (II) β = π, (IV) τ = 2, (V) κ = 4, λ = 3, k = 1, (VI) κ = 4, λ = 3, and (VII,VIII) a = 0.01.
We then compared the route to the polarized state from random initial conditions for each of the models (II)–(VIII). To do this we ran 5,000 simulations for each model and recorded the polarization of the group over time from the start until a polarized group had formed. Then, for each model, we calculated the median and the median absolute deviation (mad) of the polarization values at each time step (t) over the 5,000 simulations to obtain trajectories that illustrate how, on average, the group polarization process proceeds over time. We also collected the time to polarized group formation in each simulation and used this data to create “time to polarization” distributions and to calculate the median and mad “time to polarization” for each model. For these analyses, we used one c value from each region of the c-parameter space where a particular model is known to generate polarized groups. More specifically, we used c = 1 for (II), c = 0.2 for (III,V,VI,VII), c = 0.2 and c = 2 for (IV).
We also measured the polarization over time in simulations (with the same parameter values as above) where as soon as the group has polarized it is strongly perturbed (all particles are assigned random headings in [0, 2π]) and then allowed to repolarize again, and as soon as it has repolarized it is perturbed again. We used α = 0.99 as the threshold for deciding that a polarized group had formed in all models except (V) and (VI). In these two models, the stochastic burst-and-glide/stop motion prevents complete polarization of the group and therefore we used α = 0.85 for (V) and α = 0.9 for (VI). These values are close to the max polarization values groups generated by these models have in simulations. This process was repeated until we had at least 5,000 repolarization events for each model, and we also collected the time to repolarization following each perturbation and used this data to create “time to repolarization” distributions and to calculate the median and mad “time to repolarization” for each model. We then standardized all the repolarization trajectories to run from 0 to 1 (where 0 corresponds to the perturbation timestep and 1 the timestep when full polarization was recorded) and calculated the median and mad at each standardized time point over all the standardized repolarization curves to obtain repolarization curves for each model. Given that the repolarization typically takes a different number of timesteps every time we used the Matlab function “interp1” to interpolate each of the standardized curves over 0 to 1 over a 0.0001 grid so that a pointwise median and mad could be calculated.
Finally, we ran simulations following the simulation protocols above to generate route to polarization and repolarization curves for additional particle numbers N = 50, 75, 200, 1, 000.
All code necessary to verify the results presented in this manuscript is available (see the Data availability statement).
We establish that both burst-and-glide (V) and burst-and-stop (VI) update induces polarized group formation in combination with attraction, in addition to producing mills and swarms. Figure 2A shows the group types produced by burst-and-glide, and Figure 2B the groups produced by burst-and-stop. Figure 2C shows the common modified Weibull distribution Γ(4, 3) as measured in simulations, and Figures 2D,E show the speed over time for two particles in the simulations with asynchronous burst-and-glide update (D) and asynchronous burst-and-stop update (E). Finally, Figures 2F,G show examples of the polarization process through a simulation with burst-and-glide (F) and burst-and-stop (G). We note that in both models the polarization increases up to and flattens out around α = 0.85–0.9. A detailed investigation of the polarization inducing capacity of these two mechanisms in combination with attraction, and their polarization and repolarization characteristics are described in the comparisons below.
Figure 2. Burst-and-glide and burst-and-stop simulations. (A) Group types produced in the burst-and-glide model (V). From left to right: polarized group (c = 0.2), mill (c = 1) and swarm (c = 2). Points indicate particle positions and the red rods represent the current heading of each particle. (B) Group types produced in the burst-and-stop model. From left to right: polarized group (c = 0.2), mill (c = 1) and swarm (c = 2). (C) Common modified Weibull distribution (Γ(4, 3)) as measured in simulations. (D) Speed profiles of two particles in a burst-and-glide model simulation. We note the characteristic burst-and-glide dynamics in both, with bursts to maximum speed (δ) and exponential decrease in speed between the bursts. (E) Speed profiles of two particles in burst-and-stop model simulation. We note the burst-and-stop dynamics in both, with bursts to maximum speed (δ) and speed 0 between the bursts. (F) Polarization curve through a simulation of the burst-and-glide model. At time 0 polarization is low and after about 2 × 104 timesteps polarization starts to build up and at 3.5 × 104 it begins to stabilize at around 0.85–0.9. (G) Polarization curve through a simulation of the burst and stop model. At time 0 polarization is low (uniformly random headings) and after about 5 × 103 timesteps polarization starts to build up and at 7 × 103 it begins to stabilize at around 0.85–0.9.
