- Department of Agriculture and Forest Sciences (DAFNE), University of Tuscia, Viterbo, Italy
The wheat crop is one of the most cultivated and consumed commodities all over the world. Fungal diseases are of particular concern for wheat cultivation since they cause great losses and reduced quality, and also for the accumulation of toxin compounds into the final product. In this scenario, optimal disease management strategies are a key point to boosting food production and sustainability in agriculture. Innovative and point-of-care diagnostic technologies represent a powerful weapon for early detection of fungal pathogens and preventively counteract diseases on wheat with the aim to drastically reduce the fungicides as inputs. Indeed, in-field diagnostics devices are fast, sensitive, and ready-to-use technologies able to promptly detect a low inoculum concentration even at the pre-symptomatic stage of the disease. Promising isothermal molecular and phenomics-based methods have been developed to detect wheat fungal pathogens directly in the field. Such technologies could be potentially coupled to directly detect the presence of a certain pathogen and indirectly disclose the plant-pathogen interactions since spectral-based methodologies detect host perturbations following the infection. The present review reports the main in-field isothermal molecular-based and phenomics-based detection technologies for fungal pathogens in wheat discussing their advantages, disadvantages, and potential applications in the near future.
1 Introduction
Wheat represents 20% of the total human food calories, and it is extensively grown in all temperate world regions. Furthermore, it is human beings’ most important source of protein (Gustafson et al., 2009; Khan et al., 2020; Ma et al., 2020). In the future, the world wheat supply will become even more critical, as the demand will exponentially grow, while the current agricultural systems are responsible for land degradation (Foley et al., 2011). Thus, the world crop production needs to double by 2050 (Ray et al., 2013).
Plant diseases are responsible for major production and economic losses in agriculture and forestry, which are also attributed to non-native plant pathogens (Pimentel et al., 2005). Biotic infestations result in symptoms appearing on different parts of the plants, causing tissue damage and a significant agronomic and economic impact (López et al., 2003). In a recent report developed by the Food and Agriculture Organization (FAO), about 20% to 40% of the global crop production has been lost because of biotic stresses. Particularly, plant diseases cost approximately US$220 billion per year, and it is estimated that such losses deprived more than 800 million people of adequate consumable food (FAO, 2021; Mitra, 2021). Referring to wheat, about 10% of estimated yield losses were due to fungal pathogens, whereas viral and bacterial diseases are usually less impacting (Oerke, 2006; Aboukhaddour et al., 2020; Simón et al., 2021). Thus, it is clear to the scientific community that an early disease detection system based on the fundamental understanding of host–pathogen interactions can aid in decreasing such losses, further prevent the spread of diseases, and enhance the total agricultural yield (Sankaran et al., 2010; Mitra, 2021). For such reasons, the success of wheat improvement programs to meet future demands will require the complementation of traditional and modern breeding programs (Gustafson et al., 2009) with innovative, sustainable, fast, and sensitive management and detection strategies to promptly control wheat diseases.
The most employed in-laboratory detection techniques of plant pathogens are the enzyme-linked immunosorbent assay (ELISA), which recognizes an antigen from the pathogen, and polymerase chain reaction (PCR), amplifying specific DNA sequences of the plant pathogen (Prithiviraj et al., 2004; Saponari et al., 2008; Yvon et al., 2009; Sankaran et al., 2010; Martinelli et al., 2015). Although these are well-established techniques, there is an increasing demand for fast, sensitive, effective, and, possibly, in-field methods for the detection of plant diseases (Figure 1) (Schaad et al., 2002; Schaad et al., 2003). Detection techniques can be generally classified into two main groups: direct and indirect methods. Direct methods comprise techniques able to directly detect a certain structure from the plant pathogen, such as ELISA- or PCR-based assays. Indirect methods are based on the detection of host responses (perturbation of photosynthesis, change in chlorophyll content, and temperature increase due to stomatal closure) to a certain biotic stress, thus comprising volatile, proximal, and remote sensing techniques (Sankaran et al., 2010). Although molecular and serological methods have revolutionized plant disease detection, they are sometimes not very reliable, sensitive, and accurate and, especially at the asymptomatic stage, are labor-intensive and time-consuming and do not always offer the possibility of on-site detection. However, sensor-based techniques can deliver much more high-throughput results, by allowing high spatialization of data acquisition, and can effectively detect early the infections in the field. For such reasons, coupling DNA-based molecular methods and sensor-based techniques will help plant disease management through an effective, reliable, sensitive, and rapid preliminary identification of latent infections (Li et al., 2014; Fahlgren et al., 2015; Martinelli et al., 2015; Mahlein, 2016; Mahlein et al., 2018).
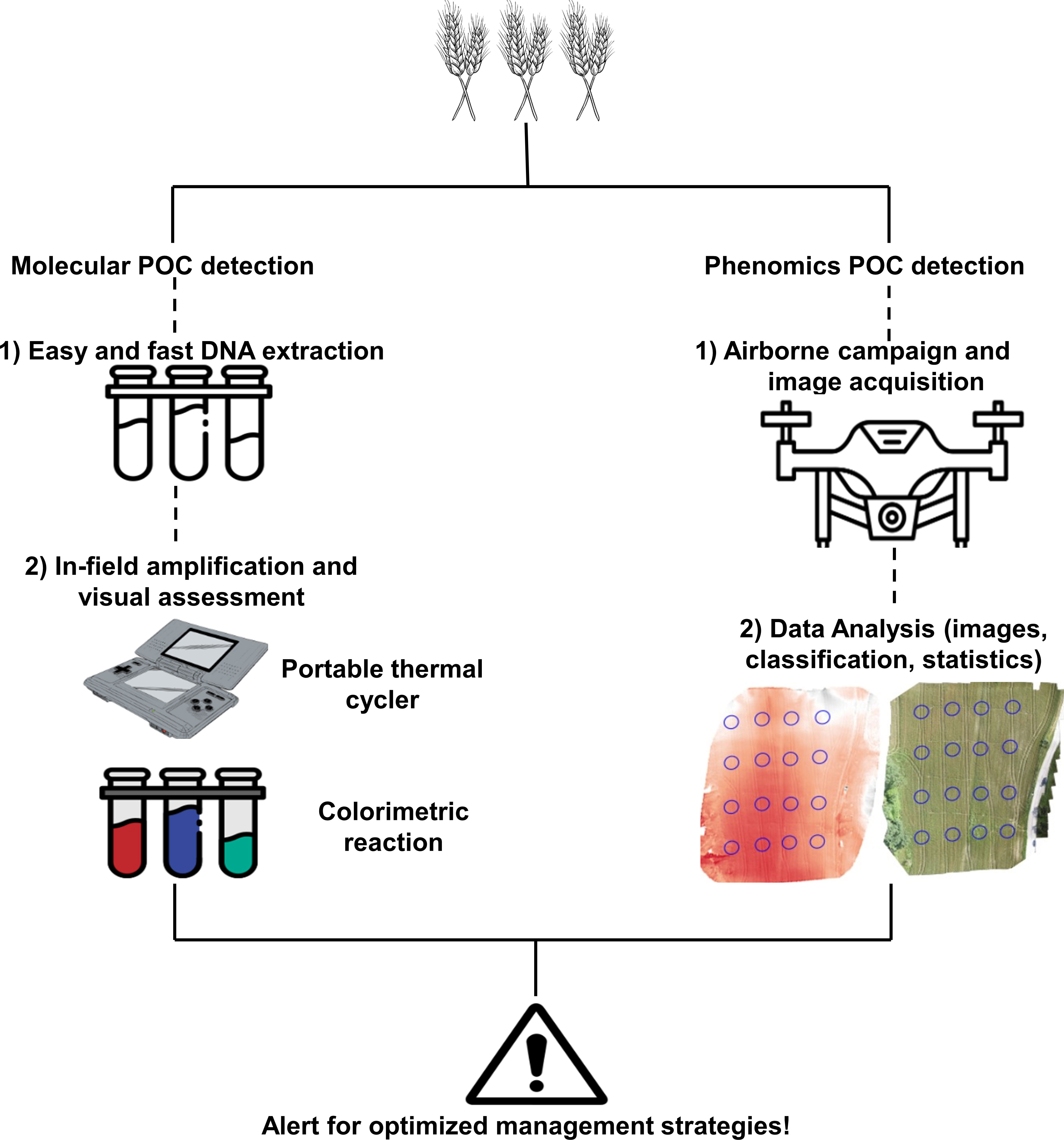
Figure 1 Graphical representation of an ideal workflow for point-of-care (POC) detection of wheat fungal pathogens. Molecular POC detection starts with an easy and fast DNA extraction by using an in-field protocol, such as simple maceration of plant materials in saline buffer. The crude extract is directly subjected to an isothermal amplification, and results are visualized on a portable thermal cycler or by colorimetric reactions. Molecular POC detection techniques are fast, sensitive, and specific and can give results in real-time, but they require precise expertise for primer design and are not extremely high-throughput since the number of processed samples is determined by the characteristics of the thermal cycler in use. Phenomics POC detection is based on the acquisition of images by a sensor mounted on an unmanned aerial vehicle (UAV) or satellite-based to boost their high-throughput capabilities since it is possible to scan a field in a few minutes. Sensors acquire images and collect a huge amount of data that need to be further analyzed, classified, and validated. Phenomics-based techniques are high-throughput and fast during image and data acquisition, but data analysis is not real-time and often requires specific expertise for data interpretation; moreover, they can be expensive, weather-dependent, and not specific for the detection of a certain stress. A prompt POC detection technique is extremely useful to optimize disease management strategies.
2 Most impacting fungal wheat diseases
Before introducing the main and innovative detection techniques, the following section will give a general overview of the most impacting fungal wheat diseases (Figure 2) against which researchers have focused their efforts on developing in-field diagnostic methodologies.
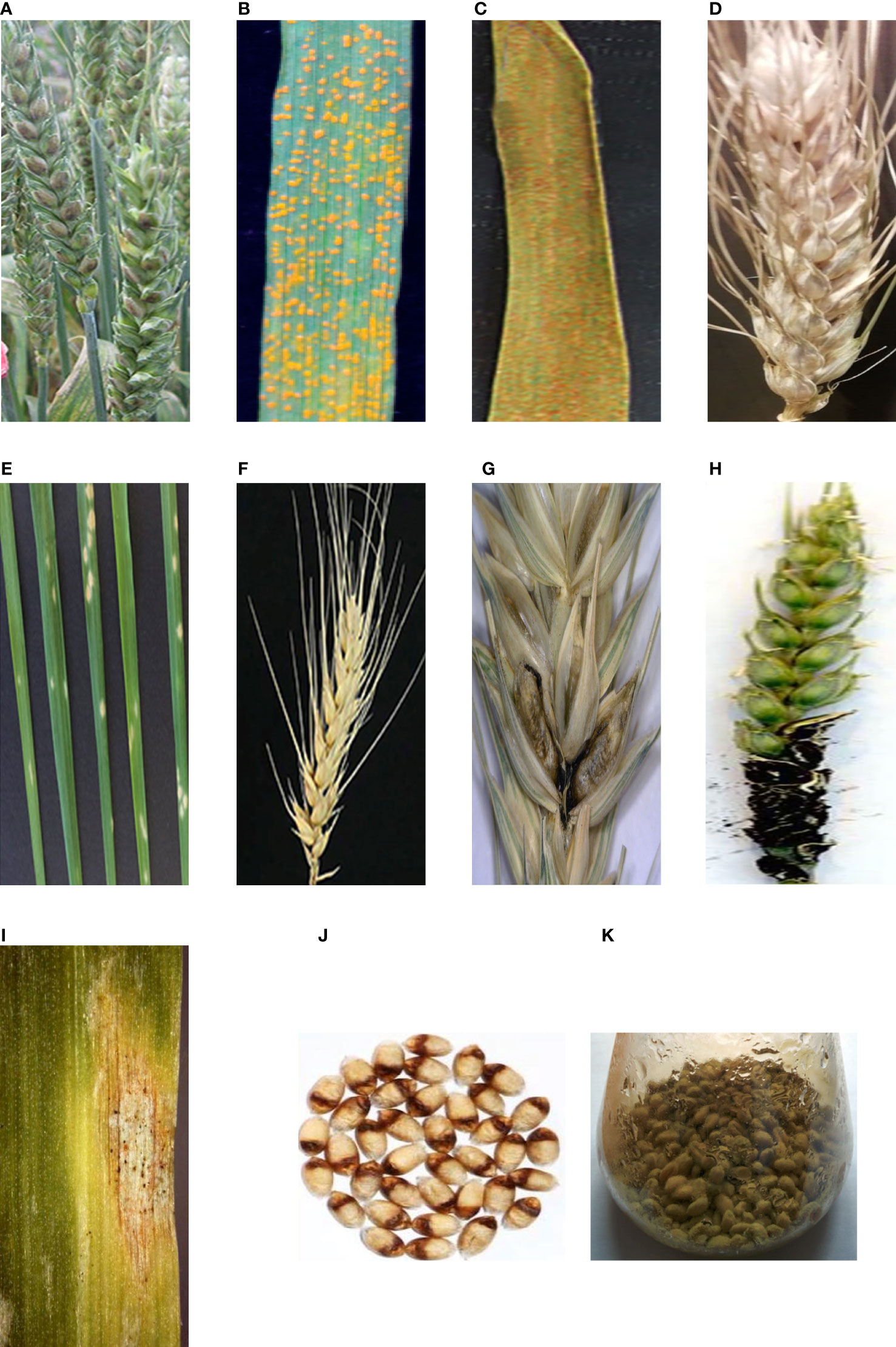
Figure 2 Representative symptoms of the main fungal diseases affecting wheat. (A) Stem or black rust by Puccinia graminis f. sp. tritici (Huerta-Espino et al., 2020). (B) Stripe or yellow rust by Puccinia striiformis f. sp. tritici (Porras et al., 2022). (C) Leaf or brown rust by Puccinia triticina (Huerta-Espino et al., 2020). (D) Fusarium head blight by Fusarium graminearum (Francesconi et al., 2021). (E) Powdery mildew by Blumeria graminis f. sp. tritici (Twamley et al., 2019). (F) Blast by Magnaporthe oryzae Triticum pathotype (Fernández-Campos et al., 2021). (G) Karnal bunt by Tilletia indica (Bishnoi et al., 2020). (H) Loose smut by Ustilago tritici (Thambugala et al., 2020). (I) Leaf blotch by Zymoseptoria tritici (Brennan et al., 2020). (J) Black point by Bipolaris sorokiniana (Li et al., 2020). (K) Molds by Aspergillus spp. (Dzhavakhiya et al., 2016).
2.1 Rusts
Rust fungi are obligate parasites characterized by high genetic variability since several races have been reported (Kolmer, 2013). They are of particular economic concern since global annual losses range between US$4.3 and 5.0 billion (Figueroa et al., 2018). In wheat, three rust diseases are reported (Ash, 1996). Three species infect wheat, Puccinia graminis f. sp. tritici, causing stem or black rust; Puccinia striiformis f. sp. tritici, causing stripe or yellow rust; and Puccinia triticina, causing leaf or brown rust. Typical symptoms appear as masses of spores on leaves, stems, and glumes, causing yield losses associated with a reduction in grain size (Kolmer, 2005; Leonard and Szabo, 2005; Huerta-Espino et al., 2011; Sabrol and Kumar, 2013; Khushboo et al., 2021).
2.2 Fusarium head blight
Fusarium head blight (FHB) stands out as the most devastating wheat disease owing to a lack of resistant varieties, great yield loss (10%–70%), grain quality reduction, and health problems because of mycotoxin accumulation in food and feed. FHB occurs in the majority of the wheat-growing regions including Asia, North America, South America, and Europe (McMullen et al., 2012; Khan et al., 2020; Ma et al., 2020; Mielniczuk and Skwaryło-Bednarz, 2020). Fusarium graminearum Schwabe is considered the most aggressive species; it is graded among the four crucial plant fungal pathogens (Dean et al., 2012) and was thought to be a single cosmopolitan species (Ma et al., 2020). The bleaching of infected spikelets inhibits the development of kernels, which causes grain number reduction in the spikes. The grains may be subjected to the accumulation of trichothecene mycotoxins produced by causal agents, such as deoxynivalenol (DON), making them unsuitable for humans and animals (Gilbert and Tekauz, 2000; McCallum and Tekauz, 2002; Jansen et al., 2005; Beyer et al., 2006; Vaughan et al., 2016; Gunupuru et al., 2017).
2.3 Powdery mildew
Powdery mildew is caused by Blumeria graminis f. sp. tritici, which has been classified as the sixth most dangerous out of 10 fungal pathogens in wheat (Dean et al., 2012). Powdery mildew can occur during the entire wheat seasonal year in all the wheat-growing regions, with crop production losses recorded from 34% to 62% (Alam et al., 2011; Mehta, 2014). Typical symptoms are visible mycelium and conidia as powder-like colonies on leaves and stem, which may be followed by black survival sexual structures, chasmothecia (formerly cleistothecia), which may overwinter and infect new host plants in the following growing season by releasing ascospores (Glawe, 2008).
