- 1Department of Psychosomatic Medicine, First Teaching Hospital of Tianjin University of Traditional Chinese Medicine, Tianjin, China
- 2Key Laboratory of Mental Health, Ministry of Health (Peking University), National Clinical Research Center for Mental Disorders (Peking University Sixth Hospital), Peking University Sixth Hospital and Peking University Institute of Mental Health, Beijing, China
- 3Tianjin Key Laboratory of Traditional Research of TCM Prescription and Syndrome, Medical Experiment Center, First Teaching Hospital of Tianjin University of Traditional Chinese Medicine, Tianjin, China
- 4Xiamen Xianyue Hospital, Xiamen, China
- 5Hebei North University, Hebei, China
Background: At present, laboratory blood tests to support major depressive disorder (MDD) diagnosis are not available. This study aimed to screen potential mRNAs for peripheral blood biomarkers and novel pathophysiology of MDD.
Methods: The present study utilized public data from two mRNA microarray datasets to analyze the hub genes changes related to MDD. Gene Ontology (GO) analysis and Kyoto Encyclopedia of Genes and Genomes (KEGG) pathway analysis of differentially expressed genes (DEGs) were performed. Finally, some potential mRNA quality biomarkers for hub gene expression in blood were identified.
Results: A total of 25 significantly co-upregulated DEGs and 98 co-downregulated DEGs were obtained from two datasets. The pathway enrichment analyses showed that co-upregulated genes were significantly enriched in the regulation of cell-matrix adhesion and mitochondrial membrane permeability which were involved in the apoptotic process. Co-downregulated genes were mainly involved in the neutrophil activation which in turn was involved in the immune response, degranulation and cell-mediated immunity, positive regulation of immune response, the Toll-like receptor signaling pathway, and the NOD-like receptor signaling pathway. From the PPI network, 14 hub genes were obtained. Among them, the subnetworks of PLCG1, BCL2A1, TLR8, FADD, and TLR4 screened out from our study have been shown to play a role in immune and inflammation responses.
Discussion: The potential molecular mechanisms that have been identified simultaneously include innate immunity, neuroinflammation, and neurotrophic factors for synapse function and development.
Introduction
Major depressive disorder (MDD) is a highly disabling mental illness involving an imbalance in brain chemicals, and it majorly contributes to the global burden of disease (1). According to the World Health Organization, an estimated 350 million people of all ages suffer from depression disorder globally (2). In a systematic review, the summary estimate of the prevalence of depression or depressive symptoms among medical students was 27.2%, and that of suicidal ideation was 11.1% (3). A psychiatric disorder is not a sign of personal weakness or a character flaw, but it reveals an opposite result with a highly prevalent heritability that accounts for major psychological (4), physical (5), and social impairments (6). At present, the criteria for MDD diagnosis and treatment are based on various signs and symptoms that do not always fit into strict diagnostic categories, such as the Diagnostic and Statistical Manual of Mental Disorders, Fifth Edition (DSM-5) (7). All of the possible causes for a set of past experiences have to be examined, including personal private medical information and confidential material, which increases stigma and makes diagnosis even more difficult (8). Recent studies implicate that functional magnetic resonance imaging (fMRI) may provide successful diagnostic information in depression disorder classification (9, 10); however, objective criteria and gold standards in early diagnosis for patients with MDD remain to be elucidated (7, 11). Previously, the microarray technique was used for life science research purposes. Bioinformatics data-mining of gene and microarray technologies has widely been used for differential expression analysis to identify novel diagnostic and therapeutic biomarkers of diseases (12, 13).
Over the past decades, several biomarkers have been proposed for MDD (13–15), but at the moment none of these biomarkers reaches sufficient sensitivity and specificity to be implemented in clinical practice (14). Recently, many potential mechanism studies have demonstrated that multiple genes and cellular pathways participate in the occurrence and development of MDD (15) and other mental illnesses (16). Numerous researchers have found that the pathophysiology of depression results from changes in oxidative stress (17), immune system effects (18), and neuroinflammation (19) in the central nervous system (CNS) through cytokines, which regulate brain activities and emotions. To understand the molecular processes that control neuronal activity and arrive at an objective diagnosis, we tried to obtain novel indicators of possible molecular mechanisms and predict peripheral blood molecular biomarkers in MDD patients and attempted to provide potential therapeutic targets for this challenging disease.
