- 1Manaaaki Whenua Landcare Research, Hamilton, New Zealand
- 2University of New England, Armidale, NSW, Australia
The adoption of new technologies and practices is fundamental to having the capacity to adapt to climate change and ameliorate resource degradation. Consequently, having the ability to predict the scale and rate of adoption by farmers of agricultural innovations is central to gauging their adaptive capacity. It is also crucial to assessing the likely compliance of farmers with change-seeking incentives and regulations. In this paper we describe a novel approach to predicting rates of adoption with respect to agricultural technologies and practices drawing on a dual-process model of consumer decision-making and a method for describing the complexity of innovations in farm systems. We tested the approach using data collected through a survey of dairy farmers in the Waikato and Waipa regions of New Zealand. In the survey we asked 200 farmers, chosen at random, about their perceptions of the complexity and relative advantage of various agricultural and resource management practices, and collected information as to how long it took them to try, and then adopt, the practices. Our results confirm that the process of forming an intention to try or adopt a technology or practice may take several months for relatively simple technologies and practices, and several years for more complex ones. Importantly, we found that novelty in terms of the originality in the components and architecture of a technology or practice does not necessarily correlate with its complexity in terms of integrating it into farm systems. This means that apparently simple technologies and practices that are promoted to reduce resource degradation can be quite difficult to integrate into farm systems and, as a consequence, the costs of integration may act as a strong deterrent to adopting them. A logical implication of our findings is that a deep understanding of the nature of the integration task is essential to anticipating how long it might take for adoption (or compliance) to occur in agriculture and, therefore, to appreciate limits on the adaptive capacity of farmers. Such an understanding requires an intimate knowledge of the, sometimes diverse, farm systems and sub-systems in which the technology or practice is to be integrated.
Introduction
The adoption of new technologies and practices is fundamental to having the capacity to adapt to climate change and ameliorate resource degradation (Zilberman et al., 2012. Price and Leviston, 2014, Westermann et al., 2018). Consequently, having the ability to predict the scale and rate of adoption of agricultural innovations by farmers is central to gauging their adaptive capacity and to evaluating the benefits to be had from relevant research, marketing, and extension programs (e.g., Benin et al., 2011, Kuehne et al., 2017; Kleinman et al., 2018; Pannell and Claassen, 2020). It is also crucial to assessing the likely response of farmers to policies offering incentives for, or compelling, the use of specific agricultural technologies and practices (e.g., Bopp et al., 2019). In principle, this ability should inform expectations of farmer compliance with regulations requiring ‘disadoption’, that is, the abandonment of practices.
Doole et al. (2019) observed that the potential for regulations such as the imposition of technology and process standards (Gunningham et al., 1998), incentives such as subsidies and discounts (Borrás & Edquist, 2013), and the dissemination of information and knowledge through extension (Anderson and Feder, 2007) to influence the scale, and rate, of the adoption of agricultural technologies and practices depended, at least partly, on the complexity of the technology or practice in question. ‘Scale of adoption’ refers to the proportion of farms in an industry for which a new technology or practice is judged by the manager to offer a ‘relative advantage’ (that is, a net benefit) compared to existing technology or practice(s) (Rogers, 2003). ‘Rate of adoption’ refers to how quickly the new technology or practice is adopted by that proportion of farms. In broad terms, a ‘simple technology or practice’ is one that is based on operating principles familiar to the farmer and is relatively easy to integrate into the farm system; a ‘complex technology or practice’ is one that is based on principles unfamiliar to the farmer and is relatively difficult to integrate into the farm system. The effort and cost involved in searching for relevant information about, understanding and evaluating, and implementing a technology or practice is greater the more complex it is (Pannell et al., 2006; Kuehne et al., 2017).
Doole et al. (2019) argued that incentives and extension have little potential to influence the scale of adoption of technologies and practices because they do not alter relative advantage.
Incentives and extension have mixed potential to influence the rate of adoption of technologies and practices depending on their complexity. Doole et al. (2019) suggested that the potential for incentives to influence the rate of adoption of simple technologies and practices is high because even a relatively small incentive can trigger trialling or offset a substantial proportion of the costs of change. With increasing complexity of innovations, this potential weakens.
The impact of complexity reverses the impact of extension on rate of adoption. By reducing the search effort and learning costs associated with adopting complex technologies and practices, extension does have the potential to influence the rate of their adoption. In contrast, there is limited potential for extension to influence the rate of adoption of simple technologies and practices, primarily because search effort and learning costs are low.
With respect to regulation, Doole et al. (2019) observed that regulation has the potential to influence the scale of adoption of both simple and complex technologies and practices by, in effect, redefining their relative advantage. Regulation also has the potential to influence rate of adoption of both simple and (especially) complex technologies and practices by restricting the time available for searching and learning. The complexity of technologies and practices is crucial, then, in understanding the role that regulations, incentives and extension can play in influencing their adoption (Rogers, 2003). However, the effects of complexity on the adoption of technologies and practices in agriculture has, relatively speaking, received limited attention in the literature (de Oca Munguia & Llewellyn, 2020).
If the effort and time involved in adopting complex agricultural innovations is greater than for less complex innovations, the intensity of the motivation, the willingness to commit effort, needed to adopt complex innovations can be expected to be greater than that needed for simpler innovations. While any innovation must be adopted only once, the magnitude, riskiness and stress associated with the prospect of a specific adoption can be expected to influence the attractiveness (relative advantage) of an innovation and the time taken to appraise and adopt (or reject). An important step, then, in predicting the rate of adoption of agricultural innovations would be to identify the impact of the complexity of agricultural innovations on the strength of motivation. This requires a method for characterising the complexity of innovations.
In this paper we describe a novel approach to predicting rates of adoption with respect to agricultural technologies and practices. The approach draws on the dual-process model of consumer decision-making (Bagozzi, 2006a; Bagozzi, 2006b) to measure motivation and a method for classifying the complexity of innovations to farm systems based on Henderson and Clark (1990).
In the next section the dual‐process model of consumer decision‐making proposed by Bagozzi (2006a) is briefly described. This is followed by a description of the classification of innovations proposed by Henderson and Clark (1990). More detailed descriptions may be found in Wright (2011) and Kaine et al. (2008), respectively. The way in which the types of innovations that these changes represent influence farmers’ motivation to change practices and technologies is then considered. We then report the results of testing this approach based on a survey of 200 dairy farmers in the Waikato and Waipa regions of New Zealand. Subsequently, the implications of the approach for predicting rates of adoption of innovations, and the role of incentives and extension in influencing those rates, are considered using the economic concept of ‘stickiness’. The ideas and methods described here could be applied to any agricultural industry in any region.
Materials and methods
Theory
A dual-process model of adoption
The material in this section draws heavily on Wright (2011) and Kaine et al. (2012).
The dual-process model proposed by Bagozzi (2006a) builds on the extensive literature linking ‘resistance to change’ (which is the opposite of ‘innovativeness’) as a personal predisposition with the likelihood and speed of adoption (Ram and Sheth, 1989; Bagozzi and Lee, 1999; Oreg, 2003). The focus in much of this literature has been on linking resistance to change to the likelihood of adoption, which is represented by the intention to adopt (see Bagozzi and Warshaw, 1990; Bagozzi, 1992). However, actual adoption involves both the intention to adopt and the translation of that intention into behaviour, which may not occur (Bagozzi and Lee, 1999).
The concept of ‘goal striving’ was developed to link intention with behaviour (Bagozzi and Dholakia, 1999; Bagozzi and Lee, 1999; Bagozzi, 2007). Consequently, the dual-process model of consumer response to innovations has two components: goal setting, which describes the process of deciding to adopt; and goal striving, which describes the process of adopting. The goal setting process provides a foundation for identifying when motivation, and the factors that influence motivation, delay adoption. The goal striving process provides a foundation for identifying when it is implementation of the decision to adopt, and the factors that influence implementation, that delays adoption.
This decomposition of the adoption process, in any context, we regard as a valuable extension of mainstream adoption process research as it explores the (otherwise) ‘black-box’ explanations such as ‘time’, ‘social communication processes’ or ‘Markov chains’ (Miller et al., 2017) for the universally recognised non-instantaneous achievement of the final scale of adoption of innovations. We are hopeful that it makes tractable the analysis, and assessment of possible deliberate influence, of the dynamic phase of implementation of choice. While choice can be modelled usefully as static, implementation cannot, in our view.
