- 1The Ohio State University, Columbus, OH, United States
- 2Veterinary Medicine, Haramaya University, Dire Dawa, Ethiopia
- 3Global One Health Initiative, The Ohio State University, Addis Ababa, Ethiopia
- 4Department of Environmental and Global Health, University of Florida, Gainesville, FL, United States
- 5Emerging Pathogens Institute, University of Florida, Gainesville, FL, United States
- 6Chan Zuckerberg Biohub, San Francisco, CA, United States
- 7Chan Zuckerberg Initiative, Redwood City, CA, United States
- 8Department of Rural Development and Agricultural Extension, Haramaya University, Dire Dawa, Ethiopia
- 9Department of Pediatrics, Washington University, St. Louis, MI, United States
High Campylobacter prevalence during early childhood has been associated with stunting and environmental enteric dysfunction (EED), especially in low resource settings. This study assessed the prevalence, diversity, abundance, and co-occurrence of Campylobacter spp. in stools from children in a rural area of eastern Ethiopia and their association with microbiome, diarrhea, and EED in children. Stool samples (n = 100) were collected from randomly selected children (age range: 360–498 days) in five kebeles in Haramaya District, Ethiopia. Diarrhea, compromised gut permeability, and gut inflammation were observed in 48, 45, and 57% of children, respectively. Campylobacter prevalence and species diversity were assessed using PCR and meta-total RNA sequencing (MeTRS). The prevalence of Campylobacter spp. in the children's stools was 50% (41–60%) by PCR and 88% (80–93.6%) by MeTRS (P < 0.01). Further, seven Campylobacter species (Campylobacter jejuni, Campylobacter upsaliensis, Campylobacter hyointestinalis, Campylobacter coli, Campylobacter sp. RM6137, uncultured Campylobacter sp., and Campylobacter sp. RM12175) were detected by MeTRS in at least 40% of children stools in high abundance (>1.76-log read per million per positive stool sample). Four clusters of Campylobacter species (5–12 species per cluster) co-occurred in the stool samples, suggesting that Campylobacter colonization of children may have occurred through multiple reservoirs or from a reservoir in which several Campylobacter species may co-inhabit. No associations between Campylobacter spp., EED, and diarrhea were detected in this cross-sectional study; however, characteristic microbiome profiles were identified based on the prevalence of Campylobacter spp., EED severity, and diarrhea. Forty-seven bacterial species were correlated with Campylobacter, and 13 of them also correlated with gut permeability, gut inflammation and/or EED severity. Forty-nine species not correlated with Campylobacter were correlated with gut permeability, gut inflammation, EED severity and/or diarrhea. This study demonstrated that (1) in addition to C. jejuni and C. coli, multiple non-thermophilic Campylobacter spp. (i.e., Campylobacter hyointestinalis, Campylobacter fetus, and Campylobacter concisus) were frequently detected in the children's stools and (2) the Campylobacter, gut permeability, gut inflammation, EED severity, and diarrhea were associated with characteristic microbiome composition. Additional spatial and longitudinal studies are needed to identify environmental reservoirs and sources of infection of children with disparate Campylobacter species and to better define their associations with EED in low-income countries.
Introduction
Campylobacter species are the most common zoonotic pathogens and the most frequent bacterial cause of foodborne disease worldwide (1). Campylobacter infection is frequently asymptomatic, and clinical cases may present with symptoms ranging from diarrhea, abdominal pain, and fever to severe consequences like reactive arthritis and, although rarely occurring, Guillain–Barré syndrome (2). Among the cases of diarrhea in children <5 years old, 15% was caused by Campylobacter infections (3). Warm-blooded animals, and particularly avian species, are common reservoir hosts for Campylobacter and infection in animals in most cases is asymptomatic. Campylobacter transmission from animal reservoirs to humans may occur through multiple routes, including contaminated food (especially poultry meat) and water, the environment, and contact with infected animals (4, 5). Children can be exposed to Campylobacter spp. directly or indirectly through exposure to animal feces (6).
Recent studies have also shown association of both symptomatic and asymptomatic Campylobacter infections with growth faltering in children from developing countries (7, 8). Approximately 24 million (35.2%) children under five, from East Africa were stunted, 4.1 million (6%) were wasted, and 2.9 million (4.3%) were overweight in 2018 (9). High exposure to enteric pathogens may result in environmental enteric dysfunction (EED), a subclinical disorder of the small intestine characterized by villous atrophy, crypt elongation, inflammatory cells infiltration of the crypts and a loss of barrier function or increased permeability (10). EED is considered to be involved in the causal pathway from pathogen exposure to stunting (11, 12). A study in multi-country settings, Campylobacter spp. were isolated in both diarrheic and non-diarrheic children in their first and second year of life (13). In addition to these, the MAL-ED project revealed a high Campylobacter infection in children in eight low-resource settings and this was associated with growth shortfalls, increased intestinal permeability, and intestinal and systemic inflammation at 24 months of age (7).
In Ethiopia, the rate of stunting among children under five is alarmingly high (38% in 2016); and a recent study indicated that the average height-for-age Z-score (HAZ) in a large sample of Ethiopian children decreased from −0.7 to −2.0 standard deviations between 6 and 18 months of age (14). In previous studies, high prevalence of thermophilic Campylobacter spp. in humans and domestic animals have been documented (15–18). Studies from Gondar, Hawassa and Jimma, Ethiopia indicated that Campylobacter was a major cause of diarrhea in children <5 years (15, 16) with high prevalence of Campylobacter in children who had exposure to domestic animals mainly chicken (15). Evidence are still lacking in Ethiopia concerning the association between Campylobacter spp. and EED in children. Thermotolerant Campylobacter spp. (i.e., Campylobacter jejuni, Campylobacter coli, Campylobacter lari, and Campylobacter upsaliensis) are frequent causal agents of campylobacterioisis. However, infection of children with non-thermophilic Campylobacter spp. (e.g., Campylobacter hyointestinalis, Campylobacter fetus, Campylobacter showae, and Campylobacter concisus) is largely underestimated due to unsuitable culturing methods and their public health risks specifically in EED pathogenesis is not known (19, 20).
Improving gut health in children can improve growth and cognitive development and the efficacy of oral vaccines (21). The fight against stunting and wasting is the prioritized agenda of several regional, national and international interventions in low and middle-income countries (22). Understanding the causes of EED and specifically characterizing the associated pathogens provide opportunities to design effective interventions to improve the health and well-being of children. Therefore, the current study is aimed at estimating the prevalence, abundance, diversity, and co-occurrence of Campylobacter spp. in children stools collected in the Haramaya District/Woreda, East Hararghe zone, Oromia region, in rural eastern Ethiopia, and to assess their association with EED, diarrhea, and host microbiome. This study is a part of the cross-sectional formative research of the Campylobacter Genomics and Environmental Enteric Dysfunction (CAGED) project, which included an epidemiological investigation of Campylobacter exposure, EED, stunting, and their associated risk factors. Results provided by this cross-sectional study will provide a strong baseline for examining the role of Campylobacter in EED.
Materials and Methods
Study Area
The study was conducted in five rural kebeles (Biftu Geda, Damota, Finkile, Gobe Chala, and Negeya; smallest administrative unit in Ethiopia) in the Haramaya District/Woreda, East Hararghe zone of Oromia Regional state, Eastern Ethiopia (Figure 1). Haramaya District is located about 525 km from Addis Ababa (capital city of Ethiopia). The altitude of Haramaya District ranges from 1,400 to 2,340 meters above sea level. Haramaya District has 36 rural kebeles and three urban kebeles. The national census of 2007 reported a total population of 271,018 (138,282 men and 132,736 women) (23) for this District. A survey of the land in Haramaya District shows that 36.1% is arable or cultivable, 2.3% pasture, 1.5% forest, and the remaining 60.1% is considered built-up, degraded, or otherwise unusable. The livestock population of the Haramaya District was estimated as; 111,528 cattle, 69,950 sheep, 106,145 goats, 137,545 chickens, 529 camels, and 31,385 donkeys. Khat, vegetables, and fruits represent major cash crops of this district (Haramaya District Agriculture and Livestock office; unpublished data).
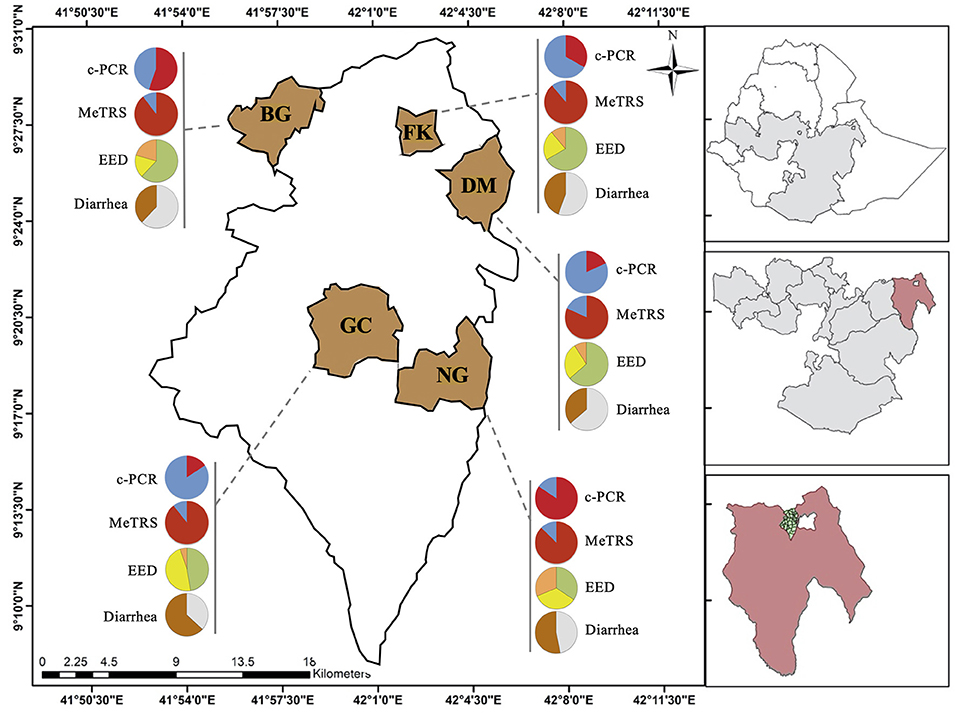
Figure 1. Prevalence of Campylobacter, environmental enteric dysfunction (EED) and diarrhea in children in the five kebeles from Haramaya District (East Ethiopia). Pie graphs in red (positive for Campylobacter) and blue (negative for Campylobacter) represent the Campylobacter prevalence in stool samples (n = 100) collected from the designated kebele. Prevalence was determined using conventional PCR (c-PCR) or MeTRS (meta-total RNA sequencing). Pie graphs in green (“normal”), yellow (“moderate” EED), and orange (“severe” EED) represent the prevalence and severity of the EED for the designated kebele. Additional details concerning the EED severity determination are presented in Table S1. Pie graphs in brown (child currently having or had diarrhea in the past 15 days), gray (child had no diarrhea in the past 15 days) represent the diarrheal status of the children. GC, Gobe Chala; NG, Negaya; FK, Finkle; DM, Damota; BG: Biftu Geda.
Study Design and Sample Size Calculation
A cross-sectional study was conducted on children between October 2018 to December 2018 (age range: 360–498 days). For the prevalence estimation, sample size was calculated based on a binomial distribution. A sample of 100 children allows estimation of 50% prevalence with a precision of 10% at 95% confidence interval, and a power of 80%. The target number of 100 children was distributed over the five kebeles (between 9 and 32 children per kebeles) based on the number of children in the sampling frame but rounded to the nearest multiple of 10. Full details of the enrollment process are described in Chen et al. (under review).