In the present study, two mRNA microarray datasets with MDD and control groups were downloaded from Gene Expression Omnibus (GEO) and screened for differentially expressed genes (DEGs). Gene Ontology (GO) functional annotation analysis and Kyoto Encyclopedia of Genes and Genomes (KEGG) pathway enrichment analysis in the online Database of Enrichr were performed for the screened DEGs. Then, we established a protein–protein interaction (PPI) network based on the Search Tool for the Retrieval of Interacting Genes (STRING) database and Cytoscape software to identify hub genes related to MDD. Subsequently, the hub gene and miRNA-mRNA pair interactions were identified. This work will provide further insight into the pathophysiology of MDD development at the molecular level and explore the potential molecular targets for new interventional strategies.
Methods
Microarray Data
In order to identify the genes expressed in MDD samples compared to normal tissues, after a careful review, two gene expression profiles (GSE76826 and GSE98793) were selected and downloaded from the Gene Expression Omnibus database (GEO, www.ncbi.nlm.nih.gov/geo/), which is a public functional genomics data repository of high-throughput gene expression data, chips, and microarrays.
The microarray dataset GSE76826 was deposited by Miyata et al. (20), and expression profiling arrays were generated using GPL17077 Agilent-039494 SurePrint G3 Human GE v2 8x60K Microarray 039381 (Agilent Technologies, Inc., Palo Alto, CA). A total of 32 samples were utilized, including 10 samples of peripheral blood cells from patients with depression (MDD group), 10 samples of patients in remission, and 12 samples from healthy controls (control group). The samples of the MDD group and control group were selected for further analysis.
Additionally, the 192 gene expression profiles of the GSE98793 dataset by Leday et al. (21) were based on the GPL570 [HG-U133_Plus_2] platform using the Affymetrix Human Genome U133 Plus 2.0 Array (Affymetrix, Inc., Santa Clara, CA). Blood samples of the dataset were collected from MDD (N = 128) and control patients (N = 64). We downloaded the raw CEL file and the probe annotation file. The probes were converted into the corresponding gene symbol according to the annotation information in the platform. All of the data were freely available online, and this study did not involve any experiment on humans or animals performed by any of the authors.
Data Pretreatment and Identification of DEGs
The raw microarray data of GSE98793 in CEL format were initially preprocessed into expression values through the Affy package (22) (http://www.bioconductor.org/packages/release/bioc/html/affy. html) in R software (version 3.5.2, https://www.r-project.org/), and then we used background correction, normalization, and summarization to create a robust multiarray average (RMA). The series matrix files of the GSE76826 dataset were the normalized log-expression values available for further analysis.
To characterize differentially expressed genes (DEGs), the control group and the MDD group were analyzed using the LIMMA (linear models for microarray data) package (23) in the R/Bioconductor platform. Benjamini–Hochberg's method was used to control the false discovery rate, and the adjusted P-value < 0.05 and |Log2 fold-change| > 0.6 were defined as the threshold. The Venn diagram was also constructed using the VennDiagram package (24) in R. All significant DEGs are shown in a volcano plot generated using R software.
Gene Ontology and KEGG Pathway Analysis
Gene Ontology (GO) analysis is a common and useful method for large-scale functional enrichment research. To further analyze the potential biological process (BP), molecular function (MF), and cellular component (CC), the Kyoto Encyclopedia of Genes and Genomes (KEGG) pathway enrichment analysis of the overlapping DEGs between two the groups was submitted to the online Database of Enrichr (http://amp.pharm.mssm.edu/Enrichr/) to conduct functional and pathway enrichment analysis in this study. Enrichr is a useful online tool for annotating genes (25–27), which provides the functionality to perform simultaneous GO and KEGG analysis. P < 0.05 was considered to indicate a statistically significant difference.
Protein-Protein Interaction (PPI) Network and Hub Gene Identification
To systematically analyze the biological functions of the obtained DEGs between the two groups, the DEGs identified previously were mapped into the online search tool STRING database (STRING, V11.0; https://string-db.org/) (28) that could predict the protein functional associations and protein-protein interactions (PPI). A combined score ≥ 0.4 of PPI pairs was considered significant. Then, the Cytoscape software (http://www.cytoscape.org/, version 3.7.1; Institute for Systems Biology, Seattle, WA, USA) (29) was used for constructing and visualizing the transcriptional regulatory network of common DEGs. To further identify key elements in the biological process (BP), the hub genes in the network defined as possessing a connective degree ≥ 3 were identified and visualized using the CentiScaPe v2.2 plugin (30), and the degree of each protein node using MCODE in Cytoscape was calculated. All the parameters were set as defaults.
