- 1Department of Soil System Science, Helmholtz Centre for Environmental Research – UFZ, Halle, Germany
- 2Soil Science, Martin-Luther-University Halle-Wittenberg, Halle, Germany
- 3Soil Physics, Bayreuth Center of Ecology and Environmental Research (BayCEER), University of Bayreuth, Bayreuth, Germany
- 4Chair of Soil Science, TUM School of Life Sciences Weihenstephan, Technical University of Munich, Freising, Germany
- 5Institute for Advanced Study, Technical University of Munich, Garching, Germany
- 6Leibnitz Institute of Plant Genetics and Crop Plant Research – IPK, Metalloid Transport, Gatersleben, Germany
- 7Institute for Epidemiology and Plant Pathogen Diagnostics, Julius-Kühn Institut, Braunschweig, Germany
- 8Department of Forest and Soil Science, Institute of Soil Research, University of Natural Resources and Life Sciences, Tulln, Austria
- 9Institute for Bio- and Geosciences – IBG-3: Agrosphere, Forschungszentrum Jülich GmbH, Jülich, Germany
- 10Department of Ecological Modelling, Helmholtz Centre for Environmental Research – UFZ, Leipzig, Germany
- 11Department of Soil Ecology, Helmholtz Centre for Environmental Research – UFZ, Halle, Germany
- 12German Centre for Integrative Biodiversity Research (iDiv) Halle-Jena-Leipzig, Leipzig, Germany
Resilience of soils, i.e., their ability to maintain functions or recover after disturbance, is closely linked to the root-soil interface, the soil's power house. However, the limited observability of key processes at the root-soil interface has so far limited our understanding of how such resilience emerges. Here, we hypothesize that resilience emerges from self-organized spatiotemporal patterns which are the result of complex and dynamic feedbacks between physical, chemical, and biological processes occurring in the rhizosphere. We propose that the combination of modern experimental and modeling techniques, with a focus on imaging approaches, allows for understanding the complex feedbacks between plant resource acquisition, microbiome-related plant health, soil carbon sequestration, and soil structure development. A prerequisite for the identification of patterns, underlying processes, and feedback loops is that joint experimental platforms are defined and investigated in their true 2D and 3D geometry along time. This applies across different scientific disciplines from soil physics/chemistry/microbiology to plant genomics/physiology and across different scales from the nano/microscopic scale of the root soil interface, over the radial profiles around single roots, up to the root architecture and plant scale. Thus, we can move beyond isolated reductionist approaches which have dominated in rhizosphere research so far.
Motivation
This review paper is motivated by the need to bring together the different aspects, processes, and scales of rhizosphere research under a common framework in order to improve our understanding of soil and rhizosphere functions, their stability under disturbances and change (resilience), and their role for robust functioning of agricultural systems. In the future, this will facilitate a more informed management of agricultural systems actively considering rhizosphere processes.
The main knowledge gaps in rhizosphere research are related to the difficulty in mechanistically linking the physical, chemical, and biological processes taking place at different spatial and temporal scales in the rhizosphere (nm to cm and minutes to months) and then upscaling them to the root system and the soil profile (Figure 1). The key for overcoming these knowledge gaps is to link the spatial arrangement of the different interconnected components of the rhizosphere and their temporal dynamics (Roose et al., 2016). We propose that this challenge can be successfully tackled by applying tools and principles of self-organization. In the following we: (1) give a short introduction to rhizosphere processes, (2) introduce the principles and tools of self-organization from an ecosystem perspective, and show how these can be applied to the rhizosphere. We (3) formulate a number of testable hypotheses based on these principles. Furthermore, we: (4) identify a number of focal topics for the application of this new approach, (5) highlight analytical developments with the potential to measure “below-ground” patterns, and (6) outline how such data can be merged and interpreted using a pipeline of image analyses, spatial correlation, interpolation, and modeling. Finally, we (7) call upon the soil science community for action, providing one example, and end with (8) the vision about what to achieve in the future.
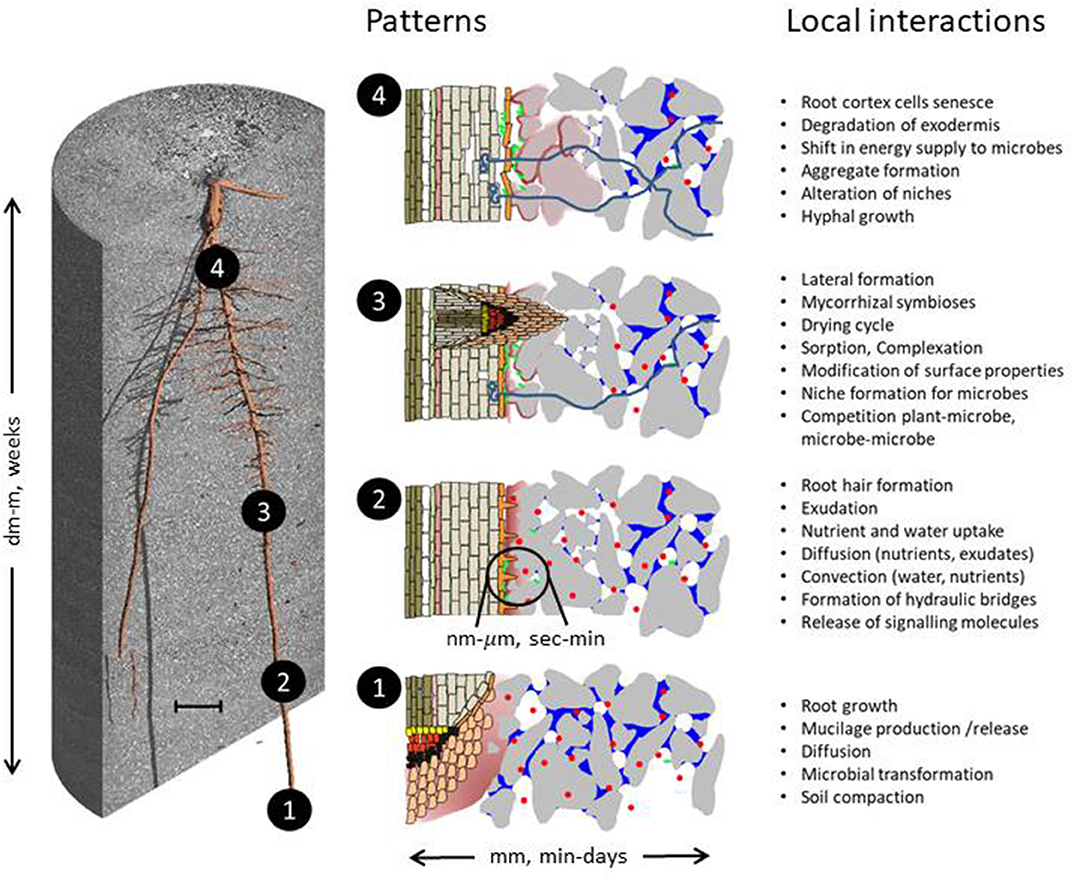
Figure 1. Hypothetical patterns reflecting rhizosphere self-organization based on numerous feedback loops resulting from local interaction of lower-level components. Illustrated are mucilage (rose) and water distribution (blue), compaction of soil particles (gray) and aggregate formation (gray-rose), colonies of microorganisms (green), depletion of phosphorous (red) and their potential change with root ontogeny. The latter is illustrated on the left for a 7-day old Zea mays root system growing in loam (scale bar 10 mm). Note that the patterns are not to scale. Local interactions/mechanisms are sorted according to their potential relevance at a certain developmental stage—not suggesting that they are absent at other stages. The integration of patterns for the whole root system is expected to determine emergent properties like water and nutrient acquisition, soil structure, and carbon storage as well as plant health.
Brief Introduction to Rhizosphere Processes
Life on earth is sustained by the small volume of soil surrounding roots, the rhizosphere (Hinsinger et al., 2009). Indeed, all substances taken up from and released into the soil have to cross the rhizosphere and their flow, transport, and reactions depend on rhizosphere-specific and time-dependent properties. The rhizosphere is where myriad biophysical and biochemical processes occur in parallel sustaining all other trophic levels in the biosphere. As roots forage for resources, nutrients are solubilized and redistributed between organic and inorganic pools, mediated by the soil microbial community, and protozoa and invertebrates grazing across trophic levels. The functioning of the rhizosphere has major implications for food and fiber production and related acquisition of resources (York et al., 2016), as well as for climate change with regards to carbon sequestration (Keiluweit et al., 2015). In the rhizosphere, roots and microorganisms shape and organize the soil physical (pore volume, connectivity, and aggregation) and biogenic structure (surface coatings, mineral associated carbon), which at the soil profile scale control infiltration, water storage, and aeration. The rhizosphere microbiome, impacted by the plant species and soil type, is fundamental for plant health and matter turnover (Berg and Smalla, 2009; Philippot et al., 2013; Berg et al., 2017). Despite its central importance to all life, we still know little about rhizosphere functioning and about how we can encourage rhizosphere health to create robust and sustainable agricultural production (Hinsinger et al., 2009).
As roots grow (Figure 1), root tips displace soil particles and mechanical impedance is alleviated by the lubrication effect of shed root border cells and secreted mucilage, a viscous substance with high water holding capacity consisting of polysaccharides, proteins, and lipids. The apical meristem in root tips provides new cells for the growing root. As root tissues differentiate along the longitudinal root axis in the elongation and root hair zone, uptake of water and nutrients and release and reabsorption of a large range of organic substances (consisting of organic acid anions, sugars, amino acids, enzymes, complexing agents like phytosiderophores but also volatiles like ethylene which act as plant hormones, etc.) are initiated (Jones et al., 2009). Due to root and microbial respiration, O2 is consumed and CO2 is released. The cylindrical geometry of roots induces (additional) non-linearity to the radial concentration profiles of different rhizosphere components and thus affects the gradients that drive flow and transport in the rhizosphere, which will be discussed in detail below (sections Rhizosphere Self-Organization Patterns–Spatial and Rhizosphere Self-Organization Patterns–Temporal). Further determining factors are soil chemical properties (sorption sites on the surface of soil particles), pore size distribution (strongly related to texture), and soil moisture as well as the plants' uptake capacity. The width of the rhizosphere is not constant: the impact of root activities on highly mobile substances like water or nitrate can be detected several centimeters from the root surface while for elements with low mobility like phosphorus or more complex organic molecules a concentration gradient toward the root surface is only found over distances of a few mm or even only a few μm (Kuzyakov and Razavi, 2019). For all transport processes in the soil, water content is of prime importance as it determines the connectivity and transport properties (i.e., unsaturated hydraulic conductivity and diffusion coefficients) in both the liquid as well as the gas phase within a given pore system.
The release of organic compounds stimulates the growth of microorganisms and creates microbial habitats that differ from the bulk soil in the availability of carbon sources in general, but also in the availability of specific substrates that can only be metabolized by certain microbial functional groups. As a result, the rhizosphere microbiome differs from that of the bulk soil (i.e., soil not influenced by plant roots) and is specific for certain plant species or even genotypes (Berg and Smalla, 2009; Philippot et al., 2013; Reinhold-Hurek et al., 2015). Changes of the microbial abundance and composition are primarily reported for the root surface and the soil particles adhering to roots (Schmidt et al., 2018). Their spatial and temporal gradients have hardly been investigated because of methodological constraints. Modeling microbial establishment on the surfaces of growing roots indicated the importance of root growth kinematics (Dupuy and Silk, 2016). These root-induced biogeochemical changes in soil and their numerous feedback loops are not constant over time. Roots adapt their metabolic activity to the actual availability of resources. As individual root segments age, the formation of new laterals from these root segments occurs (Vetterlein and Doussan, 2016). Therefore, during root growth an adjacent soil particle experiences biogeochemical change as the root tip develops into an older root segment (Figure 1).
Previously, rhizosphere size was defined operationally, i.e., by the sampling method (soil adhering to root; slicing of soil with increasing distance from the root mat, positioning of micro-samplers at the root surface, and at distance). However, following the definition of Darrah (1991), the rhizosphere reaches as far as any change caused by the root in bulk soil can be detected.
Self-Organization to Overcome Disciplinary Fragmentation of the Research Field and Gain New, Comprehensive Insights
In the past, reductionist approaches have dominated in rhizosphere research-i.e., the individual components (soil, roots, microorganisms, chemicals) have been investigated in isolation or in simplified model approaches. For the study of a complex system like the rhizosphere this was the best the science community could do at the time; however, disciplinary fragmentation tends to coincide with limited scientific progress (Balietti et al., 2015), and the complex process interactions and their effects on rhizosphere and the soil profile cannot be elaborated by a reductionist approach. But, it is a major challenge to initiate and successfully maintain interdisciplinary research (Ferlian et al., 2018). There are major differences in e.g., concepts and terminologies of the different disciplines, allowed experimental designs and the levels of replication. We propose that future research should be structured in the framework of self-organization. The principles and tools of the self-organization allow investigating process interactions and their relation to rhizosphere functions. Relevant processes and interactions are illustrated in Figure 2.
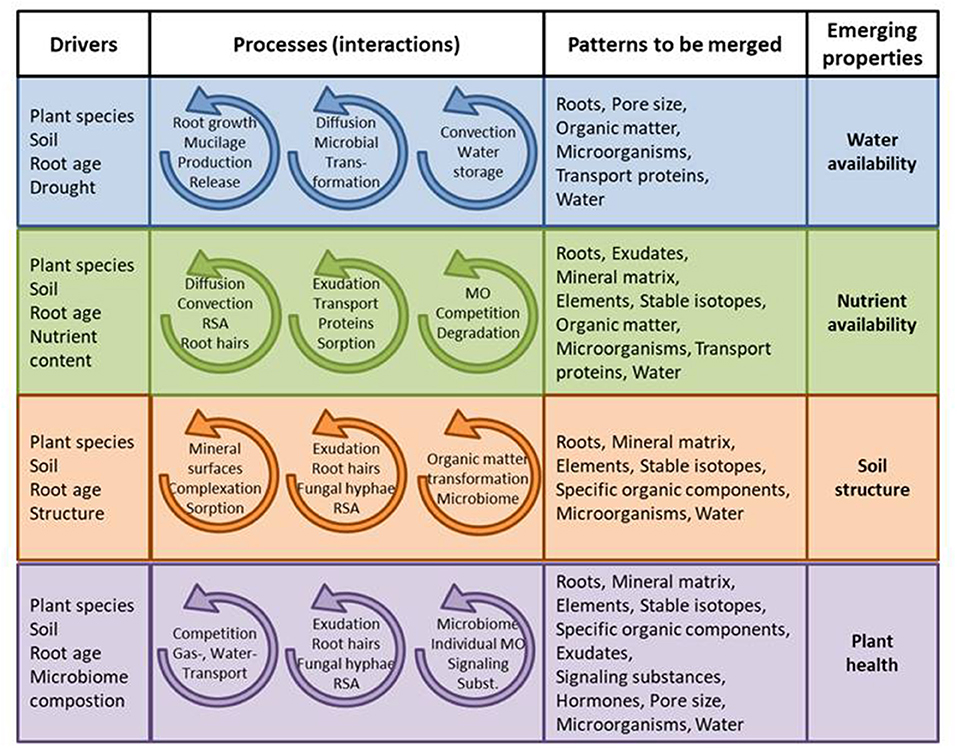
Figure 2. Examples for applying the concept of self-organization to facilitate interdisciplinary research. Including qualitative and quantitative information for the parameter in question [abundance, amount, age, chemical composition, microorganisms (MO), root system architecture (RSA), size, surface properties, expression, activity].
“Self-organization is a process in which a pattern at the global level of a system emerges solely from numerous interactions among the lower-level components of the system. Moreover, the rules specifying interactions among the system's components are executed using only local information, without reference to the global pattern. In short, the pattern is an emergent property of the system rather than a property imposed on the system by an external ordering influence. [….] Emergent properties are features of a system that arise unexpectedly from interactions among the system's components. An emergent property cannot be understood simply by examining in isolation the properties of the system's components, but requires a consideration of the interactions among the system's components. It is important to point out that system components do not necessarily have to interact directly. [….] Individuals may interact indirectly if the behavior of one individual modifies the environment and thus affects the behavior of other individuals” [quoted from Camazine et al. (2003), page 8]. The terms used in this definition with respect to rhizosphere research are explained in Table 1. The concept self-organization thus refers to a broad range of pattern-formation processes in physical, chemical, and biological multi-component systems. It is distinguished from the concept self-assembly by the energy required to maintain order in a self-organized system.
