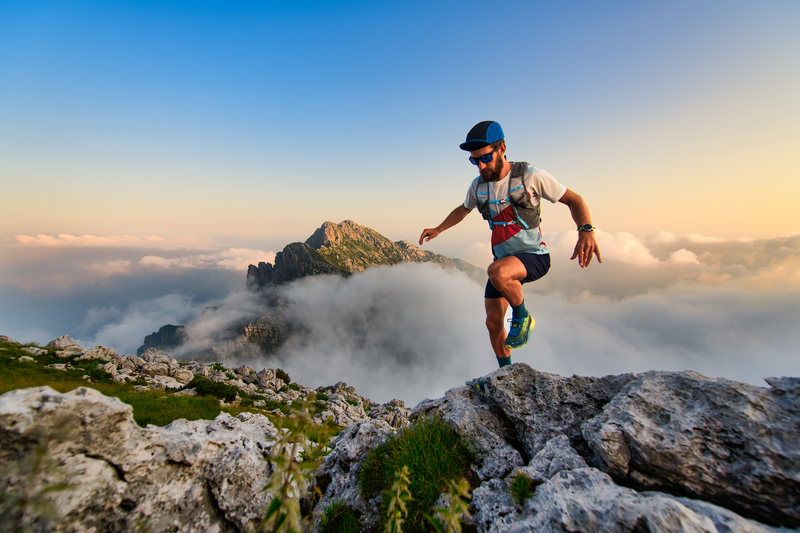
95% of researchers rate our articles as excellent or good
Learn more about the work of our research integrity team to safeguard the quality of each article we publish.
Find out more
ORIGINAL RESEARCH article
Front. Agron. , 07 May 2024
Sec. Climate-Smart Agronomy
Volume 6 - 2024 | https://doi.org/10.3389/fagro.2024.1339410
The vulnerability of corn yield to high temperature and insufficient rainfall in the US mid-west is widely acknowledged. The impact of extreme weather and genetic development on corn yield is less well known. One of the main reasons is that the multicollinearity in the variables can lead to confounding results. Here we model the impact of climate and genetic development by employing an elastic net regression model to address the multicollinearity issue. This allows us to develop a more robust multiple regression model with higher predictive accuracy. Using granular data for Iowa from 1981-2018, we find that corn yield is vulnerable to high mean summer temperatures particularly in July, a widening diurnal temperature range in June and dry summer conditions (due to extremely low rainfall) from June-August. We find that overall climate impact reduced average annual yield by 0.7%. We also find that genetic development which led to earlier planting dates, widening duration of the reproductive interval, higher growing degree day accumulation and larger net planted area had a beneficial impact on the Iowa corn yield during 1981-2018 resulting in an average annual yield improvement of 1.8% per annum. This provides a basis for optimism that these genetic developments and management practices will continue to adapt and improve in the future to counter the impact of climate change on corn yield. We have also modelled the impact of future climate change using the latest climate projections from the Sixth Assessment Report of the Intergovernmental Panel on Climate Change (IPCC AR6). These climate projections show that the average temperature during the growing season (MayO-October) will increase by 2.4 -2.9 o C by mid-century while the average spring temperature (March and April) will increase by a relatively slower 1.9 -2.3 o C by mid-century. Additionally, climate projections show that both temperature and rainfall will also become more extreme in the future with the changes varying from spring to summer. Our results show that, just due to climate change alone in Iowa corn yield will decline between 1.4-1.7% per annum until mid-century (or 1.2-2.1% per annum until the late twenty first century).
Corn (Zea mays L.) is one of the three most important cereals for global food security (Shiferaw et al., 2011). The US is one of the largest producers of corn in the world with mainly rain-fed production spread over the wide Corn Belt region in the US mid-west. According to the USDA the US accounted for 36% of the total global corn production in 2018 with a yield which is almost twice the global average corn yield (USDA, 2019). Corn yield in the mainly rainfed US mid-west is particularly vulnerable to climate change therefore, understanding the impact of climate change on corn is important to enable farmers and policy makers to devise policies to adapt to climate change. To further the existing research on the impact of climate change on corn yield, the state of Iowa (IA) in the central US mid-west was chosen as the area of interest. IA was selected for this study because it is consistently the largest corn producing US state and accounted for 17% of the total corn produced in the US in 2018. Importantly rich granular phenological growth data is available at the IA district level which is used in this study.