2.4 Wheat blast
Wheat blast is a devastating disease caused by the ascomycetous fungus Magnaporthe oryzae Triticum pathotype (MoT) (Couch and Kohn, 2002; Zhang et al., 2016; Cruz and Valent, 2017). The disease was confined to South America since its first emergence in Brazil in 1985, where it is a serious concern to 3 million ha of wheat cultivated area (Kohli et al., 2011; Islam et al., 2020). In 2016, MoT has been discovered for the first time in Bangladesh and India, where it devastated more than 15,000 ha of wheat, causing yield losses of up to 100% (Islam et al., 2016; Malaker et al., 2016; Figueroa et al., 2018). Recently, a MoT strain was also detected and characterized in Zambia ( (Tembo et al., 2020). The most distinguishable symptoms are observed in the head, even though they can be easily confused with FHB symptoms, since the spikes become partially or fully bleached, causing shriveled grains, low test weight, and accumulation of poor nutrients (Goulart et al., 2007; Islam et al., 2016; Surovy et al., 2020). The affected grains are not suitable for human consumption; thus, they can be discarded during the post-harvest process (Urashima et al., 2009; Surovy et al., 2020).
2.5 Karnal bunt
Karnal bunt is caused by the basidiomycetes fungus Tilletia indica, and it was firstly reported in India in 1931. Nowadays, the disease is present in India, Iran, Iraq, Mexico, Nepal, Pakistan, South Africa, and the United States (Jones, 2007; Emebiri et al., 2019a; Emebiri et al., 2019b; Gurjar et al., 2019; Singh et al., 2020). Moreover, it is an internationally recognized quarantine disease (classified as A1 by the European and Mediterranean Plant Protection Organization (EPPO)); thus, restrictions prevent the international trading of wheat grains from the affected regions (Carris et al., 2006; Figueroa et al., 2018; Pandey et al., 2019; Bishnoi et al., 2020). After the infection, the grains become a black powdery mass of teliospores, which secrete trimethylamine, thereby negatively impacting the quality and marketability of the kernels by altering the chemical composition and rendering them inedible (Kumari et al., 2020; Iquebal et al., 2021; Kumar et al., 2021). Yield losses are of particular concern, since, in addition to the unmarketable kernels, costs are also associated with control measures, quarantine, and regulatory and monitoring restrictions (Kumar et al., 2021).
2.6 Loose smut
Loose smut, a wheat fungal disease present worldwide, caused by the fungal pathogen Ustilago tritici, occurs in wheat during early and mid-anthesis. The pathogen infects the spikes, leading to the damage of head tissues, with the exception of the rachis, and producing a black powder mass of smut spores (Abrahim, 2019). Despite that loose smut is not considered a devastating disease, it can cause moderate reductions in both yield and quality of kernels. A disease incidence of approximately 1%–2% can reduce profit for the farmers by approximately 5%–20% (Abrahim, 2019; Chaudhary and Pujari, 2021).
2.7 Leaf blotch
Leaf blotch is caused by the hemibiotrophic pathogen Zymoseptoria tritici (formerly known as Mycosphaerella graminicola or Septoria tritici), which represents the primary leaf disease of wheat in temperate regions (Duba et al., 2018; Figueroa et al., 2018; Brennan et al., 2019). Nowadays, leaf blotch is the primary threat to wheat production, and its estimated annual losses in Europe are about 280–1200 million euros, including direct losses and control costs (Fones and Gurr, 2015). In particular, high fungicide usage poses a strong selection on pathogen populations (McDonald and Stukenbrock, 2016), leading to a rapid evolution of fungal resistance to the major classes of fungicides (Dooley et al., 2016; Hayes et al., 2016; Corkley et al., 2022).
2.8 Diseases caused by Bipolaris sorokiniana
Bipolaris sorokiniana (teleomorph Cochliobolus sativus) is one of the major biotic constraints to wheat production since losses can reach up to 50% (Sharma and Duveiller, 2006; Figueroa et al., 2018). B. sorokiniana causes spot blotch, black point, and root rot (Al-Sadi, 2021). Spot blotch can cause significant losses (15%–43%) in warm areas (Gupta et al., 2018; Al-Sadi, 2021; Alkan et al., 2022). Symptoms situated on the leaf, sheath, node, and glumes appear as small light brown and oval lesions. The affected leaves are affected by chlorophyll deficiency and eventually die. Spikes and grains could also be affected, causing black points (Gupta et al., 2018). Black point is caused at the kernel site, in association often with other fungal pathogens such as Alternaria, Fusarium, and Penicillium spp., causing a brown-to-black tip in the embryo. The disease negatively affects the quality and market value of grains, with an accumulation of fungal toxins in the kernels, and favors seedling blight, root rot, and different diseases. Moreover, seed germination, seedling emergence, total photosynthetic area, and normal growth of plants can be drastically reduced (Al-Sadi and Deadman, 2010; Li et al., 2019; Somani et al., 2019; Al-Sadi, 2021). Seeds infected by black points can favor the development of root rot and crown rot. Root rot and crown rot of cereals are characterized by necrotic dark brown lesions on the roots and crown (Al-Sadi and Deadman, 2010; Qostal et al., 2019). The disease is caused by B. sorokiniana and other fungal pathogens, such as Fusarium pseudograminearum, Fusarium culmorum, Microdochium nivale, Pythium spp., and Rhizoctonia cerealis (Moya-Elizondo et al., 2011; Saremi and Saremi, 2013; Kazan and Gardiner, 2018; Xu et al., 2018; White et al., 2019). Yield and quality are reduced in infected plants because of the reduced number of tillers and the number and size of kernels (Al-Sadi, 2021).
2.9 Molds by Aspergillus spp.
Aspergillus is considered a unique fungal genus since there are more than 180 accepted anamorphic species (Sheikh-Ali et al., 2014; Khan et al., 2021; Magallanes López and Simsek, 2021). High temperature, high humidity, and incorrect food storage conditions enhance Aspergillus growth and mycotoxin development, such as aflatoxins (AFs) (Pankaj et al., 2018; Frisvad et al., 2019). The FAO estimated that approximately 25% of the world’s cereal grains are contaminated by AFs (FAO, 2004), since AF contamination may occur during pre- or post-harvest stages (Shuaib et al., 2010; Iimura et al., 2017). Warm temperature and high humidity conditions favor fungal growth in the field and during storage, contributing to AF accumulation, which is responsible for substantial commercial losses (Sheikh-Ali et al., 2014; Khan et al., 2021). Importantly, AFs are among the most toxic compounds, since they are considered mutagenic, teratogenic, genotoxic, and carcinogenic (classified into group A1 by the International Agency for Research on Cancer), causing severe diseases in animals and humans (Giovati et al., 2015; Monson et al., 2016; Yuan et al., 2016b; Peles et al., 2019).
3 Molecular-based point-of-care detection methods
The desirable characteristics of plant pathogen diagnostic assays are specificity, sensitivity, reproducibility, quickness, affordable costs, and, possibly, high-throughput multiplex detection capability (Lau and Botella, 2017). Point of care (POC) describes any treatments or technology given at the “site-of-need”. POC diagnostic methods are essential tools to reduce the agricultural yield losses caused by biotic stresses (Lau and Botella, 2017; Cassedy et al., 2020). Thus, POC assays that do not require sophisticated and specific laboratory equipment can be rapidly and cheaply performed in the field and, possibly, show multiplexing capability, which are in high demand (Yager et al., 2006). For such purpose, isothermal DNA amplification methods overcome the main limitation of PCR and real-time PCR, since they can obviate the need for a thermal cycler (Gill and Ghaemi, 2008; Craw and Balachandran, 2012). Such characteristics depend not only on the detection methodology itself but also on sampling and further nucleic acid extraction procedures. Fungal spores are easily transported by wind and water splashes; thus, the airborne inoculum can be captured by several types of spore samplers, such as passive or active ones. Passive traps are based on spore deposition on adhesive surfaces, such as slides or filters. These samplers have the advantage of low-cost equipment even though they do not allow spore counting. However, active traps allow the calculation of spore concentrations by using a scanning electron microscope. They mechanically collect the airborne inoculum by using a rotor or a pump, but since they require the most sophisticated equipment, costs are less affordable (Van der Heyden et al., 2021). The captured airborne inoculum can be further processed for DNA extraction. More interestingly, optimized protocols for sampling fresh plant material and DNA/RNA extraction are particularly requested for efficient amplification. Soft leaves can be homogenized or macerated in an appropriate buffer without mechanical pre-treatments, and this is particularly suitable for in-field applications. After that, extraction and purification of nucleic acids require cell lysis and remotion of all the metabolites that can negatively affect downstream applications. Nitrocellulose lateral flow discs were found to be extremely useful since they allow DNA absorption from macerated samples without using laboratory equipment or specific reagents for DNA precipitation. Another method is based on alkaline polyethylene glycol (PEG-OH) extraction buffer, which allows a fast DNA extraction from several tissues and cells ready to be processed by PCR. These methods are amply reviewed by Donoso and Valenzuela (2018) and Ivanov et al. (2021) and have great potential to be applied for POC detection since they are fast and cheap and require a minimum usage of or no laboratory equipment. Nevertheless, nucleic acid amplification can be strongly affected by the impurities in the crude extract, but this is also connected to the efficiency of the isothermal amplification technique. Interestingly, many companies have developed portable thermal cyclers to directly submit the crude extract to the amplification processes. Some examples are the MiniAmp Thermal Cycler (Thermo Fisher Scientific) and the Labnet Multigene™ Mini PCR Thermal Cycler (Sigma-Aldrich), which are compact thermal cyclers suitable also for POC applications. However, there are some especially designed as portable cyclers such as the Palm PCR™ G3 (Ahram Biosystems, Inc.), the GenePro LAMP Cycler (Gencurix), and the LAMP thermal cycler iAmpDX (K-DOD Korea).
Among the isothermal amplification methods, loop-mediated isothermal amplification (LAMP) has been developed by Notomi et al. (2000). LAMP requires two outer primers (forward outer F3 and backward outer B3) and two inner primers (forward inner FIP and backward inner BIP) recognizing six specific sequences in the target DNA. The Bst polymerase mediates strand displacement of DNA by producing a single-stranded DNA, which acts as a template for the second inner and outer primers, generating a DNA molecule with a loop structure. The addition of two extra loop primers (loop forward (LF) and loop backward (LB)) may accelerate the LAMP reaction. LAMP can be carried out at a constant temperature (60°C–65°C) with a short reaction time, which makes it ideal for POC plant pathogens detection, even though there is the need for a heat block to maintain a temperature of 60°C–65°C. Moreover, LAMP has high efficiency and sensitivity, as it generates a large amount of amplicons from low amounts of input DNA. However, the main limitations of LAMP reside in the primers’ design, which is complicated and non-intuitive, and its possible inhibitions by impurities in the crude extract. Moreover, this technique does not allow proper quantification of DNA, which is a useful tool to individuate the starting amount of inoculum (Chang et al., 2012; Zhang et al., 2014c; Lau and Botella, 2017; Le and Vu, 2017; Donoso and Valenzuela, 2018; Rani et al., 2019; Cassedy et al., 2020; Hariharan and Prasannath, 2021; Ivanov et al., 2021; Leonardo et al., 2021). To potentiate the LAMP applications for POC diagnostics, LAMP has been combined with simple read-out methods to overcome the traditional in-laboratory analyses. For example, the amplification product can be detected by the naked eye by adding color indicators into the LAMP amplification reaction prior to amplification. Alternatively, LAMP has been combined with ELISA by incorporating antigen-labeled nucleotides into the reaction; thus, the amplicons incorporate them during the amplification process. Reverse Transcriptase LAMP (RT-LAMP) assays have been also developed for virus detection (Chang et al., 2012; Zhang et al., 2014c; Lau and Botella, 2017; Le and Vu, 2017; Donoso and Valenzuela, 2018; Baldi and La Porta, 2020; Buja et al., 2021).
Helicase-dependent amplification (HDA) is an isothermal technique developed by Vincent et al. (2004). This technique works quite similarly to the standard PCR, but it does not require heat denaturation and the design of complicated primers like LAMP. A DNA helicase denatures the double-stranded DNA, following primer annealing and extension at isothermal conditions. Moreover, single-stranded DNA-binding protein (SSB) and MutL endonuclease have the role of preventing the rehybridization of complementary single-stranded DNA. Diverse POC applications for HDA also improved its sensitivity by combining HDA amplification with ELISA or gold nanoparticles, showing a 90% increase in sensitivity and specificity as compared to the standard HDA (Gill and Ghaemi, 2008; Andresen et al., 2009; Chang et al., 2012; Lau and Botella, 2017; Ivanov et al., 2021; Leonardo et al., 2021).
Rolling circle amplification (RCA) is an isothermal methodology to amplify circular DNA (Fire and Xu, 1995). The DNA amplification is carried out by using phi29 DNA polymerase, which enables single- or multiple-primer annealing to a circular DNA by having a strand displacement activity. A cascade of strand displacement events generates long single-stranded DNA containing 100–1,000 tandem repeats of the original target sequence. By manipulating linear DNA, this can be suitable as a template for RCA. Indeed, a linear single-stranded DNA probe can initially hybridize to the target sequence, forming a loop and ligating to generate a circular probe to start the RCA reaction. RCA is an isothermal technique offering the advantages of ease to use, high multiplexing potential, high sensitivity, and specificity. Incorporating RCA with microarrays, biosensors, and immune assays has significantly improved the POC potentiality of this technique (Chang et al., 2012; Lau and Botella, 2017; Hariharan and Prasannath, 2021; Ivanov et al., 2021).
Recombinase polymerase amplification (RPA) is an isothermal technique optimally functioning at 37°C–42°C, even though it can be also carried out at room temperature. In the RPA reaction, a recombinase scans the double-stranded target DNA to bind primers on complementary sites and displace them. The generated single-stranded DNA is then stabilized by an SSB. After that, the recombinase is released from the primers, and strand displacement polymerase adds the complementary nucleotides to form a new strand of DNA (Piepenburg et al., 2006). RPA has gained a great interest in pathogen detection due to its rapidity (the reaction lasts approximately 30 min), sensitivity, selectivity, and low cost. POC applications of RPA have been improved by combining RPA with reverse transcription reactions (RT-RPA) or with lateral flow devices (RPA-ELISA). The use of magnetic beads has also been investigated to develop a naked-eye assay for plant pathogen detection. Moreover, the RPA assay was found to be suitable for paper and plastic lateral flow devices and nanotechnology-based and microfluidics-based biosensors (Santiago-Felipe et al., 2014; Sun et al., 2016; Lau and Botella, 2017; Rani et al., 2019; Ivanov et al., 2021).
3.1 Molecular isothermal detection of wheat pathogens
Table 1 schematically summarizes the main isothermal techniques employed for the detection of wheat fungal pathogens, their peculiarities, potentialities, and drawbacks for POC applications.
