- 1Department of General Surgery, Nanjing Drum Tower Hospital, Nanjing, China
- 2Medical School of Nanjing University, Nanjing, China
Suicide is the most severe consequence of depression which has become a leading cause of disability and a global disease burden. Recent evidence indicates a central role of small molecules in the pathogenesis of depression and associated suicidal behaviors. However, there lacks a systemic exploration of small molecules in the development of depression-associated suicide, and it remains unclear how they affect an individual’s behavior. In order to compare the metabonomic profiles between drug-naïve patients with depression-associated suicidal behaviors and healthy individuals, we conducted a systemic database search for studies of metabolic characteristics in depression-associated suicidal behavior. Manual data curation and statistical analysis and integration were performed in Excel. We further performed an enrichment analysis of signaling pathway prediction using the Reactome Pathway Analysis tool. We have identified 17 metabolites that expressed differently between drug-naïve patients with depression-associated suicidal behaviors and healthy controls. We have integrated these metabolites into biological signaling pathways and provided a visualized signaling network in depressed suicidal patients. We have revealed that “transport of small molecules”, “disease”, “metabolism” and “metabolism of proteins” were the most relevant signaling sections, among which “transport of inorganic cations/anions and amino acids/oligopeptides”, “SLC-mediated transmembrane transport”, and “metabolism of amino acids and derivatives” should be further studied to elucidate their potential pathogenic mechanism in the development of depression and associated suicidal behavior. In conclusion, our findings of these 17 metabolites and associated signaling pathways could provide an insight into the molecular pathogenesis of depression-associated suicidal behavior and potential targets for new drug inventions.
Introduction
Depression is a common mental disease that affects approximately 350 million individuals globally (1). The incidence varies between continents, among which Asia ranks second worldwide with a pooled incidence of 13.5~20.4% in the recent two decades (2). Depression has become one of leading causes of disability and global disease burdens (3).
Suicide is the most severe consequence of depression (4), of which the incidence is estimated around 2~9% among depressed patients (5). However, some consider the number to be far underestimated because suicidal ideation, plans or attempts have not been fully included (6). Notably, the prevalence of depression in physicians (7, 8) and the risk of depression-associated suicide in physicians (9–11) are considerably higher than those in the ordinary population.
A series of studies have suggested a crosstalk between inflammation-associated signaling pathways and the neuroimmune system in the brain that drives the development of depression and behaviors such as suicide (12, 13). Disruption in peripheral and central immune systems appears to contribute to a vulnerability to mental disorders (14).
Emerging evidence strongly indicates a key role of small molecules, especially metabolites, in the pathogenesis of depression (15–18). However, it remains unclear how small molecules act on the brain and affect an individual’s behavior, which is essential for improving the sensitivity of current antidepressant drugs and exploring the diagnostic and therapeutic potential of targeting small molecules for future management of depression.
The utilization of metabonomic approaches can provide high-throughput data that enables simultaneous measurement of numerous small molecules in one specific sample. Considerable studies using the metabonomic technique have been performed to find differentially expressed small molecules between depressed patients versus healthy population, drug-naïve versus drug-treated depressed patients, and drug-sensitive versus drug-resistant depressed patients.
Nevertheless, there appears to be a lack of a systemic summary of significant metabolites in depression, especially in depression-associated suicidal behavior. In the paper, we aim to systemically summarize current evidences of metabolic characterization in depression-associated suicidal behavior, including the name of metabolite, source of tissue type and expression pattern of metabolites. Furthermore, we sought to perform an enrichment analysis to integrate all significant metabolic molecules into biological signaling pathways. We expect that the identification of metabolites and the prediction of signaling pathways can help to elucidate the role of small molecules in the development of depression and associated suicide.
Methods
Literature Search Strategy
We conducted a systemic literature search of studies for metabolic characteristics in depression-associated suicidal behavior within the following databases: PubMed/Medline, Embase, Cochrane Library and regional Chinese databases including CNKI, VIP and Wanfang. For instance, the search strategy in PubMed was as follows: ((((((((depression[MeSH Terms]) AND suicide[MeSH Terms]) AND small molecular libraries[MeSH Terms]) AND brain disease, metabolic[MeSH Terms]) AND brain disorder, metabolic[MeSH Terms]) AND depress*[Title/Abstract]) AND suicid*[Title/Abstract]) AND metabo*[Title/Abstract]) AND molecu*[Title/Abstract].
The inclusion criteria were (1) clinical studies involving human samples, (2) studies comparing metabolites between drug-naïve depressed suicide attempters and healthy controls. We accepted studies that utilized nuclear magnetic resonance (NMR), magnetic resonance spectroscopy (MRS), gas chromatography-mass spectrometry (GC-MS), liquid chromatography-mass spectrometry (LC-MS) and other NMR- or MS- based metabolomics technologies.
The exclusion criteria were (1) non-human studies such as animal, primary cell or cell line experiment, (2) other detection technology than those described above, (3) other types of reports such as editorial, case report, commentary, review and study protocol, (4) other language studies than English or Chinese, (5) single-arm studies (non-comparative studies), absence of healthy individuals as control group, irrelevant epidemiology or duplicate studies.
Data Extraction
The following data were manually extracted from the original publication: name of metabolite, source of tissue type (including both peripheral and central system) and change of expression (either up- or down- regulated).
Distribution of tissue type was generated and presented as a pie chart by Excel (Microsoft, ver. 2016). Manual data curation, statistical analysis and integration of the metabolite map were performed in Excel as well. All significant metabolites were shown in this map as white circles and were connected with their relevant tissue type, shown as black circles. The weight of lines was positively correlated with the number of supporting references. The size of the black circles was positively correlated with the frequency of having been studied in the literature.
Enrichment Analysis of Signaling Pathways
All significant metabolites were labelled using KEGG C-number and brought into the Reactome Pathway Analysis tool for enrichment of signaling pathways (19, 20). All non-human identifiers were converted to the human equivalents.
Ethics
As this was a study of literature review and analysis, patient content was not required by the Ethics Committee at Nanjing Drum Tower Hospital.