Goal setting
In the dual-process model the first process triggered by awareness of an opportunity to achieve a goal is a sequence of reflective, deliberative processes: ‘consider-imagine-appraise-decide’ (Bagozzi, 2006a). This process determines the degree of interest the decision-maker has in achieving a goal, that is, ‘goal desire’ (Wright, 2011). The absence of sufficient interest will halt any move to the evaluative, cognitive use of attitudes and norms. Presumably, the greater the time and effort envisaged in adopting an innovation, the correspondingly greater goal desire must be to provoke movement beyond goal desire to ‘goal intention’, and onto ‘behavioural desire’ and ‘behavioural intention’. Goal desire determines whether a goal will be adopted.
Bagozzi (2006a) proposes that there are five elements in the consider-imagine-appraise-decide process. Two of these elements are ‘positive’ and ‘negative anticipated emotions’. These emotions result from imagining success and failure, respectively, in goal attainment and their personal emotional consequences. These emotions could include happiness, excitement and pride or disappointment, anger and sadness. The likelihood of success or failure is not considered with anticipated emotions.
Another two elements in the consider-imagine-appraise-decide process are ‘anticipatory emotions’. These are emotional responses to the prospect of a future event. The emotions involved are hope and fear and depend in part on the perceived probability of an event, that is, success or failure, occurring (Wright, 2011). The final element in the process is ‘affect towards the means’ of striving for the goal. This is the personal emotional appeal of the methods, processes, actions and so on believed to be required to pursue the goal (Bagozzi, 2006a).
Moving through the model, goal desire is converted into some goal intention: a commitment to act to achieve the goal. This commitment to the goal must then be translated into a set of specific behaviours to be implemented, which is action or behavioural desire. The factors that moderate the translation of goal intention into behavioural desire are those identified in earlier, mainstream models of consumer behaviour such as the Theory of Planned Behaviour and its variants (Fishbein and Ajzen, 1975; Ajzen, 2001; Ajzen, 2002). Behavioural desire is then translated into specific behavioural intentions, which may be moderated by perceptions of behavioural control such as ‘self-efficacy’ (Bandura, 1997). The transformation of goal desire into behavioural intention is also moderated by self-regulation.
The process of goal setting has the potential to be complex and iterative, which means the process can take some time. Action will not proceed until the process of deciding has run its course (Wright, 2011).
Goal striving
Actual and intended behaviour are not always highly correlated (Bagozzi and Lee, 1999). The factors that influence the correlation between intended and actual behaviour are considered explicitly in the goal striving component of the dual-process model. Explicit consideration of these factors is particularly important, not only in forecasting rates of adoption but also in highlighting what opportunities, if any, there may be to influence the translation of intention into action.
The first stage in the goal striving component is the choice of how the behavioural intention will be fulfilled. Alternative means by which this may be done are evaluated in terms, particularly, of self-efficacy, ‘outcome expectancy’ and affect, which is like or dislike of a means. These elements of appraisal need to be integrated to make a choice (Wright, 2011). The second stage is ‘action planning’. Action planning ‘involves decisions as to when, where, how and how long, to act. In this stage situational cues for the timing of specific actions are contemplated’ (Wright, 2011: 18). The third stage in goal striving is the implementation of the plan, which is the commencement of action in pursuit of the goal.
The fourth stage consists of the control processes exercised over the planned actions such as tracking progress, identifying opportunities and hindrances, and revising plans accordingly. Appraisals of progress will lead to affective responses. For example, positive affect will evoke an intention to stay the course. A negative affect may evoke greater effort. Alternatively, it may result in changes in goals, a redefinition of success, or a judgement of failure and abandonment of goal striving (Bagozzi, 2006b). The final stage is the outcome: adoption, trial or failure to adopt, which will generate emotions. As they are experienced, outcomes will feedback to influence goal setting for subsequent innovations.
Complexity in agricultural innovations
The material in this section is drawn from Kaine et al. (2008, 2012) and Kaine and Wright (2017a, 2017b).
As noted above, if the effort and time involved in adopting complex agricultural innovations is greater than for less complex innovations, the intensity of the motivation needed to adopt complex innovations can be expected to be greater than that needed for simpler innovations. ‘Motivation’, in this sense, is captured in the dual‐process model by the strength of anticipated emotions, anticipatory emotions and affect towards means and can be analysed with respect to the impact on each of the complexity of agricultural innovations. This requires a method for characterising that complexity.
Wright (2011) suggested that Henderson and Clark’s (1990) framework for classifying product changes into types of innovations, which was adapted for innovations to agricultural systems by Kaine et al. (2008), was the most suitable in this context. The usefulness of the classification developed by Henderson and Clark (1990) is what it reveals about the magnitude of the impact of adoption (or abandonment) of a technology or practice in terms of disruption to system activity, the destruction of competencies, and the need for new skills and knowledge. See Kaine et al. (2008; 2012) for more detail on this aspect.
Henderson and Clark (1990) proposed that a product could be conceived of as a system – a collection of components that are linked together. They defined the components of a product as the physically distinct parts of a product. How the components are linked together to enable the product to function is the ‘architecture’ of the product. Consequently, product innovation can be conceptualised as changes to components, the linkages between them, or both. They then suggested that innovations could be categorised into increasing complexity depending on the degree of change introduced into the components and the linkages between them.
Crouch (1981) observed that farms consist of hierarchies of inter‐related sub‐systems. Each farm sub‐system is a set of components that link together in a specific way to perform a function (Kaine et al., 2008). For example, a pressure irrigation system is a generic description of a sub‐system that distributes water to plants using mechanical energy. Integrated pest management is a generic description of a sub‐system for managing pests and diseases based on the use of beneficial insects and species‐specific chemicals. Other sub‐systems include animal health, feed management and breeding management.
The components of a farm sub‐system are the physically distinct elements of the sub‐system and may include technology, techniques, and practices. The architecture of the sub‐system describes how the components are arranged or linked together to enable the sub‐system to function.
Consistent with Henderson and Clark (1990), the extent of change to the components and architecture of farm sub‐systems prompted by adopting an agricultural innovation provides a basis for describing the complexity of the innovation. The complexity of an innovation increases the greater the number of sub-systems affected when adopting it, and the more severely they are affected. Depending on the complexity of the innovation, incorporating it into a farm sub‐system will require knowledge about the sub‐system to be changed, and knowledge about how to realign other sub‐systems to accommodate that change. It follows, then, that the adoption of more complex innovations could be expected to mean that different skills and competencies will be needed with respect to (i) the relevant sub‐systems, (ii) the interactions between them and, (iii) planning the implementation of the innovation. This means that qualitative differences can be expected in the time and effort involved in implementing chosen innovations depending on their complexity, and that there will be differences in the rate of adoption (or abandonment) of innovations as a result.
This approach to describing complexity means that, as complexity increases, the decision to adopt an innovation changes category from being a decision about a change in production tactics to being a decision about making a change to technical production strategy. When changing the technical production strategy, the changes to the dimensions of output and/or input characteristics, and associated risks, are more numerous. Compared to changes in production tactics, changes to the technical production strategy are likely to be more idiosyncratic to the farm system and the decision based on projections about the new technical production strategy and the benefits it creates.
Assuming the perceived benefits associated with the innovation are sufficient to attract the farmer’s attention, detailed analysis of the adoption decision is only likely to commence once they accept the innovation is relevant to their farm system, which is based on its perceived integration potential or ‘fit’. The detailed contemplation of how, and thus whether, an innovation ‘fits’ with the farm system sets the decision maker up to promptly implement a favourable adoption decision. In other words, the investigative effort involved in forming an intention to adopt an innovation that will change the technical production strategy generates enough knowledge to act promptly on that intention (goal desire translates promptly into goal striving).
How much complexity is required to trigger this category shift will vary from farmer to farmer in line with personality factors such as self-efficacy and innovativeness (in relevant domains), and financial status, potentially in play. The weaker any of these, the lower will be the trigger to categorise the innovation as a strategic change, and the higher the perceived overall risks associated with adoption.
