- 1Genebank Department, Leibniz Institute of Plant Genetics and Crop Plant Research (IPK), Gatersleben, Germany
- 2Department of Agronomy, Faculty of Agriculture, Assiut University, Assiut, Egypt
- 3Department of Genetics, Faculty of Agriculture, Assiut University, Assiut, Egypt
- 4Department of Soil and Water Resources, Faculty of Agriculture, Assiut University, Assiut, Egypt
Introduction: Understanding the dynamics of Pb tolerance across different growth stages is essential for breeding wheat varieties with comprehensive tolerance to Pb contamination throughout their life cycle. Lead (Pb) in soil affects wheat plants at all growth stages. However, the seedling stage is considered a sensitive growth stage for the tolerance of most abiotic stresses. Genetic variation in lead tolerance is poorly understood, and genetic control has still not been investigated.
Materials: In this study, 103 highly diverse wheat genotypes were investigated under normal and lead stress conditions at the seedling stage. Different seedling growth traits were scored under each treatment. GWAS was performed via two different sets of markers: 21,750 (25K Infinium iSelect array) and 37,790 SNPs (genotyping-by-sequencing) to identify SNP markers associated with the studied seedling traits.
Results: High genetic variation was found among all the genotypes for all the traits. High broad-sense heritability estimates ranging from 0.25-0.93 were observed. A highly significant correlation was found among all the traits scored under normal and lead stress conditions. Low or no significant phenotypic correlations were found for lead tolerance between the seedling and adult growth stages. A set of eight genotypes were classified as lead-tolerant genotypes. The GWAS revealed a set of 222 significant markers associated with lead tolerance. Markers with pleiotropic effects were detected under Pb stress and between lead and normal conditions. Interestingly, five significant markers were found to be associated with lead tolerance at the seedling and adult growth stages.
Discussion: The results of this study provide new and novel insights into genetic control and genetic variation in lead tolerance at the early growth stage. All the genes and genotypes reported in this study will be very useful for further investigations of lead tolerance in wheat at different growth stages.
Introduction
Lead (Pb) is one of the most toxic heavy metals that affects wheat crop. The high level of Pb in wheat can reduce growth and development, leading to a significant reduction in wheat yield and affecting the final grain quality. Mourad et al. (2021b) studied the effects of Pb on kernel traits (diameter, width, and length) and thousand-kernel weight. These authors reported a significant reduction in all the wheat grain traits. The kernel diameter and thousand-kernel weight decreased by ~50% due to the effect of Pb. Lead toxicity aggravates important fundamental physiological processes in wheat plants, such as photosynthesis, nutrient uptake, and water absorption (Souahi et al., 2021). It was reported that the acceptable level of lead in wheat flour is 0.15 mg/kg (Saatloo et al., 2023). Wheat contaminated with Pb has detrimental effects on human health, as it affects the nervous system, kidneys, and blood (Flora et al., 2012). Many human diseases, such as kidney dysfunction, diabetes, osteoporosis, high blood pressure, cancer, and infertility, occur due to the consumption of wheat contaminated with Pb (Morias et al., 2012). Wheat contamination with Pb can occur due to many factors, such as contaminated soil (McBride, 2003), water irrigation (Alloway, 2013), the atmosphere (Gupta et al., 2013), and fertilizers (Alloway, 2013). In wheat, lead stress was found to reduce the thousand-kernel weight by 50%, the kernel diameter by 64.4%, the kernel length by 15.8%, and the kernel weight by 32.3% (Mourad et al., 2021b).
The development of lead-tolerant wheat genotypes is key for the production of healthy and nongenetic modified wheat cultivars. To achieve this goal, wheat germplasm should be screened for lead tolerance to capture all genetic variation among genotypes in terms of lead absorbance in soil; hence, tolerant genotypes can be selected (Mourad et al., 2019; Mondal et al., 2021). Many studies have investigated the effects of pb on wheat plants at different growth stages (Lamhamdi et al., 2011, 2013; Semenova et al., 2019; Aslam et al., 2021; Souahi et al., 2021; Nessem et al., 2022; Ma et al., 2023). However, most of these previous studies were performed on a few wheat genotypes. Therefore, it was hard to figure out the different response of wheat seedlings to Pb contamination using few number of genotypes. Moreover, selecting promising genotypes that can tolerate Pb stress across different growth stages will be useful for producing wheat cultivars that are highly tolerant to lead stress. Mourad et al. (2021b) identified eight, ten, and ten genotypes on the basis of stress indices for thousand-kernel weight, kernel length, and kernel width, respectively, and only one genotype was found to be tolerant of all the traits scored in their study. Therefore, more studies are needed to identify genotypes that are more tolerant to Pb stress.
Exploring the genetic control of lead (Pb) tolerance in wheat involves identifying the genes and genetic mechanisms that alleviate the high levels of Pb in the soil (Mourad et al., 2021b, 2023b). This is crucial for developing Pb-tolerant wheat cultivars, especially in areas where soil contamination is a concern. One approach is to identify and characterize the genes involved in Pb tolerance. This can be accomplished through techniques such as quantitative trait loci (QTL) mapping, genome-wide association studies (GWASs), and gene expression analysis (Bálint et al., 2007; Alqudah et al., 2020; Mourad et al., 2024). QTL mapping involves identifying regions of the wheat genome associated with Pb tolerance by analyzing the segregation of phenotypic traits in mapping populations. GWASs look for associations between genetic markers and phenotypic variation across diverse populations of wheat lines. Gene expression analysis helps identify genes whose expression is upregulated or downregulated in response to Pb stress, providing insights into the molecular mechanisms of tolerance. The key genes and pathways involved in Pb tolerance in wheat may include those related to metal transport and sequestration, antioxidant defense systems, and stress signaling pathways. For example, genes encoding transporters such as metal transporters (e.g., the ZIP family), chelators (e.g., metallothionein), and detoxification enzymes (e.g., glutathione S-transferases) are important for Pb uptake, sequestration, and detoxification in plant cells (Singh et al., 2016). Genes involved in antioxidant defense, such as superoxide dismutase (SOD), catalase (CAT), and peroxidases, play a role in mitigating oxidative stress caused by Pb exposure (Rajput et al., 2021). Additionally, stress-responsive genes and signaling pathways, such as those regulated by phytohormones such as abscisic acid (ABA) and jasmonic acid (JA), are implicated in the plant response to Pb stress (Wang et al., 2020).
By understanding the genetic basis of Pb tolerance in wheat, breeders can use molecular breeding techniques to develop improved wheat varieties with increased Pb tolerance, contributing to sustainable agriculture in Pb-contaminated environments. Although traditional breeding programs can be used to address this challenge by selecting and crossing highly tolerant lead genotypes, these tools are labor intensive and time-consuming. Integrating genomic tools in breeding programs will lead to rapid genetic improvement of target traits. Genome-wide association studies (GWAS) can be used to identify alleles associated with important traits (Alqudaha et al., 2019). Candidate genes associated with lead tolerance can subsequently be identified and utilized to develop robust markers that can be used to screen large germplasms and hence accelerate breeding programs (Mourad et al., 2023c, d; Sallam et al., 2024a). Unfortunately, very few studies have been conducted to identify candidate genes associated with lead tolerance in wheat (Mourad et al., 2023b). Mourad et al. (2023b) identified a set of 126 significant single nucleotide polymorphisms in 103 highly diverse wheat genotypes. Unlocking the genetic control of lead tolerance is very important not only for selecting promising genotypes but also for developing DNA markers to accelerate breeding programs. As Pb affects wheat plants at the seedling stage, which is sensitive to biotic and abiotic stresses (Sallam et al., 2018; Amro et al., 2022), it is worth investigating the tolerance mechanism of Pb and selecting genotypes that are prone to Pb stress at this stage.
In this study, we screened the same set evaluated by Mourad et al. (2021b, 2023b) for lead tolerance at the seedling stage to investigate the genetic variation in Pb tolerance at this important growth stage and identify the most durable tolerant genotypes across different growth stages. As Mourad et al. (2021b, 2023b) identified important SNPs associated with Pb tolerance at the adult growth stage, our objectives were also to identify SNP markers associated with Pb tolerance at the seedling stage and detect common markers and genes for Pb tolerance at different growth stages.
Materials and methods
Plant material
The plant material used in this study consisted of 103 spring bread wheat genotypes representing 15 different countries. The genotypes were obtained from the U.S. National Plant Germplasm, United States Department of Agriculture (USDA), United States. The list of genotypes is presented in Supplementary Table S1.
Experimental layout
To test Pb tolerance in the tested genotypes at the seedling growth stage, a seedling experiment was conducted at the Plant Genetics Lab, Genetics Department, Faculty of Agriculture, Assiut University, Egypt, via small trays. In this laboratory, the room temperature of the laboratory was between 23°C and 25°C during the experiment. All the genotypes were tested under normal and Pb-stress conditions. Fertilized soil was collected from the Experimental Field Station at Assiut University and used for normal conditions. For Pb contamination, the same soil used in the normal treatment was contaminated with Pb(NO3)2 by adding 250 ml to each kg of soil. The target concentration was 250 mg/kg of soil (Vodyanitskii, 2016). After soil contamination, three samples were collected from normal and contaminated soils and sent for analysis at the Analytical Chemistry Unit (ACAL), Faculty of Science, Assuit University, Asyut, Egypt.
Two seeds from each genotype were handily sown in a 60-cell plant tray (35 × 21 × 4.4 cm). Each cell in this tray was filled with 50 g of fertilized soil. The soil water capacity was calculated according to Ahmed et al. (2021). The experimental layout was a randomized complete block design with three replications/treatments. After fourteen days, the plants of each genotype were carefully washed to remove the soil. For each seedling, the following traits were measured: biological weight (BW, gm), shoot weight (SW, gm), root weight (RW, gm), the ratio of shoot fresh weight to root fresh weight (SW/RW), number of roots (NR), shoot length (SL, cm), root length (RL, cm), the ratio of shoot length to root length (SL/RL), leaf width (LW, cm), and leaf area (LA, cm2). BW, RW, and SW were measured via a digital balance. SL, RL, NR, LA, and LW were measured via the ImageJ software (Schneider et al., 2012).
Using the average of the three replications of each treatment, the percentage of change in each studied seedling trait due to Pb contamination (CTH-Pb) was calculated via the following formula:
where CTH-Pb represents the change in the studied trait due to Pb contamination; Xc and XPb represent the mean performance of each studied genotype under controlled and contaminated soils, respectively; and a value of minus one (−1) was multiplied by the product to obtain positive values for the traits increased under Pb and negative values for the traits decreased under Pb.
Statistical analysis of the phenotypic data
The analysis of variance (ANOVA) was calculated for all traits via PLABSTAT software (Utz, 1997) via two different models;
where Yijk is the observation of genotype i in replication j in the treatment (normal vs. lead), k and μ are the general means, and tk, rj, and gi refer to the effects of the treatments, replications, and genotypes, respectively. tgik represents the interaction between genotype and treatment. tgrijk represents the interaction among genotype, replication, and treatment (error). Treatments were considered fixed, whereas the remaining factors were considered random. This model was used to test the differences in each studied trait between the control and Pb treatments.
where Yij is the observation of genotype i in replication j, μ is the general mean, and rj and gi refer to the effects of replications and genotypes, respectively. grij represents the interaction among genotype and replication (error). This model was used for each treatment separately to test the differences between the genotypes under each treatment.
The broad-sense heritability was estimated via PLABSTAT via the HERT command (Utz, 1997). Phenotypic correlations between each pair of studied seedling traits under each condition were calculated and visualized via the SRPlot online database available at http://www.bioinformatics.com.cn/srplot.
Selection of genotypes superior to Pb
To determine superior genotypes under Pb conditions at the seedling growth stage, the fifteen best genotypes were identified via the iPASTIC toolkit (Pour-Aboughadareh et al., 2019) average of sum ranks (ASR) for each studied seedling trait separately. Genotypes that were superior in terms of at least four traits were selected. The kernel traits of the same genotypes were previously evaluated and scored under normal and Pb stress conditions by Mourad et al. (2021b). The most tolerant wheat genotypes at the adult growth stage were compared with those detected in this study at the seedling stage to identify the most tolerant wheat genotype at different growth stages.
Genome-wide association study for seedling traits
From each genotype, DNA from two to three leaves (two-week old) was extracted via a BioSprint 96 DNA Plant Kit (Qiagen, Hombrechtikon, Switzerland). The extracted DNA was digested for genotyping-by-sequencing, which was performed at Kansas State University according to Elshire et al. (2011). Additionally, the same DNA samples were genotyped via a 25K Infinium iSelect array (25K-set) by the SGS Institute Fresenius GmbH TraitGenetics Section (Gatersleben, Germany). Genotyping revealed 36,720 and 24600 SNPs for GBS and 25K, respectively. All the markers were filtered according to Alqudah et al. (2020) and Mourad et al. (2020). Finally, a set of 11362 (GBS) and 21093 (25K set) data was used for genome-wide association mapping.