Results
After literature screening and eligibility identification, a total of 31 original studies were eventually enrolled into data analysis (21–51) (Table 1). Overall, 17 metabolites were found differentially expressed between depressed suicide attempters and healthy controls. They are acetic acid, acetone, cholesterol, d-glucose, glycine, L-alanine, LDL (low-density lipoprotein), L-glutamine, L-lactic acid, L-valine, putrescine, pyruvic acid, quinolinic acid, spermidine, taurine, unsaturated lipid, and VLDL (very low-density lipoprotein).
We summarized the distribution of involved tissue types (Supplementary Figure 1). The majority of studies (88%) investigated peripheral tissues including plasma (46%), urine (38%), serum (3%) and peripheral blood mononuclear cells (1%). The remaining 12% studies measured metabolite expression in the central system including cerebrospinal fluid (5%), frontal cortex (3%), subcortical nuclei (1%), putamen (1%), pregenual anterior cingular cortex (1%) and ventrolateral prefrontal cortex (1%). The inaccessibility of central system tissues hampers the investigation towards metabolite expression in the central system.
L-alanine was differentially expressed in peripheral tissues between depression suicide attempters and controls according to 12 references, in which a controversial expression pattern was observed (4 references supported up-regulation, while 1 supported down-regulation in urine; 4 references supported up-regulation, while 3 supported down-regulation in plasma).
L-lactic acid was found in 11 references with different expression levels between diseased patients and healthy controls. In the central system, including cerebrospinal fluid and the pregenual anterior cingulate cortex, L-lactic acid was up-regulated in depressed suicide attempters. In the peripheral system, including urine and plasma, inconsistent expression patterns were found according to respective studies.
L-glutamine was differentially expressed in both peripheral and central tissues between diseases and controls according to 8 references that measured 2 peripheral and 3 central tissues. Similar to L-alanine, these 8 studies failed to reach a consistent result in the L-glutamine expression pattern. L-valine, glycine and taurine were found differentially expressed between depression suicide attempters and healthy controls according to 7, 6 and 6 studies, respectively. Notably, inconsistent expression pattern of these metabolites is observed between studies as well.
Multiple studies suggested discrepant expressions of quinolinic acid, acetic acid, acetone and VLDL between diseased patients and controls. However, similar to the above metabolites, their expression patterns were controversial between studies as well. The other metabolites were surveyed by very few references according to which only provisional conclusions could be drawn. We generated a metabolite map to present the expression pattern of all significant metabolites in associated tissues (Figure 1).
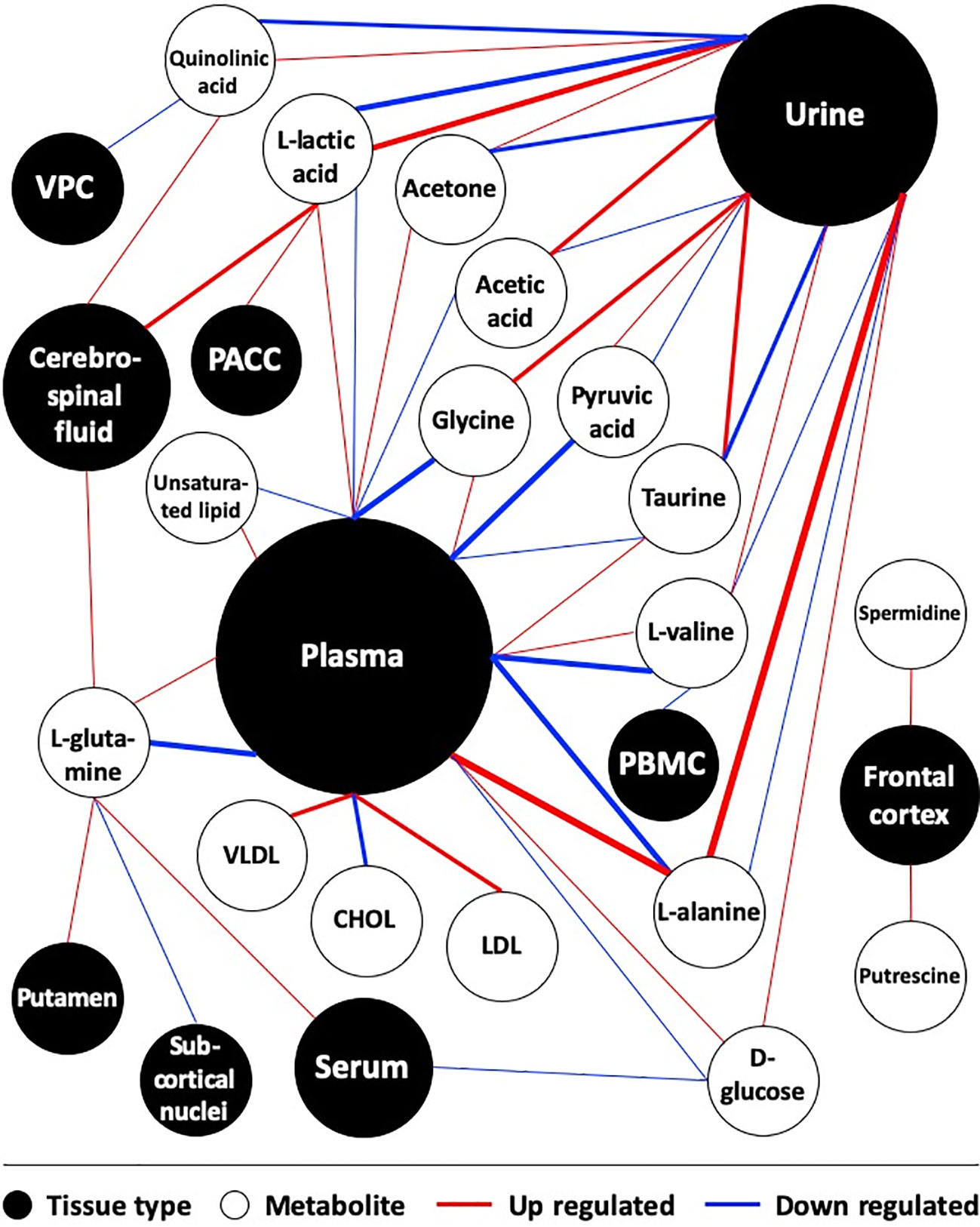
Figure 1 Map of metabolites in depression-associated suicide. Studies comparing metabolites between depressed suicide attempters and healthy controls were included. Weight of lines positively correlates with the number of supporting references. Size of circles positively correlates with the frequency of being studied in the literature. CHOL, Cholesterol; PACC, pregenual anterior cingular cortex; PBMC, peripheral blood mononuclear cell; VPC, Ventrolateral prefrontal cortex.