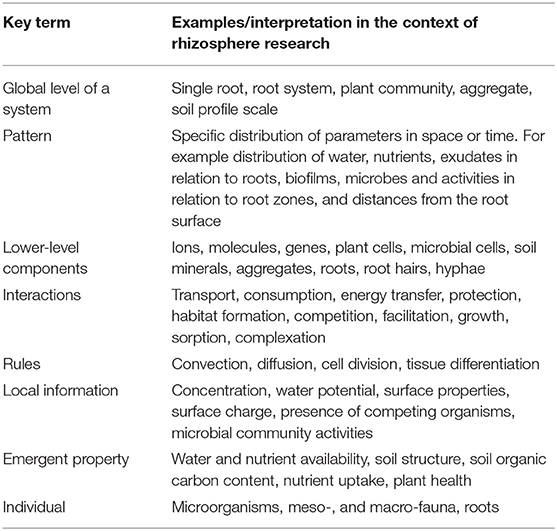
Table 1. Key terms related to principles and tools of self-organization, referring to the definition by Camazine et al. (2003).
To our knowledge, the self-organization concept has not been applied to the rhizosphere although it has been discussed for soils (Phillips, 1998; Young and Crawford, 2004; Lavelle et al., 2016), soil-microbe complexes (Young and Crawford, 2004), mycorrhizal networks (Simard, 2009), microbial biofilms (Hansen et al., 2007), assembly of biomolecules and cells (Hafner et al., 2019), root development (Leyser, 2011), plant community and landscape formation (Schwarz et al., 2018). We extend the concept to the rhizosphere (Figures 1, 2), for the following reasons: (1) It applies to individual subgroups of rhizosphere components (e.g., organic polymers, root cells, microorganisms). (2) The temporal development of radial biogeochemical gradients extending from the root surface into the soil cannot be predicted from studying the system components in isolation. (3) Recent advances in analytical methods enable the measurements of patterns at the relevant scales (Table 2). (4) The concept of self-organization, which builds on cascades of local interactions (Figures 1, 2), provides a framework for addressing the interdisciplinary and multiscale nature of the rhizosphere and unraveling how local component interactions result in macroscopic properties to be used in continuous models. (5) Self-organized systems show a strong resilience toward disturbance as the emerging properties result from a multitude of local interactions (Camazine et al., 2003; Biggs et al., 2012).
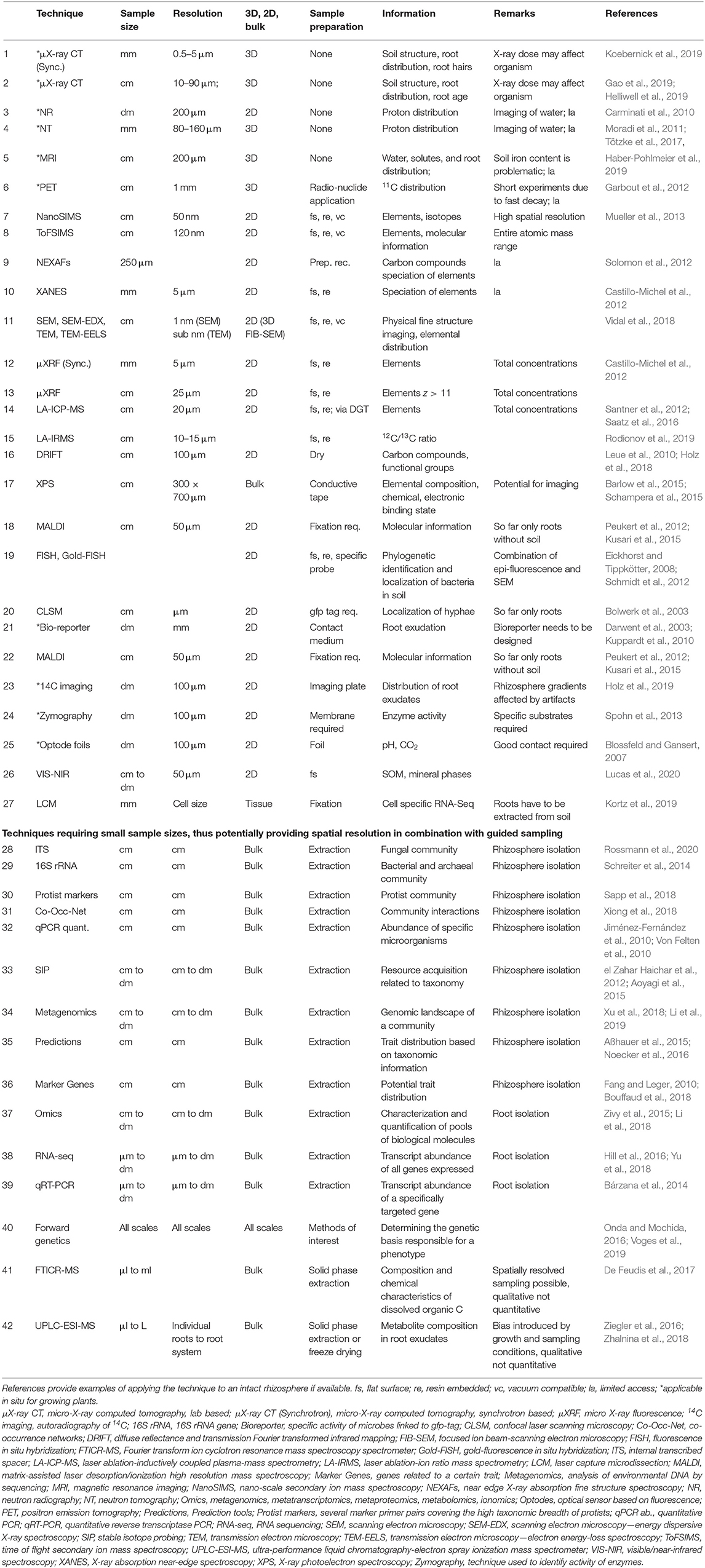
Table 2. Methods suitable or with potential for analyses of rhizosphere spatial patterns and their dynamic in time.
The last two decades have seen a segregation of research into “soil-related” investigations conducted in soil, “soil-free” approaches focusing on gene expression and membrane transport processes in roots cultivated in hydroponics, “fertilized” solidified gel plates, and pedological descriptions of rhizosphere impact on soils (Fimmen et al., 2008; Schulz et al., 2016). In addition, microbiome-related research of the rhizosphere has flourished, generally neglecting spatial structure and heterogeneity, whereas abiotic-oriented research focuses on spatial aspects but neglects or simplifies the contribution of the microbiome for turnover of substances—many of them relevant for structure formation and surface properties of the mineral matrix (Rillig et al., 2017). We propose that all those different disciplines must be brought together for a system approach following the principles of self-organization. In fact, water and nutrient availability, plant health and soil structure are prominent examples of emerging properties due to the self-organization of biotic and abiotic agents in the rhizosphere (Figure 2). These processes are meticulously studied in their respective scientific disciplines. However, rhizosphere research needs collaborative experiments in which several of these emerging properties are investigated jointly.
Rhizosphere Self-Organization Patterns – Spatial
Pattern formation in the rhizosphere (Figures 1, 3A, 4, 5) is driven by: (1) radial transport to and from the root surface, (2) temporal changes due to root growth, (3) diurnal variation of rhizosphere variables (water potential gradients, carbohydrate availability, activity of metabolic processes in roots), and (4) functional changes with root ontogeny (cell and tissue functionality, morphological and anatomical changes). In addition, some of the components in the rhizosphere are organized based on communication (calling distance) or they strictly follow chemical principles (amphiphilic substances).
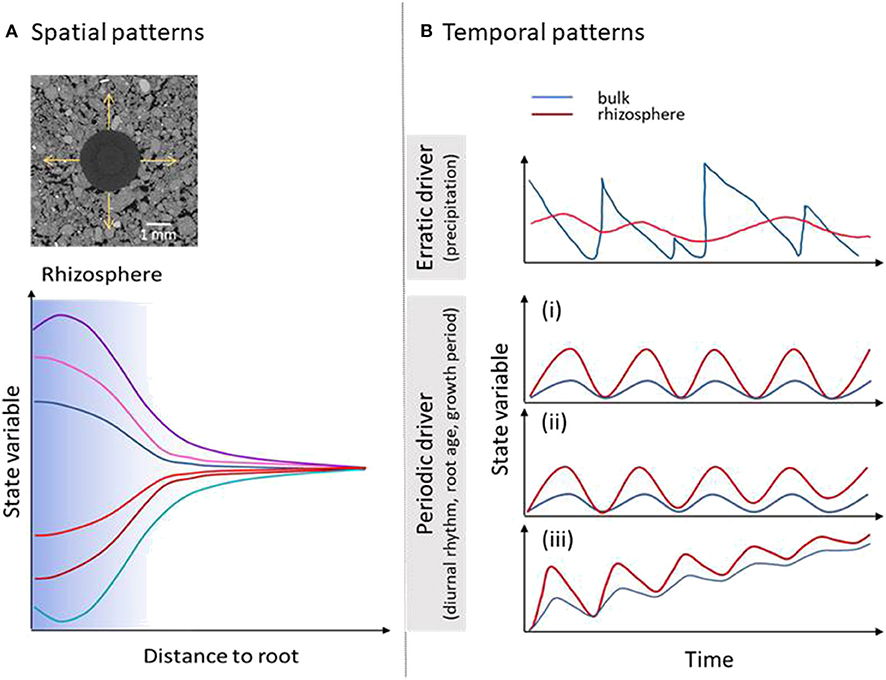
Figure 3. Hypothetical rhizosphere patterns. (A) State variables increase or decrease with increasing distance from the root surface. In specific cases the minimum or maximum is found at some distance from the root surface. Shaded area represents the width of the rhizosphere defined as the distance at which the state variable is Cbulk+5% of Cmax, Cbulk-5% of Cmin, respectively, in accordance with de Parseval et al. (2017). Please note that the width of the rhizosphere is dependent on the process in question, ranging from several μm to mm. (B) State variables fluctuate in time. For a number of processes oscillations are expected to be larger in the rhizosphere, i.e., due to day-night cycle, changes of root activity with age and time during growth period. For state variables strongly affected by erratic external drivers like the soil water content the opposite can be found, i.e., oscillations are buffered by specific rhizosphere properties like mucilage concentration. For periodic drivers three scenarios are hypothesized: (i) state variable returns to bulk soil value having no temporal trend. (ii) State variable in the rhizosphere does not return to initial bulk soil value, while bulk soil value shows no temporal trend. (iii) State variable in rhizosphere and bulk soil both change over time approaching new equilibrium. For simplicity all hypothetical examples for periodic drivers show state variable in the rhizosphere being higher than in the bulk soil, for all cases mirror images could have been drawn as well.
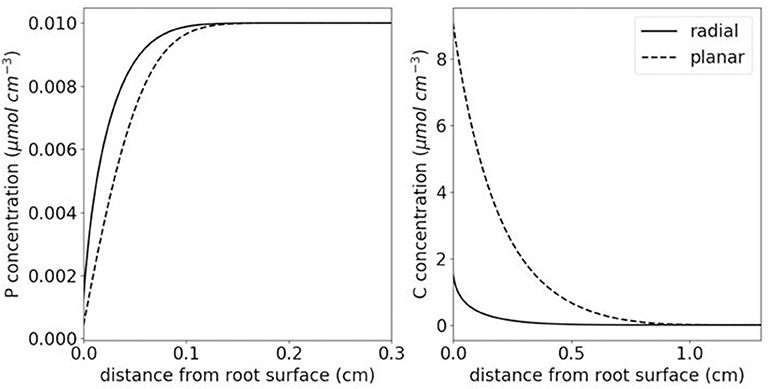
Figure 4. Differences in the shape of spatial concentration profiles for P and citrate for radial vs. planar geometry. In order to simulate either root P uptake or root citrate exudation, the 1D diffusion-reaction equation was solved in either planar or radial coordinates while all other parameters stayed the same. The root radius in the radial case was taken to be 0.01 cm. The domain was 5 cm wide, i.e., not taking into account neighboring root effects, with zero-flux outer boundary condition. Sorption was described by a Langmuir isotherm (maximal sorption capacity; affinity constant: 500 and 15 cm3 μmol−1; 2 and 1.5 cm3 μmol−1 for P and citrate, respectively), P uptake was described by Michaelis Menten kinetics (maximal influx; Michaelis Menten constant: 2.72 × 10−6 μmol cm−2 s−1; 7.17 × 10−3 μmol cm−3), citrate exudation was described by a constant rate of 3.00 × 10−6 μmol cm−2 s−1, the effective diffusion coefficient De in porous medium was taken to be De = Dlfθ (Dl: 6.9 × 10−6 and 1.00 × 10−5 cm2 s−1 for P and citrate, respectively; θ: 0.3 cm3 cm−3; f: 0.3 cm cm−1). The initial concentration of P in soil solution was assumed to be 1.00 × 10−2 μmol cm−3.
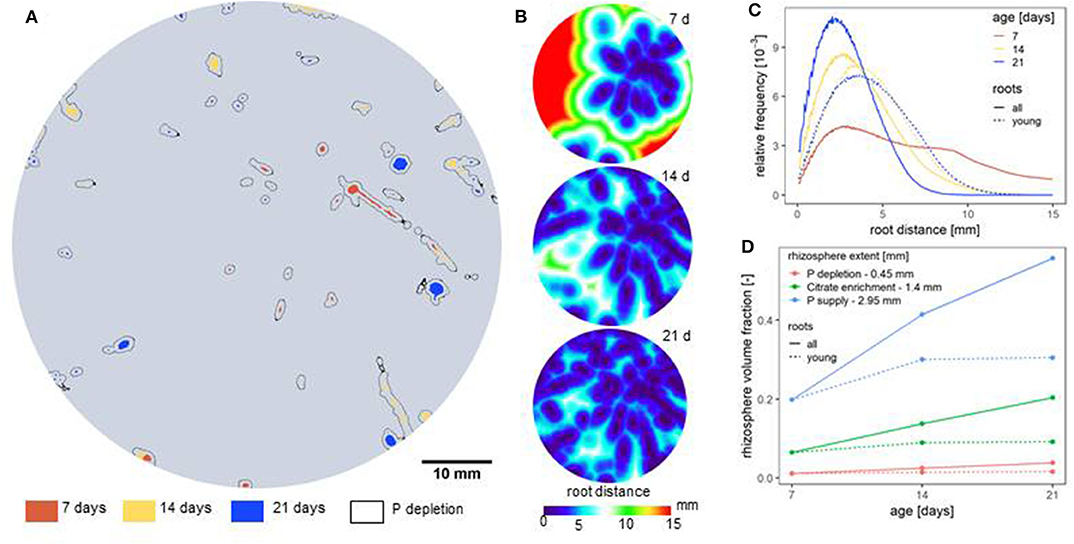
Figure 5. (A) Root distribution within a 2D cross section at 10 cm depth through a soil column planted with Zea mays. Roots of different age are distinguished by color. Root age was derived by registration of X-ray CT scans from different time points to each other. (B) Euclidean distance maps based on 3D X-ray CT scans for the cross section in (A); distance maps are provided for three time points, i.e., 7, 14, and 21 days after planting. (C) Frequency distributions of root distances in soil at three time points. Continuous lines refer to all roots present at the respective time point, dotted lines refer to young roots only for the hypothetical case, that only those are active. (D) Based on distance maps the rhizosphere volume was calculated for rhizosphere width defined in de Parseval et al. (2017) for P depletion, citrate enrichment and the P supply zone. Depending on the state variable and time point rhizosphere soil fraction accounted for 1–50% of total volume.