Previous studies show that temperature is the primary climate variable impacting corn yield in the US mid-west. Other factors such as rainfall have a second order impact (Stewart et al., 1998; Streck et al., 2008; Partridge et al., 2019). Studies have shown that at high temperature (beyond the optimal) the negative impact of increasing temperature on yield becomes nonlinear (Rosenzweig et al., 2014; Schlenker and Roberts, 2009). In terms of overall impact global corn yields are projected to decrease on average by 7.4% per 1°C increase in global mean temperature (Zhao et al., 2017) which is a significant global food security risk. The US corn yield is projected to decrease on average between 8%-10% per 1°C rise for temperature change up to 3°C (Lobell and Field, 2007; Schlenker and Roberts, 2009; Mishra and Cherkauer, 2010; Hatfield and Takle, 2014; Ummenhofer et al., 2015; Lee and Durmaz, 2016; Hatfield and Dold, 2018; Tigchelaar et al., 2018). For higher temperature change US corn yield is expected to reduce by 46% for 4°C of warming (Tigchelaar et al., 2018) and 55-60% yield reduction for a 6°C increase in temperature (Schlenker and Roberts, 2009; Huang and Khanna, 2010; Lee and Durmaz, 2016).
Rainfall is also vital for agriculture particularly in the mainly rain-fed US mid-west. Traditionally the region receives most of its rainfall in the summer months. Annual rainfall in the region increased by as much as 20% in some parts since the start of the 20th century (Easterling et al., 2017). However, climate projection models show that in the future, summer rainfall in the US mid-west will decrease by approximately 10% (Easterling et al., 2017). The combination of higher projected temperature and decreased summer rainfall is expected to deteriorate the soil moisture levels resulting in an increased risk of hydrological stress in the region (Ting et al., 2021) during the corn growing season.
Climate projections show that future climate will also become more extreme. In fact, projections show that by the middle of the 21st century the US mid-west will experience an additional 5–20 days of temperatures above 35°C (Xu et al., 2016). Projections of future rainfall indicate extreme rainfall will increase primarily in winter and spring months in the US mid-west (Easterling et al., 2017; Ummenhofer et al., 2015; Baum et al., 2019). This is important because extreme spring rainfall leads to delayed planting and exposes the young seedlings to potential soil-borne diseases leading to yield loss (Nearing et al., 2004; Sacks et al., 2011; Hatfied and Takle, 2014; Hatfield, 2015). Meanwhile extreme temperature inhibits plant growth and studies show that for corn this starts declining at extreme temperatures above 30°C, slowing by as much as 40-50% as the temperature reaches 35°C (Ben-Asher et al., 2008; Hatfield and Takle, 2014).
After allowing for climate, US agricultural output is intimately tied to genetic development. Duvick (2005) reports the vital role of genetic development and corn management practices in the improvement of US corn yields since the 1930s. As in the past, it is expected that genetic development will adapt and improve to counteract the impact of climate change. However, the measurement of genetic development is challenging because of the multicollinearity with others variables such as climate and this can lead to conflicting results (Lusk et al., 2017). Nevertheless, at a practical level the rapid adoption of genetically enhanced corn by US farmers since 1996 demonstrates its perceived beneficial impact. Lusk et al. (2017) using county data from 1980-2015, report that after controlling for weather and soil characteristics, the adoption of genetically enhanced corn was associated with a homogeneous 17% increase in the US corn yield. Xu et al. (2013) also report the positive impact of genetically enhanced corn on yield and expect yields in the US mid-west central corn belt region (Iowa, Illinois and Indiana) to increase by 19-31% during 2011-2030 due to the adoption of genetically enhanced corn.
The objective of this study is twofold. We first fit an elastic net regression model that uses IA district level data from 1981-2018 to model the impact of climate and genetic development on corn yield in IA. Next, we use this regression model to project the impact of future climate change on future corn yield in IA. For this study we have assessed the impact of future climate change using two future greenhouse gas emissions scenarios known as Shared Socioeconomic Scenarios (SSPs) scenarios (SSP2-4.5 and SSP5-8.5) from the Coupled Model Intercomparison Project Phase 6 (CMIP6).
This paper is structured as follows: we describe the materials and methods used in Section 2, followed by a discussion of the results in Section 3. We conclude in Section 4.
We have used historic and future climate data, historic corn growth interval durations, yield and planted area for each of the nine IA districts (Table 1). According to the USDA-NASS definition, “districts” represent a group of counties in a state on the basis of “geography, climate and cropping practices”. Hence it has been assumed here that districts are a homogenous group of counties with respect to corn growth conditions including weather and genotype.
The historic daily minimum and maximum temperature and rainfall data from 1981-2018 for each US county included in this study were downloaded from the Parameter-elevation Regressions on Independent Slopes Model (PRISM) daily dataset. The National Climate Data Centre (NCDC) is commonly used as a weather data source in the agronomic literature, however the PRISM data are preferred here because the NCDC has data gaps for outlying (rural) areas (Johnston and Matlock, 2011). The agricultural district level temperature is derived as the weighted average of the county temperatures.
