- 1Agroécologie, AgroSup Dijon, INRAE, Univ. Bourgogne, Univ. Bourgogne Franche-Comté, Dijon, France
- 2INRAE, UE115 Domaine Expérimental d'Epoisses, Dijon, France
The growing recognition of the environmental and health issues associated to pesticide use requires to investigate how to manage weeds with less or no herbicides in arable farming while maintaining crop productivity. The questions of weed harmfulness, herbicide efficacy, the effects of herbicide use on crop yields, and the effect of reducing herbicides on crop production have been addressed over the years but results and interpretations often appear contradictory. In this paper, we critically analyze studies that have focused on the herbicide use, weeds and crop yield nexus. We identified many inconsistencies in the published results and demonstrate that these often stem from differences in the methodologies used and in the choice of the conceptual model that links the three items. Our main findings are: (1) although our review confirms that herbicide reduction increases weed infestation if not compensated by other cultural techniques, there are many shortcomings in the different methods used to assess the impact of weeds on crop production; (2) Reducing herbicide use rarely results in increased crop yield loss due to weeds if farmers compensate low herbicide use by other efficient cultural practices; (3) There is a need for comprehensive studies describing the effect of cropping systems on crop production that explicitly include weeds and disentangle the impact of herbicides from the effect of other practices on weeds and on crop production. We propose a framework that presents all the links and feed-backs that must be considered when analyzing the herbicide-weed-crop yield nexus. We then provide a number of methodological recommendations for future studies. We conclude that, since weeds are causing yield loss, reduced herbicide use and maintained crop productivity necessarily requires a redesign of cropping systems. These new systems should include both agronomic and biodiversity-based levers acting in concert to deliver sustainable weed management.
Introduction
Since the onset of agriculture, a main objective of crop management has been the control of arable weeds, both by making the weed seed bank germinate at a time when the resulting plants would not hinder the crop and by eliminating weed plants at those times they would compete with the crop. At the beginning of the twentieth century, weed science books described losses per unit area of 20 to 50% without weed control, depending on the crop (Long, 1910; Fron, 1917). The increased availability of synthetic, highly effective herbicides in the middle of the twentieth century led to a decrease in weed species diversity and density (e.g., Andreasen et al., 1996; Andersson and Milberg, 1998; Robinson and Sutherland, 2002; Fried et al., 2009), and farmers largely lost interest in other weed management techniques. During that period, research studies focused on characterizing the harmfulness of particularly aggressive species, with most experimental studies conducted under controlled conditions and focusing on two-species situations (i.e., one crop or variety vs. one weed species) in order to determine harmfulness thresholds for triggering spraying operations (Caussanel et al., 1988; Clewis et al., 2001). Environmental and health issues (Stoate et al., 2009; Waggoner et al., 2013) have led to a recent legislation push for a reduction in pesticide use (Neumeister et al., 2007). Together with the expansion of herbicide resistance (Busi et al., 2013), this has triggered a shift from weed control exclusively based on systematic chemical herbicide applications to integrated weed management, where combinations of alternative preventive and curative techniques (which are only partially efficient) are used (Liebman and Gallandt, 1997). This shift raised the question of whether agricultural production will be impaired by weeds and how to move toward a weed management that relies little or not at all on herbicides.
Numerous studies thus investigated the effect of reducing pesticides on crop production (de Ponti et al., 2012; Seufert et al., 2012; Hossard et al., 2014; Lechenet et al., 2014, 2017a; Petit et al., 2015), the efficacy of herbicides to control weeds and to preserve crop production (Milberg and Hallgren, 2004; Fickett et al., 2013; Soltani et al., 2016), or the harmfulness of weeds for crop production (Milberg and Hallgren, 2004; Song et al., 2017). Their results and/or interpretations sometimes appear contradictory.
Consequently, this review paper critically analyses methods and results used in published studies that investigated weed harmfulness for crop production, herbicide impacts on weed flora and crop production as well as cropping-system impact on herbicide use. Our investigative framework discriminates the different conceptual models that have been used in the literature to explore the herbicide use-weed-crop yield nexus (Figure 1). Rather than writing a comprehensive review on the findings of the studies focusing on these relationships, we investigated the advantages and limits of each methodology as well as the implications of the methodological choices for interpreting results. The ultimate goal was to provide a methodological guide to answer two major questions, (1) when and how much do weeds affect crop production and (2) is it possible reconcile reduced herbicide use and yield preservation.
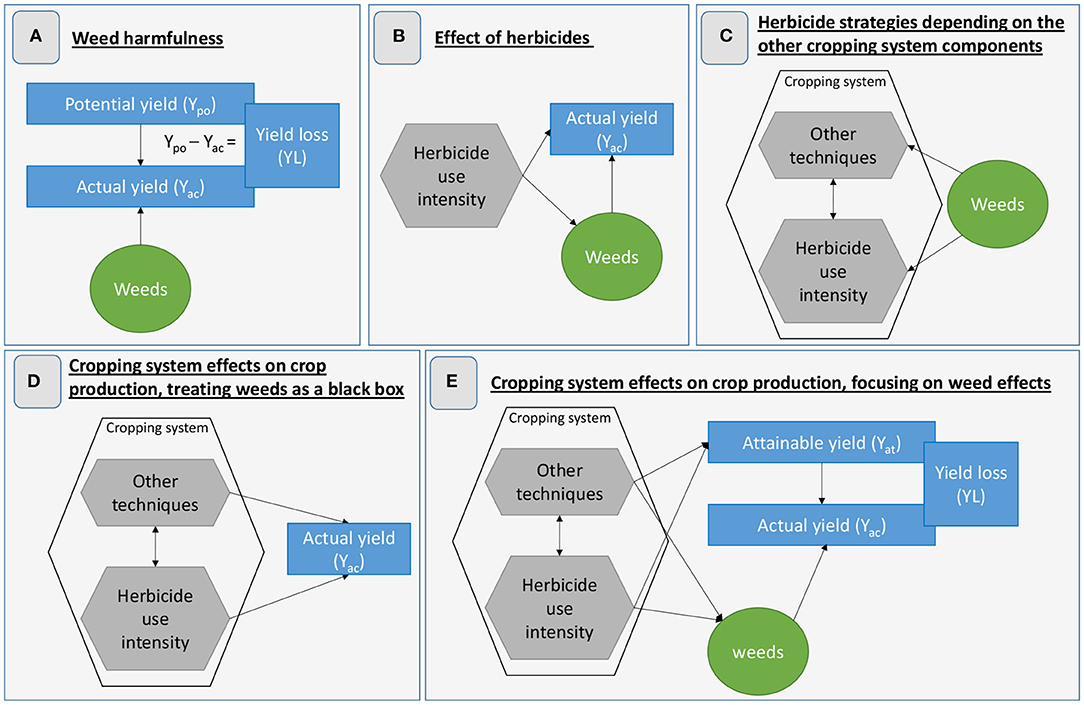
Figure 1. Methodological framework used in the present study to determine the typology of studies investigating the relationships between farming practices including herbicides (gray octagons), weeds (green ovals), and crop yield (blue rectangles). (A) Weed harmfulness (section How Crop:Weed Interference Is Quantified), (B) Effect of herbicides (section Effect of Herbicides on Crop Production), (C) Herbicide strategies depending on the other cropping system components (section How Far Farmers Adapt Their Herbicide Strategies to the Other Farming Practices); (D) Cropping system effects on crop production, treating weeds as a black box (section The Comprehensive Effects of Cropping Systems on Crop Production). (E) Cropping system effects on crop production, focusing on weed effects (section The Comprehensive Effects of Cropping Systems on Crop Production).
Impact of Weeds on Crop Production
There is a large literature on the effects of weeds on crop production (Figure 1A), revealing 1,532 articles published from 1956 to 2019 on the topic of “weed and yield loss” and available in the web of science database (see section Bibliometric Analysis of Literature and Weed-Borne Crop Yield Loss in Supplementary Material online). Oerke et al. (1994) published a book (later synthesized as a review paper (Oerke, 2006)) which remains so far the most complete report of the effect of weeds on crops around the world, revealing a high variability of yield loss due to weeds (see examples in section Range of Variation of Crop Yield Loss Due to Weeds Found in Literature online).
How and When Do Weeds Interfere With Crop Production?
Weeds interact directly with the crop through competition for water and mineral resources (Zimdahl, 2004), allelopathy (Kadioglu et al., 2005), and parasitism (Parker, 2009). Weeds can also host other organisms that can have either positive (DiTommaso et al., 2016) or negative (Mantle et al., 1977; Gutteridge et al., 2006) effects on the crop.
Most studies focused on competition between crops and non-parasitic weeds, experimentally assessing how co-habiting crop and weed plants take up resources like water (McGiffen et al., 1992), nitrogen (Teyker et al., 1991), and light (Rajcan and Swanton, 2001). Many studies aimed to identify the critical weed-free periods needed to avoid yield loss (Martin et al., 2001; Knezevic et al., 2002). Even if the impact of competition is often only visible late in the crop cycle (e.g., flowering), the weeds' harmfulness potential is determined very early (Kropff and Spitters, 1991; Hall et al., 1992; Fahad et al., 2015). For instance, if an oilseed rape (Brassica napus L.) crop remains weed-free until reaching 4–6 leaves, yield losses remain below 10%; conversely, weed control after 4 leaf stage targeting late-emerging weeds was not needed to limit yield loss (Martin et al., 2001). Because weeds compete with crops for resources, some studies advocate that increasing the resource pool diversity should alleviate crop:weed competition (Smith et al., 2010; Menalled et al., 2020). This is probably the case in conservation agriculture where the combination of no-till and cover crops modifies the resource pool diversity (Romdhane et al., 2019) in addition to filtering different weed communities compared to conventional farming (Chauhan et al., 2012; Trichard et al., 2013; Nichols et al., 2015; Cordeau et al., 2020).
How Crop: Weed Interference Is Quantified
The investigation of weed impacts on yield loss is overwrought with methodological difficulties (see the very detailed review of Swanton et al., 2015). There are roughly three types of methods (Table 1).