Sample Collection and Transportation
Stool samples were collected weekly from 10 children in one of the selected kebeles over 3 month period until the desired sample size (n = 100) was achieved. Child caretakers were invited to bring the children to the local health post for stool sampling. The samples were collected in a clean plastic sheet and then immediately transferred into four FluidX™ 2D-Barcoded 2.0 mL sample storage tubes (Thermo Scientific™, Waltham, MA, USA) and kept in an ice box and transferred to the laboratory at Haramaya University on the same day. Sample ID, date, and time of collection were verified, and samples were stored at −80°C until further use for DNA extraction. The child caretaker was asked whether the child had diarrhea during the 15 days before collection. Additional details concerning the diarrhea scoring are described in Chen et al. (under review).
Prevalence and Measurement of EED
Prevalence of EED and its measurements are described in detail in Chen et al. (under review). EED was determined by assessing both gut permeability and inflammation. The lactulose absorption (L%) was measured via sugar absorption test, as previously described (24), and was used as a maker to assess the gut permeability of the children. Classification of EED severity was performed as previously described (25). The child was considered “normal” if the L% was lower than 0.2; “moderate” if the L% was between 0.2 and 0.45, and “severe” if the L% was above 0.45. Myeloperoxidase (MPO) in the stool samples was measured using a commercially available enzyme linked immunosorbent assay (MPO RUO, Alpco, Salem, NH) to assess the gut inflammation. The child was considered having “normal” gut integrity if the [MPO] was lower than 2,000 ng/ml, “moderate” gut inflammation if the [MPO] was in between 2,000 and 11,000 ng/ml, and “severe” gut inflammation if the [MPO] was above 11,000 ng/ml. Due to the lack of correlation between the abundance of Campylobacter in stool and lactulose absorption and MPO data (r2 < 0.2; P > 0.05), the EED severity in the children was estimated by cross-tabulating the classifications generated for both L% and [MPO] (Figure S1).
Extraction of Genomic DNA
Extraction of the genomic DNA from stool samples was performed by using PurelinkTM Microbiome DNA purification kit (Invitrogen, Carlsbad, CA, USA). Traces of RNA were removed using RNAse treatment (Thermo Scientific™, Waltham, MA, USA) as previously described (26, 27). Genomic DNA was resuspended in 50 μl of nuclease free water (Qiagen, CA, USA) and stored at −20°C for further use. Quality and quantity of the extracted DNA was assessed using 1.5% agarose gel electrophoresis and Nanodrop 2000C Spectrophotometer (Thermo Scientific™, Waltham, MA, USA).
Detection of Campylobacter spp. in child Stool Samples Using Conventional PCR
Detection of Campylobacter spp. in DNA from stool samples was performed using multiplex conventional PCR with the GoTaq Green Master Mix kit (Promega Life Sciences, Madison, WI, USA). C. jejuni 81–176, C. coli ATCC33559, and sterile water were used as controls. Campylobacter genus-specific PCR was performed using 16S RNA primers and Campylobacter species-specific PCR was performed using ceuE primers for the detection of C. coli and mapA primers for the detection of C. jejuni (28). The primers used and the expected PCR product sizes are described in Table S1. The PCR was performed as described in Denis et al. (28) in a Mastercycler nexus gradient PCR system (Eppendorf, Hamburg, Germany). PCR products were visualized using 1.5% agarose gel (VWR International, Radnor, PA, USA) under UV light.
RNA Extraction, Library Preparation and Sequencing
RNA extraction was performed using approximately 0.25 g of stool sample with the Quick-RNA Fecal/Soil Microbe Microprep Kit (Zymo Research, CA, USA) according to the manufacturer's protocol. Four water samples were used as controls during extraction and library preparation. RNA concentration was measured using Qubit (Invitrogen, Carlsbad, CA, USA). Meta-total RNA sequencing (MeTRS) was used to analyze the microbiome composition of the children stools based on previously published work (29). The library generation was performed with NEBNext® Ultra™ II RNA Library Prep (New England Biolabs, MA, USA). Sequencing was performed to obtain ~400M reads using the Illumina NextSeq (Illumina, Inc., San Diego, CA, USA) across the 100 stool samples. For each sample, 25 pg of External RNA Controls Consortium (ERCC) RNA Spike-In Mix (Life Technologies, Carlsbad, CA, USA) was added prior to library preparation to determine the limit of detection for each sample. The average lower limit of detection was 49 attomoles. The average RNA input per samples was calculated using the following equation (total input RNA = ercc_pg/ercc_reads * total_reads) where “ercc_reads” is the number of reads generated from the water control and “total_reads” is the number of reads generated from test RNA including the water control. The average input was ~66 ng/sample.
Bioinformatic Analysis of the MeTRS Data
MeTRS data analysis was performed using IDseq pipeline version 3.7 available at https://github.com/chanzuckerberg/idseq-web/wiki (30). The details of the IDseq pipeline used for MeTRS data analysis is included in the Supplemental Material 1. Only identified organisms with at least 10 reads, an alignment length above 50 bp, and a Z-score above 1 were considered for the statistical analyses.
Statistical Analysis
MeTRS abundance data (reads per million; rpm) were log transformed. Statistical analyses were performed using JMP PRO 14 software (SAS Institute, Cary, NC, USA). The homogeneity of the MeTRS data for each child was analyzed using a principal component analysis (PCA) combined with restricted maximum likelihood (REML) estimation and T2 statistic test (square of the Mahalanobis distance). Similarity in the Campylobacter spp. profiles between children obtained with the MeTRS data was determined using hierarchical clustering. Discriminant analyses were performed to identify specific members of the microbial species responsible for the variability observed in the PCA and clustering data based on the designated nominal parameter used (kebeles, presence/absence of Campylobacter, EED severity, and diarrhea status). The percentage of stool samples clustering within their own group (confidence interval of 95%; CI 95%) was used to determine whether the designated nominal parameters explained the variability observed between stool samples. A Wilcoxon test was performed to identify rpm abundance differences for a given member of the microbial species based on a specific nominal parameter. Correlations between the MeTRS, EED severity, and diarrhea data were performed using a multivariate analysis combined with Pearson product-moment correlation coefficient. The co-occurrence of the Campylobacter spp. was studied using Cluster and Factoextra R packages (SAS Institute, Cary, NC, USA) on the K-means clustering data extracted from the multivariate analysis data (r2) obtained with the Campylopbacter spp. prevalence data. The optimization of the number of clusters for the PCA was performed using the Silhouette method. The abundance of each bacteria in the stool samples was estimated using MeTRS approach, which is based on RNA read quantification; therefore, we do not exclude the possibility that this approach over or under estimates the quantitative data (reads per million) described in this study.
Data Accession
MeTRS raw reads have been deposited in the IDseq platform (https://idseq.net) in accordance with the Chan Zuckerberg Biohub and Chan Zuckerberg Initiative (CZI).
Results
Prevalence of Campylobacter spp. in child Stool Using Conventional PCR
The genus-specific PCR analysis showed that 50% (40–60%, 95% CI) of the child stools (n = 100) were positive for the Campylobacter genus across the five kebeles (Table 1); however, the Campylobacter prevalence significantly differed between kebeles (P < 0.05; Figure 1 and Figure S2A). The prevalence was higher in Negeya (84.4%) compared to Biftu Geda (55.2%), followed by Finkile (33.3%), Damota (18.2%) and Gobe Chala (15.8%; Figure 1 and Figure S2A).
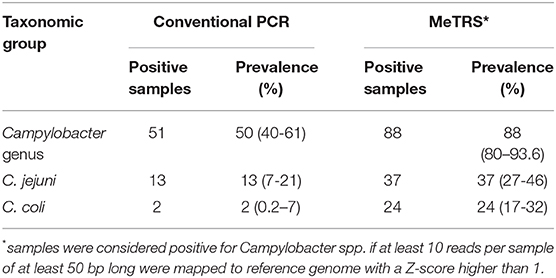
Table 1. Prevalence of Campylobacter, Campylobacter jejuni, and Campylobacter coli in child stools using conventional PCR and MeTRS approaches.
The species-specific PCR analysis showed that 13% (7–21%) of children were colonized with C. jejuni and only 2% (0.2–7%) with C. coli (Table 1). The prevalence of children positive for C. jejuni and C. coli did not differ between kebeles (Figure S2A). These results suggested that large proportion of Campylobacter in children stools likely represent other Campylobacter species, possibly including non-thermotolerant Campylobacter.
Prevalence of Campylobacter spp. in child Stools Using Meta-Total RNA Sequencing
In order to identify the full spectrum of Campylobacter species present in the stool samples, MeTRS was performed on 100 children stool samples. The MeTRS analysis showed that Campylobacter was detected in 88% (95% CI: 80–94%) of the children's stools (Table 1). A total of 27 classified Campylobacter species and 12 unclassified Campylobacter species were detected among the 88 Campylobacter positive stools based on the annotation in the NCBI database with average sequence identity of 97% for each species (https://www.ncbi.nlm.nih.gov/Taxonomy/Browser/wwwtax.cgi; Figure 2). An average of 11 Campylobacter spp. was detected per Campylobacter positive stool. Seven Campylobacter spp. (from highest to lowest prevalence; Campylobacter sp. RM12175, C. hyointestinalis, C. jejuni, Campylobacter sp. RM6137, uncultured Campylobacter sp., C. upsaliensis, and C. coli) were detected in at least 40% of the stools at high abundance (at least 1.76-log rpm per positive stool; Figure 2); and seven other Campylobacter spp. (Campylobacter sp. NCTC 13003, Campylobacter helveticus, Campylobacter lanienae, C. concisus, C. fetus, Campylobacter pinnipediorum, and C. showae) were detected in at least 40% of the stools but at lower abundance (between 0.95-log and 1.76-log rpm per positive stool; Figure 2).
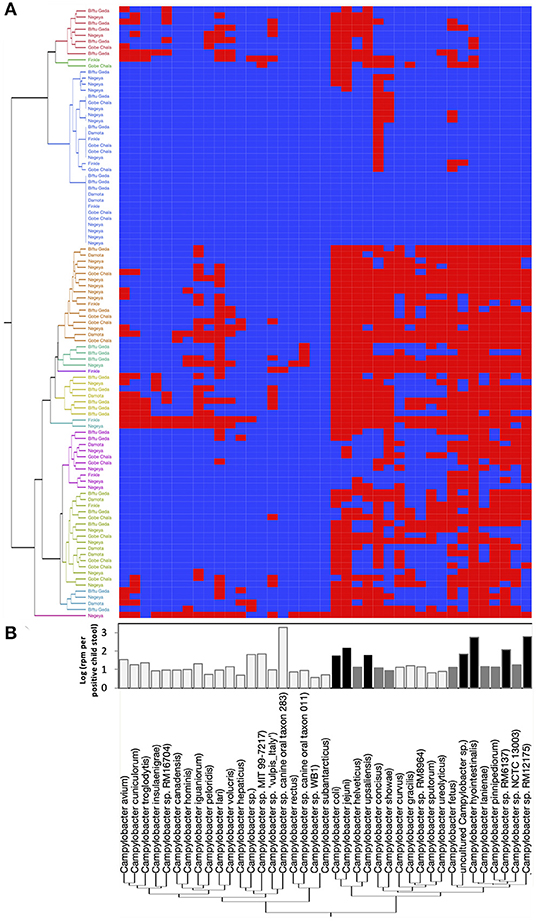
Figure 2. Campylobacter spp. prevalence, diversity, and abundance in children stools. A total of 27 classified Campylobacter spp. and 12 unclassified Campylobacter spp. were detected in the 100 child stool samples collected from children in the five kebeles using MeTRS. (A) Campylobacter spp. prevalence and diversity in the stool samples. Blue and red cells represent the absence or presence of Campylobacter spp. in the designated stool samples (cut-off; contigs number ≥ 10; Read length ≥ 50; Z-score ≥ 1). Kebeles with the same color code belong to the same cluster and therefore harbored equivalent Campylobacter sp. diversity. (B) Abundance of Campylobacter spp. in the positive stools. White bar represents Campylobacter spp. with a prevalence lower than 40% and an abundance lower than 0.95-log rpm per stool sample. Gray bar represents Campylobacter spp. with a prevalence higher than 40% and an abundance lower than 1.76-log rpm per stool sample. Black bar represents Campylobacter spp. with a prevalence higher than 40% and an abundance higher than 1.76-log rpm per stool sample. rpm: read per million.
