- 1Joint Institute of Tobacco and Health, Yunnan Academy of Tobacco Science, Kunming, China
- 2Hangzhou Global Biotechnology and Bioinformatics Co. Ltd, Hangzhou, China
Tobacco use is one of the leading causes of preventable disease worldwide. Genetic studies have elucidated numerous smoking-associated risk loci in American and European populations. However, genetic determinants for cigarette smoking in Chinese populations are under investigated. In this study, a whole-genome sequencing (WGS)-based genome-wide association study (GWAS) was performed in a Chinese Han population comprising 620 smokers and 564 nonsmokers. Thirteen single-nucleotide polymorphisms (SNPs) of the raftlin lipid linker 1 (RFTN1) gene achieved genome-wide significance levels (P < 5 x 10−8) for smoking initiation. The rs139753473 from RFTN1 and six other suggestively significant loci from CUB and sushi multiple domains 1 (CSMD1) gene were also associated with cigarettes per day (CPD) in an independent Chinese sample consisting of 1,329 subjects (805 smokers and 524 nonsmokers). When treating males separately, associations between smoking initiation and PCAT5/ANKRD30A, two genes involved in cancer development, were identified and replicated. Within RFTN1, two haplotypes (i.e., C-A-C-G and A-G-T-C) formed by rs796812630-rs796584733-rs796349027-rs879511366 and three haplotypes (i.e., T-T-C-C-C, T-T-A-T-T, and C-A-A-T-T) formed by rs879401109-rs879453873-rs75180423-rs541378415-rs796757175 were strongly associated with smoking initiation. In addition, we also revealed two haplotypes (i.e., C-A-G-G and T-C-T-T derived from rs4875371-rs4875372-rs17070935-rs11991366) in the CSMD1 gene showing a significant association with smoking initiation. Further bioinformatics functional assessment suggested that RFTN1 may participate in smoking behavior through modulating immune responses or interactions with the glucocorticoid receptor alpha and the androgen receptor. Together, our results may help understand the mechanisms underlying smoking behavior in the Chinese Han population.
Introduction
Although many programs and regulatory policies of tobacco control have been introduced, reducing the smoking prevalence to a satisfactory level remains an unsolved issue in many countries, especially low- and middle-income countries (1). It has been reported that over 1.3 billion people were tobacco users in 2018 (2). Cigarette smoking is believed to have a wide range of deleterious health effects, such as cardiovascular and pulmonary diseases, and cancers (3–6). Tobacco smoking and second-hand smoke exposure contribute to more than 6 million deaths worldwide annually, posing a serious threat to public health (7).
The establishment of daily smoking usually consists of three main stages: smoking initiation, transition from experimentation to regular smoking, and development of nicotine dependence (ND) (8, 9). Both genetic and environmental factors have been shown to influence all smoking-related stages (9). Smoking initiation, smoking quantity, smoking cessation, and nicotine dependence are commonly studied phenotypes in researches of smoking-related genetic predispositions. Of these, the most well-known is the association between ND and genetics. As primary targets in the brain for nicotine to exert its biological effects, genes encoding nicotinic acetylcholine receptors (nAChRs) represent one of the most investigated ND susceptibility gene families. It is known that nAChRs can trigger the release of dopamine and glutamate, and furthermore reinforce nicotine reward and addiction (10). Meta-analyses and genome-wide association studies (GWASs) have identified a variety of polymorphisms within nAChRs, e.g., rs3743075 in CHRNA3 (11, 12) and rs2273500 in CHRNA4 (13) associated with ND. In addition to nAChRs, nicotine metabolizing enzymes (e.g., CYP2A6), dopamine receptors and transporters (e.g., DRD2, DRD4, and SLC6A3), and neuregulin signaling pathway proteins (e.g., NRG3) are also considered to have high impacts on nicotine addiction (14–17).
China is the world’s largest producer of tobacco products, and smoking prevalence in Chinese males is among the highest in the world (18, 19). Nevertheless, GWA studies of smoking behaviors in the Han Chinese are much less reported compared with those conducted in the populations of European descent (20) and of European American or of African American populations (21, 22). In the present study, 1,184 Chinese Han adults (including 620 smokers and 564 nonsmokers) were recruited. Whole-genome sequencing (WGS) was performed to identify genome-wide variants. Genetic variants associated with smoking initiation and cigarettes per day (CPD) were determined by association tests. To verify our GWAS findings, a replication analysis was conducted in 1,329 subjects including 805 smokers and 524 nonsmokers. The possible mechanisms of how the observed variants involved in smoking behavior were also briefly discussed.
Materials and Methods
Subjects and Phenotypes
The discovery sample included a total of 1,184 unrelated Han Chinese adults from a Yunnan cigarette factory. All participants consented to participate in this project and provided a self-administered survey questionnaire including smoking status, smoking quantity, disease history, height, weight, and age (Supplementary Table 1). Among them, 620 were current smokers, 63 were former smokers, and 501 were never smokers. All smokers had smoked at least 100 cigarettes in their lifetimes. The most reported disease among the 1,184 participants was hypertension (32 cases, Supplementary Table 1). The study was approved by the institutional review board on human studies.
Sequencing and Genotyping
For each participant, 5 ml of peripheral blood was collected. DNA was extracted using a QIAamp DNA Mini Kit (Qiagen) according to the manufacturer’s recommendation. Approximately 2 μg of genomic DNA (determined by Qubit Fluorometer, Invitrogen) was prepared for DNA library construction. WGS was performed using the BGISEQ-500 platform (average depth >=30X). Quality control of raw sequences (FASTQ file reads) was conducted by FastQC (v.0.11.7, https://www.bioinformatics.babraham.ac.uk/projects/fastqc/). Clean paired-end reads were mapped against the human reference genome (GRCh37/hg19) by BWA (v.0.7.15) (23). Variants, including SNPs and insertion/deletion (indel) polymorphisms, were called by Genome Analysis Toolkit (GATK, v.3.8, https://gatk.broadinstitute.org/hc/en-us). The discovered SNPs/indels were annotated in the National Center for Biotechnology Information (NCBI) dbSNP database (https://www.ncbi.nlm.nih.gov/snp/).
Genome-Wide Association Study Quality Control
We applied the following exclusion criteria to filter the samples: 1) mean sequencing depth <10X; 2) 10X coverage <90%; 3) GC content outliers; 4) relative duplication; 5) absolute inbreeding coefficient >1; 6) principal components analysis (PCA) outliers; and 7) sex mismatch.
Quality control of variants was applied by the standard recommended GATK filters, including variant quality score recalibration (VQSR), largest contiguous homopolymer run of the variant allele (HomopolymerRun), binomial test (GetHetCoverage), root mean square of mapping quality (RMSMappingQuality), and strand bias (FisherStrand). To further reduce bias, the following exclusion criteria were adopted: 1) minor allele average depth <4X; 2) average depth in case or control <8; 3) eightfold rate for case or control <0.9; 4) and P-value of Hardy-Weinberg equilibrium test <10−4. In addition, variants without dbSNP IDs [also with minor allele frequency (MAF) <0.005] were excluded.