The two marker sets were previously reported to cover different parts of the wheat genome and were used together to identify genomic regions controlling biotic and abiotic stresses in wheat (Esmail et al., 2023b; Mourad et al., 2023b, d). All phenotypic traits scored in this study under normal and Pb stress conditions were integrated with all SNP markers to run a GWAS. The GWAS was conducted via three models: a general linear model (GLM), a mixed linear model (MLM), and FarmCPU. Principal component analysis (PCA) and kinship were considered individually and in combination in each model to correct population structure. Therefore, a total of nine different GWAS models were used to detect marker−trait associations under both conditions via the rMVP R package (Yin et al., 2021). For each trait, the best model that fits the trait was detected on the basis of the distribution of the observed and expected p values in the QQ plot. The stress tolerance index (STI) (Fernandez, 1992) for each studied trait was included in the GWAS to identify the genetic control of Pb tolerance. Markers significantly associated with tolerance were identified as those with a p-value <0.001 (-log10 >3.00). For each significant marker, the target allele has a positive effect and increases the trait as well as the STI. The phenotypic variation explained by each marker was calculated via TASSEL software (Bradbury et al., 2007).
Gene models controlling seedling traits, functional annotation, and gene enrichment
Gene models harboring significant markers were detected by comparing the location of the significant marker with the position of the gene models via the EnsemblePlants database (Bolser et al., 2007). The functional annotation of the identified gene models was performed in accordance with the International Wheat Genome Sequencing Consortium (IWGSC). Furthermore, gene enrichment of the commonly identified gene models based on the biological process pathways was identified via the ShinyGo 0.80 database (Ge et al., 2022), and a cutoff value of false discovery rate (FDR) p value<0.01 was applied. An enrichment plot of the most significant genes was generated via the SRPLOT database (https://www.bioinformatics.com.cn/srplot). A network of the identified biological processes was visualized via NetworkD3 v.4.0, R package (Allaire et al., 2017). Moreover, a network of the identified gene models representing their relationships with heavy metal tolerance was investigated via the KnetMiner database (Hassani-Pak et al., 2021).
Results
The results of the analysis of the soil samples collected after contamination and before contamination are presented in Table 1. Based on the analysis results, a higher content of Pb was detected in the contaminated soils than in the normal soils, with values of 65.81 mg/kg soil and 3.25 mg/kg soil, respectively. The pH values did not differ between the two soil samples, as they were 7.18 and 7.47 in the normal and contaminated soils, respectively. The electrical capacity and content of cations were greater in normal soils than in Pb soils. On the other hand, the Cl- content was greater in Pb soils than in normal soils. The saturation capacity was greater in Pb soils than in normal soils, with values of 57.5 and 54.4 for contaminated and normal soils, respectively.
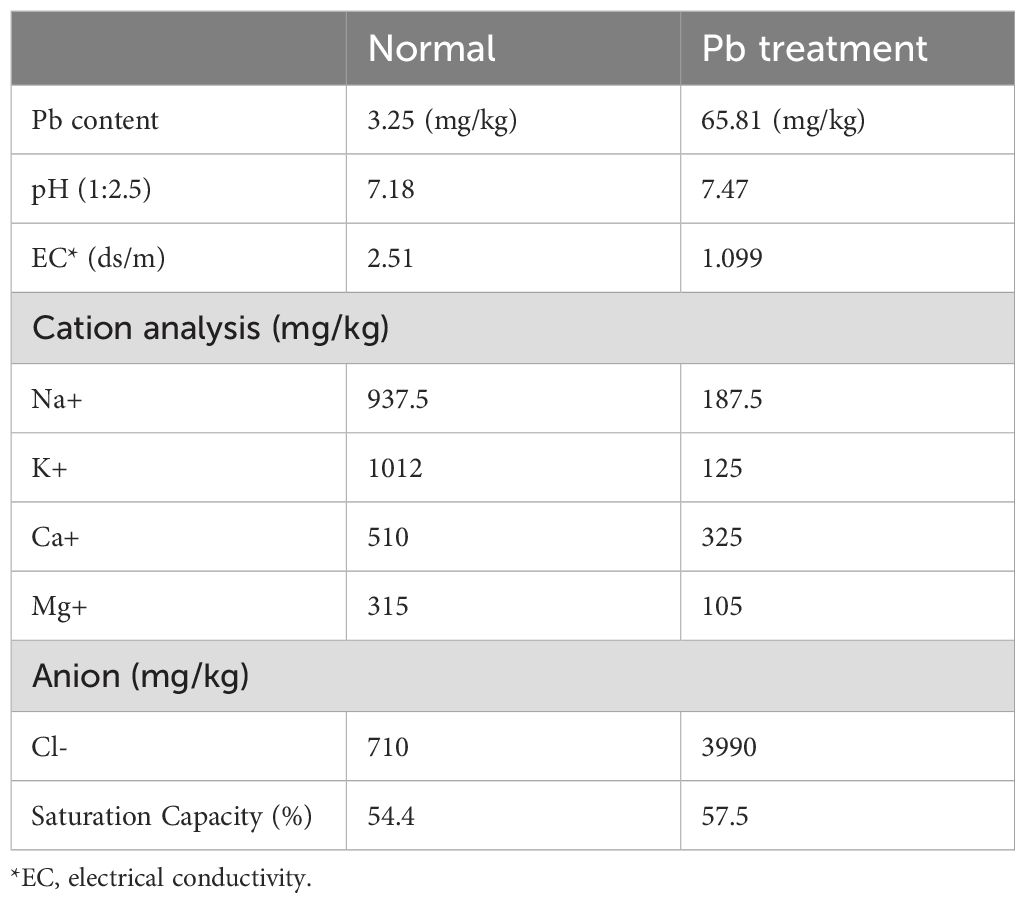
Table 1. Analysis of the soil samples used in the current study before Pb contamination (normal) and after Pb contamination (Pb treatment).
Effect of Pb on wheat seedlings
The minimum, maximum, and average values of all the genotypes for all the traits are presented in Table 2. On average, all traits were lower under Pb stress than under normal conditions. The reduction in each trait due to Pb stress is illustrated in Figure 1. Four traits, BW, RW, LW, and LA, were reduced under Pb, whereas there was a notable increase in SL, RL, and SW/RW due to Pb stress. RW was the trait that decreased the most in response to Pb, whereas BW decreased the least in response to Pb stress. An approximate increase (%) was found in RL and SL.

Table 2. Minimum, maximum, average, standard error (SE), and standard deviation (SD) of the studied seedling traits in control and lead-contaminated soils.
The ANOVA results for all traits under both treatments are presented in Table 3. Highly significant differences were found between the two treatments for all traits except SW, NR, and SL/RL. Highly significant variation was found among all the genotypes for all the traits. The G × T was highly significant for all traits except NR, RL, and LA. The broad-sense heritability (H2) ranged from 0.25 (SW/RW) to 0.93 (BW). Furthermore, highly significant differences were found among the tested genotypes for each studied trait under each condition, except for SW/RW in normal soils (Supplementary Table S2).
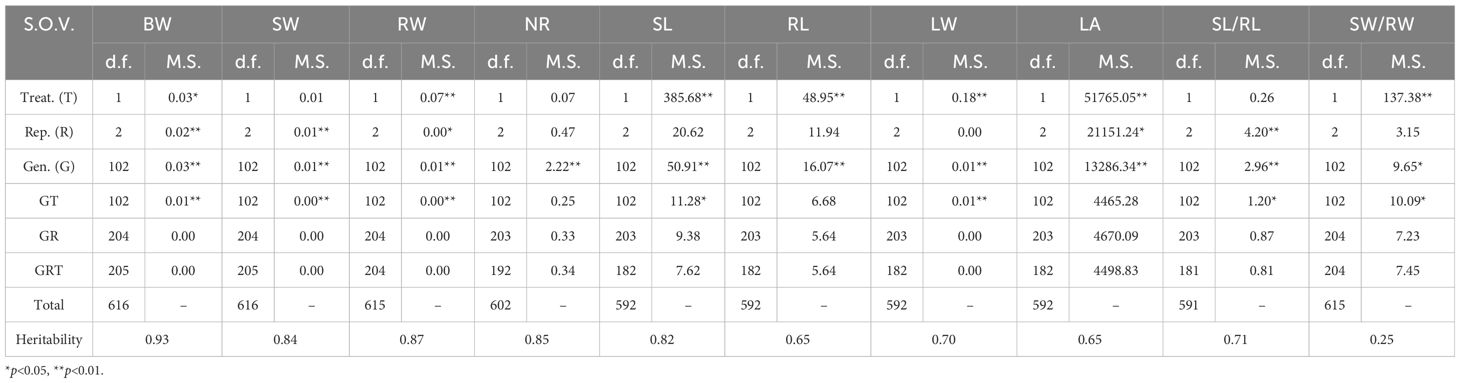
Table 3. Analysis of variance of the studied seedling traits: biological weight (BW), shoot weight (SW), root weight (RW), number of roots (NR), shoot length (SL), root length (RL), leaf width (LW), leaf area (LA), SL/RL ratio, and SW/RW ratio between the control and lead-contaminated experiments.
Phenotypic selection for the most promising lead-tolerant genotypes
The ranks of all the selection indices were used to select the superior genotypes on the basis of each studied trait (Table 4; Supplementary Table S3). On the basis of this ranking, a set of eight genotypes were classified as promising lead-tolerant genotypes on the basis of at least four seedling traits. These selected genotypes were from Iran (three genotypes) and one genotype from each of the following countries: Afghanistan, Canada, Greece, Kazakhstan, and Kenya. Among these genotypes, only one (PI381963) was found to be superior in terms of five traits. The remaining genotypes were superior in terms of four traits; however, a reduction in some traits, such as BW, was detected in some of these genotypes.
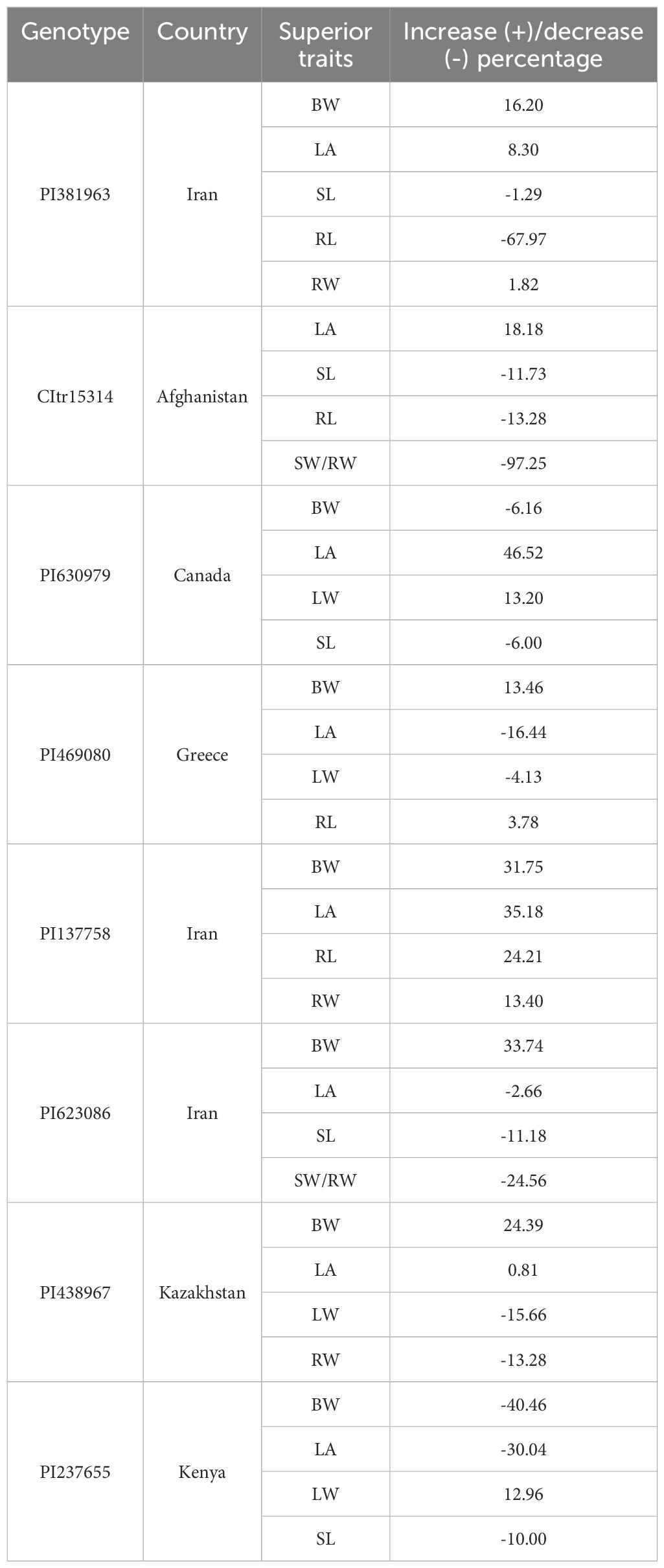
Table 4. List of superior genotypes on the basis of the stress tolerance index (STI) of the studied seedling traits, their country of origin, and their traits.