Next, we utilized the Reactome database to integrate all significant metabolic molecules into biological signaling pathways. Based on reactions being reported by the literature, all metabolites participating in reactions would be created as a network of biological interactions and integrated into signaling pathways.
Figure 2 provided a visualization of the signaling network of metabolites involved in depressed suicidal attempters. Table 2 illustrated the top 20 related signaling pathways, which was divided into 4 sections, including “transport of small molecules”, “disease”, “metabolism”, and “metabolism of proteins”. Among the 20 pathways, “transport of inorganic cations/anions and amino acids/oligopeptides” was assumed as the most relevant signaling pathway which had included 8 metabolites and demonstrated the most significant p-value. Besides, “SLC-mediated transmembrane transport” and “metabolism of amino acids and derivatives” contained the largest number of associated molecules, each of which had included 9 metabolites.
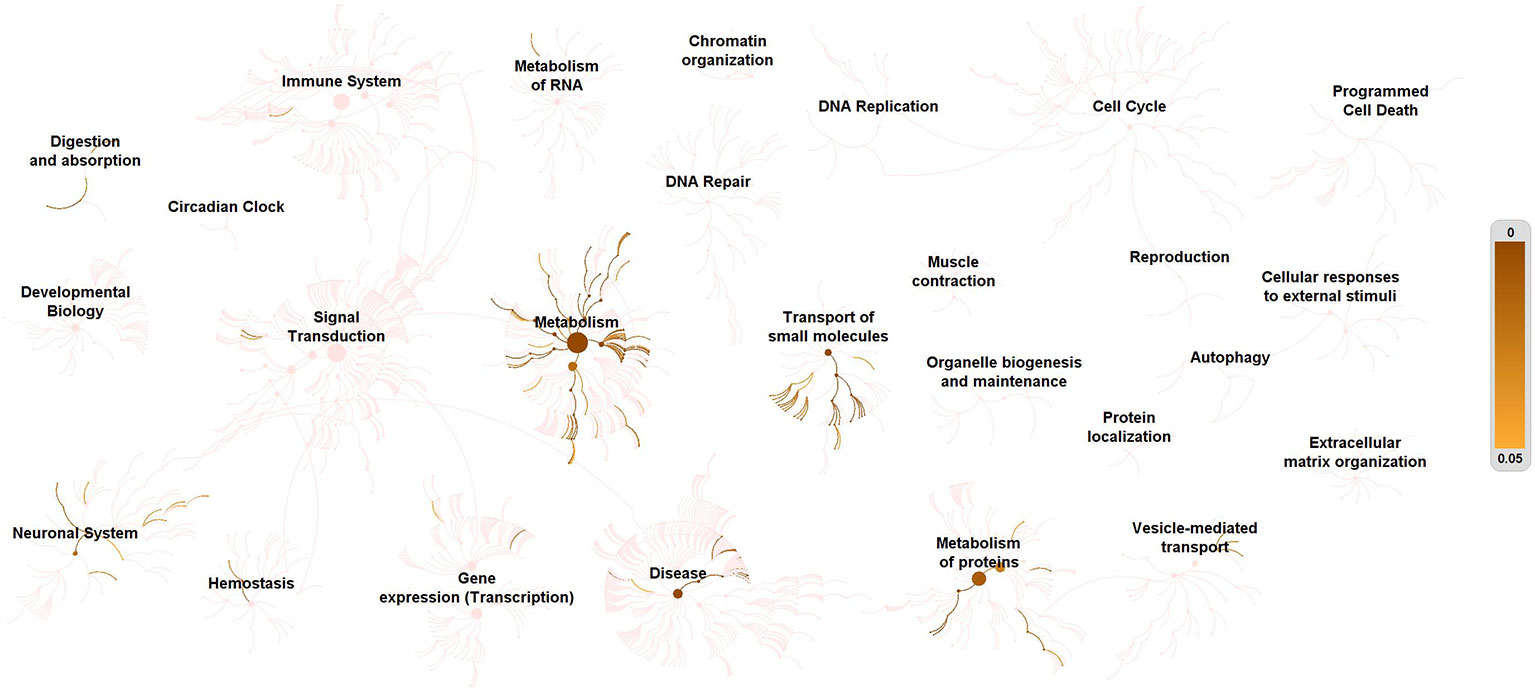
Figure 2 Enrichment of signaling pathways of metabolites in depression-associated suicide. All metabolites in depression-associated suicidal behavior were defined as KEGG C-numbers and brought into Reactome Pathway Analysis. Relevant pathways were highlighted. The right side bar indicates p-value.
