- 1Earth Observation Group, Payne Institute for Public Policy, Colorado School of Mines, Golden, CO, United States
- 2Trinity College Dublin, The University of Dublin, Dublin, Ireland
- 3School of Natural and Built Environments, University of South Australia, Adelaide, SA, Australia
- 4Department of Geography and Environment, University of Denver, Denver, CO, United States
Building Volume Per Capita (BVPC - cubic meters of building per person) is presented as a proxy measure of economic inequality and a direct measure of housing inequality. Sustainable development goal 10 (SDG 10: reduced inequalities) is synergic for achieving SDG 11 on sustainable cities and communities. Access to safe and affordable housing, transport systems, and public spaces are some of the targets of SDG 11 that mostly link with reducing inequalities. The Habitat III New Urban Agenda sets equal access to urban spaces, infrastructures and basic services as crucial for developing sustainable cities. Earth Observation (EO) data including remotely sensed satellite data, airborne data, and model outputs, in combination with demographic, and other statistical data, have been gaining importance for monitoring progress of the SDGs. High spatial resolution building footprint data derived from aerial photographs, stereo imagery, and LIDAR data, obtained for the cities of California, between 2010 and 2015, were used in this study. These measures of building volume were rasterized and juxtaposed with (divided by) a variety of demographic data including vector-based census data of 2015 and LandScan raster data of population counts of 2015. The National Landcover dataset of 2011 was used to characterize the land cover variability of the cities. Using these datasets, the spatial pattern and distribution of BVPC for nine cities in California were studied. The results showed that BVPC was inversely related with intensity of development, and positively related with median household income within cities. A BV-GINI was also developed to characterize the variability of the BVPC at the census tract level and the pixel level. This measure of income inequality, housing and population density is objective and easily executable. It can be used in other cities and countries and may help overcome lack of data in SDG indicators.
Introduction
The Sustainable Development Goals (SDGs), adopted by 193 member countries of the United Nations Development Programme (UNDP) (2015), attempt to capture and respond in sufficient detail to the suite of global civilizational challenges. One of the greatest challenges facing the current civilization is rapid urbanization. Urban population trends indicate that humanity will increasingly be living in cities. Overcrowding and congestion in dwelling units in cities affect the well-being of individuals. Because of the absence of private space, household members often suffer from stress, and develop other social and behavioral problems (Cox, 2016). Besides, the spread of infectious diseases, including asthma and other respiratory diseases (e.g., COVID 19), as well as, mental illness and anxiety are more rampant in overcrowded households (Solari and Mare, 2012). The household crowding problem is often associated with neighborhoods where the working class and immigrant population live. Thus, it is also a reflection of income inequality.
Therefore, various other sectoral goals such as poverty reduction (SDG 1), quality education (SDG 4), clean water and sanitation (SDG 6), access to energy (SDG 7) economic growth (SDG 8) and reduction in inequality (SDG 10) need be framed at city scale and contribute to SDG 11 on sustainable cities. However, inequalities at city scale limit the benefits of such sustainability progresses to a limited portion of a city's population, defeating the purpose of an urban environment that exists to facilitate access to services and means of well-being. This is reflected by some of the targets of SDG 1, which explicitly refer to inequalities, such as target 11.1 to ensure access for all to adequate, safe and affordable housing, among others.
There are 17 SDGs that are associated with 169 targets and 232 indicators (UNSTATS, 2019). Making valid and objective measurements of the 232 indicators represents a significant problem for both scientists and policy makers. In this vein, satellite observations produce objective information at different spatial resolutions and territorial scales.
In the United States, overcrowding is estimated in the American Community Surveys as the number of people living in a dwelling unit per room, or Persons per room (PPR). Overcrowding is generally defined as a household (people living in a housing unit) having more than one person per room in a dwelling unit; and severe overcrowding is more than 1.5 persons per room. While the ACS's PPR data are useful, it also has potential problems. Since the ACS surveys only a fraction of the population, the PPR data can only be released at the scale of the census tract. Thus, it has limited spatial resolution. The PPR measurement also suffers from imprecise temporal resolution and measurement of physical space. The PPR ignores the fact that rooms vary in size. Therefore, a 100 and 200 square-meter one-bedroom apartment, when occupied by one person, are considered as comparable units (Reddy and Leslie, 2015).
Hence, with the goal of developing a more objective measure of overcrowding and understanding its link to income inequality and intensity of development, we present the idea of Building Volume Per Capita (BVPC) as a spatially explicit measure of human living conditions. Because of the easy availability of Building height data for some of the cities in California, United States, we carry out our analysis in these cities. Building volume in m3 is building height times building footprint (area in m2). Interest in utilizing building volume information for scientific inquiry is growing. LIDAR measures of building volume have been used to inform high spatial resolution population estimates (Ko Ko Lwin, 2011; Lu et al., 2011; Xie et al., 2015; Zhao et al., 2017). IKONOS-2 ortho image and LIDAR data have been used in conjunction to construct 3D city models and subsequently quantify urban population (Tomás et al., 2016). The BVPC we present here takes a step back in terms of spatial aggregation and presents a moderate spatial resolution characterization of “roominess” of housing experience with the implicit assumption that the more affluent enjoy more building volume per person.
This measure blends with several of the SDGs, which are implicit for achieving SDG 111 (SDG 11, Developing sustainable cities and communities). BVPC is measured by using remotely sensed data in conjunction with census population data and land cover data. BVPC captures the average “space” people have in their local neighborhoods. This is undoubtedly compromised as a measure in commercial and industrial areas which is why we focus on residential areas. This measure also helps to overcome the drawbacks of the census measures of overcrowding such as PPR. A BV-GINI was also developed to explore the level of income or wealth dispersion represented by BVPC at the census tract level and the pixel level. Governments such as Poland are currently using GINI coefficients as one of the indicators of SDG 10 (Statistics Poland, 2020).