Statistical Analysis
Individual SNP-based association tests were performed by PLINK (v.1.9) using a logistic regression model (24). Adjusted covariates for association analysis included age, PC1, PC2, and PC3. Manhattan plots were generated using qqman implemented in R (25). The distribution of observed P-values was plotted against that of expected P-values to create a quantile-quantile (QQ) plot through snpStats (v.1.36.0). Haploview software (v.4.2) was employed to determine pairwise linkage disequilibrium (LD) and haplotype blocks (26, 27). Haplotype-based association analyses were examined with the Haplo Stats (v.1.7.9) package (28).
Replication Analysis
To replicate the GWAS associations, 1,329 participants including 805 smokers and 524 nonsmokers were recruited from local hospitals in Jincheng and Taiyuan of Shanxi Province in China during 2012–2013 (12). All 1,329 participants were males, aged 19–62 years (Supplementary Table 1). Participants with psychiatric diseases such as schizophrenia, Alzheimer’s disease, and major depression diagnosed by the Diagnostic and Statistical Manual of Mental Disorders (DSM)-IV criteria were excluded from enrollment. The project was approved by the Ethics Committee of First Affiliated Hospital of Zhejiang University School of Medicine. A set of answers to questions including age, education, income, medical history, environment, and smoking-related behaviors were collected by trained researchers. The sequencing was performed using Illumina HiSeq X10 and analyzed as reported previously (12).
Results
Sequencing and Variant Discovery
WGS was carried out in the 1,184 subjects, yielding approximately 1.3 trillion clean reads with an average read length of 100 bp. In the quality control steps for the study participants, as displayed in Supplementary Table 2, 26 subjects whose mean sequencing depth was <8X coverage and 37 subjects whose 10X coverage was <80% were removed. The estimate of the inbreeding coefficient and principal component analysis (PCA) filtered out 21 and 3 outliers, respectively.
To better demonstrate the population structure of our subjects, we performed PCA on individuals from our study along with samples from European (CEU), African (YRU), American (AMR), South Asian (SAS), and East Asian (EAS) obtained from the phase 3 release of 1000 Genomes Project (29). The distribution of the subjects in this study was concordant with the East Asian cluster (Figure 1A). During the test of relatedness, 49 samples were excluded from the analyses because duplicates and cryptic relatedness were detected. A total of 126 samples were removed (data for four subjects were marked as low quality by more than one rule) (Supplementary Table 2). In the remaining 1,058 subjects, there were 573 current smokers, 44 ex-smokers, and 441 never smokers (Table 1). Smoking prevalence was much higher among men (ever smokers, 50.8%) compared with women (ever smokers, 7.6%). The average sequencing depth was 34.78-fold coverage (Figure 1B).
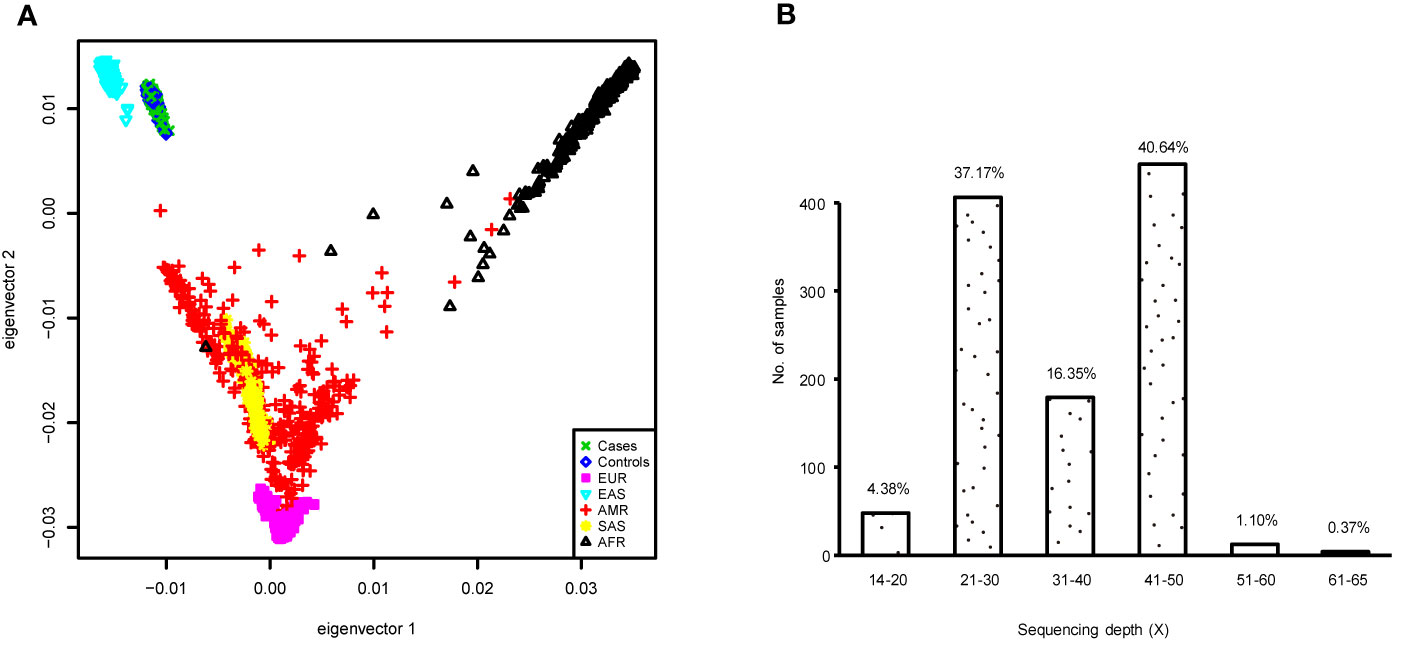
Figure 1 (A) Principal component analysis (PCA) plot of subjects enrolled in this study based on two first eigenvectors, built using all cases and controls in the genome-wide association study (GWAS) and, 2,504 individuals of European (CEU), African (YRU), American (AMR), South Asian (SAS), and East Asian (EAS) retrieved from the 1000 Genomes Project phase 3 release. (B) Distribution of the sequencing depth for the samples used in the GWAS.
After mapping all clean reads against the human hg19 reference genome, we identified a total of 46,835,008 raw variants (41,383,528 SNPs and 5,451,480 indels). Of them, 7,985,057 SNPs (19.3%) and 3,660,574 indels (67.1%) were eliminated in the variant quality control steps. Finally, 35,189,377 variants remained and were used for genome-wide association analysis (Supplementary Table 3). Among them, 1,882,293 were of low frequency (1% < MAF < 5%), and 26,578,915 were rare (MAF < 1%).
