- 1Sustainable Land Use and Climate Change, Department of Agricultural Economics, Humboldt-Universität zu Berlin, Berlin, Germany
- 2Potsdam Institute for Climate Impact Research, Member of the Leibniz Association, Potsdam, Germany
- 3Institute for Resistance Research and Stress Tolerance, Julius KühnInstitute, Federal Research Centre for Cultivated Plants, Quedlinburg, Germany
- 4Department of Plant Breeding, IFZ Research Centre for Biosystems, Land Use and Nutrition, Justus Liebig University, Giessen, Germany
- 5Department of Agricultural Economics and Rural Development, University of Göttingen, Göttingen, Germany
Improved wheat varieties have ameliorated our food production. Intensive cropping systems, as in Germany, achieve very high grain yields and thus contribute greatly to global food security. As diseases, especially fungal pathogens, pose major threats in winter wheat production, disease management is crucial to uphold high production levels and to avoid economic losses. Yet, the economic value of breeding varieties with increased resistance to fungal pathogens has not been analyzed with comprehensive data. Our analysis fills this gap by quantifying the economic effects of 50 years of resistance breeding for winter wheat production in Germany. Based on field trials and agronomic production data, we analyze how resistances have influenced the economic profitability of the crops. Thereby, we can isolate the pure breeding-induced effects of increased resistance to fungal pathogens, which cause leaf and stripe rust, powdery mildew, and Fusarium head blight. We calculated the gross margins of 176 varieties according to nitrogen and fungicide applications in field trials. Regression models show that resistance breeding had a strong positive and statistically significant effect on the gross margins of the varieties. In comparison, the specific meta-environment of the season had a larger effect, while the effect of high nitrogen as well as fungicide treatments was lower. The gross margin increased over time along with higher resistances. Simultaneously, the difference in gross margins between the fungicide-treated and untreated variants decreased, indicating an increased contribution of resistances to yield stability. Resistances have increased gross margins greatly, as the health levels of varieties remain high without the need for fungicide applications and thus production costs can be saved. We conclude that resistance breeding has increased the economic profitability and sustainability of crop production due to a reduction in the costs for fungicides. In fields of farmers, these positive effects of resistance breeding can be fully realized by an optimal variety choice and a variety- and situation-specific disease management. Such an agronomic practice would not only further improve winter wheat gross margins but also mitigate some of the pressures on agricultural productions, such as reduced disease management options and adverse climate change impacts.
Introduction
Global pressures, such as climate change and population growth, require that agricultural production must increase with less negative environmental and climatic effects. Globally, winter wheat is one of the main crops for food security (Shiferaw et al., 2013), and in Germany, it is the most grown crop, producing very high yields in intensive production systems (BMEL, 2021; FAOSTAT, 2021). Such an intensification of agriculture has effects on the environment and land use. Due to the potential production losses by pests and diseases (Savary et al., 2019), plant protection chemicals are a part of winter wheat management. Therefore, the production of wheat has special importance regarding the implementation of integrated plant protection. Also, there are political and societal pressures to reduce the use of synthetic plant protection for this crop. The most recent incorporation of these pressures is the strategy, From Farm to Fork, which is a cornerstone of the European Green Deal, released recently by the European Union. It states that by 2030, chemical pesticides shall be reduced by 50%. Also, the German National Action Plan on the Sustainable Use of Plant Protection Products intends to reduce plant protection applications to the necessary minimum that secures economic cultivation of crops. Due to these reasons, it can be expected that the spectrum and quantity of available substances for plant protection will be strongly reduced in the future (Schwarz et al., 2018) and this will have great effects on managing pathogens.
Crop resistances also play a crucial role in disease management. Plant breeders constantly develop newer and better varieties to assure or increase agricultural production and safeguard our food security. Plant breeding has upheld and improved winter wheat production even under reduced input conditions (Voss-Fels et al., 2019). It is well-understood that a large determinant in the rise of yield levels in Germany was genetic gains by plant breeding and that this breeding-induced yield increase is not stagnating (Ahlemeyer and Friedt, 2010; Laidig et al., 2014; Voss-Fels et al., 2019). New varieties have accumulated genetic variants with beneficial effects on the important yield enhancing traits, such as nutrient use and photosynthetic efficiency as well as disease resistance (Voss-Fels et al., 2019; Zetzsche et al., 2019, 2020). Over time, variety aging decreases the yield ability of varieties and is mainly induced by a steady breakdown of resistances due to pathogen evolution (Mackay et al., 2011; Fischer et al., 2014; Laidig et al., 2014; Piepho et al., 2014). Therefore, continued breeding for resistance is the key to uphold or increase the yield quantities and qualities.
Among the diseases causing losses in winter wheat production, pathogenic fungi are very dominant (Russell, 2013; Simón et al., 2021). Consequently, fungicides are the most used category of plant protection products in winter wheat production systems in Germany, regarding application rate and treatment frequency (Roßberg, 2016). Single fungal pathogens can cause yield losses of up to 70% in susceptible varieties (Zetzsche et al., 2020). In recent decades, the use of fungicides and the increasing cultivation of resistant varieties have prevented devastating epidemics in Europe (Hovmøller and Justesen, 2007; Dubin and Brennan, 2009; Singh et al., 2016; Figueroa et al., 2018), while genetic resistances are said to be the most effective and sustainable approach (Ghimire et al., 2020). In the history of breeding, genetic resistance against rusts and powdery mildew have been the most successful (Singh and Rajaram, 2002). Resistant varieties, as part of integrated disease management, can contribute to reducing the use of chemical synthetic plant protection (Schwarz et al., 2018). In Germany, contrary to the continuously increasing resistance levels of new varieties, the treatment frequency and intensity of fungicides augmented slightly over time between 2011 and 2017, a trend that was influenced by disease-promoting weather conditions and by a focus of producers on yield protection (Roßberg, 2016; Kehlenbeck, 2018).
Given this background, it is vital to better understand the economic effect of the increased resistance of the varieties to fungal pathogens. According to Marasas et al. (2003) and Nalley et al. (2010), research that analyzes the economic impacts of breeding programs often overlooks the important aspect of breeding for resistance, which has the goal to maintain high yields and quality levels. In addition, a large part of the research that addresses the subject focuses on the impact of specific breeding programs in developing countries, where cultivation is less intensive (Smale et al., 1998; Marasas et al., 2003; Dixon et al., 2006; Dubin and Brennan, 2009; Nalley et al., 2010; Lantican et al., 2016). Also, analyses for intensive cropping systems have found that the cultivation of resistant varieties is a major, cost-efficient, effective, and ecological technology to control diseases (Clifford and Lester, 1988; Hogenboom, 1993; Wiik and Rosenqvist, 2010; Hysing et al., 2012; Russell, 2013; Thompson et al., 2014). Nevertheless, the breeding of resistant varieties and further the exploitation of these resistances in practice poses difficulties due to potential risk aversion, insufficient information on the variety, high inter- and intra-annual variability in disease pressures, and other factors (Clifford and Lester, 1988; Russell, 2013; TeBeest et al., 2013). Yet, Hogenboom (1993) anticipated that new innovations in resistance breeding will increase the economic value of this method to manage diseases. Due to limited variety-specific data availability and trials, these analyses face the difficulty to approximate the pure breeding effect on yield and other parameters as well as the resulting economic impacts. In Germany, the economic assessment of different cropping systems with a special focus on disease resistance measured the economic effect of a small set of selected winter wheat varieties and showed that the resistant varieties have a high potential to reduce fungicide applications and consequently reduce costs (Kehlenbeck and Rajmis, 2018; Klocke et al., 2018; Rajmis and Kehlenbeck, 2018; Sommerfeldt, 2018).
To the best of our knowledge, so far, there is no microeconomic transformation of variety-specific resistances, purely induced by breeding, in a region where wheat is grown intensively, such as in Germany. Our research aims to contribute to closing this research gap by answering the following question: In the last 50 years, what has been the effect of resistance breeding on winter wheat gross margins in Germany? We chose Germany as a representative country in central Europe where winter wheat dominates and achieves very high yields (BMEL, 2021) due to good soils, sufficient precipitation, and moderate winters. Moreover, winter wheat breeding for this target environment is also quite intensive and competitive. We are the first to perform an economic analysis of resistance breeding with field-experimental data comprising ~180 old and new varieties cultivars that represent the most important German winter wheat varieties of the last 50 years (Voss-Fels et al., 2019; Zetzsche et al., 2020). This allows us to monetize the sole breeding-induced effect of increased resistances, without any masking effects from other production factors, and also reduces the statistical bias which can arise when only a few preselected varieties are analyzed.
Based on the trial data, we can compare the performance of varieties in treatments with and without fungicide applications, and with the help of the latter, we can deduct the impact of resistance breeding. We hypothesize that under treatments without fungicides, resistant varieties achieve higher yields than the less resistant varieties, as they withstand the fungal pathogens without external disease management while maintaining yield quantity and quality. Consequently, we assume that varietal resistances increase gross margins in winter wheat productions, as they secure or increase yields, in the absence of fungicide applications, which are costly due to their product and application costs.
To test these hypotheses, we estimate variety-specific gross margins and then employ regression and post-estimation analyses. These methods and the underlying data are explained in section Materials and Methods. In section Results: Effect of Resistance Breeding on Winter Wheat Gross Margins, we describe the results which are discussed in section Discussion.
Materials and Methods
Data
The data for this analysis are derived from several sources. The main subset is based on field trials comprising a large set of winter wheat varieties (n = 176). These varieties represent the German breeding progress of the past 50 years, as they were released from 1966 to 2013 and were selected based on their economic and agronomic importance for German winter wheat production (Zetzsche et al., 2020). In field trials, old and new varieties were grown simultaneously in the seasons, 2014/2015, 2015/2016, and 2016/2017 in two replications per treatment.
Across trial years and replications, all cultivars were treated with four different combinations of nitrogen and fungicide treatments: low nitrogen, no fungicide; low nitrogen (110 kg N ha−1), with fungicide; high nitrogen (220 kg N ha−1), no fungicide; and high nitrogen, with fungicide. Table 1 shows the fungicide applications for those treatments where fungicides were applied. The dosages represent the full amount that can be applied according to the specifications of the manufacturer.