Returning to the dual‐process model, anticipated emotions were identified as potentially important determinants of goal desire. It may be the case that there is limited emotional content associated with adopting relatively simple innovations. If so, goal desire in relation to such innovations would depend mainly on a farmer’s perceptions of the time path and reliability of the costs and benefits of changing farm practice or technology (Wright, 2011).
In contrast, it may be the case that imagined goal achievement and goal failure have significant emotional content with relatively complex innovations. If this is the case, then the relative strength of positive and negative anticipated emotions will strongly influence goal desire. The anticipatory emotions of hope and fear, and related factors such as perceived behavioural control and anticipated difficulties in striving, are also likely to strongly affect goal desire with more complex innovations.
In short, both anticipated and anticipatory emotions may play a substantial role in changing farm practices and technologies when these changes entail substantial modification of farm sub-systems; not least because of the challenges they may pose to farmer competence. The same may be said for affect towards the means. This suggests that the number of farming sub-systems, and the magnitude of changes to them, required to adopt an innovation could be most informative about rates of adoption in farming.
The adoption of more complex innovations by farmers should require, then, greater motivation, time and effort than does the adoption of simple innovations. The greater time and effort involved in adopting them means that their adoption involves greater overall costs and risks and is thus more sensitive to the strength of the motivation to adopt them. In other words, complex innovations should be intrinsically ‘stickier’ than simple innovations (Ball and Mankiw, 1994; Szulanski, 1996; Ogawa, 1998; Sims, 1998; Bils and Klenov, 2004; Mankiw and Reis, 2006): farmers will be more resistant to adopting (or being compelled to abandon) complex innovations than simpler innovations. Stickiness impacts on the speed of adoption or abandonment (the rate) but not the ultimate extent (the scale).
Hypotheses
The concepts in the dual-process model of consumer decision-making (Bagozzi, 2006a) lead us to propose the following hypotheses concerning the adoption of agricultural technologies and practices:
1. Anticipatory emotions concerning the consequences of success (or failure) in adopting agricultural technologies and practices will depend on the anticipated contribution of the technology or practice to improved performance (relative advantage) and the risk of failure which depends on the difficulty of integrating the technology or practice into the farm system, the need for new skills, and the importance of experience.
2. Feelings about success or failure (anticipated emotions) in adopting agricultural technologies and practices will depend on the consequences of success or failure ,(relative advantage), their novelty, the challenge posed by the adoption task (which concerns the novelty of the technology or practice), the difficulty of integrating it into the farm system, the need to learn new skills and to draw on experience, and feelings about the risk of failing (anticipatory emotions).
3. The enjoyment (affect towards means) of adopting agricultural technologies and practices will depend on their contribution to improved performance (relative advantage), ease of integration, feelings about the risk of failing (anticipatory emotions), and feelings about success or failure (anticipated emotions).
4. The decision effort devoted to adopting agricultural technologies and practices will depend on their contribution to improved performance (relative advantage), ease of integration, and the need to learn new skills and to draw on experience.
The concepts in Henderson and Clark’s (1990) framework for describing the complexity of product innovations lead us to propose the following hypotheses in relation to the rate of adoption of agricultural technologies and practices:
5. The relative advantage of agricultural technologies and practices will be influenced by their novelty, ease of integration, and complexity.
6. The perceived ease with which technologies and practices can be integrated into farm systems will depend on their novelty and complexity, and the enjoyment of the adoption process.
7. The time taken to adopt agricultural technologies and practices (i.e., to move from awareness to first adoption) will depend on their relative advantage, their complexity, and the ease of integrating them into the farm system (controlling for years of experience as a dairy farmer). The greater the relative advantage the shorter the time taken to adopt; the greater the complexity the longer the time taken to adopt.
8. The perceived stickiness of agricultural technologies and practices will depend on their relative advantage, complexity, ease of integration and novelty.
Methods
To test these hypotheses, we investigated the adoption of technologies and practices in the dairy sector by conducting a telephone survey of farmers in the Waikato and Waipa, two of the major dairying regions of New Zealand. The Waikato together with the Waipa is the largest dairying area in New Zealand and the two regions have, relatively speaking, similar climate, soils, topography and dairying systems. Participation in the survey was voluntary, respondents could leave the survey at any time, and all survey questions were optional. Although the research was conducted privately, the New Zealand Association of Social Science Research code of ethics was followed.
The questionnaire used in the survey was based on Kaine et al. (2012) and was piloted using a mix of face-to-face and telephone interviews with 20 randomly selected Waikato and Waipa dairy farmers. Following some minor grammatical revisions, a market research company administered the final version of the questionnaire by telephone to 180 randomly selected Waikato and Waipa dairy farmers. The survey was conducted in November 2015.
Using a randomised starting point, a list of widely known technologies and practices (Table 1) was read to participants until they identified a technology, or practice, that they had adopted. That technology or practice was the subject for subsequent questioning. They were asked, in relation to that technology or practice, to rate their agreement with the statements in each of the scales listed below using the following categories: strongly disagree (1), disagree (2), not sure (3), agree (4) and strongly agree (5). Respondents were also asked to indicate, as best they could, the period of time from when they first heard about the technology or practice until they decided to try it, and the period of time between first trying the technology or practice and finally committing to using it, that is, adoption. Note that, in the absence of trialling, the time from hearing to first trying is also time to adoption; consequently, in such cases the time from first trying to finally committing is zero.
The same procedure was followed with respect to a second set of well-known technologies and practices (Table 2). Hence, most respondents answered questions in relation to their adoption of two of the 21 technologies or practices listed in Table 1. There were 30 instances where either the respondent stated they had not adopted any of the technologies or practices in Set A or Set B; or where respondents did not completely answer one or more of the scales. Consequently, the sample for analysis consisted of 370 complete responses.
Finally, respondents were asked to provide information on their property area, size of milking herd, age, education, and years in dairy farming.
The scales in the questionnaire (see Table 2) were intended to measure:
● The relative advantage of technologies and practices (Rogers, 2003).
● The complexity of technologies based on respondents’ perceptions of difficulty of integrating the technology or practice into their farm system.
● The complexity of the technology or practice based on the number of farm sub-systems affected, and the extent to which they were affected (Henderson and Clark, 1990).
● The novelty of the technology (Gatignon et al., 2002).
● The impact of the technology or practice on the architecture of the farm system (based on the number of farm sub-systems affected) (Henderson and Clark, 1990).
● The disruptiveness of the technology or practice based on the disturbance to the farm system and costs entailed in abandoning the technology or practice.
● The anticipated emotions, anticipatory emotions and affect towards means associated with the technology or practice (Bagozzi, 2006a, b).
● The need to acquire new skills and knowledge to adopt the technology or practice (Gatignon et al., 2002).
● The role of experience in adopting the technology or practice; and
● The decision effort (the time and energy) invested in investigating the technology or practice and deciding to adopt it (Bagozzi, 2006a, b).
Respondents indicated their agreement with each statement in a scale for each technology or practice using a five-point rating from strongly disagree (1) to strongly agree (5). Scale scores for each technology or practice were then computed for each respondent as the average of their ratings for the statements in each scale for that technology or practice. The internal consistency of the scales was tested using scale reliabilities (Carmines and Zeller, 1979).
Regression analysis was used to test the hypothesis regarding motivation and complexity. Four regressions were estimated to test hypotheses 1-4 regarding motivation. Respondents’ scores on the scales for anticipatory emotions, anticipated emotion, affect towards means and decision effort were the dependent variables. Their scores on the scales for relative advantage, difficulty of integration, the need for new skills, and the importance of experience were explanatory variables. Note that anticipatory emotions were included as an explanatory variable in the regression for anticipated emotions (hypothesis 2) and both anticipatory and anticipated emotions were included as explanatory variables in the regression for affect towards means (hypothesis 3).
Two regressions were estimated to test hypotheses 5 and 6 regarding complexity using respondents’ scores on the scales for relative advantage and for difficulty of integration as the dependent variables. Their scores on the scales such as novelty, complexity and affect towards means were explanatory variables. For hypothesis 7, two regressions were estimated using the time taken from hearing to trying, and time taken from trying to adopting were treated as dependent variables. Respondents’ scores on the scales for relative advantage, difficulty of integration and complexity were explanatory variables. In addition, the length of time respondents had been a dairy farmer was included in these regressions to control for differences in the length of time available to respondents to choose to adopt a technology or practice.
