- 1Department of Radiology, Second Xiangya Hospital of Central South University, Changsha, China
- 2Hunan Judicial Police Vocational College, Changsha, China
- 3School of Computer Science and Engineering, Central South University, Changsha, China
- 4Hunan Province Engineering Technology Research Center of Computer Vision and Intelligent Medical Treatment, Changsha, China
- 5Yunnan Institute for Drug Abuse, Kunming, China
Understanding the brain mechanisms of heroin dependence is invaluable for developing effective treatment. Measurement of regional cerebral blood flow (CBF) provides a method to visualize brain circuits that are functionally impaired by heroin dependence. This study examined regional CBF alterations and their clinical associations in unmedicated heroin-dependent individuals (HDIs) using a relatively large sample. Sixty-eight (42 males, 26 females; age: 40.9 ± 7.3 years) HDIs and forty-seven (34 males, 13 females; age: 39.3 ± 9.2 years) matched healthy controls (HCs) underwent high-resolution T1 and whole-brain arterial spin labeling (ASL) perfusion magnetic resonance imaging (MRI) scans. Additionally, clinical characteristics were collected for neurocognitive assessments. HDIs showed worse neuropsychological performance than HCs and had decreased relative CBF (rCBF) in the bilateral middle frontal gyrus (MFG), inferior temporal gyrus, precuneus, posterior cerebellar lobe, cerebellar vermis, and the midbrain adjacent to the ventral tegmental area; right posterior cingulate gyrus, thalamus, and calcarine. rCBF in the bilateral MFG was negatively correlated with Trail Making Test time in HDIs. HDIs had limbic, frontal, and parietal hypoperfusion areas. Low CBF in the MFG indicated cognitive impairment in HDIs. Together, these findings suggest the MFG as a critical region in HDIs and suggest ASL-derived CBF as a potential marker for use in heroin addiction studies.
Introduction
Heroin addiction has been a large societal and health problem worldwide for many decades. Long-term use of heroin induces progressive spongiform leukodystrophy, or heroin encephalopathy, resulting in a range of mental symptoms and somatic activity disorders. It is also a major causal factor for the accelerated spread of AIDS, hepatitis C and other major infectious diseases due to the sharing of syringes among patients with addiction (1). While tremendous research advances in heroin addiction have been achieved over the past decades, heroin addiction treatment still has a high relapse rate. A total of 55.8–80% (2, 3) of patients relapsed to heroin use after being abstinent from drug use for 1–3 years at a 12-month follow-up. A major reason for the lack of an effective method for preventing relapse is the lack of a clear picture of heroin addiction-related brain mechanisms (4).
Neuroimaging has been a major tool used to examine brain alterations in heroin addiction/dependence. Brain function involves regional metabolism, which is tightly coupled with cerebral blood flow (CBF) (5). Therefore, measuring regional CBF can enable direct visualization of regional functional brain alterations (6) in heroin-dependent individuals (HDIs). A few studies (7–9) reported decreased CBF in the frontal and parietal lobes in HDIs. While these hypoperfusion patterns are consistent with the frontal and parietal functional impairments often observed in HDIs, relevant findings show large discrepancies across studies, which might be related to the sample sizes included. Some of the hypoperfusion patterns lacked explicit clinical associations. Another major issue is that early studies were based on [18F]-fluoro-deoxy-D-glucose (18F-FDG) positron emission tomography-computed tomography (PET-CT) or single-photon emission computed tomography (SPECT), which require injection of radioactive tracers and are not acceptable to all subjects.
Arterial spin labeling (ASL) perfusion magnetic resonance imaging (MRI) (10, 11) is an MR-based, entirely non-invasive technique for quantifying CBF. ASL uses magnetically labeled arterial blood as an endogenous tracer (12). The perfusion signal is extracted from MR images with labeled arterial blood compared to those without labeling (13). CBF was calculated using the one-compartment model (14) implemented in ASLtbx (15):
where f is the CBF in ml/100 g-min, α is the labeling efficiency (0.9), and TI1 and TI2 are the time of saturation and the time of imaging after applying the spin labeling pulse, respectively. Their values were given above. T1b is the T1 of blood (1.67 s) (16). M0b is the equilibrium magnetization of blood. Because we did not acquire separate M0 images, M0b was approximated by the control image intensity in this study.
CBF measured with ASL MRI has been shown to closely resemble that measured by PET or dynamic susceptibility contrast (DSC) and has high test-retest stability (17, 18). Over time, ASL MRI has been increasingly used in neuropsychiatric studies (19, 20) including drug addiction (9). However, the technique has rarely been used to study heroin addiction.
The purpose of this work was to examine baseline CBF alterations in heroin addiction using ASL perfusion MRI as well as associations of ASL-derived CBF with functional impairments observed in HDIs. Because functional deficits in the prefrontal cortex and the limbic dopamine systems are common in heroin addiction, we hypothesized that HDIs have reduced CBF in those regions, which would be correlated with the behavioral measures of their functional impairments.
Materials and Methods
Participant Characteristics
All human studies were approved by the local Institutional Review Board (IRB) of the Second Xiang-Ya Hospital of Central South University. All subjects provided signed written consent forms before participating in any experiments. A total of 72 unmedicated HDIs and 52 demographically matched healthy controls (HCs) were recruited. HDIs were recruited from drug rehabilitation centers in Changsha, Zhuzhou, and Yue Yang (three different cities in Hunan Province), following a positive urine test for heroin, the HDIs underwent compulsory abstinence for no more than 6 months. The HDIs were not taking medications before or during the current study. In total, four HDIs and five HCs were excluded due to poor MR image quality caused by severe head motion and image artifacts. All participants were right-handed Han people. Most participants were smokers, and some also reported alcohol use. The inclusion criteria for the heroin group were as follows: (1) a positive urine test for heroin; (2) a diagnosis of dependence based on criteria outlined in the fourth edition of the Diagnostic and Statistical Manual of Mental Disorders (DSM-IV); (3) a negative urine test for methamphetamine and ketamine; (4) no history of structural brain disease, epilepsy, or head trauma; (5) no contraindications to MRI; and (6) no history of mental or psychiatric illness.