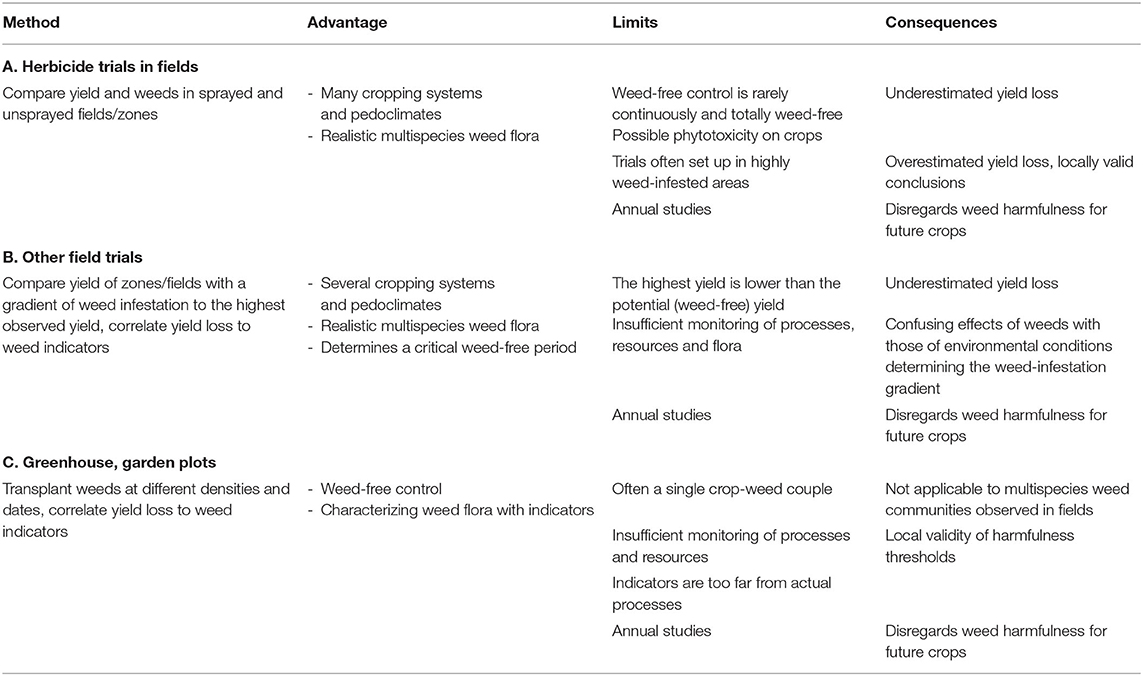
Table 1. Critical summary of methods studying the effects of weeds on crop yield loss based literature analysis.
Herbicide trials (Table 1A) are annual standardized factorial experiments designed to assess the efficacy of modalities of herbicide use (single product, association or strategies) to control weed infestation (https://pp1.eppo.int/standards/PP1-152-4, https://pp1.eppo.int/standards/herbicides). Those trials that last beyond post-spraying weed assessment until crop harvest are sometimes used to assess yield loss by comparing yields between treated plots (as a proxy for weed-free control) and untreated plots (infested by weeds) (Florez et al., 1999; Milberg and Hallgren, 2004; Fickett et al., 2013) Some studies compared more complex situations, for example several modalities with an increasing use of herbicides in order to create a gradient of weed abundances (Dieleman et al., 1999; Boström and Fogelfors, 2002).
Other harmfulness studies identified zones inside a field or similar fields with a weed-density gradient but otherwise identical (Table 1B). Yield loss is then estimated as the difference of the yield in the different zones or fields relative to the maximum observed yield, and then linked the yield-loss estimation to a series of weed flora variables (e.g., plant densities, biomass). But simple weed metrics are not sufficient to explain yield loss as recent field studies reported that yield loss decreases with increasing diversity and richness in the weed community (Storkey and Neve, 2018; Adeux et al., 2019b).
Studies in greenhouse or garden plots create weed-density gradients, by transplanting weeds (usually a single species) at different dates and densities to mimic contrasting weed emergence flushes, and this at different crop stages (Table 1C). The biomass or grain production losses due to the presence of weeds are usually linked to weed variables and used to determine thresholds for weed management (Oliver, 1988). Frequently used variables were weed density (Cousens, 1985; McDonald and Riha, 1999) or, with better results, weed species specificity (Onofri and Tei, 1994), weed leaf area (Kropff and Spitters, 1991; Lotz et al., 1996; van Acker et al., 1997), or weed biomass (Milberg and Hallgren, 2004). All these approaches suffer from methodological drawbacks (Table 1), prominently among which the difficulty to estimate the potential yield in the absence of weeds obtained in the same pedoclimatic and cultural conditions.
Herbicide trials are primarily set up to assess the effect of crop protection (i.e., the difference between yield obtained with and without weeding) and not yield loss due to weeds. Treated plots are not necessary totally and constantly weed-free. Moreover, herbicides can be phytotoxic for the crop in certain weather conditions or at early crop stages (Cabanne et al., 1985; Carvalho et al., 2009). Both events can lead to underestimating potential yield. The best way to estimate yield losses at the annual scale consists in comparing the yield in weedy zones to that in weed-free controls without chemical or mechanical weeding (Adeux et al., 2019b). Indeed, mechanical weeding is also likely to affect crop growth, e.g., through modification in the nitrogen dynamics (Gilbert et al., 2009) or by uprooting crop plants (Rasmussen et al., 2009). But even the best of these approaches neglect long-term weed harmfulness even though this is the main reason why farmers relentlessly target weeds (Macé et al., 2007).
Last, these methods produce only locally valid conclusions, with a very high risk of confusing effects. Herbicide trials are usually set up preferentially in fields with an abundant flora or difficult-to-control species (e.g., Alopecurus myosuroides Huds., Lolium multiflorum Lam.). The results, therefore, mostly have a local validity, and any national estimation based on these data would probably overestimate weed-caused yield loss. Studies monitoring weed-infestation gradients risk confusing the effect of weed pressure with that of the local environmental conditions driving weed gradients. While experiments in controlled conditions (greenhouse, garden plots) do not suffer from this deficiency, they are disadvantaged by a highly artificialized weed flora.
Implications for Weed Management
Some field experiments attempted to provide indicators for deciding when to weed, depending on the crop and/weed stages. Trials such as those of Table 1B aimed to determine the critical weed-free periods needed to avoid yield loss (Martin et al., 2001; Knezevic and Datta, 2015). Methods to determine these critical periods have been largely criticized (Knezevic et al., 2002; Knezevic and Datta, 2015). Nutrient content in crops or resource availability in the field were rarely measured, even though the resources for which crops and weeds compete vary according to year, location and cropping system. Consequently, even for a given crop (e.g., maize, Zea mays L.), the critical weed control period varied considerably according to years, locations and authors, both in terms of onset (2 to 14 leaf) and end date (12 leaf to 1 week after flowering) (Hall et al., 1992; Hugo et al., 2014).
Other studies linked weeding decisions to a weed harmfulness threshold, based on empirical relationships correlating yield loss to weed indicators estimated in fields (Table 1B) or controlled conditions (Table 1C). The concept of harmfulness threshold is highly questionable (Oliver, 1988; O'Donovan, 1996; Swanton et al., 1999). In short, even the best of these thresholds usually disregard variability in water and nutrient resources, rarely quantify yield losses due to weed assemblages (Swinton et al., 1994), and only consider annual effects (McDonald and Riha, 1999; Munier-Jolain et al., 2002). In addition, the most pertinent weed indicators (i.e., those closest to processes implicated in crop-weed competition) such as relative leaf cover (Kropff and Spitters, 1991) are impractical for taking weed control decisions. Such practical limitations explain why weed densities are usually used to establish damage thresholds, i.e., the lowest weed density for which a decrease in crop yield is detected (Coble and Mortensen, 1992). Last, the value of the threshold triggering weeding does not actually change the treatment frequency, and the sustainability of a cropping system relies on whether the decrease in herbicide use intensity is compensated by non-chemical cultural practices (Munier-Jolain et al., 2002).
Effect of Herbicides on Crop Production
This section reports on studies that investigated the impact of herbicide use intensity on weed infestation or yield loss, but without analyzing the direct impact of weeds on crop yield (Figure 1B). To simplify we will disregard here the ever increasing problem of herbicide resistance (Busi et al., 2013) but discuss it in the conclusion section (section Synthesis and Conclusions).
Herbicide Trials
Herbicide trials (see definition in section How Crop:Weed Interference Is Quantified) have established that herbicides are efficient in controlling weeds but that their efficacy depends (unsurprisingly) on weed species (Jonathan et al., 1998), herbicide rates (Streibig, 1980), application dates (Stougaard et al., 1997), and spraying conditions (Blumhorst et al., 1990) (Table 2A). These effects have been summarized in handbooks (e.g., Mamarot and Rodriguez, 2003) and various decision-support systems (e.g., Kudsk, 2008). But when these trials attempt to determine to what extent the use of crop protection prevents yield losses, they encounter the same methodological setbacks to determine yield loss as the studies of section How Crop:Weed Interference Is Quantified (Cardina et al., 1997).
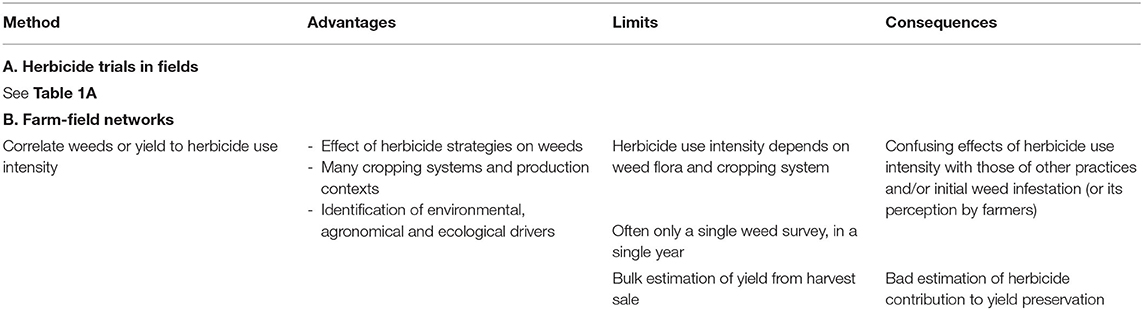
Table 2. Critical summary of methods investigating the effects of herbicides on weeds and/or crop yield based literature analysis.
Most herbicide trials last for 1 year only, thus missing future yield losses due to the descendants of the weed seed production during the trial year. One rare exception (Boström and Fogelfors, 2002) assesses the effect of dosage and treatment frequency in a 10 year multi-site experiment. It showed no difference in crop yield of fields sprayed at 25 and 100% of a full dose, even though total weed densities increased by 43 to 67%. These and other results (Salonen, 1992; Blackshaw et al., 2006) remind us that herbicides, even though considered as the most efficient “hammer” against weeds, are not a 100% efficient tool to control the whole weed community and, more importantly, that there is no generic relationship between herbicides, weeds, and yield.
Farm-Field Surveys
Farm-field surveys monitor cultural practices and real-life weed floras in a large range of contrasting situations, at a regional (Petit et al., 2016; Yvoz et al., 2020) or even national scale (Rydberg and Milberg, 2000; Fried et al., 2008). They allow assessing the environmental, agronomic and ecological drivers of the in-field weed flora, and notably the relative contribution of agronomic and environmental factors (Schumacher, 1987; Fried et al., 2008; Seifert et al., 2015), landscape context and/or of farming systems (Gabriel et al., 2005; Geiger et al., 2010; Petit et al., 2016) on weed infestation levels and/or weed community composition. This approach has proved successful to detect long-term weed response to contrasted agricultural management strategies, for example, the generic signal of higher weed cover and/or weed seed bank abundance in organic vs. conventional systems (Hawes et al., 2010).
