- School of Sustainable Engineering and The Built Environment, Arizona State University, Tempe, AZ, United States
Pervasive and accelerating climatic, technological, social, economic, and institutional change dictate that the challenges of the future will likely be vastly different and more complex than they are today. As our infrastructure systems (and their surrounding environment) become increasingly complex and beyond the cognitive understanding of any group of individuals or institutions, artificial intelligence (AI) may offer critical cognitive insights to ensure that systems adapt, services continue to be provided, and needs continue to be met. This paper conceptually links AI to various tasks and leadership capabilities in order to critically examine potential roles that AI can play in the management and implementation of infrastructure systems under growing complexity and uncertainty. Ultimately, various AI techniques appear to be increasingly well-suited to make sense of and operate under both stable (predictable) and chaotic (unpredictable) conditions. The ability to dynamically and continuously shift between stable and chaotic conditions is critical for effectively navigating our complex world. Thus, moving forward, a key adaptation for engineers will be to place increasing emphasis on creating the structural, financial, and knowledge conditions for enabling this type of flexibility in our integrated human-AI-infrastructure systems. Ultimately, as AI systems continue to evolve and become further embedded in our infrastructure systems, we may be implicitly or explicitly releasing control to algorithms. The potential benefits of this arrangement may outweigh the drawbacks. However, it is important to have open and candid discussions about the potential implications of this shift and whether or not those implications are desirable.
Introduction
If future infrastructure resembles that of the past, or even of today, it will represent a profound failure on the part of engineers and infrastructure managers. Pervasive and accelerating climatic, technological, social, economic, and institutional change signal that the challenges of the future will likely be vastly different and more complex than they are today (Allenby, 2011; Marchant et al., 2011; Markolf et al., 2018). The relationship of the human species to the planet is changing dramatically given a rapidly urbanizing global population of roughly 7.7 billion, and a parallel growing middle class with changing consumption and food demands. These dynamics play a key role in driving and accelerating the integration of human, natural, and built systems to create complex, interlinked, and rapidly evolving systems at all scales—from local infrastructure to regional and global systems (Lo and Yeung, 1998; NRC (US National Research Council)., 2003; Chester et al., 2019).
The need for infrastructure to adapt, transform, and perform competently under conditions of complexity and accelerating change is increasingly being met by integrating infrastructure and information systems [including various artificial intelligence (AI) capabilities] into infrastructure design, construction, operation, and maintenance. However, successfully implementing this strategy requires a clear and concise understanding of relevant information, communication, and computational frameworks, as well as how they functionally couple together in practice—a particularly difficult task in today's environment. Therefore, it is not surprising that the rise of a new global infrastructure with profound implications for humans, their institutions, and their planet has gone both unperceived and unremarked. This is the cognitive infrastructure, and it already permeates virtually every aspect of our world (Allenby, 2019). In particular, each infrastructure system and sector has its own companies, experts, investors, and users. But what is often not recognized is that many of these infrastructures and technologies are not only coherent entities themselves, but also being integrated into an emergent infrastructure that includes integrated functionality from many sources: the “cognitive infrastructure.”
Taking a functional definition of “cognition” (i.e., information processing, reasoning, remembering, learning, problem-solving, decision-making, etc.) (Squire, 2009), the accelerating rise of cognitive infrastructure becomes evident. For example, machine-to-machine connections are anticipated to increase from 6.1 billion in 2018 to 14.7 billion in 2023 (Cisco, 2020). Similarly, spending on sensors and other technologies related to the Internet-of-Things (IoT) is expected to reach $1.2 Trillion in 2022 (Columbus, 2018). Most of these sensors and devices will generate vast amounts of data and integrate some cognitive capability via accelerating deployment of AI technology such as neural nets (Lee, 2018). In short, accelerating capability and capacity across a number of apparently unrelated infrastructures and technologies is generating an infrastructure, tied together by AI and a vast array of institutional structures, that (1) contains the functional components of cognition and ever-more powerful networks operationally linking them together, (2) is distributed around the world, and (3) contains evolving and emergent systemic and behavioral capabilities. Simply put, we are building a pervasive cognitive infrastructure without fully recognizing it, and we are doing so rapidly and at global scale.
Cognitive infrastructure offers challenges that more traditional infrastructure systems do not. For one, it operates at a level that humans can neither fully understand nor perceive—people are relatively low bandwidth cognitive mechanisms in a world where even contemporary cognitive infrastructure operates at far higher bandwidths, much faster speeds, and higher levels of complexity than individuals can access. This can unfortunately be seen in the tragic Lion Air Flight 610 and Ethiopian Airlines Flight 302 incidents. Although many factors appeared to have been at play, the disconnect between the development of the automated flight control systems in the Boeing 737-MAX planes and the training and implementation by the pilots was a key element in the accidents (Gelles, 2019; Wise, 2019; Herkert et al., 2020; U.S. House Committee on Transportation Infrastructure., 2020). Thus, determining how to effectively integrate human and machine cognition into infrastructure systems becomes a significant professional challenge that, so far, appears to have not been adequately and effectively considered.
Integrating cognitive infrastructure is a critical capability as engineers, technologists, and policymakers try to develop infrastructure systems that are as resilient, agile, and adaptive as current (and future) conditions demand. But knowing that incorporating sensor and AI-driven adaptability into infrastructure can make it more efficient and responsive to changing conditions is only the beginning. Understanding the cognitive infrastructure as a whole is required to fully and responsibly meet the demands for better infrastructure. For example, designers of IoT devices embed sensors and communication capabilities in their products as a matter of required functionality. But, absent a systemic perspective on security and the devices' place within the overarching cognitive infrastructure, there is the potential for underappreciating/misunderstanding issues like the vulnerability to adversarial attacks that the embrace of AI technologies can create. These potential drawbacks are ultimately a symptom of understanding a few of the constituent technologies (e.g., AI) in isolation, but failing to understand that it is the cognitive infrastructure, not just those individual technologies, that their infrastructure design is integrating.
It is premature to consider tantalizing questions such as how humans should respond as critical cognitive functions migrate to higher level techno-human systems embedded in a global cognitive infrastructure. However, it is not premature to recognize that this new infrastructure, itself a reflection and driver of the complexity and challenges of the Anthropocene, is already emergent. Additionally, trying to perceive and understand some of these implications is an increasingly imperative and necessary professional responsibility. Without that first step, ethical, rational, and appropriate infrastructure design, construction, operation, maintenance (as well as the educational and institutional structures to support them) will remain beyond reach. As such, this paper provides a broad discussion about what AI is and how it relates to infrastructure. We then explore various tasks and services within infrastructure systems that may be enhanced and/or replaced by AI. Finally, we conclude with a discussion of some of the broader implications that may emerge as AI and infrastructure systems become increasingly entwined in the coming decades.