Individual Single-Nucleotide Polymorphism-Based Association Analysis
Individual SNP-based association analysis of smoking initiation was performed with 617 ever smokers vs. 441 never smokers (included both males and females). Forty-seven variants displayed statistical significance at a P-value of 10−5, including 2 SNPs without a dbSNP ID, Supplementary Data 1), 13 of which were considered to be significant at a genome-wide significance level (P < 5 x 10−8). Of them, the SNP rs139753473 showed the strongest signal [P = 2.53 x 10−10, MAF = 0.0574, odds ratio (OR) = 4.664; Figure 2 and Table 2]. The cluster consisting of rs139753473 and the other 23 SNPs (e.g., rs200713609, rs116358832, and rs796950514) is in close proximity to the Raftlin lipid raft linker 1 (RFTN1) gene (Figure 3). Of these 24 SNPs, 14 (i.e., rs796139390, rs116358832, rs796931177, rs990470344, rs796687837, rs796950514, rs796881087, rs796068970, rs796689769, rs200713609, rs796257874, rs796606528, rs796468904, and rs796525300, Supplementary Data 1) were considered to be low-frequency, i.e., 0.01 < MAF < 0.05. The next top three SNPs were located in pre-mRNA processing factor 31 (RP11-1102P16.1, an A/G polymorphism on chromosome 8 position 72324178), phosphatidylinositol transfer protein cytoplasmic 1 (PITPNC1, rs190489448), and family with sequence similarity 162 member B (FAM162B, rs11153627). In addition, 98 variants from 19 previously reported smoking-associated genes were also identified (P < 10−3, Supplementary Data 1). For instance, rs140333915 from HS6ST3 (heparan sulfate 6-O-sulfotransferase 3), rs572691375 from CYP2C19 (cytochrome P450 family 2 subfamily C member 19), and rs4875371 from CSMD1 (CUB and sushi multiple domains 1) had P-values <10−4. Of these, the rs140333915 and rs572691375 were low-frequency and rare variants (MAF = 0.0146 and 0.0073, respectively). When treating males separately, we found one genome-wide significant signal, rs11010435 (P = 4.72 x 10−8) located between PCAT5 (prostate cancer associated transcript 5) and ANKRD30A (ankyrin repeat domain 30A) and 98 variants from 31 known smoking-associated genes achieved P values of 10−3 (Supplementary Data 1). However, the corresponding QQ plot was not well-behaved (Supplementary Figure 1A).
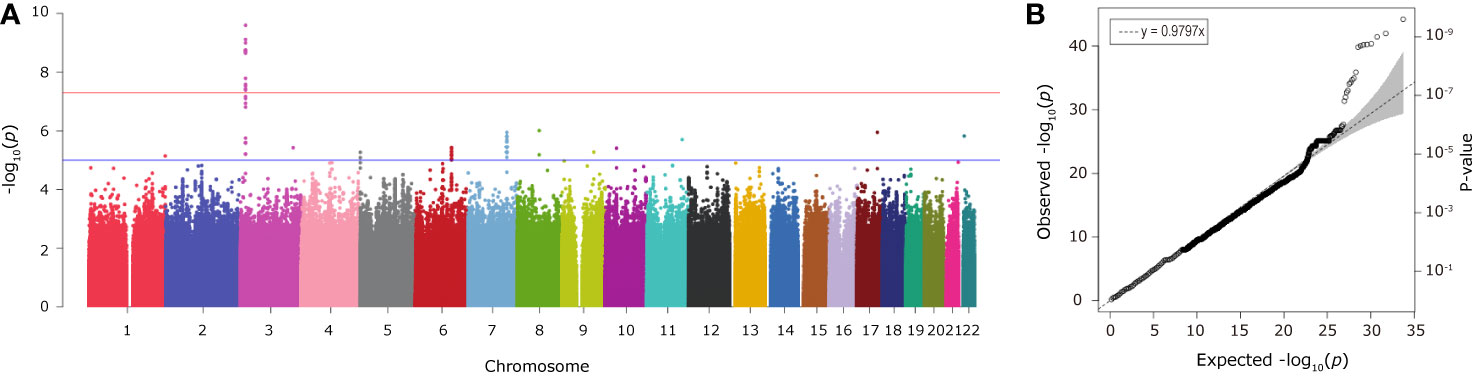
Figure 2 The Manhattan (A) and QQ (B) plots from association analysis of smoking initiation (ever vs. never smokers, with men and women combined). Approximately 12 million variants were tested in the Manhattan plot. The blue and red horizontal lines on the Manhattan plot indicate the thresholds (1 x 10−5 and 5 x 10−8, respectively). The dashed line on the QQ plot indicates the genomic inflation factor lambda.
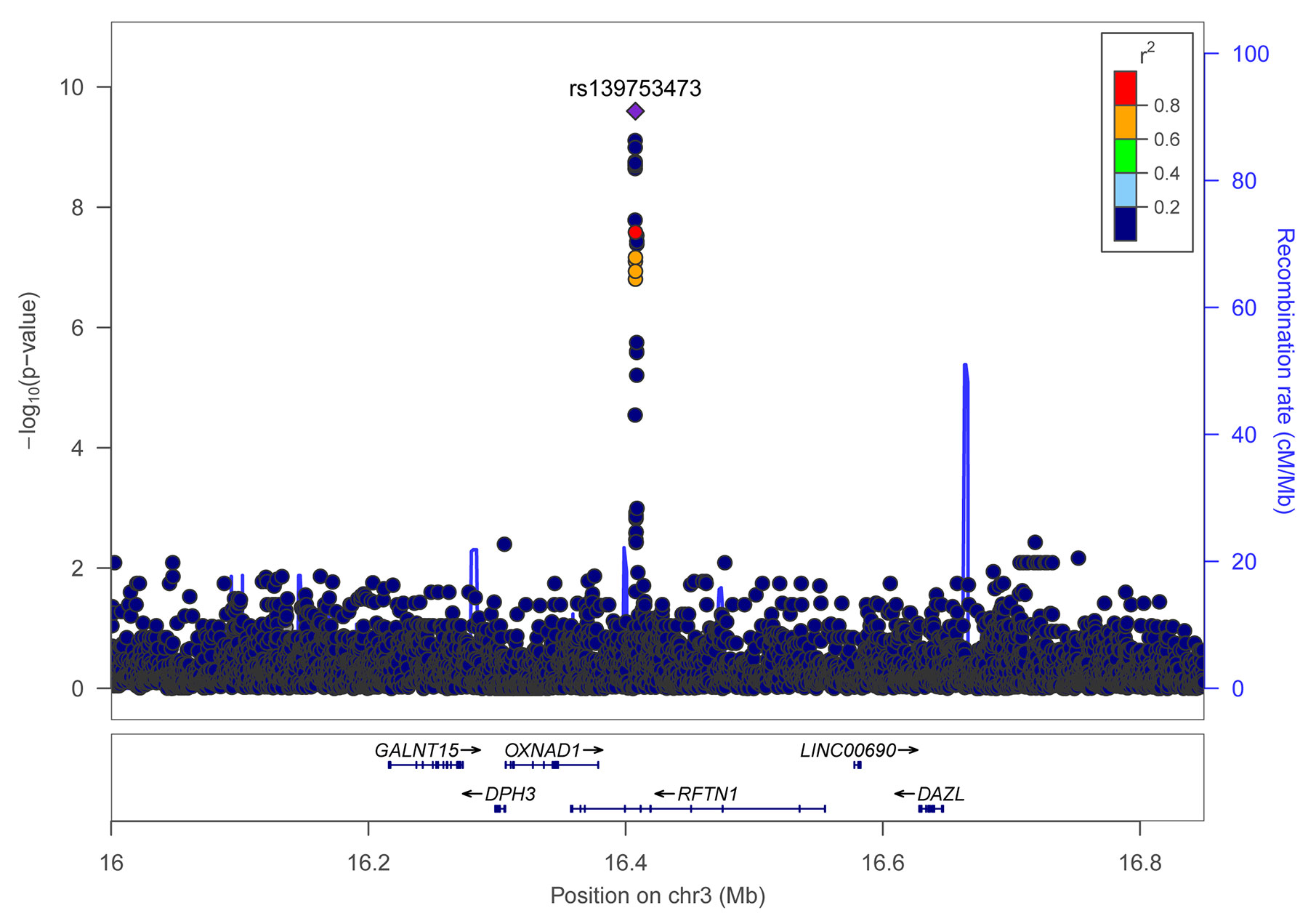
Figure 3 Regional association plot for variants of the RFTN1 gene for smoking initiation. r2 values were obtained from the hg19/1000 Genomes Nov 2014 ASN (Han Chinese and Japanese) samples. The plot was generated using LocusZoom (http://locuszoom.sph.umich.edu/).