Phenotypic correlations among seedling traits under normal and Pb-stress conditions
The phenotypic correlations among all the traits under normal and Pb stress conditions are presented in Figures 2A, B, respectively. Under each treatment, a highly significant correlation was found between shoot traits and root traits. Moreover, the phenotypic correlation among shoot traits under Pb was greater than that under normal conditions. Notably, among the shoot traits, the highest correlation under the normal and Pb treatments was found between SL and LA, with r values of 0.69** and 0.85**, respectively. In terms of the root traits, the correlations among the root traits under normal conditions were greater than those under Pb, except for the correlation between NR and RL. A significant positive correlation was detected between shoot and root traits under both treatments. The most significant correlation was found between RW and SW, with r values of 0.51** and 0.50** under normal and Pb stress conditions, respectively. SW also has a stable correlation with NR and RL under both conditions. LW had a more significant phenotypic correlation with NR and RL under normal conditions than Pb stress did. SW/RW had a positive significant correlation with SW and LW only under normal conditions. A highly negative and significant correlation was found between SW/RW and RL under normal (r= -0.81**) and Pb (r= -0.76**) stress. Interestingly, the biological weight (BW) was highly significantly correlated with all traits scored in this study except SW/RW under normal conditions. Furthermore, the results of the principal component analysis (PCA) of all the seedling traits under normal and Pb-stress conditions are presented in Supplementary Figure S1. LA was separated from all other traits, whereas BW, RW, LW, and SW/RW were clustered under both conditions.
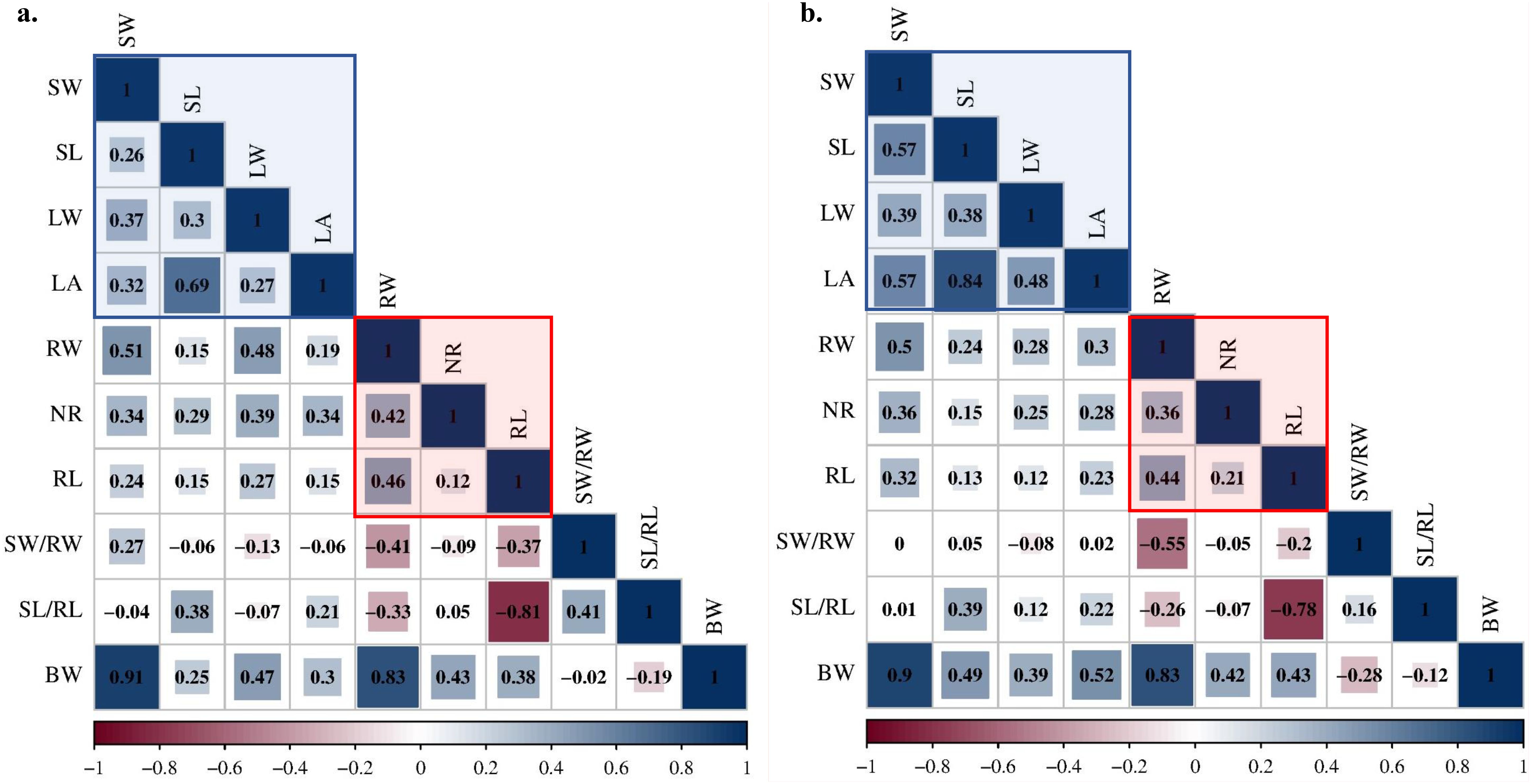
Figure 2. Phenotypic correlation between each pair of the studied seedling traits under controlled soils (A) and lead- contaminated soils (B).
Phenotypic correlation of lead tolerance between the seedling and adult growth stages
As previously mentioned, the same set was evaluated at the adult growth stage by Mourad et al. (2021a). To understand the mechanism of lead tolerance at different growth stages, the correlation between the stress tolerance index (STI) for all seedling traits scored in this study and the stress tolerance index for kernel traits reported by Mourad et al. (2021b) was calculated (Figure 3). Weak and nonsignificant correlations were found among the stress tolerance indices between the two growth stages. The majority of the significant correlations among the STIs between the two growth stages were negative, except for the positive significant correlations between BW and KL (r=0.17*), RW and KL (r=0.13*), and SL and KL (r=0.13*). Furthermore, looking for common tolerant genotypes in both stages that were selected in each study, the superior genotypes identified in this study, as well as the 20 genotypes selected in Mourad et al. (2021b), were completely different from each other.
Genome-wide association study of seedling traits in normal and Pb-contaminated soils
Two marker sets (GBS and 25K) were used to run a GWAS for all traits scored under both conditions. The analysis of the population structure of the 103 genotypes revealed three possible subpopulations (Mourad et al., 2020). On the basis of the QQ plots of the different models studied, FarmCPU+PCA+Kin was the best model for almost all the seedling traits under normal conditions and most of the seedling traits under Pb-contaminated soils. MLM+Kin+PCA was the best model for STI for most of the studied traits (Supplementary Figure S2). A summary of the GWAS results is presented in Table 5, and detailed results are presented in Supplementary Tables S4–S6. Furthermore, Manhattan plots representing the GWAS results for each trait under each condition are presented in Supplementary Figures S3–S5. A total of 198, 228, and 173 significant markers were detected for seedling traits under normal, Pb stress, and Pb tolerance (STI) conditions, respectively. The distribution of the significant SNPs across all chromosomes is presented in Figure 4A. In normal soils, only one significant marker was found to be located at 1D and 4D, while 3B presented the greatest number of significant markers. The lowest number of significant markers under Pb stress was located on 5D and 4D chromosomes, whereas the greatest number of significant markers was found on chromosome 7B.
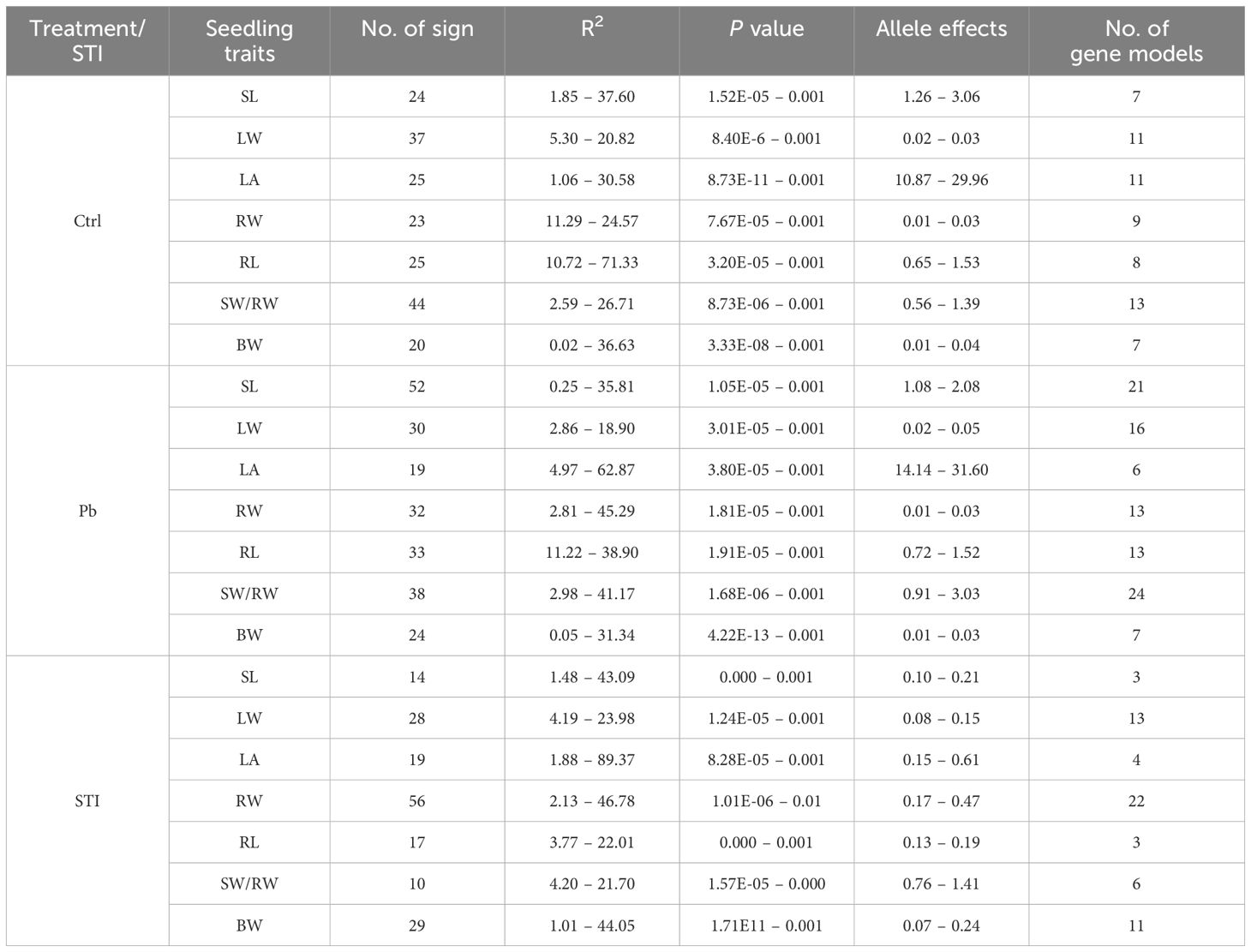
Table 5. Summary of significant markers identified for each treatment on the basis of a genome-wide association study using GBS-SNPs and the iSelect 25K-SNP array.
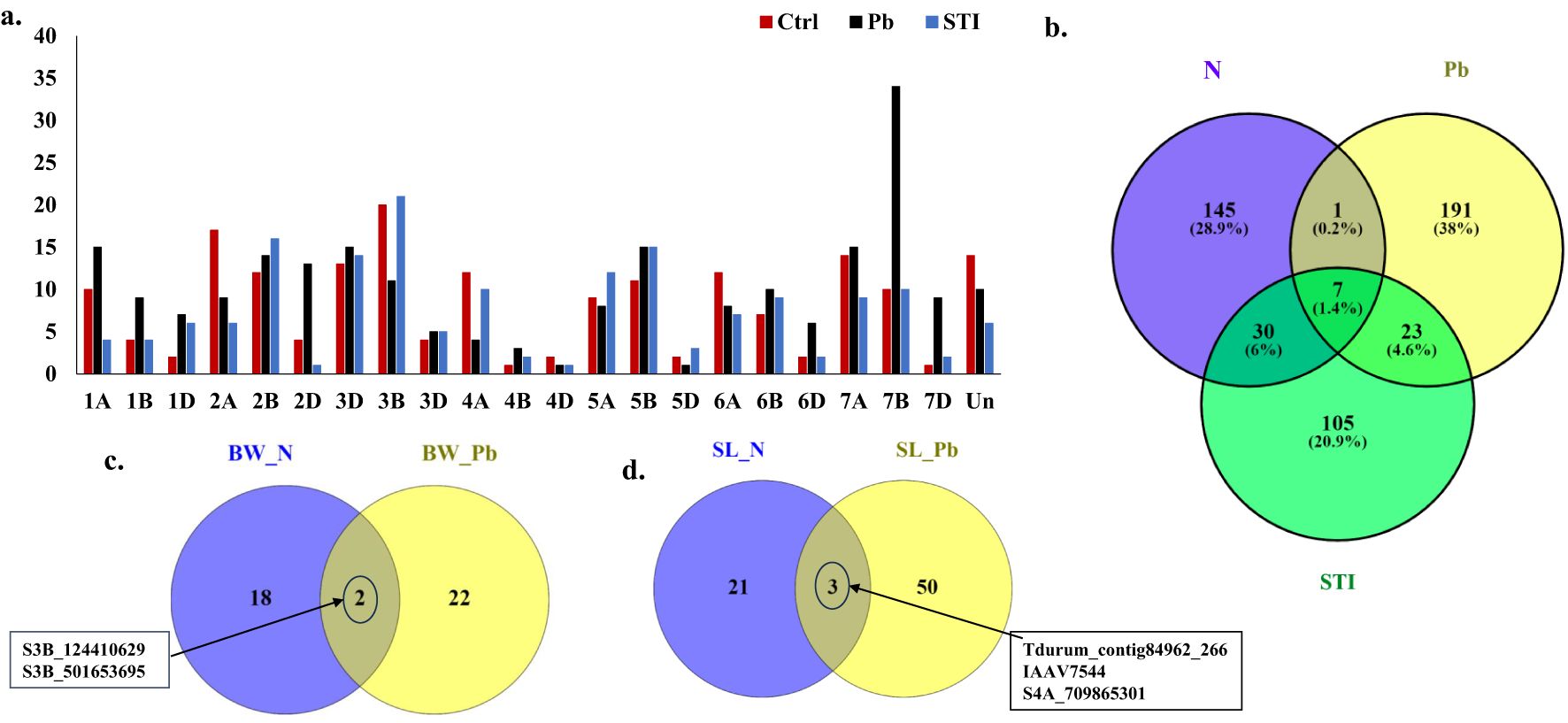
Figure 4. Results of genome-wide association study of seedling traits under controlled soils, Pb-contaminated soils and stress tolerance index (STI); (A) chromosomal location of significant markers under each condition, (B) number of common significant markers associated with the different studied seedling traits under normal soil (N), contaminated soils (Pb), and stress tolerance index (STI), (C) number of common markers associated with biological weight under Pb (BW_Pb) and normal soils (BW_N); (D) number of significant markers associated with shoot length under normal (SL_N) and Pb contaminated soils (SL_Pb).