Further, based on the prevalence data (Figure 2), it was also observed that specific Campylobacter spp. often co-occurred in the children stools (Figure 3 and Figure S3). A total of four clusters of co-occurrences (n = 5–12 Campylobacter spp. per cluster) were detected. The green cluster (C. coli, C. jejuni, C. helveticus, C. upsaliensis C. iguaniorum, and C. lari) and the red cluster (C. gracillis, C. sp. RM12175, C. sputorum, and C. ureolyticus, C. hyointestinalis, C. curvus, C. fetus, C. lanienae, C. pinnipediorum, Campylobacter sp. RM6137, Campylobacter sp. RM12175, C. showae, and uncultured Campylobacter sp.) displayed higher co-occurrence similarities compared to the blue cluster (C. rectus, C. subantarcticus, C. hepaticus, C. hominis, C. concisus, C. canadensis, and C. pelondis) and the violet cluster (C. avium, C. cuniculorum, C. insulaenigrae, C. volucris, and C. troglodytis), respectively based on dimension 1, which explained 44.4% variability between the cluster. Interestingly, the green cluster was mostly composed of the five most commonly reported thermotolerant Campylobacter species (C. coli, C. jejuni, C. helveticus, C. upsaliensis, and C. lari), while the red cluster consisted of non-thermotolerant Campylobacter species (C. hyointestinalis, C. fetus, and C. showae).
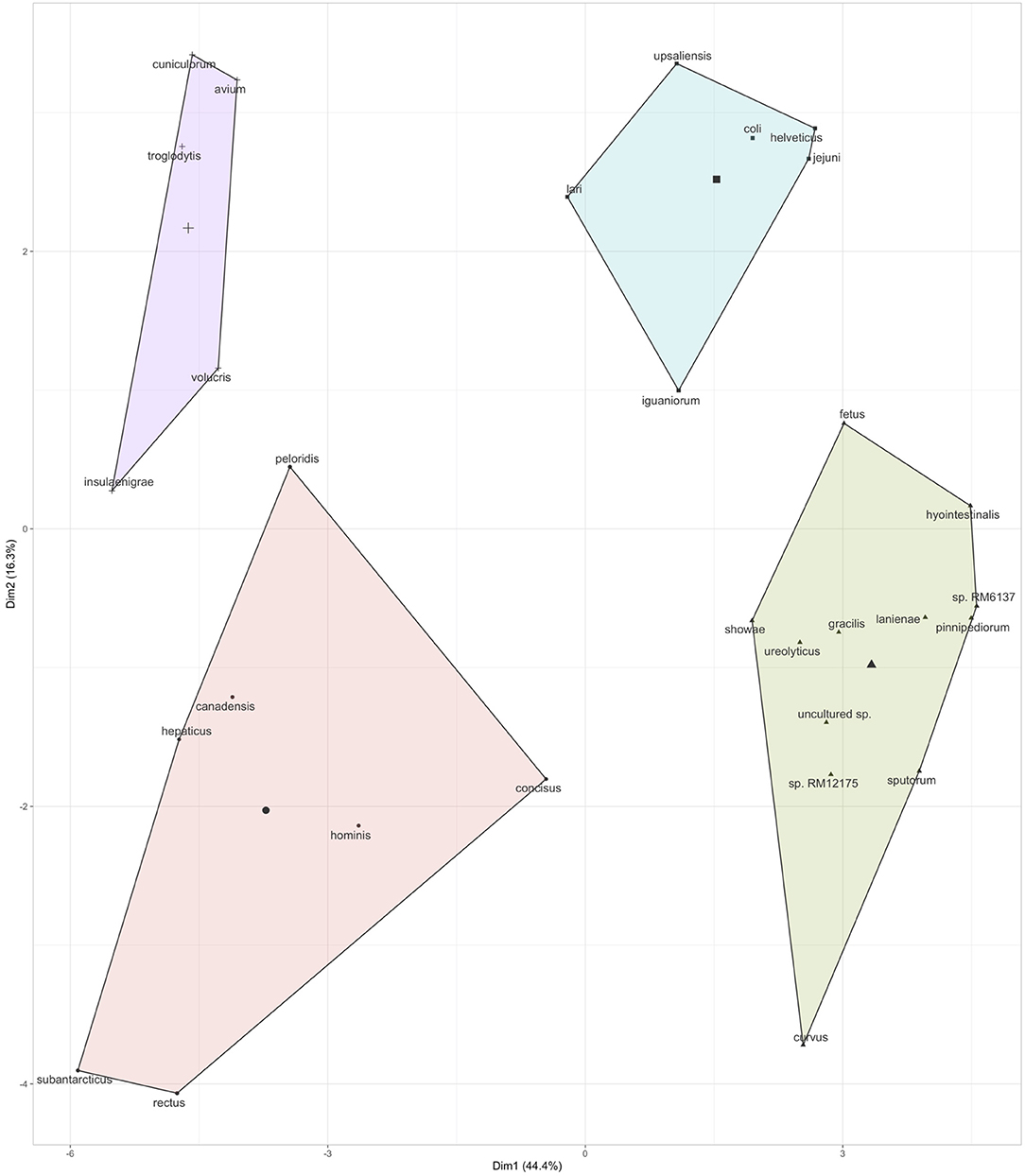
Figure 3. Co-occurrence of Campylobacter spp. in the children stool samples. Co-occurrence profiles were created using K-means clustering data extracted from the multivariate analysis data (r2) based on the prevalence of Campylobacter spp. in the stool samples (see Figure 2). Additional details regarding the multivariate data are displayed in Figure S3.
Unlike the PCR data, the distribution of the Campylobacter positive children stools (at the genus and species level), and the prevalence of the Campylobacter species in the stools were overall independent of the kebeles (P > 0.05; Figure 2, Figures S4A,B). Approximately 65% (45–84%) of the stool samples clustered within their own kebeles based on the abundance of the Campylobacter spp. detected in the stool samples (Figure S4C). Therefore, due to the high variability observed within most of the kebeles (4/5), the five kebeles were pooled into one (n = 100) to enhance the veracity of the MeTRS data described below. Only the abundance (read per million) of C. hyointestinalis and Campylobacter sp. RM12175 significantly differed between kebeles (P < 0.01; Figure S5). Finkle harbored significantly higher level of C. hyointestinalis in the stools compared to Gobe Chala, Negeya, and Biftu Geda (Figure S5A); and Damota harbored significantly higher level of Campylobacter sp. RM12175 in the stools compared to the other four kebeles (Figure S5B).
Overall, the percentage of Campylobacter positive stools was significantly higher using the MeTRS approach compared to the conventional PCR approach (P < 0.01; Figures S2A,S2B). Further, some discrepancies were observed between the two methods used (Figure S2C). Out of the 88 stools positive for Campylobacter via MeTRS, 42 of them (48%) were also identified positive via conventional PCR (16S genus-specific primers); however, five of the 50 (10%) stools positive for the Campylobacter genus via conventional PCR were not positive for the Campylobacter via MeTRS analysis (Figure S2C). Similarly, based on the species-specific PCR (ceuE and mapA primers), six of 13 (46%) and two of two (100%) stools were positive for C. jejuni and C. coli, respectively only by PCR but not with MeTRS (Figure S2C).
The Campylobacter in the Stools Was Not Associated With Diarrhea and EED Severity
To determine the role of Campylobacter in EED, the levels of lactulose and MPO were measured as indicators of EED (24, 25). Additional details concerning the prevalence of EED and stunting among children in this study are described in Chen et al. (under review).
Out of the 100 children studied, 55 children possessed “normal” gut permeability (L% below 0.2), 29 children possessed “moderate” defect in gut permeability (L% between 0.2 and 0.45), and 16 children possessed “severe” defect in gut permeability (L% ratio above 0.45). No correlations were identified when a multivariate analysis was performed between L% and the prevalence or the abundance of Campylobacter detected in the children stools (P > 0.05). On the other hand, the discriminant analysis showed that the overall Campylobacter spp. composition in the stool differed between the L% status (“normal,” “moderate,” and “severe”; r2 = 0.37; Figure S6A). Eighty two percent (45/55) of the children identified as “normal” clustered together based on the Campylobacter composition in the stool. Besides, only 66% (19/29) and 44% (7/16) of the child identified as with “moderate” and “severe” permeability defect, respectively clustered within their own group based on the Campylobacter composition in the stool (Figure S6B). Further, 28% (8/29) and 41% (7/17) of the children identified as with “moderate” and “severe” permeability defect clustered with the children identified as “normal.” Overall, the Campylobacter composition in the stool samples was associated with 58% of the children with “moderate” and “severe” gut permeability defect based on the L% data.
Similarly, based on the MPO levels, out of the 100 children studied, 43 children possessed “normal” gut ([MPO] below 2,000 ng/ml), 32 children possessed “moderate” gut inflammation ([MPO] between 2,000 and 11,000), and 25 children possessed “severe” gut inflammation ([MPO] above 11,000). No correlations were identified when a multivariate analysis was performed between [MPO] and the prevalence or the abundance of Campylobacter detected in the children stools (P > 0.05); however, the Campylobacter spp. composition in the stool differed between the inflammation status (r2 = 0.37; Figure S6C). Fifty eight percent (25/43), 88% (28/32) and 48% (12/25) of the children with inflammation status “normal,” “moderate,” and “severe,” respectively clustered within their own group based on the Campylobacter composition in the stool (Figure S6D). Only 9% (3/32) and 8% (2/25) of the children identified as having “moderate” and “severe” gut inflammation clustered with the children identified as “normal.” Overall, the Campylobacter composition in the stool was associated with 70% of the children with “moderate” and “severe” gut inflammation based on the MPO data.
Because of the lack of correlation between the Campylobacter and the L% and MPO, both parameters were combined together to build an index estimating the EED (Figure S1). Out of the 100 children studied, 50 children were classified as “normal,” 33 children having “moderate” EED, and 25 children having “severe” EED (Figure 1). As observed above, the Campylobacter spp. composition in the stool differed between the EED status (r2 = 0.38; Figure S6E). Ninety percent (45/50), 61% (20/33), and 53% (9/17) of the children identified as “normal,” “moderate,” and “severe,” respectively clustered within their own group based on the Campylobacter composition in the stool (Figure S6F). Overall, the Campylobacter composition in the stool was associated with 58% of the children with “moderate” and “severe” EED based on the EED status data.