For future climate data we have used the downscaled climate projections from the NASA Earth Exchange (NEX) Global Daily Downscaled Projections (GDDP) dataset (Thrasher et al., 2022) for 25 Global Circulation Models (GCMs) from the Coupled Model Intercomparison Project Phase 6 (CMIP6). We downloaded the daily minimum, maximum temperature and rainfall data for the period 2015-2099 data for each of the nine agricultural districts in IA for two future greenhouse gas emissions scenarios: SSP2-4.5 and SSP5-8.5, the “medium” and “high” twenty-first century greenhouse gas concentration trajectories respectively (O’Neill et al., 2017; Riahi et al., 2017).
Historic corn yield, corn planted area and growth interval data for the IA districts from 1981-2018 was downloaded from USDA-NASS website. The USDA-NASS defines yield as bushels of corn produced per acre. USDA-NASS produces a weekly national Crop Progress (CP) report during the growing season (April – November) for selected crops including corn. For corn growth interval data, it is not reasonable to assume that all the corn in a district will grow concurrently as it is unlikely that all the corn in a district will be planted on the same date. Following the difference in planting date, the corn in a district is likely to be at different stages of corn growth at anyone reporting date. It is assumed that corn in a particular district attains a particular stage of corn growth when 50% of the crop in the district is reported to have attained that corn growth stage. The CP reports do not report the date when 50% of the crops attain a particular corn growth stage but instead report the percentage of the total district corn crop at various corn growth stages on the reporting day. We estimate the calendar date that corresponds to 50% growth for each corn growth stage by interpolating between the reported calendar dates bracketing the 50% attained phenological growth. The interpolation was carried out using the logistic function which is a well-known plant growth function (Yin et al., 2002). This interpolation process provided an estimated calendar date when 50% of the corn crop in a ‘district attains a particular corn growth stage during each growing season from 1981-2018.
In this section we discuss the empirical model used in the study and the motivation behind it. We have used a multiple regression model (baseline model) to measure the impact of climate and genetic development effects on corn yield.
The multiple (baseline model) regression equation is set up as:
where is the annual percentage change in yield for year t and district d. denotes the annual change in climate explanatory variables, is a measure of the annual change in genetic explanatory variables and represents the error term.
captures the impact of climate explanatory variables such as temperature and rainfall on corn yield. While the varying nature of temperature (mean temperature (linear and quadratic terms), extreme temperature and diurnal temperature range) and rainfall (mean, extreme rainfall (both low and high), temperature and rainfall interaction) impact is discussed in various previous studies (Rosenzweig et al., 2014; Sunoj et al., 2016; Konduri et al., 2020; Sadok and Jagadish, 2020; Niu et al., 2021; Porter et al., 2014; Schlenker and Roberts, 2009; Ting et al., 2021; Ben-Asher et al., 2008; Hatfield and Takle, 2014; Nearing et al., 2004; Sacks et al., 2011) we attempt to capture these ideas in one model in this study.
is represented in the model by:
where is the average monthly mean temperature for month m in set M, where M = {April, May, June, July, August, September, October}; Diurnal.Range is the difference between average daytime (Tmax) and night time (Tmin) temperature; Ext.Temp measures the number of days with daytime temperature above the historical extreme, where this extreme is defined as the 99th percentile of the historical value for the month during 1981-2018; P is the average monthly rainfall; Dry.P is an indicator variable which measures extreme dry conditions when average monthly rainfall falls below the historical extreme low, where this extreme low is defined as the 5thpercentile of the monthly historic rainfall for the month during 1981-2018; Ext.P is an indicator variable which measures extreme high daily rainfall with the threshold for each month set equal to the 90th percentile of the historic (1981-2018) daily rainfall in the month. The percentile values for each extreme variable represent the percentile which gave the best model fit during 1981-2018. The indicator variables (Dry.P and Ext.P) are binary and take a value of 1 when the extreme conditions are met and 0 otherwise. The annual change (during 1981-2018) for temperature variables in (Equation 2) is measured as a difference while for rainfall variables the annual change is measured as a percentage change.
It is challenging to model the impact of genetic explanatory variables ( directly due to the confounding weather effect as reported in previous studies (Lusk et al., 2017). In our model we have used planting date, duration of the vegetative and reproductive growth intervals and growing degree day (GDD) accumulation during these intervals to measure the impact of genetic variables. Additionally net planted area has also been included because this has been found to interact with improved genetic practices (Chavas et al., 2014). in our model is represented by:
Where p.date is the planting date (the calendar day when 50% of the crops have been planted). p.s is the duration (in calendar days) of vegetative growth interval (i.e., when 50% of the crops in the district are at the silking stage). s.h is the duration of the reproductive interval (from the end of the vegetative interval to harvest). GDDp.s is the GDD accumulated during the p.s interval while GDDs.h measures the GDD accumulated during the s.h interval. The interaction between the GDD accumulation and the duration of the respective intervals is allowed for in the model because of the correlation between GDD accumulation and interval duration (Zai et al., 2019). planted.area measures the net planted area (acres) for corn in the district. The annual change in interval durations (during 1981-2018) in (Equation 3) is measured as a difference in days, while the changes in GDD accumulation and planted area are measured as percentage changes.