We were unable to find any previous work in which Building Volume per capita was used as a measure of the SDGs. So, this paper is a pioneering effort to explore the utility of BVPC for the SDG. However, the concept of using Volume per capita as a measure of residential space was put forward by Reddy and Leslie in their 2015 paper. They had highlighted the advantages of using Volume per capita (VPC) as a measure of residential space as it provides higher spatial detail, which could be aggregated to various scales of analysis, and quantifies actual residential space in cubic footage.
We believe Earth Observation (EO) can be used in a variety of ways to contribute to the assessment of both the state and dynamics of SDG 11. This preliminary analysis explores the use of Building Volume Per Capita (BVPC) as an indirect measure of income and income inequality and a direct measure of housing inequality. Existing building footprint datasets such as the Global Human Settlement Layer (Global Human Settlement - Home - European Commission, 2016) are increasingly being attributed with building height information which creates the opportunity for using BVPC for counties/regions for which verifiable building footprint data are available.
Materials and Methods
Detailed building footprint data with building heights allows for unprecedented spatial characterization of variation in amount of building volume people actually live with. Using just the area of the buildings to get m2/person might make sense in homogeneous regions where most of the residential buildings are single-family houses. However, many urban environments, including slums, have multi-story structures. By using m3 per person we have an Earth Observation metric of how much space people have to actually live in. Different sources of Earth Observation data were used in this analysis to portray BVPC as a measure of housing and income inequality, corresponding to SDG 10 and relevant for SDG 11.
There are undoubtedly important questions as to what the most appropriate spatial scale of analysis for understanding this variability is. We present an analysis at two spatial “resolutions”: the census tract and 500 × 500 m grid cell. This work results in two datasets at coarser spatial resolution than the higher spatial resolution building footprint data it was derived from (Figure 1). These two datasets can be juxtaposed with population, income data, and land cover data.
Building footprint data available for nine cities in California were used in this analysis. Building footprint data in shapefile format for Los Angeles County were available from “Los Angeles County GIS Data Portal” (Los Angeles County GIS Data Portal, 2016). The building footprint data including building height in feet, area and parcel number were captured from stereo imagery as part of the LARIAC2 Project (2008 acquisition) and was updated as part of the LARIAC4 (2014) imagery acquisition. The data were made available to the public on November 1, 2016.
The building footprint data for San Francisco were derived from the City and County of San Francisco, Department of Technology, Digital Services Division, San Francisco Enterprise Geographic Information Systems Program (SFGIS) in a geodatabase format2. The data were created in May 2017. The building footprints were collapsed from a former 3D building model provided by Pictometry of 2010. The “hgt_MAXcm” column depicting LIDAR derived height surface grid in centimeters were used.
The building footprint data for the rest of the cities of California, namely, Bakersfield, Del Mar, Fresno, Fullerton, Modesto, Sacramento, and Santa Barbara were obtained in shapefile format from Microsoft Building Footprint data (Microsoft Building Footprint)3. In March 2017, Microsoft released the first set of building footprint data containing approximately 9.8 million high quality building footprint data with heights in 44 US states. The height data in meters were created from very high-resolution aerial photography captures by Microsoft, and the height attribute was interpolated from a digital terrain model derived from the same data. The footprints were digitized in 2015 from imagery captures of 2014 and 2015. In June 2018, a second dataset containing 125 million computer-generated building footprints for all 50 states was released by Microsoft. The data were in “Geographic” projection.
LandScan population grid data for the year 2015 was available from Oak Ridge National Laboratory, under contract with the United States Department of Energy4. LandScan is developed by using remote sensing techniques within a multivariate dasymetric modeling framework to distribute the best available demographic (Census) data, and geographic data, and are tailored to match the data conditions and geographical nature of each individual country or region. The spatial resolution of the data is approximately 1 km (30” x 30”), and it represents ambient population (average over 24 h) distribution. Each pixel in the LandScan data demonstrates population count or the number of people per cell. The data are referenced in “Geographic” projection or latitude/longitude (WGS84) coordinates.
The Census tract Median Household Income in the past 12 months (in 2015 inflation-adjusted dollars) and the Total population of 2015 were derived from U.S. Census Bureau, 2011-2015 American Community Survey 5-year estimates, and downloaded from the U.S. Census Bureau, American Fact Finder website (U.S. Census Bureau, 2010).
The shapefiles for the state, counties, and Census Tracts for all of U.S. were downloaded from the Tiger/Line shapefile, U.S. Census Bureau (U.S. Census Bureau, 2010). The shapefile for the state of California was selected and then intersected with the county and census tract shapefiles of the U.S. to isolate the ones for California.
The building footprint polygons of the nine cities in California were converted into North America Lambert Conformal Conic projection. The building height data of Los Angeles were converted from feet to meters. For each of the nine cities, spot checks of building heights were done in Google Earth and using other information. Heights and geographic coordinates of the tallest buildings in each of the nine cities were gathered from the web. The geographic coordinates were used to check the location and building height in Google Earth. The same check was applied on the shapefiles. Some of the building footprint polygons which looked erroneous, for example, a very high building in a residential area, were removed. Moreover, all the building footprint polygons which had their height as zero, were removed. The largest set of building footprint data were available for Los Angeles, and about 2% of the data had the building heights as zero. These were mostly polygons with topological errors, such as having a polygon within a polygon, or overlapping polygons. These polygons were deleted. For DelMar, about 57% of the building footprint data had missing height information. Instead of providing an average height value based on the neighboring buildings, the polygons were deleted. For the rest of the 7 cities, there were no missing height data. These were the steps taken to rectify the freely downloaded building footprint data for the cities. Next, using the height in meters column for the building footprint polygons, and the “polygon to raster” tool in ArcGIS, one-meter building volume grids were created.