The models that include attraction induce polarization over different attraction ranges (see Figure 3). We note that three of the mechanisms induce polarization only when attraction is weak, random sequential asynchrony (III) (c = 0.1and0.2) [16], burst-and-glide (V) (c = 0.2), burst-and-stop (VI) (c = 0.1and0.2). Asymmetric interactions (II), on the other hand, only induce polarization when attraction is stronger c ≥ 0.3. Explicit alignment (VII) induces polarization for c ≤ 0.4 when a = 0.01, and this upper c limit will increase with a (see Supplementary Figure 3). In contrast to all other mechanisms anticipation (IV) reliably induces polarization in different parts of the attraction parameter space, in particular, for weak attraction c ≤ 0.2 and strong attraction c > 1 [17].
Figure 3. Comparison of models (I)–(VII) over c from 0 to 2. Each plot represents the distribution of polarization values α returned from 100 simulations for each c from 0 to 2 in increments of 0.1. For a particular c value the plot color coding shows the proportion of simulations that returned a particular polarization value, the darker the band the more simulations returned that polarization value. For example, for model (III) with c = 0.1 all simulations returned a polarization value close to 1 (so only polarized groups formed) and with c = 0.5 all simulations returned a polarization value close to 0 (so polarized groups did not form). We note that the synchronous LAM (I) does not produce polarized groups for any value of c, that there are four models that induce polarization for small c only (III,V,VI,VII), one model that induces polarized group formation for larger c only (II), and one model that induces polarized group formation for both small and larger c (IV). We note that the results for (I,III) were originally published in [16] and the result for (IV) in [17].
The route to polarization from uniformly random initial conditions is markedly different for the different mechanisms. Some are stronger polarization inducing mechanisms and polarize the groups fast, and some are weaker and polarize the group more slowly, on average. Figure 4A shows that explicit alignment alone (VIII) and anticipation with larger c (IV with c = 2) polarize groups very fast on average (t < 100). Explicit alignment in combination with attraction (VI) is initially on par with (VIII) and (IV with c = 2) but is slower in the final approach to max polarization. Anticipation when attraction is weak (IV with c = 0.2) is not far behind (t < 600) and asymmetric interactions (III) generate polarized groups at an intermediate rate (t < 2, 000), on average. Figure 4B shows that the three asynchronous update models (III,V,VI) tend to take longer to polarize the groups (t > 2, 000). (See Supplementary Figure 1) for the median curves used to create Figures 4A,B with mad error bars. Figure 4C shows the relative frequency distributions of the time to polarized group formation, and the median ± mad for the eight models are (II) 1051 ± 848, (III) 3729 ± 1414, (IV with c=0.2) 471 ± 117, (IV with c=2) 72 ± 72, (V) 12959 ± 4953, (VI) 7319 ± 1765, (VII) 365 ± 129, and (VIII) 74 ± 20. (See Supplementary Videos 1, 2 for simulations showing the polarization process for each of these models).
Figure 4. Polarization processes for the eight models. (A) Median polarization curves for the models focused on those that generate polarized groups at a fast or intermediate rate (III,IV,VII,VIII). (B) Median polarization curves for the models focused on the three models that polarize the group more slowly (II,V,VI). (C) Relative frequency distributions of the time to polarization for each model.
