- 1Plant Science Department, McGill University, Montreal, QC, Canada
- 2Laboratoire d'Écologie Microbienne, Institut de Recherche et de Développement en Agroenvironnement (IRDA), Montreal, QC, Canada
The use of biological inputs in crop production systems, as complements to synthetic inputs, is gaining popularity in the agricultural industry due to increasing consumer demand for more environmentally friendly agriculture. An approach to meeting this demand is the inoculation of field crops with beneficial microbes to promote plant growth and resistance to biotic and abiotic stresses. However, the scientific literature reports inconsistent results following applications of bio-inoculant to fields. The effects of inoculation with beneficial microbes on bulk soil and rhizospheric microbial communities is often overlooked as precise monitoring of soil microbial communities is difficult. The aim of this research was to use Illumina high throughput sequencing (HTS) to shed light on bulk soil and rhizospheric microbial community responses to two commercial microbial inoculants coated onto fertilizer granules, applied to potato fields. Bulk soil samples were collected 4 days before seeding (May 27th), 7 days after seeding (June 7th), at potato shoot emergence (June 21st) and at mid-flowering (July 26th). Rhizospheric soil was collected at the mid-flowering stage. The Illumina MiSeq HTS results indicated that the bulk soil microbial community composition, especially prokaryotes, changed significantly across potato growth stages. Microbial inoculation did not affect bulk soil or rhizospheric microbial communities sampled at the mid-flowering stage. However, a detailed analysis of the HTS results showed that bulk soil and rhizospheric microbial community richness and composition were different for the first treatment block compared to the other three blocks. The spatial heterogeneity of the soil microbial community between blocks of plots was associated with potato tuber yield changes, indicating links between crop productivity and soil microbial community composition. Understanding these links could help in production of high-quality microbial inoculants to promote potato productivity.
Introduction
In comparison to average food demand between 2005 and 2007, we will need to produce 60% more food by 2050 to meet the global demand (Alexandratos and Bruinsma, 2012). At the same time, drastic changes are needed in agricultural management practices, to be more sustainable and to reduce greenhouse gases emissions. The use of bioproducts in agriculture, to replace synthetic inputs such as fertilizers and pesticides, is an environmentally friendly approach that may be able to maintain high levels of crop productivity (Dobbelaere et al., 2003). A portion of these bioproducts are based on plant growth-promoting rhizobacteria (PGPR). These exogenous bacteria have beneficial effects on plant development and resilience to stress (Castro-Sowinski et al., 2007; Smith et al., 2017).
Plant growth promotion by plant-associated microbes occurs through various direct modes of action, such as nutrient synthesis (e.g., N2 fixation), enhanced nutrient uptake (e.g., phosphate solubilisation), phytohormone production (auxins, gibberellins, and cytokinins) and abiotic stress alleviation (Pérez-Montaño et al., 2014; Smith et al., 2015; Antar et al., 2021). PGPR can also improve plant growth by interfering with other microbes in the rhizosphere. Examples of these indirect benefits include the production of anti-microbial compounds or interference with microbial quorum sensing mechanisms (Pérez-Montaño et al., 2014; Nazari and Smith, 2020). Microbial genera such as Bacillus, Pseudomonas, Lactobacillus, and Actinobacteria include strains known for their plant-beneficial effects (Borriss, 2011; Sivasakthi et al., 2014; Fukami et al., 2018). Commercial microbial inoculant formulations include PGPR which, when applied as a seed treatment or as a soil drench, boost plant productivity and resistance to environmental stresses (Kloepper et al., 1989). However, commercial inoculants can be of poor qualities with low quantity of viable propagules, leading to inconsistent results under field conditions (Herrmann and Lesueur, 2013; Gange and Gadhave, 2018). These microbial inoculants proliferate in the vicinity of plant roots and within them, allowing them to trade-off their beneficial effects by benefiting from root exudates.
Most published studies on the application of PGPR have focused exclusively on plant variables, overlooking the persistence of inoculants in soil and their impacts on bulk soil and rhizosphere microbial communities (Rilling et al., 2019). Among the few studying the impact of PGPR application, most have focused on rhizospheric microbial communities (Mendes et al., 2011; Dagher et al., 2019). The bulk soil microbiome encompasses all soil microbial communities while the rhizospheric soil microbiome consists of microbial communities associated with soil particles closely adhering to roots (Mendes et al., 2011; Dagher et al., 2019). However, the rhizospheric microbiome may also encompass microbial communities found inside roots in addition to those found with soil particles adhering to roots (Tian and Gao, 2014). The relatively recent Illumina MiSeq HTS technology allows assessment of small changes to soil microbial communities that could have been overlooked by previous studies using culture-dependent or less sensitive molecular methods. For instance, Deng et al. (2019) showed that frequent applications of biological inputs can induce changes among bulk soil microbial communities using a HTS approach.
Within conventional agricultural production systems of Southern Quebec, where the study was conducted, the initial source of mineral fertilizer nutrients in row crops is applied at planting. The mineral fertilizer is applied in furrow, banded 5 × 5 cm (below and beside) away from the seed. In regions where seeding is done following cold winters, this banded mineral fertilizer is the main nutrient source for plants until the soil warms and nutrients are mineralized through organic matter decomposition. Placement of microbial inoculants during seeding operations is usually either on the seed, in furrow with a solid or a liquid carrier or broadcasted on the soil with a solid carrier. Previous research has indicated no difference in corn yield using either seed coating or in-furrow application (Leggett et al., 2015).
In this study, we examined bulk soil and rhizospheric microbial communities of a potato field following the banded application at seeding of granular fertilizer coated with an EVL Coating® inoculum (EVL, St-Hyacinthe, QC, Canada) or an Éra Boost® inoculum (Ulysse Biotech, Trois-Rivière, QC, Canada). We hypothesized that the banded application of granular fertilizer coated with either commercial microbial consortia of PGPR promotes plant growth by inducing favorable changes in bulk soil and rhizospheric microbial communities. We focused on two objectives by sampling bulk and rhizospheric soils: (1) to determine the impacts of granular fertilizer coated with a microbial inoculant on soil bacterial and fungal microbiomes and (2) to determine if the microbial species inoculated could be detected by HTS analysis in bulk or rhizospheric soils sampled at three time points following potato seeding. This is the first report on granular fertilizer used as a carrier for microbial inoculants and their potential impact on potato yield and on bulk and rhizsopheric soil microbial communities.
Materials and Methods
Microbial Inoculant Descriptions
The microbial inoculants used in this experiment were chosen for being able to maintain a high level of viability of microorganisms on granular fertilizer. The inoculants were Éra boost® (EB) or EVL Coating® (EVL). EB is composed of five Bacillus strains that were screened for their plant-growth promoting effects. As all strains in the EB consortia can sporulate, EB microorganisms can resist hostile environments such as granular fertilizer surface. EVL is composed of a consortium containing two Bacillus, one Lactobacillus, one Pseudomonas, and one Saccharomyces strains all combined in a bioreactor along with a proprietary substrate. The EVL consortium is added to a formulation designed for coating granular fertilizer (Garcia-Fraile et al., 2015).
Experimental Design and Sampling Methods
A field experiment was conducted on a fine sandy soil located in St-Thomas de Joliette (46°00′01.8″N 73°21′02.2″W) during 2019. Three treatments were investigated: (1) un-inoculated control (mineral fertilizer only), (2) EB or, (3) EVL inoculants. Each product was coated onto granular starter fertilizer (GSF) applied at seeding. For all treatments, the rate of GSF was 1,050 kg ha−1 with an NPK equivalent of 12–14–10 kg T−1 of GSF (Synagri, St-Hyacinthe, QC, Canada). The microbial inoculant was applied at 1 L T−1 of fertilizer for EB and 2 L T−1 of fertilizer for EVL, following the manufacturer's recommendations. The fertilizer was banded as a 5 × 5 × 5 cm application, in such a way that fertilizer was placed 5 cm to each side of the seed tuber and both strips were 5 cm below the potato seed tuber. All treatments were replicated seven times and the experiment was structured following a randomized complete block design; four of the seven blocks were used to sample bulk soil and potato roots with closely adhering soil.
Each bulk soil sample consisted of four initial samples taken at the extremities of a 2 × 2 m2 quadrant, pooled together. Bulk soil samples were collected directly on the potato row, between the seed tuber and the fertilizer rows. Within each quadrant, a 2.5 cm-diameter probe was used to sample soil to a depth of 30 cm. Samples were collected at four time points: (1) 4 days before seeding (May 27th), (2) 7 days after seeding (June 7th), (3) 21 days after seeding at shoot emergence stage (June 21st), and (4) 56 days after seeding at mid-flowering stage (July 26th). At the fourth time point, rhizospheric samples were also collected. Each rhizospheric sample consisted of fine roots and closely adhering soil sampled from three plants randomly selected from each plot. Samples of bulk and rhizospheric soils were immediately placed on ice until the arrival at the laboratory.