Genome-wide association analyses for smoking quantity were conducted among 573 current smokers. With male and female smokers were combined, no genome-wide significant signals were identified (Supplementary Figure 1B and Supplementary Data 1). Despite this, 45 variants reached a P value of <10−5, with the rs78955061 from the intergenic region of ACKR3 (atypical chemokine receptor 3) and LOC93463 had the smallest P value of 7.92 x 10−7. With a cutoff of p <10−3, 27 variants from 19 known smoking-associated genes were determined. When the study sample was restricted to males, 22 SNPs yielded a P value of <10−5, of which the rs143124048 from PALLD (a gene encoding palladin protein) had the smallest P value of 1.70 x 10−6 (Supplementary Data 1), and 28 SNPs from 15 known smoking-associated genes were identified at p <10−3. However, none of these signals reached a genome-wide significant level, which may be due to a lack of statistical power (Supplementary Figure 1C).
Replication Study
Due to the fact that very few females smoke in China, only males were included in the replication sample. Variants which had a P-value lower than 10−5 in the primary analysis were selected (for RFTN1 and CSMD1, all variants with P-value <10−3 were tested). As a result, none of the smoking initiation or CPD associated loci from the total discovery sample (with men and women combined) were significant. However, 18 male-specific smoking initiation associated loci (i.e., rs10128115, rs10128145, rs72795203, rs12241402, rs10128497, rs16936694, rs7072685, rs12261634, rs11010478, rs11010482, rs112089093, rs12248963, rs1480525, rs10128398, rs12256178, rs10128169, rs1122458, and rs7071386) yielded significant results (P < 0.05, Supplementary Table 4). All of these SNPs were located in the intergenic region between PCAT5 and ANKRD30A. At a P-value threshold of 0.10, another 15 SNPs also showed evidence for replication, 14 of which were in the intergenic region between PCAT5 and ANKRD30A. The other one, rs4590382 (P = 0.06), was an intergenic SNP between LOC101928283 and GRM8. For male-specific CPD- associated loci in the primary analysis, no evidence of replication was observed. Intriguingly, in testing for an association with CPD, the rs139753473 within RFTN1 and six SNPs within CSMD1 showed a P-value of <0.05 (Table 3). The six SNPs from CSMD1 included rs76965088, rs117740219, rs78094590, rs138695620, rs76195425, and rs148939406. Furthermore, one SNP from RFTN1 (rs796139390), and four SNPs from CSMD1 (i.e., rs114254701, rs10503200, rs56391646, and rs149909271) achieved a nominally marginal significance level (P < 0.10) for an association with CPD.
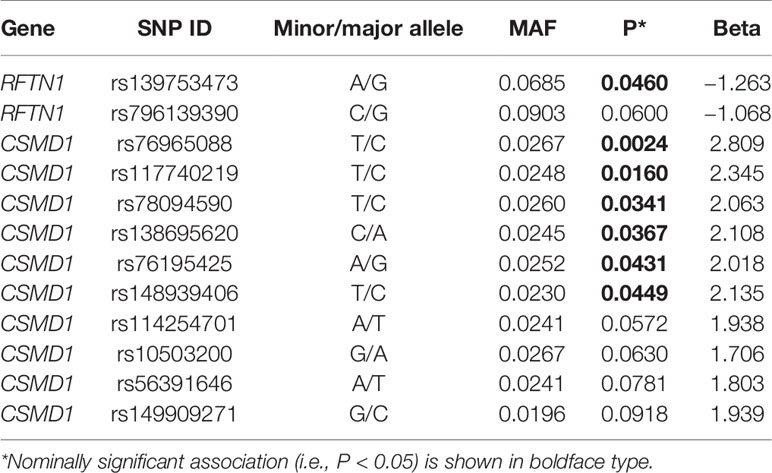
Table 3 Cigarettes per day (CPD)-associated single-nucleotide polymorphisms (SNPs) within RFTN1 and CSMD1 in the replication sample.
Haplotype-Based Association Analysis of RFTN1 and CSMD1
For the 24 SNPs in RFTN1 with a P-value of <10−3 according to the results from individual SNP-based association analysis for smoking initiation, two LD blocks were identified (D’ > 0.97) (Figure 4). Association tests between these haplotypes and smoking initiation revealed that two haplotypes, C-A-C-G and A-G-T-C, formed by rs796812630, rs796584733, rs796349027, and rs879511366 were significantly associated with smoking initiation under the additive model (Hap-Score = −4.57 and 4.63, P = 4.83 x 10−6 and 3.65 x 10−6, respectively, Table 4). In addition, three haplotypes, T-T-C-C-C, T-T-A-T-T, and C-A-A-T-T, constituted by rs879401109-rs879453873-rs75180423-rs541378415-rs796757175 also correlated with smoking initiation (Hap-Score = −5.33, 3.10 and 4.50, P = 1.00 x 10−7, 1.94 x 10−3 and 6.91 x 10−6, respectively, Table 4).