3.1.1 Detection by loop-mediated isothermal amplification
LAMP was the most studied and validated POC application. Several LAMP assays have been investigated for the detection of Fusarium spp. The first reported study validated a LAMP assay to detect F. graminearum on galactose oxidase (gaoA) gene of the pathogen. The detection was carried out in situ by using calcein fluorescence as a marker. The assay was rapid, specific, and sensitive and detected the presence of less than 2 pg of purified target DNA per reaction within 30 min. The LAMP assay was applied for DNA amplification from fungal cultures, infected barley grains, and detection of F. graminearum in total genomic DNA isolated from wheat grains (Niessen and Vogel, 2010). Similarly, LAMP was used to detect Fusarium spp. in unmalted and malted cereals during quality control in the brewing industry by targeting hydrophobin (hyd5) gene. Calcein was used as an in-tube indirect detection indicator. The LAMP reaction detected a minimum of 0.74 pg of purified DNA in 30 min. The authors also applied the LAMP reaction by directly using the mycelia as a template without any previous DNA extraction, but they obtained a false negative from F. graminearum mycelia, probably because of the thicker cell wall as compared to the other Fusarium spp., thus reducing the accessibility of the DNA. Also, barley grains were tested directly with the LAMP method. With the use of supernatants from grain samples as templates, the LAMP assay detected an infection level of 0.5% of F. culmorum in grains (Denschlag et al., 2012). The same authors (Denschlag et al., 2014) designed LAMP primers to amplify a partial sequence of Tri6 (trichothecene transcriptional regulator) gene in F. graminearum and of Tri5 (trichodeine synthases) gene in Fusarium sporotrichioides. Interestingly, the combination of both primers in one duplex assay enabled multiple detections of F. graminearum, F. culmorum, Fusarium cerealis, F. sporotrichioides, Fusarium langsethiae, and Fusarium poae. Moving to the applicability of the assay, 100 wheat samples were analyzed for the trichothecene mycotoxin DON by high-resolution mass spectrometry (HPLC) and the presence of trichothecene producers by the new real-time duplex LAMP assay. The LAMP assay showed positive results for all samples with a DON concentration exceeding 163 ppb. As an indicator of DNA amplification, the LAMP reaction employs turbidity derived from precipitating magnesium–pyrophosphate complex formed as a by-product of enzymatic DNA biosynthesis, which is directly proportional to the amount of amplified DNA. The LAMP reaction showed detection limits varying from 0.004 ng for F. graminearum to 15.74 ng for F. poae, probably depending on the interspecific sequence variations between the target genes (Denschlag et al., 2014). In another study, F. culmorum was specifically detected by a LAMP reaction targeting CYP51C (cytochrome) gene. The LAMP efficiently runs in 60 min at 63°C, demonstrating a sensitivity of 100 pg of genomic DNA. The hydroxynaphthol blue (HNB) dye was added to the amplification reaction to visually detect positive samples (Zeng et al., 2017). The same CYP51C was targeted to specifically detect Fusarium asiaticum in diseased wheat heads. The LAMP reaction was efficient in 60 min at 63°C, demonstrating a sensitivity of 100 pg of starting DNA. The specificity was compared with that for other Fusarium spp. and other fungal species, and the results were directly visualized by adding HNB prior to amplification (Xu et al., 2017). LAMP was also employed to specifically detect fumonisin producers Fusarium spp. by targeting fum1 gene encoding for a polyketide synthase present in the genome of all fumonisin-producing Fusarium spp. Therefore, a fum1-specific LAMP assay was developed and amplified 22 species belonging to the Fusarium fujikuroi species complex (FFSC) as fumonisin producers. The limit of detection of the assay was 5 pg of genomic DNA per reaction. The usefulness of the LAMP assay was demonstrated by analyzing fumonisin-contaminated grains and by using calcein as a visual indicator of the amplified DNA (Wigmann et al., 2020). Interestingly, LAMP was also used to detect Fusarium spp. strains resistant to pesticides. For example, Duan et al. (2014) detected F. graminearum strains resistant to carbendazim by targeting the point mutation F167Y into the β2-tubulin gene leading to resistance to carbendazim. The LAMP reaction was optimal at 63°C for 60 min, and positive samples were visually detected by adding HNB prior to amplification (Duan et al., 2014). Also Fusarium spp. strains resistant to benzimidazole were detected by LAMP (Komura et al., 2018). A LAMP-fluorescent loop primer (FLP) was employed by detecting genetic polymorphisms by measuring the peak temperatures of fluorescence resonance energy transfer between an FLP and a quencher probe specifically hybridizing to the sequence including a single-nucleotide polymorphism (SNP), which is characteristic of mutant genotypes F167Y, E198Q, and F200Y carrying the mutation in the β2-tubulin gene region, which results in methyl benzimidazole carbamate (MBC) resistance. The method is based on the different annealing temperatures between the selected primers and the DNA of sensitive (fully matched) and resistant (partially mismatched) strains. As a result, sensitive strains are quenched at higher annealing temperature values, while resistant strains are quenched at lower annealing temperature values.
LAMP has been also widely employed for the rapid detection of MoT by targeting the MoT3 locus. The LAMP assay showed high specificity for MoT, and its sensitivity was 5 pg of DNA per reaction. The LAMP assay was tested on MoT-infected wheat grains and spikes by using a field DNA extraction kit and the portable Genie II system to run the DNA amplification. This assay was useful for MoT field surveillance, as well as for identifying non-host species that may serve as a source of inoculum for nearby wheat fields (Yasuhara-Bell et al., 2018). More recently, a comparative genomic approach has been adopted to identify new loci specific to MoT in order to design a set of new markers to be used in LAMP for MoT detection. The assay enabled the detection of the target at an infection rate as low as 0.25% by amplifying down to 5 pg of genomic DNA per reaction in less than 5 min. Thus, this new toolkit may be particularly beneficial in preventing the trade of contaminated seeds (Thierry et al., 2020). Another genomic approach was followed by Kang et al. (2021), where, to develop an accurate and sensitive method for MoT detection, they identified two DNA fragments, MoT-6098 and MoT-6099, that are present in the MoT genome but not in the rice-infecting M. oryzae Oryzae (MoO) pathotype. Such markers were successfully employed to design a specific and sensitive LAMP assay (Kang et al., 2021).
The causal agents of karnal bunt were also successfully detected by LAMP assays. T. indica was detected by LAMP at 62°C in 30 min. LAMP sensitivity was 10 pg of DNA, and calcein was used as an indicator for the endpoint reaction (Gao et al., 2016). While the previous authors designed a LAMP assay to specifically detect T. indica, another research work detected several Tilletia spp. by LAMP in contaminated grains. Tilletia caries, Tilletia laevis, and Tilletia controversa were detected at a minimum DNA concentration of 0.001 ng/µl (Pieczul et al., 2018). More recently, Sedaghatjoo et al. (2021) established a LAMP assay to detect T. controversa by comparing 21 genomes of six Tilletia spp. to identify DNA regions unique and conserved in T. controversa isolates. The LAMP assay was specific for T. controversa DNA, with the exception of Tilletia trabutii, from pure cultures and teliospores, and its detection limit was 5 pg of genomic DNA per reaction. The LAMP assay was validated in five laboratories in Germany and resulted in 100% sensitivity and 97.7% specificity by employing an isothermal amplification at 65°C for 45 min (Sedaghatjoo et al., 2021).
Rust pathogens have been also successfully detected by LAMP. A LAMP assay was designed to detect P. striiformis f. sp. tritici during the first latent infection in leaves for the estimation of the potential initial inoculum. The LAMP assay was also validated by amplifying DNA from spores and wheat seedlings, and its detection limit was 2 pg of starting template; moreover, the latent infection was detected on leaves 24 h after the inoculation (Huang et al., 2011). Other authors established a similar LAMP assay to detect P. striiformis f. sp. tritici during latent infection. In this study, the sensitivity of the assay was 1 pg of DNA, but the latent infection was detected 48 h after the inoculation. The authors also provided the possibility to colorimetrically detect the amplified DNA by adding HNB to the reaction mixture, and the LAMP assay was run at 65°C for 1 h (Aggarwal et al., 2017). Additionally, Manjunatha et al. (2018) detected P. triticina by a rapid, reliable, efficient, and visual colorimetric LAMP method. By in silico analyses, a specific marker, PtRA68, was individuated in the genome of P. triticina PTS68, which was targeted to establish the LAMP assay. The LAMP assay was run at 65°C for 60 min by detecting P. triticina on wheat 24 h after inoculation at a pre-symptomatic stage. The sensitivity of the LAMP assay was 100 fg, and indirect detection of the amplified DNA was recorded by adding HNB to the mixture (Manjunatha et al., 2018).
Also, Aspergillus spp. were detected by LAMP. Tang et al. (2016) developed a LAMP assay for the detection of Aspergillus fumigatus. The specificity of the assay was tested by targeting the DNA of 22 non-A. fumigatus strains, and its detection limit was 10 copies of DNA per reaction, demonstrating higher sensitivity than Real-Time qPCR (102 copies of DNA) (Tang et al., 2016). A. fumigatus was also detected by Tone et al. (2017) by coupling LAMP with melting curve analysis (MCA) to reduce both the in-laboratory work required and the eventual detection of contaminants by targeting the ribosomal DNA of the large subunit of A. fumigatus. This LAMP assay demonstrated a sensitivity of 20 copies of starting DNA (Tone et al., 2017). Finally, King et al. (2019) applied LAMP to discriminate the A. fumigatus mating type. The LAMP primers targeting MAT gene were screened against 34 A. fumigatus isolates to establish whether they could distinguish MAT1-1 or MAT1-2 genotypes. The LAMP assay operating at an isothermal temperature of 65°C discriminated against the different mating types in 20 min (King et al., 2019).
Lastly, Yan et al. (2019) employed LAMP to detect U. tritici. The designed primers were specific for U. tritici, and the sensitivity of the detection method was 100 fg of starting DNA. Furthermore, the LAMP assay was successfully employed on loose smut diseased wheat plants (Yan et al., 2019).
3.1.2 Detection by rolling circle amplification and recombinase polymerase amplification
RCA and RPA have been also investigated to detect fungal wheat pathogens. Davari et al. (2012) designed an RCA assay to detect the polymorphisms in the elongation factor 1-α (EF-1α) for the identification of different species belonging to the F. graminearum species complex (FGSC). The RCA assay also enabled the detection of the Fusarium oxysporum (FOSC), Fusarium incarnatum-equiseti (FIESC), and Fusarium tricinctum (FTSC) species complexes. The RCA assays successfully detected the DNA of the target fungi in environmental and contaminated wheat samples. The amplification product was visualized by the naked eye by adding SYBR Green in combination with a UV transilluminator (Davari et al., 2012). RPA also was employed to detect MoT. The authors used the Cas12a protein and guide RNAs to target specific MoT sequences. Cas12a showed single-stranded deoxyribonuclease (ssDNase) activity; thus, the combination of the Cas12a ssDNase activity with RPA and nucleic acid lateral flow immunoassay (NALFIA) accurately detected MoT. The RPA assay was executed at 25°C–40°C for 30 min and showed a sensitivity of 0.001 µg of starting DNA (Kang et al., 2021). RPA assay was also employed to detect B. sorokiniana by targeting the calmodulin gene sequence. The specificity of the assay was tested by targeting 19 fungal species associated with wheat, while the sensitivity resulted in 10 pg of starting DNA. The RPA assay was also tested to detect B. sorokiniana from artificially infected and naturally infected wheat plants, where the amplification reaction lasted 20–40 min (Zhao et al., 2021).
4 Phenomics-based point-of-care detection methods
Despite molecular-based POC methods being sensitive, accurate, and effective, they require sampling procedures; thus, these methods are still destructive. Moreover, isothermal amplification techniques are not high throughput, since they can allow the analysis of a limited number of samples at the same time. To develop innovative, non-destructive, and high-throughput detection methods, ground-based disease detection based on plant phenotyping could be possibly integrated with an automated agricultural vehicle. Plant phenotyping disease detection methods include both field-based and laboratory-based experiments. The laboratory-based experiments provide a strong background knowledge for the field-based applications, such as spectroscopic and imaging techniques, which could be easily integrated with an unmanned vehicle for fast, reliable, and real-time disease monitoring for control and management. This could potentially lead to the early detection of plant diseases that could be a valuable source of information for executing proper management strategies to prevent the spread of disease and to apply pesticides in a cost-effective manner (Sankaran et al., 2010; Martinelli et al., 2015). With increasing options in sensor availability for image capture and open-source analysis tools, the field of high-throughput plant phenomics is exponentially growing but has also revealed some drawbacks regarding the necessary improvements of hardware and software to advance the field (Fiorani and Schurr, 2013; Li et al., 2014; Fahlgren et al., 2015; Mahlein, 2016; Ubbens and Stavness, 2017; Qiu et al., 2018; Pieruschka and Schurr, 2019). The aim of plant imaging is to qualitatively and quantitatively measure a phenotype through the interaction between light and plants. This is possible because plant cells and tissues have wavelength-specific absorbance, reflectance, and transmittance properties, thanks to differences related to biochemical features (Li et al., 2014). Particularly, a stressed plant can react with biochemical rearrangements leading to protection mechanisms, resulting in physiological perturbations such as changes in leaf area index, chlorophyll content, or surface temperature; reduction of photosynthesis rate; and stomatal closure, which induces an increase in fluorescence and heat emission (West et al., 2003). For such reasons, spectroscopic and imaging techniques are unique monitoring methods that have been used as proximal sensors to detect diseases in several plant hosts.
Certain pathogens cause a reduction of leaf plant chlorophyll content and photosynthetic efficiency, which increases reflectance in the visible range and causes a shift of the red-edge position in the spectrum (Sankaran et al., 2010). Thus, chlorophyll fluorescence has been used to monitor diseases in plants (Li et al., 2014), by estimating the plant’s efficiency of photo-assimilation, non-photochemical quenching, and other physiological plant parameters. During disease infection, photosynthesis, respiration, and nutrient flow are subjected to metabolic changes, which can be monitored by fluorescence imaging, thus detecting early the stress responses associated with biotic factors (Balachandran et al., 1997; Chaerle et al., 2007a; Chaerle et al., 2007b; Chaerle et al., 2007c; Baker, 2008). Fluorescence imaging combined with further data analysis discriminated and quantified diverse fungal infections (Konanz et al., 2014). Nevertheless, disadvantages of fluorescence imaging concern the preparation of the plants, which need to follow a strict protocol, and thus it is difficult to implement in normal agricultural greenhouses or field environments (Li et al., 2014; Mahlein, 2016).
Thermal imaging (3–14-µm spectral range) allows the detection of canopy temperature and has been of particular interest in the laboratory and field to evaluate leaf water status (Jones et al., 2009; Munns et al., 2010), as much as stomatal conductance, since leaf temperature is dependent on water transpiration. Since biotic stresses often result in decreased rates of photosynthesis and transpiration, thermal imaging can be a reliable way to detect changes in the physiological status of plants in response to different plant pathogens (Nilsson, 1995; Chaerlevan der Straeten, 2000). This was of particular interest for the detection of systemic infections (e.g., Fusarium spp.), which often influences the transpiration rate and the water flow of the entire plant or organs. The advantages of thermal sensors are spatial resolution and easy interpretation of data; however, they are often subjected to environmental factors such as environmental temperature, sunlight, rainfall, or wind speed (Mahlein, 2016).
Imaging techniques rely on vegetation indices (VIs) as simple and effective algorithms for quantitative and qualitative evaluations of plant characteristics, such as cover, vigor, water stress, and photosynthetic efficiency, thus indirectly estimating also the presence of biotic stresses. VIs are calculated from a limited number of selected spectral bands from canopies since the reflectance of light is determined by the chemical and morphological characteristics of the plant surface. Moreover, VIs are of particular interest, since they have been employed to facilitate interpretation of remotely sensed data, while minimizing confounding effects, such as sun angle, viewing angle, atmospheric composition, canopy background variation, topography, soil variations, and differences in senesced or woody vegetation (Xue and Su, 2017; Pôças et al., 2020; Ferchichi et al., 2022).
The simplest imaging method consists of the acquisition of plant phenotypes by using cameras sensitive to the visible range (400–700 nm) of the electromagnetic spectrum (Tackenberg, 2007; Hartmann et al., 2011). The projected canopy area is extracted following image pre-processing and segmentation in the RGB (red, green, blue) space. RGB imaging mimics human perception to provide useful data for plant phenotyping applications, such as breeding for interesting plant traits or detection of different stresses. For instance, RGB coupled with machine learning for data analysis has been used to successfully detect plant diseases (Camargo and Smith, 2009; Neumann et al., 2014). The advantage of RGB sensors resides in cost accessibility and ease of operation and maintenance. However, the image acquisition step can be critical. Uniform focus, sharpness, and illumination are crucial for high accuracy and reliable results. Problems with RGB imaging are most commonly caused by the overlapping of leaves and by background soil noise. Consequently, new assessments should be performed on a case-by-case basis during phenology for the developmental stage of interest (Mahlein, 2016).
Multispectral and hyperspectral sensors are capable of scanning a broader range of wavebands at high resolution since they can detect emitted reflectance in the visible spectrum (400–700 nm), the near-infrared (700–1,100 nm), and the short-wave infrared (1,100–2,500 nm). This is of particular interest in plant phenomics since the reflectance of light from plants is a complex phenomenon depending on multiple biophysical and biochemical interactions, which vary also in response to certain stress. As an example, the visible range is mainly influenced by green leaf pigment content; the near-infrared reflectance depends on the tissue structure, internal scattering processes, and the absorption by leaf water; the short-wave infrared is influenced by the composition of tissue chemicals and water (Carter and Knapp, 2001).
The use of such remote sensors can be grounded or airborne (unmanned aerial vehicles (UAVs)) and spaceborne (satellites). The Sentinel-2 satellite launched by ESA has a free and open access policy, thus overcoming the expensive inaccessibility of satellite-based images used for agricultural purposes. Sentinel-2 monitors and maps at local, regional, and global scales by using multispectral sensors, while other hyperspectral missions have been planned or recently launched, such as the Italian mission PRecursore IperSpettrale (PRIMSA), the German mission Environmental Mapping and Analysis Program (EnMAP), the Japanese mission Hyperspectral Imager SUIte (HISUI), and the National Aeronautics and Space Administration (NASA) mission Hyperspectral Infrared Imager (HyspIRI). During the last years, the costs and operability of UAVs are definitely more accessible for agricultural applications. UAVs equipped with sensors provide, in a fast and easy way, field data for precision agriculture applications. While the resolution of satellite images is from 5 to 30 m, UAV-based imaging provides resolution at pixel sizes (3–5 cm2); thus, UAVs are a useful technology for crop monitoring at different scales and can be used for agronomic experiments where space, resource, and time constraints limit manual sampling (Xue and Su, 2017; Marino and Alvino, 2019; Marino and Alvino, 2020; Pôças et al., 2020).
4.1 Phenomics-based detection of wheat pathogens
Table 2 schematically summarizes the main phenomics-based techniques employed for the detection of wheat fungal pathogens, their peculiarities, potentialities, and drawbacks for POC applications.