The census tract shapefile of California was overlaid on the one-meter building volume rasters, and the “zonal statistics as table” tool in ArcGIS was implemented to extract the “sum of building volume” for the census tracts covering each of the nine cities. Subsequently, the total population of each of the corresponding census tracts was divided by sum of building volume to derive building volume per capita (Figure 2).
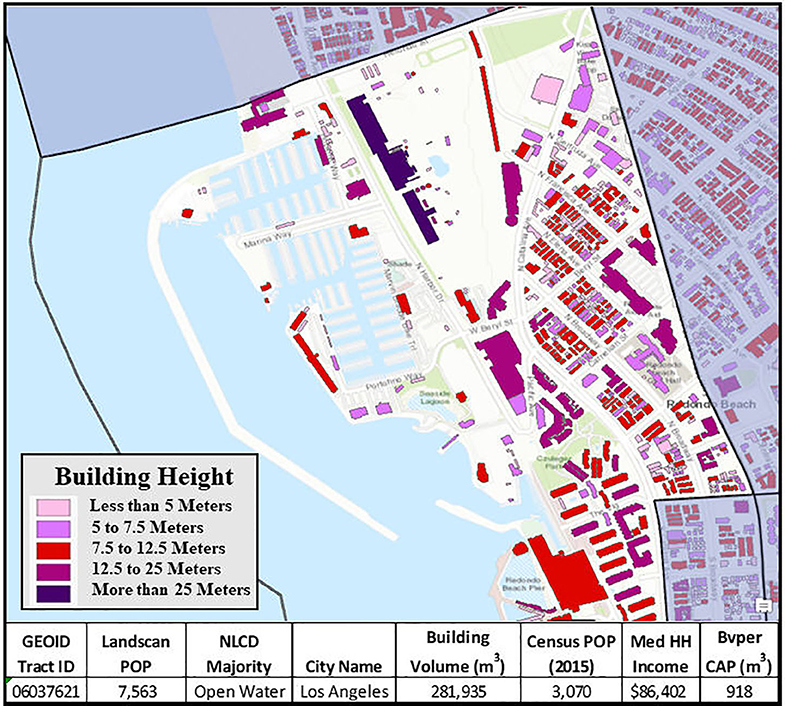
Figure 2. Inset of Raw Building Volume Data for a single census tract representing Redondo Beach and Marina Del Rey in Los Angeles.
Land cover variability within these nine cities is significant. We extracted the dominant or majority landcover from the5, National Land Cover Dataset (USGS, NLCD) 2011 database as an additional characterization of the census tract data (USGS, National Land Cover Database). The NLCD 2011 dataset is a 30 m resolution raster data derived from Landsat imagery. Most census tracts were characterized as having Developed - High Intensity, Medium Intensity, Low Intensity, or Open Space. The “Developed, Open Space” class typically captured exurban areas characterized by low density development (Sutton et al., 2006). Those areas with a majority of “Developed, High Intensity” were typical of downtown central business districts with a large fraction of commercial buildings. We did simple linear regressions using median household income to predict BVPC at the tract level only for those census tracts characterized by NLCD as “Developed – Medium, Low, or Open Space” because the High Intensity tracts were primarily commercial and industrial sites.
The second method of analysis was to compute the BVPC at the 500 m by 500 m pixel level. For this, it was necessary to calculate the sum of building volume and population within those grids. The global LandScan population grid which is originally available at 30 arcsecond resolution was resampled to 15 arcsecond resolution, each pixel representing an area of ~500 m by 500 m. Further, a box polygon was used to clip out the extent of California from the global dataset. A 500 m by 500 m fishnet polygon was created out of the LandScan grid. Then the polygon fishnet was overlaid on the LandScan grid of California to extract the population count values within each pixel using the zonal statistics tool in ArcGIS. Again, the same fishnet was overlaid on the building volume rasters to get the sum of the building volume grids within each 500 m by 500 m pixel. The BVPC was then calculated by dividing the sum of building volume by the population within each 500 m by 500 m pixel. The census tract boundaries which were used to define each of the 9 cities were dissolved and overlaid on the fishnet polygon to separate out each of the 9 cities.
Results
Aggregate Statistics
Six of the 9 cities had an aggregate BVPC of between 950 and 1,085 m3 per person. These six cities varied in total population size over an order of magnitude (roughly 100,000 to 1.4 million). The other three cities had much lower aggregate BVPC values (Los Angeles – 396, San Francisco – 478, and Del Mar – 586) (Table 1). These three cities constituted three of the four most populous cities. These three cities were all large and, on the coast, which may contain an explanation for some of this variability (Sutton, 2003). The BVPC for those census tracts with a majority NLCD value of High Intensity Development varied substantially but were almost always in excess of 1,000 m3 per person. Three of the cities had no tracts dominated by High Intensity Development and three of them had less than four tracts with that characterization. The BVPC for census tracts characterized as Medium Intensity Development (the most common characterization of census tracts in this study) showed the same pattern of six cities with an average around 1,000 m3 per person and the same other three cities with lower values (350, 519, and 408) for San Francisco, Del Mar, and Los Angeles, respectively. Note that Los Angeles dominates this dataset in that it represents 1,313 of the 2,335 census tracts evaluated. The BVPC for the Low Intensity Development tracts did not systematically vary from those of the Medium Intensity tracts. In some cities the values were higher, in others lower; however, for the most part the BVPC values went up as Development Intensity went down. This trend continued with BVPC going up generally for those census tracts dominated by “Developed Open Space.”