Five regressions were estimated to test the hypothesis regarding the perceived stickiness of agricultural technologies and practices (hypothesis 8) using respondents’ scores on the stickiness scale, and their scores on each item in the scale, as dependent variables. Their scores on the scales for relative advantage, novelty, difficulty of integration and complexity were explanatory variables. All statistical analyses were conducted using SPSS (IBM, 2020). As the differences between the pilot and final version of the questionnaire were grammatical only, the data collected during the pilot were included in the analysis.
Results
General
The scale reliabilities (Carmines and Zeller, 1979) are reported in Table 3. For the most part they are satisfactory, although the reliability of the scales measuring anticipatory emotions and experience are relatively weak. Consequently, we cannot be entirely confident that they measure only what we are seeking, nor that they do so consistently from farmer to farmer.
The mean ratings for novelty, integration and complexity for the various technologies and practices seem plausible with technologies and practices that, in our opinion, are relatively less complex scoring below or near the average on these measures and the more obviously complex, again in our opinion, technologies and practices scoring above average on these measures. For example, practices and technologies such as contour ploughing, slow-release fertilisers and new pasture varieties, which we regarded as probably being incremental or modular in nature, mostly had below-average scores whereas technologies and practices which we regarded as probably being architectural or radical in nature, such as changing calving period and installing feed pads, had above-average scores (see Appendix A). More formally, actions that involve switching among similar forms of a particular farm input, such as changing livestock breed or shifting to a purchased feed like palm kernel, were perceived as being simpler than those which involved substitution between inputs, such as installing a feed pad or reconfiguring the farm system by changing calving period.
Dual-process model of adoption
In Table 4 the regression results testing the hypotheses concerning the dual-process model are reported. All the regressions were statistically significant, and the signs of the coefficient estimates were as expected. Anticipatory emotions, which concern the consequences of success (or failure) in adopting the agricultural technology and practice were positively related to reward as measured by relative advantage. Anticipatory emotions were negatively related to the risk of failure, assuming the risk of failure was perceived to increase with the novelty of the technology or practice, the need for new skills and the difficulty of integrating it with the farm system, and to decrease with the importance of experience.
Anticipated emotions, feelings about successfully adopting (or failing to adopt) agricultural technologies and practices, were positively related to relative advantage and anticipatory emotions. They were also positively related to a sense of achievement in terms of understanding the novelty of the technology or practice and having to learn new skills and draw on their experience to adopt it. The strength of anticipated emotions was inversely related to the difficulty of integrating the technology or practice into the farm system, indicating, perhaps sensibly, increased emotional distancing as the challenge of integrating it increases.
Affect towards means, the enjoyment of the adoption process, adopting agricultural technologies and practices was positively related to relative advantage, feelings about successful adoption (anticipated emotions) and its consequences (anticipatory emotions). Affect towards means was negatively related to the perceived difficulty of integrating the technology or practice into the farm system.
As expected, the decision effort devoted to adopting agricultural technologies and practices was positively related to improved performance (relative advantage), the need to learn new skills, the need to draw on experience, and the difficulty of integrating the technology or practice into the farm system.
Complexity in agricultural technologies and practices
In Table 5 the regression results testing the hypotheses concerning Henderson and Clark (1990) framework are reported. All the regressions were statistically significant, and the signs of the coefficient estimates were as expected for the most part. To begin with, perceptions of the relative advantage of technologies and practices were positively related to their novelty and complexity, reflecting their potential to influence performance across the farm system. However, reflecting the costs entailed in adjusting and reconfiguring farm sub-systems, relative advantage was negatively related to perceptions of the difficulty of integrating technologies and practices into the farm system.
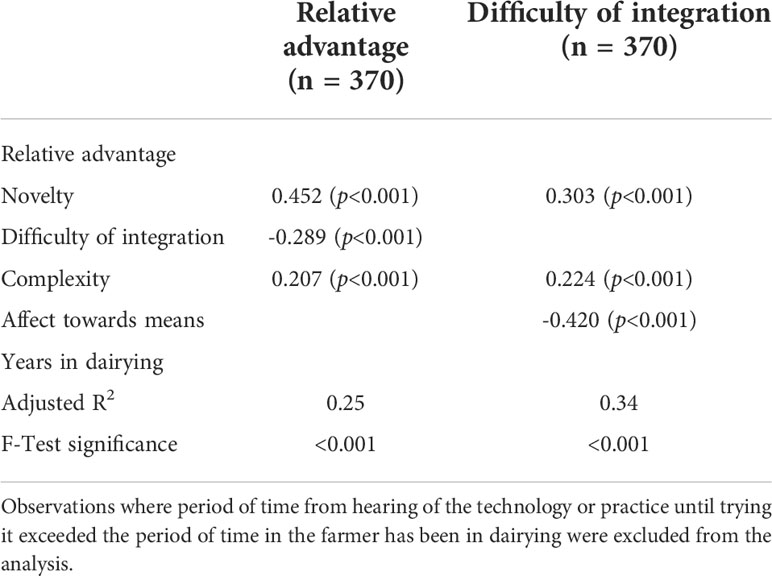
Table 5 Standardised parameter estimates for perceptions of relative advantage and difficulty of integration.
The perceived difficulty with which technologies and practices were integrated into farm systems was positively related to their novelty and complexity and negatively related to enjoyment of the adoption process, that is, affect towards means.
In Table 6 the regression results testing the hypotheses concerning Henderson and Clark (1990) framework and the time taken to try and adopt technologies and practices are reported. The regressions for the time taken to adopt agricultural technologies and practices were statistically significant but explained only a small proportion in the variance of the dependent variables (Adjusted R2 of 0.13).
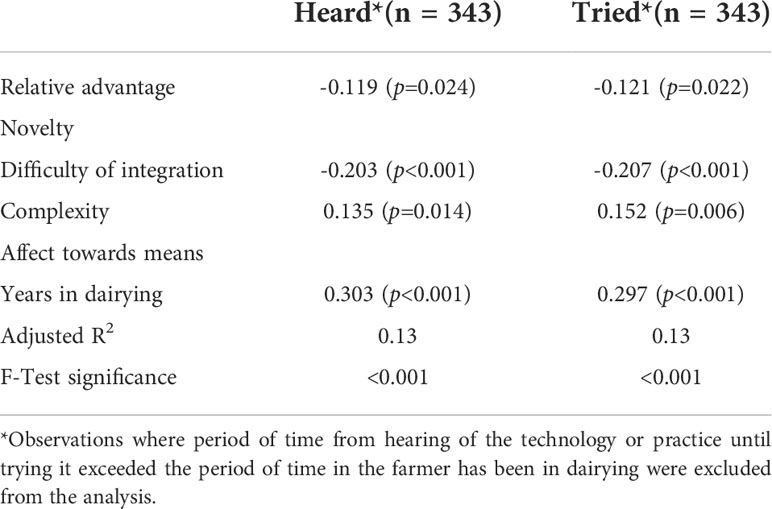
Table 6 Standardised parameter estimates for time taken to try and to adopt technologies and practices.
Controlling for respondents’ years as a farmer, the length of time between first hearing of a technology or practice and deciding to try it increased with its complexity and decreased with relative advantage and the difficulty of integrating it into the farm system. Similarly, controlling for respondents’ years as a farmer, the length of time between first trying a technology or practice and committing to it increased with its complexity and decreased with relative advantage and the difficulty of integrating it into the farm system.
The negative coefficient on perceptions of the integration task suggests that, the more difficult is the task of integrating the technology or practice adoption into the farm system, the shorter the time taken to try it, and adopt it. Note that trying and adopting are influenced by goal striving as well as goal desire. This means the time taken to try and to adopt is influenced by factors that influence action planning and implementation (Bagozzi, 2006a). Action planning encompasses when, where, how and how long, to act (Wright, 2011: 18). Consequently, the negative relationship between perceptions of the difficulty of the integration task with respect to a technology or practice and the time taken to try and to adopt it, may reflect the effect of the difficulty of the integration task on goal striving (rather than goal desire).