Demographically matched control subjects were recruited through WeChat, flyers, etc., and subjects had to meet the aforementioned inclusion criteria (4), (5), and (6) and have a negative urine test for heroin, methamphetamine, and ketamine. Table 1 provides the demographic data of the enrolled HDIs and HCs.
Acquisition/Analysis of ASL Data
MRI data acquisitions were conducted in a 3T MRI scanner (MAGNETOM Skyra, Siemens) equipped with a 32-channel receiver coil. Foam padding and a forehead restraining strap were utilized to limit head movement during the scanning procedure. To obtain high-quality ASL data, participants were instructed to remain still with their eyes open during the MRI scan. All participants were allowed a moment to relax and move their hands/feet prior to scanning to ensure the quality of MR images.
T1-weighted high-resolution MRI was acquired using a three-dimensional fast gradient echo sequence with the following parameters: repetition time (TR) = 1450 ms, echo time (TE) = 2.0 ms, inversion time (TI) = 900 ms, field-of-view (FOV) = 256 × 256 mm, slice thickness = 1 mm, and flip angle = 12°. A 5-min 3D ASL scan was performed using a pulsed-ASL sequence from a Siemens device with the following parameters: TR/TE/TI1/TI2 = 2290/16/700/1990 ms, and voxel size = 4 × 4 × 5 mm3. Background suppression was applied.
ASL images were preprocessed by ASLtbx (15) using the following steps: motion correction (MoCo) (20, 21), temporal denoising, spatial smoothing, CBF quantification, outlier cleaning, partial volume correction (PVC), spatial registration to the Montreal Neurology Institute (MNI) standard brain space, and CBF extraction for regions of interest (ROIs). Temporal filtering used a high-pass Butterworth filter (cutoff frequency = 0.01 Hz) and temporal nuisance cleaning. Temporal nuisance variables, including the time courses of head motion (three translations and three rotations), the global signal, and the mean CSF signal, were regressed out from the ASL image series at each voxel. The CSF mask was defined during the T1-weighted structural image segmentation. Spatial smoothing was performed with an isotropic Gaussian kernel with a full-width-at-half-maximum (FWHM) of 4 mm. The preprocessed ASL label and control image pairs were then successively subtracted to obtain the perfusion map. The detailed model parameters can be found in previous publications (15, 16). The mean ASL image was registered to the high-resolution structural image. Structural images were segmented into gray matter (GM), white matter (WM), and cerebrospinal fluid (CSF) using the segmentation tool provided in Statistical Parametric Mapping (https://www.fil.ion.ucl.ac.uk/spm/software/spm12/), projected into the ASL image space based on the registration transform between the mean ASL control image and the structural image and subsequently used to extract the CBF signals for temporal denoising and PVC. The relative CBF (rCBF) map (22, 23) was calculated by dividing the perfusion maps by the global mean perfusion value from the GM and WM.
Voxel-wise CBF differences between the two groups were computed using two-sample t-tests, the result was showed in Figure 1, age, gender, education, smoking, and drinking as covariates. Differences in the rCBF from group comparisons were corrected for multiple comparisons to a significance level of p < 0.05 by false discovery rate (FDR) correction. The ROIs from whole-brain voxel-wise comparisons (Figure 1) mainly include nine cerebral regions according to the automated anatomical labeling (AAL) template: the bilateral middle frontal gyrus (MFG), inferior temporal gyrus (ITG), and precuneus (PCUN); the right posterior cingulate gyrus (PCG), calcarine area (CAL), and thalamus. Then, nine sub-ROIs were generated from the intersection of voxel-wise ROIs and each regional AAL template, and each sub-ROI of rCBF values was calculated for the two groups. The rCBF values extracted by each sub-ROI between the two groups were compared using two-sample t-tests (Table 3) and evaluated for possible associations with neuropsychological characteristics.