In addition, the prevalence of child with diarrhea was recorded to determine the associations with Campylobacter spp. prevalence and abundance in the stool samples. Additional details concerning the diarrhea data are described in Chen et al. (under review). Out of the 100 children studied, 48% of the children had diarrhea on the day of the stool collection or 15 days before stool collection (Figure 1). No correlations were identified when a multivariate analysis was performed between the diarrhea data and the prevalence or abundance of Campylobacter spp. in the stool samples (P > 0.05). However, the discriminant analysis showed that overall the Campylobacter spp. composition in the stool differed based on the diarrhea status of the children (r2 = 0.32; Figure S6G). Eighty-one percent (39/48) of the children with diarrhea clustered together based on the Campylobacter composition in the stool samples, while 58% (30/52) of the children without diarrhea clustered together as a separate cluster based on the Campylobacter composition in stool (Figure S6H).
Specific Bacteria of the Stool Microbiome Correlated With the Campylobacter Prevalence and EED Severity
Post-filtering, the MeTRS identified a total of 2,353 bacteria, 642 Archaea, 17 virus/viroid, and 249 eukaryotes at the species level among the 100 children stool samples studied. The global analysis of the fecal microbiota revealed that the majority of children (n = 89) harbored similar microbiota profile (P < 0.01; Figure S7A). Between 68% and 84% of the stool samples clustered by kebeles based on the microbiota composition (Figure S7B). None of the stool samples from Damota and Biftu Geda displayed microbiome composition similarities to Finkle stool samples (Figures S7B,S7C), despite the fact that Finkle is geographically closer to Damota and Biftu Geda compared to the other kebeles (Figure 1). Further, 19 stools samples displayed higher microbiome composition similarities with Biftu Geda or Negeya stool samples compared to the original kebeles (Figures S7B,S7C), independently of the distance between the kebeles (Figure 1). Given the discriminant analysis showed that the ellipses (CI 95%) of most kebeles (4/5) were overlapping, the MeTRS data were analyzed as one population (n = 100). No distinct correlation was detected between the Campylobacter species and the virus/viroid and eukaryotes (P > 0.05, r2 < 0.25); by consequence, the majority of the results described below focused on the interconnections between Campylobacter spp. and stool bacterial community.
Our analysis showed that the bacterial composition in the stool samples was related to the overall presence of Campylobacter spp. (Figure S8A). Ninety-four percent (83/94) of the children stools positive for Campylobacter spp. clustered together based on their microbiome composition (Figure S8B). Only 58% (7/12) of the child stools negative for Campylobacter spp. clustered together in a separate cluster based on their microbiome composition. Approximately 16.9% of the bacteria identified in stool samples (n = 387/2,353) were positively or negatively correlated with the prevalence or abundance of Campylobacter in the stool samples (r2 > 0.2 or r2 < −0.20; P < 0.05). Among the bacteria correlated with Campylobacter, several of them belonged to Arcobacter, Bacillus, Bacteroides, Bifidobacterium, Capnocytophaga, Clostridium, Collinsella, Corynebacterium, Enterobacter, Enterococcus, Helicobacter, Lactobacillus, Olsenella, Paenibacillus, Pantoea, Prevotella, Serratia, Streptococcus, and Veilonella.
However, out of the 387 species mentioned above only 47 were correlated (25 negatively and 22 positively) with the prevalence or abundance of Campylobacter in the stool samples (r2 > 0.2 or r2 < −0.2; P < 0.05) and detected in at least 25 children stool samples (average of 60 children; Figure 4). Olsenella, Clostridium, and Streptococcus were the most represented genus in this subset. Anaerotignum propionicum (r2 = 0.53), Clostridium butyricum (r2 = 0.47), Hydrogenophilus islandicus (r2 = 0.46) displayed the highest positive correlation with Campylobacter abundance in the stool samples; while Burkholderiales bacterium GJ-E10 (r2 = −0.36), Pasteurellaceae bacterium NI1060 (r2 = −0.35), and Clostridium baratii (r2 = −0.38), displayed the highest negative correlation with Campylobacter abundance and/or prevalence in the stool samples.
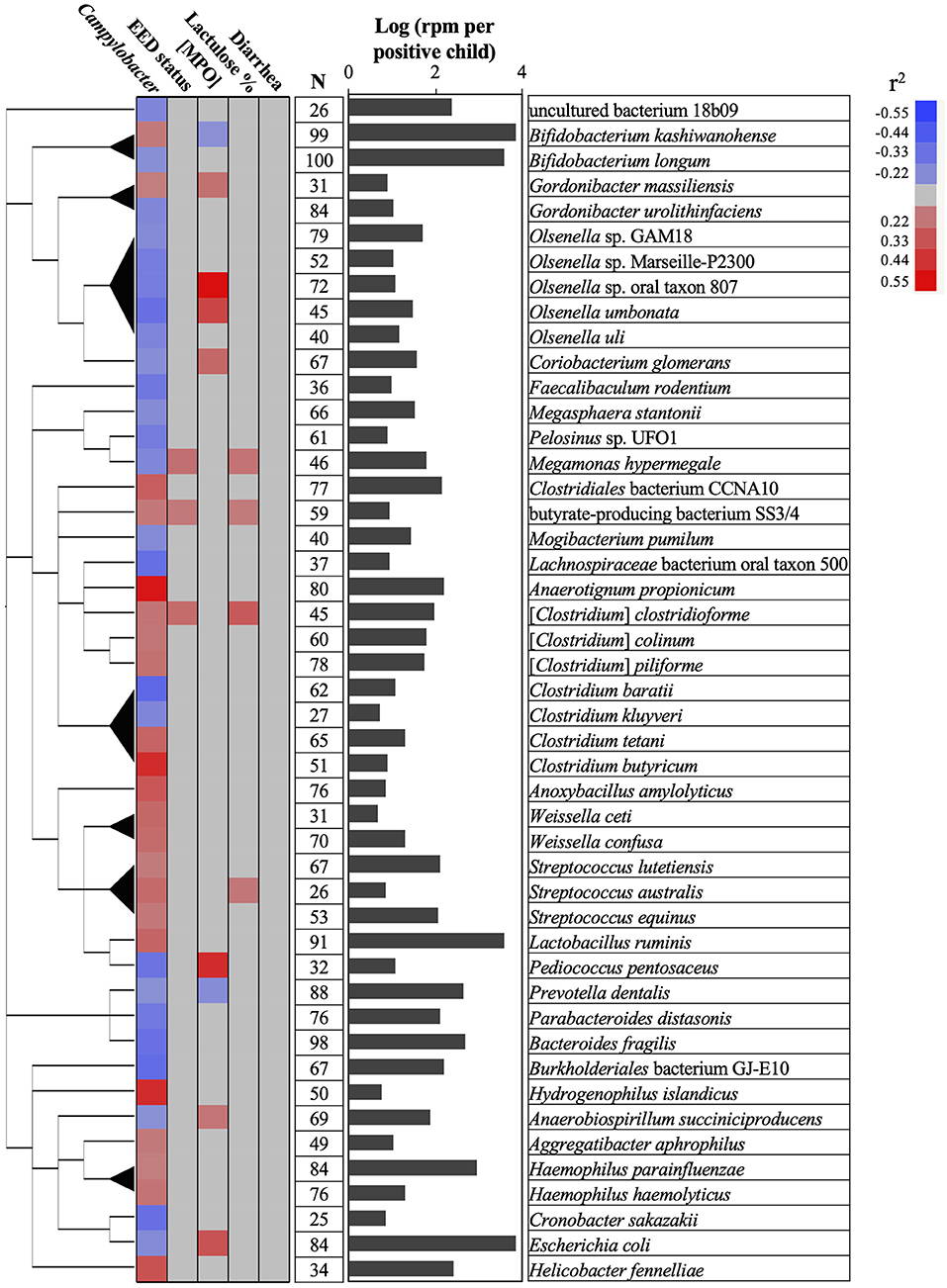
Figure 4. Associations between Campylobacter, gut permeability, gut inflammation, environmental enteric dysfunction (EED), diarrhea, and child stool microbiome. A total of 47 bacterial species positively or negatively correlated (red or blue cells, respectively) with Campylobacter prevalence and abundance (reads per million, rpm; r2 > 0.20 or r2 < −0.20; P < 0.05), gut permeability (lactulose %), [MPO] in ng/ml, EED status (“normal,” “moderate” EED, “severe” EED), and/or the diarrhea prevalence data. Additional details concerning the EED severity determination are presented in Table S1. The phylogenetic tree was built using NCBI website (https://www.ncbi.nlm.nih.gov/Taxonomy/CommonTree/wwwcmt.cgi). N: number of stools positive for the designated bacterial species. The bar graph represents the average abundance (log rpm per positive stool sample) for the selected bacterial species. rpm, read per million; MPO, myeloperoxidase.
Only Prevotella dentallis (2.63-log rpm per positive stool sample) was negatively correlated with both the Campylobacter abundance and/or prevalence in the stool samples (r2 = −0.2) and the [MPO] (r2 = −0.22). On the other hand, Butyrate-producing bacterium SS3/4, [Clostridium] clostridioforme, and Streptococcus australis were positively correlated with both the Campylobacter abundance and/or prevalence in the stool (r2 > 0.2) and at least with one of the following parameters ([MPO], lactulose%, and/or EED severity; r2 > 0.21). Anaerobiospirillum succiniciproducens, Coriobacterium glomerans, Escherichia coli, Megamonas hypermegale, Olsenella sp. oral taxon 807, Olsenella umbonata, and Pediococcus pentosaceus were negatively correlated with the Campylobacter abundance and/or prevalence in the stool (r2 < -0.2) but positively correlated with at least one of the following parameters ([MPO], lactulose%, and/or EED severity; r2 > 0.23). Only Bifidobacterium kashiwanohense was positively correlated with the Campylobacter abundance and/or prevalence in the stool (r2 > 0.21) and negatively correlated with [MPO] (r2 < −0.2).
No bacteria correlated with Campylobacter spp. were correlated with the presence or absence of diarrhea.
Specific Bacteria of the Stool Microbiome Correlated With the Gut Permeability, Gut Inflammation, EED Severity, and Diarrhea in Children
The microbiome composition was related to the gut permeability (L%), gut inflammation (MPO), and EED status (Figure S9B), as observed with the Campylobacter composition in the stool samples (Figure S6); however, higher correlations between the L%, EED status and the microbiome composition were observed compared to the correlation obtained with Campylobacter. Out of the 100 children studied, 80% (44/55), 79% (23/29), and 81% (13/16) of the children identified as “normal,” “moderate,” and “severe” based on the L%, respectively, clustered within their own group based on the microbiome composition (Figures S9A,S9B). Eighty one percent (35/43), 72% (23/32), and 68% (17/25) of the children identified as “normal,” “moderate,” and “severe” based on MPO levels, respectively, clustered within their own group based on the microbiome composition in the stool (Figures S9C,S9D). Eighty percent (40/50), 79% (26/33), and 76% (13/17) of the children identified as “normal,” “moderate,” and “severe” based on the EED status (both L% and MPO combined; Table S1), respectively, clustered within their own group based on the microbiome composition in the stool (Figures S9E,S9F). Overall, the microbiome composition was associated with 80% of the gut permeability data, 70% of the gut inflammation data, and 78% of the EED severity data observed in the children with “moderate” and “severe” status. Similarly, children with diarrhea displayed different microbiome composition compared to children with no diarrhea (Figure S9G). Out of the 100 children, 81% (42/52) of the stools from children with no diarrhea and 85% (41/48) of the stools from children with diarrhea clustered to their respective groups based on the microbiome composition (Figure S9H).