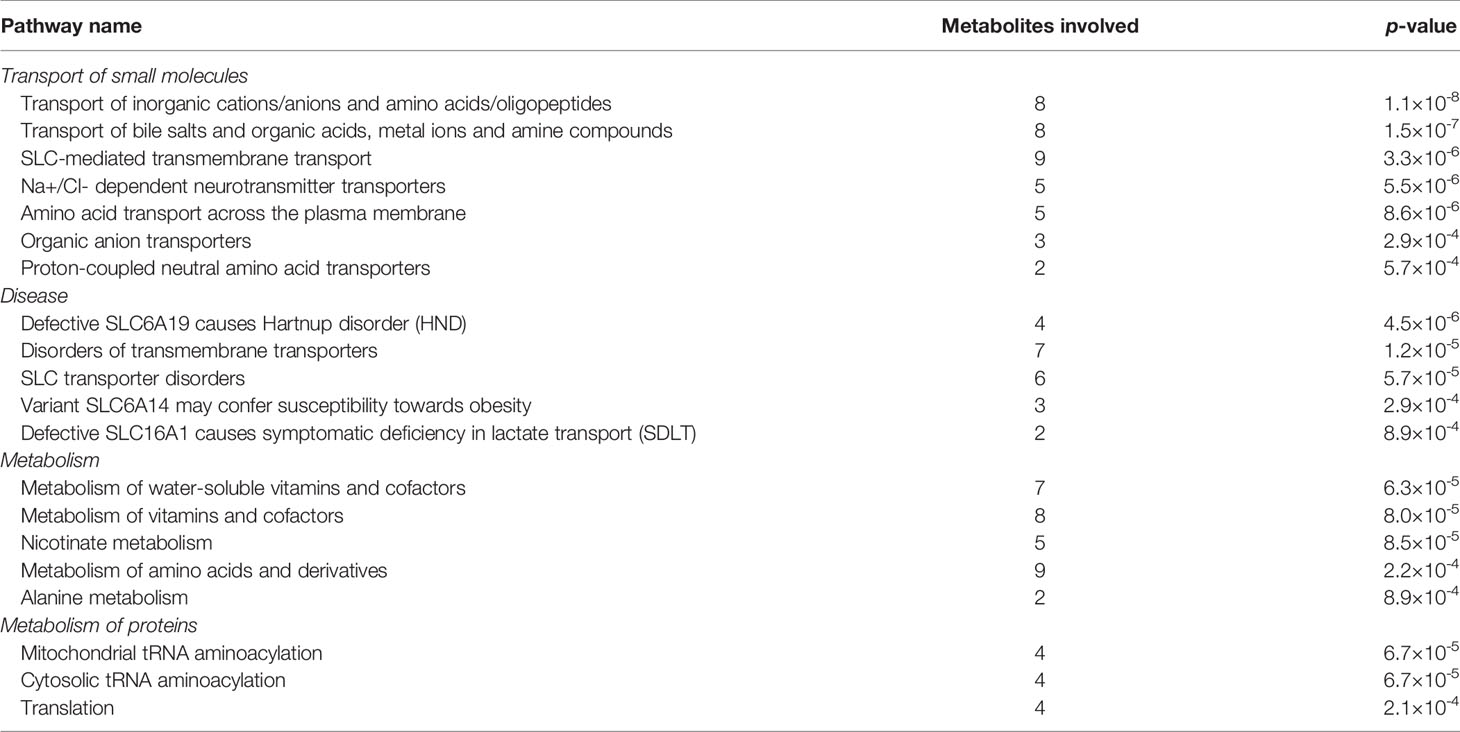
Table 2 Top 20 signaling pathways that differentially-expressed metabolites participated in depression-associated suicide.
Discussion
An initial purpose of this study was to identify all metabolites that expressed significantly different between drug-naïve depression patients with suicidal behavior and healthy population, according to current metabonomic studies. A subsequent purpose was to predict relevant signaling pathways that these metabolites theoretically participated in. In summary, we have successfully identified 17 metabolites that expressed differently between drug-naïve patients with depression-associated suicidal behaviors and healthy controls. The expression pattern of metabolites was inconsistent and even contrasted between studies. Peripheral tissues, especially plasma and urine, were extensively investigated in contrast to central system tissues that were difficult to obtain. We have integrated these 17 metabolites into biological signaling pathways and provided a visualized signaling network of metabolites involved in depressed suicidal patients. We have revealed that “transport of small molecules”, “disease”, “metabolism” and “metabolism of proteins” were the most relevant signaling sections, among which “transport of inorganic cations/anions and amino acids/oligopeptides”, “SLC-mediated transmembrane transport”, and “metabolism of amino acids and derivatives” should be further studied to elucidate their potential pathogenic mechanism in the development of depression and associated suicidal behavior.
SLCs (solute carriers) are the largest cluster of transmembrane transporters in charge of various substances exchange, including cations/anions and nutrients as well as transfer of drugs across the blood-brain barrier (52, 53). The function of SLCs is essential for the homeostasis of the brain, since they participate in energy and glutamate metabolism, neurotransmitter release, blood-brain barrier, etc. (54). Notably, both mice and human studies emphasized the importance of SLC6A15/v7-3 in the development of depression, of which the mechanism is associated with a neuronal circuits alteration that raises susceptibility to depression (55, 56).
The association between SLCs and a genetic predisposition of suicide was reported by Ernet et al. in 2009. They identified that SLC38A1 (also known as SNAT1) was significantly decreased in the brain of suicidal patients (57). A series of SLCs control multiple steps of GABA-glutamate metabolism, which is critical for brain homeostasis as well (58, 59). Dysfunction of SLCs or a disturbed GABA-glutamate cycle is related to the structural, functional and neurochemical impairment in neurons that are observed in patients with major depression (60). Whole genome analysis in brains of depression-associated suicidal victims found alteration of glutamate and GABA receptor genes in this condition (61), which was confirmed by subsequent studies (62–64). Nevertheless, the control subjects in these studies had not suffered from depression or committed suicide. To better clarify whether these gene alterations were caused by depression or suicide per se, Zhao et al. conducted a comparative study including major depression-committed suicide, major depression/non-suicide and matched healthy controls. They observed that the glutate-glutamine cycle was significantly changed in depressed suicide patients (65).
The pathophysiological role of liver in the development of depression was suggested by previous studies. Bile salt sulfotransferase 1 (SULT2A1) was associated with inflammation response, immune regulation and lipid metabolism in patients with major depressive disorder (66). Organic acid transportation that contributes to depression has been found, and a blocking therapy of organic cation transporter has shown antidepressant efficacy in patients with depression (67). In addition, several transporters of mental ions such as copper and zinc have been confirmed to promote the development of depression (68, 69).
Current diagnosis of depression is based on behavioral symptoms, and traditional anti-depressive drugs are ineffective for a considerable portion of depression patients. Although suicide is the most severe form of reaction to depression, the majority of depressed patients never attempt or commit suicide, implying a pathogenic mechanism of suicidal behavior distinct from other forms of reaction. Recent evidence is emerging to indicate a central role of small molecules in the development of depression. Understanding the pathogenic mechanism of small molecules in depression-associated suicide could promote the prevention, diagnosis and treatment of depressed patients at risk of suicide.
