- Crop Health, Faculty of Agricultural and Environmental Sciences, University of Rostock, Rostock, Germany
Introduction: Arable weeds adapt to any changes in disturbance and management. On arable fields, tilling disturbs the soil, while cropping practices like rotation, pesticide use, fertilizer use, and the use of subsidiary crops characterize the management of the field. On a species level, weeds adapt in their abundance and composition. The species have certain traits to use the on-site resources best. Our objective was to investigate if traits beyond just species describe the adaptation of weeds to tillage and cropping systems. Therefore, we present a use case of weeds on fields cropped with maize in Germany.
Material and methods: Over 2 years, we conducted a nationwide weed survey on 577 maize fields. On each field, the abundance of each weed species was counted on 10 randomly sampled square plots of 0.1 m² not treated with herbicides at the fourth to eighth leaf stages of the maize. Weed species frequent on at least 5% of the fields were assigned traits. Traits were taken from published updated databases. We interviewed all farmers about the management practices of their fields.
Results: The management practice data identified three management clusters of tillage and cropping sequence on maize fields. The standard system so far is a management system characterized by inversion tillage and maize cropped in rotations (“Traditional”). The two transformation systems are maize cropped in rotations but with non-inversion tillage as the method of soil disturbance (“Conservational”) and maize cropped continuously regardless of the tillage system (“Monoculture”). Ordination techniques showed that both weed species and traits are assigned to these management systems. Traditional disfavored weed species with a greater plant height. Conservational selected dicot weed species with a high seed weight and a long flowering duration. Monoculture mainly filtered monocot weed species and favored weeds that germinate in spring.
Discussion: Our study describes weed responses on transformations of maize cropping in tillage and cropping systems on both the species and the trait level. This application expresses the importance and value of collecting weed surveys with field management data on a geographically widespread and repeated timescale.
1 Introduction
Plants are able to adapt to environmental factors like location, climate, and soil (Lascoux et al., 2005-2010). For plants spontaneously growing on arable fields, commonly called arable weeds, it is indispensable to adapt to any changes in disturbance and management of fields. On arable fields, tilling disturbs the soil. At the same time, cropping practices like rotation, pesticide use, such as glyphosate, fertilizer use, and the use of subsidiary crops characterize the further management of the field.
Weeds on arable fields are monitored for various purposes, spanning from vegetation to weed science (Bürger et al., 2022). The approaches differ in their general focus: in vegetation science, complete species inventories allow ecological and phytosociological applications such as classification of arable weed communities and analyzing habitat and land use effects on species (e.g., Manthey, 2003; Chytrý et al., 2016); in weed science, the focus is on applied agronomic aspects. Most frequent species, together with management data, allow to disentangle drivers of change (Bürger et al., 2022). Bürger et al. (2020) collected weed vegetation records from arable fields in Europe in a database: “Arable Weeds and Management in Europe” (AWME). Using the AMWE database, Metcalfe et al. (2023) derived case studies to elaborate challenges and opportunities from a joint European-wide database.
Weed monitoring generally delivers species present in the fields, their frequencies, and abundances in various spatial and time contexts (Hanzlik and Gerowitt, 2016). These data provide insight into weed species composition and whether changes indicate an adaptation to altered arable management practices (Pinke et al., 2012; Salonen et al., 2013; Nguyen and Liebman, 2022). The effects of management factors like tillage and fertilization are investigated in several experiments; e.g., Travlos et al. (2018) describe these effects on weed communities and diversity indices in a comprehensive review.
The current crop generally appears significant for the composition of weed species in surveys (Fried et al., 2008; Andreasen and Skovgaard, 2009). Surveying weeds in just one crop excludes this factor and thereby prevents the effects of disturbance and crop management. Prominent examples use this by exclusively targeting one crop (Salonen, 1993; Pinke et al., 2022; Salaudeen et al., 2022).
In Germany, maize (Zea mays L.) has continuously increased in acreage during the past 60 years. Today, maize is cultivated on 15% of the total farmed area of Germany, making maize now the second most frequent crop after wheat. Maize is today used as feed and for producing biogas (Statista, 2023a; Statista, 2023b; Statista, 2023c).
A weed monitoring of maize fields in Germany from the years 2001–2009 identified Chenopodium spp., Stellaria media, Polygonum convolvulus, Echinochloa crus-galli, Matricaria spp., and Viola arvensis as the most frequent weed species (de Mol et al., 2015). The authors further elaborated that the environment has a more significant influence on the weed species composition than management practices. However, crop sequence had more effect on the weeds than tillage. Redwitz and Gerowitt (2018) analyzed weeds monitored in maize in four Northern German regions from 2011 to 2013. They revealed that the continuous maize cropping history had a powerful effect on the weed species composition. Species are the common unit for assessing weeds in the fields, which are focused in the sophisticated analyses of these two monitoring.
To use the on-site resources best, species have certain traits. In ecology, numerous trait databases were compiled (e.g., Fitter and Peat, 1994; Kleyer et al., 2008) and used to analyze differences in strategies to assemble communities (e.g., Grime et al., 1997). Recently, several authors used this approach to predict weed communities’ responses to management changes in agricultural systems (e.g., Hawes et al., 2009; Storkey et al., 2010; Pinke and Gunton, 2014). Due to these traits, weed species can somewhat replace each other (Hofmeijer et al., 2021). Thus, weed traits successfully allow for overcoming the species focus (Fried et al., 2012; Bàrberi et al., 2018; Hofmeijer et al., 2021). Today, traits are collected and publicly available in databases (e.g., LEDA1; BioFlor2).
Multivariate methods were developed to analyze whether changes in weed species are connected to the traits they possess. Two complementary approaches, the RLQ and the fourth-corner statistic, can reveal whether there is a relationship between the environment of a field, the recorded weed species, and the weed traits (Dolédec et al., 1996; Dray et al., 2014). The RLQ analysis considers the intercorrelation of the environment and weed traits through weed species abundance. The methods have been recently used to describe the temporal change of weed communities in wheat in France (Fried et al., 2012) and weed communities in the Baltic region (Hofmeijer et al., 2021) or to describe the effect of tillage on weed communities in wheat and soybean (Bàrberi et al., 2018).
We present data from a nationwide weed survey on fields cropped with maize in Germany in 2017 and 2018. This case study shall be used as an exercise for analyzing weed communities and weed traits in maize fields. We expect that the weeds present in maize fields have continued to change in the last decade, facilitated by the management of the maize fields. Frequencies and abundances of main weed species indicate these changes. Weed communities are expected to respond to field management. Though both management and weed data were surveyed in an applied agronomic approach, we hypothesize that the traits of the surveyed weeds reflect essential management changes.
2 Materials and methods
We conducted a nationwide weed survey on 577 maize fields in Germany in 2017 and 2018 (Figure 1). The fields represent all maize-growing regions in Germany. The network of sales consultants of Syngenta Agro GmbH assisted in finding fields and farmers nationwide. Universities of applied science and national authorities in Germany regionally acquired maize fields. Geographically, the sites were distributed all over Germany (42,72 to 54,78 N° and 5,96 to 14,81 E°), covering a height above sea level from -1 to 731 m. Not randomly distributed, the number of surveyed fields instead mirrored regional maize-cropping densities.