The polarized groups generated by the different mechanisms exhibit different repolarization behavior following strong perturbations. Figure 5A shows the standardized median repolarization curve for each model. We see that the alignment based models (VII,VIII) accumulate most of the polarization very early in the process, on average, and that some models accumulate polarization at an almost linear rate (II,V). (See Supplementary Figure 2) for the median curves used to create Figure 5A with mad error bars. Figure 5B shows the relative frequency distributions of the time to polarization, and the median ± mad for the eight models are (II) 714 ± 848, (III) 799 ± 1203, (IV with c=0.2) 280 ± 140, (IV with c=2) 8 ± 181, (V) 9853 ± 4904, (VI) 5353 ± 1560, (VII) 466 ± 134, and (VIII) 460 ± 138.
Figure 5. Repolarization processes for the eight models. (A) Median standardized repolarization curves for all models. 0 corresponds to the time the strong perturbation occurred and 1 corresponds to when full polarization was reached. We note that explicit alignment alone (VIII) and in combination with attraction (VII) are virtually indistinguishable, the curves overlap, and they exhibit an exponential repolarization process. We also note that (II) and (III) do start of recovering polarization at an exponential rate, but the process is interrupted and from then on the polarization accumulation for (II) is almost linear, and for (IV) with c = 0.2 moderately superlinear. The curve for (IV) with c = 2 exhibits a similar polarization accumulation process as (IV) with c = 0.2, but the initial accumulation is interrupted later within the repolarization period and the post interruption increase is faster. (II) and (V) effectively accumulate polarization at a linear rate throught the process, and (VI) exhibits a moderately superlinear accumulation. (B) Relative frequency distributions of the (simulation) time to polarization for each model. Here, we can visually confirm the calculated median (simulation) time steps to repolarization for each of the models. (II) 714, (III) 799, (IV with c=0.2) 280, (IV with c = 2) 8, (V) 9853, (VI) 5353, (VII) 466, and (VIII) 460. The main thing to note here is that the median repolarization time of the anticipation with strong attraction model (IV with c = 2) is only 8 time steps. Far faster than the second and third fastest models which repolarize in 466 (VII) and 460 (VIII) time steps, respectively.
The route to polarization (Figure 4) and repolarization behavior (Figure 5) are significantly affected by particle number (N) for some mechanisms, but left effectively unchanged for others (see Figure 6). The top panels in Figure 6 shows that both burst-and-glide (V) and burst-and-stop (VI) generates polarized groups faster as the number of particles increase, and that the polarization curves for the other mechanisms are relatively unaffected by change in particle number. The bottom panels in Figure 6 shows that the repolarization behavior of burst-and-glide (V), and to a lesser extent burst-and-stop (VI), are affected by particle number. In particular, for particle numbers less than 100, burst-and-glide exhibits sublinear polarization curves, but for N > 100 the curves become superlinear. The repolarization behavior of burst-and-stop (VI) also becomes more superlinear with increasing particle number, but the basic shape of the repolarization curves for the other mechanisms remain relatively unaffected.
Figure 6. Impact of particle number on the polarization and repolarization processes. Top panels show the route to polarization for the eight models with N = 50, 75, 200, and 1,000 particles, and the bottom panels the corresponding repolarization curves. We note that the polarization curves for models V and VI change significantly with increasing particle number. In particular, the polarization process of model V is, on average, more than four times faster with N = 1, 000 than with N = 50. The polarization processes of models II–IV and VII–VIII do not show any drastic changes with increasing particle number. Similarly, the repolarization curves for models V and VI are dramatically changed by particle number. In particular, the repolarization process of model V is sublinear for N = 50 − 75, but distinctly superlinear for N = 1, 000. The repolarization curves for models II–IV and VII–VIII retain their basic shape for increasing particle number, but the curves are moderately shifted. In particular, for the models with alignment (VII,VIII) more polarization is accumulated early in the process as particle number increases, whereas the alignment-free models accumulate less of the polarization early in the process as particle number increases.
How collective motion in moving animal groups emerges has been a question of debate. Explicit alignment was, and to a large extent still is, the standard explanation. However, motivated by both experimental and theoretical findings over the past decade the role of explicit alignment as the driver of collective motion has come into question. In particular, a number of biologically plausible auxiliary mechanisms have been shown to induce polarized collective motion in combination with attraction, another fundamental interaction operating in moving animal groups. However, a comparison of the effects of these recently discovered mechanisms between themselves or with explicit alignment has not been conducted and it is still unclear exactly how one can distinguish the effects of the different mechanisms. Here, we present such a comparison, including two previously unreported mechanisms that generate collective motion in combination with attraction; burst-glide asynchrony in update, burst-stop asynchrony, in update.