AI and Infrastructure Leadership in the Context of Complexity
“AI” is a fuzzy term. As the U. S. National Science and Technology Council says in its 2016 report, “There is no single definition of AI that is universally accepted by practitioners. Some define AI loosely as a computerized system that exhibits behavior that is commonly thought of as requiring intelligence. Others define AI as a system capable of rationally solving complex problems or taking appropriate actions to achieve its goals in whatever real world circumstances it encounters.” Herein, we use “AI” to include big data and analytics dimensions, but ultimately describe the leadership and intelligence capabilities that are needed to replace or augment people. In doing so we envision a future where humans employ AI to make sense of an increasingly complex world.
In managing dynamic and complex systems and environments, several leadership capabilities are needed to address continually changing conditions (Uhl-Bien et al., 2007). Administrative Leadership, what we largely practice today, is well-suited for stable conditions and is made up of bureaucracies that formalize the structure and function of organizations. However, in the changing or chaotic conditions that define complex environments, Adaptive Leadership is preferred. Under this approach, adaptability, creativity, and learning are emphasized in order to make sense of and navigate complex and uncertain conditions. Perhaps of most importance is Enabling Leadership, the ability to shift between Administrative and Adaptive Leadership practices as conditions shift from stable to chaotic. Enabling Leadership involves creating structural, financial, and knowledge conditions for flexibility (Uhl-Bien et al., 2007). In assessing the AI landscape, evaluating which techniques are best positioned to support each leadership style is increasingly useful.
Given this context, there are several tasks for which AI applications in infrastructure are well-suited, including pattern recognition, classification, clustering, categorization, system control, function approximation (e.g., regression analysis), optimization, and prediction/forecasting (Chen et al., 2008; Brynjolfsson and Mcafee, 2017; Eggimann et al., 2017). In order to accomplish these tasks, a variety of techniques and approaches can be applied, such as rule-based systems (RBS), genetic algorithms, cellular automata, Fuzzy Systems, Multi-agent systems, Swarm Intelligence, Case-based reasoning (CBR), and Artificial Neural Networks (ANN) (Chen et al., 2008). For example, AI (particularly genetic algorithms, Artificial Neural Networks, and Deep Learning) has been applied in a variety of civil engineering contexts including optimum design of structures (Hajela and Berke, 1991; Adeli and Park, 1995; Camp et al., 2003; Hadi, 2003), concrete strength modeling (Yeh, 1999; Ni and Wang, 2000; Lee and Ahn, 2003; Al-Salloum et al., 2012), predicting geotechnical settlement and liquefaction (Shahin et al., 2002; Young-Su and Byung-Tak, 2006), earthquake engineering (Lee and Han, 2002; Arslan, 2010; Yilmaz, 2011), concrete design mix (Jayaram et al., 2009), prediction and forecasting of water resources and flooding (Maier and Dandy, 2000; Mitra et al., 2016; Alexander et al., 2018; Lin et al., 2018; Yu et al., 2018; Zamanisabzi et al., 2018; Li et al., 2019), water quality and sediment modeling (Nagy et al., 2002; Zhang et al., 2010; Barzegar et al., 2016; Sabouri et al., 2016), irrigation and water-delivery scheduling (Nixon et al., 2001; Karasekreter et al., 2013), rainfall-runoff modeling (Minns and Hall, 1996; Tokar and Johnson, 1999; Cheng et al., 2005, 2017; Dixon, 2005; Jeong and Kim, 2005; Abrahart and See, 2007; Young et al., 2017), and evapo-transpiration modeling (Tabari et al., 2010; Kumar et al., 2020)—additional examples can also be found in Figure 1 (e.g., Liu et al., 2016; Mounce et al., 2016; Amanollahi et al., 2017; Beh et al., 2017; Conniff, 2017; Ghalehkhondabi et al., 2017; Matias, 2017; Rezaeianzadeh et al., 2017; Yang et al., 2017; Zhang et al., 2017, 2018; Corominas et al., 2018; Pisa et al., 2019; Rastegaripour et al., 2019; Suh, 2019). The scope and purpose of this article is not to provide a comprehensive overview and discussion of these different techniques. For that, we refer the readers to works by Flood and Kartam, 1994a,b; Kartam et al., 1997; Adeli, 2001; Flood, 2001; Flintsch and Chen, 2004; Chandwani et al., 2013; Ye et al., 2019); and (Falcone et al., 2020). Nonetheless, a brief discussion about the ways in which various AI techniques may (or may not) support infrastructure leadership in stable and chaotic environments appears warranted and is included below.
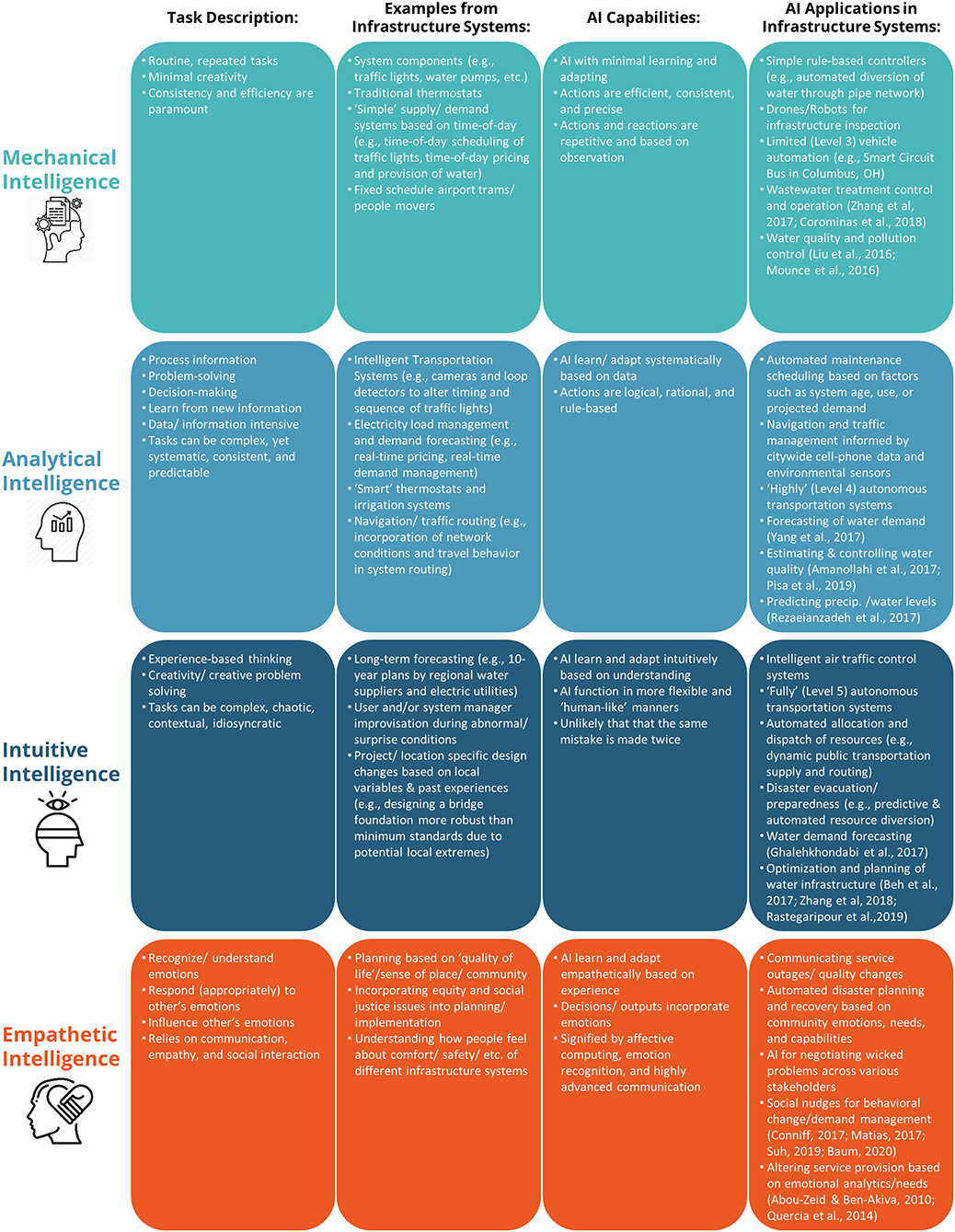
Figure 1. Summary of the “Four Intelligences,” examples from infrastructure systems, and current/potential applications of AI to infrastructure systems across each level of intelligence.