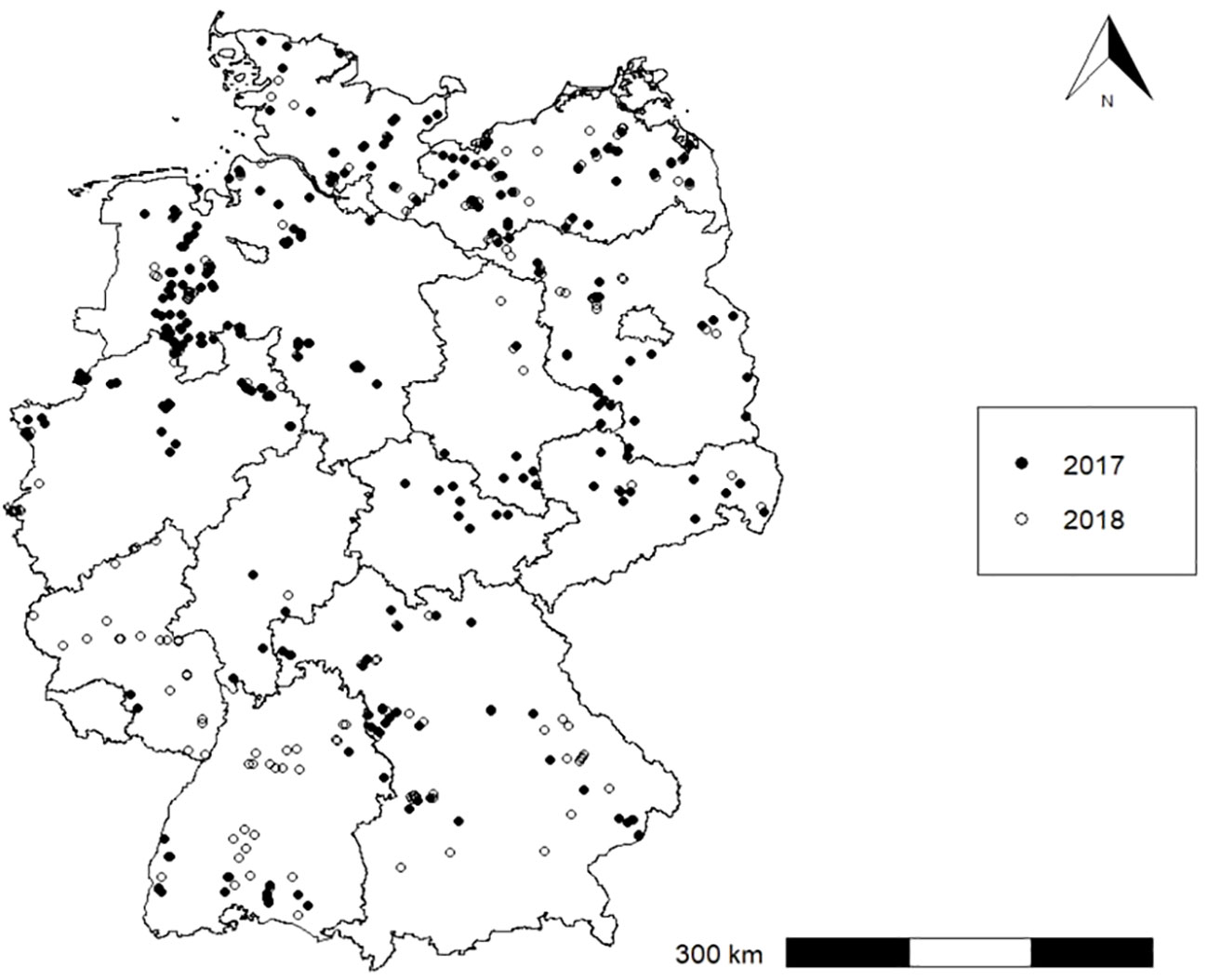
Figure 1 Locations of maize fields in Germany (n = 577) in 2017 and 2018. Location of n = 19 missing.
2.1 Management data
Data acquired through interviewing farmers included site conditions, tillage, and the maize-cropping practice. Soil characteristics, such as texture (sand, sandy loam, sandy clay, silt loam, silty loam, clay loam, and clay), humus content (low, moderate, and high), and soil pH (5–5.5, 5.5–6.0, 6–6.5, and ≥6.5) describe the sites. Two main tillage systems were distinguished: inversion tillage with a moldboard plow and non-inversion tillage mainly with chisel plowing. We asked whether farmers treated with glyphosate products before tillage. Cropping practices include fertilizer management (organic, mineral) and crop-sowing patterns (date, density). No GMO maize varieties (e.g., for glyphosate resistance) were cropped in Germany. The preceding crops were grouped (i) either continuously cropping maize or (ii) cropping maize in rotation with other crops (e.g., oilseed rape, winter wheat, winter barley, and winter rye). Farmers documented the use of subsidiary crops (e.g., Lolium perenne, Festuca rubra, Raphanus sativus var. oleiformis, Guizotia abyssinica, Phacelia tanacetifolia), if at all.
2.2 Weed field data
In every surveyed field, weeds were assessed in a 100 m² plot untreated with herbicides. These plots were at least 20 m away from the field edge. The survey took place between the fourth and eighth leaf stages of the maize crop. A trained professional identified the weed species and counted their abundance in 10 randomly selected subplots of 0.1 m². For the analysis, the abundance per weed species in every field was summed up to 1 m². The frequency was then calculated using presence or absence in each field about all fields. After removing species with frequencies less than 5% in the maize field, 31 weed species remained in the data set for further analysis.
2.3 Weed trait data
A trait database was linked to the remaining 31 weed species. Most traits were selected from published data collections (Bàrberi et al., 2018; Bourgeois et al., 2019); LEDA1; BioFlor2). Seventeen traits describe the disservice and service of weeds, and one trait (herbicide resistance documented for Germany) was selected from the International Herbicide-Resistance Weed Database3. The complete list of traits and their trait levels/values is given in Table A1. In addition, the individual traits of the remaining 31 weed species are provided in Table A2.
2.4 Data analysis
Agglomerate hierarchical clustering identified three clusters in the management data. For a strong clustering structure, dissimilarities in binary management data were analyzed with the “Manhattan” distance. Then, the agglomeration method “Ward. D2” merged pairs of clusters with the smallest linkage distance.
From the recorded weed species data per field, the true diversity, an exponent of the Shannon index, was calculated, in addition to the mean number of weed species. Multivariate unconstrained ordination elucidated how maize management clusters relate to weed species. Weed species density data were Hellinger-transformed and underwent a principal component analysis (PCA). Weed species and clusters on maize management patterns were projected a posteriori as binary data.
The RLQ analysis combines three matrices: the R-matrix describes the fields by the environment, the L-matrix describes the fields by the density of weed species, and the Q-matrix adds the traits for the weed species. We used the management clusters for the R-matrix. Each matrix in an RLQ analysis runs separately: a correspondence analysis (CA) on the L-matrix and a Hill-and-Smith analysis on the R- and Q-matrix. The significant link between the R and Q matrices was tested in a Monte Carlo permutation (n = 999). The fourth-corner method pairwise tests the significant relationship between environment and weed trait data. The fourth-corner method used a permutation model based on 49,999 permutations.
Statistical analysis was performed with R software version 4.3.1 (R Core Team, 2022). The R package “cluster” (Rousseeuw et al., 2022) was used for cluster analysis, and “factoextra” (Kassambara and Mundt, 2020) was used to visualize the cluster results. Diversity indices were calculated, and the PCA on the weed community was performed with the package “vegan” (Oksanen et al., 2022). For the fourth-corner matrix, we used the package “ade4” (Dray and Dufour, 2007). The packages “prettymapr,” “raster,” and “sp” were used to display the field map (Dunnington, 2023; Pebesma et al., 2023; Robert, 2023).
3 Results
3.1 Weed species
In total, 119 species were identified. The most frequently found species were Chenopodium album (82%), P. convolvulus (56%), E. crus-galli (50%), V. arvensis (43%), and S. media (39%). Frequent species also had the highest abundance per field (Table 1).