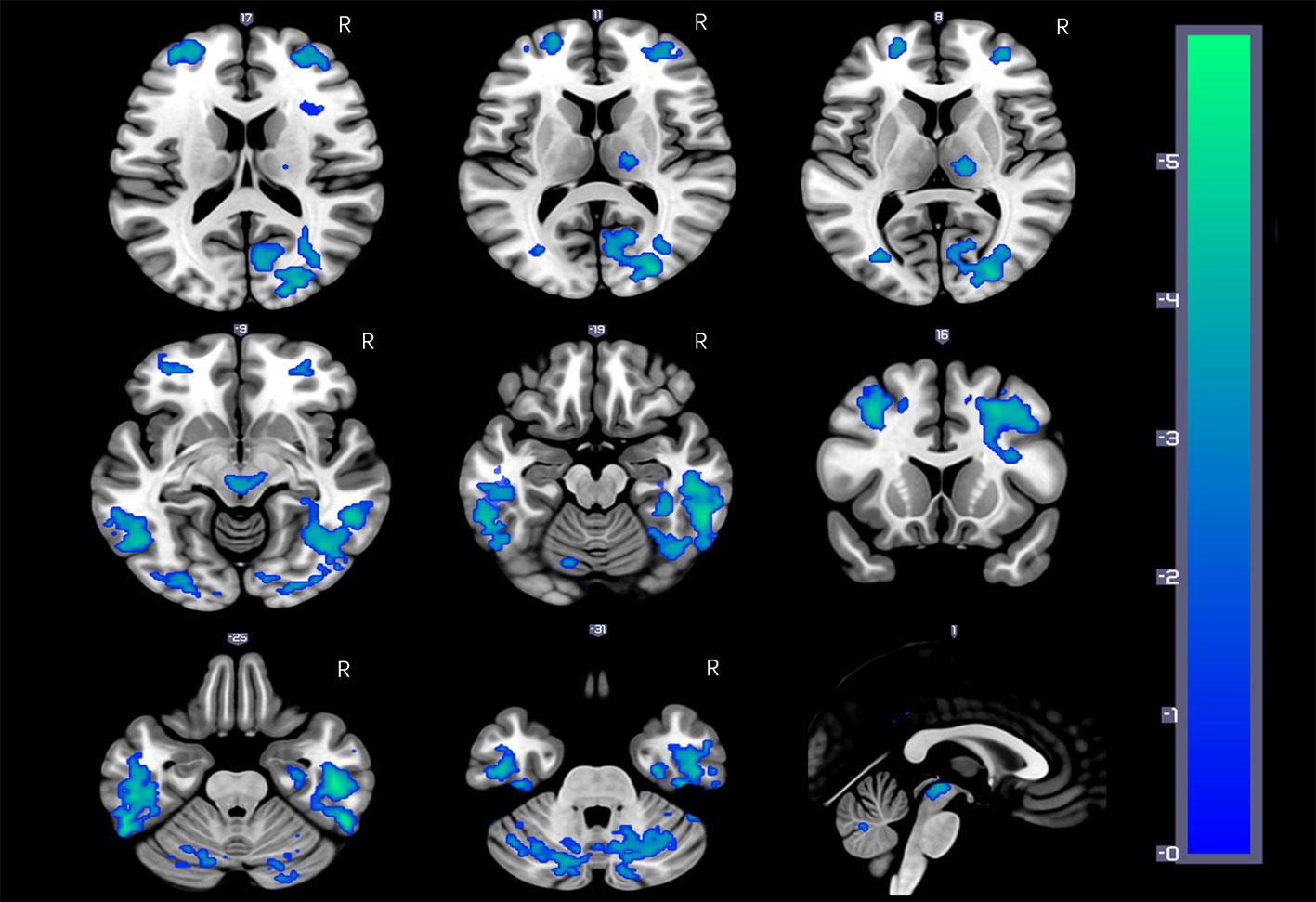
Figure 1 HDIs show significantly reduced rCBF in the bilateral inferior temporal gyrus (ITG), middle frontal gyrus (MFG), cerebellar vermis, and posterior cerebellar lobe, precuneus; the right posterior cingulate gyrus (PCG) and thalamus; and the midbrain adjacent to the ventral tegmental area (VTA) (FDR correction, q = 0.05, p = 0.00158, t = 3.012).
Clinical Characteristics and Neurocognitive Measures
The duration of heroin use, the dose consumed, and other clinical characteristics was also collected from the HDIs. Cognitive function tests, including the Wechsler Adult Intelligence Scale Third Edition (WAIS-3) Digit Symbol Test (including the first and second minutes), forward and backward digit memory span tasks, and Trail Making Test (TMT) were completed by all patients following MRI examination. The Digit Symbol Test score is a part of the WAIS and an indicator of the executive function of the frontal lobe based on visual, spatial and motor processing speed. Participants were asked to write as many numbers as possible that were paired with symbols within 2 min (24–26). The forward and backward digit memory span tasks have been widely used for simple clinical measurements of working memory. The digit span task required the subjects to remember the numbers on an answer sheet in both forward and backward order. This test involves working memory, which can reflect particular aspects of the cognitive function of the brain (27–29). The TMT (30) required the subjects to connect scattered numbers in the correct sequence on a sheet of paper quickly and accurately. They could not skip numbers. The TMT was used to evaluate psychomotor performance (31).
Statistical Analysis
Statistical analyses were performed using SPSS 18.0. The HDI and HC groups were compared using a two-sample t-test for age, the chi-square test for smoking and drinking and rank sum tests for education levels, and the significance level was set to p < 0.05. The data distributions were tested for normality using the Kolmogorov-Smirnov test. Normally distributed data were analyzed using independent-samples t-tests, while non-normally distributed data were analyzed using rank sum tests.
Voxel-wise CBF differences between the two groups were computed using two-sample t-tests. Differences in rCBF from group comparisons were corrected for multiple comparisons to a significance level of p < 0.05 by FDR correction [q = 0.05, p = 0.00158, t (113) = −3.012] and a cluster size threshold of 100. Correlations between rCBF levels and clinical characteristics were evaluated using Pearson’s correlation coefficients. The level of statistical significance was set at p < 0.05.
Results
ASL Results
Figure 1 and Table 2 show the whole-brain cross-sectional rCBF comparison results. The statistical threshold of p = 0.00158 was corrected for multiple comparisons with False FDR correction. Compared to HCs, HDIs showed significant rCBF decreases in the bilateral cortical and subcortical ITG, MFG, cerebellar vermis, posterior cerebellar lobe, and PCUN; the right PCG and thalamus; and the midbrain adjacent to the ventral tegmental area (VTA) (Figure 1, Table 2). Many significant differences were shown in the rCBF values extracted from overlapping ROI masks, and 3 rCBF values were excluded due to negative values (1 in the bilateral PCUN and 1 in the right thalamus) (Table 3). No increased regional rCBF areas were detected in HDIs compared with the control group (FDR correction).