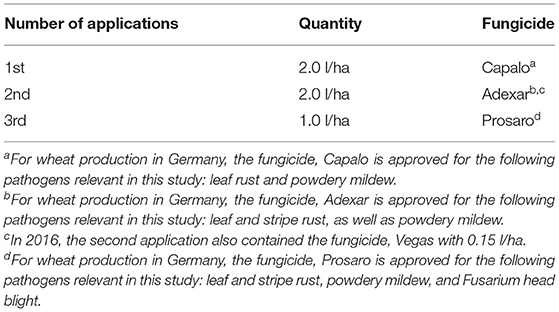
Table 1. Details on fungicide applications for all treatments with fungicides for 2015–2017, source: authors based on Zetzsche et al. (2020).
The plots with low nitrogen treatment had a target quantity of 110 kg N ha−1 and those with high nitrogen had 220 kg N ha−1. The high soil quality at the trial location retained high nitrogen levels and the quantities applied were adjusted based on the available mineralized nitrogen in the plot soils (for more details, refer to Supplements of Voss-Fels et al., 2019 and Zetzsche et al., 2020).
Hence, the field trial data is a balanced panel data set. The trials were conducted on the experimental fields of the German Federal Research Centre for Cultivated Plants (JKI) in central Germany near the city of Quedlinburg located in the Federal State Saxony-Anhalt (51.7694 N, 11.147 E, 140 m altitude). It is an area with very good soil; the soil type is Chernozem with a silty loam texture and a long-term average annual precipitation of 497 mm per year (Zetzsche et al., 2020). But the trial years had above average temperatures (+1.3°C) and an average annual precipitation deficit of 29 mm (Zetzsche et al., 2020). In comparison to the longtime mean, drought periods occurred in spring and early summer in the trial years, 2015 and 2017 as well as during the winter of 2016/17 (Zetzsche et al., 2020). During the trial years, variety-specific phenotypic data were collected, e.g., grain yield, protein content, and susceptibility of the varieties to fungal pathogens. This field trial data represents a unique data set that enables us to compare the performance of many varieties with different years of release under the same conditions, and it also allows us to single out the effect of breeding and more particularly, the resistance breeding on variety performance.
To analyze the fungal diseases, stripe and leaf rust, and Fusarium head blight, the plants were artificially inoculated to create a high disease pressure. A high abundance of the powdery mildew pathogen led to a spontaneous natural infection. Afterward, the infected plant area was estimated as the share of a diseased leaf or spike area in relation to the overall leaf or spike area up to four times per season in the untreated trial plots. Based on these observations, the area under the disease progress curve (AUDPC) was computed and the average ordinate (AO) was subsequently calculated for each disease and variety (Zetzsche et al., 2020). For our analysis, it is important to note that the sub-trials treated with fungicides were just monitored for their susceptibility to fungal pathogens due to very low infestation rates (Zetzsche et al., 2020). Thus, it is assumed that they are pathogen-free; therefore, their disease scores were set to 0 in our data set.
Based on this treatment information, we calculated the costs for the pure fungicide and nitrogen products and their application costs. First, we calculated the specific fungicide product costs based on prices from a large agricultural retailer and the nitrogen costs based on KTBL (2020). Second, the application costs were added based on the Kuratorium für Technik und Bauwesen in der Landwirtschaft e.V. (KTBL, 2020). These include the costs for labor, diesel, repairs, and farm supplies. These application costs were matched with the corresponding quantities and frequencies of the trial setup. No diesel costs were saved by applying several types of plant protection in one ride, as fungicides were applied separately.
The third subset for the economic estimation contains the producer wheat price, which is composed of two different elements: base price and markup or markdown for the quality group of a variety. The base price is derived by matching the producer price index for bread wheat (DESTATIS, 2019) with the reference wheat price from FAOSTAT (2020). Then, using the field trial data and the GRIS database (Martynov et al., 2017), we added the quality group of each variety to assign the corresponding markup or markdown. To derive a flat-rate surcharge or deduction according to the quality group, we calculated a 3-year average of the different quality group prices (Agrarzeitung, 2020) and then created a premium factor to combine it with the bread wheat base price. After matching all three data sets (trial data, costs, and prices), we generated a data set with 176 varieties over 3 years that have complete information for our economic analyses.
With all three data sets, we create a multidimensional data set, representing winter wheat gross margins per variety, trial year, replication within this trial year, and treatment.
Methods
To conduct this multidisciplinary research, we combined the fields of agricultural economics, agronomy, and plant breeding.
Gross Margin Calculation
To determine the economic profitability of certain production methods or work steps, the gross margin is a standard indicator in farm economics (KTBL, 2020; Mußhoff and Hirschauer, 2020; LfL, 2021), which is also used in other analyses to assess the economic profitability of specific winter wheat varieties (Kehlenbeck and Rajmis, 2018). We established a gross margin by calculating the revenue which would have been generated if the winter wheat harvest of the field trials had been sold and then subtracted the costs for nitrogen and fungicide applications (product and application costs) from the revenue (see Equation 1). Due to the field trial setup, we assumed that all other gross margins influencing factors were constant across varieties, treatments, replications, and trial years.
The gross margin is calculated as follows:
where the Gross marginv,t,r,a,q represents the economic profitability for a specific variety v in a specific trial year t, repetition r, and for the treatment variant a. Also, yield depends on these indices. As explained in the data section, the winter wheat producer price (priceq,t) varies with the quality group q of the variety and also the production year which is in our case, the trial year t, while the nitrogen costs (Nitrogen costst a) and fungicides costs (Fungicide costst,a) differ by trial year t and treatment variant a.
Regression Model
To determine how the efforts of the last five decades of resistance breeding in winter wheat have influenced the gross margin of the crop, we applied a regression model with multidimensional data. The regression model allows us to single out the effects of resistance breeding and validate their robustness when other gross margins influencing factors are introduced. The dependent variable of all models is the gross margin as defined in Equation (1) because our primary research interest is to detect the effect of the resistances of the varieties on their economic performance or profitability.
As we want to detect the impact of breeding-induced resistances, the first group of independent variables represents resistance breeding. This group contains the year of release and resistance variables. The variable, year of release shows how the gross margin changed over time with increased breeding progress (Cormier et al., 2016; Voss-Fels et al., 2019; Zetzsche et al., 2019, 2020). An important aspect of winter wheat breeding is the increasing resistance of the varieties to fungal pathogens. During the field trial, the most important fungal diseases were examined (stripe and leaf rust, powdery mildew, and Fusarium head blight). The resistance variables in the model were measured by subtracting the diseased leaf or spike area from the overall leaf area; hence, they quantify the non-diseased or resistant area. These variables, rust resistance, powdery mildew resistance, and Fusarium resistances show the effect of resistance breeding on gross margins when plants are infected with the most damaging pathogens of winter wheat production (Carmona et al., 2020; Figlan et al., 2020; Ghimire et al., 2020). For this study, we combined the rust diseases to one variable, rusts resistances, as these two diseases sequentially occupy a very similar ecological niche and have a similar effect on grain yield (Zetzsche et al., 2020). The full regression model is defined in Equation (2). We call the regression model, Core model when we only look at these resistance breeding variables and set the coefficients of all other variable categories (β5 to β7) to zero. We hypothesize that resistance breeding had a positive effect on the gross margin of the crops, as it secures high grain yield quantities as well as quality, which are the important factors to calculate the microeconomic indicator. The next group of independent variables is variables for agronomic management. According to standard calculators and literature, the gross margin is strongly influenced by the costs of agronomic management practices and the related inputs, such as fertilizers and fungicides and the labor and machinery costs for applying these (KTBL, 2020;Mußhoff and Hirschauer, 2020). Research shows that these inputs explain about half (49%) of the total actual wheat yield volatility in Germany (Albers et al., 2017). Therefore, we added the variables, fungicide application and high nitrogen. According to the field trial, we can distinguish between data points with and without fungicide applications, as well as those with high and low nitrogen dosage. The remaining management was the same across varieties, treatments, and replications. The two variables are dummy variables. Hence, fungicide application takes the value 1 when fungicides are applied, and 0 when not. The variable, high nitrogen remains 1 when a high dosage of nitrogen was applied, and 0 for a low dosage. When the inputs were applied, their product and application costs are included in our model. This model is named Core model+ agronomic management, where, β7, the coefficient for the trial years is set to zero. We hypothesize that high nitrogen and fungicide treatments will have a negative effect on the gross margin, as they also imply high costs. These input costs are not necessarily compensated for by higher yields secured with fungicide applications, as modern varieties can maintain high yield levels without such applications. Also, we hypothesize that under treatments without fungicides, resistant varieties achieve higher yields than less resistant varieties, as they withstand the fungal pathogens without external disease management while maintaining yield quantity and quality.
Apart from the above-mentioned determinants, the gross margin is heavily influenced by the specific growing conditions during the respective growing season. Albers et al. (2017) estimated that 43% of the total actual wheat yield volatility is driven by weather. In addition, as yield is one major determinant of the dependent variable, gross margin, the growing conditions must be included. To this end, we included the variable, trial year, in the model. Due to the field trial setup, the management and input quantities were the same for each treatment and year. Hence, the variable trial year measured the different growing conditions during the three trial years. The results will show the effect of trial years, 2015 and 2017 in comparison to the reference year 2016, as this year had the least drought conditions among all three trial years. This model is termed, Core model + trial year, where, β5 and β6 are set to zero.
Furthermore, a Full model is run; it includes all variable categories: resistance breeding, agronomic management, and the trial year. Equation (2) defines the regression model with all variable categories and Table 2 summarizes all dependent and independent variables.
With ∝ representing the intercept parameter and ε, the error or disturbance term, containing unobserved factors.
In the Results section, we describe for all regression models, the resulting coefficients, which quantify the direction and size of the effect of each variable on the gross margin. To show the absolute effects of the variables, a non-standardized version of the regression results is presented, and to compare the effect size of differently scaled variables, in addition, standardized results are presented. This provides a better understanding of which variables have the largest impact on the economic benefits of resistance breeding. We also show the prediction accuracy or estimation performance for each model with the R2; nevertheless, the focus of this research is not to best predict a gross margin but to quantify the effects of resistance breeding on the gross margin, and this effect is best described with the regression coefficients. Furthermore, we verify if the model assumptions are met.