For each composite bulk soil sample, a representative subsample of about 100 g was prepared and stored at −20°C. Around 400 mg of the 100 g were collected in 2 mL collection tubes before DNA extraction. For each rhizospheric composite sample, a subsample of homogenized roots and closely adhering roots of 1 g was ground with liquid nitrogen. After grinding, 200 mg were collected and stored at −20°C in 2 mL collection tubes, for DNA extraction.
Soil Physicochemical Analysis
Analysis of soil chemical properties was conducted by Laboratoire Géosol (St-Hyacinthe, QC, Canada). P, K, Mg, Ca, Al, Zn, Mn, Cu, Fe, and B were extracted with Mehlich III solution (Mehlich, 1984) and quantified by Inductively Coupled Plasma Optical Emission Spectroscopy (ICP-OES, Perkin Elmer Optima 4,300 DV, Shelton, CT). Soil pHwater, pHSMP and organic matter content were estimated with a 1:1 dilution in water (Hendershot et al., 1993), SMP (Shoemaker et al., 1961) and Walkley-Black solutions (Walkley and Black, 1934), respectively.
DNA Extraction and Sequencing
All DNA extractions were performed using a FastDNA Spin Kit for Soil (MPBio, Irvine, CA, USA). Bulk soil and rhizospheric subsamples were added to tubes containing 1.4 g of the bead matrix E and 1 mL of the lysis buffer supplied with the kit. Microbial inoculant samples consisted of the centrifuged cells contained in 5 mL of each commercial product. DNA extraction was performed according to the manufacturer's instructions. The resulting DNA pellet was suspended in 100 μL of sterile molecular-grade water.
The quality and quantity of the DNA extracts were evaluated by spectrophotometry using a Biophotometer (Eppendorf, Mississauga, ON, Canada) with a G1.0 μCuvette (Eppendorf, Mississauga, ON, Canada) with readings at 260, 280, 230, and 320 nm. The V4 region of prokaryota (archaea and bacteria) rRNA 16S gene was amplified using 515FB and 806RB primers (Apprill et al., 2015; Parada et al., 2016). For the fungi, the eukaryotic (fungal) ITS1 gene was amplified (Bokulich and Mills, 2013). Both genes were amplified in a two-step dual approach PCR designed for Illumina instruments by Plateforme d'analyses génomiques (IBIS, Université Laval, Quebec City, QC, Canada). DNA sequencing was performed by IBIS on an Illumina MiSeq platform, following the methods of Jeanne et al. (2019).
Obtained sequences were demultiplexed based on the tag used. Sequence quality control and feature table construction was performed using QIIME2 (Caporaso et al., 2010) and DADA2 plugin (Callahan et al., 2016). Reference databases Greengenes 13.8 (Desantis et al., 2006) and UNITE version 8 (Kõljalg et al., 2013) were used for taxonomic identification of amplicon sequence variants (Callahan et al., 2017).
Quantitative PCR of Bacterial and Fungal DNA
From the isolated DNA, 4 μL of DNA diluted at 1:20 in molecular-grade water was mixed with 6 μL of a master mix of SYBR green qPCR mix (Qiagen, Toronto, ON, Canada). Samples were prepared on 96-well plates with four water-filled wells acting as blanks. PCR conditions were 15 min at 95°C, followed by 40 cycles of 95°C for 1 min, 30 s at the annealing temperature and 72°C for 1 min. For prokaryotic DNA, the primer pair EUB-338/518 was used with an annealing temperature of 53°C (Fierer et al., 2005). For fungal DNA, the primer pair FF390/FR1 was used with an annealing temperature of 51°C (Emerson et al., 2015) all samples were replicated at least twice. Standard curves were generated from a known quantity of amplified DNA fragments, diluted over a 4-log range (efficiency of 89.1% and r2 = 0.99 for prokaryotes; efficiency of 91.7% and r2 = 1 for fungi). Fluorescence detection was performed on a CFX96 (Biorad, Hercules, CA, USA).
Amplification units (AU) were derived from the average CT values of samples using linear regressions designed by the Microbial Ecology Laboratory of Institut de Recherche et Développement en Agroenvironnement (IRDA) in which samples with known concentrations of DNA were used to fit the regressions. AU g−1 of dry soil were obtained using moisture content and the weight of soil used during DNA extraction.
Downstream Data Analysis and Statistical Analysis
The entire analysis was performed using the R software with version 4.3 R, Core Team (2020). Before doing alpha and beta diversity analysis, normalization of the number of reads was performed using the rarefy-even-depth function from the phyloseq R package (Mcmurdie and Holmes, 2014).
Alpha diversity was measured by Shannon and Chao1 indices, evenness and number of observed ASVs. A three-way ANOVA was performed on alpha-diversity measurements of soil samples with microbial inoculants product, sampling time and block numbers as the three effects. A two-way ANOVA was performed on alpha-diversity measurements of rhizospheric samples with microbial inoculants product and block as effects. Bonferroni adjustment was used to determine significant differences during post-hoc testing.
To compare alpha-diversity measurements and relative abundances of certain microbial genera between rhizospheric and bulk soil communities at the same sampling date (July 26th), a paired T-test of rhizospheric against bulk soil samples was performed.
Beta-diversity was calculated using Bray-Curtis dissimilarity matrices with the ordinate function from the phyloseq package. Ordination of bulk soil and rhizospheric samples was determined using principal coordinate analysis (PCoA). Distance matrices were computed using the distance function from phyloseq. PERMANOVA was performed on these distance matrices using the Adonis function from the Vegan package with the explanatory variables set as microbial treatment, sampling date and treatment block number and 999 permutations. A post-hoc test was conducted using the pairwiseAdonis package with p-values adjusted using a Bonferroni adjustment.
A distance-based redundancy analysis (dbRDA) was performed using the “capscale” function from the Vegan package. Prokaryotic and fungal ASV features table was used with all soil chemical variables measured. PERMANOVA of soil chemical variables revealed statistically significant variables. These variables were represented as vectors on a constrained ordination for both prokaryotic and fungal communities.
Differentially abundant ASVs between the first block and the other three blocks were identified for bulk soil and rhizospheric soil. To identify these ASVs, the DESeq2 package was used (Love et al., 2014). To convert the biom files in DESeq files, the phyloseq_to_deseq2 function from phyloseq was used.
Potato tuber yield expressed as T ha−1 were analyzed following a two-way ANOVA with microbial inoculant and block number as explanatory variables. Physicochemical properties of soil such as CEC, organic matter content, pH and P, Al, K, Mg, Ca, Zn, Mn, Cu, Fe, and B contents were analyzed following a three-way ANOVA with microbial inoculant, block number and sampling time as explanatory variables. The Tukey post-hoc test was used to differentiate significantly different pairwise comparisons for yield and physicochemical values.
Results
Potato Tuber Yield
Potato tuber yield (Table 1) was not significantly impacted by microbial inoculation of granular fertilizer (P = 0.396). The block number of each plot had a significant effect on tuber yield (P = 0.001). Tukey post-hoc test revealed that block 1 had significantly lower yields than block 2 (Padj = 0.004), 3 (Padj = 0.002), and 4 (Padj = 0.004) while blocks 2, 3, and 4 were not statistically different from each other.
Microbial Inoculant Compositions and Fate in Bulk and Rhizospheric Soils
The HTS analysis of EVL bacterial and fungal mixed inoculant provided 17,110 and 16,311 reads, respectively, for prokaryotic and fungal communities. EVL inoculant was quite diverse with 30 ASVs encompassing 15 prokaryotic and 15 fungal ASVs. In EVL inoculant the major prokaryotic genera were Lactobacillus and Clostridium, and the major fungal genus was Saccharomyces (Figures 1A,B). The HTS analysis of EB bacterial inoculant provided 21,950 reads for the prokaryotic community. The eight ASVs identified in EB all belonged to the order Bacillales with seven Bacillus and one Paenibacillus (Figure 1A).