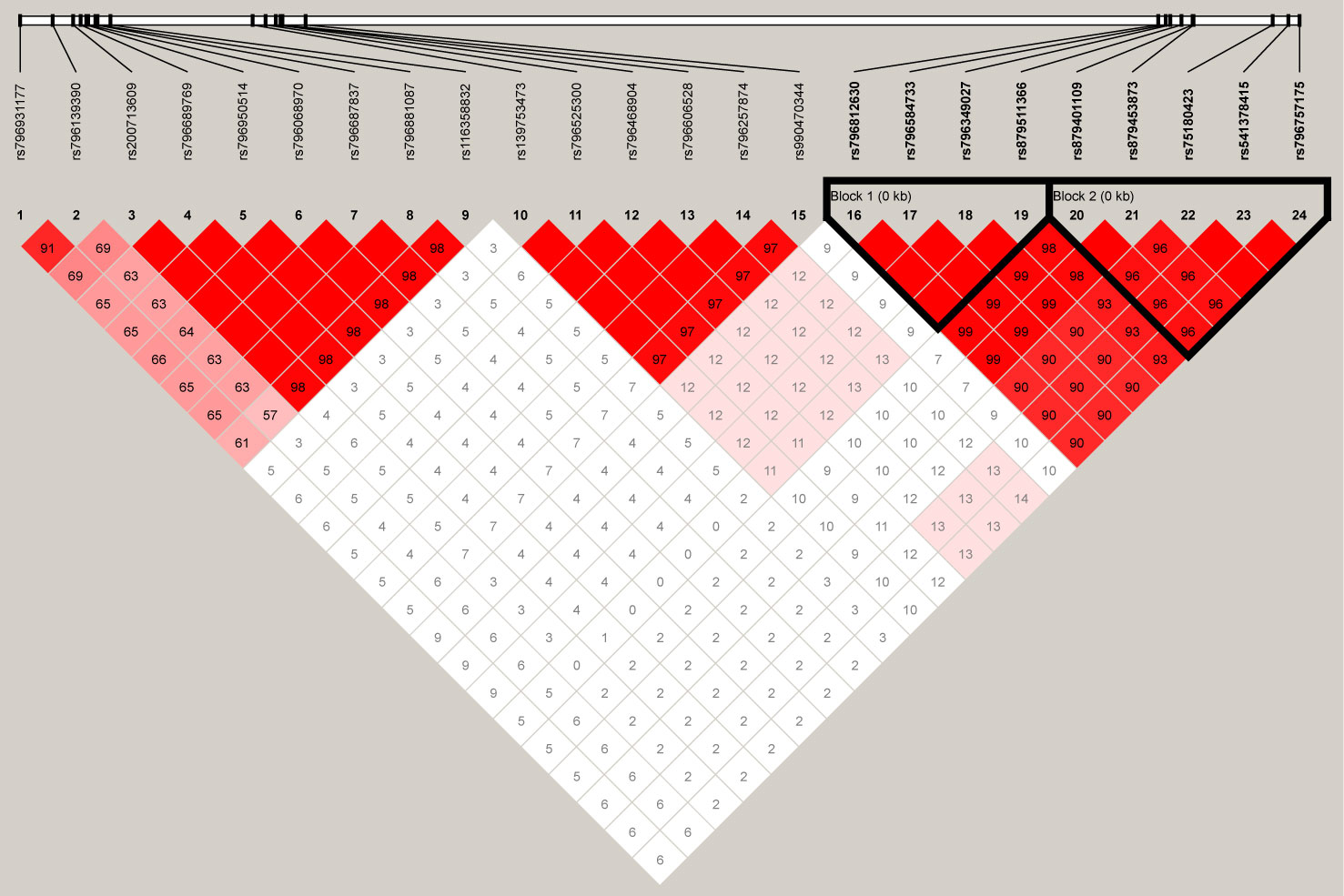
Figure 4 The linkage disequilibrium (LD) plot depicts the structure of haplotype blocks encompassing the 24 single-nucleotide polymorphisms (SNPs) located in the RFTN1 gene in our Chinese Han population. This plot was generated using Haploview (https://www.broadinstitute.org/haploview/haploview). The standard Haploview LD color scheme based on D’ has been applied. The value of 100 x D’ is displayed for each SNP pair’s tile unless D’ = 1.
Given that in the individual SNP-based association analysis of smoking initiation, the CSMD1 had the largest number of SNPs with P <10−3, of the reported smoking-associated genes, haplotype-based association analysis was also performed on the 29 SNPs in CSMD1. One LD block exhibited a D’ larger than 0.97 (Supplementary Figure 2). As shown in Table 4, two haplotypes, C-A-G-G and T-C-T-T, derived from rs4875371-rs4875372-rs17070935-rs11991366, were strongly associated with smoking initiation (Hap-Score = −3.35 and 3.77, P = 3.36 x 10-4 and 1.61 x 10-4, respectively).
Bioinformatics Functional Assessment of RFTN1
In silico functional analyses based on the RegulomeDB (https://regulomedb.org/) and HaploReg (https://pubs.broadinstitute.org/mammals/haploreg/haploreg.php) databases were performed for SNPs with P <10−8, i.e., rs139753473, rs200713609, rs116358832, rs796950514, rs796881087, rs796687837, rs796068970, and rs796689769. All of these SNPs were intron variants within RFTN1. The male-specific smoking associated rs11010435 was omitted because the GWAS did not have adequate power and this locus was not replicated. Although evidence of regulatory potential was weak for rs139753473 (RegulomeDB score = 0.008), this SNP alters E2F, Egr-1, MOVO, Nrf1, UF1H3BETA, YY1, and SP1 transcription factor binding motifs according to HaploReg (Supplementary Table 5). A further investigation using the PROMO prediction tool (30) suggested that the locus of rs139753473 interacted with two transcription factors, including the glucocorticoid receptor alpha and the androgen receptor. Interestingly, the glucocorticoid receptor has been reported to be associated with the probability of smoking severity and cessation in a sample of obstructive airway disease patients (31). It has been suggested that cigarette smoking could increase androgen receptor activity (32). Additionally, rs200713609 had a RegulomeDB score of 0.61 and could alter the PEBP transcription factor binding motif. The RegulomeDB score for rs116358832 was 0.13. Motifs altered by rs116358832 included CEBPB and GATA. For rs796950514, rs796881087, rs796687837, rs796068970, and rs796689769, the RegulomeDB score ranked from 0.13 to 0.61, and no altered motif was found in either RegulomeDB or HaploReg. In the examination of the correlation between these variants and the expression of RFTN1, no available expression quantitative trait loci (eQTL) data could be found. Furthermore, according to the expression pattern retrieved from GTEX PORTAL (https://gtexportal.org/home/), RFTN1 had the highest expression in lymphocytes and was also expressed in various brain tissues, such as the cortex, the frontal cortex (BA9), and cerebellum (Supplementary Figure 3).
Discussion
Associations between genetic variants and cigarette smoking have been largely deciphered for European- and American-ancestry populations (21, 33). For the Chinese Han population, studies on genetic factors conferring smoking susceptibility are still limited in the literature. Here we performed deep WGS of 1,184 Chinese samples and discovered 35 million variants. Of them, 1,882,293 (5%) and 26,578,915 (76%) were found to be low-frequency and rare variants, respectively. Follow up replication analyses revealed risk alleles in RFTN1, CSMD1, and PCAT5/ANKRD30A genes likely contributing to smoking behavior.
In the discover stage, 13 SNPs from RFTN1 were significantly associated with smoking initiation, i.e., rs139753473, rs200713609, rs116358832, rs796950514, rs796881087, rs796687837, rs796068970, rs796689769, rs796931177, rs796525300, rs75180423, rs796757175, and rs541378415. The rs11010435 from the intergenic region of PCAT5 and ANKRD30A was also significantly associated with smoking initiation in male smokers. For CPD, we found no genome-wide significant signals, but there were 45 and 22 variants in the total sample and the male subgroup, respectively, at the threshold of P <10−5. To validate the preliminary findings, we performed a replication study for the variants with a P-value less than 10−5 (for RFTN1 and CSMD1, variants with P <10−3 were included), using another Chinese Han sample containing 1,329 male subjects. Although variants associated with smoking initiation and CPD in the total discovery sample were not replicated, we replicated 18 loci for their association with smoking initiation in men, which including rs10128115, rs10128145, rs72795203, rs12241402, rs10128497, rs16936694, rs7072685, rs12261634, rs11010478, rs11010482, rs112089093, rs12248963, rs1480525, rs10128398, rs12256178, rs10128169, rs1122458, and rs7071386 from the intergenic region between PCAT5 and ANKRD30A (P < 0.05). For male-specific associations with CPD, no evidence of replication was found. Furthermore, although RFTN1 and CSMD1 were originally identified in the test of smoking initiation, in the replication test of CPD, the RFTN1 gene’s rs139753473 and the CSMD1 gene’s rs76965088, rs117740219, rs78094590, rs138695620, rs76195425, and rs148939406 reached a P-value of <0.05. Given that smoking initiation and CPD are moderately correlated smoking behavior traits (correlation coefficient r = 0.425, P = 2.6 x 10−15) (34), these association results for RFTN1 and CSMD1 are of great interest and warranted to elucidate their biological roles in smoking.