However, the suitability of this approach to assess the impacts of herbicide use on weed infestation in conventional fields is highly questionable (Table 2B). Annual surveys, particularly those disregarding past field history and initial weed pressure (e.g., Gaba et al., 2016), are meaningless to link herbicide use and post-weeding weed infestation or yield as farmers adapt herbicide use intensity to the initial weed infestation and to other cultural techniques (Figure 2). The absence of any correlation between herbicide use intensity and weed abundance sometimes reported in literature (Gabriel et al., 2005; Gaba et al., 2016; Petit et al., 2016) cannot be attributed to a lack of efficacy of herbicide use, as reported by some authors (Gaba et al., 2016) but either results from farmers' mental models (i.e., reduce herbicides to the benefit of non-chemical operations, trigger spraying based on observed weed communities; Kings, 2014) or from unsuitable protocols (i.e., assessing the effect of herbicide on weeds based on post-spraying weed surveys only, without any knowledge on pre-spraying floras; Gaba et al., 2016). To properly address the question of the impact of herbicide use on weed infestation, the weed flora should at the very least be surveyed twice a year, i.e., before and after chemical weeding (Milberg and Hallgren, 2004).
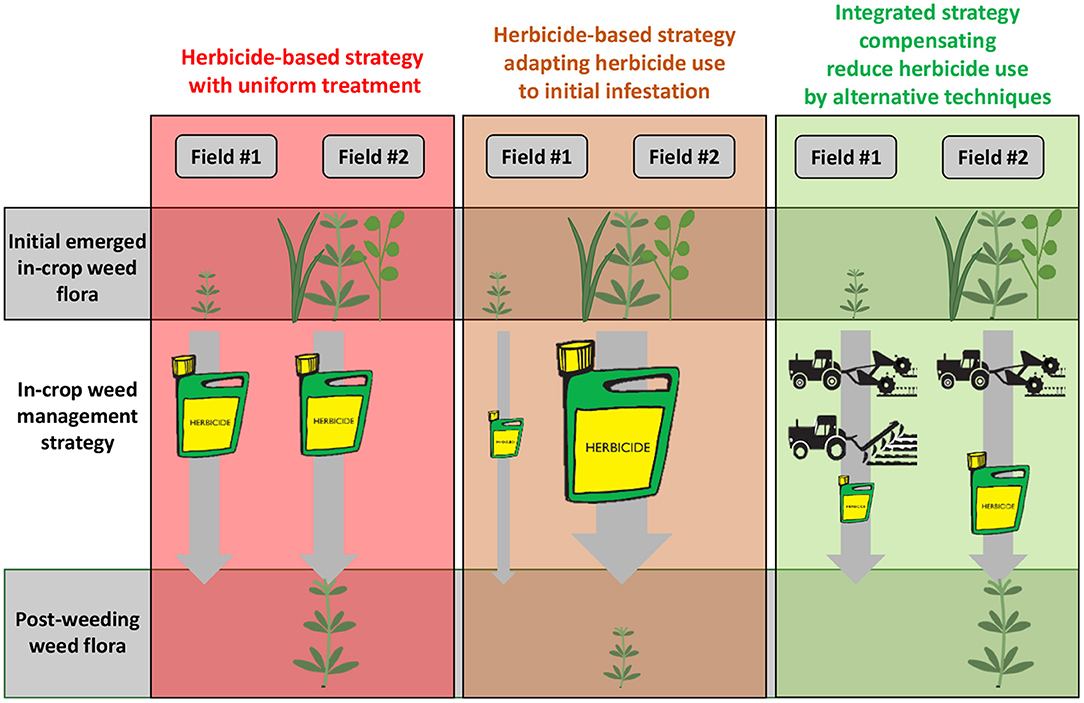
Figure 2. Impact of initial weed infestation and of the farmer's herbicide strategy on herbicide use intensity and final weed flora.
How Far Farmers Adapt Their Herbicide Strategies to the Other Farming Practices
This section deals with interaction between herbicide use and other farming practices (Figure 1C) Herbicide use intensity depends not only on initial weed infestation and the farmer's weeding strategy (Figure 2) but also on other practices (Beltran et al., 2013; Colbach and Cordeau, 2018), particularly in Integrated Weed Management (Swanton and Weise, 1991). The intensity decreases if fields are tilled, weeded mechanically and/or grown with diversified rotations (Yvoz et al., 2020), depending not only on the frequency but also the timing of non-chemical disturbances (Table 3).
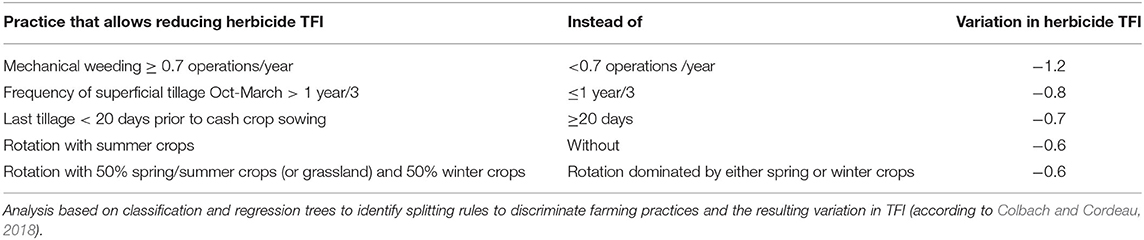
Table 3. Main variations in herbicide use intensity (expressed by the treatment frequency index, TFI) as a function of other farming practices, identified in 272 cropping systems provided by farm surveys, agricultural statistics, and crop advisors.
These interactions results largely from the farmer's attitude and perceptions. Low herbicide use requires a long-term strategic management of weeds, aiming to prevent rather than to control weeds (Macé et al., 2007). Many farmers though focus on control rather than on prevention (Wilson et al., 2008), and the type of approach depends, among others, on the production situation. For instance, farmers with access to varieties tolerant to non-selective herbicides such as glyphosate frequently simplify rotations (Fausti et al., 2014) and tillage (Trigo and Cap, 2003; Cerdeira and Duke, 2006). They accept to plant into a weedy seedbed and rely on glyphosate applications on crop canopy to control weeds (Johnson et al., 2007). So, often farmers include integrated weed management options only when no other choice is available, for instance when weeds become resistant to herbicides (Llewellyn et al., 2004; Colas et al., 2020).
Risk aversion also influences weed management strategies, with farmers focusing on minimizing the risk of failure even at the cost of lowering their average economic performance (Wossink et al., 1997; Doohan et al., 2010; Ridier et al., 2013). This explains why herbicide use intensity tends to be higher in cropping systems taken from farm surveys and field monitoring networks than those tested in research stations (−3% averaged over rotation), proposed by advisors (−15%) or designed with simulations (−26%) (Colbach and Cordeau, 2018). Economic factors (e.g., herbicide prices, farm size) and labor requirements are also important determinants in the selection of a weed control technique by farmers (Wossink et al., 1997; Llewellyn et al., 2004; Hammond et al., 2006; Beltran et al., 2013; Jabbour et al., 2014b).
The way farmers perceive weeds is a major obstacle to reducing herbicide use (Rioux, 1994; Wossink et al., 1997), particularly if they attribute weeds to factors outside their control, such as weather events or uncontrolled weed growth in neighboring fields (Wilson et al., 2008; Doohan et al., 2010). Their preferences reflect a typical inverse relationship between perceived risk and benefit (Doohan et al., 2010), underestimating the risks resulting from overreliance on herbicides (Doohan et al., 2010) and overestimating the incidence of problematic weeds (Borger et al., 2012). Knowledge about weed biology and the effects of crop management practices is essential to overcome this deadlock. Indeed, farmers that exhibit great knowledge on these aspects and that critically discuss risks of weeds and benefits of management practices tend to have fields with a lower weed infestation (Jabbour et al., 2014a).
The Comprehensive Effects of Cropping Systems on Crop Production
This section reports on studies that investigated the effect of cropping systems on crop production, either without assessing weed floras or other pests (“black box” approach, Figure 1D), or by unraveling all components and effects of the general framework of Figure 1E, including weeds.
Cropping System Experiments
Cropping system experiments have been set up all over Europe aiming at a detailed, multiannual and multicriteria evaluation of novel cropping practices such as low-input or pesticide-free systems (Lechenet et al., 2017b). Most cropping system experiments were designed to test the feasibility of these systems with a wide range of objectives (e.g., reducing pesticide use or increasing crop diversity while maintain profitability, decreasing impact on soil structure, etc.) without looking at weeds (Deytieux et al., 2012; Giuliano et al., 2016), implemented in single sites or multi-site networks (Deytieux et al., 2016). Few experiments monitored weed floras (Chikowo et al., 2009; Debaeke et al., 2009; Adeux et al., 2017, 2019a; Jernigan et al., 2017) and even fewer assessed weed-driven yield loss, for instance by comparing yield in weedy and weed-free (manually weeded) zones (Teasdale and Cavigelli, 2010; Adeux et al., 2017). This lack of monitoring results in a high risk of confusing effects (Table 4A).