In conclusion, we report here a total of 17 metabolites that express differently between drug-naïve patients with depression-associated suicidal behaviors and healthy individuals. We also predict several signaling pathways that could participate in the development of depression and associated suicide. Our findings could provide an insight into the molecular pathogenesis of depression and potential targets for new drug inventions.
Data Availability Statement
The datasets generated during and analyzed during the current study are available from the corresponding author on reasonable request.
Ethics Statement
The studies involving human participants were reviewed and approved by Ethics Committee at Nanjing Drum Tower Hospital. Written informed consent for participation was not required for this study in accordance with the national legislation and the institutional requirements.
Author Contributions
SL performed data analysis and drafted the manuscript. All members of the Class 2005 of Medical School of Nanjing University designed the research, collected the data, reviewed and approved the manuscript for submission.
Consortium
Name list of the Class 2005 of Medical School of Nanjing University (Chinese name is shown in parentheses): CHEN Jinyun (陈锦云) 2, CHEN Kang (陈康) 2, CHEN Liang (陈亮) 2, CUI Shiyun (崔诗允) 2, DAI Xinjue (戴欣珏) 2, DING Chao (丁超) 2, DUAN Guihua (段桂华) 2, GAO Yibing (高逸冰) 2, GENG Linyu (耿林玉) 2, GOU Liming (苟黎明) 2, GU Xiaoling (顾晓凌) 2, GUO Wei (郭伟) 2, GUO Zhen (郭振) 2, HAN Lijuan (韩丽娟) 2, JIANG Kuan (蒋宽) 2, LEI Peng (雷鹏) 2, LI Jianhua (李建华) 2, LI Liyun (李励芸) 2, LI Ran (李苒) 2, LIANG Fengjing (梁凤晶) 2, LING Zhonghua (凌中华) 2, LIU Bing (刘兵) 2, LIU Song (刘颂) 2, LIU Shu (刘澍) 2, LIU Yuan (柳元) 2, MA Chunyan (马春燕) 2, MA Hucheng (马虎成) 2, NIU Yuxiang (牛宇翔) 2, OU Wei (欧伟) 2, QIN Jizheng (覃基政) 2, SHA Shifu (沙士甫) 2 DECEASED, SHEN Juanhong (沈娟红) 2, SUN Jing (孙晶) 2, SUN Qing (孙青) 2, WANG Fei (汪飞) 2, WANG Kongcheng (王孔成) 2, WANG Xinqiang (王鑫强) 2, WANG Xinyi (王昕怡) 2, WEN Juan (闻娟) 2, WU Buyun (邬步云) 2, WU Han (吴韩) 2, WU Li (吴丽) 2, WU Puyuan (吴浦嫄) 2, WU Xiaodong (吴晓东) 2, XIA Haiyan (夏海燕) 2, XIA Qiuyuan (夏秋媛) 2, XIE Zhigang (谢志刚) 2, XU Weiwei (徐维玮) 2, YAN Jizhang (延吉璋) 2, YANG Nan (杨楠) 2, YANG Yan (羊妍) 2, YAO Danhua (姚丹华) 2, Ye Xie (叶叶) 2, ZHANG Haifeng (张海峰) 2, ZHANG Luyao (张璐瑶) 2, ZHANG Xiaolei (张小磊) 2, ZHANG Yiyan (张艺艳) 2, ZHAO Xin (赵鑫) 2, ZHENG Yang (郑阳) 2, ZHOU Wei (周薇) 2, ZHU Genfei (祝根飞) 2, ZHU Jianhong (祝剑虹) 2.
Funding
This study is supported by the National Natural Science Foundation of China (SL) (81602103), Natural Science Foundation of Jiangsu Province (SL) (BK20160114), Distinguished Young Scholar Project of Medical Science and Technology Development Foundation of Nanjing Department of Health (SL) (JQX17005), Key Project of Medical Science and Technology Development Foundation of Nanjing Department of Health (SL) (YKK16114), Medical Research Program of Jiangsu Provincial Commission of Health and Family Planning (SL) (Q2017007), and Wu Jieping Medical Foundation (SL) (320.2710.1817).
Conflict of Interest
The author declares that the research was conducted in the absence of any commercial or financial relationships that could be construed as a potential conflict of interest.
Acknowledgments
We all lament the decease of our dearest classmate Dr. Shifu SHA, MD, PhD (Class 2005, Medical School of Nanjing University) who committed suicide due to depression on Feb 12th, 2018. His death raises our concerns about depression-associated suicidal behavior and inspires the draft of this article. May he rest in peace.
Supplementary Material
The Supplementary Material for this article can be found online at: https://www.frontiersin.org/articles/10.3389/fpsyt.2020.00269/full#supplementary-material
Supplementary Figure 1 | Distribution of tissue type in metabolite-associated studies. Studies that compare metabolites between depressed suicide attempters and healthy controls were included. PACC, pregenual anterior cingular cortex; PBMC, peripheral blood mononuclear cell; VPC, Ventrolateral prefrontal cortex.
References
1. Summergrad P. Investing in global mental health: the time for action is now. Lancet Psychiatry (2016) 3:390–1. doi: 10.1016/S2215-0366(16)30031-1
2. Lim GY, Tam WW, Lu Y, Ho CS, Zhang MW, Ho RC. Prevalence of depression in the community from 30 countries between 1994 and 2014. Sci Rep (2018) 8:2861. doi: 10.1038/s41598-018-21243-x
3. Daly EJ, Trivedi MH, Wisniewski SR, Nierenberg AA, Gaynes BN, Warden D, et al. Health-related quality of life in depression: a STAR*D report. Ann Clin Psychiatry (2010) 22:43–55.