Within the 24 SNPs observed in the RFTN1, two LD blocks, rs796812630-rs796584733-rs796349027-rs879511366 and rs879401109-rs879453873-rs75180423-rs541378415-rs796757175, were uncovered. Two haplotypes (i.e., C-A-C-G and A-G-T-C) from the former and three (i.e., T-T-C-C-C, T-T-A-T-T, and C-A-A-T-T) from the latter were significantly associated with smoking initiation. Additionally, haplotype-based association analysis also showed that two CSMD1-derived haplotypes, C-A-G-G and T-C-T-T formed by rs4875371-rs4875372-rs17070935-rs11991366 were strongly correlated with smoking initiation.
The lead SNP (rs139753473) associated with smoking initiation is located within the intron region of the RFTN1 gene. It may bind to two transcription factors, i.e., the glucocorticoid receptor alpha and the androgen receptor, which have been proposed to play a role in smoking. Nonetheless, the functional studies were carried out in silico and need experimental validation. Although RFTN1 had the highest expression in lymphocytes, its expression can also be found in brain tissues, e.g., the cortex, frontal cortex (BA9), and cerebellum. These regions are believed to be involved in the brain’s reward and inhibitory control processes (35, 36). In addition, RFTN1 contributes to multiple immune-related biological pathways, including B and T cell receptor (BCR and TCR, respectively) signaling, toll-like receptor (TLR) 3 signaling, and interleukin-17 (IL17) production (37–40). It has been increasingly recognized that activation of central immune signaling by substances (e.g., opioids) can enhance drug reward (41, 42). In mice, TLR3 modulates cocaine reward through pro-inflammatory immune signaling (43). In particular, BCR, TCR, TLR, and IL17 induce activation of nuclear factor kappaB (NF-κB) (44–47), and NF-κB mediates the reward effects of drugs (e.g., cocaine) (48). Moreover, NF-κB is not only a transcription factor involved in inflammation and the immune response (49), but is also a regulator of synaptic plasticity and memory (50).It is plausible that RFTN1 could play a role in smoking initiation by regulating immune responsiveness.
CSMD1 is a complement-regulatory protein that is highly expressed in the central nervous system, contributing to addiction vulnerability (51). In an analysis of 4,122 psoriasis cases and 3,101 healthy controls, CSMD1 showed evidence of association with cigarette smoking (52). Regarding PCAT5 and ANKRD30A, it is reported that both of them are related to cancer progression. For instance, PCAT5 is a long noncoding RNA regulated by the ERG, an active transcription factor common in human prostate cancer (53). Similarly, the ANKRD30A encodes a DNA-binding transcription factor implicated in breast cancer (54, 55). Our findings indicated that these two genes could be potential targets to investigate the connection between smoking and cancers.
One major limitation of this study is the sample size. Due to the relatively high sequencing cost per sample and difficulty in recruiting participants, our sample size was not large enough to provide various phenotypes and adequate statistical power. Although the application of WGS in genome-wide association analysis allowed for successful detection of a few novel or low-frequency variants associated with cigarette smoking, these SNPs are infrequently reported in previous smoking or other psychiatric‐related traits, preventing further functional assessments. This also raised the question of whether the observed loci, especially those from RFTN1 and PCAT5/ANKRD30A, were specifically associated with smoking in the Chinese population. Further studies with particular attention to these genes are therefore required to address this issue. Another concern is that smoking prevalence is usually different by sex, while in this study, association analyses were not performed in a female-specific manner, because the discovery cohorts only included 74 current female smokers and there were no females in the replication sample. In addition, the Fagerström Test for Nicotine Dependence (FTND) is a widely used instrument to estimate nicotine dependence. It is possible that GWAS of this phenotype may produce more robust results. Additionally, as a major metabolite of nicotine, cotinine represents a direct biomarker of smoking quantity. Measuring the cotinine level in serum, urine, or saliva could benefit further validation studies, by providing more objective information on smoking quantity.
In summary, to the best of our knowledge, we have reported the first WGS-based GWAS of smoking phenotypes in a Chinese Han cohort. We provided exploratory evidence that RFTN1 and CSMD1 are involved in smoking. Associations between smoking initiation and PCAT5/ANKRD30A were also detected and replicated in a male-specific manner. RFTN1 might function in smoking initiation through interactions with the immune system, the glucocorticoid receptor alpha and androgen receptor signaling. These findings provide extensive insight into the biological mechanisms of smoking behavior in the Chinese Han population.
Data Availability Statement
The datasets presented in this article are not readily available because due to the restriction of “Regulation of the People’s Republic of China on the Administration of Human Genetic Resources”, sequencing data of this study can not be shared publicly. Requests to access the datasets should be directed to zhuzhouhai@gmail.com.
Ethics Statement
The studies involving human participants were reviewed and approved by Biomedical Ethics Committee of Joint Institute of Tobacco and Health. The patients/participants provided their written informed consent to participate in this study.
Author Contributions
ML, JY, and ZZ conceived the study. YC, ML, and QL performed the data analysis. ML wrote the manuscript. All authors contributed to the article and approved the submitted version.
Conflict of Interest
The Joint Institute of Tobacco and Health received research grants from China Tobacco Yunnan Industrial CO. LTD. Author QL was employed by the Hangzhou Global Biotechnology and Bioinformatics CO. LTD. However, this study was carried out independently from either the funding agency or employer.
The remaining authors declare that the research was conducted in the absence of any commercial or financial relationships that could be construed as a potential conflict of interest.
Acknowledgments
The authors are grateful to all participants of this study. We also would like to thank Dr. Chuan Liu and Dr. Yupeng Cun for their helpful suggestions.
Supplementary Material
The Supplementary Material for this article can be found online at: https://www.frontiersin.org/articles/10.3389/fpsyt.2020.564239/full#supplementary-material
Supplementary data 1 | Association analysis results of smoking initiation and CPD in this study.
Supplementary data 2 | This file contains Supplementary Figures 1-3.
Supplementary data 3 | This file contains Supplementary Tables 1-5.
References
1. Islami F, Stoklosa M, Drope J, Jemal A. Global and Regional Patterns of Tobacco Smoking and Tobacco Control Policies. Eur Urol Focus (2015) 1(1):3–16. doi: 10.1016/j.euf.2014.10.001
2. WHO. WHO report on the global tobacco epidemic 2019: Offer help to quit tobacco use. Geneva: WHO Press (2019).
3. Messner B, Bernhard D. Smoking and cardiovascular disease: mechanisms of endothelial dysfunction and early atherogenesis. Arterioscler Thromb Vasc Biol (2014) 34(3):509–15. doi: 10.1161/ATVBAHA.113.300156
4. Salvi S. Tobacco smoking and environmental risk factors for chronic obstructive pulmonary disease. Clin Chest Med (2014) 35(1):17–27. doi: 10.1016/j.ccm.2013.09.011
5. Pfeifer GP, Denissenko MF, Olivier M, Tretyakova N, Hecht SS, Hainaut P. Tobacco smoke carcinogens, DNA damage and p53 mutations in smoking-associated cancers. Oncogene (2002) 21(48):7435–51. doi: 10.1038/sj.onc.1205803
6. Siegel RL, Jacobs EJ, Newton CC, Feskanich D, Freedman ND, Prentice RL, et al. Deaths Due to Cigarette Smoking for 12 Smoking-Related Cancers in the United States. JAMA Intern Med (2015) 175(9):1574–6. doi: 10.1001/jamainternmed.2015.2398
7. Gakidou E, Afshin A, Abajobir AA, Abate KH, Abbafati C, Abbas KM, et al. Global, regional, and national comparative risk assessment of 84 behavioural, environmental and occupational, and metabolic risks or clusters of risks, 1990–2016: a systematic analysis for the Global Burden of Disease Study 2016. Lancet (2017) 390(10100):1345–422. doi: 10.1016/S0140-6736(17)32366-8
8. Baker E, Webb Hooper M. Smoking Behavior. In: Gellman MD, Turner JR, editors. Encyclopedia of Behavioral Medicine. New York, NY: Springer New York (2013). p. 1818–20.