Hypothetical spatial patterns are summarized in Figure 3A. State variables increase or decrease with increasing distance from the root surface. In specific cases, like porosity (Koebernick et al., 2019; Lucas et al., 2019a) and P depletion (Kirk et al., 1999; de Parseval et al., 2017), the minimum or maximum is found at some distance from the root surface. The width of the rhizosphere depends on the process in question, ranging from several μm up to mm. It can be defined as a relative difference in respect to bulk soil values. Spatial patterns may also occur along the root axis, as it has been shown for proton release, enzyme activity, and nutrient uptake (Hinsinger et al., 2009; Spohn and Kuzyakov, 2014).
Information on parameter patterns currently suffers from being derived from systems not properly reflecting radial geometry in 2D or 3D (Figure 4). Compartment systems are linearized systems based on a root mat along a surface and are not suitable to reflect the radial geometry of rhizosphere processes and related gradients (Roose et al., 2016). In rhizoboxes, roots grow against and along a transparent plane, which may cause artifacts in gas and water transport and altered root growth (branching, root hair formation). While the effect of the radial geometry can be accounted for in models (Figure 4), it has rarely been considered experimentally, mainly due to the lack of suitable techniques. As illustrated in Figure 4, not accounting for the radial geometry of the rhizosphere in planar experimental setups leads to an overestimation of nutrient depletion zone and the concentration of released organic compounds as a function of distance from the root surface.
The most common sampling technique, in particular in soil microbiology, is the operational definition of rhizosphere soil as the fraction of soil adhering to the complete root system after shaking the uprooted plant, ignoring spatiotemporal differences (Berg and Smalla, 2009). The amount of soil adhering depends on soil texture, moisture, root age, plant species, and plants' physiological condition. Spatial dimensions of the extent of the rhizosphere can only be estimated. Few unbiased images of the rhizosphere, i.e., of roots in their original spatial context in the soil matrix, have been obtained; for resin embedded soil samples in the 1960's and 1970's by electron microscopy (Roose et al., 2016) and for frozen samples by Watt et al. (2006). Only with the methodological advances in the last decade, we have now access to parameter patterns. X-ray computed tomography (X-ray CT) can be used in situ in the soil for visualization of roots at varying scales, from root system scale (Koebernick et al., 2014) to the scale of root hairs (Keyes et al., 2013). From such data, 3D information of soil structure, root age and distribution, root distances, bulk density, and root-soil contact (Carminati et al., 2013; Koebernick et al., 2018) can be derived (Figure 5). While non-invasive methods overcome the problems associated with the opaque nature of soil, they all show a trade-off between field of view and spatial resolution (Table 2). However, for rhizosphere research both the cm- as well as the submicron scales are relevant.
Rhizosphere Self - Organization Patterns – Temporal
From past studies, we have fragmented knowledge on how system components and some of the emerging properties change with the age of the system (Vetterlein and Doussan, 2016). However, we lack a clear understanding of temporal patterns and their function. State variables fluctuate in time; for instance, the soil water content depends, among other factors, on erratic variables such as precipitation (Figure 3B). For a number of processes oscillations are expected to be larger in the rhizosphere, i.e., due to the day-night cycle, root growth, and root development. For state variables strongly affected by erratic external drivers like water content the opposite can be found, i.e., oscillations are buffered by specific rhizosphere properties like mucilage concentration (Carminati and Vetterlein, 2012). For periodic drivers, three scenarios are hypothesized; (i) state variable returns to bulk soil value having no temporal trend—such behavior has been observed for diurnal oscillation of rhizosphere pH value (Blossfeld et al., 2010). (ii) state variable in the rhizosphere does not return to initial bulk soil value, while bulk soil value shows no temporal trend—such behavior can be hypothesized for accumulation of elements in the rhizosphere like Ca which has been found to precipitate as CaSO4 or CaCO3 (Jaillard et al., 1991). (iii) state variable in rhizosphere and bulk soil both change over time approaching equilibrium—this is hypothesized for soil C content (Poirier et al., 2018) and soil structure (Lucas et al., 2019b).
Like spatial patterns, temporal patterns are observed at different scales. Within a period of several weeks, individual root segments undergo ontogenesis (Figure 1), changing from the state of meristematic cells within the root tip, to root elongation zone, to root hair formation, to mature roots with well-differentiated exo- and endodermis, to roots with intact vascular tissue but already senescing root cortex. Along with ontogenesis, there are functional changes related to the amount and quality of substances released, pathways, and capacity for uptake as well as their capacity for direct interaction with the microbiome. A prominent example for this are root hairs, short-lived protuberances of specialized root epidermal cells which increase root surface area and apparent root diameter, which is important for absorption of nutrients (Leitner et al., 2010; Keyes et al., 2013; Miguel et al., 2015) and water (Segal et al., 2008; Carminati et al., 2017b). Root hairs are involved in rhizosheath formation and hence very likely in microaggregate formation (Bengough, 2012). In addition, the root hair zone is generally regarded as the zone showing the highest uptake activity for many nutrients (Gilroy and Jones, 2000). Likewise, exudation patterns (quality and quantity) have been shown to be specific for the root hair zone (Badri and Vivanco, 2009).
Many metabolic activities in roots show a diurnal rhythm and functional responses to stress, such as the expression of certain transporters can be induced within minutes (Bienert and Chaumont, 2011). The temporal resolution at which rhizosphere properties are measured in many instances is low (time scale of weeks), in particular for destructive sampling approaches.
The temporal cycle which is observed around an individual root segment at a certain point in the soil is restarted when a lateral root emerges from the primordia of the higher order segment. With X-ray CT, neutron imaging, and magnetic resonance imaging (MRI) there are now methods available which can measure root growth patterns dynamically and non-destructively for the same system. This is of particular relevance for root age, which cannot be derived with other methods (Vetterlein and Doussan, 2016).
Hypotheses Related to Rhizosphere as a Self-Organized System
The overarching hypothesis is that part of the resilience in agricultural systems emerges from self-organized spatiotemporal pattern formation in the rhizosphere. In detail, we hypothesize that:
(1) Self-organization in the rhizosphere leads to specific spatiotemporal patterns of water, nutrients, root-derived organic compounds, soil particles, and the microbiome. The interplay between these patterns is the key for efficient resource acquisition by the plant.
(2) Self-organization in the rhizosphere leads to soil structure (emergent property) formation and thus increases microbial habitat diversity. Structures in part are long-lasting, i.e., they will still be present, when the processes initiating them are no longer present as particles stay in place through cohesive and adhesive forces until they are displaced by another disturbance.
(3) The size of the rhizosphere, as determined by the radial extent of pattern formation controlled by root activity and morphology, is specific for different rhizosphere components and modulated by soil texture, plant species, microbial activity, and driving forces for transport.
(4) The complex process of self-organization in the rhizosphere follows a prevailing general pattern of interactions between roots, the associated microbiome, and the soil. External drivers, like climate, agriculture management, soil, plant species, or introduction of new players in the microbiome, result in quantitative but not qualitative changes in the self-organized rhizosphere system.
Experimental systems to test the above hypotheses have to enable merging and correlation of patterns obtained with different techniques (Table 2, Figure 6). Ideally, for the same experimental systems the measurement of emerging properties like water and nutrient availability, soil structure, plant health needs to be conducted (Figure 2). The analysis of these four main hypotheses is enabled by implementing joint experiments and applying as many as possible of the techniques described in the Table 2. For instance, using μXRCT facilitates, in its part, the assessments of hypothesis 1, spatiotemporal patterns, hypothesis 3, specific volumes for different rhizosphere components controlled by root activity and morphology, and hypothesis 4, a template for general pattern of interactions between the components of the rhizosphere. And, by investigating the abundance and diversity of fungal communities using fungal markers, the four hypotheses can be assessed at the level of fungal populations, including the hypothesis 3 which suggests that rhizosphere formation leads to soil structure formation and thus increases microbial habitat diversity, but its role in assessing hypothesis 4 is to represent a component of the general pattern of interactions.
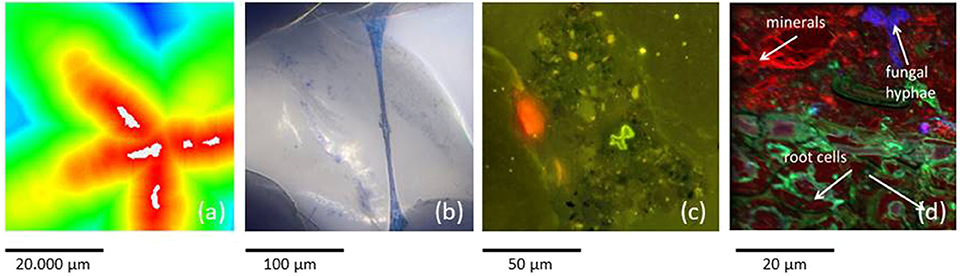
Figure 6. Examples of 3D or 2D spatial patterns of components or relevance for rhizosphere research covering different scales. (a) Root distance map derived from X-ray CT scanning (Koebernick et al., 2014). (b) Light microscopy image of mucilage strands in quartz sand (Carminati, unpublished data). (c) FISH-stained bacteria colonizing quartz mineral and clay humus complex (Eickhorst and Tippkötter, 2008). (d) Composite image (NanoSIMS) of an embedded and polished root-soil interface. Minerals indicated by 16O-(red); root cells by 12C14N (green); fungal hyphae by 32S-(blue), (Mueller, unpublished data).
Focal Topics of Interest to be Addressed by the New Approach
Plant Microbe Interplay in the Rhizosphere
Due to the traditional strategies used for rhizosphere, root and exudation sampling, very little is known about the temporal and spatial patterns of rhizosphere microbiome proliferation, root defense responses and rhizodeposition, and the microbial and plant signaling molecules mediating the interactions among the microbiota and with the host plant. This is of course also true for the spatial distribution of functions related to microbiome-plant interactions, like the spatial and temporal changes in the representation of plant receptors for microbe-associated patterns or degradation and transformation of organic molecules.
The seed bank of the rhizosphere microbiome is the soil. The composition and quantity of minerals and pH have been shown to be major drivers of soil microbial diversity (Ding et al., 2013; Babin et al., 2014; Fierer, 2017; Lucas et al., 2018). Community structure analysis of DNA extracted directly from soils showed that the diversity is higher in bulk soil than in the rhizosphere (Schreiter et al., 2014), and it is established that the interactions among the microbiota have a central role in rhizosphere microbiome assembly (Xu et al., 2018; El Sayed et al., 2019; Li et al., 2019). Bacteria forming biofilms and fungal mycelia do not uniformly colonize the root surface, and since certain root zones physically move during root development, the microbial community adapts and constantly reforms (Dupuy and Silk, 2016). Introduction of isolates or synthetic microbial consortia can enhance the ability of plants to cope with pests or pathogens, and during colonization, the resident rhizosphere community is modulated (Berg et al., 2014; Vorholt et al., 2017; Eltlbany et al., 2019). During colonization by both pathogenic and commensal members of the rhizosphere microbiota, the plant perceives and reacts to common molecular patterns of them, such as bacterial flagellin or fungal chitin, or more taxa-specific microbial compounds (Hacquard et al., 2017). Many of these molecules activate immune responses, but some attenuate plant defenses (Berendsen et al., 2012; Pieterse et al., 2014). In response, the plant immune system exerts a key role in shaping microbiome assembly (Teixeira et al., 2019). Apart from the microorganisms, plant immunity is further adjusted by abiotic factors such as changes in nutrient levels, and by plant development, with e.g., more pronounced plant defense responses in the differentiation zone than in the younger sections of the root (Stelpflug et al., 2016). Plant-derived rhizodeposits are acknowledged as the major drivers that shape microbial community composition in the rhizosphere (Badri and Vivanco, 2009) as they initiate and modulate the dialogue between roots and soil microorganisms (el Zahar Haichar et al., 2014). The major substance classes are organic acids, amino acids, sugars and vitamins, purines and secondary metabolites, and these substance classes may act on the microorganisms as nutrient sources, chemoattractants, growth promoters, or inhibitors (el Zahar Haichar et al., 2014; Watson et al., 2015). Differences in root exudate composition of plant species/genotypes have been shown to differently shape the rhizosphere microbiome community (Zhalnina et al., 2018). However, root exudation patterns also vary among different soils and change during plant growth (Chaparro et al., 2014; Neumann et al., 2014; Canarini et al., 2019). Apart from the rhizodeposits, other root physiological functions may also shape the microbiota. For instance, the competition for nutrients and water in the rhizosphere can be intense and effective plant uptake systems may indirectly influence the microbiome (Marschner et al., 2011; Verbon et al., 2017). In turn, a wide variety of microbes can improve plant nutrition, by promoting the development of the root system or by mobilizing nutrients (Lugtenberg and Kamilova, 2009). By stimulating microbial activity, root exudates also drive microbial N mining and mineralization of N, from the OM associated with minerals in the rhizosphere (Jilling et al., 2018).
Water Flux/Drought/Mucilage/Hydrophobicity
There is increasing evidence that mucilage exuded by the root tip impacts the soil physical and hydraulic properties. Mucilage of some plant species such as maize increases the water retention of the rhizosphere, but turns hydrophobic upon drying, causing water repellency in the rhizosphere (Carminati and Vetterlein, 2012). Mucilage has a higher viscosity than water, reducing the saturated hydraulic conductivity. However, the low surface tension and high viscosity prevent the break-up of the liquid phase during drying, thereby maintaining the physical connection between the root surface and the soil matrix (Carminati et al., 2017a). Despite this general behavior, significant variabilities in water holding capacity, water repellency and viscosity exist among plant species and mucilage age (Zickenrott et al., 2016; Naveed et al., 2019). Mucilage is, besides root hairs, one of the main factors responsible for the formation of stable rhizosheath (layer of soil adhering to the root surface) which is expected to maintain the physical contact between the root surface and the soil during severe drying events (Bengough, 2012). It is suggested that the interplay between low surface tension and high viscosity of mucilage increases the connectivity of the liquid phase in dry conditions, which is particularly important when air-filled gaps are expected to disconnect the roots from the soil. Little is known about the extent and conditions under which mucilage affects the ability of plant roots to extract water and nutrients from the soil, eventually playing a role in drought tolerance. Many open questions regard the differences in mucilage composition among plant species and environmental conditions, the spatial distribution of mucilage, its alteration due to microbial degradation and drying/wetting cycles, the detailed physics of how mucilage interacts with the soil particles and the soil water forming stable strands (Figure 6b), and how this impacts the configuration and connectivity of the liquid phase in the rhizosphere.
An additional open question concerning water fluxes across the rhizosphere is the role of root hairs in water uptake. Despite increasing evidence that root hairs increase nutrient uptake, their role in water relations remains controversial (Carminati et al., 2017b).
Cycling/Acquisition of Nutrients
The spatial and temporal root patterning (root system architecture) adapts permanently to changing nutrient availabilities; the response depends on the type and quantity of the nutrient and the plant species (Gruber et al., 2013; Meyer et al., 2019). Though information is scarce, root responses are suggested to be species- and ecotype-specific and evolved as adaptation to ecophysiological niches (Osmont et al., 2007). Under a given soil type and a given plant species, nutrient availability has a major impact on rhizosphere organization and regulative processes. A change in overall or local root patterning and cellular tissue functioning in response to one nutrient has severe consequences for: (1) uptake of other nutrients, (2) water fluxes in the soil and into the plant, (3) mucilage-efficiency, (4) carbon-status (photosynthesis and biomass built-up) and partitioning (root exudation), and (5) the microbiome (Hart et al., 2001; Hodge, 2006; Marschner, 2012; Gruber et al., 2013; Bouain et al., 2019). These effects on other rhizosphere-modifying drivers will entail various feedback loops independent of the causative nutrient itself, which in turn will demand a re-adaptation to the initial nutrient status. Certain nutrient deficiencies cause plant tissue deformations, plant tissue differentiations, and metabolic alterations, which unavoidably impair water and carbon fluxes, and root signal pathways, causing further re-organizations of the rhizosphere (Marschner, 2012).