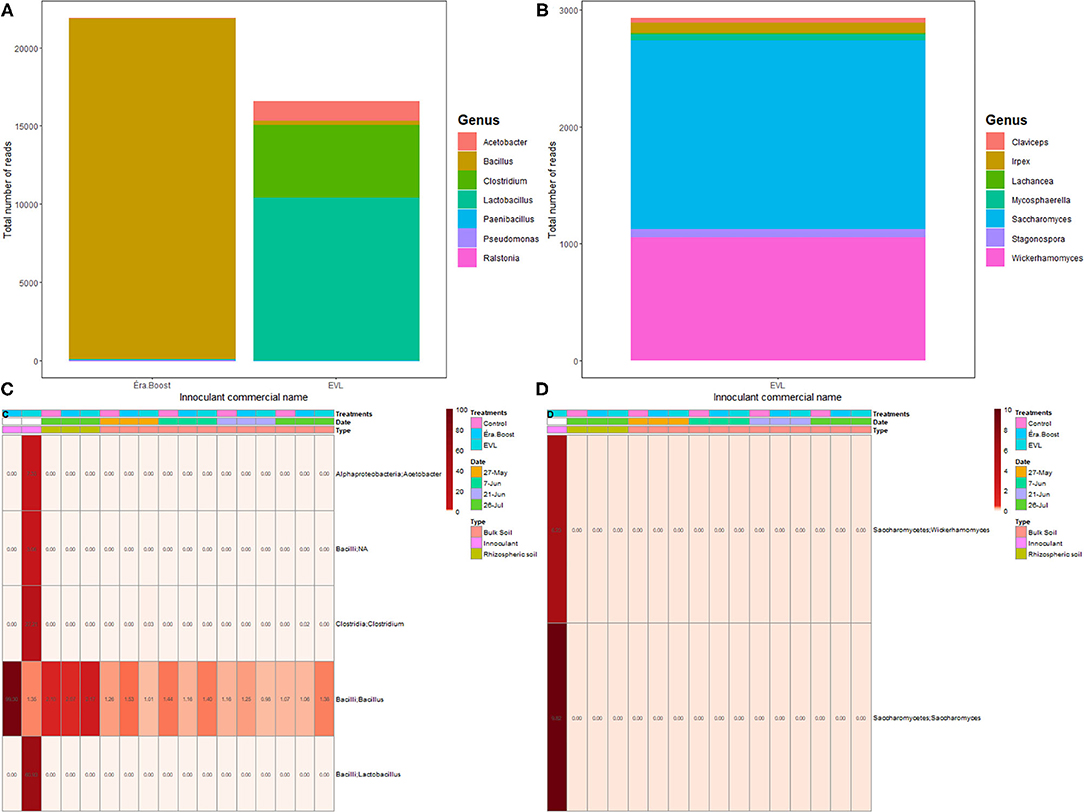
Figure 1. Commercial inoculants EVL and EV. The prokaryotic (A) and fungal (B) compositions at the Genus level. Heatmaps of prokaryotic (C), and fungal (D) genera found in commercial inoculants with relative abundances >1% in all samples collected. Inoculation of soil occurred between May 27th (orange annotation) and June 07th (teal annotation).
Bulk Soil Prokaryotic and Fungal Community Dynamics
The average number of reads for soil samples was 30,068 ± 1,905 for prokaryotes and 22,717 ± 2,341 for fungi. Standardization of the number of reads resulted in 16,685 and 12,302 reads for prokaryote and fungal groups, respectively.
Prokaryotic and fungal genera present in EVL and EB inoculants were not significantly enriched in bulk soil microbial communities following microbial inoculation at seeding or in comparison to un-inoculated plots. Although the Bacillus genus was present in bulk soil samples, the relative proportions of this genus were not significantly different in EB treated plots compared to those in control plots. The predominant prokaryotic genus in the EVL consortium, Lactobacillus, was not detected in any soil samples. Similar results were observed for fungal genera present in the EVL consortium, in that the genera Saccharomyces and Wickerhamomyces were not observed in bulk soil samples (Figures 1C,D).
Microbial inoculation of fertilizer did not have a significant effect on any alpha-diversity indices, observed ASVs or evenness of soils at any time point (Supplementary Table 1). Sampling date had a significant effect on Chao1 (Pprok = 0.003, Pfung < 0.001) index and evenness (Pprok = 0.01, Pfung = 0.02) for prokaryote and fungal populations, respectively (Figure 2). Significant differences were observed in prokaryotic diversity between May 27th and June 21st for Chao1 index and between May 27th and July 26th for Chao1 index and evenness (Figure 2). Fungal Shannon and Chao1 indices and observed ASVs were significantly different between July 26th and all other sampling dates, while there were no significant differences in evenness for May 27th and July 26th.
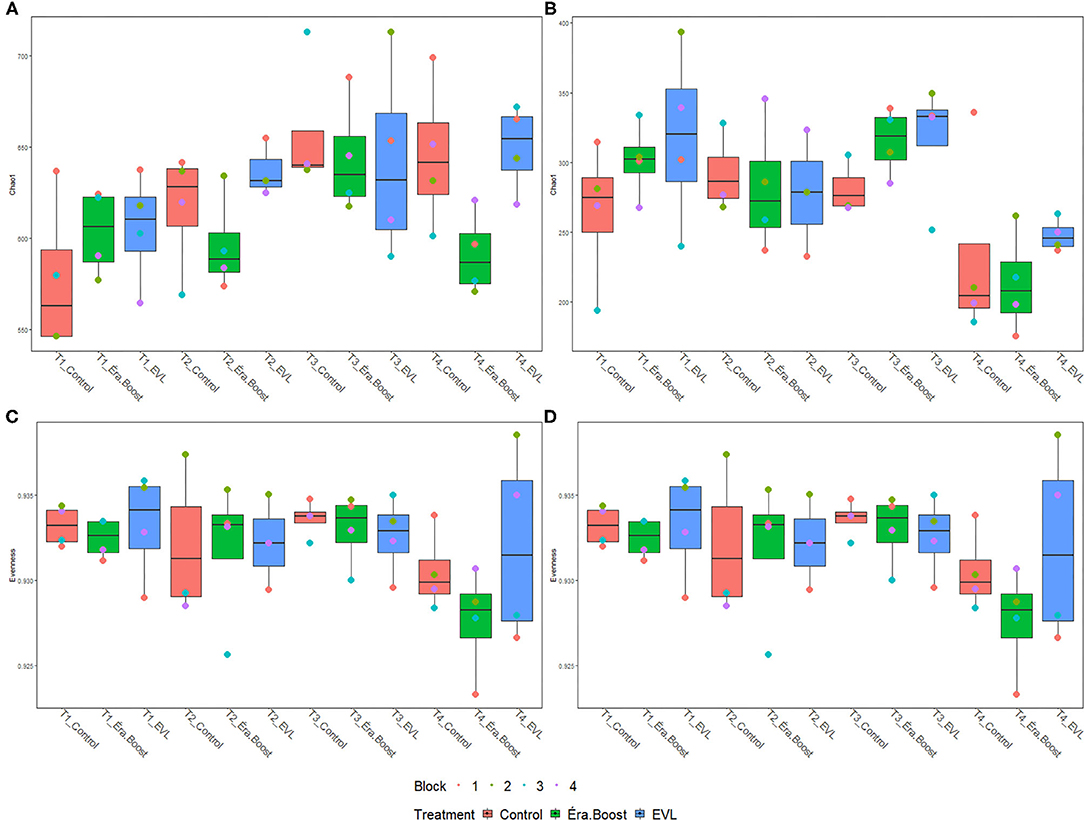
Figure 2. Boxplots of Chao1 and evenness measurements for bulk soil samples. (A,B) Display Chao1 values for prokaryotic and fungal communities, respectively, while (C,D) display evenness values for prokaryotic and fungal communities, respectively; T1: May 27th, T2: June 7th, T3: June 21st, T4: July 26th.
The two bacterial phyla showing highest relative proportions were Proteobacteria and Actinobacteria across all sampling time points, while the fungal classes showing highest relative proportions were Sordariomycetes, Leotiomycetes, and Eurotiomycetes, with the latter having a noticeable enrichment at the final sample date (Figures 3C,D).