The finding that burst-glide and burst-stop asynchrony induce polarization in combination with attraction here is important in its own right. While it was previously known that sequential random asynchrony induces polarization [16], this particular type of asynchrony is less biologically plausible than the burst-and-glide/stop asynchrony that has been observed in animals across taxa [38–44]. Our findings suggest that it might be the attraction and burst-and-glide/stop dynamics that leads to the polarization observed in some of these groups, not explicit alignment interactions. In particular, [41, 46] has modelled experiments involving burst-and-glide moving rummy nose tetra fish using a model based on attraction and explicit alignment interactions. Investigating how a model based on attraction and burst-and-glide asynchrony compare with the attraction and alignment model originally proposed for this system could be useful as a benchmark to examine if their respective effects can be distinguished when parameterized by, and compared to, the same data of a specific system. Similarly, while the current explanations for the schooling behavior of fish in [10, 11] do not involve explicit alignment, they do involve attraction and asymmetric interactions (potentially via blind zones). Given that some fish species have a very large field of vision [48], including the Golden shiner used in [11], perhaps blind zones are not the main polarization inducing mechanism at play in these systems. At least, attraction and burst-and-glide dynamics might offer an alternative explanation.
It is well-known that a large number of different spp models can produce the same type of groups, in particular, polarized groups, mills and swarms, and thus we cannot infer which mechanisms led to a particular group from the final result alone [2, 49]. Our work shows that focusing on the polarization process might provide additional ways to distinguish between mechanisms proposed to be operating in groups that tend to polarize. As a complement to currently used methods focusing on macroscopic properties of the groups and microscopic analysis of individual behavior and interaction patterns [2, 25, 49]. In particular, our comparison shows that some of the polarization inducing mechanisms included here induce polarization in different parts of the parameter space (Figure 3). This observation might help narrow down the potential candidate mechanisms, given that attraction may be gauged from trajectory data [25]. For example, for animals that exhibit polarized collective motion and very strong attraction, asymmetric interactions or anticipation may be more plausible explanations than sequential random and burst-and-glide/stop, because the latter do not induce polarization for strong attraction, at least in our framework. The observation that some mechanisms polarize the groups faster than others (Figure 4) may also be used to distinguish between proposed candidate mechanisms for a given situation. For example, if very rapid polarization from uniformly random initial configurations are observed, out of the mechanisms included here, only explicit alignment, anticipation, and asymmetric interactions are likely candidates. However, if the process is slower the other mechanisms might be more plausible drivers. The repolarization curves (Figure 5) could similarly be used to distinguish between mechanisms from trajectory data in experiments where groups are repeatedly perturbed. Given that inferring the interaction rules from data of steady state configurations often is less informative than inferences based on the approach towards the stable configuration [49] a perturbation approach might be particularly fruitful to address certain questions. In particular, it seems unlikely that any statistical method that allows for the detection of an explicit alignment interaction will fail to detect it in data collected mainly from a stable polarized group, regardless of how that group level alignment was induced. Perturbation experiments, similar in setup to experiments designed to study fish escape behavior [50, 51], but with repeated perturbations may help resolve this issue in some cases.
Here, we have focused on the polarization inducing capacity of the mechanisms, and thus on polarized groups, however, it is worth noting that all included models, except explicit alignment (VIII), also generate mills and swarms. While this is not surprising given that mills and swarms are produced by the synchronous local attraction model [16], to which each of the mechanisms were added in (II)–(VII), the fact that all attraction based alternatives here produce all the three standard groups: polarized groups, mills, and swarms, but the polarization is induced by different mechanisms in each case suggests that this is a useful and versatile class of models for collective motion.