Notably, SL, RW, RL, and BW presented a higher number of significant markers under Pb stress than under normal conditions. LW, LA, and SW/RW, on the other hand, presented greater numbers of significant markers under normal stress than under Pb stress conditions (Table 5). SW/RW had the greatest number of significant SNPs (44) under normal conditions, while the greatest number of significant SNPs (52) was found for SL under Pb. Among the stress tolerance indices, STI_RW had the greatest number of significant SNPs. Most of the markers had R2 values > 10% under Pb stress (142, with a range of 10.63--62.87%) compared with normal conditions (120, with a range of 10--71%). Notably, all the markers detected for RW and RL presented R2 values > 10% under normal (11.29--24.57%) and Pb-stress (11.61--38.90%) conditions. The GWAS identified markers that were associated with more than one trait (Figure 4B; Table 5). Eight markers were found to be common between normal and Pb-stress conditions. STI had 30 and 37 shared markers detected under Pb and normal conditions, respectively. Under controlled conditions, seven markers located on chromosome 2A were found to be associated with LW and RL (Table 5). Two markers were located on 2B and 6B and were shared between RL and RW, and between SL and SL, and between SW and RW. S3B_124410629 and S7A_2974203 were significantly associated with BW and RW. Under Pb stress, five shared markers, 1D, 1A, 2B, and 3B, were found to be associated with LA and SL. S3B_501653695 was associated with BW and RW, whereas S6B_196678015 was associated with RL and RW. By examining the common markers associated with the same traits under both conditions, two markers (S3B_124410629 and S3B_501653695) located on 3B were found to be associated with BW (Figure 4C), whereas three markers (Tdurum_contig84962_266, IAAV7544, and S4A_709865301) were found to be associated with SL (Figure 4D).
Gene models harboring significant markers were detected, and a total of 46 and 98 gene models were found under normal and Pb stress conditions, respectively (Figure 5A). Interestingly, two candidate genes were found to be common among normal, Pb-susceptible and Pb-tolerant plants. STI shares 15 and 10 candidate genes with normal and Pb stresses, respectively. The positions of important common markers and candidate genes on the chromosomes are illustrated in Figure 5B. Clustered genomic regions that were associated with more than one trait were observed on 3A, 3B, 5A, 6B, and 7B.
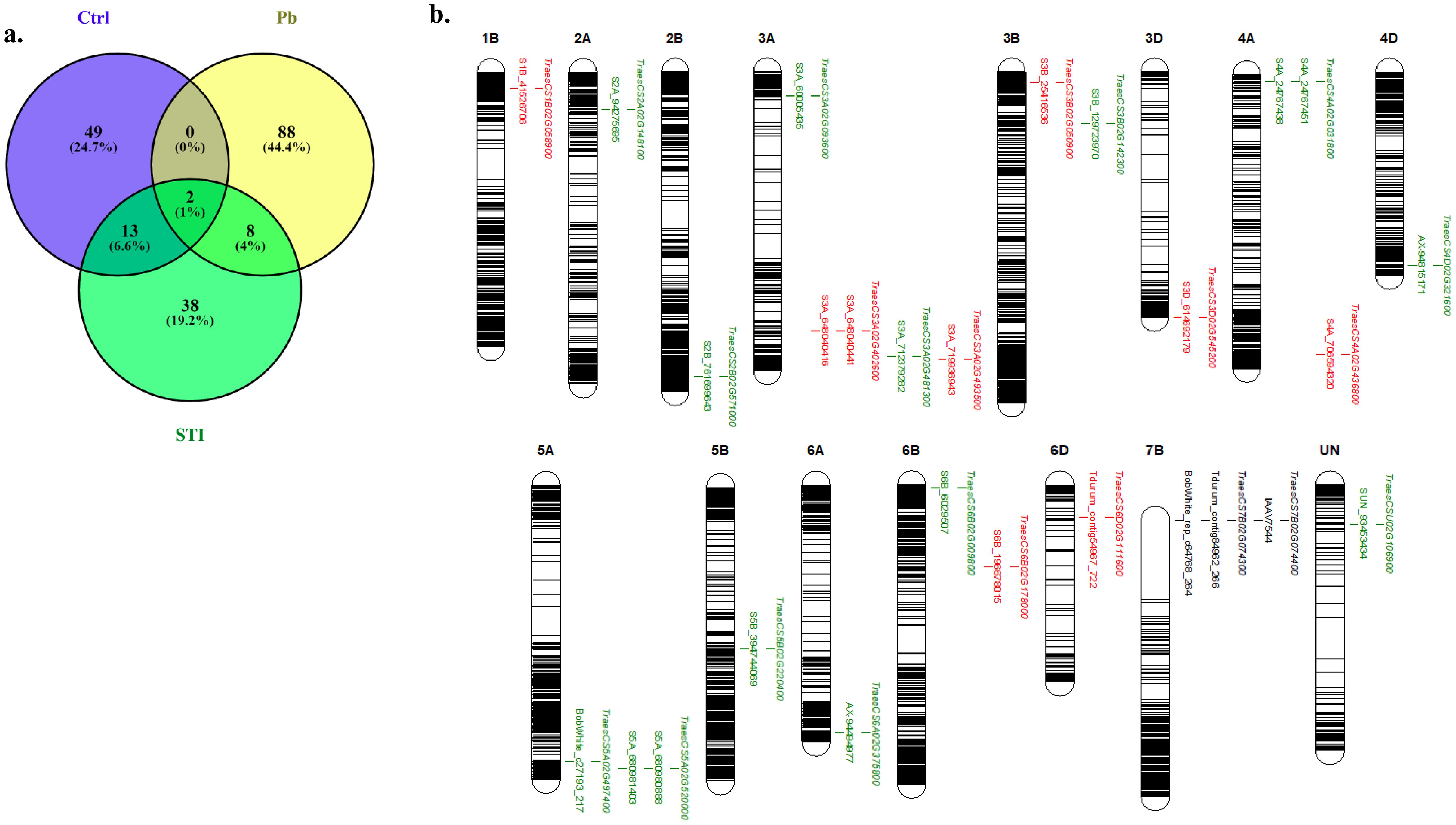
Figure 5. Gene models harboring significant markers associated with seedling traits under normal soil (Ctrl), lead contaminated soil (Pb), and stress tolerance index (STI) for lead tolerance: (A) venn diagram represents number of gene models for each treatment, (B) chromosomal location of markers and common gene models among Ctrl, PB, and STI (Black), between Pb and STI (red), and between Ctrl and STI (green).
Analysis of gene enrichment for the common genes
To provide more information about the genetic control of Pb tolerance in the seedling growth stage, gene enrichment of the 23 common gene models presented in Figure 5A was identified. On the basis of gene enrichment, a total of 16 biological process (BP), two cellular component (CC), and one molecular function (MF) pathways were found to be controlled by these 23 common genes (Figure 6A). The 16-BP pathways were found to work in three different networks (Figure 6B). The first network was found to be controlled by the TRAESCS6B02G178000 gene model, which controls the biological process of wax and fatty acid derivative metabolism in wheat. The second network controlled by TRAESCS6D02G111600 was found to control circadian rhythm regulation. The last network, network 3, was controlled by the TRAESCS3A02G402600 gene and was found to regulate the transportation of proteins within the endoplasmic reticulum.
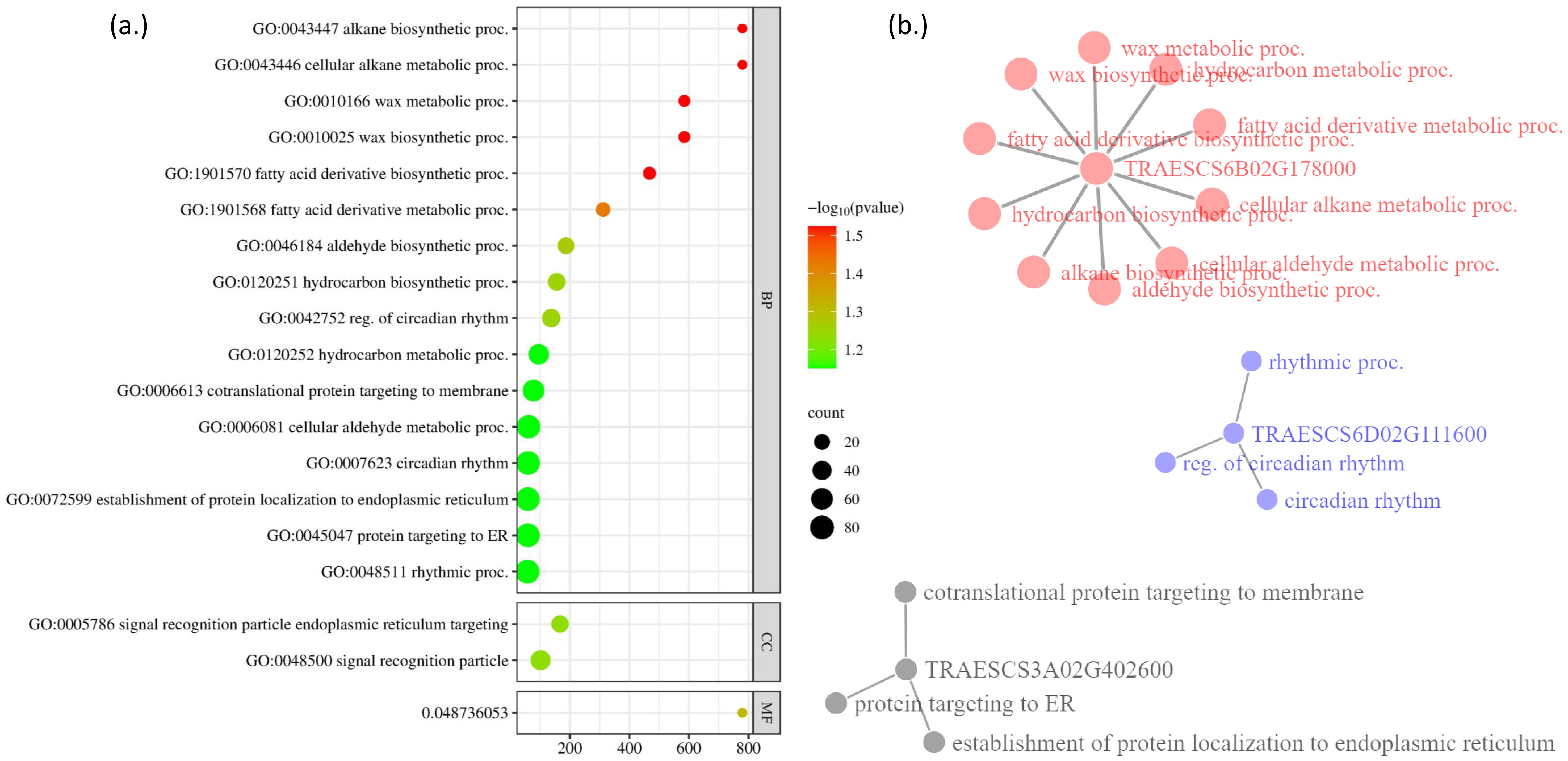
Figure 6. Gene enrichment of the common 23 gene models associated with seedling traits under normal soils and Pb-contaminated soil: (A) biological process (BP), cellular component (CC), and molecular function (MF) pathways of the identified gene, (B) network of the BP pathways controlled by the common genes.
Genomic regions controlling lead tolerance on chromosome 7B
The greatest number of significant markers was found on chromosome 7B (34 significant markers, Figure 4A). These markers were located within fourteen gene models. Therefore, focused analyses were performed to provide a better understanding of the genomic regions located on this chromosome (Figure 7).