Some AI techniques may be well-suited for enhancing operations during stable conditions, while others may be more appropriate for supporting leadership during unstable times (e.g., extreme events, funding uncertainty, pandemics, etc.). For example, techniques that establish algorithms to solve novel problems by recalling and referencing similar problems from the past (e.g., CBR) are particularly suitable for the well-defined and stable conditions endemic of Administrative Leadership. In this context, these approaches can be particularly useful for applications related to system control, planning, prediction, and diagnosis (Chen et al., 2008). Conversely, techniques that mimic the manner in which human brains process information via a series of layered and interconnected processing units (e.g., ANN) are increasingly well-suited for the complex, data-intensive, multivariable, and dynamic conditions (i.e., instability) that warrant Adaptive Leadership. In this context, AI can help make predictions (based on a series of input patterns) and/or intuit relationships between various inputs—even in situations where the underlying rules and structure of the problem may be unknown or hard to express (Chen et al., 2008). Overall, various forms of AI appear poised to greatly complement (or even in some cases replace) Administrative and Adaptive Leadership activities and roles within our infrastructure systems. In turn, the humans and institutions that interact with and govern our infrastructure systems may play an increasingly important role as the primary source of Enabling Leadership within our systems. Thus, it will be crucial for humans and institutions to recognize the benefits and tradeoffs among the different types of leadership, roles, and services provided by various AI. Perhaps most importantly, additional consideration appears warranted regarding the frameworks, resources, structures, and knowledge systems that may be needed to facilitate the smooth and agile transition between leadership approaches as future conditions continually fluctuate between stable and chaotic. The following section explores this issue further by examining some of the various roles and tasks AI may fill in infrastructure systems moving forward.
AI Intelligences and Tasks Within Infrastructure Systems
Evaluating the potential for AI to augment or replace existing capabilities requires a critical examination of the intelligences involved. Huang and Rust (2018) assert that AI job replacement fundamentally occurs at the task level, and that “lower” intelligence tasks (e.g., repetitive, routine tasks) are easier for AI to replace than “higher” intelligence tasks (e.g., highly emotional/empathetic tasks). Given that, at their core, infrastructure systems are service providers, we adapt Huang and Rust's framework to (1) link various infrastructure services to the four types of intelligences described by Huang and Rust (i.e., Mechanical, Analytical, Intuitive, and Empathetic), and (2) outline cases (and examples where possible) of how AI has and/or could potentially replace various infrastructure-related tasks at each level of intelligence—see Figure 1.
Mechanical Intelligence
The “lowest” level of intelligence is Mechanical, which is defined by routine and repeated tasks, minimal creativity, and an emphasis on efficiency and consistency (Huang and Rust, 2018). AI at this level are rule-based and are well-suited for homogenous tasks that are repetitive, performed often, and unsophisticated (Sawhney, 2016; Huang and Rust, 2018). As a result, AI at this level often have an advantage over humans with respect to consistency, reliability, and work-rate (Huang and Rust, 2018).
One of the primary challenges associated with Mechanical AI is that it can be difficult to scale to the systems level, which in turn can limit its applicability to the large-scale and dynamic infrastructure systems typical of modern cities. Mechanical tasks are typically conducted by a single unit (or small, tightly integrated group of components). As a result, this type of AI is best suited for well-bounded and tightly constrained situations. Thus, increasing the network, scale, and/or state of operations adds complexity that can eventually overwhelm the system. Under these circumstances, AI at higher levels of intelligence will likely be more appropriate and effective.
Analytical Intelligence
The second level of intelligence is Analytical, which relies on the ability to process information, make decisions, problem solve, and adjust to new information (Huang and Rust, 2018). Analytical Intelligence is defined by tasks that can be complex (often data-intensive), yet consistent and predictable. AI at this level use algorithms to iteratively learn and gain insights from large and/or continuous data sets. Analytical AI increasingly consist of networked units rather than a stand-alone machine. Human interpretation and intuition are still vital complements to AI at this level. AI provides increasingly varied and valuable decision support, but humans are still the ones ultimately making the decision.
One of the biggest potential challenges with Analytical AI is that it is likely not well-suited for problems that do not have similar analogs from the past (Chen et al., 2008). This drawback is particularly important to consider in the context of managing infrastructure systems under a changing climate. Non-stationarity, the concept that past conditions and data are not indicative of future trends and conditions, is increasingly a reality for urban and infrastructure systems (Milly et al., 2008; Koutsoyiannis, 2011; Lins, 2012). Thus, Analytical AI should not be treated as an “off-the-shelf” or “plug-and-play” solution for a wide range of problems. Engineers and infrastructure managers should take great care to understand the nuances, strengths, and weaknesses of AI when applying it to infrastructure that has significant interaction with climatic variables (e.g., weather prediction, stormwater systems, flood management systems, etc.).
Intuitive Intelligence
The next level of intelligence is Intuitive, which relies on experience-based thinking and creativity. Tasks related to Intuitive Intelligence are contextual, chaotic, complex, and idiosyncratic (Huang and Rust, 2018). AI at this level function in a more human-like manner by learning and adapting based on previous experience and new information. Understanding a problem or situation based on context and prior experience is a hallmark characteristic of Intuitive Intelligence in both humans and AI.