It is quite normal for climate variables in the baseline model regression model (Equation 1) to exhibit a large degree of multicollinearity. To address this issue, we have used the elastic net regression due to its ability to mitigate both multicollinearity and model overfitting through regularization (Zou and Hastie, 2005). Regularisation techniques such as ridge regression or lasso regression introduce penalties on the size of the coefficients, preventing them from becoming too large and preventing overfitting. The elastic net regression model represents a balance between these two penalties. It deals with multicollinearity among the explanatory variables while simultaneously selecting the important features out of a large set of explanatory variables such as the one we have.
For the elastic net regression, the underlying regression process starts with a general baseline multiple regression model defined as follows:
The regression coefficients for a multiple regression model (Equation 4) are determined by minimizing the traditional sum of squared differences i.e. least sum of squares (Equation 5):
On the other hand, the estimated regression coefficients in the elastic net regression model are determined by minimizing a penalized sum of squares (Equation 6). The penalised sum of squares (L) for any fixed non-negative and , is defined as:
We have applied the elastic net regression process to the baseline model defined in Equation 1. The elastic net regression coefficients are determined by using 10-fold cross validation repeated five times. We calculated the root mean squared error (RMSE) for both the baseline model and elastic net regression model to verify the predictive accuracy of the elastic net model relative to the baseline model. In addition to RMSE, R2 is also calculated for both the elastic net and baseline models to verify the elastic net’s ability to mitigate model overfitting.
In this section we discuss the results of the model fitting exercise. The fitted elastic net regression model has a RMSE of 13.5% (baseline model 13.4%) and a R2 of 77.5% (baseline model 77.4%). Overall, the RMSE and the R2 for elastic net model are only slightly better than the baseline model in this case. A possible explanation for this is that individually the explanatory variables in the baseline model have low regression coefficients and there is little need for aggressive penalised regularisation, thus yielding similar results to the baseline multiple regression model.
Table 2 shows the regression coefficients for selected variables in the fitted elastic net regression model. Traditional statistical inference using p-values is not possible with elastic net regression models as standard errors for the estimated coefficients are not computed. It is possible to use bootstrapping to carry out statistical inference however this is time consuming for large data sets with a high number of independent (predictor) variables as in our case (Chatterjee and Lahiri, 2011). Therefore, the variables selected in Table 2 have been prioritised in terms of the strength of their correlation with yield. The selected variables have a correlation which is significantly different from zero at the 5% significance level.
Table 2 Identification of the main variables impacting corn yield in Iowa and their elastic net regression coefficients (column 5).
The results in Table 2 show that historically corn yield in IA was negatively impacted by high average monthly temperature during July – September (Rows 3-4, Table 2). This is in line with the relationship between yield and high growing season temperatures reported in previous studies (Lobell and Field, 2007; Schlenker and Roberts, 2009; Mishra and Cherkauer, 2010). Our results highlight the yield sensitivity to the July mean temperature in particular, when a 1°C increase in temperature reduced yield by 6.3% on average (regression coefficient of -6.3% in Row 3, Table 2). The sensitivity to the August mean temperature is slightly lower when a 1°C increase in temperature reduced yield by 4.6% on average (Row 4, Table 2). In terms of the diurnal temperature range, we find that the historical yield was most sensitive to the June diurnal range with a regression coefficient of -4.1% (Row 8, Table 2) implying that and a widening of the June diurnal range by 1°C reduced yield by 4.1% on average. The negative impact of widening diurnal range on yield is well recorded (Sunoj et al., 2016; Sadok and Jagadish, 2020; Niu et al., 2021). However, the April diurnal range had an opposite impact (Row 7, Table 2) where a widening of the April diurnal range increased yield by 1.5%. As expected, the positive coefficients (Rows 10 and 11, Table 2) confirm that the average monthly rainfall during the growing season had a beneficial impact on the historical yield. Rainfall in June/July has been particularly important and a 1% increase in average June/July rainfall contributed to a 0.04% (Rows 10 and 11, Table 2) increase in the average yield historically. Rows 12-14 in Table 2 measure the impact on yield of dry conditions brought on by abnormally low monthly rainfall (below the 5th percentile) from June – August. The negative coefficients in Rows 12-14 of Table 2 show that historically the yield declined respectively by 4.2% and 4.0% due to abnormally low rainfall in June and July respectively, while abnormally low August rainfall had the biggest impact reducing yield by 8% on average.