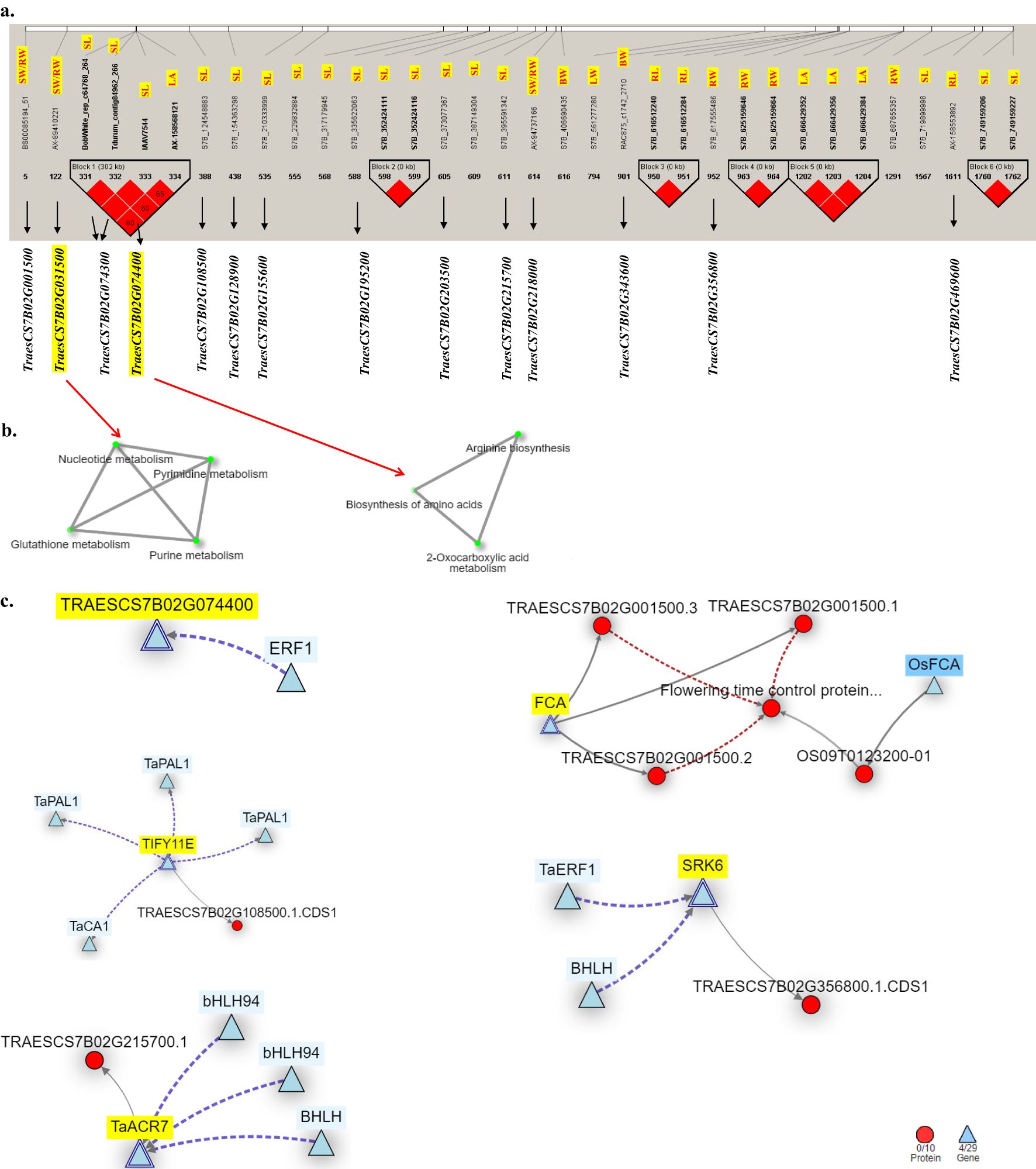
Figure 7. Genomic regions identified on 7B chromosome associated with lead tolerance in wheat seedlings: (A) haplotype blocks of the identified 34 significant markers, their associated traits (highlighted in yellow), and gene models, (B) gene enrichment analysis of gene models harboring the significant markers, and (C) gene network of the identified gene models represent their relationship with heavy metals tolerance.
On the basis of haplotype block analysis, the 34 significant markers were distributed separately along the chromosome, with the exception of 13 markers that were located within six blocks (Figure 7A). The greatest number of markers was found in Block 1, which contained four markers, followed by Block 4 (three markers), Block 2, Block 1, Block 5, and Block 6, which contained two markers in each block. Among the 14 gene models harboring significant markers on this chromosome, only two were found to be located on the same block (Block 1).
Gene enrichment analysis for all 14 gene models identified on chromosome 7B was performed. Seven important biological process pathways were identified that work together in two different networks and are controlled by two genes, TraesCS7B02G031500 and TraesCS7B02G074400 (Figure 7B; Supplementary Table S7). Network 1, which is controlled by TraesCS7B02G031500, was found to control the metabolism of pyrimidine, urine, glutathione, and nucleotides (Figure 7B). Network 2, controlled by TraesCS7B02G074400, included 2-oxocarboxylic acid metabolism, arginine biosynthesis, and amino acid biosynthesis pathways.
Furthermore, the networks representing the functions of these gene models in relation to heavy metal tolerance were investigated and are represented in Figure 7C. Among these 14 genes, five genes, ERF1, FCS, TIFY11E, TaACR7, and SRK6, were found to be correlated with known genes controlling the production of some important antioxidants in wheat under heavy metal stress. The remaining nine genes were not previously reported to be related to heavy metal tolerance in wheat.
Discussion
On the basis of the results of the soil analysis, a greater increase in soil Pb was detected in contaminated soils than in normal soils. The critical limit of Pb in soils is 30 mg/kg soil (Vries et al., 2007). In Pb-contaminated soils, the level of Pb exceeded this critical limit, as it was 65.81 mg/kg soil. Furthermore, the pH in both soils was ~7.5. It has been reported that Pb becomes available in soils and can be absorbed by plants when the pH ranges from 3–8.5 (Sharma and Dubey, 2005). It was reported that the bio-accessibility of Pb is negatively correlated with EC (Yan et al., 2019). In normal soils, the EC was lower than soil contaminated with Pb, hance Pb is more accessible to be absorbed by wheat roots in contaminated soils. Therefore, we can conclude that soil contamination was successful and that the wheat plants in the current study were exposed to high levels of Pb that are available for absorption.
Genetic variation in lead tolerance in wheat
The seedling stage in wheat is critically important, as it sets the foundation for plant growth and development throughout its life cycle. Therefore, improving seedling traits for tolerance to various biotic and abiotic stresses is an essential task. The accumulation of lead in soil can have a detrimental effect on wheat seedlings and subsequently affect all subsequent growth stages (Aslam et al., 2021). In this study, we investigated the effects of lead on shoot and root traits at the seedling stage in a set of highly diverse wheat genotypes. Pb, on average, reduced BW, RW, LW, and LA. The same effects were previously reported at a Pb concentration of 60 ppm in wheat (Shafiq et al., 2018). Most wheat studies on the effect of lead at the seedling stage reported a negative effect of Pb on SL and RL; however, in this study, Pb at a concentration of 65 mg/kg increased the SL, RL, and SL/RL ratio. The changes in SL and RL due to the effects of Pb on each genotype are presented in Supplementary Figure S6. In our study, the majority of the genotypes (75%) were taller (more SL) under Pb stress than under normal conditions (Supplementary Figure S6A). In terms of RL, 61% of the genotypes presented taller roots under Pb stress than under normal conditions (Supplementary Figure S6B). Therefore, these differences in genotype response to Pb stress are often attributed to genetic variability in Pb tolerance, detoxification mechanisms, the oxidative stress response, and gene regulation. The size of the population tested in this study was larger than the number of wheat genotypes tested in earlier studies under Pb stress (Lamhamdi et al., 2011, 2013; Kumar et al., 2018; Semenova et al., 2019; Jańczak-Pieniążek et al., 2023). Therefore, more understanding of the response of wheat seedlings to Pb could be provided in this recent study.
The analysis of variance revealed highly significant differences between the two treatments for all traits except SW, NR, and SL/RL, indicating that Pb in the soil had a significant effect on the seeding traits compared with those in normal soils. The significant differences found among genotypes in all traits were very useful in selecting promising lead-tolerant genotypes for each trait. Furthermore, highly significant differences were found among the tested genotypes in normal and Pb-contaminated soils (Supplementary Table S2), which confirmed the high genetic variation present in the tested genotypes and the possibility of selecting tolerant genotypes from them. This high genetic variation is expected due to the diversity that exists among genotypes (Mourad et al., 2020). The same set of genotypes also presented high genetic variation in important traits, such as alkaline-saline tolerance (Mourad et al., 2023c), Sn tolerance (Mourad et al., 2021b, 2023b), drought tolerance (Sallam et al., 2024b), salt tolerance (Hasseb et al., 2022), and disease resistance (Abou-Zeid and Mourad, 2021; Mourad et al., 2021a, 2022, 2023d; Esmail et al., 2023a). Furthermore, the highly significant G × T interaction for all the studied traits indicated that the response of the tested genotypes to the two treatments was different and not uniform.
The high genetic variation among genotypes allowed the selection of the most lead-tolerant genotypes. Different stress indices were used to precisely select the target genotype. The results of the selection identified eight genotypes representing different countries. These genotypes were among the 15 most lead-tolerant genotypes in terms of at least four traits. Multiple trait selection is more useful than single trait selection for improving target traits through breeding programs (Sallam and Martsch, 2015). These eight genotypes not only presented genetic variation in tolerance to lead stress but also high genetic diversity, as they are from different countries. Crossing among highly divergent genotypes is very useful for producing cultivars with desirable traits (Eltaher et al., 2018; Mourad et al., 2020, 2023a; Ghazy et al., 2021; Esmail et al., 2023a). The same genotypes were previously tested for lead tolerance at the adult growth stage by Mourad et al. (2021b), and the lead-tolerant genotypes at different growth stages should be investigated. Interestingly, none of the superior-eight genotypes were selected based on their kernel traits suggesting the presence of different response at each growth stage.
The phenotypic correlation among stress indices between the seedling and adult growth stages indicated that tolerance at the seedling stage was completely different from tolerance at the adult growth stage. Therefore, each growth stage should have a specific breeding program. The seedling stage is a very important growth stage because it affects the subsequent growth stages. The evaluation of large germplasms for Pb stress is highly recommended to identify lead-tolerant genotypes for both growth stages. These genotypes can subsequently be used to produce wheat cultivars with high tolerance to lead at all growth stages. The development of Pb-tolerant wheat varieties is very important, as they can (I) thrive in such environments without compromising yield or quality, (II) contribute to safer food production in contaminated areas, and (III) have economic benefits by reducing the need for costly soil remediation efforts in contaminated areas. They can also provide farmers with a sustainable solution for utilizing land that would otherwise be abandoned or underutilized.
Genomic regions associated with lead tolerance
The GWAS in this study was performed via two genotyping methods with completely different sequence techniques. The GWAS was run with a total set of 38,000 SNPs that were distributed across all chromosomes in the three wheat genomes. This high number of SNPs allows the identification of important markers and genes associated with Pb tolerance in wheat. The population structure and genetic diversity analyses among the 103 genotypes were extensively discussed by Mourad et al. (2020). The analysis of population structure divided the 103 genotypes into three subpopulations; therefore, PCA and kinship alone and in combination were used to correct the effect of population structure, which could lead to spurious associations in GWAS analysis. In each QQ plot for each trait, the observed p values perfectly aligned with the expected distribution along the diagonal line under the null hypothesis except for the significant SNPs, indicating the reliability of the GWAS results and the appropriateness of the selected statistical model.
The number of significant SNPs detected under Pb was greater than the number of SNPs detected under normal conditions. These results indicated that Pb had highly significant effects on the performance of the wheat genotypes tested in this study, and these effects allowed the identification of a high number of SNPs. The number of genes involved in the stress response tends to be greater than the number active under normal conditions (Moro et al., 2021). All significant SNPs associated with Pb and those associated with Pb tolerance were distributed across all chromosomes and the three genomes, indicating that Pb tolerance is a polygenic and complex trait, and more research efforts are needed to understand its genetic control. The greatest number of SNPs detected under Pb stress was located on chromosome 7B. Therefore, 7B may include important genes that play important roles in potential biological mechanisms or pathways contributing to the variation in Pb tolerance. Among the 222 significant SNPs detected under Pb stress, 142 (36%) had R2 values >10%, indicating that the majority of the detected SNPs had major effects on all traits scored under Pb stress. The application of SNPs with major effects in plant breeding facilitates the development of improved crop varieties with desirable traits (e.g., lead tolerance) through marker-assisted selection, genomic selection, quantitative trait loci mapping, gene pyramiding, and genome editing for target SNPs. These major-effect SNPs can be converted to KASP markers for use in selecting wheat genotypes with high tolerance to lead stress. Furthermore, SNPs associated with different major-effect genes can be combined through gene pyramiding to develop cultivars with multiple desirable traits, such as high yield, quality, and resistance to multiple stresses. Genome editing targeting SNPs can be used to precisely modify SNPs associated with major-effect genes, leading to the confirmation and validation of gene function under lead stress.
To the best of our knowledge, no previous GWAS reports or studies have focused on lead tolerance in wheat at the seedling stage. Therefore, the significant SNPs detected in this study under Pb stress and for STI provide important and valuable information on the genetic architecture of Pb tolerance. Mourad et al. (2023b) performed a GWAS on the same population and same markers at the adult growth stage. The authors scored kernel traits under normal and Pb stresses. A set of 279 significant markers was found to be associated with kernel traits under Pb stress. The highest number of significant SNPs detected at the adult growth stage for kernel traits were located on chromosomes 1A and 2B. These findings confirm that Pb tolerance is controlled by many genes at different growth stages.