The compiled data set for the regressions can also be treated as panel data. In this study, we defined panel data as two-dimensional with observations for the same panel subjects (varieties) over time (trial years). Therefore, several tests were run to choose the best fitting model with fixed, random, or pooled effects for panel data regressions (Croissant and Millo, 2008; Torres-Reyna, 2010). The Breusch-Pagan Lagrange Multiplier analysis indicated that random effects have no advantage for our analysis; hence, we can run a linear regression with pooled effects (Torres-Reyna, 2010). Linear regression also better fits our data which is multidimensional because there are not only observations for the dimensions varieties and trial years, but also for further dimensions treatment and replications.
Post-estimation Analyses
To further show the effects of resistance breeding, post-estimation analyses were conducted. These provide a general overview of how the gross margin changed over time. While the regression shows the effects of independent variables on single varieties, this section describes the effects of resistance breeding with data aggregated across the different fungicide and nitrogen treatments as well as resistance levels. These resistance levels will be expressed as leaf and spike health indexes. Like the resistance variables, these health indices measure the percentage of healthy leaf or spike area of the plant. The leaf health index gives the proportion of leaf area which is not infected with a stripe or leaf rust, as well as powdery mildew. The spike health gives the proportion not infected with Fusarium head blight, in percent. A low index includes all plant health values up to the first quantile, medium are all values between the first quantile and the average, and high are all values above the average.
Further, a specific subset of 18 winter wheat varieties was selected, which represents prominent varieties in Germany with different levels of resistance to fungal diseases. We define all varieties with an average leaf health index under 80% as susceptible and those with a higher health index as resistant. Under the high nitrogen treatment, we calculated several indicators for the selected varieties. The indicators measure a variety of traits that are important for the profitability of the varieties and adoption decisions of farmers (grain yield, protein content, gross margin, leaf, and spike health). This aggregation is a helpful overview for practitioners, agronomists, and breeders when they want to examine the performance of specific varieties.
Results: Effect of Resistance Breeding on Winter Wheat Gross Margins
Disentangling the Effect of Resistance Breeding on the Gross Margin
To see the economic effect of resistance breeding, a core and several validation regressions were estimated. The (non-standardized) regression results show that all variables measuring resistance breeding have a positive and statistically significant impact on the gross margin (Table 3; Figure 1). In the core model, all resistance breeding variables, year of release, Fusarium resistance, rusts resistance, and Powdery mildew resistance, have a positive impact on the dependent variables. The coefficient of the variable, the year of release, is 4.87 and it indicates that, on average, the gross margin increases by about 4.87 EUR ha−1, when the year of release increases by 1 year. This means that the gross margin increases by about 5 EUR ha−1 when a variety is chosen, which is 1 year younger. Hence, annual microeconomic progress is created. The forest plot (Figure 1) provides a graphical summary of all model results. It displays that the described effect direction and size of the first four variables, those that represent resistance breeding, remain stable across models. Hence, we can conclude that the validation models validate the effect of resistance breeding on the gross margin.
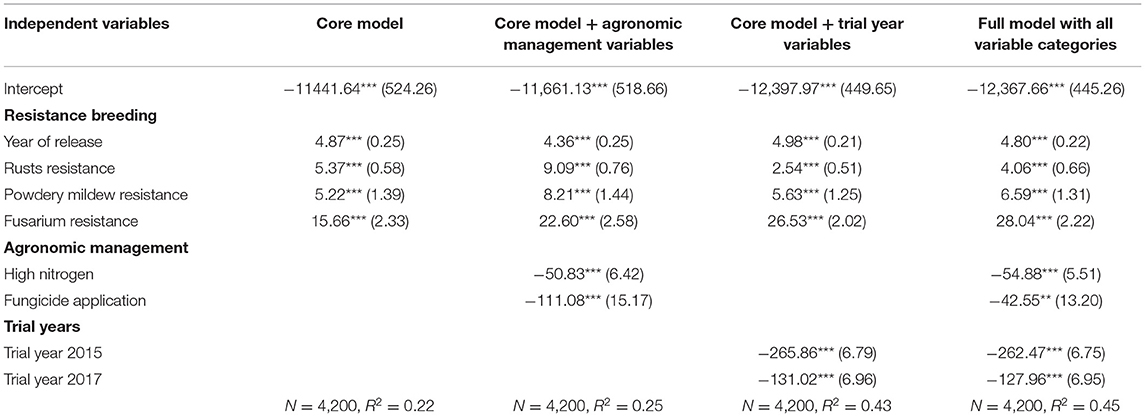
Table 3. Coefficients (standard errors) of the non-standardized regression models with gross margin as the dependent variable (significance codes: ***p < 0.001, **p < 0.01).
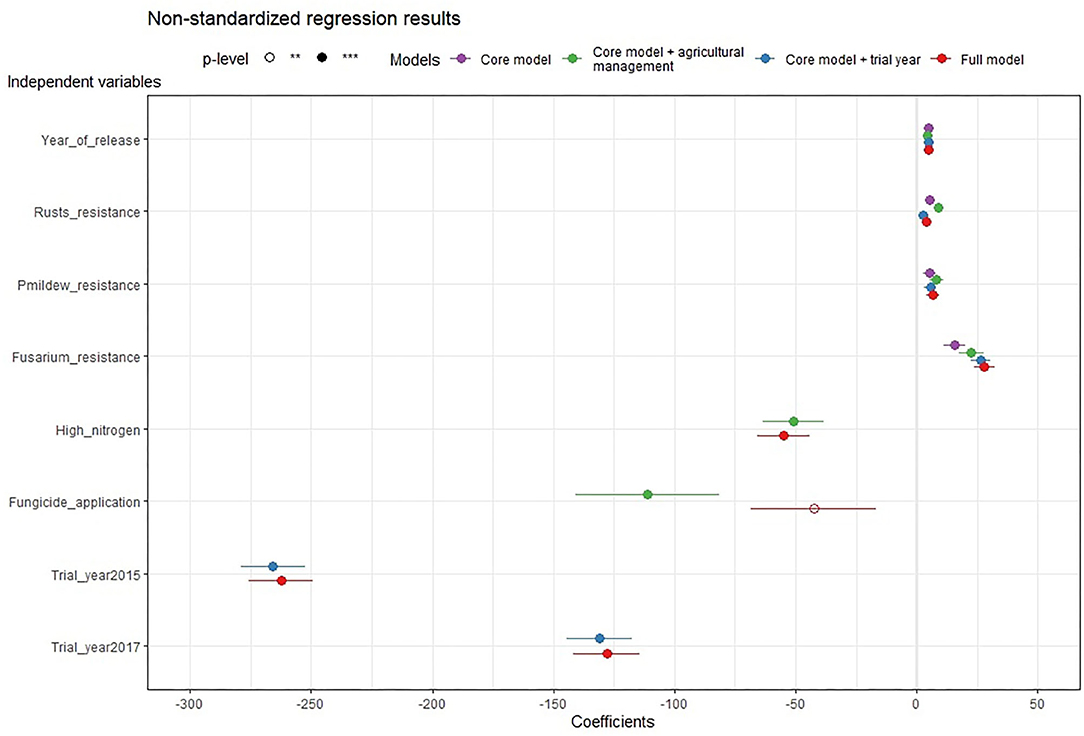
Figure 1. Forest plot of the non-standardized regression results for all regression models. The dependent variable of all models is the Gross margin. Different models are identified by different colors. The plot shows the magnitude (indicated by the circle position) and uncertainty (tails around the circles show the 0.95 confidence interval) of variable coefficients. A filled circle indicates statistical significance at the level p < 0.001 and a not filled circle at the level p < 0.01. When a circle is positioned to the right of the vertical zero lines, it means that the variable increases the gross margin of the dependent variable. For every additional unit of a variable (e.g., one additional percentage point of healthy leaf area), the gross margin increases the coefficient of the variable. And when a point is located to the left of the zero lines, the variable is estimated to have a negative influence on the dependent variable so that it decreases the gross margin.
The standardized regression results allow us to compare more easily the effect sizes among differently scaled variables (Table 4; Figure 2). Across models, the effect of the year of release is as large as the effect size of the rusts and Fusarium resistance. Increased powdery mildew resistance had a positive but small effect. The effects of high nitrogen and fungicide applications are lower than the effects of the four variables that represent resistance breeding, while the effect of the trial year (weather effect) is larger. In summary, all models let us observe a positive interaction of the resistance breeding variables with the variety-specific gross margin outcomes.
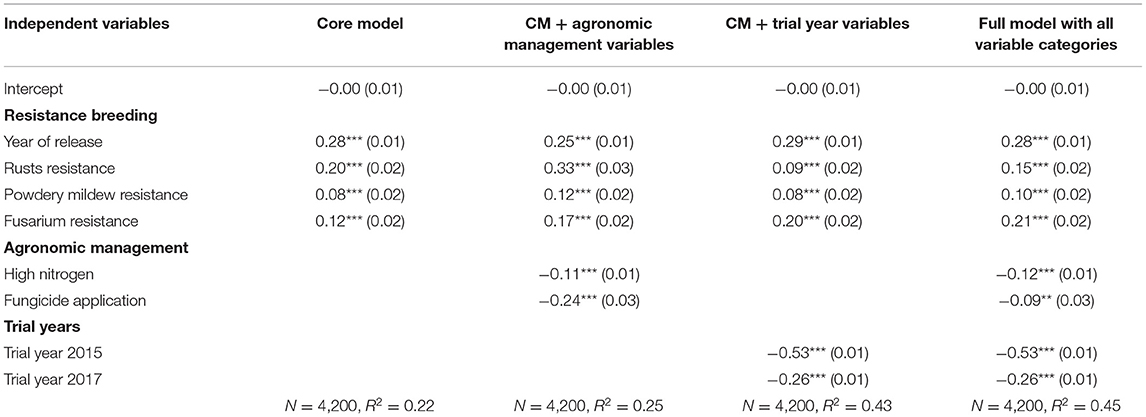
Table 4. Standardized coefficients (standard errors) of the regression models with scaled variables and gross margin as the dependent variable (significance codes: ***p < 0.001, **p < 0.01).