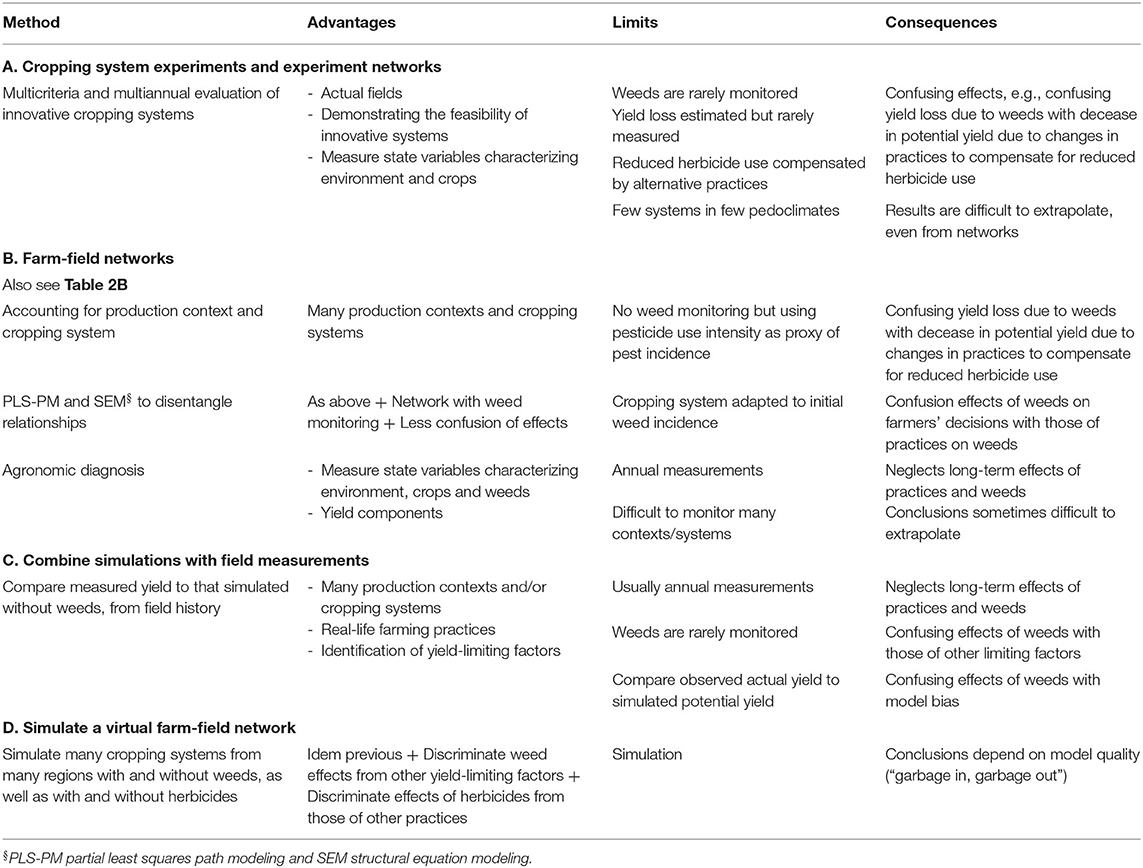
Table 4. Critical synthesis of the methods investigating cropping system effects on weeds and crop yield based literature analysis.
The holistic approach of cropping-system experiments compares coherent systems instead of factors, which can lead to seemingly contradictory results. For instance, a recent analysis of long-term experiments conducted in different regions concluded that yield would decrease by 5 to 13% compared to the yield obtained with current pesticide use if pesticide use was reduced by 50% (Hossard et al., 2014). This study was though unable to determine which pest was actually responsible for the yield decrease and, most importantly, how much of this yield decrease was due to a lower yield potential resulting from changes in management practices. For instance, delayed wheat sowing was reported to reduce emergence and survival of broad-leaved weed species (by about 20–30%) but in the absence of weeds decreased yield (by up to 30%), resulting from deteriorated weather or less productive cultivars (Christensen et al., 1994). If experiments specifically account for weeds, no correlation between herbicide use intensity, weed plant density and crop production was found, irrespective of the location (Eastern France, Chikowo et al., 2009; central France, Colbach et al., 2016; South-Western France, Adeux et al., 2017).
Consequently, most cropping-system trials and studies demonstrate local feasibility of reconciling reduced herbicide use and reduced crop yield loss but cannot explain causes and thus do not offer advice applicable elsewhere (Deytieux et al., 2016). Moreover, results collected in experimental stations (Deytieux, 2017) can differ from those collected from farms (Lechenet et al., 2017a) because experimenters do not farm as farmers do as they tend to explore extreme alternative strategies without having the economic survival of their farms depending on their success (Deytieux et al., 2012).
Farm Field Networks
To increase the number of investigated production situations and cropping systems, farm-field surveys (Seufert et al., 2012; Lechenet et al., 2014; Petit et al., 2016) and demonstration-farm networks like the French DEPHY network (Lechenet et al., 2017a) were set up but many methodological problems remain (Table 2B). Some of these could be alleviated by new statistical and survey methods. For instance, by including the production context in their analysis, Lechenet et al. (2017a) failed to detect any conflict between low herbicide use on one hand, and, on the other hand, high productivity at the cropping-system level (in 71% of the farms) or high profitability (in 79% of the farms). However, these authors did not monitor weeds or any other biotic or abiotic components, which hampers the identification of the causes of variability in farm-field networks (Table 4B).
Structural equation modeling (SEM) has been used to overcome these limits (Lamb et al., 2011; McLeod et al., 2015; Quinio et al., 2017). Among the few studies that include weed surveys, Quinio et al. (2017) thus discriminated the three pathways linking farming intensity (fallow management, sowing, chemical pest control and fertilization), crop yield, and weed pressure (Table 4B). This analysis considerably reduced the risk of confusing effects, showing that crop yield increased with farming intensity and decreased with weed pressure (section Pathway Analysis of Field Survey Data online). Overall, the authors showed that farming intensity reduced weed pressure sufficiently to cancel any negative effects that weeds had on yield.
Diagnostic studies of farmers' fields go even further and investigate a large range of limiting factors of yield (Valantin-Morison and Meynard, 2008; Subedi and Ma, 2009). These studies measure state variables describing weeds and other pests (e.g., weed density and biomass at different stages), nutrition status (e.g., nitrogen absorption by both the crop and the weeds), resource availability in the soil, as well as crop yield components. Yield components are linked to potential limiting factors, often showing that weeds are the most important limiting factor (oilseed rape in France, Valantin-Morison and Meynard, 2008; maize in Eastern Canada, Subedi and Ma, 2009), identifying the weed variables that are the most linked to yield component variance (e.g., weed dry biomass and plant density accounting for nearly 40% of variance of oilseed rape grain number per m2, Valantin-Morison and Meynard, 2008) or the main drivers of the weed floras (e.g., previous crop, tillage and oilseed rape sowing density, Valantin-Morison and Meynard, 2008). However, the cost of these measurements limits both the number of monitored situations and the duration of monitoring, thus disregarding any long-term effects of weeds and cultural practices.
Combining Simulation Models With Field Data
Simulation-based studies can go further, by using process-based crop models (with or without weeds) to estimate the potential yield (i.e., yield in the absence of weeds) which is so difficult to estimate in fields (Table 4C), the actual yield (in the presence of weeds) in many situations and cropping systems (Table 4D). Depending on which processes are included in the model, this approach allows identifying different yield-limiting factors to reduce the risk of confusing effects. The first approach compares the simulated potential yield to actual yield measured in fields (Affholder et al., 2003, 2013; van Ittersum et al., 2003; Silva et al., 2017). In addition to previously mentioned methodological drawbacks (Table 4C), this approach risks to confuse effects of agronomic and environmental conditions in the field with model bias and error.
In order to limit this risk, to cover more situations and to move beyond the annual scale, some teams went completely virtual (Colbach and Cordeau, 2018), which would not have been possible without the more recent development of mechanistic multispecies multiannual crop-weed dynamics models (see short description in section Weed Dynamics Models online). These authors simulated several hundred cropping systems provided by farm surveys, agricultural statistics or crop advisors over 30 years (to assess long-term effects) and with 10 weather scenarios. This approach allowed disentangling the effect of herbicide use intensity from that of other management practices by comparing the simulated weed floras and yields of the recorded cropping systems to those of these same systems minus herbicides (and without any other changes in practices). The relative effects of weeds and management practices on crop production were differentiated by comparing the yields of simulations run with and without weeds. As a result, this study could confirm and/or demonstrate the key conclusions of the present paper, and quantify them with values valid for a large range of production contexts and cropping systems. For instance, yield loss exceeds 50% when weed biomass exceeds crop biomass (further details in section Simulation Results Linking Yield Loss to Weed Biomass Online), or weed biomass during crop growth and yield loss increased by +116% and +62% (averaged over rotation), respectively, when herbicides were eliminated without redesigning the cropping system.
Synthesis and Conclusions
Impact of Weeds on Crop Production
Weed harmfulness for crop production is usually studied disregarding the complexity of the cultural practices, the multispecies nature of the weed flora (Massinga et al., 2001) and the processes underlying weed harmfulness, which limits the validity and the genericity of the results. Yield loss due to weeds tends to be underestimated because of the annual investigating scale and a poorly estimated potential yield. Despite existing methodological shortcomings, almost all studies conclude that weeds reduce yield if they are not controlled (Zimdahl, 2004), particularly if they emerge earlier or at crop emergence (Chikoye et al., 1995). The most accurate method to estimate yield loss at the annual scale consists in comparing the yield in weedy zones to that in weed-free controls without chemical or mechanical weeding. This approach allowed Adeux et al. (2019b) to conclude that weed diversity mitigates winter wheat yield loss and that not all weed communities are detrimental to crop productivity. Yield loss is more correlated to weed metrics closer to processes driving crop-weed competition than to weed density. Weed thresholds based on weed density are useless to forecast yield loss.
Effect of Herbicides on Crop Production
Because studies are usually annual and often limited to a single observation per field and per year, the contribution of herbicides to controlling weeds and yield loss can be underestimated. The lack of correlation between herbicide use intensity and weed abundance sometimes reported in literature (Gaba et al., 2016) cannot be attributed to a lack of efficacy of herbicide use, but rather to unsuitable survey protocols (i.e., assessing the effect of herbicide on weeds on post-spraying weed surveys only, without accounting for pre-spraying weed infestation). The weed flora must be assessed before and after weeding to evaluate its efficiency. And though herbicides are not always totally effective (even when weeds did not acquire resistance), they do reduce weed infestation and yield loss due to weeds.
How far Farmers Adapt Their Herbicide Strategies to the Other Farming Practices
The herbicide strategy used by a farmer in terms of commercial products, rates and timings of application depends on the other practices applied in the field as well as on the weed flora perceived by the farmer, his risk strategy and his production situation (Yvoz et al., 2020). Any attempt to assess the impact of herbicide use intensity on weed flora and yield loss must account for these interactions to avoid confusing effects (Quinio et al., 2017).
A Conceptual Framework Embedding the Herbicide-Weed-Yield Relationships
Based on the present review, we proposed a conceptual framework to synthesize the key variables and effects driving the relationships between herbicides, weeds and crop production (Figure 3), inspired by structural equation modeling using expert knowledge (Smith et al., 2014). This diagram not only illustrates that the effects of herbicides or weeds on crop productivity cannot be considered without accounting for the technical, and biophysical and socio-economic context. It also shows frequent feed-backs, e.g., herbicides indeed reduce weed densities but farmers increase herbicide use if they observed many emerging weeds. It is as yet difficult to quantify the individual links, particularly as the correlations are not necessarily linear, though some field and simulation studies cited above attempted to do this (Lamb et al., 2011; McLeod et al., 2015; Quinio et al., 2017).