A total of 49 bacteria not-associated with Campylobacter were correlated (30 positively [r2 > 0.21; P < 0.05] and 19 negatively [r2 < −0.20; P < 0.05]) with the L%, MPO levels, and/or the EED severity and detected in at least 25 children stool samples (Figure S9I). Only Neisseria elongata was positively correlated with the EED severity (r2 = 0.31; 2.2-fold increased between the “normal” children and the children with “severe” EED) and also L% (lactulose%; r2 = 0.21; 1.7-fold increased between the children with “normal” and “severe” gut permeability). Eight bacteria (Lachnoclostridium sp. YL32, [Clostridium] bolteae, Bifidobacterium bifidum, Mordavella sp. Marseille-P3756, Bacteroides thetaiotaomicron, Streptococcus salivarius, and Libanicoccus massiliensis) highly prevalent (>71%) and abundant (>2.0-log rpm per stool sample in children with “severe” status) in the stools were positively correlated (r2 between 0.24 and 0.36) with L% or MPO levels. On the other hand, Lactobacillus mucosae and Pasteurella multocida were less prevalent (39 and 60%, respectively) and less abundant (below 1.0 and 1.5-log rpm per stool, respectively) in the stools, but they were higher (2.5- and 2-fold, respectively) in the children with “severe” gut inflammation compared to the “normal” children.
Five bacteria (Mogibacterium diversum, Ethanoligenens harbinense, Roseburia hominis, Ruminococcus sp. SR1/5, and Bacteroides dorei) were negatively correlated with the EED severity (r2 between −0.21 and −0.27) and detected in at least 40% of the children stools (Figure S9I). Interestingly, only M. diversum was negatively correlated with all the parameters studied (EED severity [r2 = −0.27], L% [r2 = −0.20], MPO [r2 = −0.26], and diarrhea prevalence [r2 = −0.25]). Further, M. diversum abundance in stools was higher in the “normal” children compared to the children with “severe” EED (2.7-fold), with “severe” L% (4-fold), and with diarrhea (2-fold). Similarly, E. harbinense was higher (2.3-fold) in the stools of “normal” children compared to the children with “severe” EED. Four bacteria (Clostridium taeniosporum, Hungatella hathewayi, Selenomonas ruminantium, and Cryotobacterium curtum) were less prevalent (between 40% and 58%) and abundant (<1.3-log rpm per stool) in the stools, but they were detected at higher level (2.2-, 2-, 2.6-, and 2-fold, respectively) in the stools of “normal” children compared to the children with “severe” MPO. Further, Selenomonas ruminantium was also detected at higher level (2-fold) in children with no diarrhea compared to the ones with diarrhea.
Three additional bacteria (Prevotella scopos, S. ruminantium, and Measphaera els) highly prevalent (>79%) and abundant (>2.1-log rpm per stool) in the stools and not-associated with Campylobacter were negatively correlated (r2 between −0.2 and −0.22) with the diarrhea data (Figure S9I).
Discussion
The purpose of this study was to estimate the prevalence, diversity, abundance and co-occurrence of Campylobacter in the stool of young children from Eastern Ethiopia and assess potential associations between Campylobacter and diarrhea and EED (31). To accomplish this task, the use of traditional microbiology approaches to isolate thermophilic Campylobacter spp. was attempted; however, due to technical and logistical challenges faced in Ethiopia, the results generated were deemed unreliable (data not shown). The fastidious nature of Campylobacter and difficulty of isolation, rendered culture and isolation unreliable for estimation of prevalence (32). By consequence, other studies utilized different approaches to estimate the prevalence of Campylobacter in stool samples, including enzyme immunoassay (EIA), PCR, and shotgun metagenomics (7, 33, 34). Two different culture independent approaches (conventional PCR and MeTRS) were used in this study to estimate the prevalence of Campylobacter in the child stool samples.
The number of child stools detected positive for the Campylobacter genus was significantly higher using the MeTRS approach (88% [80–93.6%]) compared to the genus-specific PCR approach (50% [40–61%]; 16S RNA primer). Several studies showed that shotgun metagenomics and MeTRS had at least equal sensitivity to real-time PCR based approach for different pathogens (29, 35–37). Further, discrepancies between the two detection methods was observed, especially with the species-specific PCR approach (ceuE primer for C. coli and mapA primer for C. jejuni). However, the reason for this discrepancy is unknown and needs further investigation. Many factors could affect the sensitivity of both PCR and MeTRS approaches, including the type of the sample, the quality and quantity of the extracted DNA, the agreement between the method used, the type of the organism under investigation, and the tools used to analyze the data. A recent study suggested that shotgun metagenomics might overestimate the prevalence of microbial population due to the presence of plasmid sequences within the assembled genome and the high sequences similarities between bacterial species (38). In our study, by taking an approach of sequencing the total RNA component, we are able to better identify species rather than by sequencing DNA alone. However, the genome coverage obtained with the MeTRS approach was not high enough to provide information about strain relatedness within the same species. Therefore, metagenomic studies using long read technologies or whole genome sequencing of pure isolates are still needed for source attribution purposes.
It is commonly known that C. jejuni and C. coli are leading causes of campylobacteriosis in the western world (39); however, our study revealed that most of the children stools positive for Campylobacter (94%) harbored more than one Campylobacter species. This finding suggests that Campylobacter colonization of children may have occurred through multiple reservoirs or from a reservoir in which several Campylobacter species may co-inhabit. This hypothesis is supported by the existing literature which describes colonization of multiple hosts by a given Campylobacter species; for example, C. hyointestinalis is commonly found in swine, sheep, dog, and in cattle (40). Further, twelve Campylobacter spp. (Campylobacter sp. RM12175, C. hyointestinalis, Campylobacter sp. RM6137, uncultured Campylobacter sp., C. upsaliensis, Campylobacter sp. NCTC 13003, C. helveticus, C. lanienae, C. concisus, C. fetus, C. pinnipediorum, and C. showae) in addition to C. jejuni and C. coli were detected in at least 40% of the stool samples at relatively high abundance. Our study also showed that several Campylobacter species were commonly co-occurring within the same stool samples, while others had opposite trends. However, none of the clusters representing different Campylobacter species (Figure 3) were correlated with the gut permeability, gut inflammation, EED severity, and diarrhea (data not shown). These facts raise many questions about the mechanisms underlying the Campylobacter species diversity observed and their potential effect on gut health and children's growth. A possible contributor to this diversity could be the diverse environment surrounding these children. A previous study demonstrated significant associations between Campylobacter isolated from children (under 5 years of age) and the exposure to domestic animal (pets, chickens, and pigeons) in Gondar, North Western Ethiopia (15). It was estimated that approximately 83% of the rural households in Ethiopia possess livestock (cattle, goat, and sheep and chicken) (23). Further, most of these Campylobacter species mentioned above (except Campylobacter sp. RM6137) were reported in livestock such as cattle, sheep, and goat (41). Therefore, livestock most likely plays a major role as reservoir and in the horizontal transmission of Campylobacter to children in Ethiopia. Hence, concurrently studying the prevalence and diversity of Campylobacter in livestock and children would provide key information concerning the horizontal transmission of Campylobacter between livestock and children. It is also important to notice that four of the most prevalent Campylobacter species (C. hyointestinalis, C. helveticus, C. fetus, and C. upsaliensis) detected in the children stools in this study have not been commonly reported in previous studies, compared to other species such as C. jejuni, C. coli, C. fetus, C. showae, and C. concisus, which have been associated with human disease (41, 42). The reservoirs of non-thermotolerant Campylobacters are very diverse and not well-understood especially in low to middle income countries.
Among all the Campylobacter species detected in our study (27 classified species and 12 unclassified species), the non-thermotolerant C. hyointestinalis was the second most abundant and the third most prevalent Campylobacter species in the children stools. C. hyointestinalis was previously isolated from numerous livestock species such as swine (43), cattle (43–48), sheep (43). However, its implication in human diseases is rare and sporadic (49–53). C. hyointestinalis has also been reported in developed countries, more precisely in feces collected from dairy farms in Canada (19.3%), in Finland (10.8%) and from dairy goat farms in New Zealand (2%), and in 3.2% milk samples collected from dairy farms in Italy (45, 47, 48, 54). C. hyointestinalis, as well as, other Campylobacter species were also isolated from wild boar in Japan and dogs in Canada (55, 56). By consequence, studying the prevalence, diversity, and abundance of Campylobacter species in livestock, also in domestic- and wild animals that have a high likelihood of interacting with livestock or humans is essential to understand the transmission dynamics of Campylobacter to children.
Overall, the MeTRS data showed that the microbial population in the stools in different kebeles was homogeneous between the children. No correlations between Campylobacter spp. and gut permeability, gut inflammation, EED severity, or diarrhea status were observed in our study; however, characteristic stool microbiome composition profiles were detected based on the prevalence and abundance of Campylobacter spp. in the stools, gut permeability, gut inflammation, EED severity, and diarrhea status. Up to 0.9% (n = 22 bacterial species) of the microbiome was positively correlated (r2 > 0.20) and 1.1% (n = 25 bacterial species) was negatively correlated (r2 < −0.21) with the prevalence and/or abundance of Campylobacter spp. in the stools. Among them, only Prevotella dentalis was highly prevalent (88%) and abundant (2.63-log rpm per stool) in the stools, and negatively correlated with both Campylobacter prevalence (r2 = −0.2) and the gut inflammation (r2 = −0.21). Prevotella is associated with plant-rich diets and also promotes chronic inflammation (57, 58). Prevotella is known to provide key nutrients to other bacteria of the human microbiota and may act on the pathogenicity of specific pathobionts (59–63); however, no studies demonstrated yet potential antagonistic interactions between P. dentalis and Campylobacter.
Bifidobacterium kashiwanohense was highly prevalent (99%) and abundant (3.84-log rpm per stool) in the stools and was also negatively correlated with gut inflammation (r2 = −0.2). This bacterium possesses high iron sequestration properties and might produce indole-3-lactic acids, which was shown to be a successful strategy to inhibit and compete with enteric pathogens (i.e., Salmonella and E. coli) (64, 65). However, B. kashiwanohense was also positively correlated (r2 = 0.21) with Campylobacter in our study, suggesting that the modulation of B. kashiwanohense may reduce the impact of pathobiont on the intestinal homeostasis but facilitates the persistence of Campylobacter in the child intestinal tract. On the other hand, Bifidobacterium longum was negatively correlated with Campylobacter in our study, which concords with previous published studies (66). Similarly, other bacteria (Gordonibacter urolithinfaciens, Mogibacterium pumilum, Megasphaera stantonii, E. coli, Clostridium spp., Olsenella spp., and Bacteroides fragilis) were negatively correlated with Campylobacter in the stools. Several studies showed that Bacteroides, Gordonibacter, and Escherichia produce molecules with antimicrobial and anti-inflammatory properties (lactic acids, short chain fatty acids, enterocins, and urolithins), which could modulate the microbiome quality, intestinal homeostasis, and host immune responses and could be effective against Campylobacter (67–74). Interestingly, several Olsenella spp. (n = 5) were frequently detected in the stool samples and were negatively correlated with Campylobacter prevalence. Olsenella was previously identified as a part of the microbiome associated with the infant health status (stunting, autism spectrum disorder, and chronic malnutrition) in China and Bangladesh (75–77). Therefore, the anti-Campylobacter properties of several species identified in this study and their potential application as dietary supplement to control Campylobacter in developing countries needs further investigation.