4. Large M. Study on suicide risk assessment in mental illness underestimates inpatient suicide risk. BMJ. (2016) 532:i267. doi: 10.1136/bmj.i267
5. Bostwick JM, Pankratz VS. Affective disorders and suicide risk: a reexamination. Am J Psychiatry (2000) 157:1925–32. doi: 10.1176/appi.ajp.157.12.1925
6. Hawton K, Casanas I Comabella C, Haw C, Saunders K. Risk factors for suicide in individuals with depression: a systematic review. J Affect Disord (2013) 147:17–28. doi: 10.1016/j.jad.2013.01.004
7. Mata DA, Ramos MA, Bansal N, Khan R, Guille C, Di Angelantonio E, et al. Prevalence of depression and depressive symptoms among resident physicians: a systematic review and meta-analysis. JAMA. (2015) 314:2373–83. doi: 10.1001/jama.2015.15845
8. Goldman ML, Shah RN, Bernstein CA. Depression and suicide among physician trainees: recommendations for a national response. JAMA Psychiatry (2015) 72:411–2. doi: 10.1001/jamapsychiatry.2014.3050
9. Shanafelt TD, Balch CM, Dyrbye L, Bechamps G, Russell T, Satele D, et al. Special report: suicidal ideation among American surgeons. Arch Surg (2011) 146:54–62. doi: 10.1001/archsurg.2010.292
10. Panagioti M, Panagopoulou E, Bower P, Lewith G, Kontopantelis E, Chew-Graham C, et al. Controlled interventions to reduce burnout in physicians: a systematic review and meta-analysis. JAMA Intern Med (2017) 177:195–205. doi: 10.1001/jamainternmed.2016.7674
11. Center C, Davis M, Detre T, Ford DE, Hansbrough W, Hendin H, et al. Confronting depression and suicide in physicians: a consensus statement. JAMA. (2003) 289:3161–6. doi: 10.1001/jama.289.23.3161
12. Miller AH, Raison CL. The role of inflammation in depression: from evolutionary imperative to modern treatment target. Nat Rev Immunol (2016) 16:22–34. doi: 10.1038/nri.2015.5
13. Hodes GE, Kana V, Menard C, Menard C, Merad M, Russo SJ. Neuroimmune mechanisms of depression. Nat Neurosci (2015) 18:1386–93. doi: 10.1038/nn.4113
14. Wohleb ES, Franklin T, Iwata M, Duman RS. Integrating neuroimmune systems in the neurobiology of depression. Nat Rev Neurosci (2016) 17:497–511. doi: 10.1038/nrn.2016.69
15. Murrough JW, Abdallah CG, Mathew SJ. Targeting glutamate signalling in depression: progress and prospects. Nat Rev Drug Discovery (2017) 16:472–86. doi: 10.1038/nrd.2017.16
16. Zanos P, Moaddel R, Morris PJ, Georgiou P, Fischell J, Elmer GI, et al. NMDAR inhibition-independent antidepressant actions of ketamine metabolites. Nature. (2016) 533:481–6. doi: 10.1038/nature17998
17. Valles-Colomer M, Falony G, Darzi Y, Tigchelaar EF, Wang J, Tito RY, et al. The neuroactive potential of the human gut microbiota in quality of life and depression. Nat Microbiol (2019) 4:623–32. doi: 10.1038/s41564-018-0337-x
18. Lumsden EW, Troppoli TA, Myers SJ, Zanos P, Aracava Y, Kehr J, et al. Antidepressant-relevant concentrations of the ketamine metabolite (2R,6R)-hydroxynorketamine do not block NMDA receptor function. Proc Natl Acad Sci U S A. (2019) 116:5160–9. doi: 10.1073/pnas.1816071116
19. Fabregat A, Jupe S, Matthews L, Sidiropoulos K, Gillespie M, Garapati P, et al. The reactome pathway knowledgebase. Nucleic Acids Res (2018) 46:D649–55. doi: 10.1093/nar/gkx1132
20. Fabregat A, Sidiropoulos K, Viteri G, Forner O, Marin-Garcia P, Arnau V, et al. Reactome pathway analysis: a high-performance in-memory approach. BMC Bioinf (2017) 18:142. doi: 10.1186/s12859-017-1559-2
21. Hou L, Xu Q, Duan S, Wang H, Li W, Song X, et al. Diagnosis of major depression in hepatitis B patients by urine metabolomics. J Shanghai Jiao Tong Univer Med Sci (2014) 34:1647–56. doi: 11.3969/j.issn.1674-8115.2014.11.018
22. Zheng P, Chen JJ, Zhou CJ, Zeng L, Li KW, Sun L, et al. Identification of sex-specific urinary biomarkers for major depressive disorder by combined application of NMR- and GC-MS-based metabonomics. Transl Psychiatry (2016) 6:e955. doi: 10.1038/tp.2016.188
23. Lin L, Chen XM, Liu RH. Novel urinary metabolite signature for diagnosing postpartum depression. Neuropsychiatr Dis Treat (2017) 13:1263–70. doi: 10.2147/NDT.S135190
24. Zheng P, Gao H, Qi Z, Jia J, Li F, Chen J, et al. Peripheral metabolic abnormalities of lipids and amino acids implicated in increased risk of suicidal behavior in major depressive disorder. Metabolomics. (2013) 9:688–96. doi: 10.1007/s11306-012-0474-9
25. Hou LJ, Wang HW, Wei XX, Duan SP, Zhuo Y, Song XW, et al. Urinary metabonomics for diagnosis of depression in Hepatitis B Virus-infected patients. Iran Red Crescent Med J (2015) 17:e27359. doi: 10.5812/ircmj.17(4)2015.27359
26. Chen JJ, Zhou CJ, Zheng P, Cheng K, Wang HY, Li J, et al. Differential urinary metabolites related with the severity of major depressive disorder. Behav Brain Res (2017) 332:280–7. doi: 10.1016/j.bbr.2017.06.012
27. Ding X, Yang S, Li W, Liu Y, Li Z, Zhang Y, et al. The potential biomarker panels for identification of major depressive disorder (MDD) patients with and without early life stress (ELS) by metabonomic analysis. PloS One (2014) 9:e97479. doi: 10.1371/journal.pone.0097479
28. Zheng P, Chen JJ, Huang T, Wang MJ, Wang Y, Dong MX, et al. A novel urinary metabolite signature for diagnosing major depressive disorder. J Proteome Res (2013) 12:5904–11. doi: 10.1021/pr400939q