In terms of the genetic variables included in our regression model , we find that historically a 1% increase in the GDD accumulation during the vegetative interval increased the historic yield by 1.7% on average (Row 17, Table 2) whereas a 1% increase in the GDD accumulation during the reproductive interval increased yield by 0.9% (Row 18, Table 2). Our results further show that planting a 1 day earlier increased the historic yield by 1.3% (regression coefficient -1.3% in Row 15, Table 2); increasing the length of the reproductive interval by 1 day increased the yield by 0.6% (Row 16, Table 2). Additionally, our model shows that the increase in net planted area in IA historically also led to an improvement in the yield with a 1% increase in net planted area resulting in a 0.2% improvement in the historic yield on average (Row 19, Table 2).
We next combine the impact of the climate and genetic variables to summarise the historic impact of climate and genetic variables for IA in Figure 1. There are 9 districts in IA: 3 northern, 3 central and 3 southern districts. Instead of showing the results for each of the 9 individual districts, the results in Figure 1 are shown for the combined northern, central and southern districts to remove the noise within the districts and observe trends in the results. The results in Figure 1 show that the impact of the combined climate variables during 1981-2018 on the average yield was noticeably worse in the northern districts (-1.3% per annum) relative to the southern districts (0.1% per annum). On the other hand, the average yield improvement due to the genetic variables in the northern districts (2.3% per annum) was noticeably higher than in the southern districts (1.2% per annum) during 1981-2018. Overall, for IA (averaged across all districts), the combined climate variables accounted for a decline of 0.7% per annum, while the combined genetic variables accounted for an increase of 1.8% per annum in yield from 1981-2018.
Figure 1 Historic impact of combined climate and genetic variables on corn yield in Iowa during 1981-2018. The results are shown for the southern, central and northern Iowa districts as well the average across Iowa districts.
In this section we use the climate change predictions to model the future impact on corn yield at the state level in IA. The GCMs used in this study show that the average growing season temperature in IA is projected to increase by 2.4 – 2.9°C by mid-century and 3.4–6.3°C by late-century. On the other hand, the average spring temperature (March-April) is predicted to increase by 1.9– 2.3°C by mid-century (3.0 – 5.0°C by late-century) which is less than the predicted increase in the average growing season temperature. The relatively slower spring warming is significant because this will mean that farmers might not be able to plant as early as they would like to (with the current technology) to escape the much hotter temperature in the growing season. The GCMs further predict that the growing season temperature will also become progressively more extreme as we move further towards the end of the century. Climate projections show that future average monthly rainfall in IA is expected to increase in the spring months and reduce slightly in the summer by mid-century. Spring rainfall will also become more extreme as we approach mid-century which could lead to delayed planting. The growing season rainfall is also projected to become more extreme. Using the regression coefficients from our model and multiplying these by the projected change in future temperature and rainfall variables, we find that IA corn yield will decline by 1.4% to 1.7% per annum by mid-century (1.2% to 2.1% per annum by late century) for medium/high emissions scenarios relative to the historic average yield. (Figure 2).
Figure 2 Projected impact of climate change on average annual corn yield for IA (combined for all districts). The projections are for mid and late 21st century for medium and high emissions scenarios.
It is not known how genetic development and will adapt to counteract the impact of climate change on yield in the future. Indeed, some studies report that the historic gains in agricultural yield improvement appear to be slowing down (Rizzo et al., 2022). For the purpose of projecting the impact of genetic variables on future yield in IA, we have considered three scenarios for future development in genetic variables: base, optimistic and pessimistic. The base scenario is a constant 1.8% per annum average yield improvement (this is the average historic improvement across IA), the optimistic scenario is a constant 2.3% per annum average yield improvement (this is the historical improvement for the northern IA districts) and the pessimistic scenario is a constant 1.2% per annum average yield improvement (this is the historical improvement for the southern IA districts).
Table 3 shows the projected impact of climate change and genetic variables on IA corn yield for the base, optimistic and pessimistic genetic development scenarios. The projections are shown for mid and late 21st century under both the medium and high emissions scenarios. Instances where the impact on yield of genetic variables is not sufficient to offset the negative impact of future climate change are highlighted red in the table. The results in Table 3 show that improvement in genetic variables will not be able to offset the projected impact of future climate change in IA under the pessimistic scenario. Even under the base genetic development scenario, if we continue with high emissions, by late 21st century the improvement in genetic variables will not be able to offset the negative impact of climate change on yield. Worryingly even for instances where the climate impact is completely offset by genetic development, food security is likely to be challenged by the growing demand driven by the projected human population growth of 50% by mid-century (relative to the start of the 21st century) (United Nations, 2019).