In conclusion, our work shows that alignment based and non-alignment based mechanisms may be distinguished from their polarization processes and how they interact with attraction. In particular, models containing explicit alignment exhibit exponential polarization accumulation processes whereas most non-alignment based processes exhibit more moderate polarization accumulation processes, anticipation being the exception. In addition, explicit alignment based models polarize faster from uniformly random initial configurations than they repolarize following strong perturbations, in contrast to all attraction based models that repolarize faster than they polarize from uniformly random configurations. These insights could potentially be used to probe whether explicit alignment is operating in a particular group or not via perturbation experiments. However, as described in the simulation and analysis section the analysis presented here is based on the limited ranges/values of the auxiliary model parameters (c, R, δ, τ) used in [13, 16, 17], and the results may depend on the actual parameters used. Therefore, anyone planning to utilize the approach introduced here for situations beyond these parameter ranges should generate the polarization and repolarization curves corresponding to their situation and parameter values of interest first. To facilitate such analysis for specific experimental systems, or further theoretical study, we provide the full code needed to generate the polarization curves (Figure 4) and repolarization curves (Figure 5) for parameter values beyond those considered here. See the Data availability statement for how to access it.
All code required to verify the results in this manuscript, with information about how to modify/extend the analysis, can be found at: https://github.com/danielstrombom/AttractionVsAlignment.
DS conceived the study and analyzed the simulation results. DS and GT finalized the design, performed simulations, and wrote the manuscript. Both authors contributed to the article and approved the submitted version.
The authors declare that the research was conducted in the absence of any commercial or financial relationships that could be construed as a potential conflict of interest.
All claims expressed in this article are solely those of the authors and do not necessarily represent those of their affiliated organizations, or those of the publisher, the editors and the reviewers. Any product that may be evaluated in this article, or claim that may be made by its manufacturer, is not guaranteed or endorsed by the publisher.
We thank the reviewers for their useful and constructive comments that has helped improve this manuscript significantly.
The Supplementary Material for this article can be found online at: https://www.frontiersin.org/articles/10.3389/fams.2021.717523/full#supplementary-material
1. ^But note that time to polarization is a positive quantity.
4. Reynolds CW. Flocks, herds and schools: a distributed behavioral model. SIGGRAPH Comput Graph. (1987) 21:25–34.
5. Couzin ID, Krause J, James R, Ruxton GD, Franks NR. Collective memory and spatial sorting in animal groups. J Theor Biol. (2002) 218:1–11. doi: 10.1006/jtbi.2002.3065
6. Lukeman R, Li YX, Edelstein-Keshet L. Inferring individual rules from collective behavior. Proc Natl Acad Sci USA. (2010) 107:12576–80. doi: 10.1073/pnas.1001763107
7. Kisoma LN, Torney C, Kuznetsov D, Treydte AC. An investigation of power law distribution in wildebeest (Connochaetes taurinus) herds in Serengeti National Park, Tanzania. Commun Math Biol Neurosci. (2020) 2020:66. doi: 10.28919/cmbn/4943
8. Mudaliar RK, Schaerf TM. Examination of an averaging method for estimating repulsion and attraction interactions in moving groups. PLoS ONE. (2020) 15:e0243631. doi: 10.1371/journal.pone.0243631
9. Cao F, Motsch S, Reamy A, Theisen R. Asymptotic flocking for the three-zone model. Math Biosci Eng. (2020) 17:7692–707. doi: 10.3934/mbe.2020391