Neuropsychological Tests
Compared to HCs, HDIs showed significant differences in the WAIS-R Digit Symbol Test 2nd-minute scores [t (73) = −2.663, p = 0.011], backward digit memory span [t (70) = −2.557, p = 0.013], and TMT times [t (76) = 3.556, p = 0.001]. No significant differences were observed between forward digit memory span [t (70) = −1.730, p = 0.086] and Digit Symbol Test 1st-minute scores [t (73) = −1.689, p = 0.095].
Correlations Between CBF and Neuropsychological Test Performance
Data from the neuropsychological tests completed by 36 patients and 42 HCs were used to assess the significance of correlations between test performance and rCBF. rCBF in the left MFG and right MFG was negatively correlated with the TMT times (left: r = −0.413, p < 0.001; right: r = −0.466, p < 0.001) (Figures 2 and 3). No significant correlation was observed between rCBF in the bilateral MFG/ITG and the other neuropsychological test results.
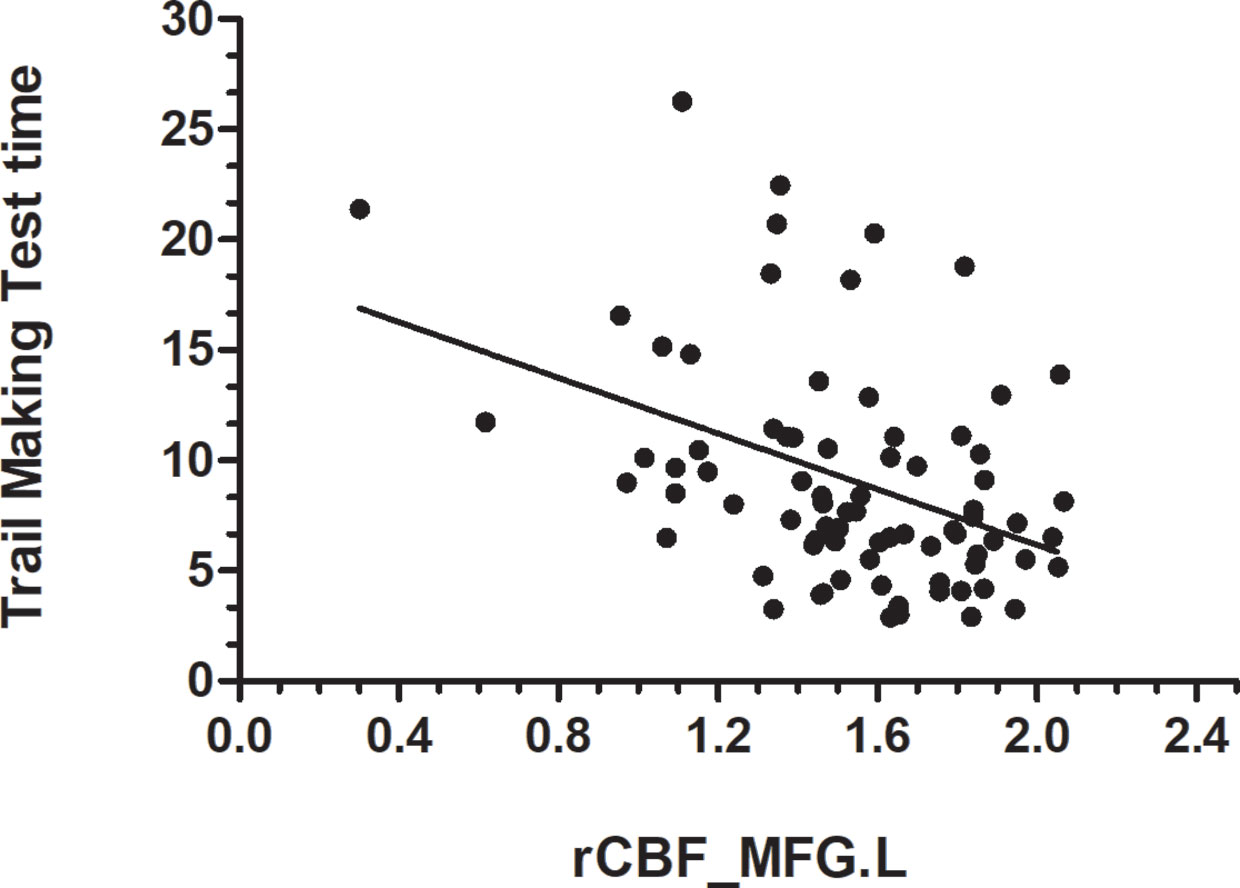
Figure 2 rCBF value in MFG.L was negatively correlated with Trail Making Test completion time (s) (r = −0.413, p < 0.001; N=36 HDIs + 42 HCs); MFG. L, left middle frontal gyrus.