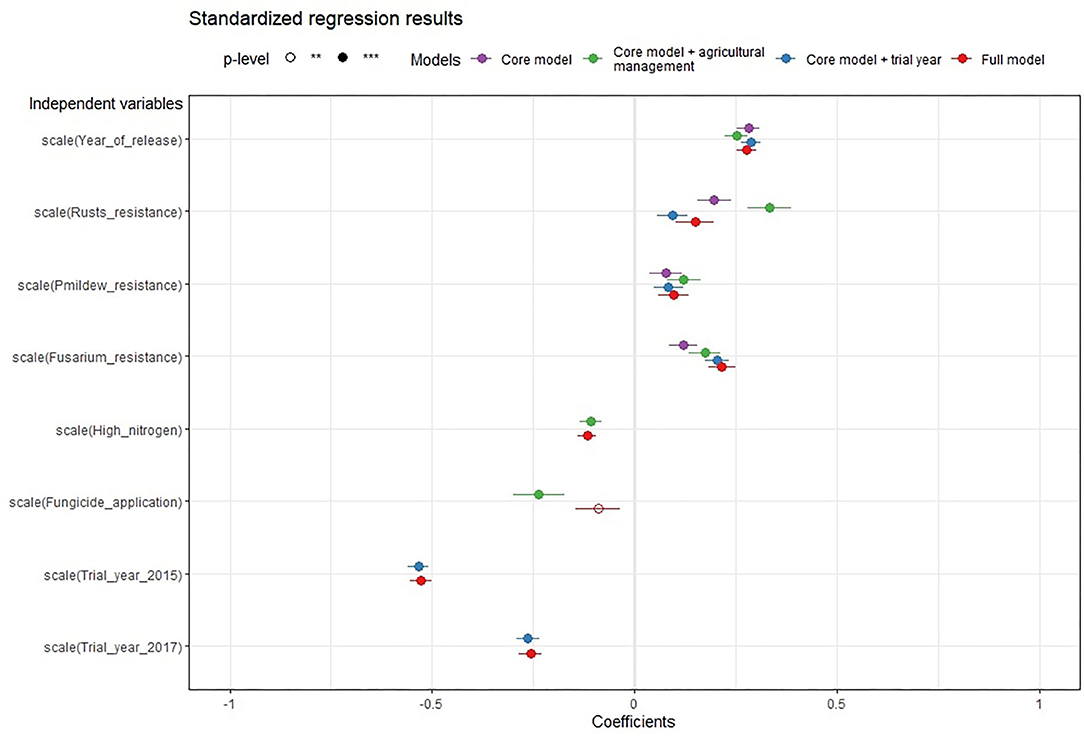
Figure 2. Forest plot of the regression results with standardized variables for all regression models. The dependent variable of all models is gross margin. Different models are identified by different colors. The plot shows the magnitude (indicated by the circle position) and uncertainty (tails around the circles show the 0.95 confidence interval) of coefficients of the variables in all regression models. A filled circle indicates statistical significance at the level of p < 0.001 and a not filled circle at the level of p < 0.01. As variables were standardized, the coefficient magnitude of differently scaled variables can be better compared. When a circle is positioned to the right of the vertical zero lines, it means that the variable increases the gross margin of the dependent variable. For every additional unit of a variable (e.g., one additional percentage point of healthy leaf area), the gross margin increases by the coefficient of the variable, and when a point is located to the left of the zero lines, the variable is estimated to have a negative influence on the dependent variable so that it decreases the gross margin.
The prediction performance of the models, measured with the R2, ranges from 0.22 to 0.45 (Table 3). For example, the independent variables of the core model explain 22% of the variance in the dependent variable. The predictive power increases when adding other variables in the validation models. Several validation models were run along with the model diagnostics to test the regression assumptions. Also, the linear regression model assumptions were tested and graphically displayed (Figure A1 in Annex). According to the Durbin-Watson test, residuals are not autocorrelated. Furthermore, the variance in errors is constant (homoscedasticity). The normal distribution of residuals was tested with the Shapiro-Wilk test, which rejected the null hypothesis, but histograms show nearly normally distributed residuals. In combination with the unchanged results of the validation models, we conclude that the rejection of one test does not distort the regression parameters.
Post-estimation Analyses
Considering all decades, the highest gross margin was calculated for the treatment with high nitrogen and fungicide applications (902.5 EUR ha−1), followed by the low nitrogen treatment with fungicides (892.1 EUR ha−), which is closely followed by low nitrogen without fungicides (853.02 EUR ha−1). The treatment variant, high nitrogen without fungicides has by far the lowest gross margin (697.45 EUR ha−1). When aggregating the gross margin of all the varieties and treatments per decade, the gross margin grew consistently from the 1960s to the 2010s (Figure 3). This finding is consistent with the multiple regression results for the variable, year of release.
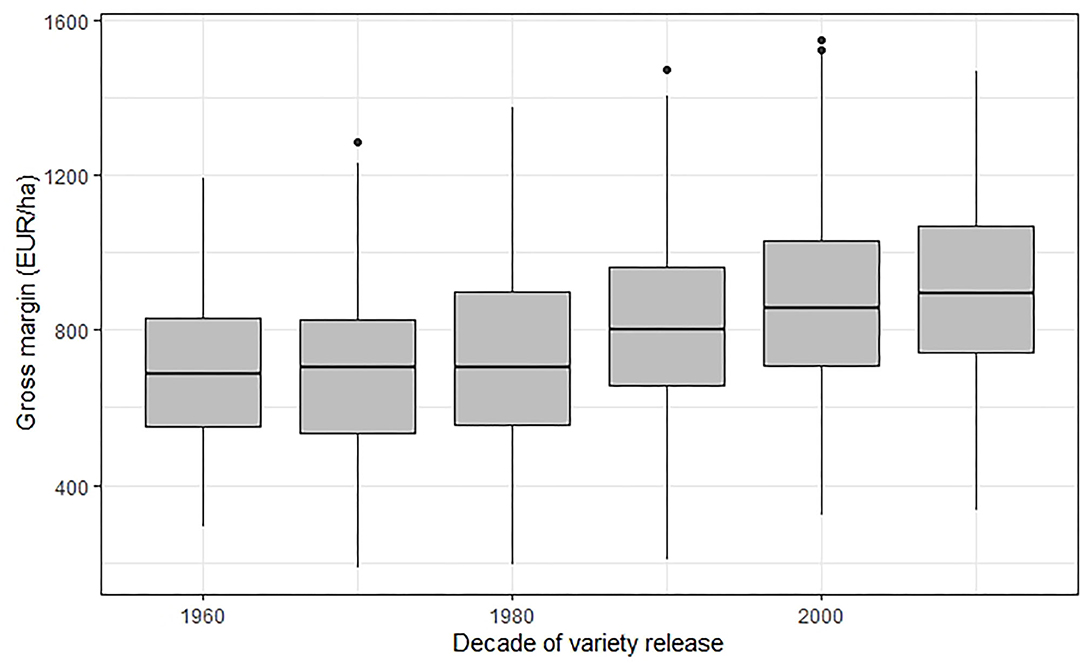
Figure 3. Boxplots of the gross margins (EUR ha−1) per decade of variety release, for all treatment groups and varieties.
In addition, distinguishing by treatment group, Table 5 shows that the gross margin and also resistances of the plant to fungal diseases (here expressed as leaf and spike health) increase from decade to decade in all treatment groups. Please note again that all plots in the treatment groups with fungicides are considered to be not infected with fungal pathogens, therefore having a health index of 100. This is not only a theoretical assumption, as these trial plots were also examined and showed nearly no infections. The gross margin of the newest varieties is the highest for the treatment variants with high nitrogen but only differs a little for those treatment variants with a low nitrogen level. The difference between cultivating with and without fungicides decreased over the years and is more pronounced in the high nitrogen treatment.
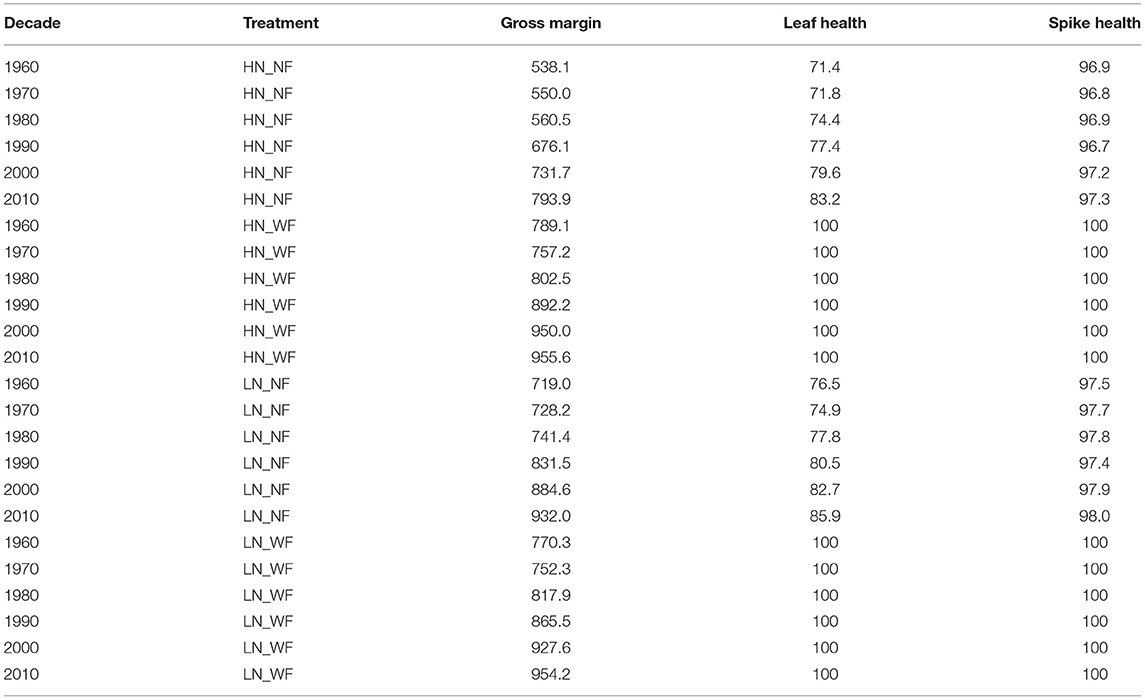
Table 5. Average gross margin (EUR ha−1) and health index per decade and treatment group; Explanation of treatments: high nitrogen application (HN), low nitrogen (LN), no fungicides (NF), with fungicides (WF).