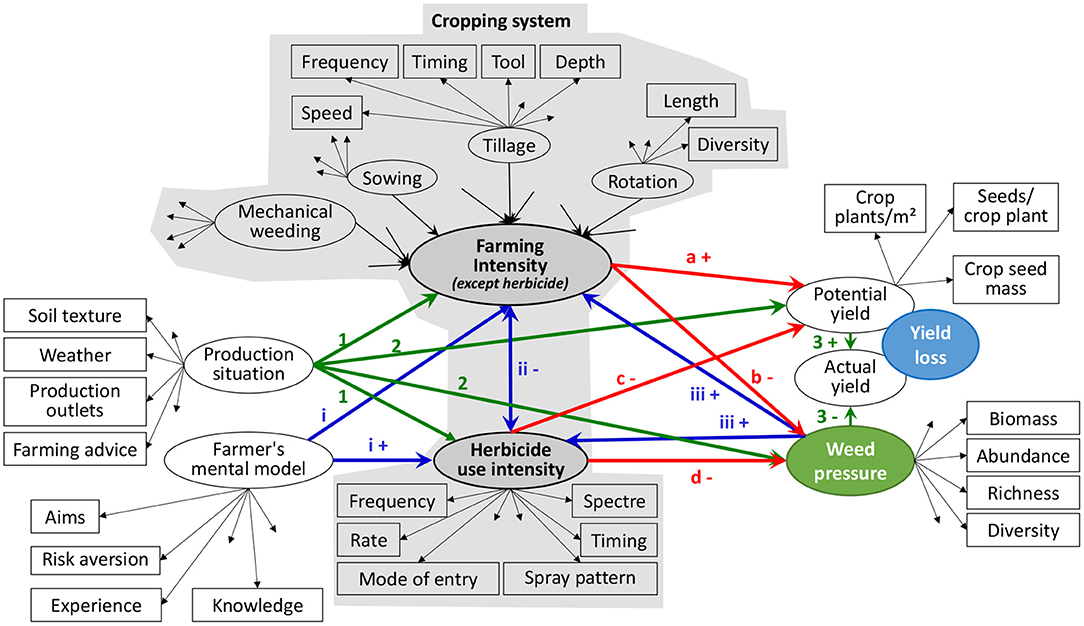
Figure 3. Conceptual representation of cropping system effects on weeds and crop production in farmers' fields inspired by structural equation modeling to synthesize the relationships between herbicide use intensity, weed pressure, and yield loss based on the present literature analysis. Circles show latent variables (in bold the targets investigated in the present study), and rectangles manifest variables. The gray zone delimits the cropping system. Note that the arrows point from the latent variables to the manifest variables. Red arrows are effects of cropping system components (a. to create favorable conditions for crop growth, b. to create unfavorable conditions for weeds and to destroy them; c. phytotoxicity, d. weed destruction); green arrows show environmental effects (1. cropping choices depend on biophysical and socio-economical constraints; 2. crop growth and weed community depend on biophysical environment; 3: yield loss is the difference between attainable and actual yield due to weeds); blue arrows show farmers' decisions (i. farmers choose cropping techniques, including herbicides, based, among others, on their risk aversion; ii: the choice of the herbicide strategy depends on other techniques and vice-versa; iii: the choice of cropping techniques, particularly herbicides, depends on the past and present weed community). Positive (+) and negative (–) effects were shown for latent variables.
Implications for Future Research and Weed Management
Our review demonstrates that understanding the herbicide use-weed-crop yield nexus requires to include all the components of the studied system (Figure 1E) and to deconstruct them in detail as illustrated in Figure 3. The apparent inconsistencies identified in the literature appear to have resulted from differences in methodological approaches and a few precautions are essential to avoid confusing effects (Table 5). The critical analysis of a large range of studies contrasting in terms of objectives and methodologies allowed us to answer the two questions addressed in this review, namely how harmful weeds are and whether herbicide use can be reduced without affecting yield. In summary, weeds are harmful for crop production but this harmfulness varies considerably and decreases when (1) weed biomass decreases, (2) weeds emerge later than the crop, (3) the weed community consists of many diverse species, (4) available resources increase (in highly fertilized/irrigated systems). Crop yield loss is highly correlated to weed variables closely linked to processes driving crop-weed competition but the best indicator variables (e.g., weed biomass at crop flowering) are useless for weed control decisions because they are measured too late.
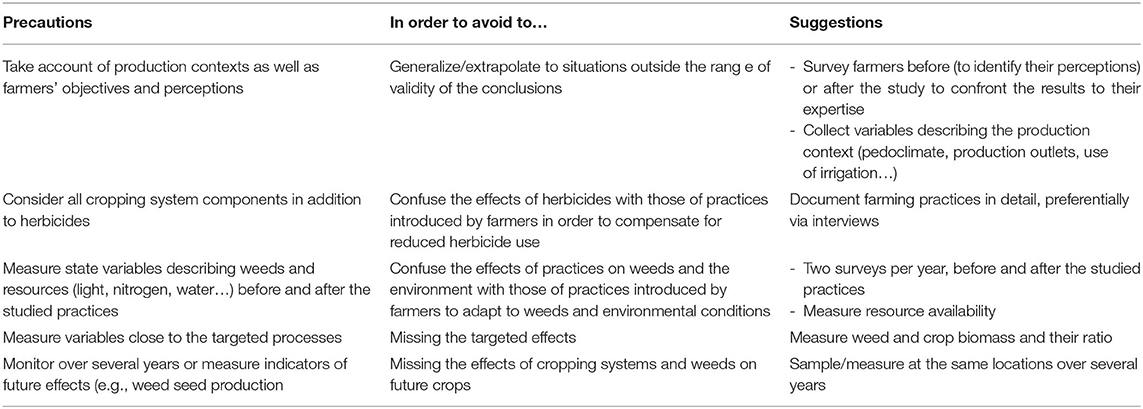
Table 5. Major precautions needed to study the relationships between herbicides, weeds and yield to avoid methodological defects.
These results advocate for a strategic long-term reasoning of weed management instead of tactical decisions based on current weed communities. Indeed, despite the undeniable weed harmfulness for crop production, well-reasoned integrated weed management can preserve crop production in cropping systems with reduced herbicide use even though herbicides remain to date the most efficient weed control technique, except in the case of herbicide-resistant weeds. However, in this particular case, many field studies show that integrated weed management can be highly efficient to control herbicide-resistant populations (e.g., Chauvel et al., 2001, 2009). These results have been synthesized already in reviews on strategies for managing herbicide-resistant populations (Beckie, 2006; Busi et al., 2013; Riar et al., 2013).
There is thus no unique single solution that is valid everywhere. Flexible solutions are required, considering the agronomical logic underlying cropping systems and the production context but also other potential levers that could be mobilized to enhance the biological regulation of weeds (Petit et al., 2018). These solutions might require to accept a certain level of weed presence in the field, and should also consider potential weed benefits for crop production, i.e., habitat provision for natural enemies (Dassou and Tixier, 2016; DiTommaso et al., 2016).
Data Availability Statement
The original contributions presented in the study are included in the article/supplementary materials, further inquiries can be directed to the corresponding author/s.
Author Contributions
All the authors contributed to the analysis of the literature and writing.
Funding
This work was supported specifically by INRAE (Environment and Agronomy Department, Plant Health and Environment Department), the French CoSAC project (ANR-15-CE18-0007) and ADVHERB projects (ANR-08-001), the European Union's Horizon 2020 Research and Innovation programme under grant agreement N 727321 (IWMPRAISE project), and the DEPHY-farm and DEPHY-EXPE grants.
Conflict of Interest
The authors declare that the research was conducted in the absence of any commercial or financial relationships that could be construed as a potential conflict of interest.
Acknowledgments
This review is the result of many past projects conducted within the Sustainable Weed Management Department of the Agroecology research unit (Dijon, France). The authors are grateful to several reviewers that helped to improve the paper.
Supplementary Material
The Supplementary Material for this article can be found online at: https://www.frontiersin.org/articles/10.3389/fagro.2020.615470/full#supplementary-material
References
Adeux, G., Giuliano, S., Cordeau, S., Savoie, J.-M., and Alletto, L. (2017). Low-input maize-based cropping systems implementing IWM match conventional maize monoculture productivity and weed control. Agriculture 7:74. doi: 10.3390/agriculture7090074
Adeux, G., Munier-Jolain, N., Meunier, D., Farcy, P., Carlesi, S., Barberi, P., et al. (2019a). Diversified grain-based cropping systems provide long-term weed control while limiting herbicide use and yield losses. Agron. Sustain. Dev. 39:42. doi: 10.1007/s13593-019-0587-x
Adeux, G., Vieren, E., Carlesi, S., Bàrberi, P., Munier-Jolain, N., and Cordeau, S. (2019b). Mitigating crop yield losses through weed diversity. Nat. Sustain. 2, 1018–1026. doi: 10.1038/s41893-019-0415-y
Affholder, F., Poeydebat, C., Corbeels, M., Scopel, E., and Tittonell, P. (2013). The yield gap of major food crops in family agriculture in the tropics: assessment and analysis through field surveys and modelling. Field Crops Res. 143, 106–118. doi: 10.1016/j.fcr.2012.10.021
Affholder, F., Scopel, E., Madeira Neto, J., and Capillon, A. (2003). Diagnosis of the productivity gap using a crop model. Methodology and case study of small-scale maize production in central Brazil. Agronomie 23, 305–325. doi: 10.1051/agro:2003004
Andersson, T. N., and Milberg, P. (1998). Weed flora and the relative importance of site, crop, crop rotation, and nitrogen. Weed Sci. 46, 30–38. doi: 10.1017/S0043174500090135
Andreasen, C., Stryhn, H., and Streibig, J. (1996). Decline of the flora in Danish arable fields. J. Appl. Ecol. 33, 619–626. doi: 10.2307/2404990
Beckie, H. J. (2006). Herbicide-resistant weeds: management tactics and practices. Weed Technol. 20, 793–814. doi: 10.1614/WT-05-084R1.1
Beltran, J. C., White, B., Burton, M., Doole, G. J., and Pannell, D. J. (2013). Determinants of herbicide use in rice production in the Philippines. Agric. Econ. 44, 45–55. doi: 10.1111/j.1574-0862.2012.00631.x
Blackshaw, R. E., O'Donovan, J. T., Harker, K. N., Clayton, G. W., and Stougaard, R. N. (2006). Reduced herbicide doses in field crops: a review. Weed Biol. Manag. 6, 10–17. doi: 10.1111/j.1445-6664.2006.00190.x
Blumhorst, M. R., Weber, J. B., and Swain, L. R. (1990). Efficacy of selected herbicides as influenced by soil properties. Weed Technol. 4, 279–283. doi: 10.1017/S0890037X00025392
Borger, C., Michael, P., Mandel, R., Hashem, A., Bowran, D., and Renton, M. (2012). Linking field and farmer surveys to determine the most important changes to weed incidence. Weed Res. 52, 564–574. doi: 10.1111/j.1365-3180.2012.00950.x
Boström, U., and Fogelfors, H. (2002). Long-term effects of herbicide-application strategies on weeds and yield in spring-sown cereals. Weed Sci. 50, 196–203. doi: 10.1614/0043-1745(2002)050[0196:LTEOHA]2.0.CO;2
Busi, R., Vila-Aiub, M. M., Beckie, H. J., Gaines, T. A., Goggin, D. E., Kaundun, S. S., et al. (2013). Herbicide-resistant weeds: from research and knowledge to future needs. Evol. Appl. 6, 1218–1221. doi: 10.1111/eva.12098
Cabanne, F., Gaillardon, P., and Scalla, R. (1985). Phytotoxicity and metabolism of chlortoluron in two wheat varieties. Pestic. Biochem. Physiol. 23, 212–220. doi: 10.1016/0048-3575(85)90008-2
Cardina, J., Johnson, G. A., and Sparrow, D. H. (1997). The nature and consequence of weed spatial distribution. Weed Sci. 45, 364–373. doi: 10.1017/S0043174500092997
Carvalho, S. J. P. D., Nicolai, M., Ferreira, R. R., Figueira, A. V. D. O., and Christoffoleti, P. J. (2009). Herbicide selectivity by differential metabolism: considerations for reducing crop damages. Sci. Agric. 66, 136–142. doi: 10.1590/S0103-90162009000100020
Caussanel, J.-P., Kafiz, B., and Carteron, A. (1988). Analyse expérimentale des effets de concurrence d'une graminée adventice dans un blé de printemps en relation avec le désherbage. Weed Res. 28, 309–322. doi: 10.1111/j.1365-3180.1988.tb00809.x
Cerdeira, A. L., and Duke, S. O. (2006). The current status and environmental impacts of glyphosate-resistant crops: a review. J. Environ. Q 35, 1633–1658. doi: 10.2134/jeq2005.0378
Chauhan, B. S., Singh, R. G., and Mahajan, G. (2012). Ecology and management of weeds under conservation agriculture: a review. Crop Protect. 38, 57–65. doi: 10.1016/j.cropro.2012.03.010
Chauvel, B., Guillemin, J. P., and Colbach, N. (2009). Evolution of a herbicide-resistant population of Alopecurus myosuroides Huds. in a long-term cropping system experiment. Crop Protect. 28, 343–349. doi: 10.1016/j.cropro.2008.11.013
Chauvel, B., Guillemin, J. P., Colbach, N., and Gasquez, J. (2001). Evaluation of cropping systems for management of herbicide-resistant populations of blackgrass (Alopecurus myosuroides Huds.). Crop Protect. 19, 127–137. doi: 10.1016/S0261-2194(00)00065-X
Chikowo, R., Faloya, V., Petit, S., and Munier-Jolain, N. M. (2009). Integrated weed management systems allow reduced reliance on herbicides and long-term weed control. Agric. Ecosyst. Environ. 132, 237–242. doi: 10.1016/j.agee.2009.04.009
Chikoye, D., Weise, S. F., and Swanton, C. J. (1995). Influence of common ragweed (Ambrosia artemisiifolia) time of emergence and density on white bean (Phaseolus vulgaris). Weed Sci. 43, 375–380. doi: 10.1017/S0043174500081352
Christensen, S., Rasmussen, G., and Olesen, J. E. (1994). Differential weed suppression and weed control in winter wheat. Aspects Appl. Biol. 40, 335–342.