10. Herbert-Read JE, Perna A, Mann RP, Schaerf TM, Sumpter DJ, Ward AJ. Inferring the rules of interaction of shoaling fish. Proc Natl Acad Sci USA. (2011) 108:18726–31. doi: 10.1073/pnas.1109355108
11. Katz Y, Tunstrøm K, Ioannou CC, Huepe C, Couzin ID. Inferring the structure and dynamics of interactions in schooling fish. Proc Natl Acad Sci USA. (2011) 108:18720–25. doi: 10.1073/pnas.1107583108
12. Romanczuk P, Couzin ID, Schimansky-Geier L. Collective motion due to individual escape and pursuit response. Phys Rev Lett. (2009) 102:010602. doi: 10.1103/PhysRevLett.102.010602
13. Strömbom D. Collective motion from local attraction. J Theor Biol. (2011) 283:145–51. doi: 10.1016/j.jtbi.2011.05.019
14. Ferrante E, Turgut AE, Dorigo M, Huepe C. Elasticity-based mechanism for the collective motion of self-propelled particles with springlike interactions: a model system for natural and artificial swarms. Phys Rev Lett. (2013) 111:268302. doi: 10.1103/PhysRevLett.111.268302
15. Barberis L, Peruani F. Large-scale patterns in a minimal cognitive flocking model: incidental leaders, nematic patterns, and aggregates. Phys Rev Lett. (2016) 117:248001. doi: 10.1103/PhysRevLett.117.248001
16. Strömbom D, Hassan T, Hunter Greis W, Antia A. Asynchrony induces polarization in attraction-based models of collective motion. R Soc Open Sci. (2019) 6:190381. doi: 10.1098/rsos.190381
17. Strömbom D, Antia A. Anticipation induces polarized collective motion in attraction based models. Northeast J Complex Syst (NEJCS). (2021) 3:2. doi: 10.22191/nejcs/vol3/iss1/2
18. Strömbom D, Siljestam M, Park J, Sumpter DJ. The shape and dynamics of local attraction. Eur Phys J Spec Top. (2015) 224:3311–23. doi: 10.1140/epjst/e2015-50082-8
19. Tunstrøm K, Katz Y, Ioannou CC, Huepe C, Lutz MJ, Couzin ID. Collective states, multistability and transitional behavior in schooling fish. PLoS Comput Biol. (2013) 9:e1002915. doi: 10.1371/journal.pcbi.1002915
20. Romey WL, Vidal JM. Sum of heterogeneous blind zones predict movements of simulated groups. Ecol Model. (2013) 258:9–15. doi: 10.1016/j.ecolmodel.2013.02.020
21. Huang XQ, Zhu WJ, Liao JJ, Ai BQ. Directed transport of self-propelled particles with local attraction. Phys A Stat Mech Appl. (2020) 553:124632. doi: 10.1016/j.physa.2020.124632
22. Newman JP, Sayama H. Effect of sensory blind zones on milling behavior in a dynamic self-propelled particle model. Phys Rev E. (2008) 78:011913. doi: 10.1103/PhysRevE.78.011913
23. Ekesten B, Ofri R. Fundamentals of animal vision. In: Veterinary Ophthamology, Vol. 1, 6th ed. Hoboken, NJ: John Wiley & Sons (2021). p. 225–259.
24. McComb DM, Kajiura SM. Visual fields of four batoid fishes: a comparative study. J Exp Biol. (2008) 211:482–90. doi: 10.1242/jeb.014506
25. Herbert-Read JE. Understanding how animal groups achieve coordinated movement. J Exp Biol. (2016) 219:2971–83. doi: 10.1242/jeb.129411
26. Olberg RM. Visual control of prey-capture flight in dragonflies. Curr Opin Neurobiol. (2012) 22:267–71. doi: 10.1016/j.conb.2011.11.015
27. Ghose K, Horiuchi TK, Krishnaprasad P, Moss CF. Echolocating bats use a nearly time-optimal strategy to intercept prey. PLoS Biol. (2006) 4:e108. doi: 10.1371/journal.pbio.0040108
28. Kane SA, Fulton AH, Rosenthal LJ. When hawks attack: animal-borne video studies of goshawk pursuit and prey-evasion strategies. J Exp Biol. (2015) 218:212–22. doi: 10.1242/jeb.108597
29. Johansson A. Constant-net-time headway as a key mechanism behind pedestrian flow dynamics. Phys Rev E. (2009) 80:026120. doi: 10.1103/PhysRevE.80.026120
30. Moussaïd M, Helbing D, Theraulaz G. How simple rules determine pedestrian behavior and crowd disasters. Proc Natl Acad Sci USA. (2011) 108:6884–8. doi: 10.1073/pnas.1016507108
31. Bailo R, Carrillo JA, Degond P. Pedestrian models based on rational behaviour. In: Crowd Dynamics, Vol. 1. Springer, 2018. p. 259–292.