One potential challenge with Intuitive AI is that the problems to which it may be applied are often “wickedly complex” and do not have one “right” solution (e.g., the allocation and management of natural resources) (Chester and Allenby, 2019a). The algorithms supporting this type of AI often learn from human-defined data as to what the outcome should be. Thus, the training of and learning by the AI can be severely inhibited in situations where the outcome/solution is not clear (Meserole, 2018). Under these circumstances, AI can still be very helpful in generating, exploring, and analyzing various scenarios. However, human stakeholders will ultimately be responsible for deciding on the final outcomes or course of action.
Another potential challenge associated with Intuitive AI is that there can be a “black-box” element to the analysis and outcomes due to the fact that it provides solutions and insights with minimal knowledge of the underlying systems and processes (Chen et al., 2008). For example, the AI may produce outputs that are non-intuitive and/or fail to converge on a solution, and it may be difficult to ascertain why. Ultimately, some level of this “black box” is likely unavoidable. Presumably, one of the main reasons to deploy Intuitive AI is because the system in question is already operating at a scale and/or level of complexity beyond human cognitive capabilities. If total understanding and mastery of system dynamics and complexity (i.e., elimination of the “black box”) is achievable, then Intuitive AI was likely not needed in the first place. Thus, the critical question is not “how do we eliminate the black-box?,” but rather, “what degree of black-box are we comfortable with?” As AI systems continue to evolve and become further embedded in our infrastructure systems, we may be implicitly or explicitly releasing control of our infrastructure systems to software and algorithms. The potential benefits of this arrangement may very well outweigh the drawbacks in certain circumstances. However, it is important for communities, policy-makers, and infrastructure managers to have open and candid discussions about the potential implications of this shift in control and whether or not those implications are desirable.
Empathetic Intelligence
The “highest” level of intelligence is Empathetic, which relies on empathy, social interaction, and communication. Empathetic tasks relate to the ability to understand emotions, appropriately respond to emotions in others, and influence other's emotions (Huang and Rust, 2018). AI at this level “relates to, arises from, or influences emotions (Picard, 1995),” and behaves as if it has feeling. Empathetic AI are still in the nascent stages of development, with initial applications tending to relate to emotional analytics (Abou-Zeid and Ben-Akiva, 2010; Quercia et al., 2014). Nonetheless, the high level of social and communication skills needed for Empathetic Intelligence seem to indicate that humans will remain integral at this level for the foreseeable future.
Similar to Intuitive AI, aspects of wicked complexity and wicked problems can be especially challenging for Empathetic AI. One of the elements of a wickedly complex problem is the presence of a wide degree of norms and values among the various stakeholders within the system. These values/interests may not always be clearly stipulated or coded in anyway. Additionally, they can shift and fluctuate over time. As a result, it is very difficult for the AI to understand the different (and often conflicting) values among the stakeholders, let alone “train” the AI around a centrally agreed upon solution/outcome (Baum, 2020).
Related to the issue above, Empathetic AI can be particularly susceptible to various biases. The biases may be implicit or explicit, and can be the result of the individuals who wrote the algorithms or the data from which the algorithm was trained (Tomer, 2019). For example, facial recognition AI has been found to contain racial bias (Grother et al., 2019). It is unlikely that biases can fully be eliminated from Empathetic (and other) AI systems. Thus, similar to the “black box” issue, perhaps the best approach is for citizens, decision makers, and AI developers to have open and candid discussions about the appropriate applications of Empathetic AI given the potential unintended consequences that may result from these biases.
Figure 1 provides a summary of the key elements of each intelligence, examples from infrastructure systems, and current/potential applications of AI in infrastructure across each level of intelligence.
How Might AI Disrupt Infrastructure Services and Introduce New Capabilities?
Exploration of the four levels of intelligences in the context of infrastructure systems reveals a few key insights. First, it appears that AI (or at least automation) has already been widely implemented for Mechanical tasks. Although there is still some potential for AI growth and evolution at this level, it appears that we may have already reached a saturation point, thereby making fundamental transformations less likely. This outcome further underscores the potential for AI to complement and supplement Administrative Leadership roles within infrastructure systems. On the other hand, Analytical tasks are where AI appears poised to have the largest disruption (at least in the near-to-medium term). As AI capabilities continue to improve (especially due to the combination of ever-increasing data availability, ever-decreasing computing costs, and advancements in techniques like ANNs), Analytical tasks (and Adaptive Leadership roles) will increasingly be accomplished by AI. Considering that the vast majority of engineering and infrastructure jobs are analytical by nature, the augmentation and/or replacement of Analytical tasks by AI is likely to have a fundamental, profound, and transformative impact on infrastructure systems as we know them. Thus, moving forward, a key adaptation for engineers and infrastructure managers will be to strengthen and place increasing emphasis on Intuitive and Empathetic tasks/intelligences, which in turn should strengthen Enabling Leadership capabilities. This is particularly important, because even though humans exhibit much higher levels of Intuitive and Empathetic Intelligence than AI (and are likely to remain that way for quite a while), there is still room for improvement. Human error is always a concern when operating under both mundane and surprise conditions. Similarly, Empathetic Intelligence currently does not appear to be widely incorporated or considered in the development of engineered/infrastructure systems. Thus, in order to most effectively balance the Mechanical (i.e., Administrative Leadership) and Analytical (i.e., Adaptive Leadership) advantages of AI with the Intuitive and Empathetic (i.e., Enabling Leadership) advantages of humans, we (humans) will need to continually learn from past mistakes and develop skills to make effective decisions under surprise conditions. Additionally, substantial and continual efforts should be made toward enhancing our ability to incorporate social, emotional, and equity dynamics into engineering/infrastructure planning and implementation.
Discussion and Conclusion
It is useful to consider how AI technologies in infrastructure are likely to create new capabilities that, if leveraged correctly, can help us adapt to the rapidly changing conditions in which infrastructure systems must thrive. As evidence emerges of the accelerating and increasingly uncertain conditions that characterize infrastructure environments (Steffen et al., 2015), design and management must be able to respond to these conditions with agility and flexibility (Chester and Allenby, 2019b; Gilrein et al., 2019). With any new technology, control processes are created to harness and guide the new capabilities toward the goals of the managing institution (Beniger, 1989). For example, the advent of engines and novel processes during the industrial revolution released energy at rates and scales never before seen. In turn, these technological advancements required new institutions and processes to channel this power. Whether AI follows historical patterns of technological control is questionable. AI technologies are fundamentally focused on augmenting and replacing cognition. Cognitive infrastructure that learns and makes decisions for us implies that control may not be fully attainable (like it was for the steam engines in the industrial era). Instead, our control efforts may need to focus on establishing relationships with AI that recognize that cyber-technologies will be guiding us in ways that we may not always fully understand.
AI may be uniquely positioned to help us learn about and navigate increasingly complex environments. In designing knowledge systems, institutions enable sensing and analytical capabilities (coupled with different leadership styles) to operate in both calm and chaotic environments (Miller and Munoz-Erickson, 2018). As our systems, and the environments in which they operate, become increasingly complex and beyond the cognitive understanding of any group of individuals or institutions, AI may offer critical cognitive insights to ensure that systems adapt, services continue to be provided, and needs continue to be met.