Clewis, S. B., Askew, S. D., and Wilcut, J. W. (2001). Common ragweed interference in peanut. Weed Sci. 49, 768–772. doi: 10.1614/0043-1745(2001)049[0768:CRIIP]2.0.CO;2
Coble, H. D., and Mortensen, D. A. (1992). The threshold concept and its application to weed science. Weed Technol. 6, 191–195. doi: 10.1017/S0890037X00034552
Colas, F., Cordeau, S., Granger, S., Jeuffroy, M.-H., Pointurier, O., Queyrel, W., et al. (2020). Co-development of a decision support system for integrated weed management: contribution from future users. Eur. J. Agron. 114:126010. doi: 10.1016/j.eja.2020.126010
Colbach, N., Bertrand, M., Busset, H., Colas, F., Dugué, F., Farcy, P., et al. (2016). Uncertainty analysis and evaluation of a complex, multi-specific weed dynamics model with diverse and incomplete data sets. Environ. Model. Softw. 86, 184–203. doi: 10.1016/j.envsoft.2016.09.020
Colbach, N., and Cordeau, S. (2018). Reduced herbicide use does not increase crop yield loss if it is compensated by alternative preventive and curative measures. Eur. J. Agron. 94, 67–78. doi: 10.1016/j.eja.2017.12.008
Cordeau, S., Baudron, A., and Adeux, G. (2020). Is tillage a suitable option for weed management in conservation agriculture? Agronomy 10:1746. doi: 10.3390/agronomy10111746
Cousens, R. (1985). A simple model relating yield loss to weed density. Ann. Appl. Biol. 107, 239–252. doi: 10.1111/j.1744-7348.1985.tb01567.x
Dassou, A. G., and Tixier, P. (2016). Response of pest control by generalist predators to local-scale plant diversity: a meta-analysis. Ecol. Evol. 6, 1143–1153. doi: 10.1002/ece3.1917
de Ponti, T., Rijk, B., and van Ittersum, M. K. (2012). The crop yield gap between organic and conventional agriculture. Agric. Syst. 108, 1–9. doi: 10.1016/j.agsy.2011.12.004
Debaeke, P., Munier-Jolain, N. M., Betrand, M., Guichard, L., Nolot, J. M., Faloya, V., et al. (2009). Iterative design and evaluation of rule-based cropping systems: methodology and case studies. A review. Agron. Sustain. Dev. 29, 73–86. doi: 10.1051/agro:2008050
Deytieux, V. (2017). Performance et durabilité de systèmes de grande culture en production intégrée (Ph.D. thesis). Université de Bourgogne Franche-Comté, Besançon, France.
Deytieux, V., Munier-Jolain, N., and Caneill, J. (2016). Assessing the sustainability of cropping systems in single- and multi-site studies. A review of methods. Eur. J. Agron. 72, 107–126. doi: 10.1016/j.eja.2015.10.005
Deytieux, V., Nemecek, T., Freiermuth Knuchel, R., Gaillard, G., and Munier-Jolain, N. M. (2012). Is integrated weed management efficient for reducing environmental impacts of cropping systems? A case study based on life cycle assessment. Eur. J. Agron. 36, 55–65. doi: 10.1016/j.eja.2011.08.004
Dieleman, J. A., Mortensen, D. A., and Martin, A. R. (1999). Influence of velvetleaf (Abutilon theophrasti) and common sunflower (Helianthus annuus) density variation on weed management outcomes. Weed Sci. 47, 81–89. doi: 10.1017/S004317450009069X
DiTommaso, A., Averill, K. M., Hoffmann, M. P., Fuchsberg, J. R., and Losey, J. E. (2016). Integrating insect, resistance, and floral resource management in weed control decision-making. Weed Sci. 64, 743–756. doi: 10.1614/WS-D-16-00052.1
Doohan, D., Wilson, R., Canales, E., and Parker, J. (2010). Investigating the human dimension of weed management: new tools of the trade. Weed Sci. 58, 503–510. doi: 10.1614/WS-D-09-00086.1
Fahad, S., Hussain, S., Chauhan, B. S., Saud, S., Wu, C., Hassan, S., et al. (2015). Weed growth and crop yield loss in wheat as influenced by row spacing and weed emergence times. Crop Protect. 71, 101–108. doi: 10.1016/j.cropro.2015.02.005
Fausti, S. W., Sluis, E. V. D., Qasmi, B. A., and Lundgren, J. (2014). “The effect of biotechnology and biofuels on U.S. corn belt cropping systems: updated version,” Economics Staff Paper - Department of Economics, South Dakota State University (Brookings, SD), 23.
Fickett, N. D., Boerboom, C. M., and Stoltenberg, D. E. (2013). Soybean yield loss potential associated with early-season weed competition across 64 site-years. Weed Sci. 61, 500–507. doi: 10.1614/WS-D-12-00164.1
Florez, J. A., Fischer, A. J., Ramirez, H., and Duque, M. C. (1999). Predicting rice yield losses caused by multispecies weed competition. Agron. J. 91, 87–92. doi: 10.2134/agronj1999.00021962009100010014x
Fried, G., Norton, L. R., and Reboud, X. (2008). Environmental and management factors determining weed species composition and diversity in France. Agric. Ecosyst. Environ. 128, 68–76. doi: 10.1016/j.agee.2008.05.003
Fried, G., Petit, S., Dessaint, F., and Reboud, X. (2009). Arable weed decline in Northern France: crop edges as refugia for weed conservation? Biol. Conserv. 142, 238–243. doi: 10.1016/j.biocon.2008.09.029
Fron, G. (1917). Plantes Nuisibles à L'agriculture. Encyclopédie agRicole. Paris: J. B. Baillières & Fils.