9. Bierut LJ. Nicotine dependence and genetic variation in the nicotinic receptors. Drug Alcohol Depend (2009) 104:S64–S9. doi: 10.1016/j.drugalcdep.2009.06.003
10. Wu J. Understanding of nicotinic acetylcholine receptors. Acta Pharmacol Sin (2009) 30(6):653–5. doi: 10.1038/aps.2009.89
11. Thorgeirsson TE, Gudbjartsson DF, Surakka I, Vink JM, Amin N, Geller F, et al. Sequence variants at CHRNB3-CHRNA6 and CYP2A6 affect smoking behavior. Nat Genet (2010) 42(5):448–53. doi: 10.1038/ng.573
12. Liu Q, Han H, Wang M, Yao Y, Wen L, Jiang K, et al. Association and cis-mQTL analysis of variants in CHRNA3-A5, CHRNA7, CHRNB2, and CHRNB4 in relation to nicotine dependence in a Chinese Han population. Transl Psychiatry (2018) 8(1):83. doi: 10.1038/s41398-018-0130-x
13. Hancock DB, Reginsson GW, Gaddis NC, Chen X, Saccone NL, Lutz SM, et al. Genome-wide meta-analysis reveals common splice site acceptor variant in CHRNA4 associated with nicotine dependence. Transl Psychiatry (2015) 5:e651. doi: 10.1038/tp.2015.149
14. Vandenbergh DJ, O’Connor RJ, Grant MD, Jefferson AL, Vogler GP, Strasser AA, et al. Dopamine receptor genes (DRD2, DRD3 and DRD4) and gene-gene interactions associated with smoking-related behaviors. Addict Biol (2007) 12(1):106–16. doi: 10.1111/j.1369-1600.2007.00054.x
15. Tobacco and Genetics Consortium. Genome-wide meta-analyses identify multiple loci associated with smoking behavior. Nat Genet (2010) 42(5):441–7. doi: 10.1038/ng.571
16. Batra V, Patkar AA, Berrettini WH, Weinstein SP, Leone FT. The genetic determinants of smoking. Chest (2003) 123(5):1730–9. doi: 10.1378/chest.123.5.1730
17. Loukola A, Hällfors J, Korhonen T, Kaprio J. Genetics and smoking. Curr Addict Rep (2014) 1(1):75–82. doi: 10.1007/s40429-013-0006-3
18. Li Q, Hsia J, Yang G. Prevalence of smoking in China in 2010. N Engl J Med (2011) 364(25):2469–70. doi: 10.1056/NEJMc1102459
19. Yang G, Wang Y, Wu Y, Yang J, Wan X. The road to effective tobacco control in China. Lancet (2015) 385(9972):1019–28. doi: 10.1016/S0140-6736(15)60174-X
20. Tozzi F, Teumer A, Munafo M, Rawal R, Kazeem G, Gerbaulet M, et al. A genomewide association study of smoking relapse in four European population-based samples. Psychiatr Genet (2013) 23(4):143–52. doi: 10.1097/YPG.0b013e32835fc94b
21. Gelernter J, Kranzler HR, Sherva R, Almasy L, Herman AI, Koesterer R, et al. Genome-wide association study of nicotine dependence in American populations: identification of novel risk loci in both African-Americans and European-Americans. Biol Psychiatry (2015) 77(5):493–503. doi: 10.1016/j.biopsych.2014.08.025
22. Hancock DB, Guo Y, Reginsson GW, Gaddis NC, Lutz SM, Sherva R, et al. Genome-wide association study across European and African American ancestries identifies a SNP in DNMT3B contributing to nicotine dependence. Mol Psychiatry (2018) 23(9):1911–9. doi: 10.1038/mp.2017.193
23. Li H, Durbin R. Fast and accurate short read alignment with Burrows-Wheeler transform. Bioinformatics (2009) 25(14):1754–60. doi: 10.1093/bioinformatics/btp324
24. Chang CC, Chow CC, Tellier LC, Vattikuti S, Purcell SM, Lee JJ. Second-generation PLINK: rising to the challenge of larger and richer datasets. Gigascience (2015) 4:7. doi: 10.1186/s13742-015-0047-8
25. Turner SD. qqman: an R package for visualizing GWAS results using QQ and manhattan plots. J Open Source Softw (2018) 3(25):731. doi: 10.21105/joss.00731
26. Barrett JC, Fry B, Maller J, Daly MJ. Haploview: analysis and visualization of LD and haplotype maps. Bioinformatics (2005) 21(2):263–5. doi: 10.1093/bioinformatics/bth457
27. Gabriel SB, Schaffner SF, Nguyen H, Moore JM, Roy J, Blumenstiel B, et al. The structure of haplotype blocks in the human genome. Science (2002) 296(5576):2225–9. doi: 10.1126/science.1069424
28. Lake SL, Lyon H, Tantisira K, Silverman EK, Weiss ST, Laird NM, et al. Estimation and tests of haplotype-environment interaction when linkage phase is ambiguous. Hum Hered (2003) 55(1):56–65. doi: 10.1159/000071811
29. Sudmant PH, Rausch T, Gardner EJ, Handsaker RE, Abyzov A, Huddleston J, et al. An integrated map of structural variation in 2,504 human genomes. Nature (2015) 526(7571):75–81. doi: 10.1038/nature15394
30. Farre D, Roset R, Huerta M, Adsuara JE, Rosello L, Alba MM, et al. Identification of patterns in biological sequences at the ALGGEN server: PROMO and MALGEN. Nucleic Acids Res (2003) 31(13):3651–3. doi: 10.1093/nar/gkg605
31. Rogausch A, Kochen MM, Meineke C, Hennig J. Association between the BclI glucocorticoid receptor polymorphism and smoking in a sample of patients with obstructive airway disease. Addict Biol (2007) 12(1):93–9. doi: 10.1111/j.1369-1600.2006.00045.x
32. Shiota M, Ushijima M, Imada K, Kashiwagi E, Takeuchi A, Inokuchi J, et al. Cigarette smoking augments androgen receptor activity and promotes resistance to antiandrogen therapy. Prostate (2019) 79(10):1147–55. doi: 10.1002/pros.23828
33. Yang J, Wang S, Yang Z, Hodgkinson CA, Iarikova P, Ma JZ, et al. The contribution of rare and common variants in 30 genes to risk nicotine dependence. Mol Psychiatry (2015) 20(11):1467–78. doi: 10.1038/mp.2014.156
34. Chang LH, Ong JS, An J, Verweij KJH, Vink JM, Pasman J, et al. Investigating the genetic and causal relationship between initiation or use of alcohol, caffeine, cannabis and nicotine. Drug Alcohol Depend (2020) 210:107966. doi: 10.1016/j.drugalcdep.2020.107966