3.2 Weed traits
Weeds of 16 botanical plant families were found. The families Gramineae (7) and Polygonaceae (5) were the most frequent. The majority (26 of 31 dominant weed species) were therophytes, according to Raunkiaer life form. Eighteen species were ascending/creeping growth style (GTF), seven built rosettes, and another seven grew graminoid. Annuals dominated (23 out of 31); five were biannual, and four were perennials. The Grimes life forms are competitive ruderals (15) and ruderals (13). Spring (15) and autumn (14) germinating species were frequent, while only two summer germinating and one year-round germinating species were found. The Ellenberg indicator values for nitrogen mainly categorize the species with 6–8, indicating a need or even a strong need for nitrogen. None of the species was avoiding or strongly avoiding N. On average, the species had a specific leaf area of 29.2 cm² g-1, a plant height of 0.8 m, and a seed weight of 3.04 mg.
3.3 Management
Maize was typically cropped on fields with sandy soils with a moderate humus content and a soil pH of 6–6.5 and commonly sown at the end of April (Julian day; 119 ± 7) with 9 ± 7 plants m-2. Fertilizer was applied as organic (249 sites with slurry, 200 sites with residuals from biogas plants, and 102 with manure) and mineral fertilizer. Total fertilization resulted in 164.2 kg ha-1 N ± 23%, 82.46 kg ha-1 P ± 47%, and 133.06 kg ha-1 K ± 54%; values are given as mean ± coefficient of variance.
The surveyed maize fields differed in their cropping sequence. Maize was cropped in rotation with other crops on 85% of all fields, 15% were continuously cropped with maize, and the most extended period was 35 years. The tillage systems were moldboard plowing at 52% and conservational tillage at 48%. Conservational tillage was characterized by not inverting the soil but mulching the upper layer. Zero tillage, meaning no intervention into the soil at all, was not practiced. In the winter before the maize, a subsidiary crop was established on 48% of the sites. Subsidiary crops undersown in the maize were only established in 14 of the 577 fields. On 21% of the fields, glyphosate was applied before seedbed preparation.
The cluster analysis, including all management variables, splits into three clusters (Figure 2). The dominating variables were rotation and tillage. The analysis revealed that farmers practice three different management combinations of tillage and rotation. We call these management systems. In the first cluster, maize is cropped in rotation combined with inversion tillage by moldboard plowing. This system is called “Traditional” (blue in Figure 2). The second cluster applying a crop rotation is “Conservational” (red in Figure 2). In “Conservational,” the soil is not inverted by plowing; the topsoil is tilled by mulching or chisel plowing. In both Traditional and Conservational, roughly the same percentage of fields carried subsidiary crops (51% in the Traditional and 55% in the Conservational). In the Conservational system, glyphosate was applied more frequently than in the Traditional (10% in the Traditional and 36% in the Conservational). Maize following maize characterizes the third system—we refer to this as “Monoculture” (green in Figure 2). The type of tillage is equally either inversion or non-inversion tillage. In this system, subsidiary crops were not often established (17%), and glyphosate before maize sowing was not frequently applied (14%).
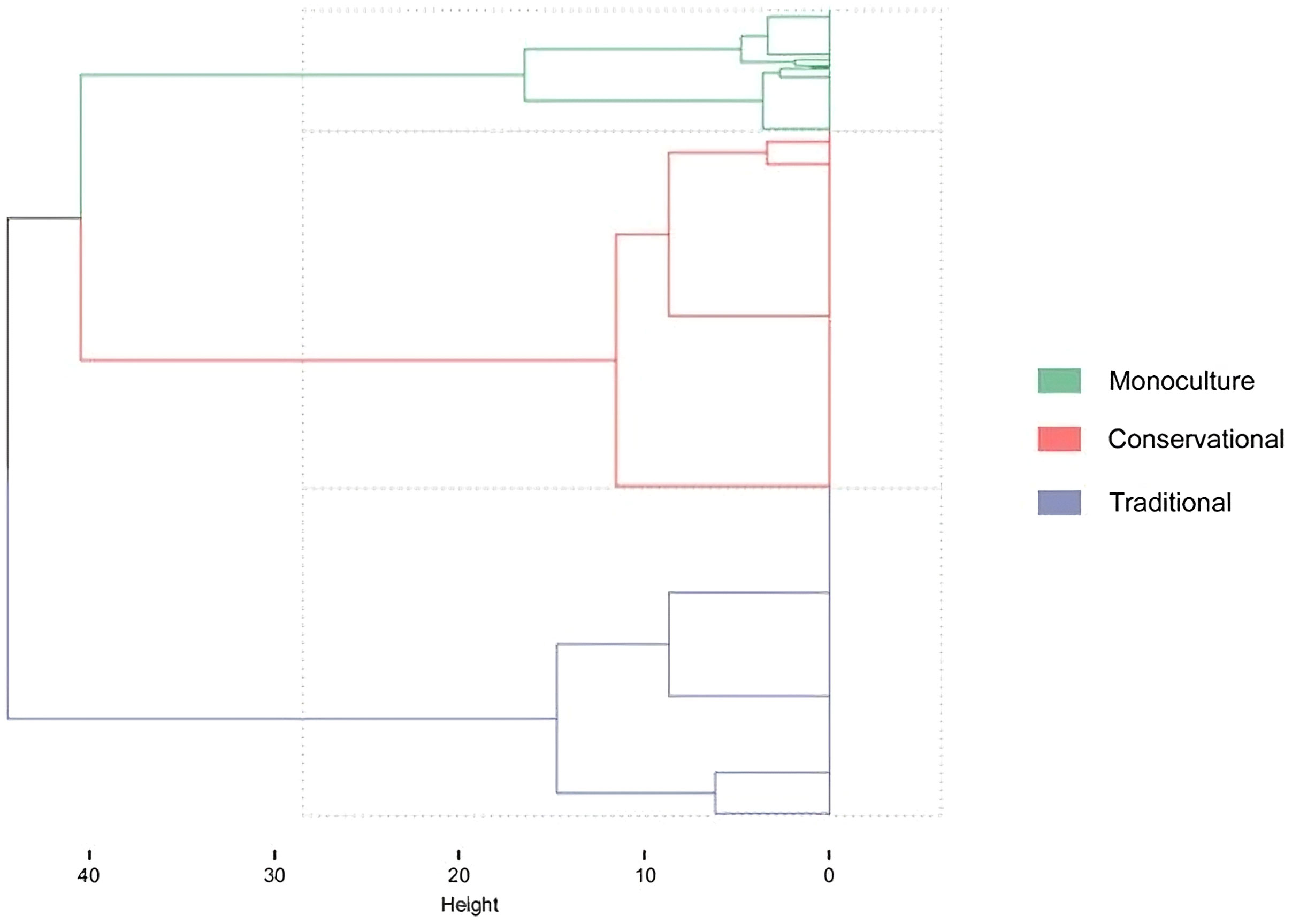
Figure 2 Hierarchical clustered all management variables of maize fields (n = 577) using the cluster method Ward.D2 and distance metric Manhattan. Split-off into three clusters: “Traditional” (blue), “Conservational” (red), and “Monoculture” (green).
3.4 Management system and weed species diversity
The mean number of species and true diversity varied across management systems (Table 2). Traditional had the highest, while Conservational and Monoculture had the lowest mean number of species and true diversity, respectively. The types of species differed within the systems. This is reflected by the true diversity, as the value is reported to be almost twice as high in all fields as in each system.