32. Morin A, Caussin JB, Eloy C, Bartolo D. Collective motion with anticipation: flocking, spinning, and swarming. Phys Rev E. (2015) 91:012134. doi: 10.1103/PhysRevE.91.012134
33. Baggaley AW. Stability of model flocks in a vortical flow. Phys Rev E. (2016) 93:063109. doi: 10.1103/PhysRevE.93.063109
34. Gerlee P, Tunstrøm K, Lundh T, Wennberg B. Impact of anticipation in dynamical systems. Phys Rev E. (2017) 96:062413. doi: 10.1103/PhysRevE.96.062413
35. Bode NW, Faria JJ, Franks DW, Krause J, Wood AJ. How perceived threat increases synchronization in collectively moving animal groups. Proc Roy Soc London Biol Sci. (2010) 277:3065–70. doi: 10.1098/rspb.2010.0855
36. Bode NW, Franks DW, Wood AJ. Making noise: emergent stochasticity in collective motion. J Theor Biol. (2010) 267:292–9. doi: 10.1016/j.jtbi.2010.08.034
37. Ariel G, Ayali A. Locust collective motion and its modeling. PLoS Comput Biol. (2015) 11:e1004522. doi: 10.1371/journal.pcbi.1004522
38. Kramer DL, McLaughlin RL. The behavioral ecology of intermittent locomotion. Am Zool. (2001) 41:137–53. doi: 10.1093/icb/41.2.137
39. Gleiss AC, Jorgensen SJ, Liebsch N, Sala JE, Norman B, Hays GC, et al. Convergent evolution in locomotory patterns of flying and swimming animals. Nat Commun. (2011) 2:1–7. doi: 10.1038/ncomms1350
40. Kalueff AV, Gebhardt M, Stewart AM, Cachat JM, Brimmer M, Chawla JS, et al. Towards a comprehensive catalog of zebrafish behavior 1.0 and beyond. Zebrafish. (2013) 10:70–86. doi: 10.1089/zeb.2012.0861
41. Calovi DS, Litchinko A, Lecheval V, Lopez U, Escudero AP, Chaté H, et al. Disentangling and modeling interactions in fish with burst-and-coast swimming reveal distinct alignment and attraction behaviors. PLoS Comput Biol. (2018) 14:e1005933. doi: 10.1371/journal.pcbi.1005933
42. Williams TM, Fuiman LA, Davis RW. Locomotion and the cost of hunting in large, stealthy marine carnivores. Integr Comp Biol. (2015) 55:673–82. doi: 10.1093/icb/icv025
43. Ribak G, Weihs D, Arad Z. Submerged swimming of the great cormorant Phalacrocorax carbo sinensis is a variant of the burst-and-glide gait. J Exp Biol. (2005) 208:3835–49. doi: 10.1242/jeb.01856
44. Miller P. A possible sensory function for the stop–go patterns of running in phorid flies. Physiol Entomol. (1979) 4:361–70. doi: 10.1111/j.1365-3032.1979.tb00628.x
45. Vicsek T, Czirók A, Ben-Jacob E, Cohen I, Shochet O. Novel type of phase transition in a system of self-driven particles. Phys Rev Lett. (1995) 75:1226. doi: 10.1103/PhysRevLett.75.1226
46. Gautrais J, Ginelli F, Fournier R, Blanco S, Soria M, Chaté H, et al. Deciphering interactions in moving animal groups. PLoS Comput Biol. (2012) 8:e1002678. doi: 10.1371/journal.pcbi.1002678
47. Iliass T, Cambui D. The combined effect of attraction and orientation zones in 2D flocking models. Int J Mod Phys B. (2016) 30:1650002. doi: 10.1142/S0217979216500028
48. Pita D, Moore BA, Tyrrell LP, Fernández-Juricic E. Vision in two cyprinid fish: implications for collective behavior. PeerJ. (2015) 3:e1113. doi: 10.7717/peerj.1113
49. Mann R. Bayesian inference for identifying interaction rules in moving animal groups. PLoS ONE. (2011) 6. doi: 10.1371/journal.pone.0022827
51. Herbert-Read JE, Buhl J, Hu F, Ward AJ, Sumpter DJ. Initiation and spread of escape waves within animal groups. R Soc Open Sci. New York, NY: John Wiley & Sons (2015) 2:140355. doi: 10.1098/rsos.140355
As outlined in the main text, the burst-and-glide (V) and burst-and-stop (VI) models are modified versions of the local attraction model (LAM) [13]. The LAM is a self-propelled particle model where N particles interact via local attraction only (see Figure 1A). With the notation in Figure 1A the positional update formula for the local attraction model can be expressed as