The mapping of AI applications to intelligences and leadership roles appears to support the varying approaches needed to address domains of complexity. The Cynefin framework classifies systems as simple, complicated, complex, or chaotic, and as we transition from one domain to another, disorder governs (Snowden and Boone, 2007; Chester and Allenby, 2019a). Each domain requires a fundamentally different approach to address challenges. Infrastructure have historically been complicated systems and are now increasingly viewed as complex (Chester and Allenby, 2019b). A complicated system calls for data collection, analyzing and decision-making, while a complex system shifts toward probing, testing, and a commitment to adaptability and transformation. The intelligence mapping presented in Figure 1 provides a useful set of AI applications that can be applied to infrastructure in complicated and complex environments. The Mechanical and Analytical Intelligences appear to align well with complicated situations where the emergent behaviors of systems are predictable and their environments somewhat stable. The Intuitive and Empathetic Intelligences appear to align with complex systems, where perturbations can result in unpredictable emergent behaviors, and “satisficing” is needed to manage wicked problems across technical and social requirements (Chester and Allenby, 2019a). While all intelligences are needed at various times during the operation of a system, the development and deployment of Intuitive and Empathetic Intelligences (and Enabling Leadership) in humans and institutions, as well as the development and deployment of Administrative and Adaptive Leadership via AI appears necessary to address the growing complexity and non-stationarity of our systems and the environments in which they operate.
Ultimately, we are in the nascent stages of AI development and application to infrastructure systems. The topics in this paper are intended to be an initial discussion of some of the key opportunities and challenges associated with AI in infrastructure systems—especially in the context of the leadership and skills needed to face the complex challenges of the Anthropocene. Avenues for future work that can build on this endeavor include interviews and surveys aimed at gaining a better understanding of infrastructure practitioner's current thoughts and expectations about the possible benefits and downsides of AI. Additionally, it would be beneficial to further explore which level of intelligence appears most appropriate for specific problems/contexts, as well as a more detailed assessment of the specific AI techniques likely to be most effective/appropriate in these circumstances. Finally, in conjunction with (if not prior to) these efforts, open, candid, and iterative discussions are required amongst society writ large to debate what level of cognitive infrastructure we are comfortable with and the level of “control” (or at least perceived control) we are comfortable offloading to cognitive infrastructure. By doing so, engineers and infrastructure users/managers can hopefully ensure that they are striking the right balance between human and AI capabilities required to effectively and equitably navigate our increasingly complex world.
Data Availability Statement
The original contributions presented in the study are included in the article/supplementary materials, further inquiries can be directed to the corresponding author/s.
Author Contributions
SM: conception of the work, drafting of the work, and revisions. MC: conception of the work, drafting of the work, and review and editing of the work. BA: drafting of the work and review and editing of the work. All authors contributed to the article and approved the submitted version.
Funding
This work is supported by multiple U.S. National Science Foundation grants including the Urban Resilience to Extremes Sustainability Research Network (UREx SRN) (Award # SRN-1444755) and Resilience Convergence (Award # GCR-1934933).
Conflict of Interest
The authors declare that the research was conducted in the absence of any commercial or financial relationships that could be construed as a potential conflict of interest.
References
Abou-Zeid, M., and Ben-Akiva, M. (2010). “A model of travel happiness and mode switching,” in Choice Modelling: The State-of-the-art and The State-of-practice, ed S. Hess (Bingley: Emerald Group Publishing Limited), 289–305. doi: 10.1108/9781849507738-012
Abrahart, R. J., and See, L. M. (2007). Neural network modelling of non-linear hydrological relationships. Hydrol. Earth Syst. Sci. 11, 1563–1579. doi: 10.5194/hess-11-1563-2007
Adeli, H. (2001). Neural networks in civil engineering: 1989-2000. Comp. Aided Civil Infrastruct. Eng. 16, 126–142. doi: 10.1111/0885-9507.00219
Adeli, H., and Park, H. S. (1995). A neural dynamics model for structural optimization-theory. Comput. Struct. 57, 383–390. doi: 10.1016/0045-7949(95)00048-L
Alexander, A., Thampi, S. G., and Chithra, N. R. (2018). Development of hybrid wavelet-ANN model for hourly flood stage forecasting. ISH J. Hydraulic Eng. 24, 266–274. doi: 10.1080/09715010.2017.1422192
Allenby, B. R. (2011). The Theory and Practice of Sustainable Engineering. Upper Saddle River, NJ: Pearson/Prentice-Hall.
Allenby, B. R. (2019). 5G, AI, and big data: We're building a new cognitive infrastructure and don't even know it. Bull Atomic Sci. Available online at: https://thebulletin.org/2019/12/5g-ai-and-big-data-were-building-a-new-cognitive-infrastructure-and-dont-even-know-it/?utm_source=Newsletter&utm_medium=Email&utm_campaign=Newsletter12232019&utm_content=DIsruptiveTechnology_CognitiveInfrastructure_12192019 (accessed December 19, 2019).
Al-Salloum, Y. A., Shah, A. A., Abbas, H., Alsayed, S. H., Almusallam, T. H., and Al-Haddad, M. S. (2012). Prediction of compressive strength of concrete using neural networks. Comp. Concrete 10, 197–217. doi: 10.12989/cac.2012.10.2.197
Amanollahi, J., Kaboodvandpour, S., and Majidi, H. (2017). Evaluating the accuracy of ANN and LR models to estimate the water quality in Zarivar International Wetland, Iran. Nat. Hazards 85, 1511–1527. doi: 10.1007/s11069-016-2641-1
Arslan, M. H. (2010). An evaluation of effective design parameters on earthquake performance of RC buildings using neural networks. Eng. Struct. 32, 1888–1898. doi: 10.1016/j.engstruct.2010.03.010
Barzegar, R., Adamowski, J., and Moghaddam, A. A. (2016). Application of wavelet-artificial intelligence hybrid models for water quality prediction: a case study in Aji-Chay River, Iran. Stochast. Environ. Res. Risk Assess. 30, 1797–1819. doi: 10.1007/s00477-016-1213-y
Baum, S.D. (2020). Social choice ethics in artificial intelligence. AI Soc. 35, 165–176. doi: 10.1007/s00146-017-0760-1
Beh, E. H. Y., Zheng, F., Dandy, G. C., Maier, H. R., and Kapelan, Z. (2017). Robust optimization of water infrastructure planning under deep uncertainty using metamodels. Environ. Modell. Softw. 93, 92–105. doi: 10.1016/j.envsoft.2017.03.013
Beniger, J. R. (1989). The Control Revolution: Technological and Economic Origins of the Information Society. Cambridge, Massachusetts: Harvard University Press.