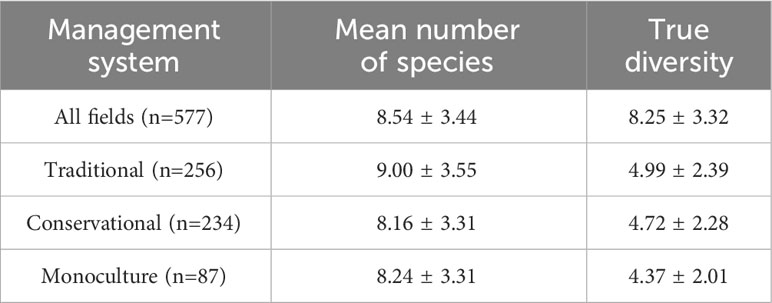
Table 2 Mean number of species and true diversity index (± standard deviation) of all observed fields and in the three maize management systems.
Ordination analysis of the weed species community in maize indicated certain patterns (Figure 3). The first, second, and third axes of the PCA represented 20.1% of the total variance. Figures 3C, D allowed a closer examination of the center of Figures 3A, B. Management systems were plotted as centroids with their levels (0 or 1; 1 indicated the belonging to the management system) in Figure 3. The weed species loaded differently on the three management systems. The centroid for Traditional (T1 blue) is most centered in the perspective of the first and second axes, also confirmed if the third axis is plotted. Frequent species in the center of the ordination like Polygonum lapathifolium, Trifolium repens, Lamium purpureum, and Matricaria chamomilla are associated with Traditional. Perennials such as Agropyron repens, Cirsium arvensis, and Rumex obtusifolius are also loaded on the management system Traditional.
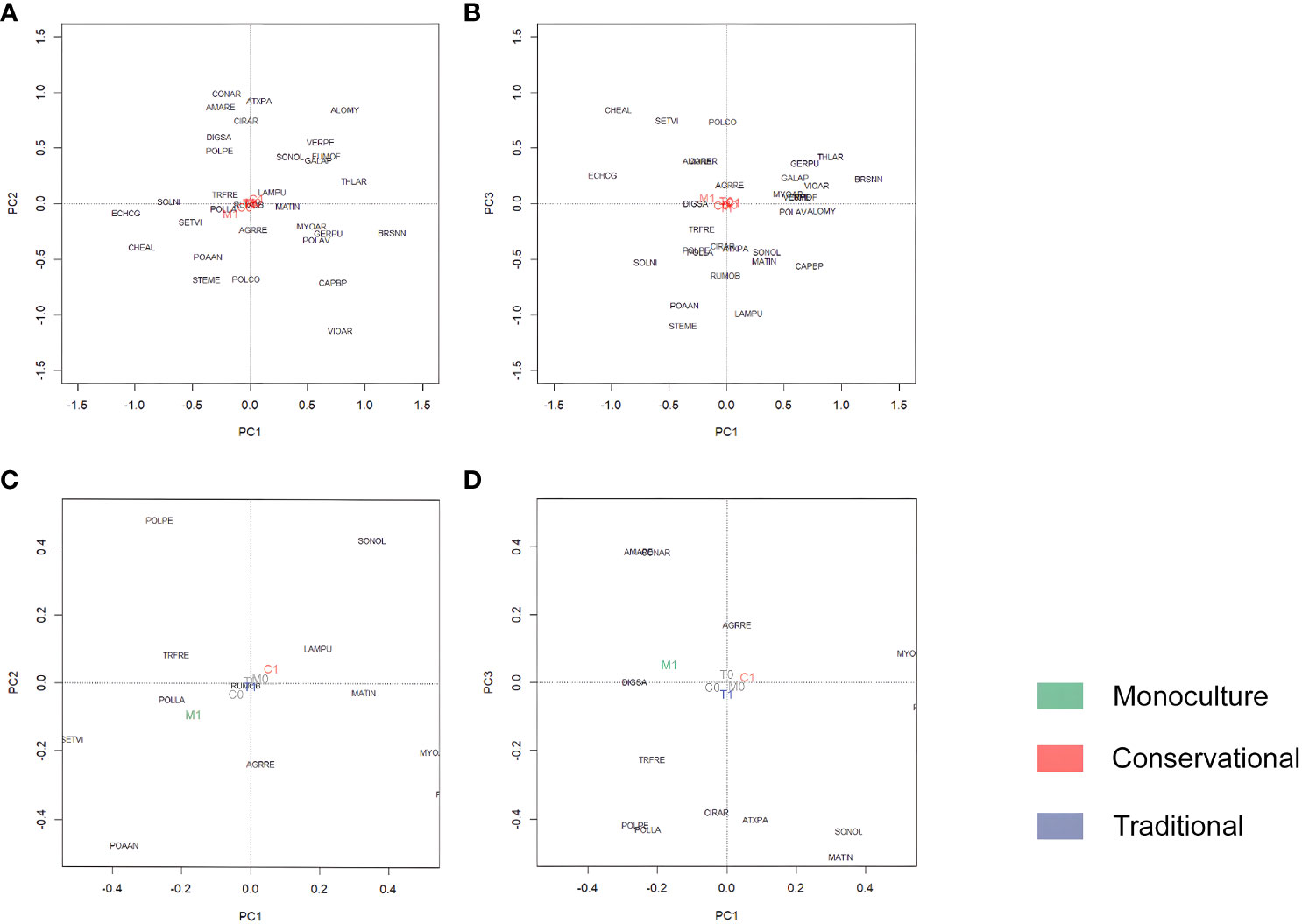
Figure 3 Principle component analysis of the weed species [n = 31, indicated by EPPO-code (http://eppt.eppo.org)]. Centroid results from the management systems (0,1; 1 indicates the belonging to the management system). Conservational (C0, C1; C and D: red), Traditional (T0, T1; C and D: blue), and Monoculture (M0, M1; C and D: green). (A) represents PC1 and PC2 with a total variance of 14.2%, and (B) represents PC1 and PC3 with a total variance of weed species of 12.5%. (C, D) are zoomed in the center of (A, B), respectively.
Opposite centroids (C1 red and M1 green) for the other two management systems indicate a clear difference in the weed species communities they favor. Conservational is associated with V. arvensis, Veronica persica, Geranium pussilum, Fumaria officinalis, Galium aparine, Alopecurus myosuroides, Thlaspi arvensis, and Myosotis arvensis. Brassica napus, the only volunteer crop occurring frequently enough in maize fields, is also clearly associated with the Conservational management cluster. Sited opposite in both perspectives, species like C album, E. crus-galli, Fallopia convolvulus, S. media, Solanum nigrum, Setaria viridis, and Poa annua load on Monoculture.
3.5 Management system and weed traits
Figure 4 summarizes the results of the fourth-corner approach, which followed the RLQ analysis. Gray cells indicate an empty fourth corner complementing the three corners resulting from the three matrices management, species, and traits. Positive correlations are indicated with yellow-striped cells and negative with blue-dotted cells.
Correlations with traits and their functional group appeared in all management systems. In Traditional, three correlations were identified. Weeds in this system belong to the family Labiatea and begin flowering in March. There was also a negative correlation with plant height. In Monoculture and Conservational, the trait correlations alternated. The five traits, being a monocot/dicot, belonging to the family Graminae, growing graminoid, long duration of flowering (6 months), and supporting pollinators were advantageous in converse directions in these two systems, as negative correlation in one corresponded with a positive in the other. Conservational correlated positively and exclusively with a high seed weight. Besides favoring monocots and graminoid-growing species, Monoculture was advantageous for spring germinators, while autumn germinators suffered.
4 Discussion
The analysis of our survey revealed that weeds present in the current maize crop mirror management in terms of rotation and tillage systems. This result appeared based on the weed species composition. Using analyzed traits, weed species deliver interpretable reactions in the management systems, allowing estimations of adaptations to changes.