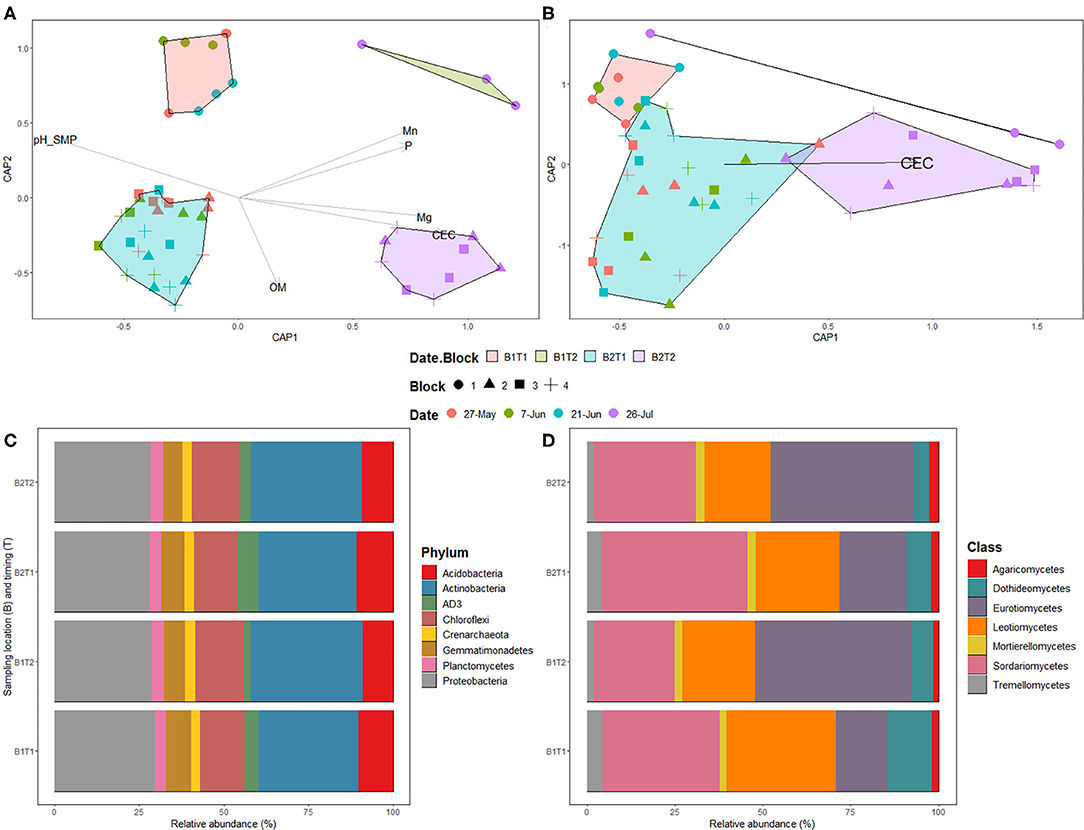
Figure 3. Distance-based redundancy analysis (Db-rda) of prokaryotic (A) and fungal (B) communities in bulk soil samples. Db-rda were based on Bray-Curtis distance at the ASV level; Relative abundance of prokaryotic phyla (C) and fungal classes (D) in bulk soil samples. The Date.Block categories is as follow: B1T1: Block 1 on May 27th, June 7th, and 21st; B1T2: Block 1 on July 26th; B2T1: Blocks 2, 3, and 4 on May 27th, June 7th and 21st; B2T2: Blocks 2, 3, and 4 on July 26th.
A principal coordinate analysis (PCoA) was used to visualize the community assemblages at the ASV level using Bray-Curtis dissimilarities (Supplementary Figure 1). The prokaryote community displayed a strong clustering of samples along the first PCoA axis, which explained 17% of the variation, with strong loading of sampling dates; the fourth sampling time date (July 26th) was segregated from the first three. The second axis, which explained 6.9% of the variation, showed a clear clustering of physical location indicated as block number. Fungal communities displayed a weak clustering effect of sampling time along the first axis, which explained 16.1% of the variation. The second axis does not represent any relevent biological variables and explained 9.4% of the variation (Supplementary Figure 1).
The PERMANOVA results indicated that microbial inoculants did not have a significant effect on bulk soil microbial community while sampling date and physical location (block number) significantly affected prokaryotic and fungal communities. The pairwise differences between sampling date revealed that May 27th prokaryotic composition was significantly different from that of June 21st (P = 0.01), and the July 26th prokaryotic composition was significantly different from the three other sampling dates (P = 0.006). Fungal composition was significantly different on July 26th from the three other sampling times (P = 0.006). The first block had a significantly different prokaryotic composition from the three other blocks (P = 0.006), as observed in the PCoAs (Supplementary Figure 1). Fungal community composition was significantly different between blocks 1 and 2 (P = 0.036).
Both alpha-diversity indices and beta-diversity showed a significant shift in prokaryotic and fungal community compositions between the third and fourth sampling dates. Many ASVs showed differential expression between June 21st and July 26th with the majority belonging to the classes Gammaproteobacteria, Actinobacteria, and Betaproteobacteria (Figures 4A,C). Additionally, many prokaryotic ASVs were unique to each block for bulk and rhizospheric prokaryotic communities (Figures 4B,D).
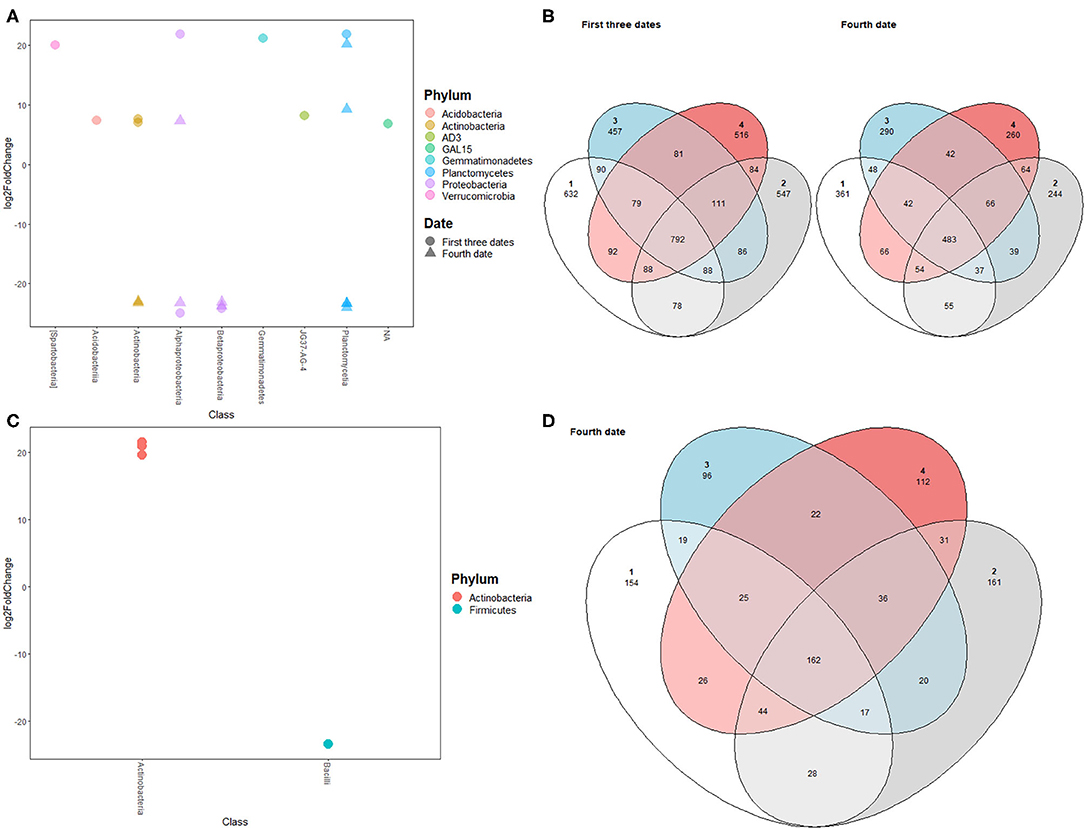
Figure 4. (A) Prokaryotic ASVs differentially detected between block 1 and blocks 2, 3, and 4 for bulk soil prokaryotic communities during the first three sampling dates (circle) and the fourth sampling date (triangle); (B) Venn diagram showing shared prokaryotic ASVs between different blocks for bulk soil at first three sampling dates (left diagram) and fourth sampling date (right diagram); (C) Prokaryotic ASVs differentially detected between block 1 and blocks 2, 3, and 4 for rhizospheric prokaryotic communities; (D) Venn diagram showing shared ASVs between different blocks for rhizospheric prokaryotic communities.
The microbial inoculation, sampling dates or sampling blocks for either prokaryotic or fungal communities did not significantly impact the number of prokaryotes and fungi detected in bulk soil. The average number of prokaryotes and fungi were, respectively, 3.57 × 109 and 3.39 × 107 AU g−1 dry soil (Supplementary Table 1).