35. Goldstein RZ, Volkow ND. Drug addiction and its underlying neurobiological basis: neuroimaging evidence for the involvement of the frontal cortex. Am J Psychiatry (2002) 159(10):1642–52. doi: 10.1176/appi.ajp.159.10.1642
36. Moulton EA, Elman I, Becerra LR, Goldstein RZ, Borsook D. The cerebellum and addiction: insights gained from neuroimaging research. Addict Biol (2014) 19(3):317–31. doi: 10.1111/adb.12101
37. Saeki K, Miura Y, Aki D, Kurosaki T, Yoshimura A. The B cell-specific major raft protein, Raftlin, is necessary for the integrity of lipid raft and BCR signal transduction. EMBO J (2003) 22(12):3015–26. doi: 10.1093/emboj/cdg293
38. Uchimura T, Oyama Y, Deng M, Guo H, Wilson JE, Rampanelli E, et al. The Innate Immune Sensor NLRC3 Acts as a Rheostat that Fine-Tunes T Cell Responses in Infection and Autoimmunity. Immunity (2018) 49(6):1049–61 e6. doi: 10.1016/j.immuni.2018.10.008
39. Yan K, Liang J, Zhang X, Deng L, Feng D, Ling B. Polyinosinic-polycytidylic acid induces innate immune responses via Toll-like receptor 3 in human ovarian granulosa cells. Immunol Cell Biol (2019) 97(8):753–65. doi: 10.1111/imcb.12269
40. Hurtado CG, Wan F, Housseau F, Sears CL. Roles for Interleukin 17 and Adaptive Immunity in Pathogenesis of Colorectal Cancer. Gastroenterology (2018) 155(6):1706–15. doi: 10.1053/j.gastro.2018.08.056
41. Jacobsen JH, Hutchinson MR, Mustafa S. Drug addiction: targeting dynamic neuroimmune receptor interactions as a potential therapeutic strategy. Curr Opin Pharmacol (2016) 26:131–7. doi: 10.1016/j.coph.2015.10.010
42. Liu JF, Li JX. Drug addiction: a curable mental disorder? Acta Pharmacol Sin (2018) 39(12):1823–9. doi: 10.1038/s41401-018-0180-x
43. Zhu R, Bu Q, Fu D, Shao X, Jiang L, Guo W, et al. Toll-like receptor 3 modulates the behavioral effects of cocaine in mice. J Neuroinflammation (2018) 15(1):93. doi: 10.1186/s12974-018-1130-8
44. Schuman J, Chen Y, Podd A, Yu M, Liu HH, Wen R, et al. A critical role of TAK1 in B-cell receptor-mediated nuclear factor κB activation. Blood (2009) 113(19):4566–74. doi: 10.1182/blood-2008-08-176057
45. Paul S, Schaefer BC. A new look at T cell receptor signaling to nuclear factor-κB. Trends Immunol (2013) 34(6):269–81. doi: 10.1016/j.it.2013.02.002
46. Kawai T, Akira S. Signaling to NF-κB by Toll-like receptors. Trends Mol Med (2007) 13(11):460–9. doi: 10.1016/j.molmed.2007.09.002
47. Hata K, Andoh A, Shimada M, Fujino S, Bamba S, Araki Y, et al. IL-17 stimulates inflammatory responses via NF-κB and MAP kinase pathways in human colonic myofibroblasts. Am J Physiol Gastrointest Liver Physiol (2002) 282(6):G1035–44. doi: 10.1152/ajpgi.00494.2001
48. Russo SJ, Wilkinson MB, Mazei-Robison MS, Dietz DM, Maze I, Krishnan V, et al. Nuclear factor κB signaling regulates neuronal morphology and cocaine reward. J Neurosci (2009) 29(11):3529–37. doi: 10.1523/JNEUROSCI.6173-08.2009
49. Robison AJ, Nestler EJ. Transcriptional and epigenetic mechanisms of addiction. Nat Rev Neurosci (2011) 12(11):623–37. doi: 10.1038/nrn3111
50. Mattson MP, Meffert MK. Roles for NF-κB in nerve cell survival, plasticity, and disease. Cell Death Differ (2006) 13(5):852–60. doi: 10.1038/sj.cdd.4401837
51. Liu QR, Drgon T, Johnson C, Walther D, Hess J, Uhl GR. Addiction molecular genetics: 639,401 SNP whole genome association identifies many “cell adhesion” genes. Am J Med Genet B Neuropsychiatr Genet (2006) 141B(8):918–25. doi: 10.1002/ajmg.b.30436
52. Yin XY, Cheng H, Wang WJ, Wang WJ, Fu HY, Liu LH, et al. TNIP1/ANXA6 and CSMD1 variants interacting with cigarette smoking, alcohol intake affect risk of psoriasis. J Dermatol Sci (2013) 70(2):94–8. doi: 10.1016/j.jdermsci.2013.02.006
53. Ylipaa A, Kivinummi K, Kohvakka A, Annala M, Latonen L, Scaravilli M, et al. Transcriptome Sequencing Reveals PCAT5 as a Novel ERG-Regulated Long Noncoding RNA in Prostate Cancer. Cancer Res (2015) 75(19):4026–31. doi: 10.1158/0008-5472.CAN-15-0217
54. Balafoutas D, zur Hausen A, Mayer S, Hirschfeld M, Jaeger M, Denschlag D, et al. Cancer testis antigens and NY-BR-1 expression in primary breast cancer: prognostic and therapeutic implications. BMC Cancer (2013) 13:271. doi: 10.1186/1471-2407-13-271
Keywords: CUB and sushi multiple domains 1 gene, Han Chinese, immune system, raftlin lipid linker 1 gene, whole-genome sequencing
Citation: Li M, Chen Y, Yao J, Lu S, Guan Y, Xu Y, Liu Q, Sun S, Mi Q, Mei J, Li X, Miao M, Zhao S and Zhu Z (2020) Genome-Wide Association Study of Smoking Behavior Traits in a Chinese Han Population. Front. Psychiatry 11:564239. doi: 10.3389/fpsyt.2020.564239
Received: 21 May 2020; Accepted: 17 August 2020;
Published: 09 September 2020.
Edited by:
Chad A. Bousman, University of Calgary, CanadaReviewed by:
Tianhua Niu, Tulane University, United StatesMira Jakovcevski, Max Planck Institute of Psychiatry (MPI), Germany
Copyright © 2020 Li, Chen, Yao, Lu, Guan, Xu, Liu, Sun, Mi, Mei, Li, Miao, Zhao and Zhu. This is an open-access article distributed under the terms of the Creative Commons Attribution License (CC BY). The use, distribution or reproduction in other forums is permitted, provided the original author(s) and the copyright owner(s) are credited and that the original publication in this journal is cited, in accordance with accepted academic practice. No use, distribution or reproduction is permitted which does not comply with these terms.
*Correspondence: Zhouhai Zhu, zhuzhouhai@gmail.com