Previous monitoring in Germany found that C. album, P. convolvulus, E. crus-galli, V. arvensis, and S. media were ranked as the most frequent weed species (de Mol et al., 2015). However, the absolute frequencies and abundances of just C. album and S. media changed. Despite staying very frequent, C. album was almost twice as abundant as in the previous study (de Mol et al., 2015). The frequency of S. media decreased by 20%. The German spring weather is typically humid, but in 2018, the spring was exceptionally dry. As the germination of S. media is known to depend on temperature and humidity (Grundy et al., 2000), we identify this as the main reason for the change. Based on the frequencies, we cannot confirm that weed species in maize fields have changed dramatically in the last decade.
In addition to the most frequent species, the ordinations present the complete weed community. At first glance, weed species in maize fields clump together in the center of the ordination in the two perspectives (Figures 3A, B), indicating that the weed species do not differ much in their demands. This is not surprising, as the sampled fields carried one crop, and all were conventionally fertilized.
Clustering distinguished three different management systems for maize in Germany, differing by crop rotation and tillage. “Traditional” is the past maize cropping system, with a crop rotation and plowing the soil by inversion tillage. The two other systems represent recent transformations in maize growing in Germany. “Conservational” is a management system with non-inversion tillage and higher glyphosate input than the others but with a previous crop other than maize. “Monoculture” is characterized by no crop sequence and little subsidiary crop.
A closer examination of the ordination (Figures 3C, D) yields a more distinct picture because these management systems indicate that species were more connected with one or the other. Species in the center of the ordination load on the system Traditional. The systems Conservational and Monoculture appear opposite in both ordination perspectives. Species loading on the one are much less connected to the other. Hence, the above-described transformation processes to reduce plowing and to stop rotational growing are mirrored by the weed species loading on these systems rather than on Traditional.
The RLQ analysis based on the traits of the species delivers a figure (Figure 4) comparable to that of the species ordination. Weeds in Traditional are correlated with few traits indicating an ordinary weed vegetation in maize. The weed traits correlated with Conservational and Monoculture are the opposite effects of the two transformation systems.
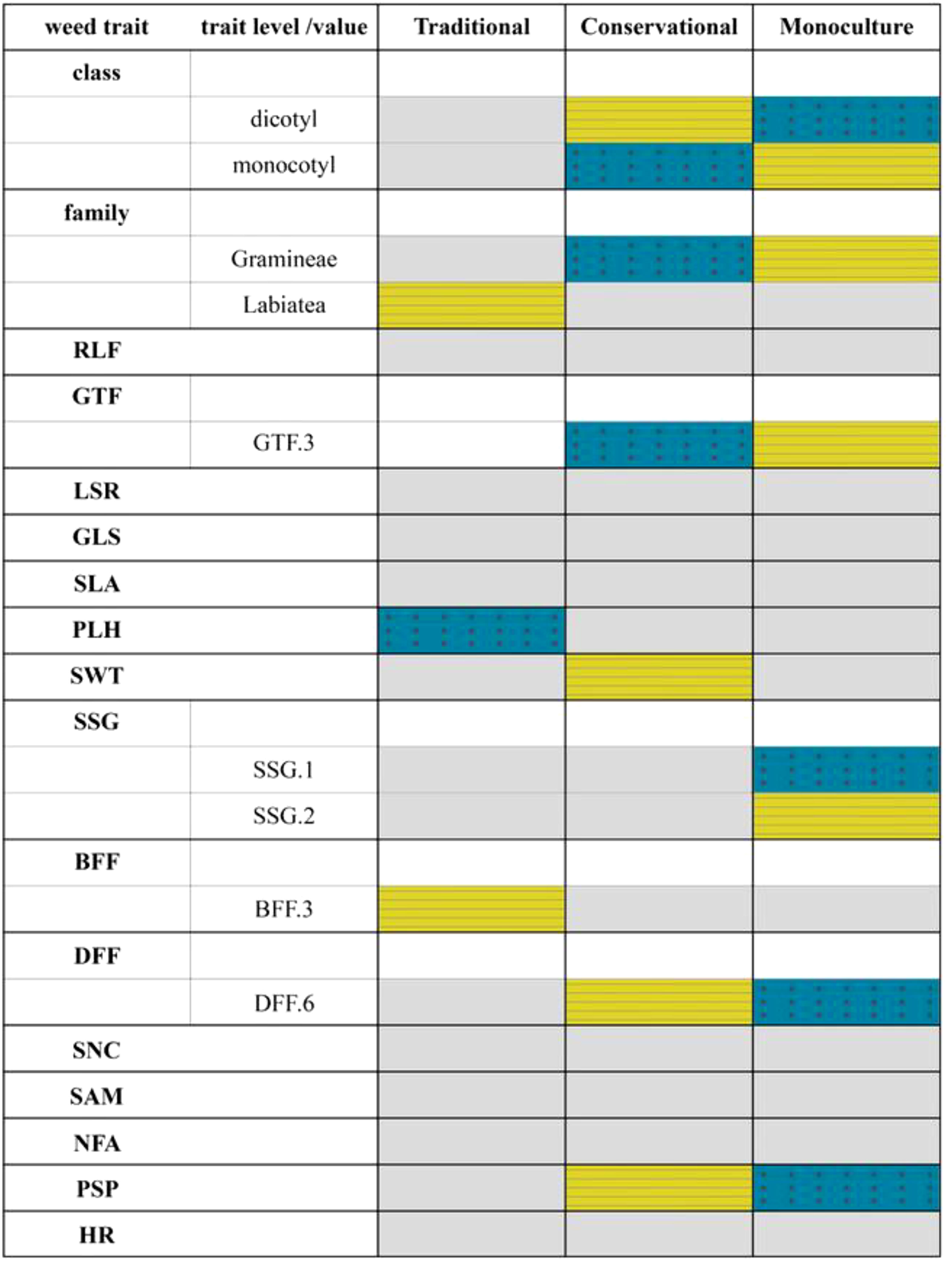
Figure 4 Weed traits, their functional groups and management system. Yellow striped = positive correlation, blue dotted = negative correlation, gray = no correlation of management system and weed trait (p ≥ 0.05). Traits and trait level/value are explained in Appendix Table A1. Raunkiaer life form (RLF), Growth form (GTF), Life span (LSR), Grime’s life strategy (GLS), Specific leaf area (SLA), Plant height (PLH), Seed weight (SWT), Seasonality of germination (SSG), Beginning of flowering (BFF), Duration of flowering (DFF), Affinity to spill nutrient conditions (SNC), Arbuscular mycorrhizal fungi (SAM), N-fixing ability (NFA), Support of pollinators (PSP), and Herbicide resistance (HR). This association is measured by a Pearson correlation coefficient for two quantitative variables (trait and environmental variable), by a Pearson chi-square and G statistic for two qualitative variables, and by a Pseudo-F and Pearson r for one quantitative variable and one qualitative variable.
In the ordination, three out of four perennial weed species are more connected to Traditional than to the two transformation systems. However, the RLQ analysis did not identify “being perennial” as a trait positively correlated to the system Traditional. Inversion tillage, characterizing Traditional, is often regarded to restrict perennial weeds better than non-inversion systems (Carr et al., 2012; Carr et al., 2013; Feledyn-Szewczyk et al., 2020). Koning et al. (2019) treated arable vegetation by inverting the soil or spraying glyphosate. It appeared that arable perennials profited from plowing. Other traits than being a perennial seem to be more important. Plant height correlated negatively with Traditional, meaning weed species have a lower height than average. It has been reported that the trait plant height is influenced by tillage (Fried et al., 2012). In Traditional, more weeds than in the other systems germinate in early spring. This is an apparent effect of the crop sequence. In Traditional, maize follows other crops, which are mainly winter annual crops in Germany like autumn sown cereals or oilseed rape (Steinmann and Dobers, 2013; Stein and Steinmann, 2018).
Weeds in both transformation systems differ from those in Traditional but with an opposite direction in the species ordination and in the trait analysis. Conservational favors weeds that are dicots and support pollinators while Monoculture favors spring germinators and monocots and disfavors flowering weeds supporting pollinators.