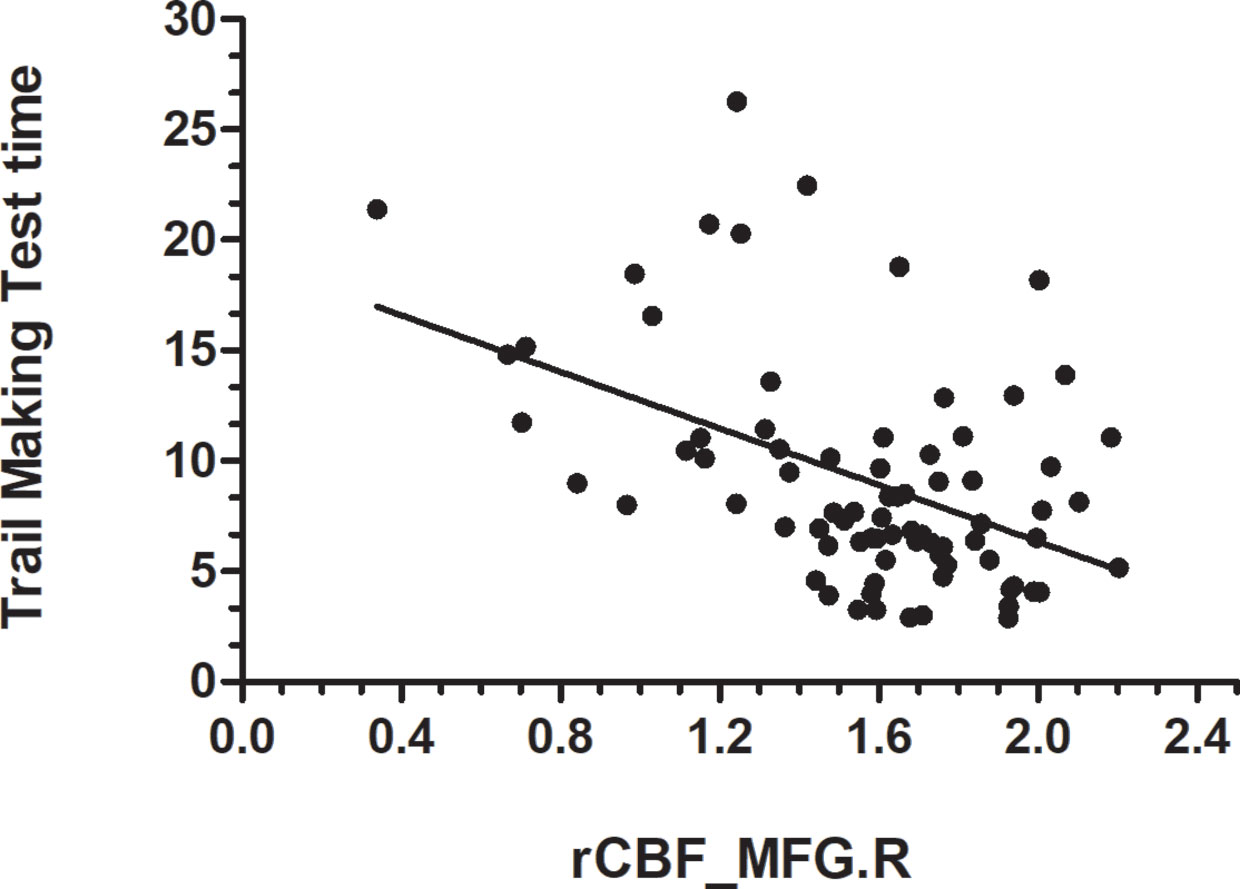
Figure 3 rCBF value in MFG.R was negatively correlatedwith Trail Making Test Time completion time (r = −0.466, p < 0.001; N=36 HDIs + 42 HCs); MFG.R, right middle frontal gyrus.
Discussion
Using ASL perfusion MRI, we identified hypoperfusion in frontal, temporal, and parietal areas in HDIs, which was correlated with neuropsychological impairments in the patients.
Hypoperfusion in HDIs has been reported in several studies. Denier et al. (9) found hypoperfusion in frontal and temporal areas following acute administration of heroin to HDIs. Using SPECT, Gilberto et al. (7) demonstrated reduced CBF in the right frontal and left temporal lobes in opioid-dependent subjects with comorbid depression. Lukas et al. (8) investigated opioid-dependent subjects with SPECT and reported decreased CBF in the bilateral cortical prefrontal lobe, right thalamus and hippocampus in opioid-dependent patients. While our hypoperfusion findings in HDIs were partially consistent with those of previous studies, we used ASL MRI, which is non-invasive and does not require radioactive materials. Another merit of our study was the relatively large sample size.
Mesolimbic system dysfunction is a hallmark change in drug addiction (32, 33), including heroin addiction (34–36). By binding to the µ-opioid receptors on GABA neurons in the midbrain, heroin suppresses the inhibitory effects of GABA on dopamine neurons, which subsequently promotes the release of dopamine, leading to elevated reward effects (37). Reduced rCBF in mesolimbic areas in HDIs may be a result of that hallmark change and is consistent with various dysfunctions (such as increased impulsivity and altered reward processing) involving those regions as identified by previous neuroimaging and neurobiological studies (33).
Frontal brain alterations represent a second hallmark symptom of drug addiction, which is often characterized by a loss of executive function, especially inhibition (38). Our frontal hypoperfusion findings in HDIs align with that symptom, which also establishes ASL MRI as a useful tool for measuring such frontal brain dysfunction in drug addiction. The clinical relevance of the frontal hypoperfusion patterns was further demonstrated by the rCBF vs TMT correlations. The TMT reflects the capabilities of mental flexibility, working memory, and executive function (38, 39). The negative frontal rCBF vs TMT processing time in HDIs indicates that patients with lower rCBF in the frontal cortex have more-impaired executive functions.
We found significantly reduced rCBF in the precuneus and ITG in HDIs. The precuneus and ITG are part of the default mode network (DMN). Hypoperfusion in the ITG and precuneus might be a result of altered facilitation function of the DMN, which has been shown to be affected by many neuropsychiatric diseases (40, 41). Reduced rCBF in the precuneus is consistent with the reduced structural and functional connectivity of the precuneus in HDIs, as reported in (42–44), and may reflect functional alterations regarding memory consolidation and information processing, especially in relation to drug cues (45–47). The ITG plays an important role in the connection of visual, linguistic and multisensory processing (47, 48). However, the role of the ITG in heroin addiction remains unclear. Our finding of reduced ITG rCBF is consistent with the hypoperfusion in the bilateral temporal gyrus as measured with SPECT (single photon emission computed tomography) (49).