4.1.1 Detection by fluorescence sensors
Kuckenberg et al. (2009) employed chlorophyll fluorescence to detect both leaf rust and powdery mildew. The authors measured the photochemical efficiency (Fv/Fm and Fv/Fo), on a daily basis over a period of 2 weeks following the inoculation of wheat leaves with B. graminis and P. triticina. Fluorescence imaging detected early the infection (2–3 days before apparent symptoms), since the initial infection of both pathogens caused a decrease in photochemical efficiency (Kuckenberg et al., 2009). Leaf rust was also detected by fluoresce imaging during a pre-symptomatic stage of the infection. Fluorescence spectra were collected from healthy and inoculated plants 2–4 days after inoculation. Fluorescence signatures revealed that inoculated leaves may be separated by healthy ones, but a high classification accuracy was difficult to achieve. The integration of a support vector machine for classification reached an accuracy of 93% in distinguishing between healthy and diseased plants 2 days after inoculation before any visible symptoms appeared (Römer et al., 2011). Another study employed chlorophyll fluorescence to detect FHB. Wheat heads were artificially inoculated with F. culmorum, and fluorescence sensors were used to detect Fv/Fm to determine the degree of the damaged ears. Results showed that Fv/Fm decreased in infected heads compared to healthy ones and that the lowest level of disease detection by chlorophyll fluorescence corresponded to a visually rated degree of disease of at least 5% (Bauriegel et al., 2010).
4.1.2 Detection by RGB and thermal sensors
RGB imaging was widely employed to detect rust diseases. Zhou et al. (2015) tested rust resistance in 12 winter wheat genotypes by assessing two RGB indices, grain yield (GY) and grain yield loss index (GYLI). GYLI values distinguished between resistant and susceptible varieties and demonstrated to be a potentially affordable approach for high-throughput phenotyping of yellow rust resistance in wheat (Zhou et al., 2015). Another study employed RGB imaging in the field to predict grain yield and yellow rust disease severity by measuring the normalized difference vegetation index (NDVI), leaf chlorophyll content, stomatal conductance, and canopy temperature. RGB imaging proved to be an accurate predictor of grain yield and grain yield losses associated with yellow rust, showing correlation coefficients of 0.581 and 0.536 (Vergara-Diaz et al., 2015). A recent study detected leaf and stripe rusts by UAV-based RGB imaging to discriminate between healthy and infected wheat plants. The study has been conducted in four wheat fields, and diseased leaf areas were determined based on green and red spectral bands for stripe rust and the combination of green, red, and blue spectral bands for leaf rust. Both diseases caused an alteration in the reflectance spectra of plants in the green and red channels. Thus, the RGB-based detection accurately identified infected leaf areas at stem elongation and booting stages for efficacious fungicide application (Dehkordi et al., 2020). RGB imaging was also employed to detect powdery mildew by using dual-green indices. The canopy spectra were measured in artificially inoculated fields, potted plants, and nurseries at different levels of disease incidence and wheat growth stages. The authors individuated that the most sensitive bands to powdery mildew were between 580 and 710 nm and constructed dual-green vegetation indices to detect the disease (Feng et al., 2016). FHB was also detected by color imaging in combination with a deep learning technique. RGB images of wheat spikes were collected in shadow at the milk stage and processed to construct datasets employed to retrain the deep learning model. The RGB detection method was compared to the manual count of infected spikes and showed to detect accurately infected spikes, demonstrating a coefficient of determination of 0.80 (Qiu et al., 2019).
Infrared thermal sensors have been employed to detect Z. tritici in the field. Twenty-five wheat genotypes were artificially inoculated in order to predict the onset of the disease before visual symptoms appeared. The results showed that the maximum temperature difference and the temperature depression significantly correlated with the presence of the disease (Wang et al., 2019).
4.1.3 Detection by multispectral sensors
Multispectral and hyperspectral sensors were the most studied spectral-based techniques to detect wheat fungal pathogens. Multispectral sensors have been widely employed to detect powdery mildew in controlled and field conditions. Graeff et al. (2006) inoculated wheat plants under controlled conditions, measured leaf reflectance in the visible and near-infrared spectra, and demonstrated that leaf reflectance highly correlated with different infection levels of powdery mildew (Graeff et al., 2006). Another study examines the potential of multispectral remote sensing for a multitemporal analysis of powdery mildew and leaf rust. An experimental field showing all the infective stages of powdery mildew and leaf rust was subjected to high-resolution remote sensing in order to execute spatial and temporal analyses of the infection dynamics. Images were used to calculate the NDVI to classify areas with different levels of disease severity, demonstrating an accuracy ranging from 56.8% to 88.6% (Franke and Menz, 2007). However, Yuan et al., 2014; Yuan et al. 2016a detected powdery mildew by satellite-based multispectral sensors. Two regions in China were selected for conducting a field survey, and five multispectral-based indices were associated with three supervised classification methods. The accuracy of such detection techniques ranged from 79% to 89% (Yuan et al., 2014; Yuan et al., 2016a). The authors also employed satellite-based multispectral sensors to detect both powdery mildew and yellow rust in winter wheat. The results revealed a significant increase of reflectance over the “red-valley” spectra at 650–680 nm, probably caused by a reduced amount of chlorophyll and destruction of cell structures due to infections (Yuan et al., 2017). Detection of rust pathogens by multispectral approaches has been also widely investigated. Devadas et al. (2009) evaluated 10 multispectral vegetation indices to distinguish rust diseases on leaves. They found that the reflectance features for non-chlorophyll pigment discriminated between diseased and healthy leaves. Nevertheless, none of the individuated indices discriminated among the three rust pathogens (Devadas et al., 2009). Ashourloo et al. (2014a) a developed two multispectral indices for leaf rust detection. The authors collected the reflectance spectra of infected and uninfected leaves at different stages, while RGB imaging was employed as ground truth to obtain the ratio of the disease-affected area and the fractions of the different symptoms. The two developed multispectral indices demonstrated a correlation coefficient of 0.94 with the RGB imaging for the estimation of infected leaves (Ashourloo et al., 2014a). The same authors developed also multispectral-based indices to classify different rust symptoms levels. The reflectance of healthy and infected leaves was collected in the 450–1,000-nm range and RGB imaging was again employed as ground reference. The authors observed that the values of the indices increased with the disease severity, demonstrating a classification accuracy from 20% to 60% (Ashourloo et al., 2014b). Satellite-based multispectral sensors have been also employed to detect yellow rust to discriminate between healthy, slight, and severe infection levels. Three sensitive spectral bands were individuated, and a novel multispectral index, the red edge disease stress index (REDSI), was proposed to classify different disease levels of yellow rust (Zheng et al., 2018). The same authors employed also multispectral sensors to detect yellow rust at different growth stages. They individuated yellow rust-sensitive bands from booting to anthesis (460–720 nm) and from filling to milky ripeness (568–1,000 nm). The band combinations were used to calculate two indices, the photochemical reflectance index (PRI) and the anthocyanin reflectance index (ARI), and employed to determine disease severity at different growth stages (Zheng et al., 2019). A more recent study trained multispectral sensors in association with a classification algorithm to detect yellow rust at different disease stages and to discriminate the infection from the defense responses occurring in a resistant variety. The method detected early the presence of yellow rust and showed an accuracy of 86% (Aharoni et al., 2021). FHB, spot blotch, and wheat blast have been also detected by multispectral imaging. Bauriegel et al. (2011a) employed multispectral sensors to detect FHB before harvesting in order to separate infected from uninfected grains. The spectra of diseased and healthy heads were analyzed by principal component analysis (PCA) and successfully detected FHB during the beginning of the medium milk stage but not at flowering; thus, early detection was not possible (Bauriegel et al., 2011a). Spot blotch was detected by calculating the NDVI in order to individuate resistant wheat genotypes. Particularly, 108 wheat germplasm were subjected to multispectral sensors, and a high negative correlation was observed between symptoms’ visual observation and NDVI, allowing an accurate mapping of quantitative trait loci (QTLs) associated with spot blotch resistance in wheat (Kumar et al., 2016). Finally, Gongora-Canul et al. (2020) used multispectral imaging to detect wheat blast. The experiments were conducted in two files in order to distinguish between symptoms of spikes and leaves. Multispectral measurements were correlated to visual estimation of symptoms, and this method was precise and accurate for wheat blast detection at different levels of disease severity (Gongora-Canul et al., 2020).
4.1.4 Detection by hyperspectral sensors
Hyperspectral sensors are the most promising and employed ones since they can analyze a wide range of wavelengths. Detection of rust diseases has been widely explored as reviewed by Maid and Deshmukh (2018). Huang et al. (2007) employed in situ and airborne hyperspectral imaging to detect yellow rust. The PRI has been developed to quantify the disease index in wheat, demonstrating a negative correlation with the disease index (Huang et al., 2007). Another study developed a continuous wavelet analysis method based on decomposing the amplitude and the scale of hyperspectral data at continuous position and scale, allowing a thorough exploration over the spectrum to detect yellow rust. This method was compared to conventional hyperspectral indices for the detection at the leaf level. Hyperspectral measurements were performed at two growth stages, and the results highlighted that the method detected yellow rust at both stages. Univariate and multivariate statistics revealed a high accuracy (coefficient of determination of 0.81) and potential ability to detect early yellow rust on leaves (Zhang et al., 2014b). Satellite-based hyperspectral imaging was also employed to detect yellow rust. Yuan et al. (2013) considered 10 satellite sensors to individuate disease-sensitive bands. Green, red, and near-infrared bands were the most useful for yellow rust detection and well correlated with conventional vegetation indices (Yuan et al., 2013). Another different approach has been proposed by Bohnenkamp et al. (2019), who established a hyperspectral method to distinguish between brown rust and yellow rust on wheat leaves by detecting pure fungal spore spectra as reference. After individuating the spectral fingerprint of both diseases, symptom quantification was verified on inoculated plants at different time points. The detection of rust diseases was enabled without pixel-wise labeling thanks to the reference spectra from rust spores (Bohnenkamp et al., 2019). A very similar method was adopted by Wójtowicz et al. (2021) to detect leaf rust by using a spectrometer connected to a microscope and measuring in the range of 350–2,500 nm. Raw spectra of uredospores, chlorotic discoloration, green leaves, senescent inoculated leaves, and senescent uninoculated leaves of wheat and rye were collected and used to individuate seven indices for the classification of different symptoms (Wójtowicz et al., 2021). Also, UAV-based hyperspectral imaging has been investigated for yellow rust detection. Zhang et al. (2019b) coupled UAV-based hyperspectral imaging with a deep convolutional neural network for automated disease detection in order to employ both spatial and spectral information for yellow rust detection. The model was calibrated with hyperspectral images collected in a well-controlled field experiment consisting of healthy and infected plots. This method resulted in a highly accurate (correlation coefficient of 0.85) detection of yellow rust (Zhang et al., 2019b). Stripe rust was also detected by hyperspectral imaging. The authors collected infected wheat leaves and measured their chlorophyll content expressed as SPAD values. Sensitive hyperspectral bands were individuated by PCA and were correlated to SPAD values. The validation accuracy of this method was high (correlation coefficient of 0.92) and was also employed to estimate distribution maps of stripe rust by allowing its detection 6 days after inoculation and 3 days before visible symptoms appeared (Yao et al., 2019). More recently, high-throughput plant phenotyping based on hyperspectral imaging was employed to predict yellow rust distribution in field trials. In this work, 40 indices were associated with the random forest model and demonstrated a good prediction accuracy when measuring the correlation between predicted and observed scores (Koc et al., 2022). Powdery mildew has been also highly investigated for the possibility of hyperspectral-based detection. Zhang et al. (2012) compared hyperspectral measures from infected and healthy leaves in laboratory. Thirty-two spectral features were tested for their ability to discriminate between three health status (normal, slightly damaged, and heavily damaged) and were found to be highly accurate (over 90%) for detection of heavily damaged leaves (Zhang et al., 2012). Cao et al. (2013) tested hyperspectral imaging on wheat varieties with different susceptibility levels to powdery mildew at several wheat growth stages. The authors calculated the difference vegetation index (DVI) and the soil-adjusted vegetation index (SAVI) and observed that as the disease level increased, the reflectance in the near-infrared spectrum decreased (Cao et al., 2013). The same authors employed hyperspectral imaging to both detect powdery mildew and estimate grain yield loss after infection and found that the wavelength range between 680 and 760 nm is well-correlated with the observed grain yield (Cao et al., 2015). A more recent work conducted by Zhao et al. (2020) describes the integration of hyperspectral imaging with machine learning algorithms to quantitatively identify powdery mildew infection on wheat leaves. The support vector machine was constructed by PCA, and the detection method showed an accuracy of 93.33% (Zhao et al., 2020). Interestingly, hyperspectral sensors were employed for a double detection of both rust and powdery mildew diseases by Huang et al. (2014). Reflectance from all possible combinations of the most relevant wavelengths was used to calculate the spectral indices. The classification accuracies of such indices to distinguish between healthy and diseased leaves ranged from 85.2% to 93.5% (Huang et al., 2014). Hyperspectral-based imaging was also employed in the open field for multiple detections of yellow rust and powdery mildew in winter wheat, and multivariate regression analysis was run to statistically validate such data. The classification accuracy of such a method was 91.9% (Shi et al., 2017). Similar research was conducted by Liang et al. (2017), who detected powdery mildew and yellow rust in an open field. Hyperspectral data were validated by PCA, and 12 disease-sensitive bands were individuated, demonstrating a classification accuracy of 92% (Liang et al., 2017). Hyperspectral imaging has been also widely investigated for its ability to detect FHB and mycotoxin content in grains, as reviewed by Femenias et al. (2020), starting from microscopic observations to high-throughput phenotyping platforms. Zhang et al. (2019a) individuated a classification index for FHB detection from hyperspectral imaging obtained by microscopy observations of wheat spikes. The classification index displayed an overall accuracy of 89.80% in classifying diseased and healthy spikes and demonstrated 30% higher accuracy as compared to six common vegetation indices (Zhang et al., 2019a). Another work employed hyperspectral imaging on selected textural parameters of wheat grains to distinguish between infected and healthy grains situated at the ventral or dorsal side of the spike. The classification accuracy for the kernels positioned on the ventral side ranged from 78% to 100%, while it was 78%–98% for the kernels positioned at the dorsal site. When combining textural parameters from the ventral and dorsal sites, the classification accuracy was 76%–98%. This classification model will be of particular interest to individuate mycotoxin-contaminated grains in spikes (Ropelewska and Zapotoczny, 2018). Hyperspectral imaging was also used to phenotype wheat varieties for resistance to FHB. An automated method based on using two hyperspectral sensors was employed to capture the most relevant bands for pigments, cell structure, water, and synthesis of secondary compounds. High correlation was found between the disease severity and the spectral signatures in 430–525, 560–710, and 1,115–2,500 nm, demonstrating that this approach can improve breeding the process to identify resistant and susceptible varieties (Alisaac et al., 2018). A more recent study proposed a novel hyperspectral classification index for ease and fast detection of Fusarium-damaged kernels (FDK). The classification accuracy of the method was 0.97, with a specificity of 0.99 and a sensibility of 0.93. Interestingly, the detection was performed in 15.07 s (Zhang et al., 2020). More recently, FHB was also detected by UAV-based hyperspectral imaging. The authors combined spectral and texture features to accurately and timely detect FHB (Xiao et al., 2021). FHB and yellow rust were also contemporarily detected by hyperspectral imaging. The authors mapped the spatial distribution of the diseases by on-line hyperspectral measurements performed in the field. Hyperspectral images were overlapped to RGB images, and the disease classification was performed by in-field visual assessments and photo interpretation assessments, which were found to be highly accurate to detect both fungal diseases (Whetton et al., 2018). More recently, hyperspectral imaging has been employed to detect Septoria diseases. Anderegg et al. (2019) detected and quantified crop diseases caused by S. tritici blotch in field. They collected canopy reflectance on 18 infected wheat genotypes and healthy plots and demonstrated that changes in temporal canopy reflectance correlated with the presence of Septoria blotch. The authors also distinguished between infected and senescent plants in order to individuate resistant genotypes directly in field (Anderegg et al., 2019). Another research work developed a novel hyperspectral-based approach to monitor and detect Septoria leaf blotch in combinations with climatic data, crop rotation, and historical data of disease appearance and severity. The final output of such methods is a map of probability of leaf blotch appearance. The monitoring method was found to be highly sensitive in detecting leaf blotch at middle-to-late period of wheat phenological stages (Malakhov, 2021). Less recently, hyperspectral sensors have been employed to detect Aspergillus molds. In these works, the authors scanned infected kernels, and data analysis was performed by PCA. Such model showed an accuracy of 97%–100% in classifying infected kernels (Singh et al., 2007; Singh et al., 2012).