Even the root system of a single plant ecotype can show a high variation in its morphological response to different nutrient deficiencies (Gruber et al., 2013). Considering the plenitude of existing ecotypes of various plant taxa illustrates the enormous plasticity of root responses at different scales. How these responses are regulated is mostly unknown. To date, comprehensive and comparative analyses of nutrient availability on holistic rhizosphere responses are in their infancy or lacking completely, even though these intrinsic root features determine the yield or stress tolerance of plants (Eshel and Beeckman, 2013; Li et al., 2016; Lynch, 2019).
Soil Structure/Microaggregate Formation/Carbon Sequestration
Roots affect soil structure, aggregate formation, and stability in multiple ways (Rasse et al., 2005; Hinsinger et al., 2009; Gregory et al., 2013), mediated and strongly coupled to the input of organic matter via root exudates and mucilage, but also as sloughed cells, root, and cell wall debris. The soil volume affected by roots and associated mycorrhizal fungi thus has a strong effect on the accrual of organic matter (Kravchenko et al., 2019b). The hyphae of mycorrhizal fungi provide an efficient mechanism for distributing plant carbon throughout the soil, facilitating its deposition into soil pores and onto mineral surfaces, where it can be protected from microbial attack. At the same time they contribute to the microbial necromass pool now considered to play a dominant role in SOM formation and stabilization (Frey, 2019). According to theory (Dexter, 1987) and experimental observations (Vollsnes et al., 2010; Aravena et al., 2011), the displacement of soil by growing roots causes a local increase in bulk density in the rhizosphere, although the magnitude and radial extent depends on soil texture, root type, and initial bulk density (Helliwell et al., 2019). Higher porosity in the direct vicinity of the root surface has also been reported and explained by gap formation (Carminati et al., 2013) and larger pore diameters caused by loose packing between convex soil particles and the convex root surface (Koebernick et al., 2019). The pressure exerted by roots when growing in the soil leads to a reorientation of clay minerals, promoting aggregation in clay microstructures (Dorioz et al., 1993; Tisdall, 1996). As the root tip moves through the soil, mucilage is distributed over mineral surfaces, sorbed to clay-sized minerals and contributes directly to the formation of microaggregates. Evidence for such a direct effect of root-released compounds is not well-documented, and it is generally believed that these compounds are easily assimilated. Small uncharged molecules such as glucose do not bind strongly to soil minerals (Pojasok and Kay, 1990) and thus do not show a direct impact. A major effect of roots on structure formation is an indirect one. Root exudates and debris are processed into the microbial biomass forming biofilms, which, together with the extracellularly produced polymers, act as binding agents in microaggregates (Chenu and Cosentino, 2011). In turn, this alters the microbial habitat toward a more porous, ordered, and aggregated structure (Feeney et al., 2006). After cell death and lysis, the microaggregate stabilized by microbial materials, especially polysaccharides, remains stable (Totsche et al., 2018). These processes are amplified by fine roots that cause intense and periodic drying in their surroundings and promote aggregation processes (Tisdall, 1996; Rasse et al., 2005). On the long term this affects the development of heterogeneity in soil structure within the soil profile (Young, 1998). Roots and associated mycorrhizal fungi also enhance macroaggregation by enmeshing soil particles (Tisdall and Oades, 1982; Rillig and Mummey, 2006; Wang et al., 2020). In an artificial root exudate experiment, the action of fungi on the formation of soil macroaggregation could be induced via the addition of model exudates, affecting the microbial community composition in favor of fungi (Baumert et al., 2018).
This is despite the fact that it has been reported that the so-called rhizosphere priming effect, if positive, can induce increased release of C. It has been observed mainly in short-term experiments (several weeks) and mechanisms underlying the rhizosphere priming effect remain elusive while several explaining hypotheses have been put forward, as discussed in detail by Lu et al. (2019). The microbial activation hypothesis states that microbial activity is stimulated by root exudates and this leads to an increased co-metabolic decomposition of SOM, responsible for a positive rhizosphere priming effect. Plant roots are considered to release exudates, which promote microbial growth and extracellular enzyme production in the rhizosphere to mine for N from SOM when soil N availability is limited, thereby increasing SOM decomposition (microbial N-mining hypothesis). The aggregate destruction hypothesis states that plant roots promote the destruction of soil aggregates more than their formation, thereby exposing the physically protected labile SOM to microbial attack that can result in increased SOM decomposition. He et al. (2020) observed in a 5 week experiment the disruption of coarse macro-aggregates, and the increased release of dissolved organic carbon (DOC) induced by roots. Their findings indicate that living roots together with rhizodeposits not only can directly stimulate rhizospheric microbial activities, but also can make soil matrix-protected organic carbon available to microbial attack, release DOC and induce a rhizosphere priming effect. Most of these investigations do not report the accumulation associated with their experiments in the rhizosphere from the addition of new C, so that taken altogether the processes described most often result in higher organic matter concentration in rhizosphere than in bulk soil.
Taken altogether, these processes result in higher organic matter concentration in rhizosphere than in bulk soil. It is to be expected that rhizodeposition has a strong effect, not only on microbial C processing, but also on the stabilization of organic C in soils and hence carbon storage (Frey, 2019; Sokol et al., 2019). Fahey et al. (2013) concluded that a small but substantial proportion of organic C released from plant roots enters non-labile organic matter pools. A recent study showed that soil in the rhizosphere of European beech is more aggregated and the aggregates have a different organic composition compared to bulk soil (Angst et al., 2016). Most information is derived from macroscopic and destructive observations of the soil structure, but detailed and in situ understanding of processes is lacking, also limiting our possibilities for modeling (Campbell and Paustian, 2015).
Analytical Developments with the Potential for Measurement of “Below-Ground” Parameter Patterns
There is a suite of imaging techniques with potential for analyses of rhizosphere spatial patterns and their dynamics in time (Table 2). Citations providing detailed information for the respective techniques are compiled in Table 2. Only few (Table 2, 1–6) enable non-invasive visualization of roots in 3D in situ over time. The information they provide is limited to structure, root architecture, water, or 11C distribution.
High-resolution chemical gradients can be analyzed using a number of 2D techniques (Table 2, 7–18), which mostly allow several elements, isotopes, or organic compounds to be studied simultaneously down to very fine resolution. The main challenge here is sample preparation, since many of these techniques require a flat surface, fixation, and vacuum stability. While these problems have been solved for samples containing only soil or only roots, the tricky part is to work with soft, elastic roots, and the hard soil minerals simultaneously. A further challenge with these techniques is the relatively small field of view, sample size, and sample number which can be analyzed. Intelligent concepts are required to select the sample locations and to embed the obtained results in a larger context through correlative imaging approaches (Schlüter et al., 2019).
Explicit localization of microorganisms is possible based on the phylogeny of the dominant players and their potential functions (Table 2, 19–22). These offer the chance to develop probes that can be used at the mm–μm scale using advanced microscopy tools to unravel the spatial and temporal patterns of microbial abundance (Schmidt et al., 2018).
Another group of techniques, which are not new but have been refined in recent years, are imaging approaches at the dm-scale (Table 2, 23–26). The critical point for some of them is the establishment of an optimal contact between measuring system and rhizosphere. Imaging approaches can be complemented by any technique requiring only small sample volumes, i.e., the image can be used to guide the point sampling.
Rhizosphere microbiome community composition can be analyzed from low sample sizes by amplicon sequencing of marker genes, that are established for bacteria, archaea (16S rRNA) and fungi (internal transcribed spacers), and developing for the analysis of protist diversity (Table 2, 28–30). Network analyses integrating the abundance patterns of microbial groups can then be used to identify taxa that are potentially linking the microbial populations, and to provide new hypotheses about interacting partners (Table 2, 31). Species-specific primers can be developed to follow quantitatively (qPCR) the abundance of enriched populations over spatio-temporal scales (Table 2, 32). Isotope labeling of substrates and subsequent amplicon sequencing of isotope-enriched DNA or RNA (Table 2, 33), or metagenomics and metatranscriptomics can be used (Table 2, 34) to combine the information on microbial community structure (who is there) with function (what are they doing). High sample volumes (labeling) and high demands of RNA quality restrict the use of these methods in rhizosphere research, but extensive metagenome and -transcriptome sequencing can identify enriched microbial traits that relate to plant host interactions, such as nutrient solubilization and uptake, consumption of rhizodeposits, and production of phytohormones or metabolites that trigger plant immunity. The steadily increasing amount of genome sequences of cultivated isolates and single cells in bacteria facilitates the use of prediction tools that assess traits (potential enzyme activities, potential metabolite patterns) of microorganisms based on taxonomic marker gene distribution, but ideally these should be confirmed at least in part by activity or metabolite analyses (Table 2, 35). The relative abundance and taxonomic distribution of established traits of microorganisms, such as root growth promotion, can be estimated by functional gene analysis at the levels of potential community activity and distribution among taxa (Table 2, 36).
For plant (root) analysis several “omics” techniques (ionomics, metabolomics, transcriptomics, proteomics, functional genomics, optical phenomics) have advanced the research field (Table 2, 37). For instance, RNA sequencing gives a snapshot of all expressed genes of the roots at different spatial levels. When the analysis is combined with correlation network analysis, the gene expression levels can be related to other measured variables (Table 2, 38). RNA sequencing generates key information on how developmental, biochemical, functional, morphologic, or genomic patterns evolve throughout the life span of a plant or in response to diverse external drivers. Further insight into the temporal and spatial regulation of gene expression provides the high-resolution quantification of targeted genes of interest by qPCR (Table 2, 39). RNA-based methods require high quality RNA which can become a major challenge. Forward genetics, analyzing the genetic basis responsible for a phenotype, has undergone recently a revolution through the development of novel statistical tools, computer algorithms, next-generation sequencing technologies, the availability of numerous plant genome sequences and high density marker maps, the development of doubled haploid technologies and the progress in quantitative trait locus (QTL), bulk segregant analysis and genome-wide association study (GWAS) methods. Mutant plants have been fundamental in the analysis of e.g., how plant nutrition or exudate spectrum play out in the establishment of rhizosphere microbiome (Table 2, 40).
In the past, analytical procedures for root exudates had to be developed specifically for individual components. Today, there are methods (Table 2, 41–42) available which enable the simultaneous determination of the whole metabolome present in exudates. Some of these methods provide even spatial resolution for intact organic molecules (Table 2, 18). While the analytical procedures have improved fundamentally, the way of sampling is still a matter of debate (Oburger and Schmidt, 2016). In addition, many studies have been performed in axenic hydroponic cultures providing information on the plant specific potential but neglecting the vast number of feedback loops between roots and soil which alter quality and quantity of exudates (Oburger et al., 2014). Alternative methods have been developed ranging from root exudate collecting tools to small-scale soil solution sampling, microdialysis, or the filter paper method (Oburger and Jones, 2018; Buckley et al., 2020). Results obtained are biased by the method of collection and a thorough methodological comparison is missing.
These novel, advanced and accessible methods and technologies in combination with advances in analytical chemistry and image analysis techniques allow today identifying genetic loci and plant mechanisms responsible for rhizosphere processes. Existing RNA, protein, metabolite, and element extraction methods of high purity down to the cellular scale, in combination with high-resolution analytics, feasible with very small-scale sample volumes, will enable analyzing age-dependent temporal and spatial processes in defined parts of the root system, and the rhizosphere. Moreover, modern technologies permit to quantitatively determine water, nutrient, and metabolite (exudates, signaling compounds, etc.) transport mechanisms over very small distances.
Image Analyses/Spatial Correlation/Interpolation/Modeling
The chance of obtaining spatially resolved data for many parameters brings along new challenges. First, for many methods, image processing has to be performed including removal of artifacts and noise, followed by edge enhancement and segmentation (Schlüter et al., 2014). Dedicated image processing protocols specialized on root segmentation continue to improve root recovery despite a heterogeneous soil background (Mairhofer et al., 2012; Flavel et al., 2017; Gao et al., 2019). Second, visual inspection has to be underpinned by quantitative image analysis in order to compare different treatments by meaningful image-based metrics.
For 3D data, there are a number of methods to quantify morphological properties of the pore space like pore size distribution, pore surface area, pore distances and pore connectivity (Vogel et al., 2010; Wildenschild and Sheppard, 2013). Likewise, for systems including roots, characteristic measures include total root surface, total root length or root length density profiles, but also equivalent hydraulic conductance or mean depth of root water uptake have been determined (Koebernick et al., 2014; Flavel et al., 2017; Schnepf et al., 2018a). There is a number of established metrics that quantify root system architecture in terms of root morphology and root topology which are summarized in Table 3. As an alternative to this root perspective, root system architecture can also be assessed from the soil perspective by investigating root distances. Such distance transforms of the binary images obtained from X-ray CT results in the Euclidean distance of each non-root voxel to the nearest root surface (Koebernick et al., 2014; Schlüter et al., 2018). Root distance histograms for the same total root length are different for different root architectures and express how efficiently the root system explores the soil (Schlüter et al., 2018). By superimposing data from different time points root age information can be derived (Blaser et al., 2018). Such data can be used in 3D mechanistic modeling (Koebernick et al., 2015; Koch et al., 2019) but can also be simplified to the 2D or 1D scale deriving frequency distributions for certain layers or the whole soil profile (de Moraes et al., 2018).
An illustrative example for such an image-based, spatial characterization of root system architecture and its implications for nutrient uptake is given in Figure 5. Root age is determined by time-lapse X-ray CT scanning to track the first emergence of any part of the root network (Figure 5A). Their distribution in space can be quantified by root distance maps in soil either for the entire root network or for young roots only as they are especially relevant in rhizodeposition and nutrient uptake (Figure 5B). These patterns need to be quantified in a meaningful way to assess rooting strategies. This can be done with root distance histograms (Figure 5C) in which the increased root length density is reflected by a shift toward shorter root distances. Alternatively, this improved soil exploration over time can be expressed with rhizosphere volume fractions for which the process-dependent extent of the rhizosphere into the soil needs to be set a priori (Figure 5D).
Biochemical mapping of the rhizosphere is typically limited to two-dimensional microscopy methods. Tools for image registration from different sources or images measured at different time points are readily available for the 2D case (Zitova and Flusser, 2003). For 3D this is more challenging especially when internal deformations and growth are involved (Peth et al., 2010; Keyes et al., 2016; Koestel and Schlüter, 2019). The superposition of 2D and 3D data, which is frequently applied in medicine, is still in its infancy for soil-based systems but protocols to do so are now emerging (Hapca et al., 2015; Juyal et al., 2019; Kravchenko et al., 2019a; Schlüter et al., 2019). Hapca et al. (2015) have shown that the information gained from successful image registration of 2D chemical maps and 3D structure data can even be used to obtain a 3D chemical characterization of the soil by geostatistical extrapolation techniques.
Once images of superimposed data are available, the next step is pattern recognition analysis as it is often applied in ecology to distinguish between random distributions or those resulting from a cascade of interactions and feedback loops (Wiegand and Moloney, 2013; Raynaud and Nunan, 2014). First attempts for application of these tools in rhizosphere research have been made (Nunan et al., 2002; Juyal et al., 2019; Schlüter et al., 2019) addressing the distribution of microorganisms in relation to soil structure and plant residues.
Data integration and mechanistic understanding can be improved by describing measured gradients or spatial patterns with single root models or root system models. There is a long tradition of single root scale models for water uptake, solute uptake, and microbial abundance. Those models are still further developed today, some of them taking more complex interactions into account (Schnepf et al., 2008) or even the explicit geometry of root and root hairs obtained by imaging (Daly et al., 2016). Patterns along the root axes and patterns in the whole rooting zone become apparent when combining single root models with functional-structural root system models (Dunbabin et al., 2013). Those models consider roots as line sources or sinks in soil and use an averaging approach to compute a sink/source term for the exchange of water or solutes between roots and soil. For the case of root water uptake, Daly et al. (2018) showed that spatially averaged models perform well in comparison to full 3D image-based models, with <2% difference in uptake. For less mobile components, it is of prime importance to represent in the model the steep gradients that may develop in the rhizosphere. The challenge is to estimate those gradients in a numerical approach in which the resolution of the soil is often larger than the extent of the radial gradient and in which roots are considered line sources or sinks (Roose et al., 2001; Dunbabin et al., 2002; Mai et al., 2018).