This result was also observed in Figure 4, where the gross margin enhancing the effect of fungicide applications decreased over time. The two lines describe the normalized gross margin differences between the treatments with fungicide applications and those without. According to the trial setup, we can additionally distinguish these differences for the treatment groups with low (purple line) and high (turquoise) nitrogen dosage.
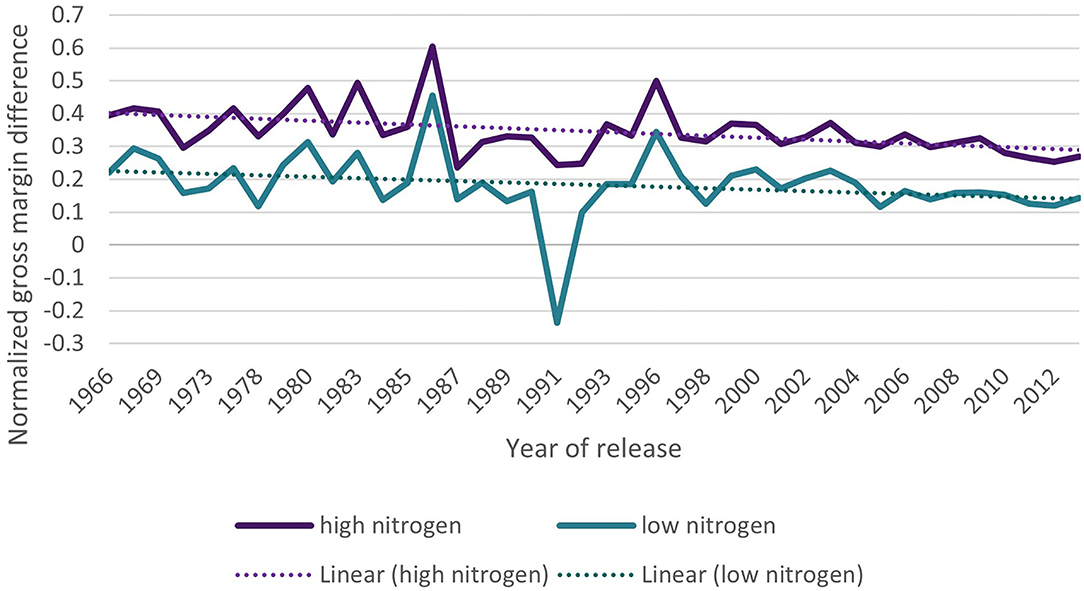
Figure 4. Normalized differences in gross margins (EUR ha-1) between treatments with and without fungicide applications in two trial set-ups with high (purple line) and low (turquoise) nitrogen application; the dotted lines indicate the linear trend.
Overall, the varieties and years of release, the average gross margin difference between a fungicide-treated and untreated system remains 122.0 EUR ha−1 with an SD of 140.0 EUR ha−1. The maximum is 502.3 EUR ha−1 and the minimum −241.3 EUR ha−1. In the untreated control, the average gross margin also increases with higher spike or leaf health (Table 6). For both health indicators, the gross margin is the highest under the low nitrogen treatment.
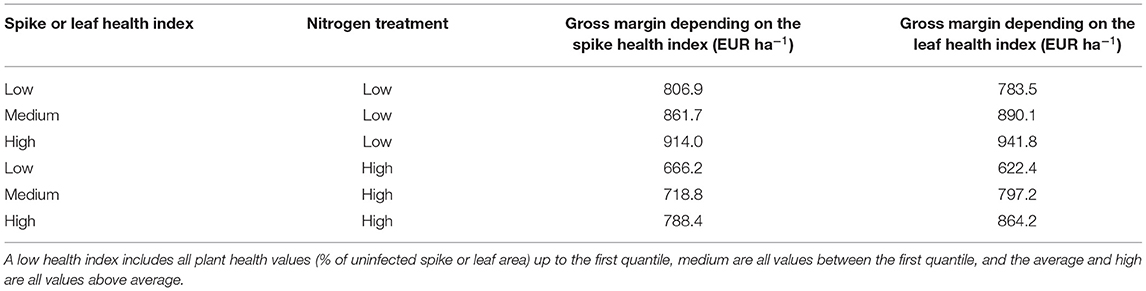
Table 6. Average gross margin according to the spike and leaf health index, as well as the nitrogen treatment for all varieties without fungicide application.
The subset of popular susceptible and resistant German winter wheat varieties is shown in Table 7. We classified those varieties as susceptible that had an average leaf health index <80%, and varieties with a higher index as resistant. The gross margin differences between a fungicide-treated and untreated cultivation are much lower for the resistant varieties. The mean difference is even negative. The difference is larger for susceptible varieties, which profit from fungicide applications as these are needed to secure their health and hence the gross margin.
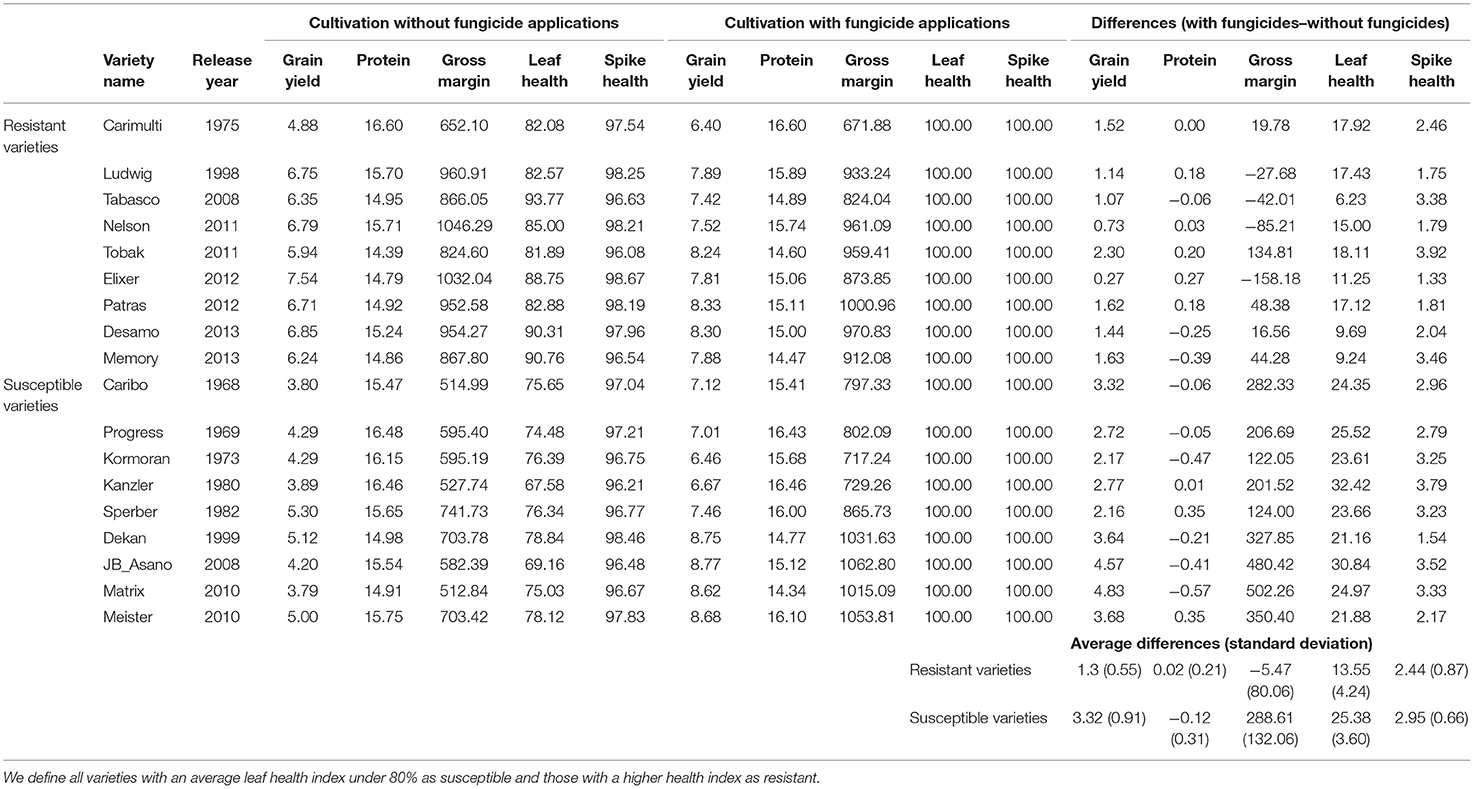
Table 7. Subset of important susceptible and resistant German winter wheat varieties with the variables average grain yield (dt ha−1), protein content (%), gross margin (EUR ha−1), leaf and spike health (uninfected leaf or spike area in %) under the high nitrogen treatment, along with the differences between the variables for the cultivation with and without fungicide applications and average differences overall susceptible and resistant varieties.
For example, consider the case of the resistant variety, Elixer which has large environmental stability. By not taking advantage of its high resistances and applying a full dosage of fungicides, the gross margin decreases, even though the fungicide treatment slightly increased yield, protein, and health levels. On the contrary, Tobak, which is also classified as resistant, produces a higher gross margin under full fungicide treatment as compared to non-application. This is due to the higher susceptibility of the variety to one particular disease, leaf rust. The susceptible variety, JB Asano profits greatly from fungicide applications, which nearly doubles its yield and gross margin. Also, the very popular variety, Dekan shows a large gross margin boosting effect induced by fungicides.