The physico-chemical properties of bulk soil sampled prior to seeding were used to determine if there are links with soil microbial communities (Supplementary Table 2). PERMANOVA following a dbRDA of prokaryotic and fungal communities' composition and soil chemical variables showed a significant effect of organic matter (P = 0.007), CEC (P = 0.001), Mg (P = 0.019), P (P = 0.001), and pHSMP (P = 0.002) measurements on prokaryotic community and of CEC (P = 0.001) on fungal community (Figures 3A,B).
Rhizospheric Bacterial and Fungal Diversity and Quantity
Rhizospheric samples had an average of 18,822 ± 1,382 and 14,718 ± 1,720 reads for prokaryotes and fungi, respectively. Similar to the reads obtained for the bulk soil samples, the number of reads normalized to 16,685 and 12,302 reads for prokaryote and fungal communities, respectively.
The highest relative proportion among potato rhizospheric prokaryotic phyla were detected for Proteobacteria, Cyanobacteria, and Actinobacteria. The fungal classes Sordariomycetes, Leotiomycetes, and Eurotiomycetes showed the highest relative proportions in rhizospheric soil (Figures 5C,D). The bacterial phylum Cyanobacteria is mostly composed of the Chloroplast genus which most likely come from lysed root cells containing plastids.
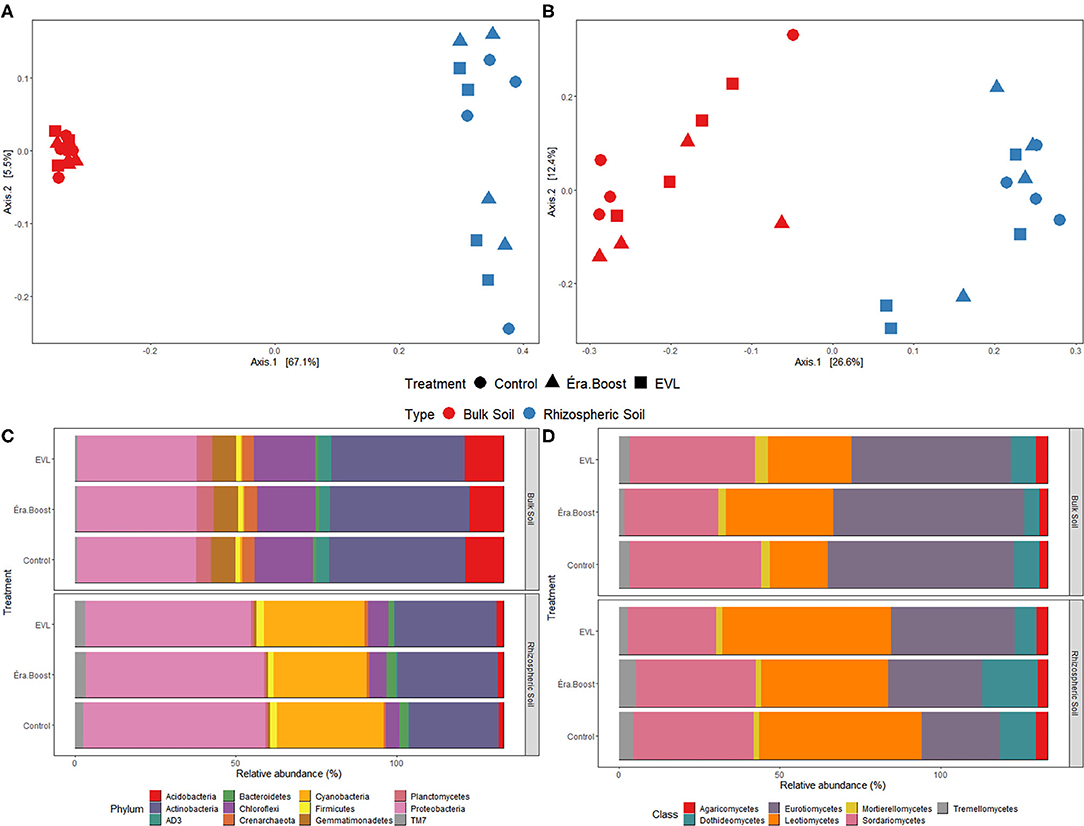
Figure 5. Principal coordinates analysis (PCoA) of prokaryotic (A) and fungal (B) communities in bulk and rhizospheric soil sampled on July 26th. PCoAs were based on Bray-Curtis distance at the ASV level; Relative abundance of prokaryotic phyla (C) and fungal classes (D) in bulk and rhizospheric soil samples.
The microbial inoculation treatment showed no significant difference for Shannon and Chao1 indices, evenness and number of observed ASVs for either prokaryotic or fungal communities (Supplementary Table 1). The microbial inoculation treatment did not significantly impact the number of prokaryotes and fungi detected in rhizospheric samples. The average number of prokaryotes and fungi were, respectively, 1.27 × 1010 and 9.17 × 107 AU g−1 dry soil (Supplementary Table 1).
As observed with the bulk soil samples, the microbial inoculant genera were not enriched in rhizopheric microbial community. Lactobacillus, Saccharomyces, and Wickerhamomyces genera, found in the EVL inoculant, were not observed in rhizospheric samples. However, the Bacillus genus, which is the dominant bacterial genus in EB inoculant, showed a significant mean increase of 68% in rhizospheric samples compared to those detected in bulk soil (t(11) = 8.3, P < 0.0001) for inoculated and non-inoculated plots.
The prokaryotic and fungal communities' diversity for bulk soil samples collected on the same date as rhizospheric soil samples (July 26th) were analyzed as paired T-tests. Prokaryotic Shannon (P < 0.001) and Chao1 (P < 0.001) indices, evenness (P < 0.001) and number of observed prokaryotic ASVs (P < 0.001) were significantly smaller in rhizospheric soil compared to bulk soil. Fungal Chao1 index (P = 0.003) and number of observed fungal ASVs (P = 0.003) were significantly smaller for rhizospheric soil than bulk soil. Mean numbers of prokaryotes and fungi estimated as AU g−1 dry soil were significantly greater in rhizospheric soil.
PERMANOVA analysis of rhizospheric and bulk soil samples collected on July 26th showed a significant effect (P < 0.001) of sample type (roots or soil) for prokaryotic and fungal communities. Microbial inoculation and block location did not have significant effects on the prokaryotic community (Ptreatment = 0.43 and Pblock = 0.15) and on fungal community (Ptreatment = 0.76 and Pblock = 0.36). Analysis of rhizospheric soil samples alone showed a significant effect of block location (P = 0.019) on prokaryotic community, but no significant effect (P = 0.415) on fungal community.
Discussion
Microbial Inoculation Impacts on the Soil Microbial Community
We hypothesized that microbial inoculation of granular fertilizer would directly influence soil microbial community by increasing relative abundances of inoculated microbial genera. We had assumed that since HTS technique and the resultant ASV can discriminate microbial genera with a higher level of precision thantechniques such as culture-dependent techniques or phospholipid-derived fatty acids, the use of HTS would detect changes in the relative abundance of the inoculated microbial genera. However, our HTS results showed that neither microbial communities of bulk soil nor rhizospheric samples were significantly affected by microbial treatments. Previous experiments using an HTS approach have successfully detected an impact from inoculants (Deng et al., 2019; Dong et al., 2019). An experiment on Fragaria × ananassa (strawberry) showed that VESTA®, a soil amendment composed of a broad spectrum of microbes, fermentation by-products and organic acids, applied at a rate of 75 L ha−1 every month for 5 months, had an effect on bulk soil prokaryotic community (Deng et al., 2019). Inoculation of P. ginseng with a one time-application of 90–270 L ha−1 of microbial inoculant consisting of Burkholderia and Rhizobium diluted in pig and cow manure as a biofertilizer significantly impacted the bulk soil prokaryotic community (Dong et al., 2019). An inoculation of 109 spores L−1 of B. subtilis in 12 different Chinese soils cultivated in a greenhouse with Cucumis sativus (cucumber) did have an effect on the rhizospheric prokaryotic community sampled 80 days after inoculation (Tian and Gao, 2014). The fertilizers coated with the microbial inoculants used in this experiment, were applied as narrow strips across the field at seeding, making their effect extremely localized within the soil. Additionally, the banded application rates of both microbial inoculants were 1–2 L ha−1 (about 4–8 × 1011 cells ha−1) which is drastically lower than the application rates used in previous experiments. That may explain the lack of direct effects on bulk and rhizospheric soil microbial communities.