In order to predict emergent properties of the rhizosphere, we need to develop multiscale and multicomponent models that include feedback loops corresponding to specific questions such as the role of membrane transporters for resource uptake, microbial activity in the rhizosphere, and resulting quality of organic matter. Mechanistic models are a prerequisite for upscaling of small-scale properties and for predicting the emerging properties at the root system scale. As the spatial scale of interest is in the range of nm to cm, models can help to bridge between pore scale and continuum scale description of the system. To study emerging properties, we see high potential for individual-based models (Railsback and Grimm, 2019) of the rhizosphere system, where the individuals can represent microorganisms (Kreft et al., 2013; Hellweger et al., 2016; Portell et al., 2018) but also individual root elements. Individuals are characterized by their biomass and physiological state, their activities in their local environment such as compound uptake or release, and their synergistic or competitive local interactions. The processes involved may be described via continuous equations or discrete rules. The most important feature of such bottom-up models is that the collective actions of all individuals determine the emergent properties and patterns at the system level and the system's responses to environmental scenarios. Hence, individual-based models can describe highly complex system dynamics based on simpler local processes and be used to decipher the underlying mechanisms. Notwithstanding these advantages, very few examples of individual-based models applied to the rhizosphere can be found (Muci et al., 2012; König et al., 2020).
Call for Action
In order to implement a system approach based on self-organization and to test the hypotheses formulated above experimental platforms are required, with meaningful drivers for the different focal topics across the different disciplines (Figure 2). Such platforms should enable (i) to relate small-scale observations to system behavior, (ii) to integrate physical, chemical, and biological sampling approaches within the same experiment, and (iii) to sample at different time points during the life cycle of the system in question. In order to motivate scientists from different disciplines to find a common specific experimental platform, drivers expected to fundamentally alter physical, chemical, and biological spatial and temporal patterns in the rhizosphere have to be identified. Such drivers could then be taken into account when targeting the sustainable development goals defined by the United Nations related to sustainable agriculture management, saving water, and coping with climate change. A first example of such an experimental platform oriented toward management strategies (soil texture, plant genotype) has recently been described, including potential pitfalls in particular related to the extrapolation from the laboratory to the field scale in order to address the whole plant life cycle (Vetterlein et al., in press).
The Vision
Our vision is an improved understanding of the relevance of individual processes for the system scale, based on the analyses of root system specific spatiotemporal rhizosphere patterns (Figure 1). This approach will enable researchers to distinguish between system-immanent dynamics and changes indicating that systems will not recover completely from disturbance. The latter is often used as a measure for system resilience (Holling, 1973).
Taking the example of mucilage (Figures 1, 2), its distribution along the root system and with increasing distance from the root surface, will be the result of the plants' nutrient status and root age-dependent mucilage release rates, microbial degradation, which depends on initial microbiome composition varying in rhizosphere competence and hence ability to colonize the root surface. Soil water flux in turn will be a function of these patterns, but will also shape them as mucilage properties like water adsorption, viscosity, or wettability affect and are affected by drying cycles. These will not only feedback on nutrient transport processes but also on the diffusion of organic compounds (organic acids, exoenzymes) into the soil, which in turn are supposed to increase availability of nutrients. Further feedback loops, based on the sensing of the internal nutrient and water status, involve increased or decreased expression of membrane nutrient and water transporters and alteration of biochemical pathways changing root growth patterns, which change root system development and so forth. In the context of the stated hypotheses, the mucilage example thus relates to hypothesis 1 that suggests specific spatiotemporal patterns, hypothesis 3 that suggests the specific volumes for different rhizosphere components controlled by root activity and morphology, and hypothesis 4 that suggests prevailing general patterns of interactions between the components of the rhizosphere.
For many patterns, we may only be able to obtain snapshots/regions of interest but for others we can get continuous information or cover the whole root system in 3D. These latter measurements help to extrapolate the local or snapshot data with the help of models. Besides disentangling and understanding complex rhizosphere dynamics, the functions of models are hence on the one hand to predict the patterns for the whole root system and on the other hand to integrate over the whole root system and predict the emergent properties. Ideally, model results can then be compared to measured emergent system properties like water and nutrient availability, biomass production, plant health, water infiltration rate, soil organic carbon storage, and alike. Comparing differences induced by different drivers will considerably help to derive mechanistic understanding of the rhizosphere as self-organized system.
Author Contributions
DV: initiated and compiled the manuscript. AC, IK-K, GB, KS, EO, AS, TB, MT, and SS: contributed discipline specific aspects and supported the integration into the concept of self-organization. AC: water-related issue and soil physics. IK-K: soil organic carbon and soil chemistry. GB: plant genetics and nutrition. KS: microbial interplay. EO: plant exudation. AS and TB: modeling. MT: microbial interplay and plant genetics. SS: imaging and soil physics. All authors contributed to the article and approved the submitted version.
Funding
We acknowledge the DFG (German Research Foundation) for funding the priority programme 2089 Rhizosphere Spatiotemporal Organization–A Key to Rhizosphere Functions (project number 403801423).
Conflict of Interest
The authors declare that the research was conducted in the absence of any commercial or financial relationships that could be construed as a potential conflict of interest.
Acknowledgments
For fruitful discussions concerning self-organization, resilience, and individual-based modeling we thank Hans-Jörg Vogel and Volker Grimm.
References
Angst, G., Kögel-Knabner, I., Kirfel, K., Hertel, D., and Mueller, C. W. (2016). Spatial distribution and chemical composition of soil organic matter fractions in rhizosphere and non-rhizosphere soil under European beech (Fagus sylvatica L.). Geoderma 264, 179–187. doi: 10.1016/j.geoderma.2015.10.016
Aoyagi, T., Hanada, S., Itoh, H., Sato, Y., Ogata, A., Friedrich, M. W., et al. (2015). Ultra-high-sensitivity stable-isotope probing of rRNA by high-throughput sequencing of isopycnic centrifugation gradients. Environ. Microbiol. Rep. 7, 282–287. doi: 10.1111/1758-2229.12243
Aravena, J. E., Berli, M., Ghezzehei, T. A., and Tyler, S. W. (2011). Effects of root-induced compaction on rhizosphere hydraulic properties-X-ray microtomography imaging and numerical simulations. Environ. Sci. Technol. 45, 425–431. doi: 10.1021/es102566j
Aßhauer, K. P., Wemheuer, B., Daniel, R., and Meinicke, P. (2015). Tax4Fun: predicting functional profiles from metagenomic 16S rRNA data. Bioinformatics 31, 2882–2884. doi: 10.1093/bioinformatics/btv287
Babin, D., Vogel, C., Zühlke, S., Schloter, M., Pronk, G. J., Heister, K., et al. (2014). Soil mineral composition matters: response of microbial communities to phenanthrene and plant litter addition in long-term matured artificial soils. PLoS ONE 9:e106865. doi: 10.1371/journal.pone.0106865
Badri, D. V., and Vivanco, J. M. (2009). Regulation and function of root exudates. Plant Cell Environ. 32, 666–681. doi: 10.1111/j.1365-3040.2009.01926.x
Balietti, S., Mäs, M., and Helbing, D. (2015). On disciplinary fragmentation and scientific progress. PLoS ONE 10:e0118747. doi: 10.1371/journal.pone.0118747
Barlow, A. J., Scott, O., Sano, N., and Cumpson, P. J. (2015). Multivariate Auger Feature Imaging (MAFI)–a new approach towards chemical state identification of novel carbons in XPS imaging. Surf. Interface Anal. 47, 173–175. doi: 10.1002/sia.5738
Bárzana, G., Aroca, R., Bienert, G. P., Chaumont, F., and Ruiz-Lozano, J. M. (2014). New insights into the regulation of aquaporins by the arbuscular mycorrhizal symbiosis in maize plants under drought stress and possible implications for plant performance. Mol. Plant-Microbe Interact. 27, 349–363. doi: 10.1094/MPMI-09-13-0268-R
Baumert, V. L., Vasilyeva, N. A., Vladimirov, A. A., Meier, I. C., Kögel-Knabner, I., and Mueller, C. W. (2018). Root exudates induce soil macroaggregation facilitated by fungi in subsoil. Front. Environ. Sci. 6:140. doi: 10.3389/fenvs.2018.00140
Bengough, A. (2012). Water dynamics of the root zone: rhizosphere biophysics and its control on soil hydrology. Vadose Zone J. 11:vzj2011.0111. doi: 10.2136/vzj2011.0111
Berendsen, R. L., Pieterse, C. M., and Bakker, P. A. (2012). The rhizosphere microbiome and plant health. Trends Plant Sci. 17, 478–486. doi: 10.1016/j.tplants.2012.04.001
Berg, G., Grube, M., Schloter, M., and Smalla, K. (2014). Unraveling the plant microbiome: looking back and future perspectives. Front. Microbiol. 5:148. doi: 10.3389/fmicb.2014.00148
Berg, G., Köberl, M., Rybakova, D., Müller, H., Grosch, R., and Smalla, K. (2017). Plant microbial diversity is suggested as the key to future biocontrol and health trends. FEMS Microbiol. Ecol. 93:fix050. doi: 10.1093/femsec/fix050
Berg, G., and Smalla, K. (2009). Plant species and soil type cooperatively shape the structure and function of microbial communities in the rhizosphere. FEMS Microbiol. Ecol. 68, 1–13. doi: 10.1111/j.1574-6941.2009.00654.x
Bienert, G. P., and Chaumont, F. (2011). “Plant aquaporins: roles in water homeostasis, nutrition, and signaling processes,” in Transporters and Pumps in Plant Signaling, Vol. 7, eds M. Geisler and K. Venema (Berlin: Springer), 3–36. doi: 10.1007/978-3-642-14369-4_1
Biggs, R., Schlüter, M., Biggs, D., Bohensky, E. L., BurnSilver, S., Cundill, G., et al. (2012). Toward principles for enhancing the resilience of ecosystem services. Annu. Rev. Environ. Resour. 37, 421–448. doi: 10.1146/annurev-environ-051211-123836
Blaser, S. R., Schlüter, S., and Vetterlein, D. (2018). How much is too much?—Influence of X-ray dose on root growth of faba bean (Vicia faba) and barley (Hordeum vulgare). PLoS ONE 13:e0193669. doi: 10.1371/journal.pone.0193669
Blossfeld, S., and Gansert, D. (2007). A novel non-invasive optical method for quantitative visualization of pH dynamics in the rhizosphere of plants. Plant Cell Environ. 30, 176–186. doi: 10.1111/j.1365-3040.2006.01616.x
Blossfeld, S., Perriguey, J., Sterckeman, T., Morel, J.-L., and Lösch, R. (2010). Rhizosphere pH dynamics in trace-metal-contaminated soils, monitored with planar pH optodes. Plant Soil 330, 173–184. doi: 10.1007/s11104-009-0190-z
Bolwerk, A., Lagopodi, A. L., Wijfjes, A. H., Lamers, G. E., Chin-A-Woeng, T. F., Lugtenberg, B. J., et al. (2003). Interactions in the tomato rhizosphere of two Pseudomonas biocontrol strains with the phytopathogenic fungus Fusarium oxysporum f. sp. radicis-lycopersici. Mol. Plant-Microbe Interact. 16, 983–993. doi: 10.1094/MPMI.2003.16.11.983
Bouain, N., Krouk, G., Lacombe, B., and Rouached, H. (2019). Getting to the root of plant mineral nutrition: combinatorial nutrient stresses reveal emergent properties. Trends Plant Sci. 24, 542–552. doi: 10.1016/j.tplants.2019.03.008
Bouffaud, M.-L., Renoud, S., Dubost, A., Moënne-Loccoz, Y., and Muller, D. (2018). 1-Aminocyclopropane-1-carboxylate deaminase producers associated to maize and other Poaceae species. Microbiome 6:114. doi: 10.1186/s40168-018-0503-7
Buckley, S., Brackin, R., Jämtgård, S., Näsholm, T., and Schmidt, S. (2020). Microdialysis in soil environments: current practice and future perspectives. Soil Biol. Biochem. 143:107743. doi: 10.1016/j.soilbio.2020.107743
Camazine, S., Deneubourg, J.-L., Franks, N. R., Sneyd, J., Bonabeau, E., and Theraula, G. (2003). Self-Organization in Biological Systems. Princeton, NJ: Princeton University Press.
Campbell, E. E., and Paustian, K. (2015). Current developments in soil organic matter modeling and the expansion of model applications: a review. Environ. Res. Lett. 10:123004. doi: 10.1088/1748-9326/10/12/123004
Canarini, A., Kaiser, C., Merchant, A., Richter, A., and Wanek, W. (2019). Root exudation of primary metabolites: mechanisms and their roles in plant responses to environmental stimuli. Front. Plant Sci. 10:157. doi: 10.3389/fpls.2019.00157
Carminati, A., Benard, P., Ahmed, M., and Zarebanadkouki, M. (2017a). Liquid bridges at the root-soil interface. Plant Soil 417, 1–15. doi: 10.1007/s11104-017-3227-8
Carminati, A., Moradi, A. B., Vetterlein, D., Vontobel, P., Lehmann, E., Weller, U., et al. (2010). Dynamics of soil water content in the rhizosphere. Plant Soil 332, 163–176. doi: 10.1007/s11104-010-0283-8
Carminati, A., Passioura, J. B., Zarebanadkouki, M., Ahmed, M. A., Ryan, P. R., Watt, M., et al. (2017b). Root hairs enable high transpiration rates in drying soils. New Phytol. 216, 771–781. doi: 10.1111/nph.14715
Carminati, A., and Vetterlein, D. (2012). Plasticity of rhizosphere hydraulic properties as a key for efficient utilization of scarce resources. Ann. Bot. 112, 277–290. doi: 10.1093/aob/mcs262
Carminati, A., Vetterlein, D., Koebernick, N., Blaser, S., Weller, U., and Vogel, H.-J. (2013). Do roots mind the gap? Plant Soil 367, 651–661. doi: 10.1007/s11104-012-1496-9
Castillo-Michel, H., Hernandez-Viezcas, J. A., Servin, A., Peralta-Videa, J. R., and Gardea-Torresdey, J. L. (2012). Arsenic localization and speciation in the root–soil interface of the desert plant prosopis juliflora-velutina. Appl. Spectrosc. 66, 719–727. doi: 10.1366/11-06336
Chaparro, J. M., Badri, D. V., and Vivanco, J. M. (2014). Rhizosphere microbiome assemblage is affected by plant development. ISME J. 8:790. doi: 10.1038/ismej.2013.196
Chenu, C., and Cosentino, D. (2011). “Microbial regulation of soil structural dynamics,” in The Architecture and Biology of Soils: Life in Inner Space, eds K. Ritz and I. Young (Wallingford, UK: CABI), 37–70.