The more difficult the integration task, the less likely the technology or practice can be easily and inexpensively trialled because:
● there is little, if any, scope to adopt the technology or practice in piecemeal or incremental fashion, and,
● until the integration task is complete the benefits of the new technology or practice cannot be realised.
Consequently, the respondent, having sufficient goal desire to form an intention to adopt (a process that has likely involved close consideration of the integration process), is prompted to translate that intention into action more quickly the greater the difficulty of the integration task. The inability to trial technologies or practices that are particularly difficult to integrate into the farm system forces the pace of implementation. Converting from furrow irrigation to mini-sprinkler irrigation in horticulture may be examples (Kaine et al., 2008).
Stickiness in agricultural technologies and practices
In Table 7 the regression results testing the hypotheses concerning ‘stickiness’ using the scale formulated by Kaine and Wright (2017b) are reported. Because more complex innovations should require greater motivation, time and effort to adopt than simple innovations we expected that farmers would be more resistant to abandoning more complex innovations than they would simpler innovations. In other words, more complex technologies and practices would be stickier than less complex technologies and practices.
Contrary to expectations, the perceived stickiness of agricultural technologies and practices only had a statistically significant relationship with relative advantage and novelty. We investigated further by estimating regressions for each of the statements in the ‘stickiness’ scale separately. The results indicate that the factors that influence perceptions about the stickiness of technologies and practices vary depending on the circumstances prompting a technology or practice to be abandoned. For example, perceptions of the emotional upset and cost of having to revert back to a previous technology or practice were related to perceptions of relative advantage and novelty. The cost of returning to a previously used practice was also related to the complexity of the practice being abandoned. The disruptiveness of replacing a technology or practice was related to both relative advantage and the difficulty of integrating it with the farm system. The disruptiveness of having to simply stop using a technology or practice (perhaps in response to regulation) was only related to relative advantage.
Discussion
Overall, the results regarding goal desire were consistent with our hypotheses. Greater relative advantage was associated with greater satisfaction with means, greater confidence that adoption will be successful, and greater emotional engagement with the outcome, be it success or failure. Consequently, relative advantage was positively associated with affect towards means and decision effort. That is to say, the greater the effort invested in deciding to adopt a technology or practice, the greater was emotional engagement with the outcome. In terms of adoption, greater relative advantage shortened the time to trying and adopting technologies and practices and was associated with greater ‘stickiness’.
Perceptions about the difficulty of the task of integrating technology and practices into the farm system were associated with lower satisfaction with means, less confidence that adoption will be successful and lower emotional engagement with the outcome, but greater decision effort. That is to say, the greater the effort involved in integrating a technology or practice into the farm system, the greater the effort invested in decision-making but the lower was emotional engagement with the outcome. In terms of adoption, increasing levels of difficulty with integration shortened the time to trying and adopting technologies and practices. The disruption associated with having to replace technologies was positively associated with greater difficulty in integrating technologies and practices.
Complexity indirectly influences goal desire through its effect on the relative advantage offered by a technology or practice, its effect on the difficulty of the integration task, and its correlation with novelty. Complexity had a direct effect on the time taken to try and to adopt technologies and practices, with greater complexity lengthening the time taken to complete the adoption process. Greater complexity was associated with greater cost at having to abandon a technology or practice. Complexity was not correlated with relative advantage, a reassuring result as the relative advantage of a technology or practice should relate to its superiority over the technology or practice it replaces, not its inherent complexity.
The greater the novelty of the technology or practice the greater was farmers’ emotional engagement with the outcome. Greater novelty increased expectations of relative advantage, but also increased the perceived difficulty of the integration task. Novelty indirectly influences the time taken to try and to adopt technologies and practices through its influence on relative advantage, complexity, the need for new skills, the difficulty of the integration task and the need to draw on experience. Greater novelty was also associated with greater cost and emotional upset at having to abandon a technology or practice.
Our results confirm that the adoption of technologies and practices in farming is complicated and slow (Burton et al., 2003; Lamb et al., 2008; Khataza et al., 2018, Lowenberg‐DeBoer et al., 2019). The process of gathering information sufficient to motivate the formation of an intention (goal desire) to try or adopt may take several months for relatively simple technologies and practices, and several years for more complex ones. The same can be said regarding the translation of intention into action (goal striving).
Our results have several implications for agricultural and natural resource policy and extension. First, we found significant positive relationships between the strength of anticipated emotions, anticipatory emotions and affect towards means. We also found that perceptions of relative advantage and difficulty of integration influenced each of these. This means that, in the right circumstances, goal setting might be responsive to extension efforts (Loock et al., 2013). Consequently, extension may influence the rate of adoption via two routes: contributing to goal setting through the provision of information; and facilitating goal striving through the provision of information and training (Bagozzi, 2007).
A second implication concerns the importance of understanding that, in terms of farm systems, complexity has more to do with the number of farm sub-systems affected by adopting a new technology or practice, and the magnitude of the effects on them, than it has to do with its novelty. Novelty in terms of the originality in the components and architecture of an innovation is only part of the story. Hence, promoting adoption (or compliance) depends on providing information and advice about how to best reconfigure farm systems and sub-systems as well as providing information about the technology or practice itself (Grieshop et al., 1988). This means a deep understanding of the nature of the integration task is essential to anticipating how long it might take for adoption (or compliance) to occur and, as a consequence, to appreciate the limitations on the adaptive capacity of farmers (Rodriguez et al., 2009; Vanlauwe et al., 2015; Jayne et al., 2019). Such an understanding requires an intimate knowledge of the, sometimes diverse, farm systems and sub-systems in which the technology or practice is to be integrated. Therefore, extension staff, and those who advise policy makers, must have an intimate knowledge of, and familiarity with, farm systems in all their diversity as well as the technology or practice of interest. Such knowledge can only come from experience.
This reasoning aligns with the justifications for participatory approaches to research and innovation in agriculture (Okali et al., 1994, Lacombe et al., 2018). The involvement of farmers in agricultural research is intended, fundamentally, to improve the likelihood of identifying and developing innovations that will offer a relative advantage in the farming contexts of interest. The criteria employed to recruit farmer participants is critical to success as the characteristics of the resulting innovations will be shaped by the participants’ farm systems (Okali et al., 1994). Given that there is relevant diversity in farm systems, extension staff must be familiar with that diversity if they are to contribute to scaling-up the products of participatory research by usefully assisting farmers to adapt their farm systems/modify the innovation, to suit their particular context (Van De Fliert et al., 2000; Snapp and Heong, 2013; Westermann et al., 2018).
Acquiring a sound knowledge of, and familiarity with, a variety of farm systems and sub-systems requires time and effort; it can only come from extensive experience with them. This means that extension staff and advisors who only interact with farmers regarding a specific sub-system may not have the knowledge and skills to provide reliable support to farmers, or reliable advice to policy makers, about the adoption of complex technologies and practices. This suggests that it is important to appropriately resource, and avoid rapid turnover among, staff who interact with farmers in those public and private organisations who rely on such interactions to acquire information about farming for guidance (Russell et al., 2019, Mkuki & Msuya 2020).
Relatedly, when contemplating the adoption of complex technologies and practices, farmers are unlikely to trust the expertise of extension staff who have limited experience, or who mainly interact with farmers regarding a specific sub-system. Farmers are, arguably justifiably, likely to discount, if not ignore, their suggestions.
Another implication relates to the fact that, in farm systems, novelty in terms of the originality in the components and architecture of an innovation does not necessarily correlate with complexity in terms of integrating technologies and practices into farm systems. This means that apparently simple innovations can be quite difficult to integrate into farm systems. As a consequence, the costs of integration may act as a strong deterrent to adopting simple technologies or practices, such as fencing to keep stock out of waterways or reducing the use of nitrogen fertiliser in winter, unless they offer a substantial relative advantage. In the absence of a substantial relative advantage, such simple innovations may not stimulate sufficient goal desire to create an intention to adopt. This implies that the adoption of ‘simple’ practices that have been advocated to improve natural resource management on farms (thereby reducing natural resource degradation), such as fencing to improve water quality by excluding stock from waterways, is not as straightforward as it might seem to those who are unfamiliar with farming systems.