City Assessments
For each city we mapped the BVPC per tract and the BVPC per 500 m x 500 m raster cell. The raster cell analysis provided greater detail as to the spatial variability of BVPC; however, we don't have corresponding income information at that spatial resolution. In addition, we characterized the mean and variability of the BVPC for those tracts according to their NLCD majorities. We also ran a simple linear regression using only those tracts with NLCD classes that are predominantly residential in character (e.g., Medium Intensity Development, Low Density Development, and Open Space Development).
Our assessment of Del Mar, California (just north of San Diego, California) included many municipalities totaling a population of 992,745. The mean BVPC for the 178 census tracts in Del Mar was 586 m3/person with a median of 543 m3/person, a minimum value of 67.5 m3/person and a maximum of 1,482 m3/person. The raster representation of BVPC had 5,167 cells with a mean BVPC value of 2,317 m3/person and a median of 487 m3/person. Not surprisingly this distribution skewed toward a few higher values and had a much higher variability of BVPC values ranging from 0 (where there were no buildings – and usually no population) to over a million m3/person in sparsely populated areas with very low ambient populations. There were 993 raster cells with a building volume of zero that accounted for an ambient population of 64,500 (roughly 7% of the total population) (Figure 3). We conducted a regression using Median Household Income of the census tract as the independent variable to predict the BVPC as the dependent variable. The idea is that median household income variability within the city is a measure of economic inequality in that city and that BVPC will correlate with that measure. We found a significant positive correlation between BVPC and median household income for all of the nine cities we studied. For Del Mar the regression equation was BVPC = 258 + 0.004 * MedHHInc with an R2 = 0.21. This regression suggests that each additional $1,000 of household income in Del Mar “buys” an additional 4 m3/person of BVPC. A figure characterizing the assessment of each of the other eight cities can be found in the Supplementary Material (Figures S1–S8).
Gini Coefficients Based on Building Volume and Median Household Income
The GINI coefficient is an established measure of economic inequality that is typically determined using a Lorenz curve of cumulative percentage of population plotted against cumulative percentage of income or wealth (Lorenz, 1905; Elvidge et al., 2012). We used the Lorenz curve methodology to derive a Building Volume GINI coefficient (BV-GINI). This was accomplished for both the raster and vector representations of the nine cities. For the raster data we sorted the value attribute table by cumulative building volume and built a Lorenz curve with the associated cumulative population. We calculated two BV-GINI coefficients by using the zero value cells in one version and excluding the zero value cells in the second (Figure 4). We also derived a BV-GINI coefficient using the tract data. We sorted the attribute table of the census tract shapefile by building volume and then plotted cumulative percent population vs. cumulative percent building volume to calculate a BV-GINI coefficient.
We also explored using median household income as a proxy measure of the traditional GINI coefficient. This is problematic because the median statistic can obscure much of the data variability and aggregates over a variable sized area for a population of roughly five thousand people. Nonetheless, we sorted the attribute table of the census tract shapefile by median household income (medHHinc) and calculated a similar GINI Coefficient (Table 2).
Discussion
Within Country Measures of Inequality
Lack of data is one limiting factor, together with lack of quantified policy targets, for the implementation of the SDGs. Globally, we lack data for 68% of SDG indicators (United Nations Environment Programme (UNEP), 2019), while progress toward 3 of the 17 goals cannot be assessed and monitored in the EU due to lack of data (Eurostat, 2020). While the contribution of Earth Observation (EO) to space-based environmental monitoring services is being (partially) explored through, for example, the EU Copernicus programme (Copernicus, 2020), EO is still underused for developing indicators for the SDGs. Furthermore, while contributions toward the goals should be measured at different scales of analysis, most effort is made for monitoring country progress, while contributions from business, citizens and municipalities is not highlighted. This study shows the importance of EO data in studying the SDGs. The building footprint data, the LandScan population data, and the National Landcover Dataset, are all remotely sensed EO data, which have been made available for free download. Because the methods are systematic, obtainable in a uniform and objective manner, and relatively low cost – we hope that more of the EO data will be used to study the Sustainable Development Goals (SDGs).
Inequality is increasingly recognized as not only a social justice issue but as a significant obstacle to achieving economic efficiency (Giridharadas, 2018); in addition, economic inequality is recognized as contributing to undesirable public health outcomes (Leigh et al., 2011). Although the evidence regarding many of the effects of economic inequality is inconsistent (Lynch et al., 2004), the majority (~70%) of findings suggest that negative consequences are the dominant effect of economic inequality (Wilkinson and Pickett, 2006). Here we measure BVPC as an absolute figure attributed to both census tracts and 500 m x 500 m grid cells as well as the distribution of these values measured across nine metropolitan areas as determined via a Lorenz curve construction.
For all the 9 cities studied in this paper, Median Household income was seen to be a significant predictor (significance level < 0.0001) of BVPC. The highest R2 was 0.4 for Bakersfield. The low R2 for the cities can be attributed to the high variability in the BVPC data. Nonetheless, it does not belittle the hypothesis that Median Household Income has a significant relationship with BVPC. Therefore, people with lower income in cities cannot afford bigger spaces and are left with small volumes of space per capita. This has a domino effect on individuals and families, and all the disadvantages slow down the progress toward achieving the goal of sustainable cities.