Clark, R. T., MacCurdy, R. B., Jung, J. K., Shaff, J. E., McCouch, S. R., Aneshansley, D. J., et al. (2011). Three-dimensional root phenotyping with a novel imaging and software platform. Plant Physiol. 156, 455–465. doi: 10.1104/pp.110.169102
Daly, K. R., Keyes, S. D., Masum, S., and Roose, T. (2016). Image-based modelling of nutrient movement in and around the rhizosphere. J. Exp. Bot. 67, 1059–1070. doi: 10.1093/jxb/erv544
Daly, K. R., Tracy, S. R., Crout, N. M., Mairhofer, S., Pridmore, T. P., Mooney, S. J., et al. (2018). Quantification of root water uptake in soil using X-ray computed tomography and image-based modelling. Plant Cell Environ. 41, 121–133. doi: 10.1111/pce.12983
Darwent, M. J., Paterson, E., McDonald, A. J. S., and Tomos, A. D. (2003). Biosensor reporting of root exudation from Hordeum vulgare in relation to shoot nitrate concentration. J. Exp. Bot. 54, 325–334. doi: 10.1093/jxb/erg017
De Feudis, M., Cardelli, V., Massaccesi, L., Hofmann, D., Berns, A., Bol, R., et al. (2017). Altitude affects the quality of the water-extractable organic matter (WEOM) from rhizosphere and bulk soil in European beech forests. Geoderma 302, 6–13. doi: 10.1016/j.geoderma.2017.04.015
de Moraes, M. T., Bengough, A. G., Debiasi, H., Franchini, J. C., Levien, R., Schnepf, A., et al. (2018). Mechanistic framework to link root growth models with weather and soil physical properties, including example applications to soybean growth in Brazil. Plant Soil 428, 67–92. doi: 10.1007/s11104-018-3656-z
de Parseval, H., Barot, S., Gignoux, J., Lata, J.-C., and Raynaud, X. (2017). Modelling facilitation or competition within a root system: importance of the overlap of root depletion and accumulation zones. Plant Soil 419, 97–111. doi: 10.1007/s11104-017-3321-y
Delory, B. M., Li, M., Topp, C. N., and Lobet, G. (2018). archiDART v3.0: a new data analysis pipeline allowing the topological analysis of plant root systems. F1000Research 7:22. doi: 10.12688/f1000research.13541.1
Dexter, A. (1987). Compression of soil around roots. Plant Soil 97, 401–406. doi: 10.1007/BF02383230
Ding, G.-C., Pronk, G. J., Babin, D., Heuer, H., Heister, K., Kögel-Knabner, I., et al. (2013). Mineral composition and charcoal determine the bacterial community structure in artificial soils. FEMS Microbiol. Ecol. 86, 15–25. doi: 10.1111/1574-6941.12070
Dorioz, J., Robert, M., and Chenu, C. (1993). “The role of roots, fungi and bacteria on clay particle organization. An experimental approach,” in Soil Structure/Soil Biota Interrelationships, eds L. Brussaard, and M. Kooistra (Wageningen: Elsevier), 179–194. doi: 10.1016/B978-0-444-81490-6.50019-4
Dunbabin, V. M., Diggle, A. J., Rengel, Z., and Van Hugten, R. (2002). Modelling the interactions between water and nutrient uptake and root growth. Plant Soil 239, 19–38. doi: 10.1023/A:1014939512104
Dunbabin, V. M., Postma, J. A., Schnepf, A., Pagès, L., Javaux, M., Wu, L., et al. (2013). Modelling root–soil interactions using three–dimensional models of root growth, architecture and function. Plant Soil 372, 93–124. doi: 10.1007/s11104-013-1769-y
Dupuy, L. X., and Silk, W. K. (2016). Mechanisms of early microbial establishment on growing root surfaces. Vadose Zone J. 15, 1–13. doi: 10.2136/vzj2015.06.0094
Eickhorst, T., and Tippkötter, R. (2008). Detection of microorganisms in undisturbed soil by combining fluorescence in situ hybridization (FISH) and micropedological methods. Soil Biol. Biochem. 40, 1284–1293. doi: 10.1016/j.soilbio.2007.06.019
El Sayed, T., Samuel, J., Nour, E., Sørensen, S. J., and Smalla, K. (2019). Biocontrol of bacterial wilt disease through complex interaction between tomato plant, antagonists, the indigenous rhizosphere microbiota and Ralstonia solanacearum. Front. Microbiol. 10:2835. doi: 10.3389/fmicb.2019.02835
el Zahar Haichar, F., Roncato, M.-A., and Achouak, W. (2012). Stable isotope probing of bacterial community structure and gene expression in the rhizosphere of Arabidopsis thaliana. FEMS Microbiol. Ecol. 81, 291–302. doi: 10.1111/j.1574-6941.2012.01345.x
el Zahar Haichar, F., Santaella, C., Heulin, T., and Achouak, W. (2014). Root exudates mediated interactions belowground. Soil Biol. Biochem. 77, 69–80. doi: 10.1016/j.soilbio.2014.06.017
Eltlbany, N., Baklawa, M., Ding, G.-C., Nassal, D., Weber, N., Kandeler, E., et al. (2019). Enhanced tomato plant growth in soil under reduced P supply through microbial inoculants and microbiome shifts. FEMS Microbiol. Ecol. 95:fiz124. doi: 10.1093/femsec/fiz124
Eshel, A., and Beeckman, T. (2013). Plant Roots: The Hidden Half . Boca Raton, FL: CRC press. doi: 10.1201/b14550
Fahey, T. J., Yavitt, J. B., Sherman, R. E., Groffman, P. M., and Wang, G. (2013). Partitioning of belowground C in young sugar maple forest. Plant Soil 367, 379–389. doi: 10.1007/s11104-012-1459-1
Fang, W., and Leger, R. J. S. (2010). Mrt, a gene unique to fungi, encodes an oligosaccharide transporter and facilitates rhizosphere competency in metarhizium robertsii. Plant Physiol 154, 1549–1557. doi: 10.1104/pp.110.163014
Feeney, D., Crawford, J., Daniell, T., Hallett, P., Nunan, N., Ritz, K., et al. (2006). Three-dimensional microorganization of the soil-root-microbe system. Microb. Ecol. 52, 151–158. doi: 10.1007/s00248-006-9062-8
Ferlian, O., Biere, A., Bonfante, P., Buscot, F., Eisenhauer, N., Fernandez, I., et al. (2018). Growing research networks on mycorrhizae for mutual benefits. Trends Plant Sci. 23, 975–984. doi: 10.1016/j.tplants.2018.08.008
Fierer, N. (2017). Embracing the unknown: disentangling the complexities of the soil microbiome. Nature Rev. Microbiol. 15:579. doi: 10.1038/nrmicro.2017.87
Fimmen, R. L., Richter, D. D, Vasudevan, D., Williams, M. A., and West, L. T. (2008). Rhizogenic Fe–C redox cycling: a hypothetical biogeochemical mechanism that drives crustal weathering in upland soils. Biogeochemistry 87, 127–141. doi: 10.1007/s10533-007-9172-5
Flavel, R. J., Guppy, C. N., Rabbi, S. M., and Young, I. M. (2017). An image processing and analysis tool for identifying and analysing complex plant root systems in 3D soil using non-destructive analysis: root1. PLoS ONE 12:e0176433. doi: 10.1371/journal.pone.0176433
Frey, S. D. (2019). Mycorrhizal fungi as mediators of soil organic matter dynamics. Annu. Rev. Ecol. Evol. Syst. 50, 237–259. doi: 10.1146/annurev-ecolsys-110617-062331
Galkovskyi, T., Mileyko, Y., Bucksch, A., Moore, B., Symonova, O., Price, C. A., et al. (2012). GiA roots: software for the high throughput analysis of plant root system architecture. BMC Plant Biol. 12:116. doi: 10.1186/1471-2229-12-116
Gao, W., Schlüter, S., Blaser, S. R., Shen, J., and Vetterlein, D. (2019). A shape-based method for automatic and rapid segmentation of roots in soil from X-ray computed tomography images: rootine. Plant Soil 441, 643–655. doi: 10.1007/s11104-019-04053-6
Garbout, A., Munkholm, L. J., Hansen, S. B., Petersen, B. M., Munk, O. L., and Pajor, R. (2012). The use of PET/CT scanning technique for 3D visualization and quantification of real-time soil/plant interactions. Plant Soil 352, 113–127. doi: 10.1007/s11104-011-0983-8
Gilroy, S., and Jones, D. L. (2000). Through form to function: root hair development and nutrient uptake. Trends Plant Sci. 5, 56–60. doi: 10.1016/S1360-1385(99)01551-4
Gregory, P. J., Bengough, A. G., George, T. S., and Hallett, P. D. (2013). “Rhizosphere engineering by plants: quantifying soil–root interactions,” in Enhancing Understanding and Quantification of Soil–Root Growth Interactions, Advances in Agricultural Systems Modeling, eds D. Timlin and L. Ahuja, Vol. 4, 1–30.
Gruber, B. D., Giehl, R. F., Friedel, S., and von Wirén, N. (2013). Plasticity of the arabidopsis root system under nutrient deficiencies. Plant Physiol. 163, 161–179. doi: 10.1104/pp.113.218453
Haber-Pohlmeier, S., Tötzke, C., Lehmann, E., Kardjilov, N., Pohlmeier, A., and Oswald, S. (2019). Combination of magnetic resonance imaging and neutron computed tomography for three-dimensional rhizosphere imaging. Vadose Zone J. 18, 1–11. doi: 10.2136/vzj2018.09.0166
Hacquard, S., Spaepen, S., Garrido-Oter, R., and Schulze-Lefert, P. (2017). Interplay between innate immunity and the plant microbiota. Annu. Rev. Phytopathol. 55, 565–589. doi: 10.1146/annurev-phyto-080516-035623
Hafner, A. E., Krausser, J., and Šarić, A. (2019). Minimal coarse-grained models for molecular self-organisation in biology. Curr. Opin. Struct. Biol. 58, 43–52. doi: 10.1016/j.sbi.2019.05.018
Hansen, S. K., Rainey, P. B., Haagensen, J. A., and Molin, S. (2007). Evolution of species interactions in a biofilm community. Nature 445, 533–536. doi: 10.1038/nature05514
Hapca, S., Baveye, P. C., Wilson, C., Lark, R. M., and Otten, W. (2015). Three-dimensional mapping of soil chemical characteristics at micrometric scale by combining 2D SEM-EDX data and 3D X-ray CT images. PLoS ONE 10:e0137205. doi: 10.1371/journal.pone.0137205
Hart, T. D., Lynch, J. M., and Chamberlain, A. H. (2001). Anion exclusion in microbial and soil polysaccharides. Biol. Fertil. Soils 34, 201–209. doi: 10.1007/s003740100400
He, Y., Cheng, W., Zhou, L., Shao, J., Liu, H., Zhou, H., et al. (2020). Soil DOC release and aggregate disruption mediate rhizosphere priming effect on soil C decomposition. Soil Biol. Biochem. 144:107787. doi: 10.1016/j.soilbio.2020.107787
Helliwell, J., Sturrock, C., Miller, A., Whalley, W., and Mooney, S. (2019). The role of plant species and soil condition in the physical development of the rhizosphere. Plant Cell Environ. 42, 1974–1986. doi: 10.1111/pce.13529
Hellweger, F. L., Clegg, R. J., Clark, J. R., Plugge, C. M., and Kreft, J.-U. (2016). Advancing microbial sciences by individual-based modelling. Nature Rev. Microbiol. 14:461. doi: 10.1038/nrmicro.2016.62
Hill, C. B., Cassin, A., Keeble-Gagnère, G., Doblin, M. S., Bacic, A., and Roessner, U. (2016). De novo transcriptome assembly and analysis of differentially expressed genes of two barley genotypes reveal root-zone-specific responses to salt exposure. Sci. Rep. 6:31558. doi: 10.1038/srep31558
Hinsinger, P., Bengough, A. G., Vetterlein, D., and Young, I. M. (2009). Rhizosphere: biophysics, biogeochemistry and ecological relevance. Plant Soil 321:117–152. doi: 10.1007/s11104-008-9885-9
Holling, C. S. (1973). Resilience and stability of ecological systems. Annu. Rev. Ecol. Syst. 4, 1–23. doi: 10.1146/annurev.es.04.110173.000245
Holz, M., Zarebanadkouki, M., Carminati, A., and Kuzyakov, Y. (2019). Visualization and quantification of root exudation using 14C imaging: challenges and uncertainties. Plant Soil 437, 473–485. doi: 10.1007/s11104-019-03956-8
Holz, M., Zarebanadkouki, M., Kaestner, A., Kuzyakov, Y., and Carminati, A. (2018). Rhizodeposition under drought is controlled by root growth rate and rhizosphere water content. Plant Soil 423, 429–442. doi: 10.1007/s11104-017-3522-4
Jaillard, B., Guyon, A., and Maurin, A. (1991). Structure and composition of calcified roots, and their identification in calcareous soils. Geoderma 50, 197–210. doi: 10.1016/0016-7061(91)90034-Q
Jilling, A., Keiluweit, M., Contosta, A. R., Frey, S., Schimel, J., Schnecker, J., et al. (2018). Minerals in the rhizosphere: overlooked mediators of soil nitrogen availability to plants and microbes. Biogeochemistry 139, 103–122. doi: 10.1007/s10533-018-0459-5
Jiménez-Fernández, D., Montes-Borrego, M., Navas-Cortés, J. A., Jiménez-Díaz, R. M., and Landa, B. B. (2010). Identification and quantification of Fusarium oxysporum in planta and soil by means of an improved specific and quantitative PCR assay. Appl. Soil Ecol. 46, 372–382. doi: 10.1016/j.apsoil.2010.10.001
Jones, D. L., Nguyen, C., and Finlay, R. D. (2009). Carbon flow in the rhizosphere: carbon trading at the soil–root interface. Plant Soil 321, 5–33. doi: 10.1007/s11104-009-9925-0
Juyal, A., Otten, W., Falconer, R., Hapca, S., Schmidt, H., Baveye, P. C., et al. (2019). Combination of techniques to quantify the distribution of bacteria in their soil microhabitats at different spatial scales. Geoderma 334, 165–174. doi: 10.1016/j.geoderma.2018.07.031
Keiluweit, M., Bougoure, J. J., Nico, P. S., Pett-Ridge, J., Weber, P. K., and Kleber, M. (2015). Mineral protection of soil carbon counteracted by root exudates. Nat. Clim. Change 5:588. doi: 10.1038/nclimate2580
Keyes, S., Gillard, F., Soper, N., Mavrogordato, M., Sinclair, I., and Roose, T. (2016). Mapping soil deformation around plant roots using in vivo 4D X-ray computed tomography and digital volume correlation. J. Biomech. 49, 1802–1811. doi: 10.1016/j.jbiomech.2016.04.023
Keyes, S. D., Daly, K. R., Gostling, N. J., Jones, D. L., Talboys, P., Pinzer, B. R., et al. (2013). High resolution synchrotron imaging of wheat root hairs growing in soil and image based modelling of phosphate uptake. New Phytol. 198, 1023–1029. doi: 10.1111/nph.12294
Kirk, G., Santos, E., and Findenegg, G. (1999). Phosphate solubilization by organic anion excretion from rice (Oryza sativa L.) growing in aerobic soil. Plant Soil 211, 11–18. doi: 10.1023/A:1004539212083
Koch, A., Meunier, F., Vanderborght, J., Garré, S, Pohlmeier, A., and Javaux, M. (2019). Functional–structural root-system model validation using a soil MRI experiment. J. Exp. Bot. 70, 2797–2809. doi: 10.1093/jxb/erz060