Autumn sown crops explain the number of winter annual weeds loading on Conservational in the ordination. The species Myosotis arvensis is, thanks to its hairy structure, able to survive the use of the nonselective herbicide glyphosate better than other species (Koning et al., 2019). The RLQ analysis indicated a high seed weight as a trait allowing weeds to cope with Conservational. G. aparine and B. napus are probably mainly responsible for this result. When the soil is not mixed by plowing, seeds are not taken close to the surface for germination. Hence, bigger seeds allow successful emergence from a wider range of germination depth than in plowed systems. This confirms findings by Fried et al. (2012), who identified tillage depth as a primary influence on the seed weight of weeds. One is Conservational, meaning non-inversion tillage was practiced in 48% of the fields. This figure indicates a substantial increase compared to that from the years 2001–2009 (de Mol et al., 2015). Less inversion tillage provides various benefits: it saves energy and labor, lowering production costs while maintaining acceptable crop yield and quality (Morris et al., 2010). Less energy input reduces the carbon footprint (Krupnik et al., 2022). Soil biodiversity generally profits if the soil is not regularly inverted (Nabel et al., 2021). However, in Conservational, glyphosate use mainly replaces the traditional inversion of the soil to control weeds or to terminate subsidiary crops before sowing the crop (Antier et al., 2020).
Deviating from Traditional is stopping rotations and continuously cropping maize with little subsidiary crop (17%) (Monoculture). The demand for maize in Germany increased since its introduction in the 1960s due to its use for biogas and feed. Despite favoring certain weeds, cropping maize after maize is in the cool-temperate climate of Germany not yet limited by soilborne insects or diseases (Redwitz and Gerowitt, 2018). The data provided in our study show that the five most frequent weed species identified load on the system Monoculture. These species are quite common, three being late summer annuals (C. album, E. crus-galli, P. convolvulus). S. media is an all-year germinator. V. arvensis is classified as winter annual but has been reported to grow in maize fields for more than 20 years (Mehrtens et al., 2005). When the seedbed for maize is prepared in late spring, species germinating in autumn are killed; therefore, Monoculture favors spring germinators. Being a monocot weed with a graminoid growth habit is advantageous in a monocot crop like maize. The more often maize is cropped, the more advantageous these traits are. Our result confirms findings by Redwitz and Gerowitt (2018), who concluded that cropping maize more often favors both already common species and exceptionally well adapted to maize cropping like E. crus-galli.
To sum up, we demonstrated the effect of transforming management practices from Traditional maize cropping to Conservational or Monoculture on the weed community emerging in maize on both the species and the trait level. The information on management and environmental field data management conducted through interviews allowed to combine plant surveys with agronomical questions, as, e.g., Bürger et al. (2022) suggest.
Crop rotation and tillage were identified as drivers of influence on weed species and traits. Hence, agronomic practices must tackle these drivers to avoid the selection of weeds with traits adapted to maize. Crop rotation is a sharp sword, frequently confirmed to work, particularly when rotating summer annual and winter annual crops and those sown broadcast and in a row (Andreasen and Skovgaard, 2009; Weisberger et al., 2019). In case crop rotations cannot meet the demands of the farm in terms of feed for livestock or energy, at least a subsidiary crop should break the continuous flow of maize over time (Nichols et al., 2020; Wienberg and Gerowitt, 2023). Crop rotation is a must in reduced tillage systems (Jalli et al., 2021). If glyphosate is used, attention must be given to “winners weeds” in these systems. “Winner weeds” are dicots. Concerning the species profiting, we regard Monoculture as the riskier transformation system.
Both transformation systems face challenges not primarily resulting from weeds with adapted traits. The common agricultural policy of the European Union (EU) demands more crop diversification. A crop rotation is required on all farms of at least 10 hectares (European Commission, 2023). In Germany, crop diversification is designed so that, at the latest, the following crop must be rotated after 3 years. In addition, farmers must ensure subsidiary crops (Federal Ministry of Food and Agriculture, 2023). Hence, EU agricultural policies are operating against monocultures anyway. Reduced tillage relies to a certain extent on the nonselective herbicide glyphosate. The future of glyphosate in the EU agriculture is currently undecided. The joint EU pesticide registration as an administrative process will be decisive for reduced tillage systems. However, it is open to what extent reduced tillage can be maintained without glyphosate and what that would mean for weeds on the fields.
The given examples of transformation processes in arable land use express the importance of weed surveys on a geographically widespread and repeated timescale together with field management data. For this purpose, the “Arable Weeds and Management” database (AWME) was established (Bürger et al., 2020). Data collection like this enables to identify drivers of change in weed communities (Metcalfe et al., 2023). The data of this study will be submitted to the AWME to serve for future applications. Meeting global challenges like climate change and land use transformations will profit from such applications.
Data availability statement
The original contributions presented in the study are included in the article/Supplementary Material. Further inquiries can be directed to the corresponding author.
Author contributions
JB: Formal Analysis, Visualization, Writing – original draft, Writing – review & editing, Conceptualization, Validation. HP: Methodology, Writing – review & editing, Conceptualization, Formal Analysis. BG: Supervision, Writing – original draft, Conceptualization, Data curation, Funding acquisition, Project administration, Resources, Writing – review & editing.
Funding
The author(s) declare financial support was received for the research, authorship, and/or publication of this article. The project was supported logistically and financially by Syngenta Agro GmbH, HP was granted, University of Rostock provided technical assistance. The work of JB is funded by the German Federal Agency for Nature Conservation with a grant from the Federal Ministry for the Environment, Nature Conservation Nuclear Safety and Consumer Protection (FKZ 351984070).
Acknowledgments
We thank all farmers participating in the monitoring. Syngenta Agro GmbH assisted to get the contacts and in the field work; Dr. Christoph Krato organized the project within the company. The teams of Dr. Manfred Kayser, University of Göttingen, Germany, Prof. Joachim Kakau, School of Applied Science Osnabrück Germany and Prof. Verena Haberlah-Korr, School of Applied Science Soest, Germany collaborated in the field assessments. Laura Tamms assisted in the monitoring in 2018. Utilizing her native skills, we were assisted by Laura Schmidt reviewing the manuscript in terms of grammar and tone. We gratefully thank them all.
Conflict of interest
The authors declare that the research was conducted in the absence of any commercial or financial relationships that could be construed as a potential conflict of interest.
The authors declare that this study received funding from Syngenta Agro GmbH. The funder had the following involvement in the study: data collection.
Publisher’s note
All claims expressed in this article are solely those of the authors and do not necessarily represent those of their affiliated organizations, or those of the publisher, the editors and the reviewers. Any product that may be evaluated in this article, or claim that may be made by its manufacturer, is not guaranteed or endorsed by the publisher.
Supplementary material
The Supplementary Material for this article can be found online at: https://www.frontiersin.org/articles/10.3389/fagro.2023.1284887/full#supplementary-material
Supplementary Table A1 | The functional traits included in the analysis.
Supplementary Table A2 | The functional traits for the 31 weeds included in the analysis.
Footnotes
- ^ LEDA: https://uol.de/en/landeco/research/leda.
- ^ BioFlor: https://www.ufz.de/index.php?de=38567.
- ^ International Herbicide-Resistant Weed Database: https://www.weedscience.org/Home.aspx.