While the rate of adoption of apparently simple technologies and practices will be relatively slow when they are difficult to integrate into farm systems, the rate of adoption should be quicker when they can be easily and inexpensively integrated into the farm system (e.g., slow-release nitrogen fertiliser). This suggests, from the perspective of natural resource policy, that setting performance standards for natural resource management on farms is generally preferable to prescribing technology and process standards (Gunningham & Sinclair 1999, Prost et al., 2018, Spicer et al., 2021). In principle, performance standards, by providing farmers with the flexibility to choose the mix of technologies and practices that will enable them to meet the standard, should enable improvements in natural resource management at lower cost, and faster, than imposing technology and process standards.
A related implication concerns farmers’ willingness to comply with regulations and prohibitions. The more complex a technology or practice, and the greater the relative advantage it offers, the stronger will be the emotional investment in adopting it. Correspondingly, the more complex a technology or practice, and the greater the relative advantage it offers, the stronger will be the resistance to being forced to abandon it, and the greater the likelihood of outrage at being compelled to do so. Conversely, where a policy compels adoption of a complex practice or technology, and the smaller the relative advantage it offers, the stronger resistance to adopting it will be, and the greater the likelihood of opposition and outrage. In these circumstances, farmers will seek to block or modify policies, or delay their implementation. At the very least, they will seek ways of appearing to comply with the letter of the policy while avoiding complying with its intent (Kaine and Johnson, 2004, Kaine & Higson 2006).
Our results suggest that technologies and practices that offer only marginal improvements in relative advantage may well fail to create goal desire that is strong enough to generate an intention to trial or adopt (e.g., Maertens et al., 2020). This is important with respect to, for example, the rapidity with which farmers may act in anticipation of climate change. The greater the complexity of any change in farm systems and practices that is suggested to reduce greenhouse gas emissions, sequester carbon, or alleviate the impacts of climate change, the smaller is the incentive to adopt those suggestions, and the greater the relative advantage must be to overcome integration costs. The implication is, perhaps unsurprisingly, that farmers should postpone making complex changes to their farm system until the benefits of doing so are obvious and reasonably immediate (provided the costs of doing so do not increase disproportionately over time).
Importantly, the detailed investigative effort involved in determining whether an innovation that changes the technical production strategy (e.g., installing a feed pad) should be adopted creates a situation where, should adoption be postponed, it can occur promptly if, and when, there is a favourable change in circumstances (that is, an increase in relative advantage).
Another implication concerns the adoption of suites of inter-related technologies and practices a such as Integrated Pest Management, precision agriculture and organic farming. In all likelihood, the task of integration of a suite of practices into a farm system as a single, collective whole would be extremely challenging. Consequently, adoption can be expected to occur in a piecemeal fashion with those elements in the suite that are less complex being adopted before those elements that are more complex, depending on relative advantage. Elements that are particularly complex, and/or offer only a marginal advantage, may not be adopted at all. This suggests that it is a mistake to use the number of elements that have been adopted as an index of the innovativeness or progressiveness of farmers (Hammond et al., 2006, Kiruthika, 2013; Lambert et al., 2015; Castle et al., 2016; Creissen et al., 2019; Steiro et al., 2020).
As a final point, the ideas and methods described here could be applied in statistical (e.g., Pierpaoli et al., 2013; Serebrennikov et al., 2020) or expert-system (e.g., Kuehne et al., 2017) models seeking to explain or predict the adoption of technologies and practices in agricultural industries in any region. The systems-based approach to describing complexity proposed by Henderson and Clark (1990) provides testable hypotheses regarding the magnitude of the impact of adopting a technology or practice in terms of disruption to system activity, the destruction of competencies, and the need for new skills and knowledge.
Our study is subject to several qualifications. First, we did not include any information on the personal characteristics of participants (apart from years in dairying) or their properties that might influence the rate of adoption such as risk preferences, personal innovativeness, expenditure priorities, or farm income) (see Lesch and Wachenheim, 2014; Liu et al., 2018; Dessart et al., 2019, for example). The unexplained variation in the dependent variables might be at least partly attributable to these characteristics and attributes. Second, there is the possibility that participants’ perceptions of the complexity and novelty of technologies and practices may be correlated with personal characteristics and property attributes. Third, personal characteristics and property attributes may well influence aspects of goal desire that we have not considered such as locus of control and self-efficacy. Fourth, we have not considered external constraining factors relating to intellectual property rights that could delay adoption such as protected plant varieties, patents, and industrial designs which may apply to agricultural equipment.
In addition, variations among participants in the time taken to try and to adopt technologies and practices will be affected by factors such as differences in investment priorities and in farm profitability at the time participants first learned of technologies and practices. The variation among participants in the time taken to try and to adopt technologies and practices may also be affected by errors in recall.
Conclusion
In this paper we described a novel approach to predicting rates of adoption with respect to agricultural technologies and practices drawing on the dual-process model of consumer decision-making (Bagozzi, 2006a, b) and a method for classifying innovations in farm systems based on Henderson & Clarke (1990).
Our results confirm that the adoption of technologies and practices in farming can be complicated and slow. The process of gathering information sufficient to motivate the formation of an intention to try or adopt may take several months for relatively simple technologies and practices, and several years for more complex ones. Importantly, we found that novelty in terms of the originality in the components and architecture of a technology or practice does not necessarily correlate with its complexity in terms of integrating it into farm systems. This means that apparently simple technologies and practices that are promoted to reduce resource degradation can be quite difficult to integrate into farm systems and, as a consequence, the costs of integration may act as a strong deterrent to adopting them. While the persistent incentive may be to seek productive change in a farm system, the process of adoption can be quite discontinuous for rational reasons.
A logical implication of our findings is that a deep understanding of the nature of the integration task is essential to anticipating how long it might take for adoption (or compliance) to occur in agriculture and, therefore, to appreciate limits on the adaptive capacity of farmers. Such an understanding requires an intimate knowledge of the, sometimes diverse, farm systems and sub-systems in which the technology or practice is to be integrated. This has important implications for agricultural and natural resource policy and extension. The ideas and methods described here could be used to explain or predict the adoption of technologies and practices in any agricultural industry in any region.
For future research, an implication of our findings is that there is a complex interaction among the factors that influence relative advantage (which drives the decision to adopt) and that influence goal striving (which is the implementation, or not, of the decision to adopt). This means that subsuming decision and implementation into a single event (adoption) may lead to a weak understanding of which factors are facilitating, and which are impeding, adoption. This may lead to missteps in the design and choice of measures employed to maximise the scale and rate of adoption.
Data availability statement
The original contributions presented in the study are included in the article/Supplementary Material. Further inquiries can be directed to the corresponding author.
Ethics statement
Ethical review and approval was not required for the study on human participants in accordance with the local legislation and institutional requirements. Written informed consent for participation was not required for this study in accordance with the national legislation and the institutional requirements. The study was conducted in accord with the New Zealand Association of Social Science Research code of ethics.
Author contributions
All authors listed have made a substantial, direct, and intellectual contribution to the work, and approved it for publication.
Acknowledgments
The original research on which this article is based was supported by Waikato Regional Council through a grant supplied by the New Zealand Ministry for the Environment. We would like to thank the staff of the Waikato Regional Council for their advice and assistance. We would also like to thank our reviewers.
Conflict of interest
The authors declare that the research was conducted in the absence of any commercial or financial relationships that could be construed as a potential conflict of interest.
Publisher’s note
All claims expressed in this article are solely those of the authors and do not necessarily represent those of their affiliated organizations, or those of the publisher, the editors and the reviewers. Any product that may be evaluated in this article, or claim that may be made by its manufacturer, is not guaranteed or endorsed by the publisher.
Supplementary material
The Supplementary Material for this article can be found online at: https://www.frontiersin.org/articles/10.3389/fagro.2022.967605/full#supplementary-material
References
Ajzen I. (2001). Nature and operation of attitudes. Annu. Rev. Psychol. 52, 27–28. doi: 10.1146/annurev.psych.52.1.27
Ajzen I. (2002). Perceived control, self-efficacy, locus of control, and the theory of planned behavior. J. Appl. Soc. Psychol. 32, 665–683. doi: 10.1111/j.1559-1816.2002.tb00236.x
Alcon F., de Miguel M. D., Burton M. (2011). Duration analysis of adoption of drip irrigation technology in southeastern Spain. Technological Forecasting Soc. Change 78 (6), 991–1001. doi: 10.1016/j.techfore.2011.02.001
Anderson J. R., Feder G. (2007). “Agricultural extension,” in Handbook of agricultural economics, (North Holland, Amsterdam: Anderson) 3. , 2343–2378.