Among the 222 significant markers, 119 were associated with gene models related to drought, heat, and salt tolerance (https://knetminer.com/cereals/). Therefore, these genes could also play important roles in lead tolerance. However, gene expression studies should be conducted to confirm the expression of these genes under Pb stress. Notably, these 119 significant markers represented 54 and 65 genes produced from GBS and 25K, respectively. This highlights the advantage of using different markers produced by different sequencing methods.
Common significant markers associated with Pb tolerance
Significant markers associated with more than one trait under each condition and both conditions were investigated and are presented in Table 6. These markers have pleiotropic effects, which are valuable for providing insights into shared genetic pathways or biological mechanisms underlying Pb tolerance. Most of the detected common markers were significantly associated with LA and SL. Only two candidate genes were annotated from the common markers. The S6B_196678015 (RL and RW) marker is located within the TraesCS6B02G178000 gene model, which encodes a protein that is among the NAD(P)-binding domain superfamily. The relationship between the NAD(P)-binding domain superfamily and lead tolerance suggests that enzymes within this superfamily could play a role in mitigating the toxic effects of lead exposure in plants (Smith and Jones, 2022). Additionally, the S1D_10740963 (LA and SL) marker was found within the TraesCS1D02G026500 gene model, which encodes the cathepsin propeptide inhibitor domain (I29). However, no direct research has reported the relationship between the cathepsin propeptide inhibitor domain superfamily and lead tolerance in plants.
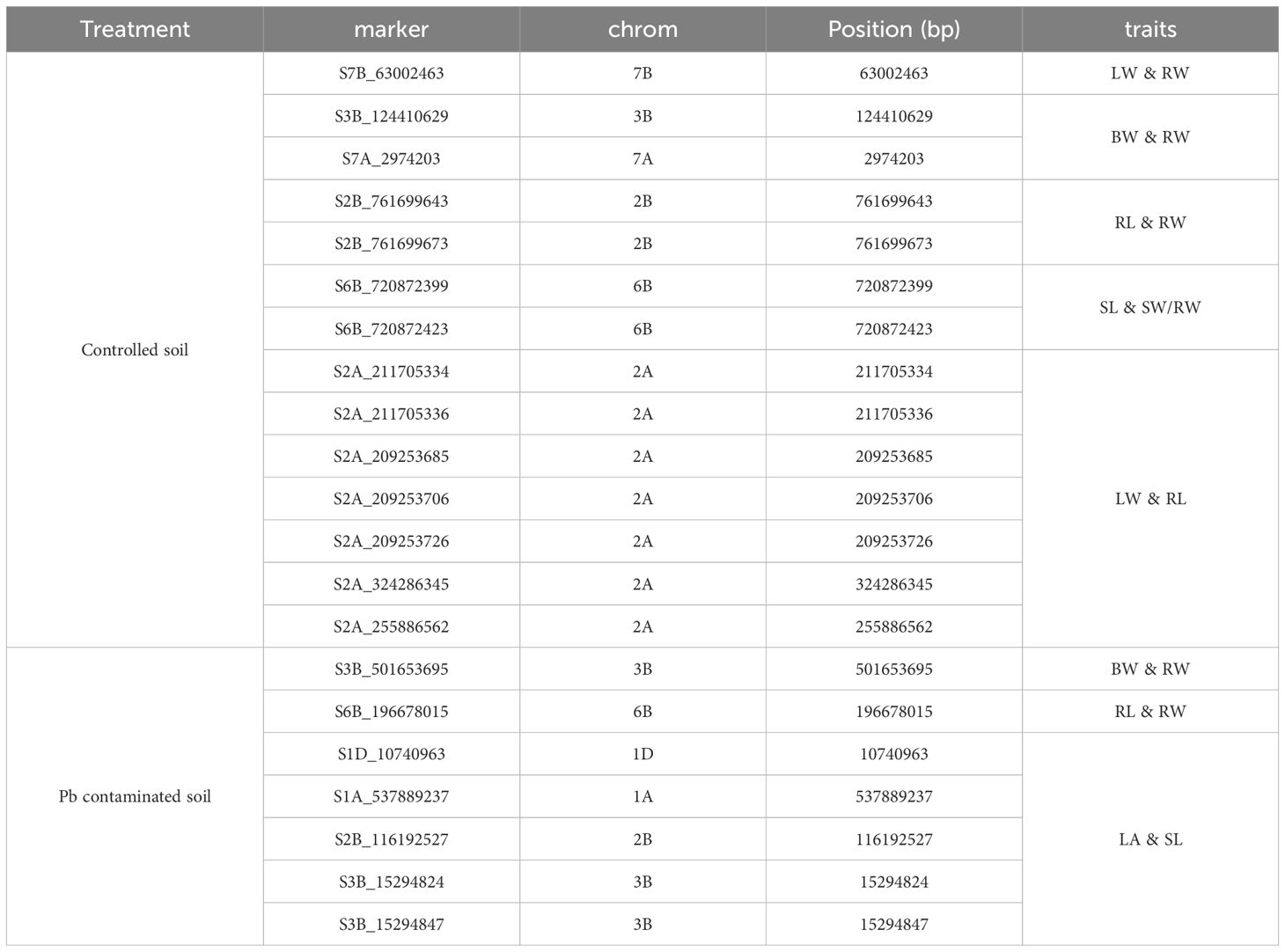
Table 6. List of SNP markers significantly associated with more than one seedling trait under the same conditions, their chromosomal position, and the trait with which they are significantly associated.
Mourad et al. (2023b) used the same population and the same types of markers to identify alleles and candidate genes associated with kernel traits under Pb stress. Identifying significant markers associated with the same trait across different growth stages can provide valuable insights into the genetic basis of trait variation over time. Therefore, it is worth investigating whether both growth stages share the same marker. Notably, four common markers were found to be associated with Pb tolerance at the seedling and adult growth stages (Table 7). The R2 of each marker varied across the growth stage. It seems that some markers showed had major effects on specific growth stages. S7A_26061799 and S7B_373077367 had major effects on Pb tolerance only at the adult growth stage, whereas the same markers had minor effects at the seedling stage and vice versa for AX-158540049. The target allele of each marker differed by growth stage. These findings suggest that the effects of the genetic variants associated with Pb tolerance may vary across different developmental stages and might support the notion that the genetic control of Pb tolerance might differ across growth stages. The negative phenotypic correlation found in the stress tolerance indices between the seedling and adult growth stages may also confirm the lack of relationship in the genetic control between the two growth stages. Moreover, these five shared markers are located in genomic regions and may have stage-specific genetic effects (temporal specificity) on trait expression (Koltunow et al., 1990). The lack of relationship between the genetic control of abiotic stress tolerance at the seedling and adult growth stages has been reported previously in wheat (Mourad et al., 2023c). Among the five shared markers, two were found within two gene models. AX-158540049 was found within TraesCS1A02G017000, which encodes an RNA recognition motif domain. In plants, RRM-containing proteins are involved in regulating gene expression, responding to environmental stresses, and modulating developmental processes (Nakaminami et al., 2012). RRM-containing proteins may indirectly contribute to Pb tolerance by regulating the expression of genes involved in stress responses, detoxification mechanisms, or metal ion homeostasis. The other marker (S7B_373077367) was found in the TraesCS7B02G203500 gene model, which encodes an Armadillo-like helical. There was no direct relationship between the Armadillo-like helicity and Pb tolerance or abiotic stress. Further investigations are needed to investigate the expression of these genes under Pb stress.
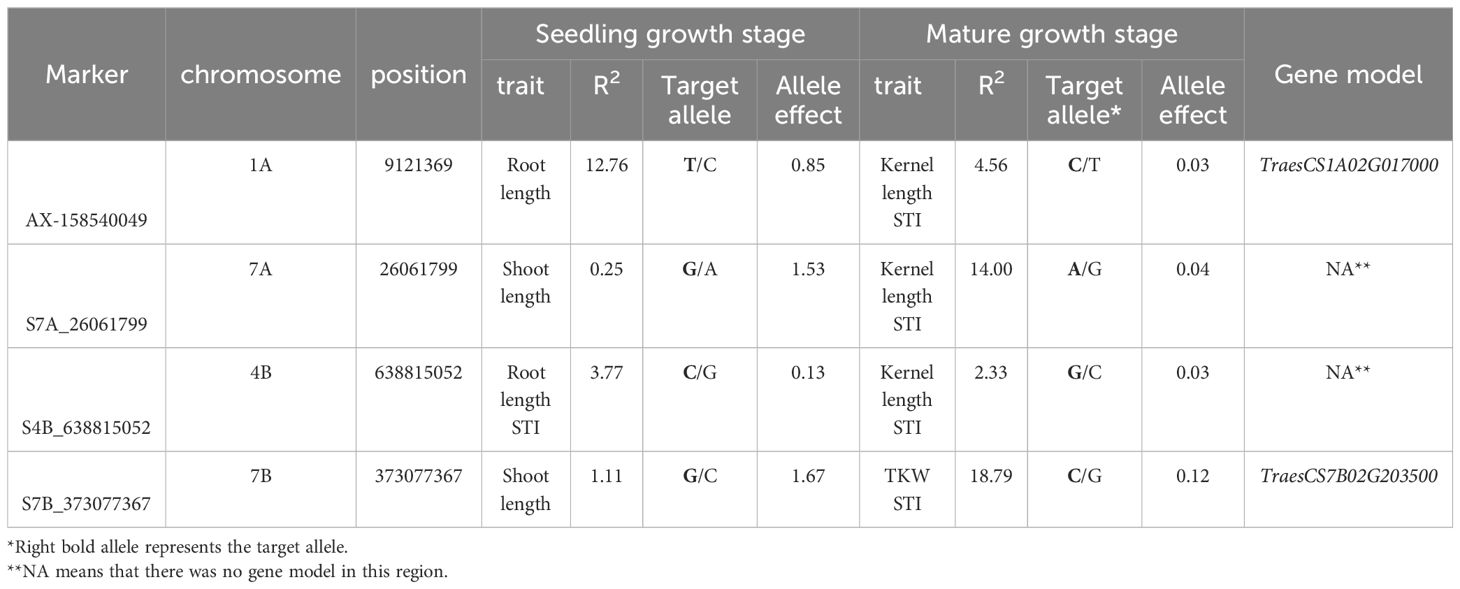
Table 7. List of markers and gene models significantly associated with lead tolerance at the seedling and mature growth stages.
Gene enrichment analysis of genes common to both normal and Pb-stress conditions
Sixteen significant biological process pathways were found to be controlled by only three gene models. Interestingly, all three gene models were found to control seedling traits in Pb-contaminated soils as well as the STI. Three networks that were controlled by one gene of these three gene models were detected. All these networks were found to be directly and indirectly associated with Pb tolerance as follows: Network 1 controlled alkane biosynthesis, alkane metabolism, wax metabolism, and aldehyde metabolism. Alkane and wax metabolism are essential for improving the response of wheat to abiotic stresses (Li et al., 2019). Plants with metal tolerance have several specific features, including waxy leaves (Pereira et al., 2016; Muszyńska et al., 2019; Sitko et al., 2022). Interestingly, the same network and its controlling gene model (TraesCS6B02G178000) were associated with several kernel traits, such as KW, TKW, KD_STI, and TKW_STI, under heavy metal conditions (Mourad et al., 2023b). However, the effect of significant markers located within this gene was minorly associated with the mentioned kernel traits. However, in the present study, TraesCS6B02G178000 and its marker were found to have a major effect on RL and a minor effect on RW (Supplementary Table S4). Therefore, we can conclude that the TraesCS6B02G178000 gene is an important gene that improves wheat tolerance to heavy metal stress at both the seedling and mature growth stages. Network 2, which is controlled by TraesCS6D02G111600, was found to control rhythmic processes, which regulate the sleep−wake cycle and various physiological functions in wheat. It has been reported to play an important role in regulating the expression of genes controlling several important biological pathways, such as the metal detoxification and stress response pathways (Grundy et al., 2015). Therefore, this network and its gene model are very important for determining the tolerance of wheat to heavy metal stress. Network 3 (controlled by the TraesCS3A02G402600 gene) was found to control protein localization in the endoplasmic reticulum (ER). Recent studies have reported the abundance of genes controlling the ER in wheat roots and leaves under nickel stress (Zhu et al., 2024). These genes were found to improve wheat tolerance to heavy metals by regulating ER stress homeostasis and the ROS balance. Moreover, in our current study, the markers S3A_648040416 and S3A_648040441, located within the TraesCS3A02G402600 gene, strongly affected RW (R2 = 45.29%) under Pb conditions and the STI (R2 = 33.00%). Therefore, these markers and their genes could be used in MAS for Pb tolerance in wheat. Moreover, including them in future breeding programs will accelerate wheat breeding for heavy metals. However, these markers should be validated in different genetic backgrounds before they are used in marker-assisted selection. Some candidate genes found in this study were associated with heavy metal tolerance, which confirmed the success of the GWAS conducted in this study, whereas the other candidate genes could be considered novel genes.