Relative abundances of the microbial inoculants of each commercial formulation in bulk soil and rhizospheric samples do not follow the same pattern. EB inoculant consisted almost exclusively of Bacillus genus. The ASVs present in EB were significantly more abundant in rhizospheric soil than bulk soil, even tough EB inoculation did not increase their abundance. This result indicates that microorganisms present in EB are recruited by plants, a sign of their potential for positive effects on plant growth (Islam et al., 2016; Backer et al., 2018). On the other hand, ASVs identified in EVL were never detected in bulk and rhizospheric samples. Their absence in our samples may indicate that they cannot compete with native soil microbial community (Khare and Arora, 2015).
In this experiment, bulk soil cores were collected from zero to 30 cm depth while the fertilizer was banded 5 cm on each side of the seed tuber and both strips were 5 cm below the potato seed tuber. A dilution effect on the microbial community caused by the bulk soil sampling depth compared to the depth of the inoculant may have impaired our capacity to detect the microbial inoculant genera. Most research has looked at the rhizospheric community instead of the bulk soil community. In this experiment, rhizospheric community samples were only taken the same day as the fourth bulk soil sampling. The root system was not well-developed at the time of the first and second samplings, and at the third sampling, rhizospheric soil was not sampled. In hindsight, analysis of rhizospheric microbial community at this stage of root development would be an ideal moment to investigate microbial inoculant effect on microbial community.
Sampling Time and Location Within the Field Effects on Bulk Soil Microbial Community
Aspects of sample collection, rather than microbial inoculation, had significant effects on microbial community composition, namely sampling date and sample location (block number). Changes in bacterial and fungal communities over the course of a growing season have been observed repeatedly on agricultural lands (Houlden et al., 2008; Lauber et al., 2013; Degrune et al., 2017). Even with minimal human inputs, microbial community composition changes are correlated with changes in soil temperature and/or humidity (Lauber et al., 2013). In addition to changes in environmental conditions, application of pesticides and in-season tillage activities influence the presence of microbial species in certain layers (strata) of the soil, as well as their relative abundance (Lo, 2010; Degrune et al., 2017; Sun et al., 2018).
In this experiment, a significant shift could be observed for both prokaryote and fungal communities between potato shoot emergence and mid-flowering, much more than between tuber seeding and shoot emergence. Examining crop management activities, between shoot emergence and mid-flowering, fungicides were applied at a weekly interval and soil was tilled once around potato rows, to promote tuber production and apply additional fertilizer. In comparison, between seeding and shoot emergence, when the first three sampling time points occurred, only seeding of seed tubers and application of banded fertilizer were carried out; there was no pesticide application or tillage. Therefore, the changes observed in microbial community composition seem to be more important during the mid-season than the early season in field potato production, around the same time when pesticides applications and tillage are frequently conducted.
Since this experiment followed an RCBD design, we had physical treatment replicates pooled into seven blocks across a field of <1 ha. Our hypothesis that microbial inoculation would have a stronger effect than the block effect on microbial community composition was rejected. Prokaryotic community composition and soil organic matter, P, Ca, Mn, Fe, and B contents were significantly different between the first block and other blocks. A db-RDA revealed that P content significantly explained prokaryotic community composition. The C:N:P ratio was found to be a strong predictor of bacterial diversity in previous research (Delgado-Baquerizo et al., 2017), further indicating that P content in bulk soil can be an important driver of soil bacterial community composition.
Interestingly, potato tuber yield was also significantly different between the first block and the three other blocks. Therefore, the changes in microbial community observed are associated with changes in potato yield. Previous work has shown that potato yield was correlated with microbial community indices (Jeanne et al., 2019) and that these indices could be used to estimate potato productivity. Here, we report ASVs that have relative abundances significantly different between two different yield productivity zones. ASVs with higher abundances in high yield regions (Figure 4C) could be used as potential microbial inoculants that could boost potato tuber yields.
Effect of Field Location on Rhizospheric Microbial Communities
Rhizospheric microbial community composition differed substantially from bulk soil microbial community composition (Figure 5). Enrichment of the rhizospheric community over bulk soil for Proteobacteria and in Eurotiomycetes was evident. Such differences in microbial community associated with potato roots have been reported in numerous other studies (Smalla et al., 2001; Berendsen et al., 2012; Xue et al., 2018).
According to Pfeiffer et al. (2016) rhizospheric microbial community consist of a core community along with a dynamically changing portion which varies among plant locations and growth stages. The dynamically changing portion of the rhizospheric community is not necessarily linked with changes in bulk soil community for potato (Inceoglu et al., 2011), soybean (Sugiyama et al., 2014), wheat (Donn et al., 2015), and wild oats (Shi et al., 2015). Instead, the rhizospheric community is shaped by plant development and location, in concordance with the results observed here and in other publications (Nahar et al., 2020). In this experiment, Actinobacteria were significantly enriched in rhizospheric soils of plots with a high tuber yield. This class of prokaryotes could be utilized as plant-growth promoting microbial inoculants, especially organisms belonging to genera Streptomyces and Mycobacterium (Sathya et al., 2017). Understanding the functions related to plant growth contributed by the core and the changing portions of rhizospheric microbiome could help in designing microbial inoculants that would provide beneficial effects under a wide variety of soil conditions.
Conclusions And Future Directions
This experiment did not confirm its original hypothesis that coating granular fertilizer used during potato seeding with commercial monogenius or mixed genus microbial inoculants would significantly impact soil microbial community. Instead, soil microbial community was shaped by sample location and soil sampling time with an association between soil microbial community and potato tuber yield. Plots with high potato yield had significantly more Actinobacteria in the rhizospheric soil at mid-flowering stage. Further investigations taking a similar approach with slight modifications would deepen our understanding of soil microbial inoculants. These modifications include a more localized soil sampling, proximal to the site of inoculant application, during the early development of the potato root system. Laboratory experiments showed that microbial inoculation of fertilizer results in addition of viable cells to the soil (Overbeek, 2020), which will grow under favorable conditions. In the soil, microbial inoculant growth must happen in the few centimeters surrounding the applied fertilizer. Hence sampling at 2.5 cm above and below the fertilizer depth (7.5–12.5 cm), would ensure that soil analyzed would be the most likely to be affected by the coated microbial inoculant. Our work could serve as a benchmark for the development of new microbial inoculants specifically aimed at improving potato tuber yield.
Data Availability Statement
The data presented in the study are deposited in the SRA repository, accession number PRJNA743329.
Author Contributions
WO, TJ, RH, and DS designed the experiment. WO sampled soil and roots samples from the field, performed the laboratory measurements, and wrote the paper. WO and TJ analyzed the data and created graphs. DS provided the overall conceptual framework and made editorial input. All authors discussed the results, read, and approved the final version of the manuscript.
Funding
The authors gratefully acknowledge the financial support provided by NSERC Collaborative Research and Development Grants, Agriculture and Agri-Food Canada Biomass Canada research cluster and the Fonds de recherche du Québec—Nature et technologies (FRQNT) Master's research scholarship.
Conflict of Interest
The authors declare that the research was conducted in the absence of any commercial or financial relationships that could be construed as a potential conflict of interest.
Publisher's Note
All claims expressed in this article are solely those of the authors and do not necessarily represent those of their affiliated organizations, or those of the publisher, the editors and the reviewers. Any product that may be evaluated in this article, or claim that may be made by its manufacturer, is not guaranteed or endorsed by the publisher.
Acknowledgments
The authors thank the research and development team of Synagri for managing potato plots for the entire growing season. Our thanks also go to the farm Frank Racz Inc. which authorized the conduct of this experiment on its land.
Supplementary Material
The Supplementary Material for this article can be found online at: https://www.frontiersin.org/articles/10.3389/fagro.2021.714700/full#supplementary-material
Supplementary Figure 1. Principal coordinates analysis (PCoA) of prokaryotic (A) and fungal (B) communities in bulk soil samples. PCoAs were based on Bray-Curtis distance at the ASV level.
Supplemental Table 1. Alpha-diversity metrics and qPCR measurements for all samples grouped by sample type (n = 3). AU, Amplification units g−1 dry soil.
Supplemental Table 2. Chemical properties of soil for all soil sampling dates.
Supplemental Table 3. Statistical significance of pairwise differences of block number and sampling date effects on soil microbial community composition.
Supplemental Table 4. Variance explained by each principal coordinates from Figure 5's PCoA.
Abbreviations
AU, Amplification units; dbRDA, distance-based redundancy analysis; EB, Éra boost®; EVL, EVL coating®; GSF, Granular starter fertilizer; PCoA, principal coordinate analysis; PGPR, Plant growth-promoting rhizobacteria.
References
Alexandratos, N., and Bruinsma, J. (2012). “World agriculture towards 2030/2050: the 2012 revision,” in ESA Working Papers 12-03 (Agricultural Development Economics Division (ESA)).