Bagozzi R. P. (1992). The self-regulation of attitudes, intention and behavior. Soc. Psychol. Q. 55 (2), 178–204. doi: 10.2307/2786945
Bagozzi R. P. (2006a). “Consumer action: Automaticity, purposiveness and self-regulation,” in Review of marketing research,. Ed. Malhotra N. K. (New York: M.E. Sharpe) 2, 3–42.
Bagozzi R. P. (2006b). Explaining consumer behavior and consumer action: From fragmentation to unity. Seoul J. Business 12 (2), 111–143.
Bagozzi R. P. (2007). The legacy of the technology acceptance model and a proposal for a paradigm shift. J. Assoc. Inf. Syst. 8 (4), 243–254. doi: 10.17705/1jais.00122
Bagozzi R. P., Dholakia U. (1999). Goal setting and goal striving in consumer behavior. J. Marketing 63, 19–32. doi: 10.1177/00222429990634s104
Bagozzi R. P., Lee K.-H. (1999). Consumer resistance to, and acceptance of, innovations. Adv. Consumer Res. 26, 218–225.
Bagozzi R. P., Warshaw P. R. (1990). Trying to consume. J. Consumer Res. 17 (2), 127–140. doi: 10.1086/208543
Ball L., Mankiw N. G. (1994). “A sticky-price manifesto,” in Carnegie-Rochester Conference Series on Public Policy, 41. 127–151.
Benin S, Nkonya E, Okecho G, Randriamamonjy J, Edward K, Lubade G, Kyotalimye M (2011). eturns to spending on agricultural extension: The case of the National Agricultural Advisory Services (NAADS) program of Uganda. Agricultural Economics 42, 249–267. doi: 10.1111/j.1574-0862.2010.00512.x
Bils M., Klenov P. J. (2004). Some evidence on the importance of sticky prices. J. Political Economy 112 (5), 947–985. doi: 10.1086/422559
Bopp C., Engler A., Poortvliet P. M., Jara-Rojas R. (2019). The role of farmers’ intrinsic motivation in the effectiveness of policy incentives to promote sustainable agricultural practices. J. Environ. Manage. 244, 320–327. doi: 10.1016/j.jenvman.2019.04.107
Borrás S, Edquist C (2013). The choice of innovation policy instruments. Technological forecasting and social change 80 (8), 1513–1522.
Burton M., Rigby D., Young T. (2003). Modelling the adoption of organic horticultural technology in the UK using duration analysis. Aust. J. Agric. Resource Economics 47 (1), 29–54. doi: 10.1111/1467-8489.00202
Castle M. H., Lubben B. D., Luck J. D. (2016). Factors influencing the adoption of precision agriculture technologies by Nebraska producers. presentations, working papers, and Gray literature: Agricultural economics (Nebraska:Agricultural Economics Department, University of Nebraska –Lincoln). Available at: http://digitalcommons.unl.edu/ageconworkpap/49.
Creissen H. E., Jones P. J., Tranter R. B., Girling R. D., Jess S., Burnett F. J., et al. (2019). Measuring the unmeasurable? A method to quantify adoption of integrated pest management practices in temperate arable farming systems. Pest Manage. Sci. 75, 3144–3152. doi: 10.1002/ps.5428
Crouch B. R. (1981). Innovation and farm development: A multi-dimensional model, in extension education and rural development. Eds. Crouch B. R., Brisbane S.C. (Brisbane: Wiley and Sons).
de Oca Munguia O. M., & Llewellyn R. (2020). The adopters versus the technology: Which matters more when predicting or explaining adoption? Appl. Economic Perspect. Policy 42 (1), 80–91. doi: 10.1002/aepp.13007
Dessart F. J., Barreiro-Hurlé J., van Bavel R. (2019). Behavioural factors affecting the adoption of sustainable farming practices: A policy-oriented review. Eur. Rev. Agric. Economics 46 (3), 417–471. doi: 10.1093/erae/jbz019
Doole G. J., Kaine G., Dorner Z. (2019). The optimal diffusion of mitigation options for environmental management. Aust. J. Agric. Resource Economics. 63 (2), 354–382. doi: 10.1111/1467-8489.12291
Fishbein M., Ajzen I. (1975). Belief, attitude, intention, and behavior: An introduction to theory and research (MA: Addison-Wesley Reading).
Gatignon H., Tushman M. L., Smith W., Anderson P. (2002). A structural approach to assessing innovation: Construct development of innovation locus, type and characteristics. Manage. Sci. 48 (9), 1103–1122. doi: 10.1287/mnsc.48.9.1103.174
Grieshop J. I., Zalom F. G., & Miyao G. (1988). Adoption and diffusion of integrated pest management innovations in agriculture. Bull. ESA 34 (2), 72–79. doi: 10.1093/besa/34.2.72
Gunningham N., & Sinclair D. (1999). “Designing smart regulation,” in A reader in environmental law ( New York: Oxford University Press), 305–334.
Gunningham N., Grabosky P., & Sinclair D. (1998). Smart regulation designing environmental policy (New York: Oxford University Press).
Hammond C. M., Luschei E. C., Boerboom C. M., Nowak P. J. (2016). Adoption of integrated pest management tactics by Wisconsin farmers Vol. 20 (Weed Technology), 756–767.
Henderson R. M., Clark K. B. (1990). Architectural innovation: The reconfiguration of existing product technologies and the failure of established firms. Administrative Sci. Q. 35 (1), 9–27. doi: 10.2307/2393549
Jayne T. S., Snapp S., Place F., & Sitko N. (2019). Sustainable agricultural intensification in an era of rural transformation in Africa. Global Food Secur. 20, 105–113. doi: 10.1016/j.gfs.2019.01.008
Kaine G., & Higson M. (2006). Understanding variety in landholders' responses to resource policy. Australas. Agribusiness Rev. 14, 1–23
Kaine G., Hill M., Rowbottom B. (2008). Types of agricultural innovations and the design of extension programs, practice change research working paper 02-08 (Tatura, Victoria: Department of Primary Industries).
Kaine G., Johnson F. (2004). “Water policy in new Zealand and Australia: We’re not playing for tiddlywinks,” in Proceedings of the New Zealand Grasslands Association.(NZGA; Dunedin)
Kaine G., Longley S., Seymour E., Wright V. (2012). The rate of adoption of agricultural innovations, service design research working paper 02-12 (Tatura, Victoria: Department of Primary Industries).
Kaine G., Wright V. (2017a). Towards predicting rates of adoption and compliance in farming: Motivation, complexity and stickiness, Waikato regional council technical report 2017/05 (Hamilton: Waikato Regional Council).
Kaine G., Wright V. (2017b). Rates of adoption and compliance in dairy farming, Waikato regional council technical report 2017/06 (Hamilton: Waikato Regional Council).
Khataza R. R., Doole G. J., Kragt M. E., & Hailu A. (2018). Information acquisition, learning and the adoption of conservation agriculture in Malawi: A discrete-time duration analysis. Technological Forecasting Soc. Change 132, 299–307. https://doi.org/10.1016/j.techfore.2018.02.015
Kiruthika N. (2013). Adoption of integrated pest management (IPM) in vegetables: a case of onion in Tamil nadu. Am. Int. J. Res. Humanities Arts Soc. Sci. 2 (2), 111–115.
Kleinman P. J. A., Spiegal S., Rigby J. R., Goslee S. C., Baker J. M., Bestelmeyer B. T., et al. (2018). Advancing the sustainability of US agriculture through long-term research. J. Environ. Qual. 47 (6), 1412–1425. doi: 10.2134/jeq2018.05.0171
Kuehne G., Llewellyn R., Pannell D. J., Wilkinson R., Dolling P., Ouzman J., et al. (2017). Predicting farmer uptake of new agricultural practices: A tool for research, extension and policy. Agric. Syst. 156, 115–125. doi: 10.1016/j.agsy.2017.06.007
Lambert D. M., Paudel K. P., Larson J. A. (2015). Bundled adoption of precision agriculture technologies by cotton producers. J. Agric. Resource Economics. 1, 325–345.