We have modelled the impact of climate and genetic variables on the historic corn yield in IA from 1981-2018. We use an elastic net regression model to address the multicollinearity within the climate and genetic variables. Our regression results show that as expected, historically the IA corn yield depended primarily on the growing season temperature with high temperatures during the growing season reducing yield. We find that historically the July mean temperature has been pivotal, more so than any other month during the growing season. In terms of the impact of the diurnal temperature range, we find that the diurnal range in June has had the most impact on yield with a widening range resulting in reduced yield. As expected, rainfall during the growing season had a beneficial impact on yield. In terms of extremes, and dry summer conditions (due to low rainfall) from June-August had the most impact historically. In terms of genetic variables, we find that historically earlier planting, widening duration of the reproductive interval, higher GDD accumulation and larger net planted area increased the historic yield. This is a significant finding and provides optimism that genetic development can counter the impact of future climate change.
In order to predict the impact of future climate change, we have used the most up-to-date temperature and rainfall predictions from the CMIP6 climate projection models. Our results show that due to future change in temperature and rainfall alone, the average corn yield in IA will reduce between 1.4-1.7% per annum until mid-century (or 1.2-2.1% per annum until the late twenty first century) for medium/high emissions scenarios.
We find that historically development in genetic variables has significantly improved corn yield by an average of 1.8% during 1981-2018. We analysed three different future genetic development scenarios to analyse the impact of genetic variables on future corn yield. Our results show that by late 21st century for high emissions scenario even if we continue with the historic development in genetic variables, the resulting 1.8% per annum improvement in annual yield will not be sufficient to offset the negative impact of climate variables.
The focus of this study is IA and this can be extended to include other states in the Corn Belt as part of future research. Another aspect of this study is that our results are impacted by the genetically enhanced corn adoption phenomenon which has been high in the US relative to other major corn producing countries. As part of future research, the analysis in this study can be extended to include corn growing areas outside the US where the adoption of genetically enhanced corn has been less aggressive.
Publicly available datasets were analyzed in this study. This data can be found here: https://quickstats.nass.usda.gov/, https://www.nccs.nasa.gov/services/data-collections/land-based-products/nex-gddp-cmip6 and https://prism.oregonstate.edu/explorer/.
FZ: Writing – original draft. PM: Writing – review & editing. HH: Writing – review & editing.
The author(s) declare that no financial support was received for the research, authorship, and/or publication of this article.
The authors declare that the research was conducted in the absence of any commercial or financial relationships that could be construed as a potential conflict of interest.
The author(s) declared that they were an editorial board member of Frontiers, at the time of submission. This had no impact on the peer review process and the final decision.
All claims expressed in this article are solely those of the authors and do not necessarily represent those of their affiliated organizations, or those of the publisher, the editors and the reviewers. Any product that may be evaluated in this article, or claim that may be made by its manufacturer, is not guaranteed or endorsed by the publisher.
Baum M. E., Archontoulis S. V., Licht M. A. (2019). Planting date, hybrid maturity, and weather effects on maize yield and crop stage. Agron. J. 111, 303–313. doi: 10.2134/agronj2018.04.0297
Ben-Asher J., Garcia Y Garcia A., Hoogenboom G. (2008). Effect of high temperature on photosynthesis and transpiration of sweet corn (Zea mays L. var. rugosa). Photosynthetica 46, 595–603. doi: 10.1007/s11099-008-0100-2
Chatterjee A., Lahiri S. N. (2011). Bootstrapping lasso estimators. J. Am. Stat. Assoc. 106 (494), 608–625.
Chavas J. P., Shi G., Lauer J. (2014). The effects of GM technology on maize yield. Crop Sci. 54, 1331–1335. doi: 10.2135/cropsci2013.10.0709
Duvick D. N. (2005). Genetic progress in yield of United States maize (Zea mays L.). Maydica 50, 193–202. doi: 10.1016/S0065-2113(05)86002-X
Easterling W., Aggarwal P., Batima P., Brander K., Erda L., Howden M., et al. (2017). “Agriculture,” in Climate change 2014: Impacts, adaptation, and vulnerability. part a: Global and sectoral aspects. contribution of working group II to the fifth assessment report of the intergovernmental panel on climate change. Eds. Field C. B., Barros V. R., Dokken D. J., Mach K. J., Mastrandrea M. D., Bilir T. E., et al (Cambridge: University Press), 485–533.
Hatfield J., Takle G. (2014). “Agriculture,” in Climate change impacts in the United States. The Third National Climate Assessment, 150–174.