References
Andreasen C., Skovgaard I. M. (2009). Crop and soil factors of importance for the distribution of plant species on arable fields in Denmark. Agriculture Ecosyst. Environ. 133, 61–67. doi: 10.1016/j.agee.2009.05.003
Antier C., Kudsk P., Reboud X., Ulber L., Baret P. V., Messéan A. (2020). Glyphosate use in the European agricultural sector and a framework for its further monitoring. Sustainability 12, 5682. doi: 10.3390/su12145682
Bàrberi P., Bocci G., Carlesi S., Armengot L., Blanco-Moreno J. M., Sans F. X. (2018). Linking species traits to agroecosystem services: a functional analysis of weed communities. Weed Res. 58, 76–88. doi: 10.1111/wre.12283
Bourgeois B., Munoz F., Fried G., Mahaut L., Armengot L., Denelle P., et al. (2019). What makes a weed a weed? A large-scale evaluation of arable weeds through a functional lens. Am. J. Bot. 106, 90–100. doi: 10.1002/ajb2.1213
Bürger J., Küzmič F., Šilc U., Jansen F., Bergmeier E., Chytrý M., et al. (2022). Two sides of one medal: Arable weed vegetation of Europe in phytosociological data compared to agronomical weed surveys. Appl. Vegetation Sci. 25, e12460. doi: 10.1111/avsc.12460
Bürger J., Metcalfe H., Redwitz C., Cirujeda Ranzenberger A., Fogliatto S., Freid G.. (2020). Arable weeds and management in Europe. Available at: http://hdl.handle.net/10532/5203
Carr P., Gramig G., Liebig M. (2013). Impacts of organic zero tillage systems on crops, weeds, and soil quality. Sustainability 5, 3172–3201. doi: 10.3390/su5073172
Carr P. M., Mäder P., Creamer N. G., Beeby J. S. (2012). Editorial: Overview and comparison of conservation tillage practices and organic farming in Europe and North America. Renew. Agric. Food Syst. 27, 2–6. doi: 10.1017/S1742170511000536
Chytrý M., Hennekens S. M., Jiménez-Alfaro B., Knollová I., Dengler J., Jansen F., et al. (2016). European Vegetation Archive (EVA): an integrated database of European vegetation plots. Appl. Vegetation Sci. 19, 173–180. doi: 10.1111/avsc.12191
de Mol F., Redwitz C., Gerowitt B. (2015). Weed species composition of maize fields in Germany is influenced by site and crop sequence. Weed Res. 55, 574–585. doi: 10.1111/wre.12169
Dolédec S., Chessel D., ter Braak C. J. F., Champely S. (1996). Matching species traits to environmental variables: a new three-table ordination method. Environ. Ecol. Stat. 3, 143–166. doi: 10.1007/BF02427859
Dray S., Choler P., Dolédec S., Peres-Neto P. R., Thuiller W., Pavoine S., et al. (2014). Combining the fourth-corner and the RLQ methods for assessing trait responses to environmental variation. Ecology 95, 14–21. doi: 10.1890/13-0196.1
Dray S., Dufour A. B. (2007). The ade4 package: implementing the duality diagram for ecologists. J. Stat. Soft. (American Statistical Association), 22 (4). doi: 10.18637/jss.v022.i04
Dunnington D. (2023) prettymapr: scale bar, north arrow, and pretty margins in R. Available at: https://cran.r-project.org/web/packages/prettymapr/index.html (Accessed October 23, 2023).
European Commission (2023) Key reforms in the new CAP. Available at: https://agriculture.ec.europa.eu/common-agricultural-policy/cap-overview/cap-2023-27/key-reforms-new-cap_en (Accessed October 24, 2023).
Federal Ministry of Food and Agriculture (2023) Publikationen - Umsetzung der Gemeinsamen Agrarpolitik der Europäischen Union 2023 in Deutschland. Available at: https://www.bmel.de/SharedDocs/Downloads/DE/Broschueren/gap-2023.html (Accessed October 24, 2023).
Feledyn-Szewczyk B., Smagacz J., Kwiatkowski C. A., Harasim E., Woźniak A. (2020). Weed flora and soil seed bank composition as affected by tillage system in three-year crop rotation. Agriculture 10, 186. doi: 10.3390/agriculture10050186
Fitter A. H., Peat H. J. (1994). The ecological flora database. J. Ecol. 82, 415. doi: 10.2307/2261309
Fried G., Kazakou E., Gaba S. (2012). Trajectories of weed communities explained by traits associated with species’ response to management practices. Agriculture Ecosyst. Environ. 158, 147–155. doi: 10.1016/j.agee.2012.06.005
Fried G., Norton L. R., Reboud X. (2008). Environmental and management factors determining weed species composition and diversity in France. Agriculture Ecosyst. Environ. 128, 68–76. doi: 10.1016/j.agee.2008.05.003
Grime J. P., Thompson K., Hunt R., Hodgson J. G., Cornelissen J. H. C., Rorison I. H., et al. (1997). Integrated screening validates primary axes of specialisation in plants. Oikos 79, 259. doi: 10.2307/3546011
Grundy A. C., Phelps K., Reader R. J., Burston S. (2000). Modelling the germination of Stellaria media using the concept of hydrothermal time. New Phytol. 148, 433–444. doi: 10.1046/j.1469-8137.2000.00778.x
Hanzlik K., Gerowitt B. (2016). Methods to conduct and analyse weed surveys in arable farming: a review. Agron. Sustain. Dev. 36, 1–18. doi: 10.1007/s13593-015-0345-7
Hawes C., Haughton A. J., Bohan D. A., Squire G. R. (2009). Functional approaches for assessing plant and invertebrate abundance patterns in arable systems. Basic Appl. Ecol. 10, 34–42. doi: 10.1016/j.baae.2007.11.007
Hofmeijer M. A. J., Melander B., Salonen J., Lundkvist A., Zarina L., Gerowitt B. (2021). Weed species trait selection as shaped by region and crop diversity in organically managed spring cereals. Agriculture 11, 433. doi: 10.3390/agriculture11050433
Jalli M., Huusela E., Jalli H., Kauppi K., Niemi M., Himanen S., et al. (2021). Effects of crop rotation on spring wheat yield and pest occurrence in different tillage systems: A multi-year experiment in finnish growing conditions. Front. Sustain. Food Syst. 5. doi: 10.3389/fsufs.2021.647335
Kassambara A., Mundt F. (2020). Extract and Visualize the Results of Multivariate Data Analyses [R package factoextra version 1.0.7]: Comprehensive R Archive Network (CRAN). Available at: https://cran.r-project.org/web/packages/factoextra/index.html.
Kleyer M., Bekker R. M., Knevel I. C., Bakker J. P., Thompson K., Sonnenschein M., et al. (2008). The LEDA Traitbase: A database of life-history traits of Northwest European flora. J. Ecol. 96, 1266–1274. https://uol.de/en/landeco/research/leda. doi: 10.1111/j.1365-2745.2008.01430.x
Koning L. A., de Mol F., Gerowitt B. (2019). Effects of management by glyphosate or tillage on the weed vegetation in a field experiment. Soil Tillage Res. 186, 79–86. doi: 10.1016/j.still.2018.10.012
Krupnik T. J., Hossain M. K., Timsina J., Gathala M. K., Sapkota T. B., Yasmin S., et al. (2022). Adapted conservation agriculture practices can increase energy productivity and lower yield-scaled greenhouse gas emissions in coastal Bangladesh. Front. Agron. 4. doi: 10.3389/fagro.2022.829737
Lascoux M., Glémin S., Savolainen O. (2005-2010). “Local adaptation in plants,” in Encyclopedia of life sciences (London, New York: John Wiley & Sons), 1–7.
Manthey M. (2003). Vegetationsökologie der Äcker und Ackerbrachen - Mecklenburg-Vorpommerns (Stuttgart: Schweizerbart’sche Verlagsbuchhandlung).