Lamb D. W., Frazier P., & Adams P. (2008). Improving pathways to adoption: Putting the right p’s in precision agriculture. Comput. Electron. Agric. 61 (1), 4–9. doi: 10.1016/j.compag.2007.04.009
Lesch W. C., Wachenheim C. J. (2014). Factors influencing conservation practice adoption in agriculture: a review of the literature. agribusiness & applied economics report 722 (North Dakota: Department of Agribusiness and Applied Economics, North Dakota State University).
Liu T., Bruins R. J., Heberling M. T. (2018). Factors influencing farmers’ adoption of best management practices: A review and synthesis. Sustainability 10 (2), 432. https://doi.org/10.3390/su10020432
Loock C. M., Staake T., & Thiesse F. (2013). Motivating energy-efficient behavior with green IS: An investigation of goal setting and the role of defaults. MIS Q. 37 (4), 1313–1332. doi: 10.25300/MISQ/2013/37.4.15
Lowenberg-DeBoer J., & Erickson B. (2019). Setting the record straight on precision agriculture adoption. Agron. J. 111 (4), 1552–1569. doi: 10.2134/agronj2018.12.0779
Maertens A., Michelson H., & Nourani V. (2021). How do farmers learn from extension services? evidence from Malawi. Am. J. Agric. Economics 103 (2), 569–595. doi: 10.1111/ajae.12135
Mankiw N. G., Reis R. (2006). Pervasive stickiness. Am. Economic Rev. 96 (2), 164–169. doi: 10.1257/000282806777211937
Miller N. J., Griffin T. W., Bergtold J., Ciampitti I. A., & Sharda A. (2017). Farmers’ adoption path of precision agriculture technology. Adv. Anim. Biosci. 8 (2), 708–712. doi: 10.1017/S2040470017000528
Mkuki Z. H., & Msuya C. P. (2020). Agricultural extension officers’ perceptions towards their roles: A case study of simiyu region. Tanzania J. Agric. Sci. 19 (2), 56–66.
Ogawa S. (1998). Does sticky information affect the locus of innovation? evidence from the Japanese convenience-store industry. Res. Policy 26 (7–8), 777–790. doi: 10.1016/S0048-7333(97)00047-4
Okali C., Sumberg J., & Farrington J. (1994). Farmer participatory research: rhetoric and reality (London (UK: Intermediate Technology).
Oreg S. (2003). Resistance to change: Developing an individual difference measure. J. Appl. Psychol. 88 (4), 680–693. doi: 10.1037/0021-9010.88.4.680
Pannell D. J., Claassen R. (2020). The roles of adoption and behavior change in agricultural policy. Appl. Economic Perspect. Policy 42 (1), 31–41. doi: 10.1002/aepp.13009
Pannell D. J., Marshall G. R., Barr N., Curtis A., Vanclay F., Wilkinson R. (2006). Understanding and promoting adoption of conservation practices by rural landholders. Aust. J. Exp. Agric. 46 (11), 1407–1424. doi: 10.1071/EA05037
Pierpaoli E., Carli G., Pignatti E., & Canavari M. (2013). Drivers of precision agriculture technologies adoption: A literature review. Proc. Technol. 8, 61–69. doi: 10.1016/j.protcy.2013.11.010
Price J. C., & Leviston Z. (2014). Predicting pro-environmental agricultural practices: The social, psychological and contextual influences on land management. J. Rural Stud. 34, 65–78. doi: 10.1016/j.jrurstud.2013.10.001
Ram S., Sheth J. N. (1989). Consumer resistance to innovations: The marketing problem and its solutions. J. Consumer Marketing 6 (2), 5–14. doi: 10.1108/EUM0000000002542
Rodriguez J. M., Molnar J. J., Fazio R. A., Sydnor E., & Lowe M. J. (2009). Barriers to adoption of sustainable agriculture practices: Change agent perspectives. Renewable Agric. Food Syst. 24 (1), 60–71. doi: 10.1017/S1742170508002421
Serebrennikov D., Thorne F., Kallas Z., McCarthy S. N. (2020). Factors influencing adoption of sustainable farming practices in Europe: A systemic review of empirical literature. Sustainability 12 (22), 9719. doi: 10.3390/su12229719
Sims C. A. (1998). “Stickiness,” in Carnegie-Rochester Conference Series on Public Policy, Vol. 49. 317–356.
Snapp S., Heong K. L. (2013). “Scaling up and out,” in Managing natural resources for sustainable livelihoods (London: Routledge), 87–107.
Spicer E. A., Swaffield S., Moore K. (2021). Agricultural land use management responses to a cap and trade regime for water quality in lake taupo catchment, new Zealand. Land Use Policy 102, 105200. doi: 10.1016/j.landusepol.2020.105200
Steiro Å.L., Kvakkestad V., Breland T. A., Vatn A. (2020). Integrated pest management adoption by grain farmers in Norway: A novel index method. Crop Prot. 135 (2), 105201. doi: 10.1016/j.cropro.2020.105201
Szulanski G. (1996). Exploring internal stickiness: Impediments to the transfer of best practice within the firm. Strategic Manage. J. 17, 27–43. doi: 10.1002/smj.4250171105
Van De Fliert E., Asmunati R., Tantowijoyo W. (2000). “Participatory approaches and scaling-up,” in Paper presented at the CIAT workshop “Working with farmers: the key to adoption of forage technologies, vol. Vol. 12., 15(ACIAR. Canberra, Australia).
Vanlauwe B., Descheemaeker K., Giller K. E., Huising J., Merckx R., Nziguheba G., et al. (2015). Integrated soil fertility management in sub-Saharan Africa: unravelling local adaptation. Soil 1 (1), 491–508. doi: 10.5194/soil-1-491-2015
Westermann O., Förch W., Thornton P., Körner J., Cramer L., Campbell B. (2018). Scaling up agricultural interventions: Case studies of climate-smart agriculture. Agric. Syst. 165, 283–293. doi: 10.1016/j.agsy.2018.07.007
Wright V. (2011). Rates of adoption: The diffusion of agricultural innovations, service design research working paper 06-11 (Tatura, Victoria: Victorian Department of Primary Industries).
Zilberman D., Zhao J., Heiman A. (2012). Adoption versus adaptation, with emphasis on climate change. Annu. Rev. Resource Economics 4 (1), 27–53. doi: 10.1146/annurev-resource-083110-115954
Appendix A
Advantage, novelty, complexity and integration task for farm technologies and practices
Values in parentheses are number of observations. Values in bold are greater than the sample average. Time to trying and time to adopting is in months. In the absence of trialling time to try equals time to adoption and time from trying to adoption is zero.
Keywords: Farmers, adaptive capacity, behaviour, adoption, complexity, rate of adoption, policy
Citation: Kaine G and Wright V (2022) Relative advantage and complexity: Predicting the rate of adoption of agricultural innovations. Front. Agron. 4:967605. doi: 10.3389/fagro.2022.967605
Received: 13 June 2022; Accepted: 15 August 2022;
Published: 06 September 2022.
Edited by:
Sandra Ricart, University of Alicante, SpainReviewed by:
Shahrina Md Nordin, Universiti Teknologi Petronas, MalaysiaRick Llewellyn, Commonwealth Scientific and Industrial Research Organisation (CSIRO), Australia
Margot Leclère, Institut National de Recherche pour l’Agriculture, l’Alimentation et l’Environnement (INRAE), France
Michael Blakeney, University of Western Australia, Australia
Copyright © 2022 Kaine and Wright. This is an open-access article distributed under the terms of the Creative Commons Attribution License (CC BY). The use, distribution or reproduction in other forums is permitted, provided the original author(s) and the copyright owner(s) are credited and that the original publication in this journal is cited, in accordance with accepted academic practice. No use, distribution or reproduction is permitted which does not comply with these terms.
*Correspondence: Geoff Kaine, a2FpbmVnQGxhbmRjYXJlcmVzZWFyY2guY28ubno=
†These authors have contributed equally to this work and share first authorship