4.1.5 Detection by multiple combinations of sensors
Among the diverse methods to detect wheat pathogens, recently it was highly informative to couple two or more phenomics-based methods. RGB and chlorophyll fluorescence measurements were both employed to detect leaf rust and powdery mildew and to additionally distinguish between the two wheat diseases and nitrogen deficiency. The authors artificially inoculated wheat plants and induced nitrogen deficiency in controlled conditions and successfully discriminated between healthy and infected plants 1 and 2 days after inoculation for powdery mildew and leaf rust, respectively (Bürling et al., 2011). RGB and multispectral sensors were coupled to detect FHB in the field. Wheat varieties with different levels of susceptibilities were artificially inoculated and were scanned by RGB and multispectral sensors associated with real-time image analysis software. The phenomics-based data correlated with the visually detected disease levels, but the multispectral system was more accurate than RGB (Dammer et al., 2011). Chlorophyll fluorescence coupled with spectral sensors was employed to detect powdery mildew (Peteinatos et al., 2016) and FHB (Bauriegel et al., 2011b). Peteinatos et al. (2016) performed pot experiments in which spectral and fluorescence sensors distinguished between non-stressed and stressed plants exposed to water stress, weed invasion, and powdery mildew infection. Particularly, water stress and powdery mildew were detected before symptoms appeared (Peteinatos et al., 2016). In the second research paper, FHB was detected by proximal sensors in the laboratory. Data analysis revealed that such coupled methods successfully classified healthy and diseased spikes since photosynthetic efficiency decreased from 6 days after inoculation and correlated with disease severity, while hyperspectral imaging detected FHB at 7 days after inoculation (Bauriegel et al., 2011b). Infrared thermal and fluorescence sensors were used to detect F. culmorum, S. tritici, and B. graminis in experiments conducted in a greenhouse. The authors observed an increase in canopy temperature and a decrease in chlorophyll content 2 days after inoculation (Wang et al., 2014). Infrared and chlorophyll fluorescence sensors were also used in another work to detect Septoria blotch. This method identified photosystem II quantum yield and vegetative indices associated with the biochemical composition of leaves (chlorophyll, carotenoid, and anthocyanin content) as the most predictive variables for blotch detection (Odilbekov et al., 2018). RGB and infrared sensors were both used to detect powdery mildew (Zhang et al., 2014a) and FHB (Francesconi et al., 2021). Zhang et al. (2014a) detected powdery mildew by satellite-based and multi-temporal data of surface reflectance in RGB and infrared bands for a disease survey during a field campaign. Single-stage and multi-stage spectral features sensitive to powdery mildew have been individuated and used to generate maps showing a distribution pattern of powdery mildew (Zhang et al., 2014a). Francesconi et al. (2021) employed UAV-based RGB and infrared sensors to detect FHB in the field during two field campaigns. Infrared and RGB data were validated by proximal measurements, such as spike’s temperature and photosynthetic efficiency as much as molecular detection of FHB. This phenomics-based technique detected FHB and anthesis halfway (Francesconi et al., 2021). Finally, Mahlein et al. (2019) compared thermal, fluorescence, and hyperspectral sensors to detect FHB. Under controlled conditions, the authors acquired time-series measurements with infrared thermography, chlorophyll fluorescence, and hyperspectral imaging. Differences in temperature and photosynthetic efficiency were detected at 5 days after inoculation, while hyperspectral imaging discriminated between infected and uninfected heads at 3 days after inoculation. Moreover, the combination of the three methods improved the detected accuracy to almost 90% (Mahlein et al., 2019).
5 Conclusions and future perspectives
POC diagnostics could potentially play an important role in environmental monitoring, health, and food safety by effectively detecting plant pathogens. Modern agriculture is nowadays leading to the optimization of management practices; thus, the assumption of a homogenous pesticide application must be abandoned in order to decrease costs related to agrochemicals and to increase sustainability and benefits for agroecological health (Mahlein et al., 2018). POC molecular- and phenomics-based detection techniques aim to realize real-time, robust mapping systems for crop health status to facilitate a management decision. The occurrence of plant disease depends on specific environmental factors, spatial distribution in the field, and the presence of resistant or susceptible hosts; POC methodologies are useful to identify primary disease foci and areas differing in disease severity in fields. Nevertheless, POC detection techniques demonstrate some disadvantages that need to be further implemented for in-field applications. Despite isothermal molecular methodologies do not require specific facilities and are extremely specific, sensitive, fast, and suitable for multiplex pathogens detection (especially for RCA), they are not quantitative and can require specific expertise during primer design (especially for LAMP), and DNA amplification can be inhibited by impurities (especially LAMP and HDA), thus requiring a time-consuming DNA extraction prior amplification. Phenomics-based techniques are high-throughput methodologies allowing the non-destructive acquisition of data from hundreds of plants in a few minutes. They potentially provide information with a high temporal resolution, and they can furnish models for disease management scheduling. Nevertheless, they are still expensive, and since they are based on indirect pathogen detection by capturing spectral differences due to physiological perturbations, it is nowadays not possible to distinguish between different stresses (biotic or abiotic). Thus, novel tools need to be implemented for potential multiple stress detection to individuate peculiar spectra associated with certain stresses. Probably, complementary methodologies will be necessary in the near future to fill this gap. Moreover, some approaches are crop-specific and local-specific; thus, there is a need to validate them in different environments, and they are weather-dependent, since applications may be compromised by environmental factors. Indeed, the so-called envirotyping, defined as next-generation and high-throughput technologies aimed at investigating the environmental influences on phenomics techniques, could potentially address this issue (Song et al., 2021; Gill et al., 2022). For such reasons, these techniques, in combination with advanced methods of real-time and less labor-intensive data analysis, can be used for targeted and optimized pest management programs in sustainable wheat production, thus resulting in a potential reduction in pesticide use, economic expense, and ecological impact in agricultural crop production systems.
Author contributions
SF conceived the topic, collected and analyzed the bibliography, and wrote, prepared, reviewed, and formatted the manuscript and the figure. The author confirms being the sole contributor of this work and has approved it for publication and formatted the manuscript, the tables and the figures.
Funding
This work was supported by the Italian Ministry for University and Research (MUR), Law 232/216 Department of Excellence and Ministerial Decree (DM) 1062 (10 August 2021) PON “Research and Innovation 2014-2020 of the National Smart Specialization Strategy (SNSI) and the National Research Plan (PNR)”.
Conflict of interest
The author declares that the research was conducted in the absence of any commercial or financial relationships that could be construed as a potential conflict of interest.
Publisher’s note
All claims expressed in this article are solely those of the authors and do not necessarily represent those of their affiliated organizations, or those of the publisher, the editors and the reviewers. Any product that may be evaluated in this article, or claim that may be made by its manufacturer, is not guaranteed or endorsed by the publisher.
References
Aboukhaddour R., Fetch T., McCallum B. D., Harding M. W., Beres B. L., Graf R. J. (2020). Wheat diseases on the prairies: A Canadian story. Plant Pathol. 69, 418–432. doi: 10.1111/ppa.13147
Abrahim A. (2019). Loose smut of wheat (Ustilago tritici) and its managements: a review article. J. Biol. Agric. Healthc. 9, 25–33. doi: 10.7176/JBAH
Aggarwal R., Sharma S., Manjunatha C., Gupta S., Singh V. K. (2017). Development and validation of loop mediated isothermal amplification based detection assay for Puccinia striiformis f. sp. tritici causing stripe rust of wheat. Australas. Plant Pathol. 46, 577–583. doi: 10.1007/s13313-017-0524-x
Aharoni R., Klymiuk V., Sarusi B., Young S., Fahima T., Fishbain B., et al. (2021). Spectral light-reflection data dimensionality reduction for timely detection of yellow rust. Precis. Agric. 22, 267–286. doi: 10.1007/s11119-020-09742-2
Alam M. A., Xue F., Wang C., Ji W. (2011). Powdery mildew resistance genes in wheat: identification and genetic analysis. J. Mol. Biol. Res. 1, 20–39. doi: 10.5539/jmbr.v1n1p20
Alisaac E., Behmann J., Kuska M. T., Dehne H. W., Mahlein A. K. (2018). Hyperspectral quantification of wheat resistance to fusarium head blight: comparison of two Fusarium species. Eur. J. Plant Pathol. 152, 869–884. doi: 10.1007/s10658-018-1505-9
Alkan M., Bayraktar H., İmren M., Özdemir F., Lahlali R., Mokrini F., et al. (2022). Monitoring of host suitability and defense-related genes in wheat to Bipolaris sorokiniana. J. Fungi 8, 149. doi: 10.3390/jof8020149
Al-Sadi A. M. (2021). Bipolaris sorokiniana-induced black point, common root rot, and spot blotch diseases of wheat: a review. Front. Cell. Infect. Microbiol. 11. doi: 10.3389/fcimb.2021.584899
Al-Sadi A. M., Deadman M. L. (2010). Influence of seed-borne Cochliobolus sativus (Anamorph Bipolaris sorokiniana) on crown rot and root rot of barley and wheat. J. Phytopathol. 158, 683–690. doi: 10.1111/j.1439-0434.2010.01684.x
Anderegg J., Hund A., Karisto P., Mikaberidze A. (2019). In-field detection and quantification of Septoria tritici blotch in diverse wheat germplasm using spectral–temporal features. Front. Plant Sci. 10 1355. doi: 10.3389/fpls.2019.01355
Andresen D., von Nickisch-Rosenegk M., Bier F. F. (2009). Helicase dependent OnChip-amplification and its use in multiplex pathogen detection. Clin. Chim. Acta 403, 244–248. doi: 10.1016/j.cca.2009.03.021
Ash G. (1996). Wheat rusts: An atlas of resistance genes. Australas. Plant Pathol. 25, 70–70. doi: 10.1007/bf03214019
Ashourloo D., Mobasheri M. R., Huete A. (2014a). Developing two spectral disease indices for detection of wheat leaf rust (Puccinia triticina). Remote Sens. 6, 4723–4740. doi: 10.3390/rs6064723
Ashourloo D., Mobasheri M. R., Huete A. (2014b). Evaluating the effect of different wheat rust disease symptoms on vegetation indices using hyperspectral measurements. Remote Sens. 6, 5107–5123. doi: 10.3390/rs6065107
Baker N. R. (2008). Chlorophyll fluorescence: A probe of photosynthesis in vivo. Annu. Rev. Plant Biol. 59, 89–113. doi: 10.1146/annurev.arplant.59.032607.092759
Balachandran S., Hurry V. M., Kelley S. E., Osmond C. B., Robinson S. A., Rohozinski J., et al. (1997). Concepts of plant biotic stress. some insights into the stress physiology of virus-infected plants, from the perspective of photosynthesis. Physiol. Plant 100, 203–213. doi: 10.1034/j.1399-3054.1997.1000201.x
Baldi P., La Porta N. (2020). Molecular approaches for low-cost point-of-care pathogen detection in agriculture and forestry. Front. Plant Sci. 11. doi: 10.3389/fpls.2020.570862
Bauriegel E., Giebel A., Geyer M., Schmidt U., Herppich W. B. (2011a). Early detection of fusarium infection in wheat using hyper-spectral imaging. Comput. Electron. Agric. 75, 304–312. doi: 10.1016/j.compag.2010.12.006
Bauriegel E., Giebel A., Herppich W. B. (2010). Rapid fusarium head blight detection on winter wheat ears using chlorophyll fluorescence imaging. J. Appl. Bot. Food Qual. 83, 196–203.
Bauriegel E., Giebel A., Herppich W. B. (2011b). Hyperspectral and chlorophyll fluorescence imaging to analyse the impact of fusarium culmorum on the photosynthetic integrity of infected wheat ears. Sensors 11, 3765–3779. doi: 10.3390/s110403765
Beyer M., Klix M. B., Klink H., Verreet J. A. (2006). Quantifying the effects of previous crop, tillage, cultivar and triazole fungicides on the deoxynivalenol content of wheat grain - a review. J. Plant Dis. Prot. 113, 241–246. doi: 10.1007/BF03356188
Bishnoi S. K., He X., Phuke R. M., Kashyap P. L., Alakonya A., Chhokar V., et al. (2020). Karnal bunt: a re-emerging old foe of wheat. Front. Plant Sci. 11. doi: 10.3389/fpls.2020.569057
Bohnenkamp D., Kuska M. T., Mahlein A. K., Behmann J. (2019). Hyperspectral signal decomposition and symptom detection of wheat rust disease at the leaf scale using pure fungal spore spectra as reference. Plant Pathol. 68, 1188–1195. doi: 10.1111/ppa.13020
Brennan C. J., Benbow H. R., Mullins E., Doohan F. M. (2019). A review of the known unknowns in the early stages of Septoria tritici blotch disease of wheat. Plant Pathol. 68, 1427–1438. doi: 10.1111/ppa.13077
Brennan C. J., Zhou B., Benbow H. R., Ajaz S., Karki S. J., Hehir J. G., et al. (2020). Taxonomically restricted wheat genes interact with small secreted fungal proteins and enhance resistance to Septoria tritici blotch disease. Front. Plant Sci. 11. doi: 10.3389/fpls.2020.00433
Buja I., Sabella E., Monteduro A. G., Chiriacò M. S., De Bellis L., Luvisi A., et al. (2021). Advances in plant disease detection and monitoring: from traditional assays to in-field diagnostics. Sensors 21, 2129. doi: 10.3390/s21062129
Bürling K., Hunsche M., Noga G. (2011). Use of blue-green and chlorophyll fluorescence measurements for differentiation between nitrogen deficiency and pathogen infection in winter wheat. J. Plant Physiol. 168, 1641–1648. doi: 10.1016/j.jplph.2011.03.016
Camargo A., Smith J. S. (2009). Image pattern classification for the identification of disease causing agents in plants. Comput. Electron. Agric. 66, 121–125. doi: 10.1016/j.compag.2009.01.003
Cao X., Luo Y., Zhou Y., Duan X., Cheng D. (2013). Detection of powdery mildew in two winter wheat cultivars using canopy hyperspectral reflectance. Crop Prot. 45, 124–131. doi: 10.1016/j.cropro.2012.12.002
Cao X., Luo Y., Zhou Y., Fan J., Xu X., West J. S., et al. (2015). Detection of powdery mildew in two winter wheat plant densities and prediction of grain yield using canopy hyperspectral reflectance. PLoS One 10, e0121462. doi: 10.1371/journal.pone.0121462
Carris L. M., Castlebury L. A., Goates B. J. (2006). Nonsystemic bunt fungi - Tilletia indica and T. horrida: A review of history, systematics, and biology. Annu. Rev. Phytopathol. 44, 113–133. doi: 10.1146/annurev.phyto.44.070505.143402
Carter G. A., Knapp A. K. (2001). Leaf optical properties in higher plants: linking spectral characteristics to stress and chlorophyll concentration. Am. J. Bot. 88, 677–684. doi: 10.2307/2657068
Cassedy A., Mullins E., O’Kennedy R. (2020). Sowing seeds for the future: The need for on-site plant diagnostics. Biotechnol. Adv. 39, 107358. doi: 10.1016/j.biotechadv.2019.02.014
Chaerle L., Hagenbeek D., De Bruyne E., van der Straeten D. (2007a). Chlorophyll fluorescence imaging for disease-resistance screening of sugar beet. Plant Cell Tissue Organ Culture 97–106. doi: 10.1007/s11240-007-9282-8
Chaerle L., Leinonen I., Jones H. G., van der Straeten D. (2007b). Monitoring and screening plant populations with combined thermal and chlorophyll fluorescence imaging. J. Exp. Bot. 58, 773–784. doi: 10.1093/jxb/erl257
Chaerle L., Lenk S., Hagenbeek D., Buschmann C., van der Straeten D. (2007c). Multicolor fluorescence imaging for early detection of the hypersensitive reaction to tobacco mosaic virus. J. Plant Physiol. 164, 253–262. doi: 10.1016/j.jplph.2006.01.011
Chaerle L., van der Straeten D. (2000). Imaging techniques and the early detection of plant stress. Trends Plant Sci. 5, 495–501. doi: 10.1016/S1360-1385(00)01781-7
Chang C. C., Chen C. C., Wei S. C., Lu H. H., Liang Y. H., Lin C. W. (2012). Diagnostic devices for isothermal nucleic acid amplification. Sensors 12, 8319–8337. doi: 10.3390/s120608319
Chaudhary R., Pujari M. (2021). Major diseases of wheat and their management: a review. Plant Arch. 21, 240–245. doi: 10.51470/PLANTARCHIVES.2021.v21.no2.037MAJOR
Corkley I., Fraaije B., Hawkins N. (2022). Fungicide resistance management: maximizing the effective life of plant protection products. Plant Pathol. 71, 150–169. doi: 10.1111/ppa.13467
Couch B. C., Kohn L. M. (2002). A multilocus gene genealogy concordant with host preference indicates segregation of a new species, Magnaporthe oryzae, from M. grisea. Mycologia 94, 683–693. doi: 10.1080/15572536.2003.11833196
Craw P., Balachandran W. (2012). Isothermal nucleic acid amplification technologies for point-of-care diagnostics: A critical review. Lab. Chip 12, 2469–2486. doi: 10.1039/c2lc40100b
Cruz C. D., Valent B. (2017). Wheat blast disease: danger on the move. Trop. Plant Pathol. 42, 210–222. doi: 10.1007/s40858-017-0159-z
Dammer K. H., Möller B., Rodemann B., Heppner D. (2011). Detection of head blight (Fusarium ssp.) in winter wheat by color and multispectral image analyses. Crop Prot. 30, 420–428. doi: 10.1016/j.cropro.2010.12.015
Davari M., van Diepeningen A. D., Babai-Ahari A., Arzanlou M., Najafzadeh M. J., van der Lee T. A. J., et al. (2012). Rapid identification of Fusarium graminearum species complex using rolling circle amplification (RCA). J. Microbiol. Methods 89, 63–70. doi: 10.1016/j.mimet.2012.01.017
Dean R., Van Kan J. A. L., Pretorius Z. A., Hammond-Kosack K. E., Di Pietro A., Spanu P. D., et al. (2012). The top 10 fungal pathogens in molecular plant pathology. Mol. Plant Pathol. 13, 414–430. doi: 10.1111/j.1364-3703.2011.00783.x
Dehkordi R. H., El Jarroudi M., Kouadio L., Meersmans J., Beyer M. (2020). Monitoring wheat leaf rust and stripe rust in winter wheat using high-resolution UAV-based red-green-blue imagery. Remote Sens. 12, 3696. doi: 10.3390/rs12223696
Denschlag C., Rieder J., Vogel R. F., Niessen L. (2014). Real-time loop-mediated isothermal amplification (LAMP) assay for group specific detection of important trichothecene producing Fusarium species in wheat. Int. J. Food Microbiol. 177, 117–127. doi: 10.1016/j.ijfoodmicro.2014.02.010
Denschlag C., Vogel R. F., Niessen L. (2012). Hyd5 gene-based detection of the major gushing-inducing Fusarium spp. in a loop-mediated isothermal amplification (LAMP) assay. Int. J. Food Microbiol. 156, 189–196. doi: 10.1016/j.ijfoodmicro.2012.03.009
Devadas R., Lamb D. W., Simpfendorfer S., Backhouse D. (2009). Evaluating ten spectral vegetation indices for identifying rust infection in individual wheat leaves. Precis. Agric. 10, 459–470. doi: 10.1007/s11119-008-9100-2
Donoso A., Valenzuela S. (2018). In-field molecular diagnosis of plant pathogens: recent trends and future perspectives. Plant Pathol. 67, 1451–1461. doi: 10.1111/ppa.12859
Dooley H., Shaw M. W., Mehenni-Ciz J., Spink J., Kildea S. (2016). Detection of Zymoseptoria tritici SDHI-insensitive field isolates carrying the SdhC-H152R and SdhD-R47W substitutions. Pest Manage. Sci. 72, 2203–2207. doi: 10.1002/ps.4269
Duan Y., Zhang X., Ge C., Wang Y., Cao J., Jia X., et al. (2014). Development and application of loop-mediated isothermal amplification for detection of the F167Y mutation of carbendazim-resistant isolates in Fusarium graminearum. Sci. Rep. 4, 7094. doi: 10.1038/srep07094
Duba A., Goriewa-Duba K., Wachowska U. (2018). A review of the interactions between wheat and wheat pathogens: Zymoseptoria tritici, Fusarium spp. and Parastagonospora nodorum. Int. J. Mol. Sci. 19, 1138. doi: 10.3390/ijms19041138
Dzhavakhiya V. G., Voinova T. M., Popletaeva S. B., Statsyuk N. V., Limantseva L. A., Shcherbakova L. A. (2016). Effect of various compounds blocking the colony pigmentation on the aflatoxin B1 production by Aspergillus flavus. Toxins 8, 313. doi: 10.3390/toxins8110313
Emebiri L., Singh P. K., Tan M. K., Fuentes-Davila G., He X., Singh R. P. (2019a). Reaction of Australian durum, common wheat and triticale genotypes to karnal bunt (Tilletia indica) infection under artificial inoculation in the field. Crop Pasture Sci. 70, 107–112. doi: 10.1071/CP18235
Emebiri L., Singh S., Tan M. K., Singh P. K., Fuentes-Dávila G., Ogbonnaya F. (2019b). Unravelling the complex genetics of karnal bunt (Tilletia indica) resistance in common wheat (Triticum aestivum) by genetic linkage and genome-wide association analyses. G3 Genes Genomes Genet. 9, 1437–1447. doi: 10.1534/g3.119.400103
Fahlgren N., Gehan M. A., Baxter I. (2015). Lights, camera, action: high-throughput plant phenotyping is ready for a close-up. Curr. Opin. Plant Biol. 24, 93–99. doi: 10.1016/j.pbi.2015.02.006
FAO (2004). Worldwide regulations for mycotoxyns in food and feed in 2003. Food and nutrition paper 81. Food and Nutrition Paper. Available at: https://www.fao.org/3/y5499e/y5499e00.htm#Contents
FAO (2021). New standards to curb the global spread of plant pests and diseases. Available at: https://www.fao.org/news/story/en/item/1187738/icode/.