Hatfield J., Dold C. (2018). “Climate change impacts on corn phenology and productivity,” in Corn - production and human health in changing climate (InTech). doi: 10.5772/intechopen.76933
Hatfield J., Swanston C., Janowiak M., Steele R., Hempel J., Bochicchio J., et al. (2015). Midwest and northern forests regional climate hub assessment of climate change vulnerability and adaptation and mitigation strategies. Ed. Anderson T. (United States Department of Agriculture), 55 pp.
Huang H., Khanna M. (2010). An econometric analysis of U.S. Crop yield and cropland acreage: Implications for the impact of climate change. SSRN Electronic J. 0(33). doi: 10.22004/ag.econ.61527
Johnston R. Z., Matlock M. D. (2011). “Geospatial climate data,” in Sustainability consortium white paper #104.
Konduri V. S., Vandal T. J., Ganguly S., Ganguly A. R. (2020). Data science for weather impacts on crop yield. Front. Sustain. Food Syst. 4. doi: 10.3389/fsufs.2020.00052
Lee J., Durmaz N. (2016). Impact of climate change on corn production in the U.S.: Evidence from panel study. Appl. Econ. Int. Dev. 16 (1), 93–104.
Lobell D. B., Field C. B. (2007). Global scale climate–crop yield relationships and the impacts of recent warming 1), 014002. doi: 10.1088/1748-9326/2/1/014002
Lusk J. L., Tack J., Hendricks N. P. (2017). Heterogeneous yield impacts from adoption of genetically engineered corn and the importance of controlling for weather (Working Paper No. 23519). National Bureau of Economic Research. doi: 10.3386/w23519
Mishra V., Cherkauer K. A. (2010). Retrospective droughts in the crop growing season: Implications to corn and soybean yield in the Midwestern United States. Agric. For. Meteorology 150, 1030–1045. doi: 10.1016/j.agrformet.2010.04.002
Nearing M. A., Pruski F. F., O'Neal M.R. (2004). [Journal article]. J Soil Water Conserv. 59 (1), 43–50.
Niu J., Feng J., Zhang X., Chen S., Shao L. (2021). Open field simulating nocturnal warming on summer maize performance in the North China plain. Agronomy 11, 992. doi: 10.3390/agronomy11050992
O’Neill B. C., Kriegler E., Ebi K. L., Kemp-Benedict E., Riahi K., Rothman D. S., et al. (2017). The roads ahead: Narratives for shared socioeconomic pathways describing world futures in the 21st century. Global Environ. Change 42, 169–180. doi: 10.1016/j.gloenvcha.2015.01.004
Partridge T. F., Winter J. M., Liu L., Kendall A. D., Basso B., Hyndman D. W. (2019). Mid-20th century warming hole boosts US maize yields. Environ. Res. Lett. 14 (11). doi: 10.1088/1748-9326/ab422b
Porter J. R., Xie L., Challinor A. J., Cochrane K., Howden S. M., Iqbal M. M., et al. (2014). “Food security and food production systems,” in Climate change 2014: Impacts, adaptation, and vulnerability. part a: Global and sectoral aspects. contribution of working group II to the fifth assessment report of the intergovernmental panel on climate change. Eds. Field C. B., Barros V. R., Dokken D. J., Mach K. J., Mastrandrea M. D., Bilir T. E., et al (Cambridge: University Press), 485–533.
Riahi K., van Vuuren D. P., Kriegler E., Edmonds J., O Neill B. C., Fujimori S., et al (2017). The Shared Socioeconomic Pathways and their energy, land use, and greenhouse gas emissions implications: An overview. Global Environmental Change 42, 153–168. doi: 10.1016/j.gloenvcha.2016.05
Rosenzweig C., Elliott J., Deryng D., Ruane A. C., Müller C., Arneth A., et al. (2014). Assessing agricultural risks of climate change in the 21st century in a global gridded crop model intercomparison. Proc. Nat. Acad. Sci. 11 (9), 3268–3273. doi: 10.1073/pnas.1222463110
Sacks W. J., Kucharik C. J. (2011). Crop management and phenology trends in the U.S. corn belt: Impacts on yields, evapotranspiration and energy balance 7), 882–894. doi: 10.1016/j.agrformet.2011.02.010
Sadok W., Jagadish S.V. K. (2020). The Hidden Costs of Nighttime Warming on Yields. Trends Plant Sci. 25, 644–651.