Koebernick, N., Daly, K. R., Keyes, S. D., Bengough, A. G., Brown, L. K., Cooper, L. J., et al. (2019). Imaging microstructure of the barley rhizosphere: particle packing and root hair influences. New Phytol. 221, 1878–1889. doi: 10.1111/nph.15516
Koebernick, N., Huber, K., Kerkhofs, E., Vanderborght, J., Javaux, M., Vereecken, H., et al. (2015). Unraveling the hydrodynamics of split root water uptake experiments using CT scanned root architectures and three dimensional flow simulations. Front. Plant Sci. 6:370. doi: 10.3389/fpls.2015.00370
Koebernick, N., Schlüter, S., Blaser, S. R., and Vetterlein, D. (2018). Root-soil contact dynamics of Vicia faba in sand. Plant Soil 431, 417–431. doi: 10.1007/s11104-018-3769-4
Koebernick, N., Weller, U., Huber, K., Schlüter, S., Vogel, H.-J., Jahn, R., et al. (2014). In situ visualization and quantification of three-dimensional root system architecture and growth using X-Ray computed tomography. Vadose Zone J. 13, 1–10. doi: 10.2136/vzj2014.03.0024
Koestel, J., and Schlüter, S. (2019). Quantification of the structure evolution in a garden soil over the course of two years. Geoderma 338, 597–609. doi: 10.1016/j.geoderma.2018.12.030
König, S., Vogel, H.-J., Harms, H., and Worrich, A. (2020). Physical, chemical and biological effects on soil bacterial dynamics in microscale models. Front. Ecol. Evol. 8:53. doi: 10.3389/fevo.2020.00053
Kortz, A., Hochholdinger, F., and Yu, P. (2019). Cell type-specific transcriptomics of lateral root formation and plasticity. Front. Plant Sci. 10:21. doi: 10.3389/fpls.2019.00021
Kravchenko, A., Guber, A., Razavi, B., Koestel, J., Blagodatskaya, E., and Kuzyakov, Y. (2019a). Spatial patterns of extracellular enzymes: combining X-ray computed micro-tomography and 2D zymography. Soil Biol. Biochem. 135, 411–419. doi: 10.1016/j.soilbio.2019.06.002
Kravchenko, A., Guber, A., Razavi, B., Koestel, J., Quigley, M., Robertson, G., et al. (2019b). Microbial spatial footprint as a driver of soil carbon stabilization. Nat. Commun. 10, 1–10. doi: 10.1038/s41467-019-11057-4
Kreft, J.-U., Plugge, C. M., Grimm, V., Prats, C., Leveau, J. H., Banitz, T., et al. (2013). Mighty small: observing and modeling individual microbes becomes big science. Proc. Natl. Acad. Sci. 110, 18027–18028. doi: 10.1073/pnas.1317472110
Kreuzeder, A., Santner, J., Scharsching, V., Oburger, E., Hoefer, C., Hann, S., et al. (2018). In situ observation of localized, sub-mm scale changes of phosphorus biogeochemistry in the rhizosphere. Plant Soil 424, 573–589. doi: 10.1007/s11104-017-3542-0
Kuppardt, A., Vetterlein, D., Harms, H., and Chatzinotas, A. (2010). Visualisation of gradients in arsenic concentrations around individual roots of Zea mays L. using agar-immobilized bioreporter bacteria. Plant Soil 329, 295–306. doi: 10.1007/s11104-009-0154-3
Kusari, S., Sezgin, S., Nigutova, K., Cellarova, E., and Spiteller, M. (2015). Spatial chemo-profiling of hypericin and related phytochemicals in hypericum species using MALDI-HRMS imaging. Anal. Bioanal. Chem. 407, 4779–4791. doi: 10.1007/s00216-015-8682-6
Kuzyakov, Y., and Razavi, B. S. (2019). Rhizosphere size and shape: temporal dynamics and spatial stationarity. Soil Biol. Biochem. 135, 343–360. doi: 10.1016/j.soilbio.2019.05.011
Landl, M., Schnepf, A., Vanderborght, J., Bengough, A. G., Bauke, S. L., Lobet, G., et al. (2018). Measuring root system traits of wheat in 2D images to parameterize 3D root architecture models. Plant Soil 425, 457–477. doi: 10.1007/s11104-018-3595-8
Lavelle, P., Spain, A., Blouin, M., Brown, G., Decaëns, T., Grimaldi, M., et al. (2016). Ecosystem engineers in a self-organized soil: a review of concepts and future research questions. Soil Sci. 181, 91–109. doi: 10.1097/SS.0000000000000155
Leitner, D., Klepsch, S., Ptashnyk, M., Marchant, A., Kirk, G., Schnepf, A., et al. (2010). A dynamic model of nutrient uptake by root hairs. New Phytol. 185, 792–802. doi: 10.1111/j.1469-8137.2009.03128.x
Leue, M., Ellerbrock, R. H., and Gerke, H. H. (2010). DRIFT mapping of organic matter composition at intact soil aggregate surfaces. Vadose Zone J. 9, 317–324. doi: 10.2136/vzj2009.0101
Leyser, O. (2011). Auxin, self-organisation, and the colonial nature of plants. Curr. Biol. 21, R331–R337. doi: 10.1016/j.cub.2011.02.031
Li, H., Wang, X., Rukina, D., Huang, Q., Lin, T., Sorrentino, V., et al. (2018). An integrated systems genetics and omics toolkit to probe gene function. Cell Syst. 6, 90–102. doi: 10.1016/j.cels.2017.10.016
Li, X., Jousset, A., de Boer, W., Carrión, V. J., Zhang, T., Wang, X., et al. (2019). Legacy of land use history determines reprogramming of plant physiology by soil microbiome. ISME J. 13, 738–751. doi: 10.1038/s41396-018-0300-0
Li, X., Zeng, R., and Liao, H. (2016). Improving crop nutrient efficiency through root architecture modifications. J. Integr. Plant Biol. 58, 193–202. doi: 10.1111/jipb.12434
Lobet, G., Pagès, L., and Draye, X. (2011). A novel image-analysis toolbox enabling quantitative analysis of root system architecture. Plant Physiol. 157, 29–39. doi: 10.1104/pp.111.179895
Lobet, G., Pound, M. P., Diener, J., Pradal, C., Draye, X., Godin, C., et al. (2015). Root system markup language: toward a unified root architecture description language. Plant Physiol. 167, 617–627. doi: 10.1104/pp.114.253625
Lu, J., Dijkstra, F. A., Wang, P., and Cheng, W. (2019). Roots of non-woody perennials accelerated long-term soil organic matter decomposition through biological and physical mechanisms. Soil Biol. Biochem. 134, 42–53. doi: 10.1016/j.soilbio.2019.03.015
Lucas, M., Balbín-Suárez, A., Smalla, K., and Vetterlein, D. (2018). Root growth, function and rhizosphere microbiome analyses show local rather than systemic effects in apple plant response to replant disease soil. PLoS ONE 13:e0204922. doi: 10.1371/journal.pone.0204922
Lucas, M., Pihlap, E., Steffens, M., Vetterlein, D., and Kögel-Knabner, I. (2020). Combination of imaging infrared spectroscopy and X-ray computed microtomography for the investigation of bio-and physicochemical processes in structured soils. Front. Environ. Sci. 8:42. doi: 10.3389/fenvs.2020.00042
Lucas, M., Schlüter, S., Vogel, H.-J., and Vetterlein, D. (2019a). Roots compact the surrounding soil depending on the structures they encounter. Sci. Rep. 9, 1–13. doi: 10.1038/s41598-019-52665-w
Lucas, M., Schlüter, S., Vogel, H.-J., and Vetterlein, D. (2019b). Soil structure formation along an agricultural chronosequence. Geoderma 350, 61–72. doi: 10.1016/j.geoderma.2019.04.041
Lugtenberg, B., and Kamilova, F. (2009). Plant-growth-promoting rhizobacteria. Annu. Rev. Microbiol. 63, 541–556. doi: 10.1146/annurev.micro.62.081307.162918
Lynch, J. P. (2019). Root phenotypes for improved nutrient capture: an underexploited opportunity for global agriculture. New Phytol. 223, 548–564. doi: 10.1111/nph.15738
Mai, T. H., Schnepf, A., Vereecken, H., and Vanderborght, J. (2018). Continuum multiscale model of root water and nutrient uptake from soil with explicit consideration of the 3D root architecture and the rhizosphere gradients. Plant Soil 439, 273–292. doi: 10.1007/s11104-018-3890-4
Mairhofer, S., Zappala, S., Tracy, S. R., Sturrock, C., Bennett, M., Mooney, S. J., et al. (2012). RooTrak: automated recovery of three-dimensional plant root architecture in soil from X-ray microcomputed tomography images using visual tracking. Plant Physiol. 158, 561–569. doi: 10.1104/pp.111.186221
Marschner, P., Crowley, D., and Rengel, Z. (2011). Rhizosphere interactions between microorganisms and plants govern iron and phosphorus acquisition along the root axis–model and research methods. Soil Biol. Biochem. 43, 883–894. doi: 10.1016/j.soilbio.2011.01.005
Meyer, R. C., Gryczka, C., Neitsch, C., Müller, M., Bräutigam, A., Schlereth, A., et al. (2019). Genetic diversity for nitrogen use efficiency in Arabidopsis thaliana. Planta 250, 41–57. doi: 10.1007/s00425-019-03140-3
Miguel, M. A., Postma, J. A., and Lynch, J. P. (2015). Phene synergism between root hair length and basal root growth angle for phosphorus acquisition. Plant Physiol. 167, 1430–1439. doi: 10.1104/pp.15.00145
Moradi, A. B., Carminati, A., Vetterlein, D., Vontobel, P., Lehmann, E., Weller, U., et al. (2011). Three-dimensional visualization and quantification of water content in the rhizosphere. New Phytol. 192, 653–663. doi: 10.1111/j.1469-8137.2011.03826.x
Muci, A. L., Jorquera, M. A., Ávila, Á. I., Rengel, Z., Crowley, D. E., and de la Luz Mora, M. (2012). A combination of cellular automata and agent-based models for simulating the root surface colonization by bacteria. Ecol. Modell. 247, 1–10. doi: 10.1016/j.ecolmodel.2012.07.035
Mueller, C. W., Weber, P. K., Kilburn, M. R., Hoeschen, C., Kleber, M., and Pett-Ridge, J. (2013). “Advances in the analysis of biogeochemical interfaces: nanosims to investigate soil microenvironments,” in Advances in Agronomy, Vol. 121, ed D. L. Sparks (Perth, WA: Elsevier), 1–46. doi: 10.1016/B978-0-12-407685-3.00001-3
Naveed, M., Ahmed, M., Benard, P., Brown, L., George, T., Bengough, A., et al. (2019). Surface tension, rheology and hydrophobicity of rhizodeposits and seed mucilage influence soil water retention and hysteresis. Plant Soil 437, 65–81. doi: 10.1007/s11104-019-03939-9
Neumann, G., Bott, S., Ohler, M., Mock, H.-P., Lippmann, R., Grosch, R., et al. (2014). Root exudation and root development of lettuce (Lactuca sativa L. cv. Tizian) as affected by different soils. Front. Microbiol. 5:2. doi: 10.3389/fmicb.2014.00002
Noecker, C., Eng, A., Srinivasan, S., Theriot, C. M., Young, V. B., Jansson, J. K., et al. (2016). Metabolic model-based integration of microbiome taxonomic and metabolomic profiles elucidates mechanistic links between ecological and metabolic variation. mSystems 1:e00013-15. doi: 10.1128/mSystems.00013-15
Nunan, N., Wu, K., Young, I., Crawford, J., and Ritz, K. (2002). In situ spatial patterns of soil bacterial populations, mapped at multiple scales, in an arable soil. Microb. Ecol. 44, 296–305. doi: 10.1007/s00248-002-2021-0
Oburger, E., Gruber, B., Schindlegger, Y., Schenkeveld, W. D., Hann, S., Kraemer, S. M., et al. (2014). Root exudation of phytosiderophores from soil-grown wheat. New Phytol. 203, 1161–1174. doi: 10.1111/nph.12868
Oburger, E., and Jones, D. L. (2018). Sampling root exudates–mission impossible? Rhizosphere 6, 116–133. doi: 10.1016/j.rhisph.2018.06.004
Oburger, E., and Schmidt, H. (2016). New methods to unravel rhizosphere processes. Trends Plant Sci. 21, 243–255. doi: 10.1016/j.tplants.2015.12.005
Onda, Y., and Mochida, K. (2016). Exploring genetic diversity in plants using high-throughput sequencing techniques. Curr. Genomics 17, 358–367. doi: 10.2174/1389202917666160331202742
Osmont, K. S., Sibout, R., and Hardtke, C. S. (2007). Hidden branches: developments in root system architecture. Annu. Rev. Plant Biol. 58, 93–113. doi: 10.1146/annurev.arplant.58.032806.104006
Peth, S., Nellesen, J., Fischer, G., and Horn, R. (2010). Non-invasive 3D analysis of local soil deformation under mechanical and hydraulic stresses by μCT and digital image correlation. Soil Tillage Res. 111, 3–18. doi: 10.1016/j.still.2010.02.007
Peukert, M., Matros, A., Lattanzio, G., Kaspar, S., Abadía, J., and Mock, H. P. (2012). Spatially resolved analysis of small molecules by matrix-assisted laser desorption/ionization mass spectrometric imaging (MALDI-MSI). New Phytol. 193, 806–815. doi: 10.1111/j.1469-8137.2011.03970.x
Philippot, L., Raaijmakers, J. M., Lemanceau, P., and Van Der Putten, W. H. (2013). Going back to the roots: the microbial ecology of the rhizosphere. Nature Rev. Microbiol. 11:789. doi: 10.1038/nrmicro3109
Phillips, J. D. (1998). On the relations between complex systems and the factorial model of soil formation (with discussion). Geoderma 86, 1–21. doi: 10.1016/S0016-7061(98)00054-8
Pieterse, C. M., Zamioudis, C., Berendsen, R. L., Weller, D. M., Van Wees, S. C., and Bakker, P. A. (2014). Induced systemic resistance by beneficial microbes. Annu. Rev. Phytopathol. 52, 347–375. doi: 10.1146/annurev-phyto-082712-102340
Poirier, V., Roumet, C., and Munson, A. D. (2018). The root of the matter: linking root traits and soil organic matter stabilization processes. Soil Biol. Biochem. 120, 246–259. doi: 10.1016/j.soilbio.2018.02.016
Pojasok, T., and Kay, B. (1990). Effect of root exudates from corn and bromegrass on soil structural stability. Can. J. Soil Sci. 70, 351–362. doi: 10.4141/cjss90-036
Portell, X., Pot, V., Garnier, P., Otten, W., and Baveye, P. C. (2018). Microscale heterogeneity of the spatial distribution of organic matter can promote bacterial biodiversity in soils: insights from computer simulations. Front. Microbiol. 9:1583. doi: 10.3389/fmicb.2018.01583
Pound, M. P., French, A. P., Atkinson, J. A., Wells, D. M., Bennett, M. J., and Pridmore, T. (2013). RootNav: navigating images of complex root architectures. Plant Physiol. 162, 1802–1814. doi: 10.1104/pp.113.221531
Railsback, S. F., and Grimm, V. (2019). Agent-Based and Individual-Based Modeling: A Practical Introduction. Princeton, NJ: Princeton University Press.