Femenias A., Gatius F., Ramos A. J., Sanchis V., Marín S. (2020). Use of hyperspectral imaging as a tool for Fusarium and deoxynivalenol risk management in cereals: a review. Food Control 108, 106819. doi: 10.1016/j.foodcont.2019.106819
Feng W., Shen W., He L., Duan J., Guo B., Li Y., et al. (2016). Improved remote sensing detection of wheat powdery mildew using dual-green vegetation indices. Precis. Agric. 17, 608–627. doi: 10.1007/s11119-016-9440-2
Ferchichi A., Abbes A.B., Barra V., Farah I. R. (2022). Forecasting vegetation indices from spatio-temporal remotely sensed data using deep learning-based approaches: a systematic literature review. Ecol. Inform. 68, 101552. doi: 10.1016/j.ecoinf.2022.101552
Fernández-Campos M., Huang Y. T., Jahanshahi M. R., Wang T., Jin J., Telenko D. E. P., et al. (2021). Wheat spike blast image classification using deep convolutional neural networks. Front. Plant Sci. 12. doi: 10.3389/fpls.2021.673505
Figueroa M., Hammond-Kosack K. E., Solomon P. S. (2018). A review of wheat diseases–a field perspective. Mol. Plant Pathol. 19, 1523–1536. doi: 10.1111/mpp.12618
Fiorani F., Schurr U. (2013). Future scenarios for plant phenotyping. Annu. Rev. Plant Biol. 64, 267–291. doi: 10.1146/annurev-arplant-050312-120137
Fire A., Xu S. Q. (1995). Rolling replication of short DNA circles. Proc. Natl. Acad. Sci. U. S. A. 92, 4641–4645. doi: 10.1073/pnas.92.10.4641
Foley J. A., Ramankutty N., Brauman K. A., Cassidy E. S., Gerber J. S., Johnston M., et al. (2011). Solutions for a cultivated planet. Nature 478, 337–342. doi: 10.1038/nature10452
Fones H., Gurr S. (2015). The impact of Septoria tritici blotch disease on wheat: An EU perspective. Fungal Genet. Biol. 79, 3–7. doi: 10.1016/j.fgb.2015.04.004
Francesconi S., Harfouche A., Maesano M., Balestra G. M. (2021). UAV-based thermal, RGB imaging and gene expression analysis allowed detection of fusarium head blight and gave new insights into the physiological responses to the disease in durum wheat. Front. Plant Sci. 12. doi: 10.3389/fpls.2021.628575
Franke J., Menz G. (2007). Multi-temporal wheat disease detection by multi-spectral remote sensing. Precis. Agric. 8, 161–172. doi: 10.1007/s11119-007-9036-y
Frisvad J. C., Hubka V., Ezekiel C. N., Hong S. B., Nováková A., Chen A. J., et al. (2019). Taxonomy of Aspergillus section Flavi and their production of aflatoxins, ochratoxins and other mycotoxins. Stud. Mycol. 93, 1–63. doi: 10.1016/j.simyco.2018.06.001
Gao Y., Tan M. K., Zhu Y. G. (2016). Rapid and specific detection of Tilletia indica using loop-mediated isothermal DNA amplification. Australas. Plant Pathol. 45, 361–367. doi: 10.1007/s13313-016-0422-7
Gilbert J., Tekauz A. (2000). Review: recent developments in research on fusarium head blight of wheat in Canada. Can. J. Plant Pathol. 22, 1–8. doi: 10.1080/07060660009501155
Gill P., Ghaemi A. (2008). Nucleic acid isothermal amplification technologies - a review. Nucleosides Nucleotides Nucleic Acids 27, 224–243. doi: 10.1080/15257770701845204
Gill T., Gill S. K., Saini D. K., Chopra Y., de Koff J. P., Sandhu K. S. (2022). A comprehensive review of high throughput phenotyping and machine learning for plant stress phenotyping. Phenomics 2, 156–183. doi: 10.1007/s43657-022-00048-z
Giovati L., Magliani W., Ciociola T., Santinoli C., Conti S., Polonelli L. (2015). AFM1 in milk: physical, biological, and prophylactic methods to mitigate contamination. Toxins (Basel). 7, 4330–4349. doi: 10.3390/toxins7104330
Glawe D. A. (2008). The powdery mildews: a review of the world’s most familiar (yet poorly known) plant pathogens. Annu. Rev. Phytopathol. 46, 27–51. doi: 10.1146/annurev.phyto.46.081407.104740
Gongora-Canul C., Salgado J. D., Singh D., Cruz A. P., Cotrozzi L., Couture J., et al. (2020). Temporal dynamics of wheat blast epidemics and disease measurements using multispectral imagery. Phytopathology 110, 393–405. doi: 10.1094/PHYTO-08-19-0297-R
Goulart A. C. P., Sousa P. G., Urashima A. S. (2007). Damages in wheat caused by infection of Pyricularia grisea. Summa Phytopathol. 33, 358–363. doi: 10.1590/S0100-54052007000400007
Graeff S., Link J., Claupein W. (2006). Identification of powdery mildew (Erysiphe graminis sp. tritici) and take-all disease (Gaeumannomyces graminis sp. tritici) in wheat (Triticum aestivum l.) by means of leaf reflectance measurements. Cent. Eur. J. Biol. 1, 275–288. doi: 10.2478/s11535-006-0020-8
Gunupuru L. R., Perochon A., Doohan F. M. (2017). Deoxynivalenol resistance as a component of FHB resistance. Trop. Plant Pathol. 42, 175–183. doi: 10.1007/s40858-017-0147-3
Gupta P. K., Chand R., Vasistha N. K., Pandey S. P., Kumar U., Mishra V. K., et al. (2018). Spot blotch disease of wheat: the current status of research on genetics and breeding. Plant Pathol. 67, 508–531. doi: 10.1111/ppa.12781
Gurjar M. S., Aggarwal R., Jogawat A., Kulshreshtha D., Sharma S., Solanke A. U., et al. (2019). De novo genome sequencing and secretome analysis of Tilletia indica inciting karnal bunt of wheat provides pathogenesis-related genes. 3 Biotech. 9 (6), 2019. doi: 10.1007/s13205-019-1743-3
Gustafson P., Raskina O., Ma X., Nevo E. (2009). “Wheat evolution, domestication, and improvement,”. Wheat Sci. Trade 5–30, 5–30. doi: 10.1002/9780813818832.ch1
Hariharan G., Prasannath K. (2021). Recent advances in molecular diagnostics of fungal plant pathogens: a mini review. Front. Cell. Infect. Microbiol. 10. doi: 10.3389/fcimb.2020.600234
Hartmann A., Czauderna T., Hoffmann R., Stein N., Schreiber F. (2011). HTPheno: an image analysis pipeline for high-throughput plant phenotyping. BMC Bioinf. 12, 148. doi: 10.1186/1471-2105-12-148
Hayes L. E., Sackett K. E., Mundt C. C., Anderson N. P., Flowers M. D. (2016). Evidence of selection for fungicide resistance in Zymoseptoria tritici populations on wheat in Western Oregon. Plant Dis. 100, 483–489. doi: 10.1094/PDIS-02-15-0214-RE
Huang W., Guan Q., Luo J., Zhang J., Zhao J., Liang D., et al. (2014). New optimized spectral indices for identifying and monitoring winter wheat diseases. IEEE J. Sel. Top. Appl. Earth Obs. Remote Sens. 7, 2516–2524. doi: 10.1109/JSTARS.2013.2294961
Huang W., Lamb D. W., Niu Z., Zhang Y., Liu L., Wang J. (2007). Identification of yellow rust in wheat using in-situ spectral reflectance measurements and airborne hyperspectral imaging. Precis. Agric. 8, 187–197. doi: 10.1007/s11119-007-9038-9
Huang C., Sun Z., Yan J., Luo Y., Wang H., Ma Z. (2011). Rapid and precise detection of latent infections of wheat stripe rust in wheat leaves using loop-mediated isothermal amplification. J. Phytopathol. 159, 582–584. doi: 10.1111/j.1439-0434.2011.01806.x
Huerta-Espino J., Singh R., Crespo-Herrera L. A., Villaseñor-Mir H. E., Rodriguez-Garcia M. F., Dreisigacker S., et al. (2020). Adult plant slow rusting genes confer high levels of resistance to rusts in bread wheat cultivars from Mexico. Front. Plant Sci. 11. doi: 10.3389/fpls.2020.00824
Huerta-Espino J., Singh R. P., Germán S., McCallum B. D., Park R. F., Chen W. Q., et al. (2011). Global status of wheat leaf rust caused by puccinia triticina. Euphytica 179, 143–160. doi: 10.1007/s10681-011-0361-x
Iimura K., Furukawa T., Yamamoto T., Negishi L., Suzuki M., Sakuda S. (2017). The mode of action of cyclo(L-Ala-L-Pro) in inhibiting aflatoxin production of Aspergillus flavus. Toxins 9, 9070219. doi: 10.3390/toxins9070219
Iquebal M. A., Mishra P., Maurya R., Jaiswal S., Rai A., Kumar D. (2021). Centenary of soil and air borne wheat karnal bunt disease research: A review. Biol. (Basel). 10, 1152. doi: 10.3390/biology10111152
Islam M. T., Croll D., Gladieux P., Soanes D. M., Persoons A., Bhattacharjee P., et al. (2016). Emergence of wheat blast in Bangladesh was caused by a south American lineage of Magnaporthe oryzae. BMC Biol. 14, 1–11. doi: 10.1186/s12915-016-0309-7
Islam M. T., Gupta D. R., Hossain A., Roy K. K., He X., Kabir M. R., et al. (2020). Wheat blast: a new threat to food security. Phytopathol. Res. 2, 28. doi: 10.1186/s42483-020-00067-6
Ivanov A. V., Safenkova I. V., Zherdev A. V., Dzantiev B. B. (2021). The potential use of isothermal amplification assays for in-field diagnostics of plant pathogens. Plants 10, 2424. doi: 10.3390/plants10112424
Jansen C., von Wettstein D., Schafer W., Kogel K.-H., Felk A., Maier F. J. (2005). Infection patterns in barley and wheat spikes inoculated with wild-type and trichodiene synthase gene disrupted Fusarium graminearum. Proc. Natl. Acad. Sci. 102, 16892–16897. doi: 10.1073/pnas.0508467102
Jones D. R. (2007). Arguments for a low risk of establishment of karnal bunt disease of wheat in Europe. Eur. J. Plant Pathol. 118, 93–104. doi: 10.1007/s10658-006-9097-1
Jones H. G., Serraj R., Loveys B. R., Xiong L., Wheaton A., Price A. H. (2009). Thermal infrared imaging of crop canopies for the remote diagnosis and quantification of plant responses to water stress in the field. Funct. Plant Biol. 36, 978–989. doi: 10.1071/FP09123
Kang H., Peng Y., Hua K., Deng Y., Bellizzi M., Gupta D. R., et al. (2021). Rapid detection of wheat blast pathogen Magnaporthe oryzae triticum pathotype using genome-specific primers and Cas12a-mediated technology. Engineering 7, 1326–1335. doi: 10.1016/j.eng.2020.07.016
Kazan K., Gardiner D. M. (2018). Transcriptomics of cereal–Fusarium graminearum interactions: what we have learned so far. Mol. Plant Pathol. 19, 764–778. doi: 10.1111/mpp.12561
Khan R., Ghazali F. M., Mahyudin N. A., Samsudin N. I. P. (2021). Biocontrol of aflatoxins using non-aflatoxigenic aspergillus flavus: A literature review. J. Fungi 7 (381), 2–17. doi: 10.3390/jof7050381
Khan M. K., Pandey A., Athar T., Choudhary S., Deval R., Gezgin S., et al. (2020). Fusarium head blight in wheat: Contemporary status and molecular approaches. 3 Biotech. 10. doi: 10.1007/s13205-020-2158-x
Khushboo S. S., Gupta V., Pandit D., Abrol S., Choskit D., Farooq S., et al. (2021). Epidemiology of stripe rust of wheat: a review. Int. J. Curr. Microbiol. Appl. Sci. 10, 1158–1172. doi: 10.20546/ijcmas.2021.1001.140
King K. M., Hawkins N. J., Atkins S., Dyer P. S., West J. S., Fraaije B. A. (2019). First application of loop-mediated isothermal amplification (LAMP) assays for rapid identification of mating type in the heterothallic fungus Aspergillus fumigatus. Mycoses 62, 812–817. doi: 10.1111/myc.12959
Koc A., Odilbekov F., Alamrani M., Henriksson T., Chawade A. (2022). Predicting yellow rust in wheat breeding trials by proximal phenotyping and machine learning. Plant Methods 18, 1–11. doi: 10.1186/s13007-022-00868-0
Kohli M. M., Mehta Y. R., Guzman E., de Viedma L., Cubilla L. E. (2011). Pyricularia blast-a threat to wheat cultivation. Czech J. Genet. Plant Breed. 47, 130–134. doi: 10.17221/3267-cjgpb
Kolmer J. A. (2005). Tracking wheat rust on a continental scale. Curr. Opin. Plant Biol. 8, 441–449. doi: 10.1016/j.pbi.2005.05.001
Kolmer J. (2013). Leaf rust of wheat: Pathogen biology, variation and host resistance. Forests 4, 70–84. doi: 10.3390/f4010070
Komura R., Kawakami T., Nakajima K., Suzuki H., Nakashima C. (2018). Simultaneous detection of benzimidazole-resistant strains of fusarium head blight using the loop-mediated isothermal amplification-fluorescent loop primer method. J. Gen. Plant Pathol. 84, 247–253. doi: 10.1007/s10327-018-0788-1
Konanz S., Kocsányi L., Buschmann C. (2014). Advanced multi-color fluorescence imaging system for detection of biotic and abiotic stresses in leaves. Agriculture 4, 79–95. doi: 10.3390/agriculture4020079
Kuckenberg J., Tartachnyk I., Noga G. (2009). Temporal and spatial changes of chlorophyll fluorescence as a basis for early and precise detection of leaf rust and powdery mildew infections in wheat leaves. Precis. Agric. 10, 34–44. doi: 10.1007/s11119-008-9082-0
Kumari P., Maurya S., Kumar L., Pandia S. (2020). Karnal bunt disease a major threatening to wheat crop: A review. Int. J. Appl. Res. 6, 157–160.