Antar, M., Gopal, P., Msimbira, L. A., Naamala, J., Nazari, M., Overbeek, W., et al. (2021). “Inter-organismal signaling in the rhizosphere,” in Rhizosphere Biology: Interactions Between Microbes and Plants, eds V. V. S. R. Gupta and A. K. Sharma (Singapore: Springer Singapore), 255–293.
Apprill, A., Mcnally, S., Parsons, R., and Weber, L. (2015). Minor revision to V4 region SSU rRNA 806R gene primer greatly increases detection of SAR11 bacterioplankton. Aquat. Microb. Ecol. 75, 129–137. doi: 10.3354/ame01753
Backer, R., Rokem, J. S., Ilangumaran, G., Lamont, J., Praslickova, D., Ricci, E., et al. (2018). Plant growth-promoting rhizobacteria: context, mechanisms of action, and roadmap to commercialization of biostimulants for sustainable agriculture. Front. Plant Sci. 9:1473. doi: 10.3389/fpls.2018.01473
Berendsen, R. L., Pieterse, C. M. J., and Bakker, P. A. H. M. (2012). The rhizosphere microbiome and plant health. Trends Plant Sci. 17, 478–486. doi: 10.1016/j.tplants.2012.04.001
Bokulich, N. A., and Mills, D. A. (2013). Improved selection of internal transcribed spacer-specific primers enables quantitative, ultra-high-throughput profiling of fungal communities. Appl. Environ. Microbiol. 79, 2519–2526. doi: 10.1128/AEM.03870-12
Borriss, R. (2011). “Use of Plant-Associated Bacillus Strains as Biofertilizers and Biocontrol Agents in Agriculture,” in Bacteria in Agrobiology: Plant Growth Responses, ed D. K. Maheshwari. (Berlin; Heidelberg: Springer), 41–76.
Callahan, B. J., Mcmurdie, P. J., and Holmes, S. P. (2017). Exact sequence variants should replace operational taxonomic units in marker-gene data analysis. ISME J. 11, 2639–2643. doi: 10.1038/ismej.2017.119
Callahan, B. J., Mcmurdie, P. J., Rosen, M. J., Han, A. W., Johnson, A. J. A., and Holmes, S. P. (2016). DADA2: High-resolution sample inference from Illumina amplicon data. Nat. Methods 13, 581–583. doi: 10.1038/nmeth.3869
Caporaso, J. G., Kuczynski, J., Stombaugh, J., Bittinger, K., Bushman, F. D., Costello, E. K., et al. (2010). QIIME allows analysis of high-throughput community sequencing data. Nat. Methods 7, 335–336. doi: 10.1038/nmeth.f.303
Castro-Sowinski, S., Herschkovitz, Y., Okon, Y., and Jurkevitch, E. (2007). Effects of inoculation with plant growth-promoting rhizobacteria on resident rhizosphere microorganisms. FEMS Microbiol. Lett. 276, 1–11. doi: 10.1111/j.1574-6968.2007.00878.x
Dagher, D. J., De La Providencia, I. E., Pitre, F. E., St-Arnaud, M., and Hijri, M. (2019). Plant identity shaped rhizospheric microbial communities more strongly than bacterial bioaugmentation in petroleum hydrocarbon-polluted sediments. Front. Microbiol. 10:2144. doi: 10.3389/fmicb.2019.02144
Degrune, F., Theodorakopoulos, N., Colinet, G., Hiel, M.-P., Bodson, B., Taminiau, B., et al. (2017). Temporal dynamics of soil microbial communities below the seedbed under two contrasting tillage regimes. Front. Microbiol. 8:1127. doi: 10.3389/fmicb.2017.01127
Delgado-Baquerizo, M., Reich, P. B., Khachane, A. N., Campbell, C. D., Thomas, N., Freitag, T. E., et al. (2017). It is elemental: soil nutrient stoichiometry drives bacterial diversity. Environ. Microbiol. 19, 1176–1188. doi: 10.1111/1462-2920.13642
Deng, S., Wipf, H. M. L., Pierroz, G., Raab, T. K., Khanna, R., and Coleman-Derr, D. (2019). A plant growth-promoting microbial soil amendment dynamically alters the strawberry root bacterial microbiome. Sci. Rep. 9:17677. doi: 10.1038/s41598-019-53623-2
Desantis, T. Z., Hugenholtz, P., Larsen, N., Rojas, M., Brodie, E. L., Keller, K., et al. (2006). Greengenes, a chimera-checked 16S rRNA gene database and workbench compatible with ARB. Appl. Environ. Microbiol. 72, 5069–5072. doi: 10.1128/AEM.03006-05
Dobbelaere, S., Vanderleyden, J., and Okon, Y. (2003). Plant growth-promoting effects of diazotrophs in the rhizosphere. CRC. Crit. Rev. Plant Sci. 22, 107–149. doi: 10.1080/713610853
Dong, L., Li, Y., Xu, J., Yang, J., Wei, G., Shen, L., et al. (2019). Biofertilizers regulate the soil microbial community and enhance Panax ginseng yields. Chin. Med. 14:20. doi: 10.1186/s13020-019-0241-1
Donn, S., Kirkegaard, J. A., Perera, G., Richardson, A. E., and Watt, M. (2015). Evolution of bacterial communities in the wheat crop rhizosphere. Environ. Microbiol. 17, 610–621. doi: 10.1111/1462-2920.12452
Emerson, J., Keady, P., Brewer, T., Clements, N., Morgan, E., Awerbuch, J., et al. (2015). Impacts of flood damage on airborne bacteria and fungi in homes after the 2013 Colorado front range flood. Environ. Sci. Tech. 49, 2675–2684. doi: 10.1021/es503845j
Fierer, N., Jackson, J. A., Vilgalys, R., and Jackson, R. B. (2005). Assessment of soil microbial community structure by use of taxon-specific quantitative PCR assays. Appl. Environ. Microbiol. 71, 4117–4120. doi: 10.1128/AEM.71.7.4117-4120.2005
Fukami, J., Cerezini, P., and Hungria, M. (2018). Azospirillum: benefits that go far beyond biological nitrogen fixation. AMB Express 8:73. doi: 10.1186/s13568-018-0608-1
Gange, A. C., and Gadhave, K. R. (2018). Plant growth-promoting rhizobacteria promote plant size inequality. Sci. Rep. 8:13828. doi: 10.1038/s41598-018-32111-z
Garcia-Fraile, P., Menendez, E., and Rivas, R. (2015). Role of bacterial biofertilizers in agriculture and forestry. AIMS J. 2, 183–205. doi: 10.3934/bioeng.2015.3.183
Hendershot, W. H., Lalande, H., and Duquette, M. (1993). “Ion exchange and exchangeable cations,” in Soil Sampling and Methods of Analysis, ed M. R. Carter. (Boca Raton, Fl: Lewis Publishers), 167–176.
Herrmann, L., and Lesueur, D. (2013). Challenges of formulation and quality of biofertilizers for successful inoculation. Appl. Microbiol. Biotechnol. 97, 8859–8873. doi: 10.1007/s00253-013-5228-8
Houlden, A., Timms-Wilson, T. M., Day, M. J., and Bailey, M. J. (2008). Influence of plant developmental stage on microbial community structure and activity in the rhizosphere of three field crops. FEMS Microbiol. Ecol. 65, 193–201. doi: 10.1111/j.1574-6941.2008.00535.x
Inceoglu, Ö., Al-Soud, W. A., Salles, J. F., Semenov, A. V., and Van Elsas, J.D. (2011). Comparative analysis of bacterial communities in a potato field as determined by pyrosequencing. PLoS ONE 6:e23321. doi: 10.1371/journal.pone.0023321
Islam, S., Akanda, A. M., Prova, A., Islam, M. T., and Hossain, M. M. (2016). Isolation and identification of plant growth promoting rhizobacteria from cucumber rhizosphere and their effect on plant growth promotion and disease suppression. Front. Microbio. 6:1360. doi: 10.3389/fmicb.2015.01360
Jeanne, T., Parent, S.-É., and Hogue, R. (2019). Using a soil bacterial species balance index to estimate potato crop productivity. PLoS ONE 14:e0214089. doi: 10.1371/journal.pone.0214089
Khare, E., and Arora, N. K. (2015). “Effects of soil environment on field efficacy of microbial inoculants,” in Plant Microbes Symbiosis: Applied Facets, ed. N. K. Arora (New Delhi: Springer), 353–381.