Rasse, D. P., Rumpel, C., and Dignac, M.-F. (2005). Is soil carbon mostly root carbon? Mechanisms for a specific stabilisation. Plant Soil 269, 341–356. doi: 10.1007/s11104-004-0907-y
Raynaud, X., and Nunan, N. (2014). Spatial ecology of bacteria at the microscale in soil. PLoS ONE 9:e87217. doi: 10.1371/journal.pone.0087217
Reinhold-Hurek, B., Bünger, W., Burbano, C. S., Sabale, M., and Hurek, T. (2015). Roots shaping their microbiome: global hotspots for microbial activity. Annu. Rev. Phytopathol. 53, 403–424. doi: 10.1146/annurev-phyto-082712-102342
Rellán-Álvarez, R., Lobet, G., Lindner, H., Pradier, P.-L., Sebastian, J., Yee, M.-C., et al. (2015). GLO-roots: an imaging platform enabling multidimensional characterization of soil-grown root systems. eLife 4:e07597. doi: 10.7554/eLife.07597.058
Rillig, M. C., Muller, L. A., and Lehmann, A. (2017). Soil aggregates as massively concurrent evolutionary incubators. ISME J. 11, 1943–1948. doi: 10.1038/ismej.2017.56
Rillig, M. C., and Mummey, D. L. (2006). Mycorrhizas and soil structure. New Phytol. 171, 41–53. doi: 10.1111/j.1469-8137.2006.01750.x
Rodionov, A., Lehndorff, E., Stremtan, C. C., Brand, W. A., Königshoven, H.-P., and Amelung, W. (2019). Spatial microanalysis of natural 13C/12C abundance in environmental samples using laser ablation-isotope ratio mass spectrometry. Anal. Chem. 91, 6225–6232. doi: 10.1021/acs.analchem.9b00892
Roose, T., Fowler, A., and Darrah, P. (2001). A mathematical model of plant nutrient uptake. J. Math. Biol. 42, 347–360. doi: 10.1007/s002850000075
Roose, T., Keyes, S., Daly, K., Carminati, A., Otten, W., Vetterlein, D., et al. (2016). Challenges in imaging and predictive modeling of rhizosphere processes. Plant Soil 407, 9–38. doi: 10.1007/s11104-016-2872-7
Rossmann, M., Pérez-Jaramillo, J. E., Kavamura, V. N., Chiaramonte, J. B., Dumack, K., Fiore-Donno, A. M., et al. (2020). Multitrophic interactions in the rhizosphere microbiome of wheat: from bacteria and fungi to protists. FEMS Microbiol. Ecol. 96:fiaa032. doi: 10.1093/femsec/fiaa032
Saatz, J., Stryhanyuk, H., Vetterlein, D., Musat, N., Otto, M., Reemtsma, T., et al. (2016). Location and speciation of gadolinium and yttrium in roots of Zea mays by LA-ICP-MS and ToF-SIMS. Environ. Pollut. 216, 245–252. doi: 10.1016/j.envpol.2016.05.069
Santner, J., Zhang, H., Leitner, D., Schnepf, A., Prohaska, T., Puschenreiter, M., et al. (2012). High-resolution chemical imaging of labile phosphorus in the rhizosphere of Brassica napus L. cultivars. Environ. Exp. Bot. 77, 219–226. doi: 10.1016/j.envexpbot.2011.11.026
Sapp, M., Ploch, S., Fiore-Donno, A. M., Bonkowski, M., and Rose, L. E. (2018). Protists are an integral part of the Arabidopsis thaliana microbiome. Environ. Microbiol. 20, 30–43. doi: 10.1111/1462-2920.13941
Schampera, B., Solc, R., Woche, S., Mikutta, R., Dultz, S., Guggenberger, G., et al. (2015). Surface structure of organoclays as examined by X-ray photoelectron spectroscopy and molecular dynamics simulations. Clay Miner. 50, 353–367. doi: 10.1180/claymin.2015.050.3.08
Schlüter, S., Blaser, S. R., Weber, M., Schmidt, V., and Vetterlein, D. (2018). Quantification of root growth patterns from the soil perspective via root distance models. Front. Plant Sci. 9:1084. doi: 10.3389/fpls.2018.01084
Schlüter, S., Eickhorst, T., and Mueller, C. W. (2019). Correlative imaging reveals holistic view of soil microenvironments. Environ. Sci. Technol. 53, 829–837. doi: 10.1021/acs.est.8b05245
Schlüter, S., Sheppard, A., Brown, K., and Wildenschild, D. (2014). Image processing of multiphase images obtained via X-ray microtomography: a review. Water Resour. Res. 50, 3615–3639. doi: 10.1002/2014WR015256
Schmidt, H., Eickhorst, T., and Mußmann, M. (2012). Gold-FISH: a new approach for the in situ detection of single microbial cells combining fluorescence and scanning electron microscopy. Syst. Appl. Microbiol. 35, 518–525. doi: 10.1016/j.syapm.2012.04.006
Schmidt, H., Nunan, N., Höck, A., Eickhorst, T., Kaiser, C., Woebken, D., et al. (2018). Recognizing patterns: spatial analysis of observed microbial colonization on root surfaces. Front. Environ. Sci. 6:61. doi: 10.3389/fenvs.2018.00061
Schnepf, A., Huber, K., Landl, M., Meunier, F., Petrich, L., and Schmidt, V. (2018a). Statistical characterization of the root system architecture model CRootBox. Vadose Zone J. 17, 1–11. doi: 10.2136/vzj2017.12.0212
Schnepf, A., Leitner, D., Landl, M., Lobet, G., Mai, T. H., Morandage, S., et al. (2018b). CRootBox: a structural–functional modelling framework for root systems. Ann. Bot. 121, 1033–1053. doi: 10.1093/aob/mcx221
Schnepf, A., Roose, T., and Schweiger, P. (2008). Impact of growth and uptake patterns of arbuscular mycorrhizal fungi on plant phosphorus uptake–a modelling study. Plant Soil 312, 85–99. doi: 10.1007/s11104-008-9749-3
Schreiter, S., Ding, G.-C., Heuer, H., Neumann, G., Sandmann, M., Grosch, R., et al. (2014). Effect of the soil type on the microbiome in the rhizosphere of field-grown lettuce. Front. Microbiol. 5:144. doi: 10.3389/fmicb.2014.00144
Schulz, M., Stonestrom, D., Lawrence, C., Bullen, T., Fitzpatrick, J., Kyker-Snowman, E., et al. (2016). Structured heterogeneity in a marine terrace chronosequence: upland mottling. Vadose Zone J. 15, 1–14. doi: 10.2136/vzj2015.07.0102
Schwarz, C., Gourgue, O., Van Belzen, J., Zhu, Z., Bouma, T. J., Van De Koppel, J., et al. (2018). Self-organization of a biogeomorphic landscape controlled by plant life-history traits. Nat. Geosci. 11, 672–677. doi: 10.1038/s41561-018-0180-y
Segal, E., Kushnir, T., Mualem, Y., and Shani, U. (2008). Water uptake and hydraulics of the root hair rhizosphere. Vadose Zone J. 7, 1027–1034. doi: 10.2136/vzj2007.0122
Simard, S. W. (2009). The foundational role of mycorrhizal networks in self-organization of interior douglas-fir forests. For. Ecol. Manage. 258, S95–S107. doi: 10.1016/j.foreco.2009.05.001
Sokol, N. W., Sanderman, J., and Bradford, M. A. (2019). Pathways of mineral-associated soil organic matter formation: integrating the role of plant carbon source, chemistry, and point of entry. Glob. Chang. Biol. 25, 12–24. doi: 10.1111/gcb.14482
Solomon, D., Lehmann, J., Harden, J., Wang, J., Kinyangi, J., Heymann, K., et al. (2012). Micro-and nano-environments of carbon sequestration: multi-element STXM–NEXAFS spectromicroscopy assessment of microbial carbon and mineral associations. Chem. Geol. 329, 53–73. doi: 10.1016/j.chemgeo.2012.02.002
Spohn, M., Carminati, A., and Kuzyakov, Y. (2013). Soil zymography–a novel in situ method for mapping distribution of enzyme activity in soil. Soil Biol. Biochem. 58, 275–280. doi: 10.1016/j.soilbio.2012.12.004
Spohn, M., and Kuzyakov, Y. (2014). Spatial and temporal dynamics of hotspots of enzyme activity in soil as affected by living and dead roots—a soil zymography analysis. Plant Soil 379, 67–77. doi: 10.1007/s11104-014-2041-9
Stelpflug, S. C., Sekhon, R. S., Vaillancourt, B., Hirsch, C. N., Buell, C. R., de Leon, N., et al. (2016). An expanded maize gene expression atlas based on RNA sequencing and its use to explore root development. Plant Genome 9, 1–15. doi: 10.3835/plantgenome2015.04.0025
Symonova, O., Topp, C. N., and Edelsbrunner, H. (2015). DynamicRoots: a software platform for the reconstruction and analysis of growing plant roots. PLoS ONE 10:e0127657. doi: 10.1371/journal.pone.0127657
Teixeira, P. J. P., Colaianni, N. R., Fitzpatrick, C. R., and Dangl, J. L. (2019). Beyond pathogens: microbiota interactions with the plant immune system. Curr. Opin. Microbiol. 49, 7–17. doi: 10.1016/j.mib.2019.08.003
Tisdall, J. M. (1996). “Formation of soil aggregates and accumulation of soil organic matter,” in Structure and Organic Matter Storage in Agricultural Soils, eds M. R. Carter and B. A. Stewart (Boca Raton, FL: Lewis), 57–96.
Tisdall, J. M., and Oades, J. M. (1982). Organic matter and water-stable aggregates in soils. Eur. J. Soil Sci. 33, 141–163. doi: 10.1111/j.1365-2389.1982.tb01755.x
Totsche, K. U., Amelung, W., Gerzabek, M. H., Guggenberger, G., Klumpp, E., Knief, C., et al. (2018). Microaggregates in soils. J. Plant Nutr. Soil Sci. 181, 104–136. doi: 10.1002/jpln.201600451
Tötzke, C., Kardjilov, N., Manke, I., and Oswald, S. E. (2017). Capturing 3D water flow in rooted soil by ultra-fast neutron tomography. Sci. Rep. 7, 1–9. doi: 10.1038/s41598-017-06046-w
Verbon, E. H., Trapet, P. L., Stringlis, I. A., Kruijs, S., Bakker, P. A., and Pieterse, C. M. (2017). Iron and immunity. Annu. Rev. Phytopathol. 55, 355–375. doi: 10.1146/annurev-phyto-080516-035537
Vetterlein, D., and Doussan, C. (2016). Root age distribution: how does it matter in plant processes? A focus on water uptake. Plant Soil 407, 145–160. doi: 10.1007/s11104-016-2849-6
Vetterlein, D., Lippold, E., Schreiter, S., Phalempin, M., Fahrenkampf, T., and Hochholdinger, F. et al. (in press). Experimental platforms for the investigation of spatiotemporal patterns in the rhizosphere—laboratory and field scale. J. Plant Nutr. Soil Sci. doi: 10.1002/jpln.202000079.
Vidal, A., Hirte, J., Bender, S. F., Mayer, J., Gattinger, A., Höschen, C., et al. (2018). Linking 3D soil structure and plant-microbe-soil carbon transfer in the rhizosphere. Front. Environ. Sci. 6:9. doi: 10.3389/fenvs.2018.00009
Vogel, H.-J., Weller, U., and Schlüter, S. (2010). Quantification of soil structure based on minkowski functions. Comput. Geosci. 36, 1236–1245. doi: 10.1016/j.cageo.2010.03.007
Voges, M. J., Bai, Y., Schulze-Lefert, P., and Sattely, E. S. (2019). Plant-derived coumarins shape the composition of an Arabidopsis synthetic root microbiome. Proc. Natl. Acad. Sci. 116, 12558–12565. doi: 10.1073/pnas.1820691116
Vollsnes, A., Futsaether, C., and Bengough, A. (2010). Quantifying rhizosphere particle movement around mutant maize roots using time-lapse imaging and particle image velocimetry. Eur. J. Soil Sci. 61, 926–939. doi: 10.1111/j.1365-2389.2010.01297.x
Von Felten, A., Défago, G., and Maurhofer, M. (2010). Quantification of Pseudomonas fluorescens strains F113, CHA0 and Pf153 in the rhizosphere of maize by strain-specific real-time PCR unaffected by the variability of DNA extraction efficiency. J. Microbiol. Methods 81, 108–115. doi: 10.1016/j.mimet.2010.02.003
Vorholt, J. A., Vogel, C., Carlström, C. I., and Müller, D. B. (2017). Establishing causality: opportunities of synthetic communities for plant microbiome research. Cell Host Microbe 22, 142–155. doi: 10.1016/j.chom.2017.07.004
Wang, X. J., Sale, P., Hayden, H., Tang, C., Clark, G., and Armstrong, R. (2020). Plant roots and deep-banded nutrient-rich amendments influence aggregation and dispersion in a dispersive clay subsoil. Soil Biol. Biochem. 141:107664. doi: 10.1016/j.soilbio.2019.107664
Watson, B. S., Bedair, M. F., Urbanczyk-Wochniak, E., Huhman, D. V., Yang, D. S., Allen, S. N., et al. (2015). Integrated metabolomics and transcriptomics reveal enhanced specialized metabolism in Medicago truncatula root border cells. Plant Physiol. 167, 1699–1716. doi: 10.1104/pp.114.253054
Watt, M., Silk, W. K., and Passioura, J. B. (2006). Rates of root and organism growth, soil conditions, and temporal and spatial development of the rhizosphere. Ann. Bot. 97, 839–855. doi: 10.1093/aob/mcl028
Wiegand, T., and Moloney, K. A. (2013). Handbook of Spatial Point-Pattern Analysis in Ecology. New York, NY: Chapman and Hall/CRC. doi: 10.1201/b16195
Wildenschild, D., and Sheppard, A. P. (2013). X-ray imaging and analysis techniques for quantifying pore-scale structure and processes in subsurface porous medium systems. Adv. Water Resour. 51, 217–246. doi: 10.1016/j.advwatres.2012.07.018
Xiong, W., Jousset, A., Guo, S., Karlsson, I., Zhao, Q., Wu, H., et al. (2018). Soil protist communities form a dynamic hub in the soil microbiome. ISME J. 12, 634–638. doi: 10.1038/ismej.2017.171
Xu, J., Zhang, Y., Zhang, P., Trivedi, P., Riera, N., Wang, Y., et al. (2018). The structure and function of the global citrus rhizosphere microbiome. Nat. Commun. 9, 1–10. doi: 10.1038/s41467-018-07343-2
York, L. M., Carminati, A., Mooney, S. J., Ritz, K., and Bennett, M. J. (2016). The holistic rhizosphere: integrating zones, processes, and semantics in the soil influenced by roots. J. Exp. Bot. 67, 3629–3643. doi: 10.1093/jxb/erw108
Young, I. M. (1998). Biophysical interactions at the root–soil interface: a review. J. Agric. Sci. 130, 1–7. doi: 10.1017/S002185969700498X
Young, I. M., and Crawford, J. W. (2004). Interactions and self-organization in the soil-microbe complex. Science 304, 1634–1637. doi: 10.1126/science.1097394
Yu, P., Wang, C., Baldauf, J. A., Tai, H., Gutjahr, C., Hochholdinger, F., et al. (2018). Root type and soil phosphate determine the taxonomic landscape of colonizing fungi and the transcriptome of field-grown maize roots. New Phytol. 217, 1240–1253. doi: 10.1111/nph.14893
Zhalnina, K., Louie, K. B., Hao, Z., Mansoori, N., da Rocha, U. N., Shi, S., et al. (2018). Dynamic root exudate chemistry and microbial substrate preferences drive patterns in rhizosphere microbial community assembly. Nature Microbiol. 3, 470–480. doi: 10.1038/s41564-018-0129-3
Zickenrott, I. M., Woche, S. K., Bachmann, J., Ahmed, M. A., and Vetterlein, D. (2016). An efficient method for the collection of root mucilage from different plant species—a case study on the effect of mucilage on soil water repellency. J. Plant Nutr. Soil Sci. 179, 294–302. doi: 10.1002/jpln.201500511
Ziegler, J., Schmidt, S., Chutia, R., Müller, J., Böttcher, C., Strehmel, N., et al. (2016). Non-targeted profiling of semi-polar metabolites in Arabidopsis root exudates uncovers a role for coumarin secretion and lignification during the local response to phosphate limitation. J. Exp. Bot. 67, 1421–1432. doi: 10.1093/jxb/erv539
Zitova, B., and Flusser, J. (2003). Image registration methods: a survey. Image Vision Comput. 21, 977–1000. doi: 10.1016/S0262-8856(03)00137-9
Keywords: root, resilience, pattern analyses, resource efficiency, nutrient acquisition, soil structure, maize, self-organization
Citation: Vetterlein D, Carminati A, Kögel-Knabner I, Bienert GP, Smalla K, Oburger E, Schnepf A, Banitz T, Tarkka MT and Schlüter S (2020) Rhizosphere Spatiotemporal Organization–A Key to Rhizosphere Functions. Front. Agron. 2:8. doi: 10.3389/fagro.2020.00008
Received: 23 March 2020; Accepted: 22 June 2020;
Published: 28 July 2020.
Edited by:
Antonio Rafael Sánchez-Rodríguez, University of Cordoba, SpainReviewed by:
Huadong Zang, China Agricultural University, ChinaJessica L. M. Gutknecht, University of Minnesota Twin Cities, United States
Zhenke Zhu, Institute of Subtropical Agriculture (CAS), China
Copyright © 2020 Vetterlein, Carminati, Kögel-Knabner, Bienert, Smalla, Oburger, Schnepf, Banitz, Tarkka and Schlüter. This is an open-access article distributed under the terms of the Creative Commons Attribution License (CC BY). The use, distribution or reproduction in other forums is permitted, provided the original author(s) and the copyright owner(s) are credited and that the original publication in this journal is cited, in accordance with accepted academic practice. No use, distribution or reproduction is permitted which does not comply with these terms.
*Correspondence: Doris Vetterlein, ZG9yaXMudmV0dGVybGVpbiYjeDAwMDQwO3Vmei5kZQ==