Kumar S., Röder M. S., Singh R. P., Kumar S., Chand R., Joshi A. K., et al. (2016). Mapping of spot blotch disease resistance using NDVI as a substitute to visual observation in wheat (Triticum aestivum l.). Mol. Breed. 36, 1–11. doi: 10.1007/s11032-016-0515-6
Kumar S., Singroha G., Singh G. P., Sharma P. (2021). Karnal bunt of wheat: etiology, breeding and integrated management. Crop Prot. 139, 105376. doi: 10.1016/j.cropro.2020.105376
Lau H. Y., Botella J. R. (2017). Advanced DNA-based point-of-care diagnostic methods for plant diseases detection. Front. Plant Sci. 8 2016. doi: 10.3389/fpls.2017.02016
Leonardo S., Toldrà A., Campàs M. (2021). Biosensors based on isothermal DNA amplification for bacterial detection in food safety and environmental monitoring. Sensors 21, 602. doi: 10.3390/s21020602
Leonard K. J., Szabo L. J. (2005). Stem rust of small grains and grasses caused by Puccinia graminis. Mol. Plant Pathol. 6, 99–111. doi: 10.1111/j.1364-3703.2005.00273.x
Le D. T., Vu N. T. (2017). Progress of loop-mediated isothermal amplification technique in molecular diagnosis of plant diseases. Appl. Biol. Chem. 60, 169–180. doi: 10.1007/s13765-017-0267-y
Liang D., Liu N., Zhang D., Zhao J., Lin F., Huang L., et al. (2017). Discrimination of powdery mildew and yellow rust of winter wheat using high-resolution hyperspectra and imageries. Infrared Laser Eng. 46, 1–9. doi: 10.3788/IRLA201746.0138004
Li Q. Y., Xu Q. Q., Jiang Y. M., Niu J. S., Xu K. G., He R. S. (2019). The correlation between wheat black point and agronomic traits in the north China plain. Crop Prot. 119, 17–23. doi: 10.1016/j.cropro.2019.01.004
Li Q., Xu K., Wang S., Li M., Jiang Y., Liang X., et al. (2020). Enzymatic browning in wheat kernels produces symptom of black point caused by Bipolaris sorokiniana. Front. Microbiol. 11. doi: 10.3389/fmicb.2020.526266
Li L., Zhang Q., Huang D. (2014). A review of imaging techniques for plant phenotyping. Sensors (Switzerland) 14, 20078–20111. doi: 10.3390/s141120078
López M. M., Bertolini E., Olmos A., Caruso P., Gorris M. T., Llop P., et al. (2003). Innovative tools for detection of plant pathogenic viruses and bacteria. Int. Microbiol. 6, 233–243. doi: 10.1007/s10123-003-0143-y
Magallanes López A. M., Simsek S. (2021). Pathogens control on wheat and wheat flour: a review. Cereal Chem. 98, 17–30. doi: 10.1002/cche.10345
Mahlein A. K. (2016). Plant disease detection by imaging sensors – parallels and specific demands for precision agriculture and plant phenotyping. Plant Dis. 100, 241–254. doi: 10.1094/PDIS-03-15-0340-FE
Mahlein A.-K., Alisaac E., Al Masri A., Behmann J., Dehne H.-W., Oerke E.-C. (2019). Comparison and combination of thermal, fluorescence, and hyperspectral imaging for monitoring fusarium head blight of wheat on spikelet scale. Sensors 19, 2281. doi: 10.3390/s19102281
Mahlein A. K., Kuska M. T., Behmann J., Polder G., Walter A. (2018). Hyperspectral sensors and imaging technologies in phytopathology: State of the art. Annu. Rev. Phytopathol. 56, 535–558. doi: 10.1146/annurev-phyto-080417-050100
Maid M. K., Deshmukh R. R. (2018). Hyperspectral analysis of wheat leaf rust (WLR) disease: A review. Int. J. Comput. Sci. Eng. 6, 215–219. doi: 10.26438/ijcse/v6i1.215219
Malaker P. K., Barma N. C. D., Tiwari T. P., Collis W. J., Duveiller E., Singh P. K., et al. (2016). First report of wheat blast caused by Magnaporthe oryzae pathotype Triticum in Bangladesh. Plant Dis. 100, 2330. doi: 10.1094/pdis-05-16-0666-pdn
Malakhov D. (2021). The septoria leaf blotch of wheat in central Kazakhstan: prognosis, evaluation and monitoring with remotely sensed data. J. Geoinformatics Environ. Res. 2, 28–44. doi: 10.38094/jgier2124
Manjunatha C., Sharma S., Kulshreshtha D., Gupta S., Singh K., Bhardwaj S. C., et al. (2018). Rapid detection of Puccinia triticina causing leaf rust of wheat by PCR and loop mediated isothermal amplification. PLoS One 13, e0196409. doi: 10.1371/journal.pone.0196409
Marino S., Alvino A. (2019). Detection of spatial and temporal variability of vegetation indices. Agronomy 9, 226. doi: 10.3390/agronomy9050226
Marino S., Alvino A. (2020). Agronomic traits analysis of ten winter wheat cultivars clustered by UAV-derived vegetation indices. Remote Sens. 12, 249. doi: 10.3390/rs12020249
Martinelli F., Scalenghe R., Davino S., Panno S., Scuderi G., Ruisi P., et al. (2015). Advanced methods of plant disease detection. a review. Agron. Sustain. Dev. 35, 1–25. doi: 10.1007/s13593-014-0246-1
Ma Z., Xie Q., Li G., Jia H., Zhou J., Kong Z., et al. (2020). Germplasms, genetics and genomics for better control of disastrous wheat fusarium head blight. Theor. Appl. Genet. 133, 1541–1568. doi: 10.1007/s00122-019-03525-8
McCallum B. D., Tekauz A. (2002). Influence of inoculation method and growth stage on fusarium head blight in barley. Can. J. Plant Pathol. 24, 77–80. doi: 10.1080/07060660109506976
McDonald B. A., Stukenbrock E. H. (2016). Rapid emergence of pathogens in agro-ecosystems: Global threats to agricultural sustainability and food security. Philos. Trans. R. Soc B Biol. Sci. 371, 20160026. doi: 10.1098/rstb.2016.0026
McMullen M., Bergstrom G., De Wolf E., Dill-Macky R., Hershman D., Shaner G., et al. (2012). Fusarium head blight disease cycle , symptoms , and impact on grain yield and quality frequency and magnitude of epidemics since 1997. Plant Dis. 96, 1712–1728. doi: 10.1094/PDIS-03-12-0291-FE
Mehta Y. R. (2014). Wheat diseases and their management (Cham: Springer). doi: 10.1007/978-3-319-06465-9
Mielniczuk E., Skwaryło-Bednarz B. (2020). Fusarium head blight, mycotoxins and strategies for their reduction. Agronomy 10, 1–26. doi: 10.3390/agronomy10040509
Mitra D. (2021). Emerging plant diseases: Research status and challenges. Emerg. Trends Plant Pathol. (Singapore: Springer), 1–17. doi: 10.1007/978-981-15-6275-4_1
Monson M. S., Cardona C. J., Coulombe R. A., Reed K. M. (2016). Hepatic transcriptome responses of domesticated and wild turkey embryos to aflatoxin B1. Toxins (Basel). 8, 16. doi: 10.3390/toxins8010016
Moya-Elizondo E. A., Rew L. J., Jacobsen B. J., Hogg A. C., Dyer A. T. (2011). Distribution and prevalence of fusarium crown rot and common root rot pathogens of wheat in Montana. Plant Dis. 95, 1099–1108. doi: 10.1094/PDIS-11-10-0795
Munns R., James R. A., Sirault X. R. R., Furbank R. T., Jones H. G. (2010). New phenotyping methods for screening wheat and barley for beneficial responses to water deficit. J. Exp. Bot. 61, 3499–3507. doi: 10.1093/jxb/erq199
Neumann M., Hallau L., Klatt B., Kersting K., Bauckhage C. (2014). Erosion band features for cell phone image based plant disease classification. in. Proc. - Int. Conf. Pattern Recognition, 3315–3320. doi: 10.1109/ICPR.2014.571
Niessen L., Vogel R. F. (2010). Detection of fusarium graminearum DNA using a loop-mediated isothermal amplification (LAMP) assay. Int. J. Food Microbiol. 140, 183–191. doi: 10.1016/j.ijfoodmicro.2010.03.036
Nilsson H. E. (1995). Remote sensing and image analysis in plant pathology. Can. J. Plant Pathol. 17, 154–166. doi: 10.1080/07060669509500707
Notomi T., Okayama H., Masubuchi H., Yonekawa T., Watanabe K., Amino N., et al. (2000). Loop-mediated isothermal amplification of DNA. Nucleic Acids Res. 28, e63. doi: 10.1093/nar/28.12.e63
Odilbekov F., Armoniené R., Henriksson T., Chawade A. (2018). Proximal phenotyping and machine learning methods to identify Septoria tritici blotch disease symptoms in wheat. Front. Plant Sci. 9. doi: 10.3389/fpls.2018.00685
Pandey V., Gupta A. K., Singh M., Pandey D., Kumar A. (2019). Complementary proteomics, genomics approaches identifies potential pathogenicity/virulence factors in Tilletia indica induced under the influence of host factor. Sci. Rep. 9, 553. doi: 10.1038/s41598-018-37810-1
Pankaj S. K., Shi H., Keener K. M. (2018). A review of novel physical and chemical decontamination technologies for aflatoxin in food. Trends Food Sci. Technol. 71, 73–83. doi: 10.1016/j.tifs.2017.11.007
Peles F., Sipos P., Győri Z., Pfliegler W. P., Giacometti F., Serraino A., et al. (2019). Adverse effects, transformation and channeling of aflatoxins into food raw materials in livestock. Front. Microbiol. 10. doi: 10.3389/fmicb.2019.02861
Peteinatos G. G., Korsaeth A., Berge T. W., Gerhards R. (2016). Using optical sensors to identify water deprivation, nitrogen shortage, weed presence and fungal infection in wheat. Agriculture 6, 24. doi: 10.3390/agriculture6020024
Pieczul K., Perek A., Kubiak K. (2018). Detection of Tilletia caries, Tilletia laevis and Tilletia controversa wheat grain contamination using loop-mediated isothermal DNA amplification (LAMP). J. Microbiol. Methods 154, 141–146. doi: 10.1016/j.mimet.2018.10.018
Piepenburg O., Williams C. H., Stemple D. L., Armes N. A. (2006). DNA Detection using recombination proteins. PLoS Biol. 4, 1115–1121. doi: 10.1371/journal.pbio.0040204
Pieruschka R., Schurr U. (2019). Plant phenotyping: past, present, and future. Plant Phenomics 2019, 1–6. doi: 10.34133/2019/7507131
Pimentel D., Zuniga R., Morrison D. (2005). Update on the environmental and economic costs associated with alien-invasive species in the united states. Ecol. Econ. 52, 273–288. doi: 10.1016/j.ecolecon.2004.10.002
Pôças I., Calera A., Campos I., Cunha M. (2020). Remote sensing for estimating and mapping single and basal crop coefficientes: A review on spectral vegetation indices approaches. Agric. Water Manage. 233, 106081. doi: 10.1016/j.agwat.2020.106081
Porras R., Miguel-Rojas C., Pérez-de-Luque A., Sillero J. C. (2022). Macro- and microscopic characterization of components of resistance against Puccinia striiformis f. sp. tritici in a collection of spanish bread wheat cultivars. Agronomy 12, 1239. doi: 10.3390/agronomy12051239
Prithiviraj B., Vikram A., Kushalappa A. C., Yaylayan V. (2004). Volatile metabolite profiling for the discrimination of onion bulbs infected by Erwinia carotovora ssp. carotovora, fusarium oxysporum and Botrytis allii. Eur. J. Plant Pathol. 110, 371–377. doi: 10.1023/B:EJPP.0000021058.81491.f8
Qiu R., Wei S., Zhang M., Li H., Sun H., Liu G., et al. (2018). Sensors for measuring plant phenotyping: a review. Int. J. Agric. Biol. Eng. 11, 1–17. doi: 10.25165/j.ijabe.20181102.2696
Qiu R., Yang C., Moghimi A., Zhang M., Steffenson B. J., Hirsch C. D. (2019). Detection of fusarium head blight in wheat using a deep neural network and color imaging. Remote Sens. 11, 2658. doi: 10.3390/rs11222658
Qostal S., Kribel S., Chliyeh M., Serghat S., KarimaSelmaoui A. O. T., Zaarati H., et al. (2019). Study of the fungal complex responsible for root rot of wheat and barley in the north-west of Morocco. Plant Arch. 19, 2143–2157.
Rani A., Donovan N., Mantri N. (2019). Review: The future of plant pathogen diagnostics in a nursery production system. Biosens. Bioelectron. 145, 111631. doi: 10.1016/j.bios.2019.111631
Ray D. K., Mueller N. D., West P. C., Foley J. A. (2013). Yield trends are insufficient to double global crop production by 2050. PLoS One 8, e66428. doi: 10.1371/journal.pone.0066428
Römer C., Bürling K., Hunsche M., Rumpf T., Noga G., Plümer L. (2011). Robust fitting of fluorescence spectra for pre-symptomatic wheat leaf rust detection with support vector machines. Comput. Electron. Agric. 79, 180–188. doi: 10.1016/j.compag.2011.09.011
Ropelewska E., Zapotoczny P. (2018). Classification of Fusarium-infected and healthy wheat kernels based on features from hyperspectral images and flatbed scanner images: A comparative analysis. Eur. Food Res. Technol. 244, 1453–1462. doi: 10.1007/s00217-018-3059-7
Sabrol H., Kumar S. (2013). An identification of wheat rust diseases in digital images: a review. Int. J. Comput. Sci. Eng. Inf. Technol. Res. 3, 85–94.