Decreased CBF was detected in the cerebellar vermis and cerebellar posterior lobe. Studies have shown that the vermis is involved in the regulation of both emotional and cognitive processes (49–52). Thus, further research is needed to clarify the relationship between alterations in CBF and cognitive function in HDIs.
Using ASL, we demonstrated that frontal cognitive impairment is a major element of dysfunction in abstinent HDIs. The limitation of this study is that it had a cross-sectional design. Further studies are required to confirm the relationship between alterations in brain CBF and impaired cognitive function after abstinence.
Conclusions
In summary, using a relatively large sample, we identified hypoperfusion patterns in mesolimbic, frontal, parietal, and temporal regions in HDIs. Hypoperfusion in frontal brain regions might be a primary marker for heroin addiction as supported by the cross-sectional differences and correlations with cognitive impairment identified.
Data Availability Statement
All datasets generated for this study are included in the article/supplementary material.
Ethics Statement
The studies involving human participants were reviewed and approved by Institutional Review Board of the Second Xiang-Ya Hospital of Central South University. The patients/participants provided their written informed consent to participate in this study. Written informed consent was obtained from the individual(s) for the publication of any potentially identifiable images or data included in this article.
Author Contributions
WY, JiL, JZ, and JuL conceptualized and designed the study. WY, JiL, FT, and LF conducted the behavioral and imaging analyses. RY, CC, CD, and YD conducted the assessments. JuL modified the manuscript and supervised the study. WY wrote the first draft and all authors provided input on the final version of the manuscript.
Funding
The National Key Research and Development Program of China (Grant number: 2016YFC0800908) and the National Natural Science Foundation of China (U1052225). National Natural Science Foundation of China, Grant Number: 61971451; Innovative Province special construction foundation of Hunan Province, China, Grant Number: 2019SK2131.
Conflict of Interest
The authors declare that the research was conducted in the absence of any commercial or financial relationships that could be construed as a potential conflict of interest.
Acknowledgments
This work was funded by the National Key Research and Development Program of China (Grant number: 2016YFC0800908) and the National Natural Science Foundation of China (U1052225). The authors express their appreciation to their patients and volunteers for participating in this study.
References
1. Benyamin R, Trescot AM, Datta S, Buenaventura R, Adlaka R, Sehgal N, et al. Opioid complications and side effects. Pain Physcian (2008) 11:S105–20.
2. Wang W. Illegal drug abuse and the community camp strategy in China. J Drug Educ (1999) 29:97–114. doi: 10.2190/J28R-FH8R-68A9-L288
3. Tang YL, Zhao D, Zhao C, Cubells JF. Opiate addiction in China: current situation and treatments. Addiction (2006) 101:657–65. doi: 10.1111/j.1360-0443.2006.01367.x
4. Wang Z, Suh J, Duan D, Darnley S, Jing Y, Zhang J, et al. A hypo-status in drug-dependent brain revealed by multi-modal MRI. Addict Biol (2017) 22:1622–31. doi: 10.1111/adb.12459
5. Detre JA, Wang J, Wang Z, Rao H. Arterial spin-labeled perfusion MRI in basic and clinical neuroscience. Curr Opin Neurol (2009) 22:348–55. doi: 10.1097/WCO.0b013e32832d9505
6. Adinoff B, Gu H, Merrick C, McHugh M, Jeon-Slaughter H, Lu H, et al. Basal Hippocampal Activity and Its Functional Connectivity Predicts Cocaine Relapse. Biol Psychiatry (2015) 78:496–504. doi: 10.1016/j.biopsych.2014.12.027
7. Gerra G, Calbiani B, Zaimovic A, Sartori R, Ugolotti G, Ippolito L, et al. Regional cerebral blood flow and comorbid diagnosis in abstinent opioid addicts. Psychiatry Res (1998) 83:117–26. doi: 10.1016/S0925-4927(98)00030-4
8. Pezawas L, Fischer G, Podreka I, Schindler S, Brücke T, Jagsch R, et al. Opioid addiction changes cerebral blood flow symmetry. Neuropsychobiology (2002) 45:67–73. doi: 10.1159/000048679
9. Denier N, Schmidt A, Gerber H, Schmid O, Riecher-Rössler A, Wiesbeck GA, et al. Association of frontal gray matter volume and cerebral perfusion in heroin addiction: a multimodal neuroimaging study. Front Psychiatry (2013) 4:135. doi: 10.3389/fpsyt.2013.00135