where δ is constant. In order to extend this model to animals using burst-and-glide locomotion δ must be redefined. From the literature we know that during the burst phase, fish and other animals, quickly accelate to a certain speed and then during the glide phase the speed drops exponentially until the next burst [41]. To create a simple model of this behavior we define a speed function that is a function of time t, particle i's most recent burst time Ti, t, a constant representing the particles maximum speed δmax, and a rate of speed decay constant k > 0, defined by
We note that δ = δmax at the burst time t = Ti, t and after this time, when t > Ti, t, the term k(Ti, t−t) is negative and decreasing so δ is decreasing exponentially from δmax at a rate determined by the constant k. Substituting equation 6 in 5 gives us the positional update formula for the local attraction model with individual burst-and-glide locomotion
and the burst-and-glide behavior will be determined by the process that defines the evolution of burst times Ti, t. Here we define this process in terms of the distribution of times between two successive bursts Γ because it has been empirically estimated in experiments with fish [41]. We call the time between two successive bursts the glide time and denote the current glide time of particle i by γi, t. In order to utilize equation 7 to update the particle positions we must define the processes for Ti, t and γi, t so that they are both constant over the current glide period and then both updated when the current glide period is over. A simple way to achieve this is to define them as follows
So while particle i is still in its current glide period (Ti, t−1+γi, t−1 > t) both Ti, t−1 and γi, t−1 remain unchanged as t increases, but as soon as the current glide period ends (Ti, t−1+γi, t−1 = t) Ti, t is set to the current time t and a new current glide time γi, t is drawn from the distribution Γ. We choose the distribution Γ for the current work to be a Weibull distribution with shape parameter κ = 4 and shape parameter λ = 3 because this choice leads to a glide time distribution (Figure 2C) that is similar to Figure 1G in [41].
Above we have described the burst-and-glide model. The burst-and-stop model works exactly the same way except that δ is set to 0 after the burst rather than decrease in an exponential fashion. See the Data availablity statement for how to access the full model code.
Keywords: flocking, self-propelled particles, polarization, animal behavior, schooling, swarming, intermittent locomotion, burst and glide
Citation: Strömbom D and Tulevech G (2022) Attraction vs. Alignment as Drivers of Collective Motion. Front. Appl. Math. Stat. 7:717523. doi: 10.3389/fams.2021.717523
Received: 31 May 2021; Accepted: 24 December 2021;
Published: 31 January 2022.
Edited by:
Raluca Eftimie, University of Franche-Comté, FranceReviewed by:
Miguel Pineda, University College London, United KingdomCopyright © 2022 Strömbom and Tulevech. This is an open-access article distributed under the terms of the Creative Commons Attribution License (CC BY). The use, distribution or reproduction in other forums is permitted, provided the original author(s) and the copyright owner(s) are credited and that the original publication in this journal is cited, in accordance with accepted academic practice. No use, distribution or reproduction is permitted which does not comply with these terms.
*Correspondence: Daniel Strömbom, c3Ryb2VtYnBAbGFmYXlldHRlLmVkdQ==
Disclaimer: All claims expressed in this article are solely those of the authors and do not necessarily represent those of their affiliated organizations, or those of the publisher, the editors and the reviewers. Any product that may be evaluated in this article or claim that may be made by its manufacturer is not guaranteed or endorsed by the publisher.
Research integrity at Frontiers
Learn more about the work of our research integrity team to safeguard the quality of each article we publish.