Interestingly, M. diversum was the only bacteria of the stool microbiome with a distinct positive effect on the child gut permeability (r2 = −0.2), gut inflammation (r2 = −0.26), EED severity (r2 = −0.27), and diarrhea prevalence (r2 = −0.24), but no association with Campylobacter. No information is available concerning this bacterium. Therefore, further investigations are required to support the beneficial properties of M. diversum against EED and their potential application as dietary supplement in developing countries to improve intestinal homeostasis. On the other hand, the abundance of four bacterial species (butyrate-producing bacterium SS3/4, Megamonas hypermegale, Streptococcus australis, and Gordonibacter massiliensis) were positively correlated with the abundance of Campylobacter in the stools and with the severity of the gut inflammation, gut permeability and/or EED severity; Therefore, these bacteria might have an important impact on the gut microbiome quality, the persistence of Campylobacter in the gut, and the children's health.
In conclusion, we report in this study high prevalence and diversity of Campylobacter spp. in children in eastern Ethiopia, including the non-thermophilic Campylobacter spp. (i.e., C. hyointestinalis and C. fetus-like species). The MeTRS revealed co-occurrence of several Campylobacter spp. More studies are needed to better understand the prevalence, sources, and transmission dynamics of Campylobacter to children as well as to establish a clear link between Campylobacter infection (including non-thermotolerant species) in children with EED and stunting. Furthermore, even though no direct correlation was identified between Campylobacter prevalence and abundance, and the EED severity or the diarrhea, the metagenomic analysis highlighted the association between stool microbiome and the prevalence and abundance of Campylobacter spp., EED severity, and diarrhea in children. The unique changes in microbiome may serve as biomarkers for disease status and can help to elucidate the complex interactions between Campylobacter, the gut microbiome, EED and stunting in the context of the socio-economic environment of children in rural Ethiopia.
Data Availability Statement
The datasets generated for this study can be found in the BioProject ID: PRJNA608948 https://www.ncbi.nlm.nih.gov/sra/?term=SRR11194563.
Ethics Statement
All the study procedures were performed in accordance with the Declaration of Helsinki (78) and approvals from the HU Institutional Health Ethics Research Review Committee (Ref. No. IHRERC/152/2018), the Ethiopia National Research Ethics Review Committee (Ref. No. MoST/3-10/168/2018), the Institutional Review Board at the University of Florida (UF) (Ref. No. 201703252) and Washington University School of Medicine (Protocol No. 201806021). Additionally, the operational district officers and local village chiefs were informed about the objectives of the study and the future impacts and their consent was obtained. Written and oral consents were also obtained from child's mother/caregiver and father. Material and Data Transfer Agreements (MDTA) were signed between Haramaya University and all US-based partners. Export permits to ship biological specimens from Ethiopia to the U.S.A were approved by the Ministry of Science and Higher Education (Ref. No. SHE/SSM/19.1/008/11/19). Written informed consent to participate in this study was provided by the participants' legal guardian/next of kin.
Author Contributions
GR, SM, JY, WG, MM, AH, and LD conceived and designed the experiment. YT, AM, and GY collected samples. YT, LD, MG, YH, BM, VA, MO, and KK processed the samples for PCR and MeTRS analyses. YT, LD, AH, YH, GR, NS, MG, VA, KK, and DC analyzed the PCR and MeTRS data. YT, LD, MG, AH, and GR wrote the manuscript.
Funding
The CAGED project was funded by the Bill & Melinda Gates Foundation to address food insecurity issues in Ethiopia and Burkina Faso through the project Equip - Strengthening Smallholder Livestock Systems for the Future (grant number OPP11755487). These funds are administered by the Feed the Future Innovation Lab for Livestock Systems, which was established by funding from the United States Agency for International Development (USAID) and is co-led by the University of Florida's Institute of Food and Agricultural Sciences and the International Livestock Research Institute. Support for the Feed the Future Innovation Lab for Livestock Systems is made possible by the generous support of the American people through USAID. The contents are the responsibility of the authors and do not necessarily reflect the views of USAID or the United States Government. REDCap is hosted at the University of Florida Clinical and Translational Science Institute (CTSI), supported by NIH National Center for Advancing Translational Sciences grant UL1 TR000064. The CAGED is a collaborative project between University of Florida, The Ohio State University, Washington University, and Haramaya University (Ethiopia). The CAGED project was supported by a Technical Advisory Group consisting of Eric Fèvre (University of Liverpool and International Livestock Research Institute), Nigel French (Massey University), Aulo Gelli (International Food Policy Research Institute), Andrew Jones (University of Michigan), Vivek Kapur (Penn State University), Nick Juleff and Supriya Kumar (Bill & Melinda Gates Foundation) and James Platts-Mills (University of Virginia).
Conflict of Interest
The authors declare that the research was conducted in the absence of any commercial or financial relationships that could be construed as a potential conflict of interest.
Acknowledgments
We thank Chan Zuckerberg Biohub, San Francisco, CA, USA and Chan Zuckerberg Initiative, Redwood City, CA, USA for their support with sequencing of total RNA and the bioinformatics analysis of the MeTRS data. We thank Drs. Volker Mai and Nigel French for their critical reading of the manuscript. We also thank Ame Yousuf, Beyan Abdullahi, Yeharerwork Abebaw, Yenenesh Elias Ahmed, Ibsa Ahmed, Jafer Amin, Seyum Tezera, Dr. Nigussie Bussa, and Ibsa Usmane at Haramaya University, and Jenna Daniels and Anna Rabil at University of Florida for their contributions to the project. We thank Srevi Devaraj (Clinical Chemistry and POCT, Texas Children's Hospital, Baylor College of Medicine, Houston, TX) for analysis of sugars in the dual sugar absorption test. The study would not have been possible without cooperation of study communities and local administration of the study kebeles. We would like to express our appreciation for the study households and all who supported the study directly or otherwise.
Supplementary Material
The Supplementary Material for this article can be found online at: https://www.frontiersin.org/articles/10.3389/fpubh.2020.00099/full#supplementary-material
Pipeline used for the processing of the MeTRS data.
Figure S1. Estimation of environmental enteric dysfunction severity based on gut permeability and gut inflammation data. The gut permeability was assessed using the percentage of lactulose in the urine. The gut inflammation was assessed based on the concentration in MPO (ng/ml) in the urine. Both parameters were used to estimate the environmental enteric dysfunction (EED) severity for each infant. The gut inflammation was considered normal if [MPO] was lower than 2,000 ng/ml, moderated if [MPO] was between 2,000 and 11,000 ng/ml, and severe if [MPO] was higher than 11,000 ng/ml. The gut permeability was considered normal if the lactulose value was lower than 0.2%, moderated if the lactulose value was between 0.2 and 0.45%, and severe if the lactulose value was higher than 0.45%; MPO, myeloperoxidase.
Figure S2. Campylobacter spp. prevalence in the children stools (n = 100) collected from five kebeles using conventional PCR (A) and MeTRS (B) data. Orange and blue bars represent the percentage of the stools positive or negative, respectively for Campylobacter for a given kebele. C) Comparison of the Campylobacter prevalence using conventional PCR (c-PCR) and meta-total RNA sequencing (MeTRS). In red and blue are the stools positive or negative, respectively for Campylobacter. The heat map is composed of 100 columns (one column per child). Genus-specific PCR was performed using 16S RNA primers and species-specific PCR for C. coli and C. jejuni were performed using ceuE and mapA primers, respectively (Table S1).
Figure S3. Co-occurrence of Campylobacter spp. in the children stool samples. Co-occurrence Heatmap profile was created using the multivariate analysis data (r2) based on the prevalence of Campylobacter spp. in the stool samples (see Figure 2).
Figure S4. Campylobacter spp. profile between children stool samples based on the meta-total RNA sequencing (MeTRS). (A) Principal component analysis of the Campylobacter spp. diversity and abundance in the stools. Each colored dot represents one child stool sample (n = 100). Black dot represents the average profile for a given kebele (n = 5). (B) Discriminant analysis of the Campylobacter spp. diversity and abundance in the stools based on kebeles. The outside ellipse contains ~50% of the observations. The inside ellipse represents 95% confidence level (Cut-off for MeTRS data; contigs number ≥ 10; Read length ≥ 50; Z-score ≥ 1. (C). Clusterization profile of the stools based on the Campylobacter spp. diversity, prevalence, and abundance data.
Figure S5. Differences in abundance of Campylobacter hyointestinalis and Campylobacter RM12175 between kebeles. Letters (A,B) indicates different statistical groups (P < 0.01). Star: read per million (rpm) are significantly higher in the designated kebele compared to the other kebeles.
Figure S6. Campylobacter spp. profiles between children stool samples based EED severity and diarrhea. Discriminant analysis of the Campylobacter spp. abundance in the children stools based on (A) the gut permeability (lactulose%), (C) gut inflammation ([myeloperoxidase] in ng/ml), (E) EED severity, and (G) diarrhea prevalence data. The outside ellipse contains approximately 50% of the observations. The inside ellipse represents 95% confidence level. Clusterization profile of the Campylobacter spp. abundance in the stools based on (B) the gut permeability (lactulose%), (D) gut inflammation ([myeloperoxidase] in ng/ml), (F) EED severity, and (H) diarrhea prevalence data. Additional details concerning the EED severity determination are presented in Table S1.
Figure S7. Microbiome profiles between children stool samples based on kebeles. (A) Principal component analysis of the children stool samples based on kebeles. Each colored dot represents one stool sample (n = 100). Kebeles with the same color code belong to the same cluster and therefore harbored equivalent microbiota diversity and abundance. A T2 test revealed a total of 11 outliers (stools with significantly different microbiota profile compared to the rest of the population; outside the red oval). (B) Discriminant analysis of the stool microbiome based on kebeles. The outside ellipse contains ~50% of the observations. The inside ellipse represents 95% confidence level. (C) Clusterization profile of the stool microbiome based on kebeles.
Figure S8. Microbiome profile based on Campylobacter prevalence. (A) Discriminant analysis of the children stool microbiome based on Campylobacter prevalence. The outside ellipse contains ~50% of the observations. The inside ellipse represents 95% confidence level. (B) Clusterization profile of the stool's microbiome based on Campylobacter prevalence.
Figure S9. Microbiome profile based on environmental enteric dysfunction (EED). Discriminant analysis of the children stool microbiome composition based on (A) the gut permeability (lactulose%), (C) gut inflammation ([MPO] in ng/ml), (E) EED severity, and (G) diarrhea prevalence data. The outside ellipse contains ~50% of the observations. The inside ellipse represents 95% confidence level. Clusterization profile of the Campylobacter spp. abundance in the stools based on (B) the gut permeability (lactulose%), (D) gut inflammation ([MPO] in ng/ml), (F) EED severity, and (H) diarrhea prevalence data. Additional details concerning the EED severity determination are presented in Table S1. (I) Correlation between the specific bacterial species of the children stool microbiome and the gut permeability (lactulose%), gut inflammation ([MPO] in ng/ml), EED severity, and diarrhea prevalence data. N, number of stools positive for the designated bacterium. Values in the table represent the mean (log [read per million]) ± standard error for the designated bacterial species for a given status. Stars represent bacterial species harboring at least 2-fold difference in abundance between the “normal” and “severe” status for at least one of the parameters studied. MPO: myeloperoxidase.
Table S1. Primers used in the study. Source: Adapted from Deals et al. (28).