Brynjolfsson, E., and Mcafee, A. (2017). The business of artificial intelligence: what it can and cannot do for your organization. Harvard Business Review Digital Articles, 1–20.
Camp, C. V., Pezeshk, S., and Hansson, H. (2003). Flexural design of reinforced concrete frames by genetic algorithm. J. Struct. Eng. 129, 105–115. doi: 10.1061/(ASCE)0733-9445(2003)129:1(105)
Chandwani, V., Agrawal, V., and Nagar, R. (2013). Applications of soft computing in civil engineering: a review. Int. J. Comp. Appl. 81, 13–20. doi: 10.5120/14047-2210
Chen, S. H., Jakeman, A. J., and Norton, J. P. (2008). Artificial Intelligence techniques: an introduction to their use for modelling environmental systems. Math. Comput. Simul. 78, 379–400. doi: 10.1016/j.matcom.2008.01.028
Cheng, C.-T., Wu, X.-Y., and Chau, K. W. (2005). Multiple criteria rainfall-runoff model calibration using a parallel genetic algorithm in a cluster of computers. Hydrol. Sci. J. 50:1069. doi: 10.1623/hysj.2005.50.6.1069
Cheng, K. S., Lien, Y. T., Wu, Y. C., and Su, Y. F. (2017). On the criteria of model performance evaluation for real-time flood forecasting. Stoch. Environ. Res. Risk Assess. 31, 1123–1146. doi: 10.1007/s00477-016-1322-7
Chester, M. V., and Allenby, B. (2019a). Infrastructure as a wicked complex process. Elementa 7:360. doi: 10.1525/elementa.360
Chester, M. V., and Allenby, B. (2019b). Toward adaptive infrastructure: flexibility and agility in a non-stationarity age. Sustai Resil Infrastruc. 4, 173–191. doi: 10.1080/23789689.2017.1416846
Chester, M. V., Markolf, S., and Allenby, B. (2019). Infrastructure and the environment in the anthropocene. J. Ind. Ecol. 23, 1006–1015. doi: 10.1111/jiec.12848
Cisco. (2020). Cisco Annual Internet Report (2018–2023). Cisco. Available online at: http://grs.cisco.com/grsx/cust/grsCustomerSurvey.html?SurveyCode=4153&ad_id=US-BN-SEC-M-CISCOASECURITYRPT-ENT&KeyCode=000112137
Columbus, L. (2018). 2018 Roundup of Internet of Things Forecasts and Market Estimates. Forbes. Available online at: https://www.forbes.com/sites/louiscolumbus/2018/12/13/2018-roundup-of-internet-of-things-forecasts-and-market-estimates/#3a269b967d83
Conniff, R. (2017). Pushy AI Bots Nudge Humans to Change Behavior. Scientific American. Available online at: https://www.scientificamerican.com/article/pushy-ai-bots-nudge-humans-to-change-behavior/
Corominas, L., Garrido-Baserba, M., Villez, K., Olsson, G., Cortés, U., and Poch, M. (2018). Transforming data into knowledge for improved wastewater treatment operation: a critical review of techniques. Environ. Modell. Softw. 106, 89–103. doi: 10.1016/j.envsoft.2017.11.023
Dixon, B. (2005). Applicability of neuro-fuzzy techniques in predicting ground-water vulnerability: a GIS-based sensitivity analysis. J. Hydrol. 309, 17–38. doi: 10.1016/j.jhydrol.2004.11.010
Eggimann, S., Mutzner, L., Wani, O., Schneider, M. Y., Spuhler, D., Moy De Vitry, M., et al. (2017). The potential of knowing more: a review of data-driven urban water management. Environ. Sci. Technol. 51, 2538–2553. doi: 10.1021/acs.est.6b04267
Falcone, R., Lima, C., and Martinelli, E. (2020). Soft computing techniques in structural and earthquake engineering: a literature review. Eng. Struct. 207:110269. doi: 10.1016/j.engstruct.2020.110269
Flintsch, G. W., and Chen, C. (2004). Soft computing applications in infrastructure management. J. Infrastruct. Syst. 10, 157–166. doi: 10.1061/(asce)1076-0342(2004)10:4(157)
Flood, I. (2001). Neural networks in civil engineering: a review,” in Civil and Structural Engineering Computing: 2001, ed B. H. Topping (Kippen: Saxe-Coburg Publications), 185–209.
Flood, I., and Kartam, N. (1994a). Neural networks in civil engineering I: principles and understanding. J. Comp. Civil Eng. 8, 131–148. doi: 10.1061/(ASCE)0887-3801(1994)8:2(131)
Flood, I., and Kartam, N. (1994b). Neural networks in civil engineering II: systems and application. J. Comp. Civil Eng. 8, 149–162. doi: 10.1061/(ASCE)0887-3801(1994)8:2(149)
Gelles, D. (2019). Boeing 737 Max: What's Happened After the 2 Deadly Crashes. The New York Times. Available online at: https://www.nytimes.com/interactive/2019/business/boeing-737-crashes.html
Ghalehkhondabi, I., Ardjmand, E., Young, W. A., and Weckman, G. R. (2017). Water demand forecasting: review of soft computing methods. Environ. Monit. Assess. 189:313. doi: 10.1007/s10661-017-6030-3
Gilrein, E. J., Carvalhaes, T. M., Markolf, S. A., Chester, M. V., Allenby, B. R., and Garcia, M. (2019). Concepts and practices for transforming infrastructure from rigid to adaptable. Sustain. Resilient Infrastruct. 1–22. doi: 10.1080/23789689.2019.1599608
Grother, P., Ngan, M., and Hanaoka, K. (2019). Face Recognition Vendor Test (FRVT) Part 3: Demographic Effects. doi: 10.6028/NIST.IR.8280
Hadi, M. N. S. (2003). Neural networks applications in concrete structures. Comp. Struct. 81, 373–381. doi: 10.1016/S0045-7949(02)00451-0
Hajela, P., and Berke, L. (1991). Neurobiological computational models in structural analysis and design. Comp. Struct. 41, 657–667. doi: 10.1016/0045-7949(91)90178-O
Herkert, J., Borenstein, J., and Miller, K. (2020). The Boeing 737 MAX: Lessons for Engineering Ethics. Sci Eng Ethics. 0123456789. doi: 10.1007/s11948-020-00252-y
Huang, M. H., and Rust, R. T. (2018). Artificial intelligence in service. J. Serv. Res. 21, 155–172. doi: 10.1177/1094670517752459
Jayaram, M. A., Nataraja, M. C., and Ravikumar, C. N. (2009). Elitist genetic algorithm models: optimization of high performance concrete mixes. Mater. Manufact. Process. 24, 225–229. doi: 10.1080/10426910802612387
Jeong, D. I. L., and Kim, Y. O. (2005). Rainfall-runoff models using artificial neural networks for ensemble streamflow prediction. Hydrol. Process. 19, 3819–3835. doi: 10.1002/hyp.5983
Karasekreter, N., Basciftci, F., and Fidan, U. (2013). A new suggestion for an irrigation schedule with an artificial neural network. J. Exp. Theoret. Artif. Intell. 25, 93–104. doi: 10.1080/0952813X.2012.680071
Kartam, N., Flood, I., and Garrett, J. H., (eds.). (1997). Artificial Neural Networks for Civil Engineers: Fundamentals and Applications. New York, NY: American Society of Civil Engineers.