10. Alsop DC, Detre J, Golay X. Recommended implementation of arterial spin-labeled perfusion MRI for clinical applications: A consensus of the ISMRM perfusion study group and the European consortium for ASL in dementia. Magn Reson Med (2015) 73:102–16. doi: 10.1002/mrm.25607
11. Wang Z, Faith M, Patterson F, Tang K, Kerrin K, Wileyto EP, et al. Neural substrates of abstinence-induced cigarette cravings in chronic smokers. J Neurosci (2007) 27:14035–40. doi: 10.1523/JNEUROSCI.2966-07.2007
12. Franklin TR, Wang Z, Wang J, Sciortino N, Harper D, Li Y, et al. Limbic activation to cigarette smoking cues independent of nicotine withdrawal: a perfusion fMRI study. Neuropsychopharmacol (2007) 32:2301–9. doi: 10.1038/sj.npp.1301371
13. Chen Y, Wang DJ, Detre JA. Test-retest reliability of arterial spin labeling with common labeling strategies. J mMagn Reson Imaging (2011) 33:940–9. doi: 10.1002/jmri.22345
14. Buxton RB, Frank LR, Wong EC, Siewert B, Warach S, Edelman RR. A general kinetic model for quantitative perfusion imaging with arterial spin labeling. Magn Reson Med (1998) 40:383–96. doi: 10.1002/mrm.1910400308
15. Wang Z, Aguirre GK, Rao H, Wang J, Fernández-Seara MA, Childress AR, et al. Empirical optimization of ASL data analysis using an ASL data processing toolbox: ASLtbx. Magn Reson Imaging (2008) 26:261–9. doi: 10.1016/j.mri.2007.07.003
16. Cavusoglu M, Pfeuffer J, Ugurbil K, Uludag K. Comparison of pulsed arterial spin labeling encoding schemes and absolute perfusion quantification. Magn Reson Imaging (2009) 27:1039–45. doi: 10.1016/j.mri.2009.04.002
17. Qiao PG, Han C, Zuo ZW, Wang YT, Pfeuffer J, Duan L, et al. Clinical assessment of cerebral hemodynamics in Moyamoya disease via multiple inversion time arterial spin labeling and dynamic susceptibility contrast-magnetic resonance imaging: A comparative study. J Neuroradiol (2017) 44:273–80. doi: 10.1016/j.neurad.2016.12.006
18. Detre JA, Rao H, Wang DJ, Chen YF, Wang Z. Applications of arterial spin labeled MRI in the brain. J Magn Reson Imaging (2012) 35:1026–37. doi: 10.1002/jmri.23581
19. Delgado AF, De Luca F, Hanagandi P, van Westen D, Delgado AF. Arterial Spin-Labeling in Children with Brain Tumor: A Meta-Analysis. AJNR Am J Neuroradiol (2018) 39:1536–42. doi: 10.3174/ajnr.A5727
20. Schneider K, Michels L, Hartmann-Riemer MN, Burrer A, Tobler PN, Stampfli P, et al. Cerebral blood flow in striatal regions is associated with apathy in patients with schizophrenia. J Psychiatry Neurosci (2019) 44:102–10. doi: 10.1503/jpn.170150
21. Wang Z. Improving cerebral blood flow quantification for arterial spin labeled perfusion MRI by removing residual motion artifacts and global signal fluctuations. Magn Reson Imaging (2012) 30:1409–15. doi: 10.1016/j.mri.2012.05.004
22. Tsien C, Galban CJ, Chenevert TL, Johnson TD, Hamstra DA, Sundgren PC, et al. Parametric response map as an imaging biomarker to distinguish progression from pseudoprogression in high-grade glioma. J Clin Oncol (2010) 28:2293–9. doi: 10.1200/JCO.2009.25.3971
23. Galban CJ, Chenevert TL, Meyer CR, Tsien C, Lawrence TS, Hamstra DA, et al. The parametric response map is an imaging biomarker for early cancer treatment outcome. Nat Med (2009) 15:572–6. doi: 10.1038/nm.1919
24. Loprinzi PD. Epidemiological investigation of muscle-strengthening activities and cognitive function among older adults. Chronic Illn (2016) 12:157–62. doi: 10.1177/1742395316641998
25. Nakahachi T, Ishii R, Iwase M, Canuet L, Takahashi H, Kurimoto R, et al. Frontal activity during the digit symbol substitution test determined by multichannel near-infrared spectroscopy. Neuropsychobiology (2008) 57:151–8. doi: 10.1159/000147467
26. Frith E, Loprinzi PD. Physical Activity and Cognitive Function Among Older Adults with an Elevated Gamma Gap. Med Princ Pract (2018) 27:531–6. doi: 10.5455/JCBPR.284072
27. Jensen AR, Figueroa RA. Forward and backward digit span interaction with race and IQ: predictions from Jensen’s theory. J Educ Psychol (1975) 67:882–93. doi: 10.1037/0022-0663.67.6.882
28. Hester RL, Kinsella GJ, Ong B. Effect of age on forward and backward span tasks. J Int Neuropsychol Soc (2004) 10:475–81. doi: 10.1017/S1355617704104037
29. Wechsler D. Technical manual for the Wechsler Adult Intelligence and Memory Scale. 3rd ed. New York: The Psychological Corporation. (1997).