The average size of a single-family home in the U.S. today is 2,600 square feet (~241 m2). If we assume roughly 3 meters of elevation (8 ft plus some attic space) for the living space, then an average US home is roughly 720 m3 of building volume. Average household size in the U.S. in 2018 is roughly 2.5 people. Building Volume per person (BVPC) for single family households would then be roughly 290 m3/person or roughly 1,000 square feet per person. Building Volume per capita is a relatively recent idea as a useful measure of residential space. Persons per room (PPR) and Volume per capita (VPC) have been demonstrated to be correlated to one another in the United States and VPC values between 1,020 ft3/person (28.9 m3/person) and 29,926 ft3/person (847 m3/person) with a median value of 8,242 ft3/person (233 m3/person) were measured by Reddy and Leslie for areas in Baltimore (Reddy and Leslie, 2015). The Reddy and Leslie study, which examined Baltimore at the block and block group level, concluded that the wealth of residents and small family size leads to large amounts of residential living space per person.
Our study was summarized at the tract level and the 500 m x 500 m raster cell level. We included varying degrees of non-residential buildings in our assessment of building volume. The reasons our estimates of BVPC were significantly higher than those of Reddy and Leslie probably include the differing nature of historical development in California and Baltimore and the inclusion of more commercial structures in our study. Our measures for Los Angeles, San Francisco, and Del Mar were very similar to those in Baltimore which may be attributable to geographic characteristics such as being relatively large cities on the coasts. This sort of work is in its infancy because the technology (e.g., LIDAR, Satellite imagery, high spatial resolution demographic information, and volunteered geographic information) has only recently made this kind of data and information available. In fact, availability of such building footprint data, which can be freely downloadable is still very limited, and high-resolution building footprint data from IKONOS or LIDAR are cost prohibitive. Moreover, in working with the building footprint data we came across missing data, and other data errors. Income data are mostly available to the lowest administrative boundaries and in this study, we used the data available at the Census tract level. If income data were available at a higher spatial resolution, say, 500 m by 500 m grids we could have carried out the regression analysis of income and BVPC at the pixel level. However, since it is not available, we could present only a pictorial representation of BVPC at the pixel level. Nighttime light satellite images have been used as a proxy for income in several studies (Ghosh et al., 2010, 2013; Henderson et al., 2012; Mellander et al., 2015; Hu and Yao, 2019). We could use the VIIRS nighttime lights as a proxy for income in forthcoming studies. Nevertheless, the relationships found between household income and BVPC, and BVPC and intensities of development are interesting enough to encourage more explorations. Future research by many scholars will likely shed light on the utility of measures like this and the ideal spatial scale for measuring them with the purpose of establishing objective measures of a valid proxy for the broad idea of “inequality.” We believe this may be a useful, spatially explicit, and valid way to characterize “inequality” within a country. Of course, there is another side to the inequality question that addresses differences between countries.
Between Country Measures of Inequality
Several of SDGs lend themselves to having indicators associated with ideas of BVPC and BV-GINI. Arguably, SDG 3 Good Health and Well-being, SDG 10 Reduced Inequalities, and SDG 11 Sustainable Cities and Communities are informed by such concepts and might even benefit from using these measures as contributing indicators. SDG indicator 11.1.1 “proportion of urban population living in slums, informal settlements or inadequate housing” lends itself to EO measurement (Kuffer et al., 2018). BVPC may be a useful contribution to monitoring and assessing progress on this indicator. The viability of mapping and monitoring slums from space has developed substantially in the last decade and there is promise that this development will continue (Goldblatt et al., 2016; Kuffer et al., 2016). Nonetheless, challenges remain because urban areas (let alone slums) are spatially complex both in terms of form, function, and spectral characteristics (Powell et al., 2007; Lilford et al., 2019). This complexity raises key questions about what the appropriate spatial scales of measurement are to measure and assess what phenomena or concepts relevant to policies such as the Sustainable Development Goals.
What Is the Appropriate Spatial Scale of Analysis?
There are many reasons to develop spatially explicit representations of policy relevant phenomena such as those identified by the SDGs. Nonetheless, the spatial and temporal resolution appropriate to myriad policy interventions can vary dramatically. Epidemiologists can need specific building and address level information whereas identifying neighborhoods with inadequate housing could require information at much coarser spatial resolution. There is growing consensus that pixels rather than polygons will likely be the preferred format for mapping and monitoring phenomena that will be derived from ecological models (Blei et al., 2018); nonetheless, the raster vs. vector and related spatial resolution questions will likely never be resolved definitively. This is particularly true in light of the vector nature of the growing quantity of data generated by social media and volunteered geographic information.
In this study, we found BVPC measured at the 500 m x 500 m pixel level to more accurately represent what we knew to be true about the cities in California that we were familiar with. We examined the “reasonableness” of several buildings in these towns for their height and areal extent. Wealthy areas such as Palos Verdes in Los Angeles and Montecito in Santa Barbara had high BVPC values that were dampened when represented by census tracts. Low BVPC areas like Isla Vista in Santa Barbara and central Los Angeles were identified by both raster and vector representation because they were often in the most densely populated areas of the cities and thus had smaller census tracts. We are not convinced that the BV-GINI measured at this scale captures something valid or meaningful about inequality in our cities. Los Angeles was identified as the city with the highest BV-GINI derived from census tracts (0.406) and second highest defined from raster cells (0.263). We are not sure that this resulted from the sheer size of Los Angeles relative to the other cities. The GINI values for all 9 cities are generally lower than GINI coefficients derived for income. California has one of the higher GINI coefficients in the U.S. for income at a value of 0.490 (U.S. Census Bureau, 2010). There is no reason to believe that absolute measures of BV-GINI will correspond with income or wealth measures of GINI coefficient. Our results are generally consistent with other studies and we were pleased to see that BVPC did increase with decreasing intensity of development. We were also pleased to find that BVPC did positively correlate with median household income within cities. This exploratory work has demonstrated that BVPC can be measured at relatively fine spatial resolution and juxtaposed with corresponding demographic information. We focused on easily available building footprint data available for the cities of California and tested our hypothesis. However, we hope future research will cover a greater diversity of cities in a variety of countries and shed more light on the variability and meaning of these numbers throughout the world. This will of course entail availability of such remotely sensed building footprint data, population and land cover data becoming available for other countries around the world. With the increasing availability of global coverage of remotely sensed data, it is bound to happen sooner than later.