Mehrtens J., Schulte M., Hurle K. (2005). Unkrautflora in Mais Ergebnisse eines Monitorings in Deutschland: Weed flora in maize Results of a monitoring in Germany. Gesunde Pflanzen 57, 206–218. doi: 10.1007/s10343-005-0097-4
Metcalfe H., Bürger J., Redwitz C., Cirujeda A., Fogliatto S., Dostatny D. F., et al. (2023). The utility of the ‘Arable Weeds and Management in Europe’ database: Challenges and opportunities of combining weed survey data at a European scale. Weed Res. 63, 1–11. doi: 10.1111/wre.12562
Morris N. L., Miller P., Orson J. H., Froud-Williams R. J. (2010). The adoption of non-inversion tillage systems in the United Kingdom and the agronomic impact on soil, crops and the environment—A review. Soil Tillage Res. 108, 1–15. doi: 10.1016/j.still.2010.03.004
Nabel M., Selig C., Gundlach J., von der Decken H., Klein M. (2021). Biodiversity in agricultural used soils: Threats and options for its conservation in Germany and Europe. Soil ORG 93, 1–11-1–11. doi: 10.25674/so93iss1pp1
Nguyen H. T. X., Liebman M. (2022). Impact of cropping system diversification on vegetative and reproductive characteristics of waterhemp (Amaranthus tuberculatus). Front. Agron. 4. doi: 10.3389/fagro.2022.811359
Nichols V., English L., Carlson S., Gailans S., Liebman M. (2020). Effects of long-term cover cropping on weed seedbanks. Front. Agron. 2. doi: 10.3389/fagro.2020.591091
Oksanen J., Simpson G. L., Blanchet F. G., Kindt R., Legendre P., Minchin P. R. (2022). Community Ecology Package [R package vegan version 2.6-4]: Comprehensive R Archive Network (CRAN). Available at: https://cran.r-project.org/web/packages/vegan/index.html.
Pebesma E., Bivand R., Rowlingson B., Gomez-Rubio V., Hijmans R., Sumner M. (2023). Classes and Methods for Spatial Data [R package sp version 2.1-1]: Comprehensive R Archive Network (CRAN). Available at: https://cran.r-project.org/web/packages/sp/index.html.
Pinke G., Giczi Z., Vona V., Dunai É., Vámos O., Kulmány I., et al. (2022). Weed Composition in Hungarian Phacelia (Phacelia tanacetifolia Benth.) Seed Production: Could Tine Harrow Take over Chemical Management? Agronomy 12, 891. doi: 10.3390/agronomy12040891
Pinke G., Gunton R. M. (2014). Refining rare weed trait syndromes along arable intensification gradients. J. Veg Sci. 25, 978–989. doi: 10.1111/jvs.12151
Pinke G., Karácsony P., Czúcz B., Botta-Dukát Z., Lengyel A. (2012). The influence of environment, management and site context on species composition of summer arable weed vegetation in Hungary. Appl. Veg Sci. 15, 136–144. doi: 10.1111/j.1654-109X.2011.01158.x
R Core Team (2022). R: A language and environment for statistical computing (Vienna, Austria: R Foundation for Statistical Computing). Available at: https://www.R-project.org/.
Redwitz C., Gerowitt B. (2018). Maize-dominated crop sequences in northern Germany: Reaction of the weed species communities. Appl. Veg. Sci 21, 431–441. doi: 10.1111/avsc.12384
Robert J. H. (2023). Geographic Data Analysis and Modeling [R package raster version 3.6-26]: Comprehensive R Archive Network (CRAN). Available at: https://cran.r-project.org/web/packages/raster/index.html.
Rousseeuw P., Struyf A., Hubert M. (2022). "Finding groups in data": Cluster analysis extended Rousseeuw et al. [R package cluster version 2.1.4]: Comprehensive R Archive Network (CRAN). Available at: https://cran.r-project.org/web/packages/cluster/index.html.
Salaudeen M. T., Daniya E., Olaniyi O. M., Folorunso T. A., Bala J. A., Abdullahi I. M., et al. (2022). Phytosociological survey of weeds in irrigated maize fields in a Southern Guinea Savanna of Nigeria. Front. Agron. 4. doi: 10.3389/fagro.2022.985067
Salonen J. (1993). Weed infestation and factors affecting weed incidence in spring cereals in Finland - a multivariate approach. AFSci 2, 525–536. doi: 10.23986/afsci.72678
Salonen J., Hyvönen T., Kaseva J., Jalli H. (2013). Impact of changed cropping practices on weed occurrence in spring cereals in Finland - a comparison of surveys in 1997-1999 and 2007-2009. Weed Res. 53, 110–120. doi: 10.1111/wre.12004
Statista (2023a) Anbaufläche von Körnermais in Deutschland bis 2022 (Statista). Available at: https://de.statista.com/statistik/daten/studie/28893/umfrage/anbauflaeche-von-koernermais-in-deutschland-seit-1960/ (Accessed July 27, 2023).
Statista (2023b) Landwirtschaftliche Nutzfläche in Deutschland bis 2022 (Statista). Available at: https://de.statista.com/statistik/daten/studie/206250/umfrage/landwirtschaftliche-nutzflaeche-in-deutschland/ (Accessed July 27, 2023).
Statista (2023c) Silomais: Anbaufläche bis 2022 (Statista). Available at: https://de.statista.com/statistik/daten/studie/1389823/umfrage/anbauflaeche-von-silomais-in-deutschland/ (Accessed July 27, 2023).
Stein S., Steinmann H.-H. (2018). Identifying crop rotation practice by the typification of crop sequence patterns for arable farming systems – A case study from Central Europe. Eur. J. Agron. 92, 30–40. doi: 10.1016/j.eja.2017.09.010
Steinmann H.-H., Dobers E. S. (2013). Spatio-temporal analysis of crop rotations and crop sequence patterns in Northern Germany: potential implications on plant health and crop protection. J. Plant Dis. Prot 120, 85–94. doi: 10.1007/BF03356458
Storkey J., Moss S. R., Cussans J. W. (2010). Using assembly theory to explain changes in a weed flora in response to agricultural intensification. Weed Sci. 58, 39–46. doi: 10.1614/WS-09-096.1
Travlos I. S., Cheimona N., Roussis I., Bilalis D. J. (2018). Weed-species abundance and diversity indices in relation to tillage systems and fertilization. Front. Environ. Sci. 6. doi: 10.3389/fenvs.2018.00011
Weisberger D., Nichols V., Liebman M. (2019). Does diversifying crop rotations suppress weeds? A meta-analysis. PloS One 14, e0219847. doi: 10.1371/journal.pone.0219847
Keywords: weed, management, disturbance, species, traits, maize, tillage system, Germany
Citation: Bensch J, Pannwitt H and Gerowitt B (2023) Traits of weed species present in maize respond to tillage and cropping systems. Front. Agron. 5:1284887. doi: 10.3389/fagro.2023.1284887
Received: 29 August 2023; Accepted: 13 November 2023;
Published: 04 December 2023.
Edited by:
Leslie A. Weston, Charles Sturt University, AustraliaReviewed by:
Ahmet Uludag, Çanakkale Onsekiz Mart University, TürkiyeAndrea Basche, University of Nebraska System, United States
Copyright © 2023 Bensch, Pannwitt and Gerowitt. This is an open-access article distributed under the terms of the Creative Commons Attribution License (CC BY). The use, distribution or reproduction in other forums is permitted, provided the original author(s) and the copyright owner(s) are credited and that the original publication in this journal is cited, in accordance with accepted academic practice. No use, distribution or reproduction is permitted which does not comply with these terms.
*Correspondence: Johanna Bensch, am9oYW5uYS5iZW5zY2hAdW5pLXJvc3RvY2suZGU=
†Present address: Heike Pannwitt, Julius Kühn-Institute (JKI), Federal Research Centre for Cultivated Plants, Institute for Biosafety in Plant Biotechnology, Quedlinburg, Germany