Sankaran S., Mishra A., Ehsani R., Davis C. (2010). A review of advanced techniques for detecting plant diseases. Comput. Electron. Agric. 72, 1–13. doi: 10.1016/j.compag.2010.02.007
Santiago-Felipe S., Tortajada-Genaro L. A., Puchades R., Maquieira A. (2014). Recombinase polymerase and enzyme-linked immunosorbent assay as a DNA amplification-detection strategy for food analysis. Anal. Chim. Acta 811, 81–87. doi: 10.1016/j.aca.2013.12.017
Saponari M., Manjunath K., Yokomi R. K. (2008). Quantitative detection of Citrus tristeza virus in citrus and aphids by real-time reverse transcription-PCR (TaqMan®). J. Virol. Methods 147, 43–53. doi: 10.1016/j.jviromet.2007.07.026
Saremi H., Saremi H. (2013). Isolation of the most common Fusarium species and the effect of soil solarisation on main pathogenic species in different climatic zones of Iran. Eur. J. Plant Pathol. 137, 585–596. doi: 10.1007/s10658-013-0272-x
Schaad N. W., Frederick R. D., Shaw J., Schneider W. L., Hickson R., Petrillo M. D., et al. (2003). Advances in molecular-based diagnostics in meeting crop biosecurity and phytosanitary issues. Annu. Rev. Phytopathol. 41, 305–324. doi: 10.1146/annurev.phyto.41.052002.095435
Schaad N. W., Opgenorth D., Gaush P. (2002). Real-time polymerase chain reaction for one-hour on-site diagnosis of pierce’s disease of grape in early season asymptomatic vines. Phytopathology 92, 721–728. doi: 10.1094/PHYTO.2002.92.7.721
Sedaghatjoo S., Forster M. K., Niessen L., Karlovsky P., Killermann B., Maier W. (2021). Development of a loop-mediated isothermal amplification assay for the detection of tilletia controversa based on genome comparison. Sci. Rep. 11, 11611. doi: 10.1038/s41598-021-91098-2
Sharma R. C., Duveiller E. (2006). Spot blotch continues to cause substantial grain yield reductions under resource-limited farming conditions. J. Phytopathol. 154, 482–488. doi: 10.1111/j.1439-0434.2006.01134.x
Sheikh-Ali S. I., Ahmad A., Mohd-Setapar S. H., Zakaria Z. A., Abdul-Talib N., Khamis A. K., et al. (2014). The potential hazards of aspergillus sp. in foods and feeds, and the role of biological treatment: A review. J. Microbiol. 52, 807–818. doi: 10.1007/s12275-014-4294-7
Shi Y., Huang W., Zhou X. (2017). Evaluation of wavelet spectral features in pathological detection and discrimination of yellow rust and powdery mildew in winter wheat with hyperspectral reflectance data. J. Appl. Remote Sens. 11, 26025. doi: 10.1117/1.jrs.11.026025
Shuaib F. M. B., Ehiri J., Abdullahi A., Williams J. H., Jolly P. E. (2010). Reproductive health effects of aflatoxins: a review of the literature. Reprod. Toxicol. 29, 262–270. doi: 10.1016/j.reprotox.2009.12.005
Simón M. R., Börner A., Struik P. C. (2021). Editorial: fungal wheat diseases: Etiology, breeding, and integrated management. Front. Plant Sci. 12. doi: 10.3389/fpls.2021.671060
Singh J., Aggarwal R., Gurjar M. S., Sharma S., Jain S., Saharan M. S. (2020). Identification and expression analysis of pathogenicity-related genes in Tilletia indica inciting karnal bunt of wheat. Australas. Plant Pathol. 49, 393–402. doi: 10.1007/s13313-020-00711-x
Singh C. B., Jayas D. S., Paliwal J., White N. D. G. (2007). Fungal detection in wheat using near-infrared hyperspectral imagin. Am. Soc Agric. Biol. Eng. 50, 2171–2176. doi: 10.13031/2013.24077
Singh C. B., Jayas D. S., Paliwal J., White N. D. G. (2012). Fungal damage detection in wheat using short-wave near-infrared hyperspectral and digital colour imaging. Int. J. Food Prop. 15, 11–24. doi: 10.1080/10942911003687223
Somani D., Adhav R., Prashant R., Kadoo N. Y. (2019). Transcriptomics analysis of propiconazole-treated Cochliobolus sativus reveals new putative azole targets in the plant pathogen. Funct. Integr. Genomics 19, 453–465. doi: 10.1007/s10142-019-00660-9
Song P., Wang J., Guo X., Yang W., Zhao C. (2021). High-throughput phenotyping: breaking through the bottleneck in future crop breeding. Crop J. 9, 633–645. doi: 10.1016/j.cj.2021.03.015
Sun K., Xing W., Yu X., Fu W., Wang Y., Zou M., et al. (2016). Recombinase polymerase amplification combined with a lateral flow dipstick for rapid and visual detection of schistosoma japonicum. Parasites Vectors 9, 476. doi: 10.1186/s13071-016-1745-5
Surovy M. Z., Mahmud N. U., Bhattacharjee P., Hossain M. S., Mehebub M. S., Rahman M., et al. (2020). Modulation of nutritional and biochemical properties of wheat grains infected by blast fungus Magnaporthe oryzae triticum pathotype. Front. Microbiol. 11. doi: 10.3389/fmicb.2020.01174
Tackenberg O. (2007). A new method for non-destructive measurement of biomass, growth rates, vertical biomass distribution and dry matter content based on digital image analysis. Ann. Bot. 99, 777–783. doi: 10.1093/aob/mcm009
Tang Q., Tian S., Yu N., Zhang X., Ji X., Zhai H., et al. (2016). Development and evaluation of a loop-mediated isothermal amplification method for rapid detection of Aspergillus fumigatus. J. Clin. Microbiol. 54, 950–955. doi: 10.1128/JCM.01751-15
Tembo B., Mulenga R. M., Sichilima S., M’siska K. K., Mwale M., Chikoti P. C., et al. (2020). Detection and characterization of fungus (Magnaporthe oryzae pathotype Triticum) causing wheat blast disease on rain-fed grown wheat (Triticum aestivum l.) in Zambia. PLoS One 15, e0238724. doi: 10.1371/journal.pone.0238724
Thambugala D., Menzies J. G., Knox R. E., Campbell H. L., McCartney C. A. (2020). Genetic analysis of loose smut (Ustilago tritici) resistance in sonop spring wheat. BMC Plant Biol. 20, 314. doi: 10.1186/s12870-020-02525-x
Thierry M., Chatet A., Fournier E., Tharreau D., Ioos R. (2020). A PCR, qPCR, and LAMP toolkit for the detection of the wheat blast pathogen in seeds. Plants 9, 277. doi: 10.3390/plants9020277
Tone K., Fujisaki R., Yamazaki T., Makimura K. (2017). Enhancing melting curve analysis for the discrimination of loop-mediated isothermal amplification products from four pathogenic molds: use of inorganic pyrophosphatase and its effect in reducing the variance in melting temperature values. J. Microbiol. Methods 132, 41–45. doi: 10.1016/j.mimet.2016.10.020
Twamley T., Gaffney M., Feechan A. (2019). A microbial fermentation mixture primes for resistance against powdery mildew in wheat. Front. Plant Sci. 10 1241. doi: 10.3389/fpls.2019.01241
Ubbens J. R., Stavness I. (2017). Deep plant phenomics: a deep learning platform for complex plant phenotyping tasks. Front. Plant Sci. 8. doi: 10.3389/fpls.2017.01190
Urashima A. S., Grosso C. R. F., Stabili A., Freitas E. G., Silva C. P., Netto D. C. S., et al. (2009). “Effect of magnaporthe grisea on seed germination, yield and quality of wheat,” in Advances in genetics, genomics and control of rice blast disease (Netherlands: Springer Dordrecht), 267–277. doi: 10.1007/978-1-4020-9500-9_27
Van der Heyden H., Dutilleul P., Charron J. B., Bilodeau G. J., Carisse O. (2021). Monitoring airborne inoculum for improved plant disease management. a review. Agron. Sustain. Dev. 41, 1–23. doi: 10.1007/s13593-021-00694-z
Vaughan M., Backhouse D., Del Ponte E. M. (2016). Climate change impacts on the ecology of Fusarium graminearum species complex and susceptibility of wheat to fusarium head blight: a review. World Mycotoxin J 9, 685–700. doi: 10.3920/WMJ2016.2053
Vergara-Diaz O., Kefauver S. C., Elazab A., Nieto-Taladriz M. T., Araus J. L. (2015). Grain yield losses in yellow-rusted durum wheat estimated using digital and conventional parameters under field conditions. Crop J. 3, 200–210. doi: 10.1016/j.cj.2015.03.003
Vincent M., Xu Y., Kong H. (2004). Helicase-dependent isothermal DNA amplification. EMBO Rep. 5, 795–800. doi: 10.1038/sj.embor.7400200
Wang Y., Zia-Khan S., Owusu-Adu S., Miedaner T., Müller J. (2019). Early detection of Zymoseptoria tritici in winter wheat by infrared thermography. Agriculture 9, 139. doi: 10.3390/agriculture9070139
Wang Y., Zia S., Owusu-Adu S., Gerhards R., Müller J. (2014). Early detection of fungal diseases in winter wheat by multi-optical sensors. APCBEE Proc. 8, 199–203. doi: 10.1016/j.apcbee.2014.03.027
West J. S., Bravo C., Oberti R., Lemaire D., Moshou D., McCartney H. A. (2003). The potential of optical canopy measurement for targeted control of field crop diseases. Annu. Rev. Phytopathol. 41, 593–614. doi: 10.1146/annurev.phyto.41.121702.103726
Whetton R. L., Waine T. W., Mouazen A. M. (2018). Hyperspectral measurements of yellow rust and fusarium head blight in cereal crops: Part 2: On-line field measurement. Biosyst. Eng. 167, 144–158. doi: 10.1016/j.biosystemseng.2018.01.004
White D. J., Chen W., Schroeder K. L. (2019). Assessing the contribution of ethaboxam in seed treatment cocktails for the management of metalaxyl-resistant Pythium ultimum var. ultimum in pacific Northwest spring wheat production. Crop Prot. 115, 7–12. doi: 10.1016/j.cropro.2018.08.026
Wigmann É.F., Meyer K., Cendoya E., Maul R., Vogel R. F., Niessen L. (2020). A loop-mediated isothermal amplification (LAMP) based assay for the rapid and sensitive group-specific detection of fumonisin producing Fusarium spp. Int. J. Food Microbiol. 325, 108627. doi: 10.1016/j.ijfoodmicro.2020.108627
Wójtowicz A., Piekarczyk J., Czernecki B., Ratajkiewicz H. (2021). A random forest model for the classification of wheat and rye leaf rust symptoms based on pure spectra at leaf scale. J. Photochem. Photobiol. B Biol. 223, 112278. doi: 10.1016/j.jphotobiol.2021.112278
Xiao Y., Dong Y., Huang W., Liu L., Ma H. (2021). Wheat fusarium head blight detection using UAV-based spectral and texture features in optimal window size. Remote Sens. 13, 2437. doi: 10.3390/rs13132437
Xue J., Su B. (2017). Significant remote sensing vegetation indices: a review of developments and applications. J. Sensors 2017, 1353691. doi: 10.1155/2017/1353691
Xu F., Yang G., Wang J., Song Y., Liu L., Zhao K., et al. (2018). Spatial distribution of root and crown rot fungi associated with winter wheat in the north China plain and its relationship with climate variables. Front. Microbiol. 9. doi: 10.3389/fmicb.2018.01054
Xu M., Ye W., Zeng D., Wang Y., Zheng X. (2017). Rapid diagnosis of wheat head blight caused by Fusarium asiaticum using a loop-mediated isothermal amplification assay. Australas. Plant Pathol. 46, 261–266. doi: 10.1007/s13313-017-0487-y
Yager P., Edwards T., Fu E., Helton K., Nelson K., Tam M. R., et al. (2006). Microfluidic diagnostic technologies for global public health. Nature 442, 412–418. doi: 10.1038/nature05064
Yan H., Zhang J., Ma D., Yin J. (2019). qPCR and loop mediated isothermal amplification for rapid detection of Ustilago tritici. PeerJ 2019, e7766. doi: 10.7717/peerj.7766
Yao Z., Lei Y., He D. (2019). Early visual detection of wheat stripe rust using visible/near-infrared hyperspectral imaging. Sensors 19, 952. doi: 10.3390/s19040952
Yasuhara-Bell J., Pedley K. F., Farman M., Valent B., Stack J. P. (2018). Specific detection of the wheat blast pathogen (Magnaporthe oryzae triticum) by loop-mediated isothermal amplification. Plant Dis. 102, 2550–2559. doi: 10.1094/pdis-03-18-0512-re
Yuan L., Pu R., Zhang J., Wang J., Yang H. (2016a). Using high spatial resolution satellite imagery for mapping powdery mildew at a regional scale. Precis. Agric. 17, 332–348. doi: 10.1007/s11119-015-9421-x
Yuan S., Wu B., Yu Z., Fang J., Liang N., Zhou M., et al. (2016b). The mitochondrial and endoplasmic reticulum pathways involved in the apoptosis of bursa of Fabricius cells in broilers exposed to dietary aflatoxin B1. Oncotarget 7, 65295–65306. doi: 10.18632/oncotarget.11321
Yuan L., Zhang J., Nie C., Wei L., Yang G., Wang J. (2013). Selection of spectral channels for satellite sensors in monitoring yellow rust disease of winter wheat. Intell. Autom. Soft Comput. 19, 501–511. doi: 10.1080/10798587.2013.869108
Yuan L., Zhang J., Shi Y., Nie C., Wei L., Wang J. (2014). Damage mapping of powdery mildew in winter wheat with high-resolution satellite image. Remote Sens. 6, 3611–3623. doi: 10.3390/rs6053611
Yuan L., Zhang H., Zhang Y., Xing C., Bao Z. (2017). Feasibility assessment of multi-spectral satellite sensors in monitoring and discriminating wheat diseases and insects. Optik (Stuttg). 131, 598–608. doi: 10.1016/j.ijleo.2016.11.206
Yvon M., Thébaud G., Alary R., Labonne G. (2009). Specific detection and quantification of the phytopathogenic agent “Candidatus phytoplasma prunorum”. Mol. Cell. Probes 23, 227–234. doi: 10.1016/j.mcp.2009.04.005
Zeng D., Ye W., Xu M., Lu C., Tian Q., Zheng X. (2017). Rapid diagnosis of soya bean root rot caused by Fusarium culmorum using a loop-mediated isothermal amplification assay. J. Phytopathol. 165, 249–256. doi: 10.1111/jph.12556
Zhang X., Han L., Dong Y., Shi Y., Huang W., Han L., et al. (2019b). A deep learning-based approach for automated yellow rust disease detection from high-resolution hyperspectral UAV images. Remote Sens. 11, 1554. doi: 10.3390/rs11131554
Zhang X., Lowe S. B., Gooding J. J. (2014c). Brief review of monitoring methods for loop-mediated isothermal amplification (LAMP). Biosens. Bioelectron. 61, 491–499. doi: 10.1016/j.bios.2014.05.039
Zhang N., Luo J., Rossman A. Y., Aoki T., Chuma I., Crous P. W., et al. (2016). Generic names in Magnaporthales. IMA Fungus 7, 155–159. doi: 10.5598/imafungus.2016.07.01.09
Zhang N., Pan Y., Feng H., Zhao X., Yang X., Ding C., et al. (2019a). Development of fusarium head blight classification index using hyperspectral microscopy images of winter wheat spikelets. Biosyst. Eng. 186, 83–99. doi: 10.1016/j.biosystemseng.2019.06.008
Zhang J. C., Pu R.l., Wang J.h., Huang W.j., Yuan L., Luo J.h. (2012). Detecting powdery mildew of winter wheat using leaf level hyperspectral measurements. Comput. Electron. Agric. 85, 13–23. doi: 10.1016/j.compag.2012.03.006
Zhang J., Pu R., Yuan L., Wang J., Huang W., Yang G. (2014a). Monitoring powdery mildew of winter wheat by using moderate resolution multi-temporal satellite imagery. PLoS One 9, e93107. doi: 10.1371/journal.pone.0093107
Zhang D., Wang Q., Lin F., Weng S., Lei Y., Chen G., et al. (2020). New spectral classification index for rapid identification of Fusarium infection in wheat kernel. Food Anal. Methods 13, 2165–2175. doi: 10.1007/s12161-020-01829-w
Zhang J., yuan L., Pu R., Loraamm R. W., Yang G., Wang J. (2014b). Comparison between wavelet spectral features and conventional spectral features in detecting yellow rust for winter wheat. Comput. Electron. Agric. 100, 79–87. doi: 10.1016/j.compag.2013.11.001
Zhao W., Chi Y., di M., Wang T., Xu A.m., Qi R. (2021). Development and application of recombinase polymerase amplification assay for detection of Bipolaris sorokiniana. Crop Prot. 145, 105619. doi: 10.1016/j.cropro.2021.105619
Zhao J., Fang Y., Chu G., Yan H., Hu L., Huang L. (2020). Identification of leaf-scale wheat powdery mildew (Blumeria graminis f. sp. tritici) combining hyperspectral imaging and an SVM classifier. Plants 9, 936. doi: 10.3390/plants9080936
Zheng Q., Huang W., Cui X., Dong Y., Shi Y., Ma H., et al. (2019). Identification of wheat yellow rust using optimal three-band spectral indices in different growth stages. Sensors 19, 35. doi: 10.3390/s19010035
Zheng Q., Huang W., Cui X., Shi Y., Liu L. (2018). New spectral index for detecting wheat yellow rust using sentinel-2 multispectral imagery. Sensors (Switzerland) 18, 868. doi: 10.3390/s18030868
Keywords: detection, point-of-care (POC), isothermal DNA amplification, phenomics, spectral sensors, disease management, sustainable agriculture
Citation: Francesconi S (2022) High-throughput and point-of-care detection of wheat fungal diseases: Potentialities of molecular and phenomics techniques toward in-field applicability. Front. Agron. 4:980083. doi: 10.3389/fagro.2022.980083
Received: 28 June 2022; Accepted: 12 August 2022;
Published: 06 September 2022.
Edited by:
Sara Franco Ortega, University of York, United KingdomReviewed by:
Karansher Singh Sandhu, Bayer Crop Science, United StatesJonathan Spencer West, Rothamsted Research, United Kingdom
Copyright © 2022 Francesconi. This is an open-access article distributed under the terms of the Creative Commons Attribution License (CC BY). The use, distribution or reproduction in other forums is permitted, provided the original author(s) and the copyright owner(s) are credited and that the original publication in this journal is cited, in accordance with accepted academic practice. No use, distribution or reproduction is permitted which does not comply with these terms.
*Correspondence: Sara Francesconi, ZnJhbmNlc2Nvbmkuc0B1bml0dXMuaXQ=