Conclusions
We have measured building volume per capita at 500 m x 500 m and census tract levels of spatial resolution for 9 cities in California. Our measurements are conciliant with other findings that wealthy areas with smaller families tend to have more building volume per person as might be expected. In addition, within a given city, BVPC tended to increase as intensity of development decreased (where Intensity of Development was characterized by NLCD data from the USGS). BVPC also positively and significantly corresponds with median household income in each of the 9 cities. We characterized variability of BVPC by constructing a Lorenz curve of cumulative percent of population vs. cumulative percent of building volume to produce a BV-GINI value for each city based on both tracts and raster cells. Further research is needed to develop a larger dataset of BV-GINI values for urban areas around the world.
California is second only to Hawaii for having the status of being the “most overcrowded” state. Two main contributing factors are the high international migration rates and high housing costs (Cox, 2016). BVPC, developed using EO data, provides an accurate picture of living space, and furnishes us with information regarding income inequality and affordability of housing in cities. Moreover, BVPC can be aggregated to any required spatial unit of analysis. BVPC also helps to surmount the shortcomings associated with the established methods in census, such as PPR as a method of measuring overcrowding.
It is projected that by 2050, about two-thirds of the world population will be living in urban areas, and this will be mostly in lower and lower-middle income countries. With the increase of population density in cities, the problems of overcrowding, income inequality, and affordable housing will only be aggravated. In such circumstances, making progress toward achieving the SDG 11 goals of making cities “inclusive, safe, resilient and sustainable” requires an objective, easily executable measure of income inequality, housing and population density, which the BVPC can adequately provide. With the greater availability of remotely sensed building height data, and other associated EO data we see the possibility of expanding the measure of BVPC to other cities, and countries. While building height information is increasingly being incorporated in Global Human Settlement layer (Global Human Settlement - Home - European Commission, 2016), extending the use of BVPC as an indicator or supplemental indicator to the SDGs in other countries of the world would require global coverage of Radar or Lidar based information to develop high spatial resolution elevation models of buildings. Studies have demonstrated that laser scatterometer satellites have high correlations with LIDAR derived measures of urban built-up volume (Mathews et al., 2019), and given that lidar data is expensive, scatterometer data might provide a better alternative for monitoring vertical growth and horizontal expansion of cities across the world. Characterizing the 3-D nature of urban form is increasingly recognized as a vital component of understanding global environmental change (Mahtta et al., 2019). The use of Seawinds radar data in conjunction with nighttime satellite imagery has shed light on the aggregate trajectory of urban form noting that cities in China tend to be growing up, whereas cities in India are spreading out (Frolking et al., 2013). This study is modeled on cities in a developed country. However, we anticipate that researchers in developing countries would be prompted to undertake similar studies of understanding how BVPC varies both within a city and between regions of the world as a logical next step in understanding and monitoring many of the phenomena related to the SDGs. With a clearer picture of the spatial distribution of the disadvantaged pockets in a city it will undoubtedly be helpful for government and other social agencies to direct resources to such areas, and to monitor efforts related to sustainability and social justice.
Data Availability Statement
Publicly available datasets were analyzed in this study. This data can be found here: https://data.sfgov.org/Housing-and-Buildings/Building-Footprints-File-Geodatabase-Format-/asx6-3trm, https://egis-lacounty.hub.arcgis.com/datasets/countywide-building-outlines-2014.
Author Contributions
TG and PS: conceptualization and formal analysis. SA and TG: methodology and software. PS and TG: validation. SA and LC: investigation. TG: data curation. PS: visualization. All authors: writing, contributed to the article, and approved the submitted version.
Funding
LC was funded by an IRC/Marie Skłodowska-Curie CAROLINE Postdoctoral Fellowship (IRC-CLNE/2017/567).
Conflict of Interest
The authors declare that the research was conducted in the absence of any commercial or financial relationships that could be construed as a potential conflict of interest.
Supplementary Material
The Supplementary Material for this article can be found online at: https://www.frontiersin.org/articles/10.3389/frsc.2020.00037/full#supplementary-material
Figure S1. Census tract and raster cell representation of BVPC for Sacramento, California.
Figure S2. Census tract and raster cell representation of BVPC for Modesto, California.
Figure S3. Census tract and raster cell representation of BVPC for Fresno, California.
Figure S4. Census tract and raster cell representation of BVPC for Santa Barbara, California.
Figure S5. Census tract and raster cell representation of BVPC for San Francisco, California.
Figure S6. Census tract and raster cell representation of BVPC for Bakersfield, California.
Figure S7. Census tract and raster cell representation of BVPC for Fullerton, California.
Figure S8. Census tract and raster cell representation of BVPC for Los Angeles, California.
Footnotes
1. ^United Nations. Sustainable Development Goals, Goal 11. Sustainable Development Knowledge Platform. Available online at: https://sustainabledevelopment.un.org/sdg11 (accessed March 26, 2020).