Koutsoyiannis, D. (2011). Hurst-kolmogorov dynamics and uncertainty. J. Am. Water Res. Assoc. 47, 481–495. doi: 10.1111/j.1752-1688.2011.00543.x
Kumar, M., Raghuwanshi, N. S., Singh, R., Wallender, W. W., and Pruitt, W. O. (2020). Estimating of evapotranspiration using artificial neural network. J. Irrigat. Drainage Eng. 37, 81–94. doi: 10.21608/mjae.2020.94971
Lee, C., and Ahn, J. (2003). Flexural design of reinforced concrete frames by genetic algorithm. J. Struct. Eng. 129, 762–774. doi: 10.1061/(asce)0733-9445(2003)129:6(762)
Lee, K. (2018). AI Superpowers: China, Silicon Valley, and the New World Order. Harcourt, Boston, MI: Houghton Mifflin.
Lee, S. C., and Han, S. W. (2002). Neural-network-based models for generating artificial earthquakes and response spectra. Comp. Struct. 80, 1627–1638. doi: 10.1016/S0045-7949(02)00112-8
Li, S., Kazemi, H., and Rockaway, T. D. (2019). Performance assessment of stormwater GI practices using artificial neural networks. Sci. Total Environ. 651, 2811–2819. doi: 10.1016/j.scitotenv.2018.10.155
Lin, J., Kandra, H., Choudhury, T. A., and Barton, A. (2018). “Prediction of clogging in stormwater filters using artificial neural network,” in IEEE International Symposium on Industrial Electronics (Cairns, QLD). 771–776.
Lins, H. F. (2012). A Note on Stationarity and Nonstationarity. Available online at: http://www.wmo.int/pages/prog/hwrp/chy/chy14/documents/ms/Stationarity_and_Nonstationarity.pdf (accessed November 30, 2020).
Liu, R., Jiang, J., Guo, L., Shi, B., Liu, J., Du, Z., et al. (2016). Screening of pollution control and clean-up materials for river chemical spills using the multiple case-based reasoning method with a difference-driven revision strategy. Environ. Sci. Pollut. Res. 23, 11247–11256. doi: 10.1007/s11356-016-6283-3
Lo, F., and Yeung, Y, (eds). (1998). Globalization and the World of Large Cities. Tokyo: United Nations University Press.
Maier, H. R., and Dandy, G. C. (2000). Neural networks for the prediction and forecasting of water resources variables: a review of modelling issues and applications. Environ. Modell. Softw. 15, 101–124. doi: 10.1016/S1364-8152(99)00007-9
Marchant, G. E., Allenby, B. R., and Herkert, J, (eds). (2011). The Growing Gap Between Emerging Technologies and Legal-Ethical Oversight. New York, NY: Springer Press.
Markolf, S. A., Chester, M. V., Eisenberg, D. A., Iwaniec, D. M., Davidson, C. I., Zimmerman, R., et al. (2018). Interdependent infrastructure as linked social, ecological, and technological systems (setss) to address lock-in and enhance resilience. Earth's Future 6, 1638–1659. doi: 10.1029/2018EF000926
Matias, J. N. (2017). Persuading Algorithms With and AI Nudge. MIT Media Lab. Available online at: https://medium.com/mit-media-lab/persuading-algorithms-with-an-ai-nudge-25c92293df1d
Meserole, C. (2018). Artificial Intelligence and the Security Dilemma. Available online at: https://www.brookings.edu/blog/order-from-chaos/2018/11/06/artificial-intelligence-and-the-security-dilemma/ (accessed November 30, 2020).
Miller, C. A., and Munoz-Erickson, T. A. (2018). The Rightful Place of Science: Designing Knowledge. Tempe, AZ: Consortium for Science, Policy & Outcomes.
Milly, P. C. D., Betancourt, J., Falkenmark, M., Hirsch, R. M., Kundzewicz, Z. W., Lettenmaier, D. P., et al. (2008). Stationarity is dead: whither water management? Science 319, 573–574. doi: 10.1126/science.1151915
Minns, A. W., and Hall, M. J. (1996). Artificial neural networks as rainfall-runoff models. Hydrol. Sci. J. 41, 399–417. doi: 10.1080/02626669609491511
Mitra, P., Ray, R., Chatterjee, R., Basu, R., Saha, P., Raha, S., et al. (2016). “Flood forecasting using Internet of things and artificial neural networks,” in 7th IEEE Annual Information Technology, Electronics and Mobile Communication Conference (Vancouver, BC: IEEE IEMCON), 1–5.
Mounce, S. R., Mounce, R. B., and Boxall, J. B. (2016). Case-based reasoning to support decision making for managing drinking water quality events in distribution systems. Urban Water J. 13, 727–738. doi: 10.1080/1573062X.2015.1036082
Nagy, H. M., Watanabe, K., and Hirano, M. (2002). Prediction of sediment load concentration in rivers using artificial neural network model. J. Hydraul. Eng. 128, 588–595. doi: 10.1061/(ASCE)0733-9429(2002)128:6(588)
Ni, H.-G., and Wang, J.-Z. (2000). Prediction of compressive strength of concrete using neural networks. Comput. Concr. 30, 1245–1250. doi: 10.1016/S0008-8846(00)00345-8
Nixon, J. B., Dandy, G. C., and Simpson, A. R. (2001). A genetic algorithm for optimizing off-farm irrigation scheduling. J. Hydroinformat. 3, 11–22. doi: 10.2166/hydro.2001.0003
NRC (US National Research Council). (2003). Cities Transformed. Washington, DC: National Academies Press.
Picard, R. W. (1995). Affective Computing. MIT Media Laboratory Perceptual Computing Section Technical Report No. 321. Available online at: https://affect.media.mit.edu/pdfs/95.picard.pdf (accessed November 30, 2020).
Pisa, I., Santín, I., Vicario, J. L., Morell, A., and Vilanova, R. (2019). ANN-based soft sensor to predict effluent violations in wastewater treatment plants. Sensors 19:1280. doi: 10.3390/s19061280
Quercia, D., Schifanella, R., and Aiello, L. M. (2014). “The shortest path to happiness: Recommending beautiful, quiet, and happy routes in the city,” in HT 2014 - Proceedings of the 25th ACM Conference on Hypertext and Social Media (Santiago), 116–125.