Discussion
Breeding varieties with increased resistance to fungal pathogens is an integral part of plant breeding programs and it was an important goal of the last 50 years of winter wheat breeding around the world, and also in Germany. Such breeding ties up monetary and human resources and yet, so far, the microeconomic impact of resistance breeding has not been analyzed with a field-experimental data set which covers 50 years of breeding progress and which enables us to isolate the purely breeding-induced effect. Our research contributes to filling this research gap. We show that breeding for resistances has created large positive economic effects on winter wheat production in Germany. The results of the regression models show that resistance breeding had a largely positive and statistically significant effect on the microeconomic target variable gross margin (Tables 3, 4; Figures 1, 2). The strong and statistically significant effect of the year of release indicates that younger varieties are more profitable as they contain breeding-induced innovations, which older varieties lack on average. The effect of the resistances to individual fungal pathogens showed that rusts and Fusarium resistances had the largest effect on the economic profitability of the varieties. Confirming our hypothesis, the validation models including the agronomic management variables, show that fungicide applications had a statistically significant and large negative effect on the gross margin of a specific variety. This is due to the high costs for fungicide products and their application. These results indicate that a careful choice of resistant varieties can be more profitable than applying a full dosage of fungicides or high levels of nitrogen. Similarly, high nitrogen fertilization has a negative impact on economic performance. This was true even though in the trials, nitrogen (N) application was reduced to the necessary minimum, which means that in comparison to an average location of German winter wheat cultivation, nitrogen application was low. Due to the given high N-supply capacity of the soil at the trial site, the statements regarding the economic efficiency of the N-fertilizer application cannot be transferred to all sites. On other soils with a lower N-supply capacity, a higher economic efficiency of the N fertilization can be assumed. Appropriate fertilizer management is also important for reducing the disease intensity depending on the nutritional habit of the present pathogen (Simón et al., 2020). Overall, the trial year had a significant effect on the gross margin. As the management and input use are the same across varieties of a certain treatment, this can be explained by the different meta-environments prevailing during each trial year. During the trials, production was mainly limited by the plant-available water during late spring and summer. According to the soil moisture, 2016, the reference year in the models, was the least dry year. In 2015, soil moisture was 29.3%, in 2016 it was 31.0%, and in 2017, it was 29.1%. Hence, during the first and last trial years, plants had to cope with strong drought stress (Zetzsche et al., 2020). Moreover, drought periods need to be considered when interpreting the effects of fertilization, as they caused some fertilizer to remain undissolved so that the effect of fertilization was limited or delayed in these years.
The regression results are backed up with the results of the post-estimation analyses. These show that the gross margin increased over the decades (Figure 3; Table 5) and also with the increased breeding-induced leaf and spike health (Table 6). Furthermore, the difference between fungicide-treated and untreated winter wheat cultivation decreased over time due to increased plant health (Table 5; Figure 4). This underlines that resistant varieties are also profitable without disease management and under biotic pressures from fungal pathogens. The peaks in 1986 and 1996, as well as the strong drop down in 1991 visible in Figure 3, are caused by single varieties with particular behavior that were released in this year. The subset of popular winter wheat varieties (Table 7) shows that on average, the gross margin of more resistant varieties decreases with fungicide applications. Even though the yield and protein contents of the resistant varieties increase slightly with applications, this yield and protein-boosting effect is not large enough to compensate for the high fungicide costs. In general, the trial produced above-average protein contents due to the prevailing dry conditions.
Usual gross margin calculators include the standard gross margin categories revenue, which is influenced by yield quantity, quality, and the producer price, as well as the variable costs of production for inputs, labor, etc. (KTBL, 2020; Mußhoff and Hirschauer, 2020; LfL, 2021). Due to the trial setup and economic analysis method, our variety-specific gross-margin calculations allow us to assess the microeconomic effect of the last 50 years of breeding for resistance to fungal pathogens. Studies on winter wheat found that the continued innovation of breeding in Germany has created higher-yielding crops, which are more resistant and stable across environments and treatment variants (Voss-Fels et al., 2019; Zetzsche et al., 2020). Our research shows that this positive yield effect has translated into increased profitability of more resistant varieties.
The results show that due to their high costs, fungicide applications have a negative effect on the gross margin and that the gross margin-enhancing effect of a full fungicide rate has been diminishing over time. The profitability of fungicide applications depends on many factors: weather conditions, and corresponding disease intensity as well as risks, the efficacy of the applied active ingredients, application costs and rates, timing, agronomic management, the current wheat price, and the variety-specific resistance (Wegulo et al., 2011). Wegulo et al. (2011) conclude that foliar fungicide treatments can be profitable in years with moderate to high disease severity but can be unprofitable when fungicides are used in years with low disease severity. In line with our results, Weisz et al. (2011) found that the costs of the fungicide application need to be offset by yield gains induced by these applications. Therefore, routine applications solely based on the wheat growth stage or general experience are only profitable if such a yield gain is achieved (Weisz et al., 2011). However, when diseases are present, the likelihood that application costs and benefits break even or that benefits outweigh the costs increase (Weisz et al., 2011). In Germany, farmers increasingly plant winter wheat varieties with broad resistances to diseases; nevertheless, the fungicide application intensity in fields planted with such varieties is not significantly different from those planted with less-resistant varieties (Dachbrodt-Saaydeh, 2018). This indicates that the gross margin-enhancing potential of resistant varieties is generally not realized in practice. Weather and other factors, such as available labor and machinery as well as rules and regulations, influence the decision of an operating farm to apply or not apply fungicides (Dachbrodt-Saaydeh, 2018). Analyzing operating farms in Germany, the majority of situation-specific fungicide applications are economically superior to general applications, as farm businesses can save on product and application costs (Kehlenbeck and Rajmis, 2018). In particular, less application can save the overall costs for disease management. Depending on the weather and disease conditions, modern, resistant varieties can allow for some fungicide applications to be skipped within the season and therefore save product and application costs. As described, knowledge of the farmers and their options to allocate farm resources for disease monitoring and other practices are relevant. The high application costs for fungicides can be avoided by not applying fungicides when the variety- and site-specific damage thresholds are not surpassed. This implies using locally adapted disease management systems that adjust according to yearly and local variations in disease intensity (Jalli et al., 2020).
Despite the breeding progress, the results also show that, on average, the grain yield performance was always superior when applying fungicides. Hence, under certain conditions, fungicide applications can be beneficial for increasing yields, also if plants with higher resistance levels are sprayed. This was also found by other trials where the yield advantage highly depended on yield potential, location, and disease conditions of the varieties (Thompson et al., 2014). One explanation for this finding is a possible preventive effect of applying fungicides before a specific fungal pathogen is present. This option can be influenced by the application timing and the applied active ingredients which usually target several pathogens and not only the pathogen for which a damage threshold was surpassed. This preventive effect can decrease plant stress, delay senescence, or prolong the green leaf area retention and hence create plant health and economic benefits (Bertelsen et al., 2001; Wu and von Tiedemann, 2001; Zhang et al., 2010; Wegulo et al., 2011; Berdugo et al., 2012; Lopez et al., 2015). Recent literature analyzing new groups of fungicides reported that apart from the protective attributes of fungicides, they can also positively alter plant physiology so that grain yield and other traits improve (van Dingenen et al., 2017; Amaro et al., 2020). Along with farm resource allocation, these findings can partly explain why some farmers tend to spray fungicides rather as a preventative measure than according to variety- and site-specific disease risks (Rajmis and Kehlenbeck, 2018). Also, plant injuries caused by fungicide applications do not seem to have a yield-decreasing effect (Robinson et al., 2013) that could influence the economic profitability of the crop. Some studies conclude that the profitability of fungicide applications depends on the grain price; hence applications are unprofitable in years with low prices and in particular, when the host plant is resistant (Wiik and Rosenqvist, 2010; Wegulo et al., 2011; Hysing et al., 2012; Thompson et al., 2014). Also, an analysis of 25 years of winter wheat cultivation in southern Sweden found that the average net return from fungicide applications was not more than 12 EUR ha−1, and was even negative in 10 years (Wiik and Rosenqvist, 2010). Contrary to our findings, fungicide treatments were more profitable in the latter part of the trial period from 1995 to 2007 (Wiik and Rosenqvist, 2010). Modeling the fungicide choices of farmers, TeBeest et al. (2013) conclude that in years with high disease severity too low doses of fungicides can result in a net loss; therefore, risk-adverse farmers prefer to apply a high fungicide dosage as insurance to avoid such losses.
It is no surprise for agronomists that the specific meta-environment or weather during the field trials decisively impacted the variety-specific gross margins. Albers et al. (2017) estimated that 43% of the total actual wheat yield volatility is driven by weather. The authors also estimated that 49% of the yield volatility is driven by inputs. Our results indicate that the meta-environment had a much larger impact on the gross margin than inputs. This can be explained by the strong water-stress conditions during the trial years.
A combination of resistant varieties, intensive inventory scoring, and independent consultation is necessary to reduce fungicide applications and decrease the risks thereof (Helbig et al., 2018). Farmers need more actionable, accessible, and affordable information on the resistance traits of varieties along with current weather conditions and subplot specific pathogen risk. Digital farming and data analysis tools, such as non-uniform, sensor-controlled spraying (Tackenberg et al., 2018), can help to pursue site- and variety-specific fungal disease management, while optimizing the farm resource allocation (labor, machinery, etc.). Such tools must be adapted in such a manner that they are profitable for farmers and yet facilitate the achievement of certain standards. Therefore, it is also necessary to better understand the spraying decisions of farmers and what factors influence them. The described positive impacts of resistance breeding on winter wheat production gross margins can be further increased by practitioners, who pair a good variety selection with variety-, pathogen-, and site-specific management. This implies that less fungicide could be applied. Fungicide use could be reduced by only treating the pathogen present on a specific variety and on a specific plot. This way, the resistances of a variety to other pathogens can be exploited, which could imply that no further gross-margin decreasing applications are necessary.
Our analysis is the first to calculate the microeconomic effect of resistance breeding for such a large and historical set of 176 varieties for which we can compare the important traits of grain yield, protein content, plant health, and the resulting gross margin. All varieties received the same variants of agronomic management and growing conditions, which enables us to single out the sole breeding-induced economic effect of breeding for increased resistance to fungal pathogens. Our findings are also relevant for other regions in the world as new and more aggressive strains of pathogens, such as stripe rust are distributed around the world (Carmona et al., 2020). Therefore, international collaboration is needed to sustain the quality and quantity of wheat production and assuring profitable production methods for farmers. Future work on the economics of resistance breeding for wheat could set up trials at locations that differ in their soil conditions, and apply variety-, pathogen-, and site-specific fungicide treatments. Such fungicide application trials could further increase the economic effect of the resistance of varieties. To assure the availability of agricultural innovations, such as more resistant varieties, regulatory reforms, and a public debate with more focus on scientific evidence is needed (Qaim, 2020).