References
1. Havelaar AH, Kirk MD, Torgerson PR, Gibb HJ, Hald T, Lake RJ, et al. World Health Organization global estimates and regional comparisons of the burden of foodborne disease in 2010. PLoS Med. (2015) 12:e1001923. doi: 10.1371/journal.pmed.1001923
2. Janssen R, Krogfelt KA, Cawthraw SA, van Pelt W, Wagenaar JA, Owen RJ. Host-pathogen interactions in Campylobacter infections: the host perspective. Clin Microbiol Rev. (2008) 21:505–18. doi: 10.1128/CMR.00055-07
3. GBD 2015 Mortality and Causes of Death Collaborators. Global, regional, and national life expectancy, all-cause mortality, and cause-specific mortality for 249 causes of death, 1980-2015: a systematic analysis for the Global Burden of Disease Study 2015. Lancet. (2016) 388:1459–544. doi: 10.1016/S0140-6736(16)31012-1
4. Coker AO, Isokpehi RD, Thomas BN, Amisu KO, Obi CL. Human campylobacteriosis in developing countries. Emerg Infect Dis. (2002) 8:237–44. doi: 10.3201/eid0803.010233
5. Lee G, Pan W, Peñataro Yori P, Paredes Olortegui M, Tilley D, Gregory M, et al. Symptomatic and asymptomatic Campylobacter infections associated with reduced growth in Peruvian children. PLoS Negl Trop Dis. (2013) 7:e2036. doi: 10.1371/journal.pntd.0002036
6. Ngure F, Gelli A, Becquey E, Ganaba R, Headey D, Huybregts L, et al. Exposure to livestock feces and water quality, sanitation, and hygiene (WASH) conditions among caregivers and young children: formative research in Rural Burkina Faso. Am J Trop Med Hyg. (2019) 100:998–1004. doi: 10.4269/ajtmh.18-0333
7. Amour C, Gratz J, Mduma E, Svensen E, Rogawski ET, McGrath M, et al. Epidemiology and impact of Campylobacter infection in children in 8 low-resource settings: results from the MAL-ED study. Clin Infect Dis. (2016) 63:1171–9. doi: 10.1093/cid/ciw542
8. Rogawski ET, Liu J, Platts-Mills JA, Kabir F, Lertsethtakarn P, Siguas M, et al. Use of quantitative molecular diagnostic methods to investigate the effect of enteropathogen infections on linear growth in children in low-resource settings: longitudinal analysis of results from the MAL-ED cohort study. Lancet Glob Health. (2018) 6:e1319-28. doi: 10.1016/S2214-109X(18)30351-6
9. World Health Organization. WHO | Joint Child Malnutrition Estimates - Levels and Trends, 2019 ed. WHO (2018). Available at: http://www.who.int/nutgrowthdb/estimates2018/en/ (accessed October 16, 2019).
10. Keusch GT, Denno DM, Black RE, Duggan C, Guerrant RL, Lavery JV, et al. Environmental enteric dysfunction: pathogenesis, diagnosis, and clinical consequences. Clin Infect Dis. (2014) 59(Suppl. 4):S207–12. doi: 10.1093/cid/ciu485
11. Guerrant RL, DeBoer MD, Moore SR, Scharf RJ, Lima AAM. The impoverished gut—a triple burden of diarrhoea, stunting and chronic disease. Nat Rev Gastroenterol Hepatol. (2013) 10:220–9. doi: 10.1038/nrgastro.2012.239
12. Richard SA, McCormick BJJ, Murray-Kolb LE, Lee GO, Seidman JC, Mahfuz M, et al. Enteric dysfunction and other factors associated with attained size at 5 years: MAL-ED birth cohort study findings. Am J Clin Nutr. (2019) 110:131–8. doi: 10.1093/ajcn/nqz004
13. Platts-Mills JA, Babji S, Bodhidatta L, Gratz J, Haque R, Havt A, et al. Pathogen-specific burdens of community diarrhoea in developing countries: a multisite birth cohort study (MAL-ED). Lancet Glob Health. (2015) 3:e564–75. doi: 10.1016/S2214-109X(15)00151-5
14. Headey D, Hirvonen K. Is exposure to poultry harmful to child nutrition? An observational analysis for rural Ethiopia. PloS ONE. (2016) 11:e0160590. doi: 10.1371/journal.pone.0160590
15. Lengerh A, Moges F, Unakal C, Anagaw B. Prevalence, associated risk factors and antimicrobial susceptibility pattern of Campylobacter species among under five diarrheic children at Gondar University Hospital, Northwest Ethiopia. BMC Pediatr. (2013) 13:82. doi: 10.1186/1471-2431-13-82
16. Mulatu G, Beyene G, Zeynudin A. Prevalence of Shigella, Salmonella and Campylobacter species and their susceptibility patters among under five children with diarrhea in Hawassa town, south Ethiopia. Ethiop J Health Sci. (2014) 24:101–8. doi: 10.4314/ejhs.v24i2.1
17. Abamecha A, Assebe G, Tafa B, Beyene W. Prevalence of thermophilic campylobacter and their antimicrobial resistance profile in food animals in Lare District, Nuer Zone, Gambella, Ethiopia. J Drug Res Dev. (2015) 1:2470–1009. doi: 10.16966/2470-1009.108
18. Thomas KM, de Glanville WA, Barker GC, Benschop J, Buza JJ, Cleaveland S, et al. Prevalence of Campylobacter and Salmonella in African food animals and meat: A systematic review and meta-analysis. Int J Food Microbiol. (2019) 315:108382. doi: 10.1016/j.ijfoodmicro.2019.108382
19. Forsythe SJ. The Microbiology of Safe Food. Nottingham, UK: John Wiley and Sons; Nottingham Trent University (2011).
20. Wilkinson DA, O'Donnell AJ, Akhter RN, Fayaz A, Mack HJ, Rogers LE, et al. Updating the genomic taxonomy and epidemiology of Campylobacter hyointestinalis. Sci Rep. (2018) 8:1–12. doi: 10.1038/s41598-018-20889-x
21. Owino V, Ahmed T, Freemark M, Kelly P, Loy A, Manary M, et al. Environmental enteric dysfunction and growth failure/stunting in Global child health. Pediatrics. (2016) 138:1–10. doi: 10.1542/peds.2016-0641
22. Bado AR, Susuman AS, Nebie EI. Trends and risk factors for childhood diarrhea in sub-Saharan countries (1990-2013): assessing the neighborhood inequalities. Glob Health Action. (2016) 9:30166. doi: 10.3402/gha.v9.30166
23. Central Statistical Agency (CSA). Demographic and Health Survey, Ethiopia, 2016. (2016). Available online at: https://microdata.worldbank.org/index.php/catalog/2886 (accessed September 22, 2019).
24. Faubion WA, Camilleri M, Murray JA, Kelly P, Amadi B, Kosek MN, et al. Improving the detection of environmental enteric dysfunction: a lactulose, rhamnose assay of intestinal permeability in children aged under 5 years exposed to poor sanitation and hygiene. BMJ Glob Health. (2016) 1:66. doi: 10.1136/bmjgh-2016-000066
25. Ordiz MI, Davitt C, Stephenson K, Agapova S, Divala O, Shaikh N, et al. EB 2017 article: interpretation of the lactulose:mannitol test in rural Malawian children at risk for perturbations in intestinal permeability. Exp Biol Med. (2018) 243:677–83. doi: 10.1177/1535370218768508
26. Deblais L, Helmy YA, Kathayat D, Huang H, Miller SA, Rajashekara G. Novel imidazole and methoxybenzylamine growth inhibitors affecting Salmonella cell envelope integrity and its persistence in chickens. Sci Rep. (2018) 8:1–17. doi: 10.1038/s41598-018-31249-0
27. Kumar A, Vlasova AN, Deblais L, Huang H-C, Wijeratne A, Kandasamy S, et al. Impact of nutrition and rotavirus infection on the infant gut microbiota in a humanized pig model. BMC Gastroenterol. (2018) 18:93. doi: 10.1186/s12876-018-0810-2
28. Denis M, Soumet C, Rivoal K, Ermel G, Blivet D, Salvat G, et al. Development of a m-PCR assay for simultaneous identification of Campylobacter jejuni and C. coli. Lett Appl Microbiol. (1999) 29:406–10. doi: 10.1046/j.1472-765X.1999.00658.x
29. Cottier F, Srinivasan KG, Yurieva M, Liao W, Poidinger M, Zolezzi F, et al. Advantages of meta-total RNA sequencing (MeTRS) over shotgun metagenomics and amplicon-based sequencing in the profiling of complex microbial communities. NPJ Biofilms Microbiomes. (2018) 4:1–7. doi: 10.1038/s41522-017-0046-x
30. Saha S, Ramesh A, Kalantar K, Malaker R, Hasanuzzaman M, Khan LM, et al. Unbiased metagenomic sequencing for pediatric meningitis in Bangladesh reveals neuroinvasive Chikungunya virus outbreak and other unrealized pathogens. bioRxiv. (2019) 10:579532. doi: 10.1128/mBio.02877-19
31. Schnee AE, Petri WA. Campylobacter jejuni and associated immune mechanisms: short-term effects and long-term implications for infants in low-income countries. Curr Opin Infect Dis. (2017) 30:322–8. doi: 10.1097/QCO.0000000000000364
32. Rodgers JD, Simpkin E, Lee R, Clifton-Hadley FA, Vidal AB. Sensitivity of direct culture, enrichment and PCR for Detection of Campylobacter jejuni and C. coli in Broiler Flocks at Slaughter. Zoonoses Public Health. (2017) 64:262–71. doi: 10.1111/zph.12306
33. Platts-Mills JA, Liu J, Gratz J, Mduma E, Amour C, Swai N, et al. Detection of Campylobacter in stool and determination of significance by culture, enzyme immunoassay, and PCR in developing countries. J Clin Microbiol. (2014) 52:1074–80. doi: 10.1128/JCM.02935-13
34. Ricke SC, Feye KM, Chaney WE, Shi Z, Pavlidis H, Yang Y. Developments in rapid detection methods for the detection of foodborne Campylobacter in the United States. Front Microbiol. (2018) 9:3280. doi: 10.3389/fmicb.2018.03280
35. Lewandowska DW, Zagordi O, Geissberger F-D, Kufner V, Schmutz S, Böni J, et al. Optimization and validation of sample preparation for metagenomic sequencing of viruses in clinical samples. Microbiome. (2017) 5:94. doi: 10.1186/s40168-017-0317-z
36. Plaire D, Puaud S, Marsolier-Kergoat M-C, Elalouf J-M. Comparative analysis of the sensitivity of metagenomic sequencing and PCR to detect a biowarfare simulant (Bacillus atrophaeus) in soil samples. PloS ONE. (2017) 12:e0177112. doi: 10.1371/journal.pone.0177112
37. Gigliucci F, von Meijenfeldt FAB, Knijn A, Michelacci V, Scavia G, Minelli F, et al. Metagenomic characterization of the human intestinal microbiota in fecal samples from STEC-infected patients. Front Cell Infect Microbiol. (2018) 8:25. doi: 10.3389/fcimb.2018.00025
38. Doster E, Rovira P, Noyes NR, Burgess BA, Yang X, Weinroth MD, et al. A cautionary report for pathogen identification using shotgun metagenomics; a comparison to aerobic culture and polymerase chain reaction for Salmonella enterica identification. Front Microbiol. (2019) 10:2499. doi: 10.3389/fmicb.2019.02499
39. Kaakoush NO, Castaño-Rodríguez N, Mitchell HM, Man SM. Global epidemiology of Campylobacter infection. Clin Microbiol Rev. (2015) 28:687–720. doi: 10.1128/CMR.00006-15
40. Costa D, Iraola G. Pathogenomics of emerging Campylobacter species. Clin Microbiol Rev. (2019) 32:1–24. doi: 10.1128/CMR.00072-18
41. Spickler AR, Leedom Larson K. Campylobacteriosis (2013). Retrieved from: http://www.cfsph.iastate.edu/DiseaseInfo/factsheets.php
42. Liu F, Ma R, Wang Y, Zhang L. The Clinical Importance of Campylobacter concisus and Other Human Hosted Campylobacter Species. Front Cell Infect Microbiol. (2018) 8:243. doi: 10.3389/fcimb.2018.00243
43. Oporto B, Hurtado A. Emerging thermotolerant Campylobacter species in healthy ruminants and swine. Foodborne Pathog Dis. (2011) 8:807–13. doi: 10.1089/fpd.2010.0803
44. Inglis GD, Kalischuk LD, Busz HW. Chronic shedding of Campylobacter species in beef cattle. J Appl Microbiol. (2004) 97:410–20. doi: 10.1111/j.1365-2672.2004.02313.x
45. Hakkinen M, Heiska H, Hänninen M-L. Prevalence of Campylobacter spp. in cattle in Finland and antimicrobial susceptibilities of bovine Campylobacter jejuni strains. Appl Environ Microbiol. (2007) 73:3232–8. doi: 10.1128/AEM.02579-06
46. Salihu MD, Abdulkadir JU, Oboegbulem SI, Egwu GO, Magaji AA, Lawal M, et al. Isolation and prevalence of Campylobacter species in cattle from Sokoto state, Nigeria. Vet Ital. (2009) 45:501–5.