29. Liu CC, Wu YF, Feng GM, Gao XX, Zhou YZ, Hou WJ, et al. Plasma-metabolite-biomarkers for the therapeutic response in depressed patients by the traditional Chinese medicine formula Xiaoyaosan: a 1H NMR-based metabolomics approach. J Affect Disord (2015) 185:156–63. doi: 10.1016/j.jad.2015.05.005
30. Xia XT, Sun N, Liu CC, Qin XM, Tian JS. Discovering potential biomarkers of depression and drug intervention of paroxetine based on 1H NMR metabolomics. Yao Xue Bao. (2016) 51:595–9. doi: 10.16438/j.0513-4870.2015-0588
31. Zheng P, Wang Y, Chen L, Yang D, Meng H, Zhou D, et al. Identification and validation of urinary metabolite biomarkers for major depressive disorder. Mol Cell Proteomics (2013) 12:207–14. doi: 10.1074/mcp.M112.021816
32. Zheng P, Gao HC, Li Q, Shao WH, Zhang ML, Cheng K, et al. Plasma metabonomics as a novel diagnostic approach for major depressive disorder. J Proteome Res (2012) 11:1741–8. doi: 10.1021/pr2010082
33. Tian JS, Peng GJ, Wu YF, Zhou JJ, Xiang H, Gao XX, et al. A GC-MS urinary quantitative metabolomics analysis in depressed patients treated with TCM formula of Xiaoyaosan. J Chromatogr B Analyt Technol BioMed Life Sci (2016) 1026:227–35. doi: 10.1016/j.jchromb.2015.12.026
34. Steffens DC, Jiang W, Krishnan KR, Karoly ED, Mitchell MW, O'Connor CM, et al. Metabolomic differences in heart failure patients with and without major depression. J Geriatr Psychiatry Neurol (2010) 23:138–46. doi: 10.1177/0891988709358592
35. Woo HI, Chun MR, Yang JS, Lim SW, Kim MJ, Kim SW, et al. Plasma amino acid profiling in major depressive disorder treated with selective serotonin reuptake inhibitors. CNS Neurosci Ther (2015) 21:417–24. doi: 10.1111/cns.12372
36. Liu Y, Yieh L, Yang T, Drinkenburg W, Peeters P, Steckler T, et al. Metabolomic biosignature differentiates melancholic depressive patients from healthy controls. BMC Genomics (2016) 17:669. doi: 10.1186/s12864-016-2953-2
37. Umehara H, Numata S, Watanabe SY, Hatakeyama Y, Kinoshita M, Tomioka Y, et al. Altered KYN/TRP, Gln/Glu, and Met/methionine sulfoxide ratios in the blood plasma of medication-free patients with major depressive disorder. Sci Rep (2017) 7:4855. doi: 10.1038/s41598-017-05121-6
38. Levine J, Panchalingam K, Rapoport A, Gershon S, McClure RJ, Pettegrew JW. Increased cerebrospinal fluid glutamine levels in depressed patients. Biol Psychiatry (2000) 47:586–93. doi: 10.1016/S0006-3223(99)00284-X
39. Ajilore O, Haroon E, Kumaran S, Darwin C, Binesh N, Mintz J, et al. Measurement of brain metabolites in patients with type 2 diabetes and major depression using proton magnetic resonance spectroscopy. Neuropsychopharmacology (2007) 32:1224–31. doi: 10.1038/sj.npp.1301248
40. Godlewska BR, Masaki C, Sharpley AL, Cowen PJ, Emir UE. Brain glutamate in medication-free depressed patients: a proton MRS study at 7 Tesla. Psychol Med (2017) 48:1731–7. doi: 10.1017/S0033291717003373
41. Zhang W, Zhang XA. A novel urinary metabolite signature for non-invasive post-stroke depression diagnosis. Cell Biochem Biophys (2015) 72:661–7. doi: 10.1007/s12013-014-0472-9
42. Xiao J, Zhang J, Sun D, Wang L, Yu L, Wu H, et al. Discriminating poststroke depression from stroke by nuclear magnetic resonance spectroscopy based metabonomic analysis. Neuropsychiatr Dis Treat (2016) 12:1919–25. doi: 10.2147/NDT.S110613
43. Shungu DC, Weiduschat N, Murrough JW, Mao X, Pillemer S, Dyke JP, et al. Increased ventricular lactate in chronic fatigue syndrome. III. Relationships to cortical glutathione and clinical symptoms implicate oxidative stress in disorder pathophysiology. NMR BioMed (2012) 25:1073–87. doi: 10.1002/nbm.2772
44. Bradley KA, Mao X, Case JA, Kang G, Shungu DC, Gabbay V. Increased ventricular cerebrospinal fluid lactate in depressed adolescents. Eur Psychiatry (2016) 32:1–8. doi: 10.1016/j.eurpsy.2015.08.009
45. Ernst J, Hock A, Henning A, Seifritz E, Boeker H, Grimm S. Increased pregenual anterior cingulate glucose and lactate concentrations in major depressive disorder. Mol Psychiatry (2017) 22:113–9. doi: 10.1038/mp.2016.73
46. Zheng P, Fang Z, Xu XJ, Liu ML, Du X, Zhang X, et al. Metabolite signature for diagnosing major depressive disorder in peripheral blood mononuclear cells. J Affect Disord (2016) 195:75–81. doi: 10.1016/j.jad.2016.02.008
47. Chen GG, Fiori LM, Moquin L, Gratton A, Mamer O, Mechawar N, et al. Evidence of altered polyamine concentrations in cerebral cortex of suicide completers. Neuropsychopharmacology (2010) 35:1477–84. doi: 10.1038/npp.2010.17
48. Zheng H, Zheng P, Zhao L, Jia J, Tang S, Xu P, et al. Predictive diagnosis of major depression using NMR-based metabolomics and least-squares support vector machine. Clin Chim Acta (2017) 464:223–7. doi: 10.1016/j.cca.2016.11.039
49. Erhardt S, Lim CK, Linderholm KR, Janelidze S, Lindqvist D, Samuelsson M, et al. Connecting inflammation with glutamate agonism in suicidality. Neuropsychopharmacology (2013) 38:743–52. doi: 10.1038/npp.2012.248
50. Clark SM, Pocivavsek A, Nicholson JD, Notarangelo FM, Langenberg P, McMahon RP, et al. Reduced kynurenine pathway metabolism and cytokine expression in the prefrontal cortex of depressed individuals. J Psychiatry Neurosci (2016) 41:386–94. doi: 10.1503/jpn.150226