Insights into genomic regions on chromosome 7B
The majority of the identified significant markers are located on chromosome 7B. Despite the high number of significant markers identified on this chromosome, only six blocks were identified to contain some of the significant markers. The absence of significant linkage disequilibrium (LD) between most of these significant markers confirmed the presence of many genomic regions controlling tolerance on this chromosome. This is notable from the distribution of the markers on the short and long arms of the chromosome (Figure 7A). Furthermore, the presence of different gene models (14 genes) harboring these SNPs confirms our conclusion. It is known that significant markers located on the same block are most likely controlling the same QTL. All the identified blocks contained markers associated with the same trait, except block 1, which contained three markers associated with SL and one marker associated with LA. However, positive significant correlations were found between LA and SL under normal and Pb conditions, which suggested the presence of a common genetic system controlling both traits. Furthermore, previous studies reported that the use of blocks containing many significant markers in marker-assisted selection (MAS) is better than the use of single markers (Mokry et al., 2014; Mourad et al., 2018, 2023d). Therefore, the identified blocks on this chromosome could be used in MAS for lead tolerance in wheat seedlings.
To elucidate the genomic regions controlling Pb tolerance on the 7B chromosome, gene enrichment analysis of the 14-gene models identified on this chromosome was performed. This analysis reduced the number of genes to only two genes on the basis of an FDR of 1%. The seven biological process pathways controlled by these two genes are very important for heavy metal tolerance (Supplementary Table S7). For example, the arginine biosynthesis pathway has been reported as one of the pathways related to antioxidant defense against heavy metals in wheat (Zhou and Zheng, 2022). Furthermore, the 2-oxocarboxylic acid metabolism pathway has been reported as one of the important pathways controlling cotton tolerance to cadmium stress (Li et al., 2022). Moreover, the network of all 14 gene models related to heavy metal tolerance was investigated. Among the 14 gene models, five were related to known genes controlling heavy metal tolerance. For example, the ERF1 gene (related to the TRAESCS7B02G074400 gene model) was reported to be highly expressed in wheat seedlings tolerant to chromium (Ergün et al., 2014). The TaPAL1 gene (related to the TRAESCS7B02G108500 gene model) was reported to play an important role in the synthesis of endogenous salicylic acid, which is essential for cadmium tolerance in wheat (Wei et al., 2022). The protein family bHLH (related to TraesCS7B02G215700 and TraesCS7B02G356800 gene models) was reported as an important protein family in controlling the accumulation of lead and cadmium in wheat roots (Aprile et al., 2018, 2019; Sabella et al., 2021).
More studies are needed to elucidate the genetic role of these putative genomic regions in controlling lead tolerance in wheat. However, our preliminary study shed light on the importance of the 7B chromosome in providing a high level of Pb tolerance in wheat.
In conclusion, valuable and novel information on genetic variation in lead tolerance and candidate genes associated with lead tolerance was reported in this study for the first time at the seedling stage. The genotypes evaluated in this study presented different responses to lead tolerance in wheat, which resulted in the identification of the most promising lead-tolerant genotypes. None of the selected genotypes based on the studied traits at seedling growth stage were superior based on kernel traits suggesting the presence of different response at each growth stage. The GWAS revealed very important SNP markers and candidate genes associated with lead tolerance. Most of the markers had major effects on lead tolerance. The results of the gene enrichment analysis support the success of the GWAS conducted in this study, as most of the detected genes were previously reported to be associated with heavy metal tolerance in wheat. Lead tolerance at the seedling stage and adult growth stage is controlled by different genetic mechanisms. Extensive physiological analysis is needed to expand our knowledge of lead tolerance in wheat and interpret the different responses of the genotypes, especially for RL and SL.
Data availability statement
The original contributions presented in the study are included in the article/Supplementary Material, further inquiries can be directed to the corresponding author/s.
Author contributions
AM: Conceptualization, Data curation, Formal Analysis, Funding acquisition, Investigation, Methodology, Software, Validation, Writing – original draft, Writing – review & editing. AS: Writing – original draft, Writing – review & editing. KF: Writing – original draft, Methodology. AB: Writing – review & editing.
Funding
The author(s) declare that financial support was received for the research, authorship, and/or publication of this article. This work was financially supported by the Alexander von Humboldt Foundation, Germany. The costs for open access publishing were partially funded by the Deutsche Forschungsgemeinschaft (DFG, German Research Foundation, grant 491250510).
Conflict of interest
The authors declare that the research was conducted in the absence of any commercial or financial relationships that could be construed as a potential conflict of interest.
The author(s) declared that they were an editorial board member of Frontiers, at the time of submission. This had no impact on the peer review process and the final decision.
Publisher’s note
All claims expressed in this article are solely those of the authors and do not necessarily represent those of their affiliated organizations, or those of the publisher, the editors and the reviewers. Any product that may be evaluated in this article, or claim that may be made by its manufacturer, is not guaranteed or endorsed by the publisher.
Supplementary material
The Supplementary Material for this article can be found online at: https://www.frontiersin.org/articles/10.3389/fagro.2025.1428366/full#supplementary-material
References
Abou-Zeid M. A., Mourad A. M. I. (2021). Genomic regions associated with stripe rust resistance against the Egyptian race revealed by genome-wide association study. BMC Plant Biol. 21, 1–14. doi: 10.1186/s12870-020-02813-6
Ahmed A. A. M., Mohamed E. A., Hussein M. Y., Sallam A. (2021). Genomic regions associated with leaf wilting traits under drought stress in spring wheat at the seedling stage revealed by GWAS. Environ. Exp. Bot. 184, 104393. doi: 10.1016/j.envexpbot.2021.104393
Allaire J. J., Gandrud C., Russell K., Yetman C. (2017). networkD3: D3 JavaScript Network Graphs from R. R Packag., version 0.4. Available online at: https://cran.r-project.org/package=networkD3.
Alloway B. J. (2013). “Sources of Heavy Metals and Metalloids in Soils,” in Heavy metals in soils: trace metals and metalloids in soils and their bioavailability. Ed. Alloway B. J. (Springer, UK), 11–50. doi: 10.1002/9781119438922.ch18
Alqudah A. M., Sallam A., Baenziger P. S., Börner A. (2020). GWAS : Fast-forwarding gene identification and characterization in temperate Cereals : lessons from Barley – A review. J. Adv. Res. 22, 119–135. doi: 10.1016/j.jare.2019.10.013
Alqudaha A. M., Sallamb A., Baenzigerc P. S., Börnera A. (2019). GWAS: fast-forwarding gene identification in temperate cereals: barley as a case study-A review. J. Adv. Res. Nov 4, 119–135. doi: 10.1016/j.jare.2019.10.013
Amro A., Harb S., Youssef K., Ali M. M. F., Mohammed A. G., Mourad A. M. I., et al. (2022). Growth responses and genetic variation among highly ecologically diverse spring wheat genotypes grown under seawater stress. Front. Plant Sci. 13. doi: 10.3389/fpls.2022.996538
Aprile A., Sabella E., Francia E., Milc J., Ronga D., Pecchioni N., et al. (2019). Combined effect of cadmium and lead on durum wheat. Int. J. Mol. Sci. 20, 1–17. doi: 10.3390/ijms20235891
Aprile A., Sabella E., Vergine M., Genga A., Siciliano M., Nutricati E., et al. (2018). Activation of a gene network in durum wheat roots exposed to cadmium. BMC Plant Biol. 18, 1–16. doi: 10.1186/s12870-018-1473-4
Aslam M., Aslam A., Sheraz M., Ali B., Ulhassan Z., Najeeb U., et al. (2021). Lead toxicity in cereals: mechanistic insight into toxicity, mode of action, and management. Front. Plant Sci. 11. doi: 10.3389/fpls.2020.587785
Bálint A. F., Röder M. S., Hell R., Galiba G., Börner A. (2007). Mapping of QTLs affecting copper tolerance and the Cu, Fe, Mn and Zn contents in the shoots of wheat seedlings. Biol. Plant 51, 129–134. doi: 10.1007/s10535-007-0025-9
Bolser D., Staines D. M., Kersey E., Kersey P. (2007). Ensembl Plants: Integrating tools for visualizing, mining, and analyzing plant genomics data. Plant Bioinform.: Methods Protoc., 115–140.
Bradbury P. J., Zhang Z., Kroon D. E., Casstevens T. M., Ramdoss Y., Buckler E. S. (2007). TASSEL: Software for association mapping of complex traits in diverse samples. Bioinformatics 23, 2633–2635. doi: 10.1093/bioinformatics/btm308
Elshire R. J., Glaubitz J. C., Sun Q., Poland J. A., Kawamoto K., Buckler E. S., et al. (2011). A robust, simple genotyping-by-sequencing (GBS) approach for high diversity species. PloS One 6, e19379. doi: 10.1371/journal.pone.0019379
Eltaher S., Sallam A., Belamkar V., Emara H. A., Nower A. A., Salem K. F. M., et al. (2018). Genetic diversity and population structure of F3:6 Nebraska Winter wheat genotypes using genotyping-by-sequencing. Front. Genet. 9. doi: 10.3389/fgene.2018.00076
Ergün N., Özçubukçu S., Kolukirik M., Temizkan (2014). Effects of temperature - Heavy metal interactions, antioxidant enzyme activity and gene expression in wheat (Triticum aestivum L.) seedlings. Acta Biol. Hung. 65, 439–450. doi: 10.1556/ABiol.65.2014.4.8
Esmail S. M., Omar G. E., El-Orabey W. M., Börner A., Mourad A. M. I. (2023a). Exploring the genetic variation of stripe rust foliar and head infection in Egyptian wheat as an effect of climate change. Agronomy 13, 1509. doi: 10.3390/agronomy13061509
Esmail S. M., Omar G. E., Mourad A. (2023b). In-depth understanding of the genetic control of stripe rust resistance (Puccinia striiformis f. sp. tritici) induced in wheat (Triticum aestivum L.) by Trichoderma asperellum T34. Plant Dis. 107, 457–472. doi: 10.1094/PDIS-07-22-1593-RE
Fernandez G. C. J. (1992). Effective selection criteria for assessing PLant stress toerance. Adapt. Food Crop to Temp. Water Stress., 257–270.
Flora G., Gupta D., Tiwari A. (2012). Toxicity of lead: A review with recent updates. Interdiscip. Toxicol. 5, 47–58. doi: 10.2478/v10102-012-0009-2
Ge S. X., Jung D., Yao R. (2022). ShinyGO: a graphical enrichment tool for ani-mals and plants. Bioinformatics 36, 2628–2629. doi: 10.1093/bioinformatics/btz931
Ghazy M. I., Salem K. F. M., Sallam A. (2021). Utilization of genetic diversity and marker-trait to improve drought tolerance in rice (Oryza sativa L.). Mol. Biol. Rep. 48, 157–170. doi: 10.1007/s11033-020-06029-7
Grundy J., Stoker C., Carré I. A. (2015). Circadian regulation of abiotic stress tolerance in plants. Front. Plant Sci. 6. doi: 10.3389/fpls.2015.00648
Gupta D. K., Huang H. G., Corpas F. J. (2013). Lead tolerance in plants: Strategies for phytoremediation. Environ. Sci. pollut. Res. 20, 2150–2161. doi: 10.1007/s11356-013-1485-4
Hassani-Pak K., Singh A., Brandizi M., Hearnshaw J., Parsons J. D., Amberkar S., et al. (2021). KnetMiner: a comprehensive approach for supporting evidence-based gene discovery and complex trait analysis across species. Plant Biotechnol. J. 19, 1670–1678. doi: 10.1111/pbi.13583
Hasseb N. M., Sallam A., Karam M. A., Gao L., Wang R. R. C., Moursi Y. S. (2022). High-LD SNP markers exhibiting pleiotropic effects on salt tolerance at germination and seedlings stages in spring wheat. Plant Mol. Biol. 108, 585–603. doi: 10.1007/s11103-022-01248-x
Jańczak-Pieniążek M., Cichoński J., Michalik P., Chrzanowski G. (2023). Effect of heavy metal stress on phenolic compounds accumulation in winter wheat plants. Molecules 28, 241. doi: 10.3390/molecules28010241
Koltunow A. M., Truettner J., Cox K. H., Wallroth M., Goldberg R. B. (1990). Different temporal and spatial gene expression patterns occur during anther development. Plant Cell 2, 1201–1224. doi: 10.2307/3869340
Kumar S., Sharma P., Misra M., Misra A. N. (2018). Lead induced root and shoot growth reduction in wheat (Triticum aestivum L.) is due to increase in membrane lipid peroxidation. J. Pharmacogn. Phytochem. 1, 2080–2083.