Schlenker W., Roberts M. J. (2009). Nonlinear temperature effects indicate severe damages to U.S. crop yields under climate change. Proc. Natl. Acad. Sci. U.S.A. 106 (37), 15594–15598. doi: 10.1073/pnas.0906865106
Shiferaw B., Prasanna B. M., Hellin J., Bänziger M. (2011). Crops that feed the world 6. Past successes and future challenges to the role played by maize in global food security. Food Secur. 3 (3), 307–327. doi: 10.1007/s12571-011-0140-5
Stewart D. W., Dwyer L. M., Carrigan L. L. (1998). Phenological temperature response of maize. Agron. J. 90, 73–79. doi: 10.2134/agronj1998.00021962009000010014x
Streck N. A., Lago I., Gabriel L. F., Samboranha F. K. (2008). Simulating maize phenology as a function of air temperature with a linear and a nonlinear model. Pesqui. Agropecu. Bras. 43, 449–455. doi: 10.1590/S0100-204X2008000400002
Sunoj V. S. J., Shroyer K. J., Jagadish S. V. K., Prasad P. V. V. (2016). Diurnal temperature amplitude alters physiological and growth response of maize (Zea mays L.) during the vegetative stage. Environ. Exp. Bot. 130, 113–121. doi: 10.1016/j.envexpbot.2016.04.007
Thrasher B., Wang W., Michaelis A., Melton F., Lee T., Nemani R. (2022). NASA global daily downscaled projections, CMIP6 1), 262. doi: 10.1038/s41597-022-01393-4
Tigchelaar M., Battisti D. S., Naylor R. L., Ray D. K. (2018). Future warming increases probability of globally synchronized maize production shocks. Proc. Natl. Acad. Sci. United States America 115 (26), 6644–6649. doi: 10.1073/pnas.1718031115
Ting M., Seager R., Li C., Liu H., Henderson N. (2021). Future summer drying in the U.S. Corn belt and the role of midlatitude storm tracks. J. Climate 34, 9043–9056. doi: 10.1175/JCLI-D-20-1004.1
Ummenhofer C. C., Xu H., Twine T. E., Girvetz E. H., McCarthy H. R., Chhetri N., et al. (2015). How climate change affects extremes in maize and wheat yield in two cropping regions. J. Climate 28, 4653–4687. doi: 10.1175/JCLI-D-13-00326.1
United Nations, Department of Economic and Social Affairs, Population Division (2019). World population prospects 2019: Highlights (ST/ESA/SER.A/423).
Xu Z., Hennessy D. A., Sardana K., Moschini G. C. (2013). The realized yield effect of genetically engineered crops: U.S. maize and soybean. Crop Sci. 53, 735–745. doi: 10.2135/cropsci2012.06.0399
Xu H., Twine T. E., Girvetz E. (2016). Climate change and maize yield in Iowa. PLoS One 11 (5), 1–20. doi: 10.1371/journal.pone.0156083
Yin X., Goudriaan J., Lantinga E. A., Vos J., Spiertz H. J. (2002). A flexible sigmoid function of determinate growth. Ann. Bot. 91 (3), 361–371. doi: 10.1093/aob/mcg029
Zai F. H., Hamers H., McSharry P. E. (2019). Evaluating the predictive accuracy of corn phenological durations in Iowa and Illinois. Agron. J. 111 (3), 1128–1140. doi: 10.2134/agronj2018.04.0294
Zhao C., Liu B., Piao S., Wang X., Lobell D. B., Huang Y., et al. (2017). Temperature increase reduces global yields of major crops in four independent estimates. Proc. Natl. Acad. Sci. United States America 114 (35), 9326–9331. doi: 10.1073/pnas.170176211
Keywords: corn yield, extreme weather, elastic net regression, genetic development, climate change
Citation: Zai FH, McSharry PE and Hamers H (2024) Impact of climate change and genetic development on Iowa corn yield. Front. Agron. 6:1339410. doi: 10.3389/fagro.2024.1339410
Received: 16 November 2023; Accepted: 12 April 2024;
Published: 07 May 2024.
Edited by:
Domenico Ronga, University of Salerno, ItalyReviewed by:
Jordi Doltra, Institute of Agrifood Research and Technology (IRTA), SpainCopyright © 2024 Zai, McSharry and Hamers. This is an open-access article distributed under the terms of the Creative Commons Attribution License (CC BY). The use, distribution or reproduction in other forums is permitted, provided the original author(s) and the copyright owner(s) are credited and that the original publication in this journal is cited, in accordance with accepted academic practice. No use, distribution or reproduction is permitted which does not comply with these terms.
*Correspondence: Faisal H. Zai, ZmFpc2FsemFpQGdtYWlsLmNvbQ==
Disclaimer: All claims expressed in this article are solely those of the authors and do not necessarily represent those of their affiliated organizations, or those of the publisher, the editors and the reviewers. Any product that may be evaluated in this article or claim that may be made by its manufacturer is not guaranteed or endorsed by the publisher.
Research integrity at Frontiers
Learn more about the work of our research integrity team to safeguard the quality of each article we publish.