Kloepper, J. W., Lifshitz, R., and Zablotowicz, R. M. (1989). Free-living bacterial inocula for enhancing crop productivity. Trends Biotechnol. 7, 39–44. doi: 10.1016/0167-7799(89)90057-7
Kõljalg, U., Nilsson, R. H., Abarenkov, K., Tedersoo, L., Taylor, A. F. S., Bahram, M., et al. (2013). Towards a unified paradigm for sequence-based identification of fungi. Mol. Ecol. 22, 5271–5277. doi: 10.1111/mec.12481
Lauber, C. L., Ramirez, K. S., Aanderud, Z., Lennon, J., and Fierer, N. (2013). Temporal variability in soil microbial communities across land-use types. ISME J. 7, 1641–1650. doi: 10.1038/ismej.2013.50
Leggett, M., Newlands, N. K., Greenshields, D., West, L., Inman, S., and Koivunen, M. E. (2015). Maize yield response to a phosphorus-solubilizing microbial inoculant in field trials. J. Agric. Sci. 153, 1464–1478. doi: 10.1017/S0021859614001166
Lo, C. C. (2010). Effect of pesticides on soil microbial community. J. Environ. Sci. Health B 45, 348–359. doi: 10.1080/03601231003799804
Love, M. I., Huber, W., and Anders, S. (2014). Moderated estimation of fold change and dispersion for RNA-seq data with DESeq2. Genome Biol. 15:550. doi: 10.1186/s13059-014-0550-8
Mcmurdie, P. J., and Holmes, S. (2014). Waste not, want not: why rarefying microbiome data is inadmissible. PLoS Comput. Biol. 10:e1003531. doi: 10.1371/journal.pcbi.1003531
Mehlich, A. (1984). Mehlich 3 soil test extractant: a modification of Mehlich 2 extractant. Commun. Soil Sci. Plant Anal. 15, 1409–1416. doi: 10.1080/00103628409367568
Mendes, R., Kruijt, M., De Bruijn, I., Dekkers, E., Van Der Voort, M., Schneider, J. H. M., et al. (2011). Deciphering the rhizosphere microbiome for disease-suppressive bacteria. Science 332, 1097–1100. doi: 10.1126/science.1203980
Nahar, K., Floc'h, J.-B., Goyer, C., Zebarth, B. J., and Whitney, S. (2020). Diversity of soil bacterial community is influenced by spatial location and time but not potato cultivar. Phytobio. J. 4, 225–238. doi: 10.1094/PBIOMES-01-20-0002-R
Nazari, M., and Smith, D. L. (2020). A PGPR-produced bacteriocin for sustainable agriculture: a review of thuricin 17 characteristics and applications. Front. Plant Sci. 11:916. doi: 10.3389/fpls.2020.00916
Overbeek, W. (2020). Viable Microbial Inoculation of Granular Fertilizer to Improve Row Crop Productivity in Southern Quebec. Montreal, QC: McGill University.
Parada, A. E., Needham, D. M., and Fuhrman, J. A. (2016). Every base matters: assessing small subunit rRNA primers for marine microbiomes with mock communities, time series and global field samples. Environ. Microbiol. 18, 1403–1414. doi: 10.1111/1462-2920.13023
Pérez-Montaño, F., Alías-Villegas, C., Bellogín, R. A., Del Cerro, P., Espuny, M. R., Jiménez-Guerrero, I., et al. (2014). Plant growth promotion in cereal and leguminous agricultural important plants: from microorganism capacities to crop production. Microbiol. Res. 169, 325–336. doi: 10.1016/j.micres.2013.09.011
Pfeiffer, S., Mitter, B., Oswald, A., Schloter-Hai, B., Schloter, M., Declerck, S., et al. (2016). Rhizosphere microbiomes of potato cultivated in the High Andes show stable and dynamic core microbiomes with different responses to plant development. FEMS Microbiol. Ecol. 93:fiw242. doi: 10.1093/femsec/fiw242
R Core Team. (2020). R: A Language and Environment for Statistical Computing. Vienna: R Foundation for Statistical Computing.
Rilling, J. I., Acuña, J. J., Nannipieri, P., Cassan, F., Maruyama, F., and Jorquera, M. A. (2019). Current opinion and perspectives on the methods for tracking and monitoring plant growth–promoting bacteria. Soil Bio. Biochem. 130, 205–219. doi: 10.1016/j.soilbio.2018.12.012
Sathya, A., Vijayabharathi, R., and Gopalakrishnan, S. (2017). Plant growth-promoting actinobacteria: a new strategy for enhancing sustainable production and protection of grain legumes. 3 Biotech 7, 102–102. doi: 10.1007/s13205-017-0736-3
Shi, S., Nuccio, E., Herman, D. J., Rijkers, R., Estera, K., Li, J., et al. (2015). Successional trajectories of rhizosphere bacterial communities over consecutive seasons. MBio 6:e00746. doi: 10.1128/mBio.00746-15
Shoemaker, H. E., Mclean, E. O., and Pratt, P. F. (1961). Buffer methods for determining lime requirement of soils with appreciable amounts of extractable aluminum. Soil Sci. Soc. Am. J. 25, 274–277. doi: 10.2136/sssaj1961.03615995002500040014x
Sivasakthi, S., Usharani, G., and Saranraj, P. (2014). Biocontrol potentiality of plant growth promoting bacteria (pgpr)- pseudomonas fluorescens and bacillus subtilis: a review. Air. J. Agric. Res 9, 1265–1277. doi: 10.5897/AJAR2013.7914
Smalla, K., Wieland, G., Buchner, A., Zock, A., Parzy, J., Kaiser, S., et al. (2001). Bulk and rhizosphere soil bacterial communities studied by denaturing gradient gel electrophoresis: plant-dependent enrichment and seasonal shifts revealed. Appl. Environ. Microbiol. 67:4742. doi: 10.1128/AEM.67.10.4742-4751.2001
Smith, D., Subramanian, S., Lamont, J., and Bywater-Ekegärd, M. (2015). Signaling in the phytomicrobiome: breadth and potential. Front. Plant Sci. 6:709. doi: 10.3389/fpls.2015.00709
Smith, D. L., Gravel, V., and Yergeau, E. (2017). Editorial: signaling in the phytomicrobiome. Front. Plant Sci. 8:611. doi: 10.3389/fpls.2017.00611
Sugiyama, A., Ueda, Y., Zushi, T., Takase, H., and Yazaki, K. (2014). Changes in the bacterial community of soybean rhizospheres during growth in the field. PLoS ONE 9:e100709. doi: 10.1371/journal.pone.0100709
Sun, R., Li, W., Dong, W., Tian, Y., Hu, C., and Liu, B. (2018). Tillage changes vertical distribution of soil bacterial and fungal communities. Front. Microbiol. 9:699. doi: 10.3389/fmicb.2018.00699
Tian, Y., and Gao, L. (2014). Bacterial diversity in the rhizosphere of cucumbers grown in soils covering a wide range of cucumber cropping histories and environmental conditions. Microb. Ecol. 68, 794–806. doi: 10.1007/s00248-014-0461-y
Walkley, A., and Black, I. A. (1934). An examination of the degtjareff method for determining soil organic matter, and a proposed modification of the chromic acid titration method. Soil Sci. 37:29. doi: 10.1097/00010694-193401000-00003
Keywords: soil microbial communities, plant growth-promoting microbe, illumina sequencing, potato production, microbial inoculants, plant productivity
Citation: Overbeek W, Jeanne T, Hogue R and Smith DL (2021) Effects of Microbial Consortia, Applied as Fertilizer Coating, on Soil and Rhizosphere Microbial Communities and Potato Yield. Front. Agron. 3:714700. doi: 10.3389/fagro.2021.714700
Received: 25 May 2021; Accepted: 05 July 2021;
Published: 04 August 2021.
Edited by:
Shakeel Ahmad, Bahauddin Zakariya University, PakistanReviewed by:
Mohd Sayeed Akhtar, M. J. P. Rohilkhand University, IndiaMahaveer P. Sharma, ICAR Indian Institute of Soybean Research, India
Copyright © 2021 Overbeek, Jeanne, Hogue and Smith. This is an open-access article distributed under the terms of the Creative Commons Attribution License (CC BY). The use, distribution or reproduction in other forums is permitted, provided the original author(s) and the copyright owner(s) are credited and that the original publication in this journal is cited, in accordance with accepted academic practice. No use, distribution or reproduction is permitted which does not comply with these terms.
*Correspondence: William Overbeek, d2lsbGlhbS5vdmVyYmVlayYjeDAwMDQwO21haWwubWNnaWxsLmNh