This analysis provides compelling evidence that resistance breeding has created large positive effects on the profitability of winter wheat production. Therefore, for farmers, an optimal choice of resistant varieties can be highly profitable with regard to the gross margin of winter wheat production. Resistances can help decrease input use while producing high-yield quantities and qualities. The use of resistant varieties additionally contributes to climate protection by reducing the carbon footprint of the energy-intensive production of synthetic agrochemicals. Thus, the use of fungicides at full rates is not forward-looking when varieties are carefully chosen and cultivated, neither from an economic nor an environmental perspective. Social and political as well as climatic and ecological pressures make it clear that agricultural producers, stakeholders along the value chain, and policymakers must consider other target indicators, such as minimizing external environmental costs, apart from profit maximization. As wheat is a major staple food around the world, our findings show that resistance breeding can contribute to achieving the United Nations Sustainable Development goals. It can help reduce the poverty levels of wheat-producing farmers while decreasing hunger through larger product quantities and better qualities. It can also enable climate action and improve life on land by reducing potential negative environmental effects of intensive agricultural systems and reducing the pressure on land. Also, the German National Action Plan for Plant Protection intends to reduce fungicide applications to the necessary minimum. This target can be achieved with the support of healthier varieties. Therefore, resistance breeding creates benefits at the farm level and beyond.
Data Availability Statement
The raw data supporting the conclusions of this article will be made available by the authors, without undue reservation.
Author Contributions
SL organized and contributed to the research conceptualization and design, data gathering and analysis, and also wrote and edited the manuscript. HZ, BW, AS, and OM contributed to the research conceptualization and design, data provision, and analysis as well as manuscript editing. FO lead the project, whose data was used for the here presented research, and edited the manuscript. All authors contributed to the article and approved the submitted version.
Funding
This work was supported by the Federal Ministry of Education and Research (BMBF Grant No: 031A354 E and F). We acknowledge support by the German Research Foundation (DFG) and the Open Access Publication Fund of Humboldt-Universität zu Berlin.
Conflict of Interest
The authors declare that the research was conducted in the absence of any commercial or financial relationships that could be construed as a potential conflict of interest.
Publisher's Note
All claims expressed in this article are solely those of the authors and do not necessarily represent those of their affiliated organizations, or those of the publisher, the editors and the reviewers. Any product that may be evaluated in this article, or claim that may be made by its manufacturer, is not guaranteed or endorsed by the publisher.
Acknowledgments
We would like to thank the consortium Breeding Innovations in Wheat for Resilient Cropping Systems (BRIWECS) consortia that gave us data access and support.
References
Agrarzeitung (2020). Mein Markt–Kassamarkt Hamburg. Available online at: https://www.agrarzeitung.de/mein-markt/ (accessed October 16, 2020).
Ahlemeyer, J., and Friedt, W. (2010). Progress in winter wheat yield in Germany-what's the share of the genetic gain? in 61 Tagung der Vereinigung der Pflanzenzüchter und Saatgutkaufleute Österreichs (Raumberg-Gumpenstein), 19–23.
Albers, H., Gornott, C., and Hüttel, S. (2017). How do inputs and weather drive wheat yield volatility? the example of Germany. Food Policy 70, 50–61. doi: 10.1016/j.foodpol.2017.05.001
Amaro, A. C. E., Baron, D., Ono, E. O., and Rodrigues, J. D. (2020). Physiological effects of strobilurin and carboxamides on plants: an overview. Acta Physiol. Plant 42:4. doi: 10.1007/s11738-019-2991-x
Berdugo, C. A., Steiner, U., Dehne, H.-W., and Oerke, E.-C. (2012). Effect of bixafen on senescence and yield formation of wheat. Pestic. Biochem. Physiol. 104, 171–177. doi: 10.1016/j.pestbp.2012.07.010
Bertelsen, J. R., Neergaard, E., and de Smedegaard-Petersen, V. (2001). Fungicidal effects of azoxystrobin and epoxiconazole on phyllosphere fungi, senescence and yield of winter wheat. Plant Pathol. 50, 190–205. doi: 10.1046/j.1365-3059.2001.00545.x
BMEL (2021). Statistisches Jahrbuch über Ernährung, Landwirtschaft und Forsten der Bundesrepublik Deutschland 2020. Bonn: BMEL.
Carmona, M., Sautua, F., Pérez-Hérnandez, O., and Reis, E. M. (2020). Role of fungicide applications on the integrated management of wheat stripe rust. Front. Plant Sci. 11:733. doi: 10.3389/fpls.2020.00733
Clifford, B. C., and Lester, E.,. (1988). Control of Plant Diseases: Costs and Benefits. Oxford: Blackwell Scientific.
Cormier, F., Foulkes, J., Hirel, B., Gouache, D., Moënne-Loccoz, Y., and Le Gouis, J. (2016). Breeding for increased nitrogen-use efficiency: a review for wheat (T. aestivum L.). Plant Breed 135, 255–278. doi: 10.1111/pbr.12371
Croissant, Y., and Millo, G. (2008). Panel data econometrics in R: the plm Package. J. Stat. Softw. 27, 1–43. doi: 10.18637/jss.v027.i02
Dachbrodt-Saaydeh, S. (2018). Ergebnisse aus 10 Jahren Untersuchungen zum notwendigen Maß im Netz Vergleichsbetriebe Pflanzenschutz. Gesunde Pflanzen 70, 147–153. doi: 10.1007/s10343-018-0423-2
Dixon, J., Nalley, L., Kosina, P., La Rovere, R., Hellin, J., and Aquino, P. (2006). Adoption and economic impact of improved wheat varieties in the developing world. J. Agric. Sci. 144, 489–502. doi: 10.1017/S0021859606006459
Dubin, H., and Brennan, J. P. (2009). Combating Stem and Leaf Rust of Wheat: Historical Perspective, Impacts, and Lessons Learned. Washington, DC: International Food Policy Research Institute
Figlan, S., Ntushelo, K., Mwadzingeni, L., Terefe, T., Tsilo, T. J., and Shimelis, H. (2020). Breeding wheat for durable leaf rust resistance in Southern Africa: variability, distribution, current control strategies, challenges and future prospects. Front. Plant Sci. 11:549. doi: 10.3389/fpls.2020.00549
Figueroa, M., Hammond-Kosack, K. E., and Solomon, P. S. (2018). A review of wheat diseases-a field perspective. Mol. Plant Pathol. 19, 1523–1536. doi: 10.1111/mpp.12618
Fischer, T., Byerlee, D., and Edmeades, G. (2014). Crop yields and Global Food Security: Will Yield Increase Continue to Feed the World? Canberra, ACT: ACIAR.
Ghimire, B., Sapkota, S., Bahri, B. A., Martinez-Espinoza, A. D., Buck, J. W., and Mergoum, M. (2020). fusarium head blight and rust diseases in soft red winter wheat in the Southeast United States: state of the art, challenges, and future perspective for breeding. Front. Plant Sci. 11:1080. doi: 10.3389/fpls.2020.01080
Helbig, J., Paap, M., Kehlenbeck, H., Gummert, A., and Freier, B. (2018). 7 years “Demonstration Farms for Integrated Pest Manangement” in arable farming–results and interim conclusion, in Julius-Kühn-Archiv, Nr. 461 (2018): 61. Deutsche Pflanzenschutztagung “Herausforderung Pflanzenschutz–Wege in die Zukunft,” 11. - 14. September 2018, Universität Hohenheim: Kurzfassungen der Vorträge und Poster, ed JKI (Stuttgart: Julius-Kühn-Archiv), 94–95.
Hogenboom, N. G. (1993). Economic importance of breeding for disease resistance, in Durability of Disease Resistance, eds T. Jacobs and J. E. Parlevliet (Dordrecht: Springer Netherlands), 5–9.
Hovmøller, M. S., and Justesen, A. F. (2007). Appearance of atypical Puccinia striiformis f. sp. tritici phenotypes in north-western Europe. Aust. J. Agric. Res. 58:518. doi: 10.1071/AR06146
Hysing, S.-C., Rosenqvist, H., and Wiik, L. (2012). Agronomic and economic effects of host resistance vs. fungicide control of barley powdery mildew in southern Sweden. Crop Protect. 41, 122–127. doi: 10.1016/j.cropro.2012.05.010
Jalli, M., Kaseva, J., Andersson, B., Ficke, A., Nistrup-Jørgensen, L., Ronis, A., et al. (2020). Yield increases due to fungicide control of leaf blotch diseases in wheat and barley as a basis for IPM decision-making in the Nordic-Baltic region. Eur. J. Plant Pathol. 158, 315–333. doi: 10.1007/s10658-020-02075-w
Kehlenbeck, H. (2018). Tendenzen bei der Anwendung von Pflanzenschutzmitteln in Deutschland, in Thüringer Düngungs–und Pflanzenschutztagung, November 15 (New York, NY).
Klocke, B., Sommerfeldt, N., Baumecker, M., Ellmer, F., Jacobi, A., Petersen, J., et al. (2018). Possibilities and limits of resistant winter wheat cultivars to minimize fungicide applications, in Julius-Kühn-Archiv, Nr. 461 (2018): 61. Deutsche Pflanzenschutztagung „Herausforderung Pflanzenschutz – Wege in die Zukunft”, 11–14. September 2018, Universität Hohenheim: Kurzfassungen der Vorträge und Poster, ed JKI (Stuttgart: Julius-Kühn-Archiv), 130–131.
KTBL (2020). Leistungs-Kostenrechnung Pflanzenbau. Available online at: https://www.ktbl.de/webanwendungen/leistungs-kostenrechnung-pflanzenbau (accessed october 02, 2020).
Laidig, F., Piepho, H.-P., Drobek, T., and Meyer, U. (2014). Genetic and non-genetic long-term trends of 12 different crops in German official variety performance trials and on-farm yield trends. Theor. Appl. Genet. 127, 2599–2617. doi: 10.1007/s00122-014-2402-z
Lantican, M. A., Braun, H.-J., Payne, T. S., Singh, R. P., Sonder, K., Baum, M., et al. (2016). Impacts of international wheat improvement research: 1994-2014. Mexico: CIMMYT.
LfL (2021). Winterweizen–LfL Deckungsbeiträge und Kalkulationsdaten. Available online at: https://www.stmelf.bayern.de/idb/winterweizen.html (accessed April 23, 2021).