Gaba, S., Gabriel, E., Chadœuf, J., Bonneu, F., and Bretagnolle, V. (2016). Herbicides do not ensure for higher wheat yield, but eliminate rare plant species. Sci. Rep. 6:30112. doi: 10.1038/srep30112
Gabriel, D., Thies, C., and Tscharntke, T. (2005). Local diversity of arable weeds increases with landscape complexity. Perspect. Plant Ecol. Evol. Syst. 7, 85–93. doi: 10.1016/j.ppees.2005.04.001
Geiger, F., Bengtsson, J., Berendse, F., Weisser, W. W., Emmerson, M., Morales, M. B., et al. (2010). Persistent negative effects of pesticides on biodiversity and biological control potential on European farmland. Basic Appl. Ecol. 11, 97–105. doi: 10.1016/j.baae.2009.12.001
Gilbert, P.-A., Vanasse, A., and Angers, D. A. (2009). Harrowing for weed control: impacts on mineral nitrogen dynamics, soil aggregation and wheat production. Soil Tillage Res. 103, 373–380. doi: 10.1016/j.still.2008.12.001
Giuliano, S., Ryan, M. R., Véricel, G., Rametti, G., Perdrieux, F., Justes, E., et al. (2016). Low-input cropping systems to reduce input dependency and environmental impacts in maize production: a multi-criteria assessment. Eur. J. Agron. 76, 160–175. doi: 10.1016/j.eja.2015.12.016
Gutteridge, R. J., Jenkyn, J. F., and Bateman, G. L. (2006). Effects of different cultivated or weed grasses, grown as pure stands or in combination with wheat, on take-all and its suppression in subsequent wheat crops. Plant Pathol. 55, 696–704. doi: 10.1111/j.1365-3059.2006.01405.x
Hall, M. R., Swanton, C. J., and Anderson, G. W. (1992). The critical period of weed control in grain corn (Zea mays). Weed Sci. 40, 441–447. doi: 10.1017/S0043174500051882
Hammond, C. M., Luschei, E. C., Boerboom, C. M., and Nowak, P. J. (2006). Adoption of integrated pest management tactics by Wisconsin farmers. Weed Technol. 20, 756–767. doi: 10.1614/WT-05-095R1.1
Hawes, C., Squire, G. R., Hallett, P. D., Watson, C. A., and Young, M. (2010). Arable plant communities as indicator of farming practice. Agric. Ecosyst. Environ. 138, 17–26. doi: 10.1016/j.agee.2010.03.010
Hossard, L., Philibert, A., Bertrand, M., Colnenne-David, C., Debaeke, P., Munier-Jolain, N., et al. (2014). Effects of halving pesticide use on wheat production. Sci. Rep. 4:4405. doi: 10.1038/srep04405
Hugo, E., Morey, L., Saayman-Du Toit, A. E., and Reinhardt, C. F. (2014). Critical periods of weed control for naked crabgrass (Digitaria nuda), a grass weed in corn in South Africa. Weed Sci. 62, 647–656. doi: 10.1614/WS-D-13-00152.1
Jabbour, R., Gallandt, E. R., Zwickle, S., Wilson, R. S., and Doohan, D. (2014a). Organic farmer knowledge and perceptions are associated with on-farm weed seedbank densities in Northern New England. Weed Sci. 62, 338–349. doi: 10.1614/WS-D-13-00098.1
Jabbour, R., Zwickle, S., Gallandt, E. R., Mcphee, K. E., Wilson, R. S., and Doohan, D. (2014b). Mental models of organic weed management: comparison of New England US farmer and expert models. Renew. Agric. Food Syst. 29, 319–333. doi: 10.1017/S1742170513000185
Jernigan, A. B., Caldwell, B. A., Cordeau, S., DiTommaso, A., Drinkwater, L. E., Mohler, C. L., et al. (2017). Weed abundance and community composition in a long-term organic vegetable cropping systems trial. Weed Sci. 65, 639–649. doi: 10.1017/wsc.2017.33
Johnson, W. G., Gibson, K. D., and Conley, S. P. (2007). Does weed size matter? An Indiana grower perspective about weed control timing. Weed Technol. 21, 542–546. doi: 10.1614/WT-06-094R.1
Jonathan, K. S., Michael, J. H., Peterson, D. E., Randy, W. L., and Boyer, J. E. (1998). Herbicide efficacy on four amaranthus species in Soybean (Glycine max). Weed Technol. 12, 315–321. doi: 10.1017/S0890037X00043876
Kadioglu, I., Yanar, Y., and Asav, U. (2005). Allelopathic effects of weeds extracts against seed germination of some plants. J. Environ. Biol. 26, 169–173.
Kings, D. (2014). Farmers' understandings of weeds and herbicide usage as environmental influences on agricultural sustainability. J. Environ. Prot. 5:923. doi: 10.4236/jep.2014.511094
Knezevic, S. Z., and Datta, A. (2015). The critical period for weed control: revisiting data analysis. Weed Sci. 63, 188–202. doi: 10.1614/WS-D-14-00035.1
Knezevic, S. Z., Evans, S. P., Blankenship, E. E., van Acker, R. C., and Lindquist, J. L. (2002). Critical period for weed control: the concept and data analysis. Weed Sci. 50, 773–786. doi: 10.1614/0043-1745(2002)050[0773:CPFWCT]2.0.CO;2
Kropff, M. J., and Spitters, C. J. T. (1991). A simple model of crop loss by weed competition from early observations on relative leaf area of the weeds. Weed Res. 31, 97–105. doi: 10.1111/j.1365-3180.1991.tb01748.x
Kudsk, P. (2008). Optimising herbicide dose: a straightforward approach to reduce the risk of side effects of herbicides. Environmentalist 28, 49–55. doi: 10.1007/s10669-007-9041-8
Lamb, E., Shirtliffe, S., and May, W. (2011). Structural equation modeling in the plant sciences: an example using yield components in oat. Can. J. Plant Sci. 91, 603–619. doi: 10.4141/cjps2010-035
Lechenet, M., Bretagnolle, V., Bockstaller, C., Boissinot, F., Petit, M.-S., Petit, S., et al. (2014). Reconciling pesticide reduction with economic and environmental sustainability in arable farming. PLoS ONE 9:e97922. doi: 10.1371/journal.pone.0097922
Lechenet, M., Dessaint, F., Py, G., Makowski, D., and Munier-Jolain, N. (2017a). Reducing pesticide use while preserving crop productivity and profitability on arable farms. Nat. Plants 3:17008. doi: 10.1038/nplants.2017.8
Lechenet, M., Deytieux, V., Antichi, D., Aubertot, J.-N., Bàrberi, P., Bertrand, M., et al. (2017b). Diversity of methodologies to experiment integrated pest management in arable cropping systems: analysis and reflections based on a European network. Eur. J. Agron. 83, 86–99. doi: 10.1016/j.eja.2016.09.012
Liebman, M., and Gallandt, E. R. (1997). “Many little hammers: ecological management of crop-weed interactions,” in Ecology in Agriculture, ed L. E. Jackson (Davis, CA: Academic Press), 291–343.
Llewellyn, R. S., Lindner, R. K., Pannell, D. J., and Powles, S. B. (2004). Grain grower perceptions and use of integrated weed management. Aust. J. Exp. Agric. 44, 993–1001. doi: 10.1071/EA03115
Lotz, L. A. P., Christensen, S., Cloutier, D., Fernandez Quintanilla, C., Legere, A., Lemieux, C., et al. (1996). Prediction of the competitive effects of weeds on crop yields bases on the relative leaf area of weeds. Weed Res. 36, 93–101. doi: 10.1111/j.1365-3180.1996.tb01805.x
Macé, K., Morlon, P., Munier-Jolain, N., and Quéré, L. (2007). Time scales as a factor in decision-making by French farmers on weed management in annual crops. Agric. Syst. 93, 115–142. doi: 10.1016/j.agsy.2006.04.007
Mamarot, J., and Rodriguez, A. (2003). Sensibilité des Mauvaises Herbes aux Herbicides en Grandes Cultures. Paris: ACTA.
Mantle, P., Shaw, S., and Doling, D. (1977). Role of weed grasses in the etiology of ergot disease in wheat. Ann. Appl. Biol. 86, 339–351. doi: 10.1111/j.1744-7348.1977.tb01848.x
Martin, S. G., van Acker, R. C., and Friesen, L. F. (2001). Critical period of weed control in spring canola. Weed Sci. 49, 326–333. doi: 10.1614/0043-1745(2001)049[0326:CPOWCI]2.0.CO;2
Massinga, R. A., Currie, R. S., Horak, M. J., and Boyer, J. (2001). Interference of Palmer amaranth in corn. Weed Sci. 49, 202–208. doi: 10.1614/0043-1745(2001)049[0202:IOPAIC]2.0.CO;2
McDonald, A., and Riha, S. (1999). Model of crop: weed competition applied to maize: Abutilon theophrasti interactions. I. Model description and evaluation. Weed Res. 39, 355–369. doi: 10.1046/j.1365-3180.1999.00151.x
McGiffen, M. E., Masiunas, J. B., and Huck, M. G. (1992). Tomato and nightshade (Solanum nigrum L. and S. ptycanthum Dun.) effects on soil water content. J. Am. Soc. Horticult. Sci. 117, 730–735. doi: 10.21273/JASHS.117.5.730
McLeod, E. M., Banerjee, S., Bork, E. W., Hall, L. M., and Hare, D. D. (2015). Structural equation modeling reveals complex relationships in mixed forage swards. Crop Protect. 78, 106–113. doi: 10.1016/j.cropro.2015.08.019
Menalled, U. D., Bybee-Finley, K., Smith, R. G., DiTommaso, A., Pethybridge, S. J., and Ryan, M. R. (2020). Soil-mediated effects on weed-crop competition: elucidating the role of annual and perennial intercrop diversity legacies. Agronomy 10:1373. doi: 10.3390/agronomy10091373
Milberg, P., and Hallgren, E. (2004). Yield loss due to weeds in cereals and its large-scale variability in Sweden. Field Crops Res. 86, 199–209. doi: 10.1016/j.fcr.2003.08.006
Munier-Jolain, N. M., Chauvel, B., and Gasquez, J. (2002). Long-term modelling of weed control strategies: analysis of threshold-based options for weed species with contrasted competitive abilities. Weed Res. 42, 107–122. doi: 10.1046/j.1365-3180.2002.00267.x
Neumeister, L., Williamson, S., Parente, S., and Cannell, E. (2007). Pesticide uSe Reduction Strategies in Europe. Six Case Studies. London: Pesticide Action Network Europe.
Nichols, V., Verhulst, N., Cox, R., and Govaerts, B. (2015). Weed dynamics and conservation agriculture principles: a review. Field Crops Res.183, 56–68. doi: 10.1016/j.fcr.2015.07.012
O'Donovan, J. T. (1996). Weed economic thresholds: useful agronomic tool or pipe dream? Phytoprotection 77, 13–28. doi: 10.7202/706097ar
Oerke, E.-C., Dehne, H. W., Schonbeack, F., and Weber, A. (1994). Crop Production and Crop Protection. Amsterdam: Elsevier Science.
Oliver, L. R. (1988). Principles of weed threshold research. Weed Technol. 2, 398–403. doi: 10.1017/S0890037X00032152
Onofri, A., and Tei, F. (1994). Competitive ability and threshold levels of three broadleaf weed species in sunflower. Weed Res. 34, 471–479. doi: 10.1111/j.1365-3180.1994.tb02044.x
Parker, C. (2009). Observations on the current status of Orobanche and Striga problems worldwide. Pest Manag. Sci. 65, 453–459. doi: 10.1002/ps.1713
Petit, S., Cordeau, S., Chauvel, B., Bohan, D., Guillemin, J.-P., and Steinberg, C. (2018). Biodiversity-based options for arable weed management. A review. Agron. Sustain. Dev. 38:48. doi: 10.1007/s13593-018-0525-3
Petit, S., Gaba, S., Grison, A.-L., Meiss, H., Simmoneau, B., Munier-Jolain, N., et al. (2016). Landscape scale management affects weed richness but not weed abundance in winter wheat fields. Agric. Ecosyst. Environ. 223, 41–47. doi: 10.1016/j.agee.2016.02.031
Petit, S., Munier-Jolain, N., Bretagnolle, V., Bockstaller, C., Gaba, S., Cordeau, S., et al. (2015). Ecological intensification through pesticide reduction: weed control, weed biodiversity and sustainability in arable farming. Environ. Manage 56, 1078–1090. doi: 10.1007/s00267-015-0554-5
Quinio, M., De Waele, M., Dessaint, F., Biju-Duval, L., Buthiot, M., Cadet, E., et al. (2017). Separating the confounding effects of farming practices on weeds and winter wheat production using path modelling. Eur. J. Agron. 82, 134–143. doi: 10.1016/j.eja.2016.10.011
Rajcan, I., and Swanton, C. J. (2001). Understanding maize-weed competition: resource competition, light quality and the whole plant. Field Crops Res. 71, 139–150. doi: 10.1016/S0378-4290(01)00159-9
Rasmussen, J., Nielsen, H. H., and Gundersen, H. (2009). Tolerance and selectivity of cereal species and cultivars to postemergence weed harrowing. Weed Sci. 57, 338–345. doi: 10.1614/WS-08-109.1
Riar, D. S., Norsworthy, J. K., Steckel, L. E., Stephenson, D. O., Eubank, T. W., Bond, J., et al. (2013). Adoption of best management practices for herbicide-resistant weeds in midsouthern United States cotton, rice, and soybean. Weed Technol. 27, 788–797. doi: 10.1614/WT-D-13-00087.1
Ridier, A., Ghali, M. B. E., Nguyen, G., and Kephaliacos, C. (2013). The role of risk aversion and labor constraints in the adoption of low input practices supported by the CAP green payments in cash crop farms. Rev. Étud. Agric Environ. 94, 195–219. doi: 10.4074/S1966960713012034
Rioux, R. (1994). Influence de la fumure azotée sur la compétition entre le chiendent et l'orge. Phytoprotection 65, 61–64.