30. Wilckens KA, Tudorascu DL, Snitz BE, Price JC, Aizenstein HJ, Lopez OL, et al. Sleep moderates the relationship between amyloid beta and memory recall. Neurobiol Aging (2018) 71:142–8. doi: 10.1016/j.neurobiolaging.2018.07.011
31. Yu Y, Zhao Y, Si Y, Ren Q, Ren W, Jing C, et al. Estimation of the cool executive function using frontal electroencephalogram signals in first-episode schizophrenia patients. BioMed Eng Online (2016) 15:131. doi: 10.1186/s12938-016-0282-y
32. Koob GFAV. Neurocircuitry of addiction. Neuropsychopharmacology (2013) 35:217–38. doi: 10.1016/B978-0-444-62619-6.00003-3
33. Schluter RS, Daams JG, van Holst RJ, Goudriaan AE. Effects of Non-invasive Neuromodulation on Executive and Other Cognitive Functions in Addictive Disorders: A Systematic Review. Front Neurosci (2018) 12:642. doi: 10.3389/fnins.2018.00642
34. Karila L, Marillier M, Chaumette B, Billieux J, Nicolas F, Amine B. New synthetic opioids: Part of a new addiction landscape. Neurosci Biobehav Rev (2018) 106:133–40. doi: 10.1016/j.neubiorev.2018.06.010
35. Valentino RJ, Volkow N. Untangling the complexity of opioid receptor function. Neuropsychopharmacol (2018) 43:2514–20. doi: 10.1038/s41386-018-0225-3
36. Jay GW, Barkin RL. Perspectives on the opioid crisis from pain medicine clinicians. Dis Mon (2018) 64:451–66. doi: 10.1016/j.disamonth.2018.07.002
37. Darcq E, Kieffer BL. Opioid receptors: drivers to addiction? Nat Rev Neurosci (2018) 19:499–514. doi: 10.1038/s41583-018-0028-x
38. Ryu K, Kim Y, Kwon M, Kim H, Kim J. The frontal executive function in exercise addicts, moderate exercisers, and exercise avoiders. Am J Addict (2016) 25:466–71. doi: 10.1111/ajad.12422
39. Varjacic A, Mantini D, Demeyere N, Gillebert CR. Neural signatures of Trail Making Test performance: Evidence from lesion-mapping and neuroimaging studies. Neuropsychologia (2018) 115:78–87. doi: 10.1016/j.neuropsychologia.2018.03.031
40. Hu ML, Zong XF, Mann JJ, Zheng JJ, Liao YH, Li ZC, et al. A Review of the Functional and Anatomical Default Mode Network in Schizophrenia. Neurosci Bull (2017) 33:73–84. doi: 10.1007/s12264-016-0090-1
41. Malhi GS, Das P, Outhred T, Bryant RA, Calhoun V, Mann JJ. Default mode dysfunction underpins suicidal activity in mood disorders. Psychol Med (2019) 50:1–10. doi: 10.1017/S0033291719001132
42. Yuan K, Qin W, Dong M, Liu J, Liu P, Zhang Y, et al. Combining spatial and temporal information to explore resting-state networks changes in abstinent heroin-dependent individuals. Neurosci Lett (2010) 475:20–4. doi: 10.1016/j.neulet.2010.03.033
43. Li Q, Li Z, Li W, Zhang Y, Wang Y, Zhu J, et al. Disrupted Default Mode Network and Basal Craving in Male Heroin-Dependent Individuals: A Resting-State fMRI Study. J Clin Psychiatry (2016) 77:e1211–7. doi: 10.4088/JCP.15m09965
44. Ma X, Qiu Y, Tian J, Wang J, Li S, Zhan W, et al. Aberrant default-mode functional and structural connectivity in heroin-dependent individuals. PloS One (2015) 10:e120861. doi: 10.1371/journal.pone.0120861
45. Rugg MD, Vilberg KL. Brain networks underlying episodic memory retrieval. Curr Opin Neurobiol (2013) 23:255–60. doi: 10.1016/j.conb.2012.11.005
46. Chen J, Honey CJ, Simony E, Arcaro MJ, Norman KA, Hasson U. Accessing Real-Life Episodic Information from Minutes versus Hours Earlier Modulates Hippocampal and High-Order Cortical Dynamics. Cereb Cortex (New York N Y 1991) (2016) 26:3428–41. doi: 10.1093/cercor/bhv155
47. Engelmann JM, Versace F, Robinson JD, Minnix JA, Lam CY, Cui Y, et al. Neural substrates of smoking cue reactivity: a meta-analysis of fMRI studies. Neuroimage (2012) 60:252–62. doi: 10.1016/j.neuroimage.2011.12.024
48. Onitsuka T, Shenton ME, Salisbury DF, Dickey CC, Kasai K, Toner SK, et al. Middle and inferior temporal gyrus gray matter volume abnormalities in chronic schizophrenia: an MRI study. Am J Psychiatry (2004) 161:1603–11. doi: 10.1176/appi.ajp.161.9.1603
49. Botelho MF, Relvas JS, Abrantes M, Cunha MJ, Marques TR, Rovira E, et al. Brain blood flow SPET imaging in heroin abusers. Ann N Y Acad Sci (2006) 1074:466–77. doi: 10.1196/annals.1369.047
50. Schmahmann JD. Disorders of the cerebellum: ataxia, dysmetria of thought, and the cerebellar cognitive affective syndrome. J Neuropsychiatr Clin Neurosci (2004) 16:367–78. doi: 10.1176/jnp.16.3.367
51. Schmahmann JD. The cerebellum and cognition. Neurosci Lett (2018) 30:1409–15. doi: 10.1016/j.neulet.2018.07.005
Keywords: heroin addiction, magnetic resonance perfusion imaging, arterial spin labeling, neurocognitive, reward circuits
Citation: Yang W, Yang R, Tang F, Luo J, Zhang J, Chen C, Duan C, Deng Y, Fan L and Liu J (2020) Decreased Relative Cerebral Blood Flow in Unmedicated Heroin-Dependent Individuals. Front. Psychiatry 11:643. doi: 10.3389/fpsyt.2020.00643
Received: 20 December 2019; Accepted: 19 June 2020;
Published: 14 July 2020.
Edited by:
Maorong Hu, Nanchang University, ChinaCopyright © 2020 Yang, Yang, Tang, Luo, Zhang, Chen, Duan, Deng, Fan and Liu. This is an open-access article distributed under the terms of the Creative Commons Attribution License (CC BY). The use, distribution or reproduction in other forums is permitted, provided the original author(s) and the copyright owner(s) are credited and that the original publication in this journal is cited, in accordance with accepted academic practice. No use, distribution or reproduction is permitted which does not comply with these terms.
*Correspondence: Jun Liu, junliu123@csu.edu.cn