Lopez, J. A., Rojas, K., and Swart, J. (2015). The economics of foliar fungicide applications in winter wheat in Northeast Texas. Crop Protect. 67, 35–42. doi: 10.1016/j.cropro.2014.09.007
Mackay, I., Horwell, A., Garner, J., White, J., McKee, J., and Philpott, H. (2011). Reanalyses of the historical series of UK variety trials to quantify the contributions of genetic and environmental factors to trends and variability in yield over time. Theor. Appl. Genet. 122, 225–238. doi: 10.1007/s00122-010-1438-y
Marasas, C. N., Smale, M., and Singh, R. P. (2003). The economic impact of productivity maintenance research: breeding for leaf rust resistance in modern wheat. Agric. Econ. 29, 253–263. doi: 10.1111/j.1574-0862.2003.tb00162.x
Martynov, S., Dobrotvorskaya, T., and Dobrotvorskiy, D. (2017). Genetic Resources Information System for Wheat and Triticale (GRIS). Available online at: http://wheatpedigree.net/ (accessed October 16, 2020).
Mußhoff, O., and Hirschauer, N. (2020). Modernes Agrarmanagement: Betriebswirtschaftliche Analyse- und Planungsverfahren. München: Verlag Franz Vahlen.
Nalley, L. L., Barkley, A. P., and Featherstone, A. M. (2010). The genetic and economic impact of the CIMMYT wheat breeding program on local producers in the Yaqui Valley, Sonora Mexico. Agric. Econ. 41, 453–462. doi: 10.1111/j.1574-0862.2010.00457.x
Piepho, H.-P., Laidig, F., Drobek, T., and Meyer, U. (2014). Dissecting genetic and non-genetic sources of long-term yield trend in German official variety trials. Theor. Appl. Genet. 127, 1009–1018. doi: 10.1007/s00122-014-2275-1
Qaim, M. (2020). Role of new plant breeding technologies for food security and sustainable agricultural development. Appl. Econ. Perspect. Policy 42, 129–150. doi: 10.1002/aepp.13044
Rajmis, S., and Kehlenbeck, H. (2018). Economic assessment of wheat cropping systems with focus on resistance breeding (AWECOS), in Julius-Kühn-Archiv, Nr. 461 (2018): 61. Deutsche Pflanzenschutztagung “Herausforderung Pflanzenschutz–Wege in die Zukunft,” 11–14. September 2018, Universität Hohenheim: Kurzfassungen der Vorträge und Poster, ed JKI (Stuttgart: Julius-Kühn-Archiv), 245–246.
Robinson, M. A., Cowbrough, M. J., Sikkema, P. H., and Tardif, F. J. (2013). Winter wheat (Triticum aestivum L.) tolerance to mixtures of herbicides and fungicides applied at different timings. Can. J. Plant Sci. 93, 491–501. doi: 10.4141/cjps2012-181
Roßberg, D. (2016). Survey on application of chemical pesticides in agriculture//Erhebungen zur Anwendung von Pflanzenschutzmitteln im Ackerbau. J. Cultivat. Plants 68, 25–37. doi: 10.5073/JFK.2016.02.01
Russell, G. E. (2013). Plant Breeding for Pest and Disease Resistance: Studies in the Agricultural and Food Sciences. Burlington: Elsevier Science.
Savary, S., Willocquet, L., Pethybridge, S. J., Esker, P., McRoberts, N., and Nelson, A. (2019). The global burden of pathogens and pests on major food crops. Nat. Ecol. Evol. 3, 430–439. doi: 10.1038/s41559-018-0793-y
Schwarz, J., Klocke, B., Wagner, C., and Krengel, S. (2018). Untersuchungen zum notwendigen Maß bei der Anwendung von Pflanzenschutzmitteln in Winterweizen in den Jahren 2004 bis 2016. GesundePflanzen 70, 119–127. doi: 10.1007/s10343-018-0422-3
Shiferaw, B., Smale, M., Braun, H.-J., Duveiller, E., Reynolds, M., and Muricho, G. (2013). Crops that feed the world 10. Past successes and future challenges to the role played by wheat in global food security. Food Sec. 5, 291–317. doi: 10.1007/s12571-013-0263-y
Simón, M. R., Börner, A., and Struik, P. C. (2021). Editorial: fungal wheat diseases: etiology, breeding, and integrated management. Front. Plant Sci. 12:498. doi: 10.3389/fpls.2021.671060
Simón, M. R., Fleitas, M. C., Castro, A. C., and Schierenbeck, M. (2020). How foliar fungal diseases affect nitrogen dynamics, milling, and end-use quality of wheat. Front. Plant Sci. 11:569401. doi: 10.3389/fpls.2020.569401
Singh, R. P., and Rajaram, S. (2002). “Breeding for disease resistance in wheat,” in Bread Wheat: Improvement and Production, eds B. C. Curtis, S. Rajaram, and H. Gómez Macpherson (Rome: Food and Agriculture Organization of the United Nations), 345–366.
Singh, R. P., Singh, P. K., Rutkoski, J., Hodson, D. P., He, X., Jørgensen, L. N., et al. (2016). Disease impact on wheat yield potential and prospects of genetic control. Annu. Rev. Phytopathol. 54, 303–322. doi: 10.1146/annurev-phyto-080615-095835
Smale, M., Singh, R. P., Sayre, K., Pingali, P., Rajaram, S., and Dubin, H. J. (1998). Estimating the economic impact of breeding nonspecific resistance to leaf rust in modern bread wheats. Plant Dis. 82, 1055–1061. doi: 10.1094/PDIS.1998.82.9.1055
Sommerfeldt, N. (2018). Hingucken lohnt sich. DLG-Mitteilungen 2018, 52–55. doi: 10.30963/aramit5509
Tackenberg, M., Volkmar, C., Schirrmann, M., Giebel, A., and Dammer, K.-H. (2018). Impact of sensor-controlled variable-rate fungicide application on yield, senescence and disease occurrence in winter wheat fields. Pest Manag. Sci. 74, 1251–1258. doi: 10.1002/ps.4843
TeBeest, D. E., Paveley, N. D., Shaw, M. W., and van den Bosch, F. (2013). Accounting for the economic risk caused by variation in disease severity in fungicide dose decisions, exemplified for Mycosphaerellagraminicola on winter wheat. Phytopathology 103, 666–672. doi: 10.1094/PHYTO-05-12-0119-R
Thompson, N. M., Epplin, F. M., Edwards, J. T., and Hunger, R. M. (2014). Economics of foliar fungicides for hard red winter wheat in the USA southern Great Plains. Crop Protect. 59, 1–6. doi: 10.1016/j.cropro.2014.01.009
Torres-Reyna, O. (2010). Getting Started in Random Effects Models using R: (ver. 0.1 Draft). Princeton: Princeton University
van Dingenen, J., Antoniou, C., Filippou, P., Pollier, J., Gonzalez, N., Dhondt, S., et al. (2017). Strobilurins as growth-promoting compounds: how Stroby regulates Arabidopsis leaf growth. Plant Cell Environ. 40, 1748–1760. doi: 10.1111/pce.12980
Voss-Fels, K. P., Stahl, A., Wittkop, B., Lichthardt, C., Nagler, S., Rose, T., et al. (2019). Breeding improves wheat productivity under contrasting agrochemical input levels. Nat. Plants 5, 706–714. doi: 10.1038/s41477-019-0445-5
Wegulo, S. N., Zwingman, M. V., Breathnach, J. A., and Baenziger, P. S. (2011). Economic returns from fungicide application to control foliar fungal diseases in winter wheat. Crop Protect. 30, 685–692. doi: 10.1016/j.cropro.2011.02.002
Weisz, R., Cowger, C., Ambrose, G., and Gardner, A. (2011). Multiple mid-Atlantic field experiments show no economic benefit to fungicide application when fungal disease is absent in winter wheat. Phytopathology 101, 323–333. doi: 10.1094/PHYTO-03-10-0096
Wiik, L., and Rosenqvist, H. (2010). The economics of fungicide use in winter wheat in southern Sweden. Crop Protect. 29, 11–19. doi: 10.1016/j.cropro.2009.09.008
Wu, Y.-X., and von Tiedemann, A. (2001). Physiological effects of azoxystrobin and epoxiconazole on senescence and the oxidative status of wheat. Pestic. Biochem. Physiol. 71, 1–10. doi: 10.1006/pest.2001.2561
Zetzsche, H., Ordon, F., and Friedt, W. (2020). Breeding progress for pathogen resistance is a major driver for yield increase in German winter wheat at contrasting N levels. Sci. Rep. 10:20374. doi: 10.1038/s41598-020-77200-0
Zetzsche, H., Serfling, A., and Ordon, F. (2019). Breeding progress in seedling resistance against various races of stripe and leaf rust in european bread wheat. Crop Breed Genet. Genom. 1:e190021. doi: 10.20900/cbgg20190021
Zhang, Y.-J., Zhang, X., Chen, C.-J., Zhou, M.-G., and Wang, H.-C. (2010). Effects of fungicides JS399-19, azoxystrobin, tebuconazloe, and carbendazim on the physiological and biochemical indices and grain yield of winter wheat. Pestic. Biochem. Physiol. 98, 151–157. doi: 10.1016/j.pestbp.2010.04.007
Annex: Model Diagnostics
Keywords: gross margin analysis, economics, winter wheat breeding, resistance breeding, Germany, Europe, disease management, agronomy
Citation: Lüttringhaus S, Zetzsche H, Wittkop B, Stahl A, Ordon F and Mußhoff O (2021) Resistance Breeding Increases Winter Wheat Gross Margins–An Economic Assessment for Germany. Front. Agron. 3:730894. doi: 10.3389/fagro.2021.730894
Received: 25 June 2021; Accepted: 26 August 2021;
Published: 30 September 2021.
Edited by:
Giovanni Bubici, Institute for Sustainable Plant Protection, National Research Council (CNR), ItalyReviewed by:
Kirstin Verity Wurms, The New Zealand Institute for Plant and Food Research Ltd., New ZealandFernanda Maria Gamba, Universidad de la República, Uruguay
Copyright © 2021 Lüttringhaus, Zetzsche, Wittkop, Stahl, Ordon and Mußhoff. This is an open-access article distributed under the terms of the Creative Commons Attribution License (CC BY). The use, distribution or reproduction in other forums is permitted, provided the original author(s) and the copyright owner(s) are credited and that the original publication in this journal is cited, in accordance with accepted academic practice. No use, distribution or reproduction is permitted which does not comply with these terms.
*Correspondence: Sophia Lüttringhaus, luettria@hu-berlin.de