Robinson, R. A., and Sutherland, W. J. (2002). Post-war changes in arable farming and biodiversity in Great Britain. J. Appl. Ecol. 39, 157–176. doi: 10.1046/j.1365-2664.2002.00695.x
Romdhane, S., Spor, A., Busset, H., Falchetto, L., Martin, J., Bizouard, F., et al. (2019). Cover crop management practices rather than the composition of cover crop mixtures affect microbial communities in no-till agroecosystems. Front. Microbiol. 10:1618. doi: 10.3389/fmicb.2019.01618
Rydberg, N. T., and Milberg, P. (2000). A survey of weeds in organic farming in Sweden. Biol. Agric. Horticult. 18, 175–185. doi: 10.1080/01448765.2000.9754878
Salonen, J. (1992). Efficacy of reduced herbicide doses in spring cereals of different competitive ability. Weed Res. 32, 483–491. doi: 10.1111/j.1365-3180.1992.tb01909.x
Schumacher, W. (1987). Measures taken to preserve arable weeds and their associated communities in central Europe. Monogr. Br. Crop Protect. Council 109–112.
Seifert, C., Leuschner, C., and Culmsee, H. (2015). Arable plant diversity on conventional cropland—the role of crop species, management and environment. Agric. Ecosyst. Environ. 213, 151–163. doi: 10.1016/j.agee.2015.07.017
Seufert, V., Ramankutty, N., and Foley, J. A. (2012). Comparing the yields of organic and conventional agriculture. Nature 485, 229–U113. doi: 10.1038/nature11069
Silva, J. V., Reidsma, P., Laborte, A. G., and van Ittersum, M. K. (2017). Explaining rice yields and yield gaps in Central Luzon, Philippines: an application of stochastic frontier analysis and crop modelling. Eur. J. Agron. 82, 223–241. doi: 10.1016/j.eja.2016.06.017
Smith, R. G., Davis, A. S., Jordan, N. R., Atwood, L. W., Daly, A. B., Grandy, A. S., et al. (2014). Structural equation modeling facilitates transdisciplinary research on agriculture and climate change. Crop Sci. 54, 475–483. doi: 10.2135/cropsci2013.07.0474
Smith, R. G., Mortensen, D. A., and Ryan, M. R. (2010). A new hypothesis for the functional role of diversity in mediating resource pools and weed–crop competition in agroecosystems. Weed Res. 50, 37–48. doi: 10.1111/j.1365-3180.2009.00745.x
Soltani, N., Dille, J. A., Burke, I. C., Everman, W. J., Vangessel, M. J., Davis, V. M., et al. (2016). Potential corn yield losses from weeds in North America. Weed Technol. 30, 979–984. doi: 10.1614/WT-D-16-00046.1
Song, J.-S., Kim, J.-W., Im, J.-H., Lee, K.-J., Lee, B.-W., and Kim, D.-S. (2017). The effects of single- and multiple-weed interference on soybean yield in the far-Eastern region of Russia. Weed Sci. 65, 371–380. doi: 10.1017/wsc.2016.25
Stoate, C., Baldi, A., Beja, P., Boatman, N. D., Herzon, I., van Doorn, A., et al. (2009). Ecological impacts of early 21st century agricultural change in Europe - a review. J. Environ. Manage 91, 22–46. doi: 10.1016/j.jenvman.2009.07.005
Storkey, J., and Neve, P. (2018). What good is weed diversity? Weed Res. 58, 239–243. doi: 10.1111/wre.12310
Stougaard, R. N., Maxwell, B. D., and Harris, J. D. (1997). Influence of application timing on the efficacy of reduced rate postemergence herbicides for wild oat (Avena fatua) control in spring barley (Hordeum vulgare). Weed Technol. 11, 283–289. doi: 10.1017/S0890037X00042962
Streibig, J. (1980). Models for curve-fitting herbicide dose response data. Acta Agric. Scand. 30, 59–64. doi: 10.1080/00015128009435696
Subedi, K. D., and Ma, B. L. (2009). Assessment of some major yield-limiting factors on maize production in a humid temperate environment. Field Crops Res. 110, 21–26. doi: 10.1016/j.fcr.2008.06.013
Swanton, C. J., Nkoa, R., and Blackshaw, R. E. (2015). Experimental methods for crop–weed competition studies. Weed Sci. 63, 2–11. doi: 10.1614/WS-D-13-00062.1
Swanton, C. J., Weaver, S., Cowan, P., Acker, R. V., Deen, W., and Shrestha, A. (1999). Weed thresholds: theory and applicability. J. Crop Product. 2, 9–29. doi: 10.1300/J144v02n01_02
Swanton, C. J., and Weise, S. F. (1991). Integrated weed management - the rationale and approach. Weed Technol. 5, 657–663. doi: 10.1017/S0890037X00027512
Swinton, S. M., Buhler, D. D., Forcella, F., Gunsolus, J. L., and King, R. P. (1994). Estimation of crop yield loss due to interference by multiple weed species. Weed Sci. 42, 103–109. doi: 10.1017/S0043174500084241
Teasdale, J. R., and Cavigelli, M. A. (2010). Subplots facilitate assessment of corn yield losses from weed competition in a long-term systems experiment. Agron. Sustain. Dev. 30, 445–453. doi: 10.1051/agro/2009048
Teyker, R., Hoelzer, H., and Liebl, R. (1991). Maize and pigweed response to nitrogen supply and form. Plant Soil 135, 287–292. doi: 10.1007/BF00010918
Trichard, A., Alignier, A., Chauvel, B., and Petit, S. (2013). Identification of weed community traits response to conservation agriculture. Agric. Ecosyst. Environ. 179, 179–186. doi: 10.1016/j.agee.2013.08.012
Trigo, E. J., and Cap, E. J. (2003). The impact of the introduction of transgenic crops in Argentinean agriculture. AgBioForum 6, 87–94.
Valantin-Morison, M., and Meynard, J. M. (2008). Diagnosis of limiting factors of organic oilseed rape yield. A survey of farmers' fields. Agron. Sustain. Dev. 8, 527–539. doi: 10.1051/agro:2008026
van Acker, R. C., Lutman, P. J. W., and Froud-Williams, R. J. (1997). Predicting yield loss due to interference from two weed species using early observations of relative weed leaf area. Weed Res. 37, 287–299. doi: 10.1046/j.1365-3180.1997.d01-52.x
van Ittersum, M. K., Leffelaar, P. A., van Keulen, H., Kropff, M. J., Bastiaans, L., and Goudriaan, J. (2003). On approaches and applications of the Wageningen crop models. Eur. J. Agron. 18, 201–234. doi: 10.1016/S1161-0301(02)00106-5
Waggoner, J., Henneberger, P., Kullman, G., Umbach, D., Kamel, F., Beane Freeman, L., et al. (2013). Pesticide use and fatal injury among farmers in the agricultural health study. Int. Arch. Occup. Environ. Health 86, 177–187. doi: 10.1007/s00420-012-0752-x
Wilson, R. S., Tucker, M. A., Hooker, N. H., Lejeune, J. T., and Doohan, D. (2008). Perceptions and beliefs about weed management: perspectives of Ohio grain and produce farmers. Weed Technol. 22, 339–350. doi: 10.1614/WT-07-143.1
Wossink, G., de Buck, A., van Niejenhuis, J., and Haverkamp, H. (1997). Farmer perceptions of weed control techniques in sugarbeet. Agric. Syst. 55, 409–423. doi: 10.1016/S0308-521X(96)00097-2
Yvoz, S., Petit, S., Biju-Duval, L., and Cordeau, S. (2020). A framework to type crop management strategies within a production situation to improve the comprehension of weed communities. Eur. J. Agron. 115:126009. doi: 10.1016/j.eja.2020.126009
Keywords: weed-crop interference, cropping system, yield gap, crop loss, weeding, herbicide, trophic resource use, weed management
Citation: Colbach N, Petit S, Chauvel B, Deytieux V, Lechenet M, Munier-Jolain N and Cordeau S (2020) The Pitfalls of Relating Weeds, Herbicide Use, and Crop Yield: Don't Fall Into the Trap! A Critical Review. Front. Agron. 2:615470. doi: 10.3389/fagro.2020.615470
Received: 09 October 2020; Accepted: 07 December 2020;
Published: 23 December 2020.
Edited by:
Bhagirath Singh Chauhan, The University of Queensland, AustraliaReviewed by:
Simerjeet Kaur, Punjab Agricultural University, IndiaIlias Travlos, Agricultural University of Athens, Greece
Copyright © 2020 Colbach, Petit, Chauvel, Deytieux, Lechenet, Munier-Jolain and Cordeau. This is an open-access article distributed under the terms of the Creative Commons Attribution License (CC BY). The use, distribution or reproduction in other forums is permitted, provided the original author(s) and the copyright owner(s) are credited and that the original publication in this journal is cited, in accordance with accepted academic practice. No use, distribution or reproduction is permitted which does not comply with these terms.
*Correspondence: Stéphane Cordeau, stephane.cordeau@inrae.fr
†ORCID: Nathalie Colbach orcid.org/0000-0002-3791-037X
Sandrine Petit orcid.org/0000-0001-8781-8873
Bruno Chauvel orcid.org/0000-0001-8891-8817
Nicolas Munier-Jolain orcid.org/0000-0002-0871-3692
Stéphane Cordeau orcid.org/0000-0003-1069-8388