Lamhamdi M., Bakrim A., Aarab A., Lafont R., Sayah F. (2011). Lead phytotoxicity on wheat (Triticum aestivum L.) seed germination and seedlings growth. Comptes Rendus - Biol. 334, 118–126. doi: 10.1016/j.crvi.2010.12.006
Lamhamdi M., El Galiou O., Bakrim A., Nóvoa-Muñoz J. C., Arias-Estévez M., Aarab A., et al. (2013). Effect of lead stress on mineral content and growth of wheat (Triticum aestivum) and spinach (Spinacia oleracea) seedlings. Saudi J. Biol. Sci. 20, 29–36. doi: 10.1016/j.sjbs.2012.09.001
Li T., Sun Y., Liu T., Wu H., An P., Shui Z., et al. (2019). TaCER1-1A is involved in cuticular wax alkane biosynthesis in hexaploid wheat and responds to plant abiotic stresses. Plant Cell Environ. 42, 3077–3091. doi: 10.1111/pce.13614
Li L., Yan X., Li J., Wu X., Wang X. (2022). Metabolome and transcriptome association analysis revealed key factors involved in melatonin mediated cadmium-stress tolerance in cotton. Front. Plant Sci. 13. doi: 10.3389/fpls.2022.995205
Ma C., Lin L., Yang J., Liu F., Berrettoni M., Zhang K., et al. (2023). Mechanisms of lead uptake and accumulation in wheat grains based on atmospheric deposition-soil sources. Sci. Total Environ. 885, 163845. doi: 10.1016/j.scitotenv.2023.163845
McBride M. B. (2003). Toxic metals in sewage sludge-amended soils: Has promotion of beneficial use discounted the risks? Adv. Environ. Res. 8, 5–19. doi: 10.1016/S1093-0191(02)00141-7
Mokry F. B., Buzanskas M. E., Mudadu M. D. A., Grossi A., Higa R. H., Ventura R. V., et al. (2014). Linkage disequilibrium and haplotype block structure in a composite beef cattle breed. BMC Genomics 15, 1–9. Available at: http://www.biomedcentral.com/1471-2164/15/S7/S6.
Mondal S., Sallam A., Sehgal D., Sukumaran, Sivakumar Farhad M., Krishnan J. N., et al. (2021). Advances in breeding for abiotic stress tolerance in wheat. Genom. Design. Abiot. Stress Resist. Cereal Crops, 71–103. doi: 10.1007/978-3-030-75875-2
Morias S., Costa F., Pereira M. (2012). “Soil, heavy metals, and human health,” in Environmental health– Emerging Issues and Practice, Taylor & Francis. vol. 59–82. . doi: 10.1201/b13683
Moro S. G., Hermans C., Ruiz-Orera J., Albà M. M. (2021). Impact of uORFs in mediating regulation of translation in stress conditions. BMC Mol. Cell Biol. 22, 1–13. doi: 10.1186/s12860-021-00363-9
Mourad A. M. I., Abou-Zeid M. A., Eltaher S., Baenziger P. S., Börner A. (2021a). Identification of candidate genes and genomic regions associated with adult plant resistance to stripe rust in spring wheat. Agronomy 11, 2585–2603. doi: 10.3390/agronomy11122585
Mourad A. M. I., Alomari D. Z., Alqudah A. M., Sallam A., Salem K. F. M. (2019). Recent Advances in wheat (Triticum spp.) breeding. Adv. planr Breed. strat.: cereals 559–593. doi: 10.1007/978-3-030-23108-8_15
Mourad A. M. I., Amin A. E. E. A. Z., Dawood M. F. A. (2021b). Genetic variation in kernel traits under lead and tin stresses in spring wheat diverse collection. Environ. Exp. Bot. 192, 104646. doi: 10.1016/j.envexpbot.2021.104646
Mourad A. M. I., Baghdady S., Abdel-aleem F. A. M., Jazeri R. M., Börner A. (2024). Novel genomic regions and gene models controlling copper and cadmium stress tolerance in wheat seedlings. Agronomy 14, 2876. doi: 10.3390/agronomy14122876
Mourad A. M. I., Belamkar V., Baenziger P. S. (2020). Molecular genetic analysis of spring wheat core collection using genetic diversity, population structure, and linkage disequilibrium. BMC Genomics 21, 1–12. doi: 10.1186/s12864-020-06835-0
Mourad A. M. I., Börner A., Esmail S. M. (2023a). Alleviation of stripe rust disease in wheat seedlings using three different species of trichoderma spp. Agriculture 13, 1755. doi: 10.3390/agriculture13091755
Mourad A. M. I., Draz I. S., Omar G. E., Börner A., Esmail S. M. (2022). Genome-Wide Screening of Broad-Spectrum Resistance to Leaf Rust (Puccinia triticina Eriks ) in Spring Wheat ( Triticum aestivum L.). Front. Plant Sci. 13. doi: 10.3389/fpls.2022.921230
Mourad A. M. I., Eltaher S., Börner A., Sallam A. (2023b). Unlocking the genetic control of spring wheat kernel traits under normal and heavy metals stress conditions. Plant Soil 484, 257–278. doi: 10.1007/s11104-022-05790-x
Mourad A. M. I., Farghly K. A., Börner A., Moursi Y. S. (2023c). Candidate genes controlling alkaline-saline tolerance in two different growing stages of wheat life cycle. Plant Soil. doi: 10.1007/s11104-023-06232-y
Mourad A. M. I., Hamdy R. M., Esmail S. M. (2023d). Novel genomic regions on chromosome 5B controlling wheat powdery mildew seedling resistance under Egyptian conditions. Front. Plant Sci. 14. doi: 10.3389/fpls.2023.1160657
Mourad A. M. I., Sallam A., Belamkar V., Wegulo S., Bowden R., Jin Y., et al. (2018). Genome-wide association study for identification and validation of novel SNP markers for Sr6 stem rust resistance gene in bread wheat. Front. Plant Sci. 9. doi: 10.3389/fpls.2018.00380
Muszyńska E., Labudda M., Różańska E., Hanus-Fajerska E., Koszelnik-Leszek A. (2019). Structural, physiological and genetic diversification of Silene vulgaris ecotypes from heavy metal-contaminated areas and their synchronous in vitro cultivation. Planta 249, 1761–1778. doi: 10.1007/s00425-019-03123-4
Nakaminami K., Matsui A., Shinozaki K., Seki M. (2012). RNA regulation in plant abiotic stress responses. Biochim. Biophys. Acta - Gene Regul. Mech. 1819, 149–153. doi: 10.1016/j.bbagrm.2011.07.015
Nessem A. A., Mohsen A. A., Atlam A. M., Ragab G. A. (2022). Retrieving the growth and mineral nutrition of wheat plants subjected to lead or nickel stress by priming in Sonchus oleraceus extract. Delta J. Sci. 44, 57–73. doi: 10.21608/djs.2022.142278.1025
Pereira M. P., Rodrigues L.C. de A., Corrêa F. F., de Castro E. M., Ribeiro V. E., Pereira F. J. (2016). Cadmium tolerance in Schinus molle trees is modulated by enhanced leaf anatomy and photosynthesis. Trees - Struct. Funct. 30, 807–814. doi: 10.1007/s00468-015-1322-0
Pour-Aboughadareh A., Yousefian M., Moradkhani H., Moghaddam Vahed M., Poczai P., Siddique K. H. M. (2019). iPASTIC: An online toolkit to estimate plant abiotic stress indices. Appl. Plant Sci. 7, 1–6. doi: 10.1002/aps3.11278
Rajput V. D., Harish, Singh R. K., Verma K. K., Sharma L., Quiroz-Figueroa F. R., et al. (2021). Recent developments in enzymatic antioxidant defence mechanism in plants with special reference to abiotic stress. Biol. (Basel). 10, 1–28. doi: 10.3390/biology10040267
Saatloo N. V., Ebrahiminejad B., Sadighara P., Manafi L., Yazdanfar N., Fallahizadeh S. (2023). Quantification and human health risk assessment of cadmium and lead in wheat flour on the Iranian market by atomic absorption spectrometry. Case Stud. Chem. Environ. Eng. 8, 100438. doi: 10.1016/j.cscee.2023.100438
Sabella E., Luvisi A., Genga A., De Bellis L., Aprile A. (2021). Molecular responses to cadmium exposure in two contrasting durum wheat genotypes. Int. J. Mol. Sci. 22, 1–19. doi: 10.3390/ijms22147343
Sallam A., Awadalla R. A., Elshamy M. M., Börner A., Heikal Y. M. (2024a). Genome-wide analysis for root and leaf architecture traits associated with drought tolerance at the seedling stage in a highly ecologically diverse wheat population. Comput. Struct. Biotechnol. J. 23, 870–882. doi: 10.1016/j.csbj.2024.01.020
Sallam A., Dawood M. F. A., Jarquín D., Mohamed E. A., Hussein M. Y., Börner A., et al. (2024b). Genome-wide scanning to identify and validate single nucleotide polymorphism markers associated with drought tolerance in spring wheat seedlings. Plant Genome 1–26, e20444. doi: 10.1002/tpg2.20444
Sallam A., Martsch R. (2015). Association mapping for frost tolerance using multi-parent advanced generation inter-cross (MAGIC) population in faba bean (Vicia faba L.). Genetica 143, 501–514. doi: 10.1007/s10709-015-9848-z
Sallam A., Mourad A. M. I., Hussain W., Stephen Baenziger P. (2018). Genetic variation in drought tolerance at seedling stage and grain yield in low rainfall environments in wheat (Triticum aestivum L.). Euphytica 214, 169. doi: 10.1007/s10681-018-2245-9
Schneider C. A., Rasband W. S., Eliceiri K. W. (2012). NIH Image to ImageJ: 25 years of image analysis. Nat. Methods 9, 671–675. doi: 10.1038/nmeth.2089
Semenova G. A., Fomina I. R., Bakaeva E. A., Balakhnina T. I. (2019). The effects of lead on the meristem of wheat seedlings. CellBio 08, 41–51. doi: 10.4236/cellbio.2019.83003
Shafiq M., Mehboob S., Iqbal M. Z., Abir M. (2018). The influence of lead on seedling growth of wheat (Triticum aestivum L.). Civ. Environ. Res. 10, 42–48.
Sharma P., Dubey R. S. (2005). Lead toxicity in plants. Braz.J.Plant Physiol. 17, 35–52. doi: 10.1590/S1677-04202005000100004
Singh S., Parihar P., Singh R., Singh V. P., Prasad S. M. (2016). Heavy metal tolerance in plants: Role of transcriptomics, proteomics, metabolomics, and ionomics. Front. Plant Sci. 6. doi: 10.3389/fpls.2015.01143
Sitko K., Opała-Owczarek M., Jemioła G., Gieroń Ż., Szopiński M., Owczarek P., et al. (2022). Effect of drought and heavy metal contamination on growth and photosynthesis of silver birch trees growing on post-industrial heaps. Cells 11, 59. doi: 10.3390/cells11010053
Smith A. B., Jones C. D. (2022). The role of the NAD(P)-binding domain superfamily in lead tolerance in plants. Plant Physiol. 162, 567–580. doi: 10.1016/j.plaphy.2022.123456
Souahi H., Chebout A., Akrout K., Massaoud N., Gacem R. (2021). Physiological responses to lead exposure in wheat, barley and oat. Environ. Challenges 4, 100079. doi: 10.1016/j.envc.2021.100079
Vodyanitskii Y. N. (2016). Standards for the contents of heavy metals in soils of some states. Ann. Agrar. Sci. 14, 257–263. doi: 10.1016/J.AASCI.2016.08.011
Vries W., Römkens P. F. A. M., Schütze G. (2007). Critical soil concentrations of cadmium, lead, and mercury in view of health effects on humans and animals. Rev. Env. Contam. Toxicol. 191, 91–130. doi: 10.1007/978-0-387-69163-3_4
Wang J., Song L., Gong X., Xu J., Li M. (2020). Functions of jasmonic acid in plant regulation and response to abiotic stress. Int. J. Mol. Sci. 21, 1–17. doi: 10.3390/ijms21041446
Wei J., Liao S., Li M., Zhu B., Wang H., Gu L., et al. (2022). AetSRG1 contributes to the inhibition of wheat Cd accumulation by stabilizing phenylalanine ammonia lyase. J. Hazard. Mater. 428, 128226. doi: 10.1016/j.jhazmat.2022.128226
Yan K., Dong Z., Wijayawardena M. A. A., Liu Y., Li Y., Naidu R. (2019). The source of lead determines the relationship between soil properties and lead bioaccessibility. Environ. pollut. 246, 53–59. doi: 10.1016/j.envpol.2018.11.104
Yin L., Zhang H., Tang Z., Xu J., Yin D., Zhang Z., et al. (2021). rMVP: A memory-efficient, visualization-enhanced, and parallel-accelerated tool for genome-wide association study. Genomics Proteomics Bioinf. 19, 619–628. doi: 10.1016/J.GPB.2020.10.007
Zhou M., Zheng S. (2022). Multi-Omics Uncover the mechanism of wheat under heavy metal stress. Int. J. Mol. Sci. 23, 15968. doi: 10.3390/ijms232415968
Keywords: heavy metals, seedling tolerance, genome-wide association study, gene enrichment, functional annotation, gene network
Citation: Mourad AMI, Sallam A, Farghaly KA and Börner A (2025) Detailed genetic analyses highlight genetic variation and genomic regions for lead tolerance in spring wheat. Front. Agron. 7:1428366. doi: 10.3389/fagro.2025.1428366
Received: 06 May 2024; Accepted: 14 January 2025;
Published: 06 February 2025.
Edited by:
Mohammad Bagher Hassanpouraghdam, University of Maragheh, IranCopyright © 2025 Mourad, Sallam, Farghaly and Börner. This is an open-access article distributed under the terms of the Creative Commons Attribution License (CC BY). The use, distribution or reproduction in other forums is permitted, provided the original author(s) and the copyright owner(s) are credited and that the original publication in this journal is cited, in accordance with accepted academic practice. No use, distribution or reproduction is permitted which does not comply with these terms.
*Correspondence: Amira M. I. Mourad, bW91cmFkQGlway1nYXRlcnNsZWJlbi5kZQ==; YW1pcmFfbW91cmFkQGF1bi5lZHUuZWc=