2. ^City and County of San Francisco. Building Footprints (File Geodatabase Format), DataSF. Available online at: https://data.sfgov.org/Housing-and-Buildings/Building-Footprints-File-Geodatabase-Format-/asx6-3trm (accessed March 26, 2020).
3. ^Microsoft Building Footprint Data - OpenStreetMap Wiki Available online at: https://wiki.openstreetmap.org/wiki/Microsoft_Building_Footprint_Data (accessed March 26, 2020).
4. ^Oak Ridge National Laboratory, Home | LandScanTM Available online at: https://landscan.ornl.gov/ (accessed March 26, 2020).
5. ^United States Geological Survey (USGS). National Land Cover Database. Available online at: https://www.usgs.gov/centers/eros/science/national-land-cover-database?qt-science_center_objects=0 (accessed March 26, 2020).
References
Blei, A. M., Angel, S., Civco, D. L., Liu, Y., and Zhang, X. (2018). Accuracy Assessment and Map Comparisons for Monitoring Urban Expansion: The Atlas of Urban Expansion and the Global Human Settlement Layer. Lincoln: Institute of Land Policy.
Copernicus (2020). Europe's eyes on Earth. Available online at: https://www.copernicus.eu/en (accessed March 26, 2020).
Cox, W. (2016). Overcrowded California|Newgeography.com. New Geography. Available online at: https://www.newgeography.com/content/005452-overcrowded-california (accessed March 26, 2020).
Elvidge, C. D., Baugh, K. E., Anderson, S. J., Sutton, P. C., and Ghosh, T. (2012). The lumen gini coefficient: a satellite imagery derived human development index. Soc. Geogr. Discuss. 8, 27–59. doi: 10.5194/sgd-8-27-2012
Eurostat (2020). Your Key to European Statistics. Available online at: https://ec.europa.eu/eurostat/371 (accessed July 7, 2020).
Frolking, S., Milliman, T., Seto, K. C., and Friedl, M. A. (2013). A global fingerprint of macro-scale changes in urban structure from 1999 to 2009. Environ. Res. Lett. 8:024004. doi: 10.1088/1748-9326/8/2/024004
Ghosh, T., Anderson, S. J., Elvidge, C. D., and Sutton, P. C. (2013). Using nighttime satellite imagery as a proxy measure of human well-being. Sustain. Sci. Pract. Policy 5, 1–32. doi: 10.3390/su5124988
Ghosh, T., Powell, L. R., Elvidge, D. C., Baugh, E. K., Sutton, C. P., and Anderson, S. (2010). Shedding light on the global distribution of economic activity. Open Geog. J. 3, 147–160. doi: 10.2174/1874923201003010147
Global Human Settlement - Home - European Commission (2016). GHSL - Global Human Settlement Layer: A New Open and Free Tool for Assessing the Human Presence on the Planet. Available online at: https://ghsl.jrc.ec.europa.eu/ (accessed March 26, 2020).
Goldblatt, R., You, W., Hanson, G., and Khandelwal, A. K. (2016). Detecting the boundaries of urban areas in india: a dataset for pixel-based image classification in google earth engine. Remote Sens. 8:634. doi: 10.3390/rs8080634
Henderson, J. V., Storeygard, A., and Weil, D. N. (2012). Measuring economic growth from outer space. Am. Econ. Rev. 102, 994–1028. doi: 10.1257/aer.102.2.994
Hu, Y., and Yao, J. (2019). Illuminating Economic Growth. IMF. Available online at: https://www.imf.org/en/Publications/WP/Issues/2019/04/09/Illuminating-Economic-Growth-46670 (accessed March 26, 2020).
Ko Ko Lwin, Y. M. (2011). “Estimation of building population from lidar derived digital volume model,” in Spatial Analysis and Modeling in Geographical Transformation Process: GIS-based Applications, ed. R. B. T. Yuji Murayama (Dordrecht: Springer). 87–98. doi: 10.1007/978-94-007-0671-2_6
Kuffer, M., Pfeffer, K., and Sliuzas, R. (2016). Slums from space−15 years of slum mapping using remote sensing. Remote Sens. 8:455. doi: 10.3390/rs8060455
Kuffer, M., Wang, J., Nagenborg, M., Pfeffer, K., Kohli, D., Sliuzas, R., et al. (2018). The scope of earth-observation to improve the consistency of the SDG slum indicator. ISPRS Int. J Geoinf. 7:428. doi: 10.3390/ijgi7110428
Leigh, A., Jencks, C., and Smeeding, T. M. (2011). “Health and economic inequality,” in The Oxford Handbook of Economic Inequality, eds. B. Nolan, W. Salverda, and T. M. Smeeding. (Oxford: Oxford University Press)
Lilford, R., Kyobutungi, C., Ndugwa, R., Sartori, J., Watson, S. I., Sliuzas, R., et al. (2019). Because space matters: conceptual framework to help distinguish slum from non-slum urban areas. BMJ Glob Health 4:e001267. doi: 10.1136/bmjgh-2018-001267
Lorenz, M. O. (1905). Methods of measuring the concentration of wealth. Publications Am. Stat. Assoc. 9, 209–219. doi: 10.2307/2276207
Los Angeles County GIS Data Portal Countywide Building Outlines – 2014 Update – Public Domain Release (2016). Available online at: https://egis3.lacounty.gov/dataportal/2016/11/03/countywide-building-outlines-2014-update-public-domain-release/ (accessed March 26, 2020).