47. Serraino A, Florio D, Giacometti F, Piva S, Mion D, Zanoni RG. Presence of Campylobacter and Arcobacter species in in-line milk filters of farms authorized to produce and sell raw milk and of a water buffalo dairy farm in Italy. J Dairy Sci. (2013) 96:2801–7. doi: 10.3168/jds.2012-6249
48. Guévremont E, Lamoureux L, Loubier CB, Villeneuve S, Dubuc J. Detection and characterization of Campylobacter spp. from 40 dairy cattle herds in Quebec, Canada. Foodborne Pathog Dis. (2014) 11:388–94. doi: 10.1089/fpd.2013.1706
49. Gorkiewicz G, Feierl G, Zechner R, Zechner EL. Transmission of Campylobacter hyointestinalis from a pig to a human. J Clin Microbiol. (2002) 40:2601–5. doi: 10.1128/JCM.40.7.2601-2605.2002
50. Miller WG, Chapman MH, Yee E, On SLW, McNulty DK, Lastovica AJ, et al. Multilocus sequence typing methods for the emerging Campylobacter species, C. hyointestinalis, C lanienae, C sputorum, C concisus, and C curvus, Front Cell Infect Microbiol. (2012) 2:45. doi: 10.3389/fcimb.2012.00045
51. Patrick ME, Gilbert MJ, Blaser MJ, Tauxe RV, Wagenaar JA, Fitzgerald C. Human infections with new subspecies of Campylobacter fetus. Emerg Infect Dis. (2013) 19:1678–80. doi: 10.3201/eid1910.130883
52. Kim DK, Hong SK, Kim M, Ahn JY, Yong D, Lee K. Campylobacter hyointestinalis isolated from a human stool specimen. Ann Lab Med. (2015) 35:657–9. doi: 10.3343/alm.2015.35.6.657
53. Samosornsuk W, Asakura M, Yoshida E, Taguchi T, Eampokalap B, Chaicumpa W, et al. Isolation and characterization of Campylobacter strains from diarrheal patients in central and Suburban Bangkok, Thailand. Jpn J Infect Dis. (2015) 68:209–15. doi: 10.7883/yoken.JJID.2014.229
54. Rapp D, Ross CM. Prevalence of six Campylobacter species in a New Zealand dairy goat herd. N Z J Agric Res. (2012) 55:235–40. doi: 10.1080/00288233.2012.672427
55. Chaban B, Ngeleka M, Hill JE. Detection and quantification of 14 Campylobacter species in pet dogs reveals an increase in species richness in feces of diarrheic animals. BMC Microbiol. (2010) 10:73. doi: 10.1186/1471-2180-10-73
56. Sasaki Y, Goshima T, Mori T, Murakami M, Haruna M, Ito K, et al. Prevalence and antimicrobial susceptibility of foodborne bacteria in wild boars (Sus scrofa) and wild deer (Cervus nippon) in Japan. Foodborne Pathog Dis. (2013) 10:985–91. doi: 10.1089/fpd.2013.1548
57. Ley RE. Gut microbiota in 2015: Prevotella in the gut: choose carefully. Nat Rev Gastroenterol Hepatol. (2016) 13:69–70. doi: 10.1038/nrgastro.2016.4
58. Larsen JM. The immune response to Prevotella bacteria in chronic inflammatory disease. Immunology. (2017) 151:363–74. doi: 10.1111/imm.12760
59. Pybus V, Onderdonk AB. Evidence for a commensal, symbiotic relationship between Gardnerella vaginalis and Prevotella bivia Involving ammonia: potential significance for bacterial vaginosis. J Infect Dis. (1997) 175:406–13. doi: 10.1093/infdis/175.2.406
60. Pybus V, Onderdonk AB. A commensal symbiosis between Prevotella bivia and Peptostreptococcus anaerobius involves amino acids: potential significance to the pathogenesis of bacterial vaginosis. FEMS Immunol Med Microbiol. (1998) 22:317–27. doi: 10.1111/j.1574-695X.1998.tb01221.x
61. Margolis E, Fredricks DN. Chapter 83 - bacterial vaginosis-associated bacteria. In: Tang YW, Sussman M, Liu D, Poxton I, Schwartzman J, editors. Molecular Medical Microbiology, 2nd ed. Boston, MA: Academic Press (2015). p. 1487–96.
62. Pedersen HK, Gudmundsdottir V, Nielsen HB, Hyotylainen T, Nielsen T, Jensen BAH, et al. Human gut microbes impact host serum metabolome and insulin sensitivity. Nature. (2016) 535:376–81. doi: 10.1038/nature18646
63. Giri S, Mangalam A. Chapter 34 - the gut microbiome and metabolome in multiple sclerosis. In: Faintuch J, Faintuch S, editors. Academic Press (2019). p. 333–40.
64. Vazquez-Gutierrez P, de Wouters T, Werder J, Chassard C, Lacroix C. High Iron-sequestrating bifidobacteria inhibit enteropathogen growth and adhesion to intestinal epithelial cells in vitro. Front Microbiol. (2016) 7:1480. doi: 10.3389/fmicb.2016.01480
65. Sakurai T, Odamaki T, Xiao J-Z. Production of indole-3-lactic acid by bifidobacterium strains isolated fromhuman infants. Microorganisms. (2019) 7:340. doi: 10.3390/microorganisms7090340
66. Mundi A, Delcenserie V, Amiri-Jami M, Moorhead S, Griffiths MW. Cell-free preparations of Lactobacillus acidophilus strain La-5 and Bifidobacterium longum strain NCC2705 affect virulence gene expression in Campylobacter jejuni. J Food Prot. (2013) 76:1740–6. doi: 10.4315/0362-028X.JFP-13-084
67. Hashizume K, Tsukahara T, Yamada K, Koyama H, Ushida K. Megasphaera elsdenii JCM1772T normalizes hyperlactate production in the large intestine of fructooligosaccharide-fed rats by stimulating butyrate production. J Nutr. (2003) 133:3187–90. doi: 10.1093/jn/133.10.3187
68. Wexler HM. Bacteroides: the good, the bad, and the nitty-gritty. Clin Microbiol Rev. (2007) 20:593–621. doi: 10.1128/CMR.00008-07
69. Spanogiannopoulos P, Bess EN, Carmody RN, Turnbaugh PJ. The microbial pharmacists within us: a metagenomic view of xenobiotic metabolism. Nat Rev Microbiol. (2016) 14:273–87. doi: 10.1038/nrmicro.2016.17
70. Ščerbová J, Lauková A. Sensitivity to enterocins of thermophilic Campylobacter spp. from different poultry species. Foodborne Pathog Dis. (2016) 13:668–73. doi: 10.1089/fpd.2016.2158
71. Helmy YA, Kassem II, Kumar A, Rajashekara G. In vitro evaluation of the impact of the probiotic E. coli nissle 1917 on Campylobacter jejuni's invasion and intracellular survival in human colonic cells. Front. Microbiol. (2017) 8:1588. doi: 10.3389/fmicb.2017.01588
72. Johnson TJ, Shank JM, Johnson JG. Current and potential treatments for reducing Campylobacter colonization in animal hosts and disease in HUMANS. Front Microbiol. (2017) 8:487. doi: 10.3389/fmicb.2017.00487
73. Maki JJ, Looft T. Complete genome sequence of megasphaera stantonii ajh120t, isolated from a chicken cecum. Microbiol Resour Announc. (2018) 7:e01148-18. doi: 10.1128/MRA.01148-18
74. Ueda A, Kobayashi A, Tsuchida S, Yamada T, Murata K, Nakamura H, et al. Cecal microbiome analyses on wild Japanese Rock Ptarmigans (Lagopus muta japonica) reveals high level of coexistence of lactic acid bacteria and lactate-utilizing bacteria. Microorganisms. (2018) 6:77. doi: 10.3390/microorganisms6030077
75. Gough EK, Stephens DA, Moodie EEM, Prendergast AJ, Stoltzfus RJ, Humphrey JH, et al. Linear growth faltering in infants is associated with Acidaminococcus sp. and community-level changes in the gut microbiota. Microbiome. (2015) 3:24. doi: 10.1186/s40168-015-0089-2
76. Dinh DM, Ramadass B, Kattula D, Sarkar R, Braunstein P, Tai A, et al. Longitudinal analysis of the intestinal microbiota in persistently stunted young children in South India. PloS ONE. (2016) 11:e0155405. doi: 10.1371/journal.pone.0155405
77. Liu S, Li E, Sun Z, Fu D, Duan G, Jiang M, et al. Altered gut microbiota and short chain fatty acids in Chinese children with autism spectrum disorder. Sci Rep. (2019) 9:1–9. doi: 10.1038/s41598-018-36430-z
Keywords: Campylobacter, non-thermotolerant Campylobacter, EED, diarrhea, malnutrition, stunting, livestock reservoirs, MeTRS
Citation: Terefe Y, Deblais L, Ghanem M, Helmy YA, Mummed B, Chen D, Singh N, Ahyong V, Kalantar K, Yimer G, Yousuf Hassen J, Mohammed A, McKune SL, Manary MJ, Ordiz MI, Gebreyes W, Havelaar AH and Rajashekara G (2020) Co-occurrence of Campylobacter Species in Children From Eastern Ethiopia, and Their Association With Environmental Enteric Dysfunction, Diarrhea, and Host Microbiome. Front. Public Health 8:99. doi: 10.3389/fpubh.2020.00099
Received: 05 December 2019; Accepted: 12 March 2020;
Published: 15 April 2020.
Edited by:
Nicolae Corcionivoschi, Agri-Food and Biosciences Institute (AFBI), United KingdomReviewed by:
Brendan Wren, University of London, United KingdomChristine M. Szymanski, University of Alberta, Canada
Copyright © 2020 Terefe, Deblais, Ghanem, Helmy, Mummed, Chen, Singh, Ahyong, Kalantar, Yimer, Yousuf Hassen, Mohammed, McKune, Manary, Ordiz, Gebreyes, Havelaar and Rajashekara. This is an open-access article distributed under the terms of the Creative Commons Attribution License (CC BY). The use, distribution or reproduction in other forums is permitted, provided the original author(s) and the copyright owner(s) are credited and that the original publication in this journal is cited, in accordance with accepted academic practice. No use, distribution or reproduction is permitted which does not comply with these terms.
*Correspondence: Gireesh Rajashekara, rajashekara.2@osu.edu
†These authors have contributed equally to this work
‡Present address: Mostafa Ghanem, Department of Veterinary Medicine, Virginia-Maryland College of Veterinary Medicine, University of Maryland, College Park, MD, United States