51. Kawamura N, Shinoda K, Sato H, Sasaki K, Suzuki M, Yamaki K, et al. Plasma metabolome analysis of patients with major depressive disorder. Psychiatry Clin Neurosci (2018) 72:349–61. doi: 10.1111/pcn.12638
52. Zhang Y, Zhang Y, Sun K, Meng Z, Chen L. The SLC transporter in nutrient and metabolic sensing, regulation, and drug development. J Mol Cell Biol (2019) 11:1–13. doi: 10.1093/jmcb/mjy052
53. Cesar-Razquin A, Snijder B, Frappier-Brinton T, Isserlin R, Gyimesi G, Bai X, et al. A call for systematic research on solute carriers. Cell (2015) 162:478–87. doi: 10.1016/j.cell.2015.07.022
54. Hu C, Tao L, Cao X, Chen L. The solute carrier transporters and the brain: physiological and pharmacological implications. Asian J Pharm Sci (2019). doi: 10.1016/j.ajps.2019.09.002
55. Kohli MA, Lucae S, Saemann PG, Schmidt MV, Demirkan A, Hek K, et al. The neuronal transporter gene SLC6A15 confers risk to major depression. Neuron (2011) 70:252–65. doi: 10.1016/j.neuron.2011.04.005
56. Schuhmacher A, Lennertz L, Wagner M, Höfels S, Pfeiffer U, Guttenthaler V, et al. A variant of the neuronal amino acid transporter SLC6A15 is associated with ACTH and cortisol responses and cognitive performance in unipolar depression. Int J Neuropsychopharmacol (2013) 16:83–90. doi: 10.1017/S1461145712000223
57. Ernst C, Dumoulin P, Cabot S, Erickson J, Turecki G. SNAT1 and a family with high rates of suicidal behavior. Neuroscience (2009) 162:415–22. doi: 10.1016/j.neuroscience.2009.05.010
58. Leke R, Schousboe A. The glutamine transporters and their role in the glutamate/GABA-glutamine cycle. Adv Neurobiol (2016) 13:223–57. doi: 10.1007/978-3-319-45096-4_8
59. Jenstad M, Chaudhry FA. The amino acid transporters of the glutamate/GABA-glutamine cycle and their impact on insulin and glucagon secretion. Front Endocrinol (Lausanne) (2013) 4:199. doi: 10.3389/fendo.2013.00199
60. Wu Y, Tang J, Zhou C, Zhao L, Chen J, Zeng L, et al. Quantitative proteomics analysis of the liver reveals immune regulation and lipid metabolism dysregulation in a mouse model of depression. Behav Brain Res (2016) 311:330–9. doi: 10.1016/j.bbr.2016.05.057
61. Sequeira A, Mamdani F, Ernst C, Vawter MP, Bunney WE, Lebel V, et al. Global brain gene expression analysis links glutamatergic and GABAergic alterations to suicide and major depression. PloS One (2009) 4:e6585. doi: 10.1371/journal.pone.0006585
62. Bernard R, Kerman IA, Thompson RC, Jones EG, Bunney WE, Barchas JD, et al. Altered expression of glutamate signaling, growth factor, and glia genes in the locus coeruleus of patients with major depression. Mol Psychiatry (2011) 16:634–46. doi: 10.1038/mp.2010.44
63. Choudary PV, Molnar M, Evans SJ, Tomita H, Li JZ, Vawter MP, et al. Altered cortical glutamatergic and GABAergic signal transmission with glial involvement in depression. Proc Natl Acad Sci U S A (2005) 102:15653–8. doi: 10.1073/pnas.0507901102
64. Duric V, Banasr M, Stockmeier CA, Simen AA, Newton SS, Overholser JC, et al. Altered expression of synapse and glutamate related genes in post- mortem hippocampus of depressed subjects. Int J Neuropsychopharmacol (2013) 16:69–82. doi: 10.1017/S1461145712000016
65. Zhao J, Verwer RW, van Wamelen DJ, Qi XR, Gao SF, Lucassen PJ, et al. Prefrontal changes in the glutamate-glutamine cycle and neuronal/glial glutamate transporters in depression with and without suicide. J Psychiatr Res (2016) 82:8–15. doi: 10.1016/j.jpsychires.2016.06.017
66. Kious BM, Kondo DG, Renshaw PF. Creatine for the treatment of depression. Biomolecules (2019) 9:E406. doi: 10.3390/biom9090406
67. Orrico-Sanchez A, Chausset-Boissarie L, Alves de Sousa R, Coutens B, Amin S, Vialou V, et al. Antidepressant efficacy of a selective organic cation transporter blocker in a mouse model of depression. Mol Psychiatry (2019). doi: 10.1038/s41380-019-0548-4
68. Slupski J, Cubala WJ, Gorska N, Gałuszko-Węgielnik M, Wiglusz MS. Role of copper in depression. Relationship with ketamine treatment. Med Hypotheses (2018) 119:14–7. doi: 10.1016/j.mehy.2018.07.012
Keywords: metabolites, depression, signalling, metabonomic profile, suicidal
Citation: Liu S (2020) Metabonomic Profile and Signaling Pathway Prediction of Depression-Associated Suicidal Behavior. Front. Psychiatry 11:269. doi: 10.3389/fpsyt.2020.00269
Received: 11 November 2019; Accepted: 19 March 2020;
Published: 16 April 2020.
Edited by:
Helge Frieling, Hannover Medical School, GermanyReviewed by:
Tianmei Si, Peking University Sixth Hospital, ChinaGita A. Pathak, Yale University, United States
Copyright © 2020 Liu. This is an open-access article distributed under the terms of the Creative Commons Attribution License (CC BY). The use, distribution or reproduction in other forums is permitted, provided the original author(s) and the copyright owner(s) are credited and that the original publication in this journal is cited, in accordance with accepted academic practice. No use, distribution or reproduction is permitted which does not comply with these terms.
*Correspondence: Song Liu, medical.lis@gmail.com