Lu, Z., Im, J., and Quackenbush, L. (2011). A volumetric approach to population estimation using lidar remote sensing. Photogramm. Eng. Remote Sens. 77, 1145–1156. doi: 10.14358/PERS.77.11.1145
Lynch, J., Smith, G. D., Harper, S., Hillemeier, M., Ross, N., Kaplan, G. A., et al. (2004). Is income inequality a determinant of population health? Part 1. a systematic review. Milbank Q. 82, 5–99. doi: 10.1111/j.0887-378X.2004.00302.x
Mahtta, R., Mahendra, A., and Seto, K. C. (2019). Building up or spreading out? Typologies of urban growth across 478 cities of 1 million+. Environ. Res. Lett. 14:124077. doi: 10.1088/1748-9326/ab59bf
Mathews, A. J., Frazier, A. E., Nghiem, S. V., Neumann, G., and Zhao, Y. (2019). Satellite scatterometer estimation of urban built-up volume: validation with airborne lidar data. Int. J. Appl. Earth Obs. Geoinf. 77, 100–107. doi: 10.1016/j.jag.2019.01.004
Mellander, C., Lobo, J., Stolarick, K., and Matheson, Z. (2015). Night-time light data: a good proxy measure for economic activity? PLoS ONE 10:e0139779. doi: 10.1371/journal.pone.0139779
Powell, R. L., Roberts, D. A., Dennison, P. E., and Hess, L. L. (2007). Sub-pixel mapping of urban land cover using multiple endmember spectral mixture analysis: Manaus, Brazil. Remote Sens. Environ. 106, 253–267. doi: 10.1016/j.rse.2006.09.005
Reddy, A., and Leslie, T. F. (2015). Volume per capita as a useful measure of residential space. Urban Geogr. 36, 1099–1112. doi: 10.1080/02723638.2015.1060696
Solari, C. D., and Mare, R. D. (2012). Housing crowding effects on children's wellbeing. Soc. Sci. Res. 41, 464–476. doi: 10.1016/j.ssresearch.2011.09.012
Statistics Poland, SDG. (2020). Goal 10 - Reduce Inequality Within and Among Countries; Indicator 10.3.a - Gini Oefficient - Distribution of Income Data Metadata. Available online at: http://www.sdg.gov.pl/en/statistics_nat/10-3-a/ (accessed March 26, 2020).
Sutton, P. C. (2003). A scale-adjusted measure of “Urban sprawl” using nighttime satellite imagery. Remote Sens. Environ. 86, 353–369. doi: 10.1016/S0034-4257(03)00078-6
Sutton, P. C., Cova, T. J., and Elvidge, C. D. (2006). Mapping “Exurbia” in the conterminous united states using nighttime satellite imagery. Geocarto Int. 21, 39–45. doi: 10.1080/10106040608542382
Tomás, L., Fonseca, L., Almeida, C., Leonardi, F., and Pereira, M. (2016). Urban population estimation based on residential buildings volume using IKONOS-2 images and lidar data. Int. J. Remote Sens. 37, 1–28. doi: 10.1080/01431161.2015.1121301
U.S. Census Bureau (2010). TIGER/Line shapefiles. Available online at: https://www.census.gov/geographies/mapping-files/time-series/geo/tiger-line-file.html (accessed March 26, 2020).
United Nations Development Programme (UNDP) (2015). Sustainable Development Goals. Available online at: https://www.undp.org/content/undp/en/home/sustainable-development-goals.html (accessed July 7, 2020).
United Nations Environment Programme (UNEP). (2019). “We Lack Data for 68% of SDG Indicators” science Policy Business Forum, Editorial by Jillian Campbell (UNEP Chief Statistician). Available online at: http://un-sbpf.org (accessed March 16, 2020).
UNSTATS. (2019). Global Indicator Framework for the Sustainable Development Goals and Targets of the 2030 Agenda for Sustainable Development Goals and Targets (From the 2030 Agenda for Sustainable Development). Available online at: https://unstats.un.org/sdgs/indicators/Global%20Indicator%20Framework%20after%202019%20refinement_Eng.pdf (accessed March 26, 2020).
Wilkinson, R. G., and Pickett, K. E. (2006). Income inequality and population health: a review and explanation of the evidence. Soc. Sci. Med. 62, 1768–1784. doi: 10.1016/j.socscimed.2005.08.036
Xie, Y., Weng, A., and Weng, Q. (2015). Population estimation of urban residential communities using remotely sensed morphologic data. IEEE Geosci. Remote Sens. Lett. 12, 1111–1115. doi: 10.1109/LGRS.2014.2385597
Keywords: EO of SDGs, inequality, building volume, spatially explicit GINI proxy, SDG 10
Citation: Ghosh T, Coscieme L, Anderson SJ and Sutton PC (2020) Building Volume Per Capita (BVPC): A Spatially Explicit Measure of Inequality Relevant to the SDGs. Front. Sustain. Cities 2:37. doi: 10.3389/frsc.2020.00037
Received: 22 January 2020; Accepted: 12 June 2020;
Published: 23 July 2020.
Edited by:
Tathagata Chatterji, Xavier University, IndiaReviewed by:
Yonn Dierwechter, University of Washington Tacoma, United StatesGengyuan Liu, Beijing Normal University, China
Copyright © 2020 Ghosh, Coscieme, Anderson and Sutton. This is an open-access article distributed under the terms of the Creative Commons Attribution License (CC BY). The use, distribution or reproduction in other forums is permitted, provided the original author(s) and the copyright owner(s) are credited and that the original publication in this journal is cited, in accordance with accepted academic practice. No use, distribution or reproduction is permitted which does not comply with these terms.
*Correspondence: Tilottama Ghosh, dGdob3NoQG1pbmVzLmVkdQ==; Paul C. Sutton, cGF1bC5zdXR0b25AZHUuZWR1