Rastegaripour, F., Saboni, M. S., Shojaei, S., and Tavassoli, A. (2019). Simultaneous management of water and wastewater using ant and artificial neural network (ANN) algorithms. Int. J. Environ. Sci. Technol. 16, 5835–5856. doi: 10.1007/s13762-018-1943-0
Rezaeianzadeh, M., Kalin, L., and Anderson, C. J. (2017). Wetland water-level prediction using ANN in conjunction with base-flow recession analysis. J. Hydrol. Eng. 22, D4015003-1–D4015003-11. doi: 10.1061/(ASCE)HE.1943-5584.0001276
Sabouri, F., Gharabaghi, B., Sattar, A. M. A., and Thompson, A. M. (2016). Event-based stormwater management pond runoff temperature model. J. Hydrol. 540, 306–316. doi: 10.1016/j.jhydrol.2016.06.017
Sawhney, M. (2016). Putting products into services. Harvard Business Review, 82–89. Available online at: https://hbr.org/2016/09/putting-products-into-services (accessed November 30, 2020).
Shahin, M. A., Maier, H. R., and Jaksa, M. B. (2002). Predicting settlement of shallow foundations using neural networks. J. Geotechn. Geoenviron. Eng. 128, 785–793. doi: 10.1061/(asce)1090-0241(2002)128:9(785)
Snowden, D. J., and Boone, M. E. (2007). A Leader' s Framework for Decision Making. Harvard Business Review. Available online at: www.hbr.orgorcall800-988-0886.www.hbrreprints.org
Steffen, W., Broadgate, W., Deutsch, L., Gaffney, O., and Ludwig, C. (2015). The trajectory of the Anthropocene: the great acceleration. Anthropocene Rev. 2, 81–98. doi: 10.1177/2053019614564785
Suh, B. (2019). Can AI Nudge Us to Make Better Choices? Harvard Business Review. Available online at: https://hbr.org/2019/05/can-ai-nudge-us-to-make-better-choices
Tabari, H., Marofi, S., and Sabziparvar, A. A. (2010). Estimation of daily pan evaporation using artificial neural network and multivariate non-linear regression. Irrigat. Sci. 28, 399–406. doi: 10.1007/s00271-009-0201-0
Tokar, B. A. S., and Johnson, P. A. (1999). Rainfall-runoff modeling using artificial neural networks. J. Hyrdol. Eng. 4, 232–239. doi: 10.1061/(ASCE)1084-0699(1999)4:3(232)
Tomer, A. (2019). Artificial Intelligence in America's Digital City. Available online at: https://www.brookings.edu/research/artificial-intelligence-in-americas-digital-city/ (accessed November 30, 2020).
U.S. House Committee on Transportation Infrastructure. (2020). The Boeing 737 MAX Aircraft: Costs, Consequences, and Lessons from it Design, Development, and Certification. (Washington, DC: U.S. House Committee on Transportation Infrastructure. Available online at: https://transportation.house.gov/imo/media/doc/TI Preliminary Investigative Findings Boeing 737 MAX March 2020.pdf
Uhl-Bien, M., Marion, R., and McKelvey, B. (2007). Complexity Leadership Theory: Shifting Leadership from the industrial age to the knowledge era. Leadersh. Q. 18, 298–318. doi: 10.1016/j.leaqua.2007.04.002
Wise, J. (2019). The Boeing 737 Max and the Problems Autopilot Can't Solve. The New York Times. Available online at: https://www.nytimes.com/2019/03/14/opinion/business-economics/boeing-737-max.html
Yang, B., Zheng, W., and Ke, X. (2017). Forecasting of industrial water demand using case-based reasoning—A case study in Zhangye City, China. Water 9:626. doi: 10.3390/w9080626
Ye, X. W., Jin, T., and Yun, C. B. (2019). A review on deep learning-based structural health monitoring of civil infrastructures. Smart Struct. Syst. 24, 567–585. doi: 10.12989/sss.2019.24.5.567
Yeh, I.-C. (1999). Design of high-performance concrete mixture using neural networks and nonlinear programming. J. Comp. Civil Eng. 13, 36–42. doi: 10.1061/(ASCE)0887-3801(1999)13:1(36)
Yilmaz, S. (2011). Ground motion predictive modelling based on genetic algorithms. Nat. Hazards Earth Syst. Sci. 11, 2781–2789. doi: 10.5194/nhess-11-2781-2011
Young, C. C., Liu, W. C., and Wu, M. C. (2017). A physically based and machine learning hybrid approach for accurate rainfall-runoff modeling during extreme typhoon events. Appl. Soft Comp. J. 53, 205–216. doi: 10.1016/j.asoc.2016.12.052
Young-Su, K., and Byung-Tak, K. (2006). Use of artificial neural networks in the prediction of liquefaction resistance of sands. J. Geotech. Geoenviron. Eng. 132, 1502–1504. doi: 10.1061/(asce)1090-0241(2006)132:11(1502)
Yu, F., Li, X. Y., and Han, X. S. (2018). Risk response for urban water supply network using case-based reasoning during a natural disaster. Safety Sci. 106, 121–139. doi: 10.1016/j.ssci.2018.03.003
Zamanisabzi, H., King, J. P., Dilekli, N., Shoghli, B., and Abudu, S. (2018). Developing an ANN based streamflow forecast model utilizing data-mining techniques to improve reservoir streamflow prediction accuracy: a case study. Civil Eng. J. 4:1135. doi: 10.28991/cej-0309163
Zhang, F. X., Wai, O. W. H., and Jiang, Y. W. (2010). Prediction of sediment transportation in deep bay (Hong Kong) using genetic algorithm. J. Hydrodyn. Ser. B 22, 599–604. doi: 10.1016/S1001-6058(09)60260-2
Zhang, J., Du, C., and Feng, X. (2017). Research on a soft measurement model of sewage treatment based on a case-based reasoning approach. Water Sci. Technol. 76, 3181–3189. doi: 10.2166/wst.2017.417
Keywords: climate change, infrastructure, artificial intelligence, complexity, anthropocene
Citation: Markolf SA, Chester MV and Allenby B (2021) Opportunities and Challenges for Artificial Intelligence Applications in Infrastructure Management During the Anthropocene. Front. Water 2:551598. doi: 10.3389/frwa.2020.551598
Received: 14 April 2020; Accepted: 19 November 2020;
Published: 27 January 2021.
Edited by:
Auroop Ratan Ganguly, Northeastern University, United StatesReviewed by:
Munir Ahmad Nayak, Indian Institute of Technology Indore, IndiaAbhishek Gaur, National Research Council Canada (NRC-CNRC), Canada
Copyright © 2021 Markolf, Chester and Allenby. This is an open-access article distributed under the terms of the Creative Commons Attribution License (CC BY). The use, distribution or reproduction in other forums is permitted, provided the original author(s) and the copyright owner(s) are credited and that the original publication in this journal is cited, in accordance with accepted academic practice. No use, distribution or reproduction is permitted which does not comply with these terms.
*Correspondence: Samuel A. Markolf, smarkolf@asu.edu