Construction of the mRNA-miRNA Interaction Network
To construct and analyze the miRNA-mRNA regulatory network, we applied the online prediction tools TargetScan (Release 7.2; http://www.targetscan.org/vert_72/) (31) and miRTarBase (Release 7.0, http://mirtarbase.mbc.nctu.edu.tw) (32) to predict the possible target mRNAs. Those mRNA–miRNA pairs with inverse expression relationships were included for network construction. Finally, we used Cytoscape software to construct interaction networks of mRNAs and related miRNAs.
Results
Identification of Differentially Expressed Genes
We studied two microarray MDD datasets (GSE76826 and GSE98793) from independent experiments to detect DEGs that were dysregulated in MDD samples compared to normal samples. In the GSE76826 gene chip, 1130 DEGs were identified; 556 genes were upregulated, and 574 genes were downregulated (Figure 1A). In addition to GSE98793, 4052 DEGs, including 1,014 upregulated genes and 3,038 downregulated genes, were identified (Figure 1B). The overlap analysis between the two datasets contained 25 significantly co-upregulated genes and 98 co-downregulated genes, as shown in the Venn diagram in R (Figures 1C,D). As a result, the number of co-downregulated DEGs is larger than the number of co-upregulated DEGs.
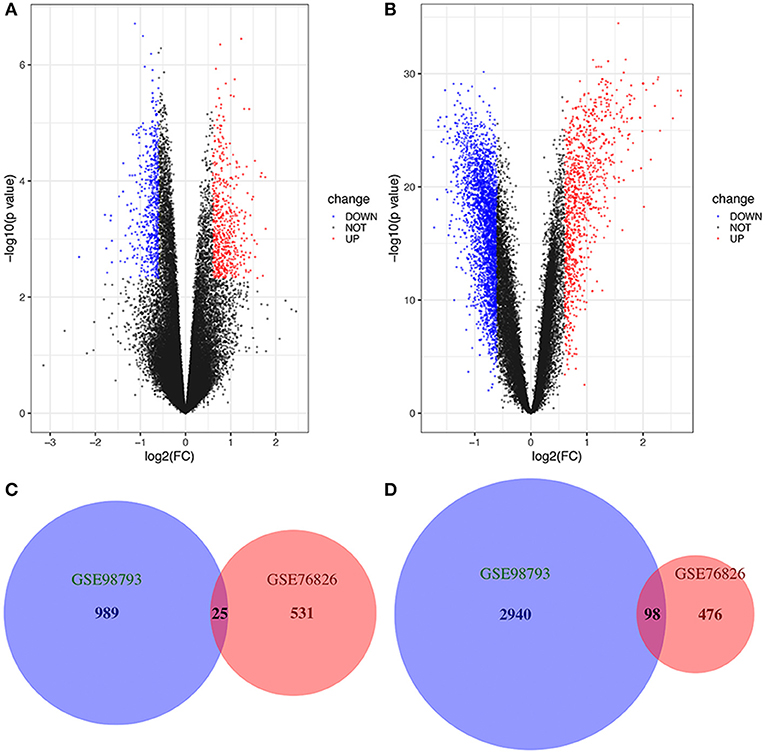
Figure 1. Volcano plot and Venn diagram of DEGs in the mRNA expression profiling datasets. Volcano plots of DEGs in normal and MDD samples in the (A) GSE76826 and (B) GSE98793 datasets (FC, fold-change). Colors represent different genes: black nodes represent genes without significantly different expression, red nodes represent upregulated genes, and blue nodes represent downregulated genes. Venn diagrams illustrating the number of (C) upregulated and (D) downregulated genes in the two datasets. The intersection in red represents the DEGs that are common between the two datasets.
GO Functional and KEGG Pathway Enrichment Analysis of DEGs
To further investigate the functions and mechanisms of DEGs, GO and KEGG pathway enrichment analyses of upregulated and downregulated genes were performed in the online Enrichr database. According to the results of the enrichment analysis, a total of 337 GO terms and 27 pathways of DEGs (FDR < 0.05), including 269 biological processes (BPs), 36 cellular components (CCs), and 32 molecular functions (MFs), were obtained, and the top five of each items are presented (Table 1).
The GO analysis results showed that for BP, upregulated DEGs were significantly enriched in glucocorticoid and corticosteroid receptor signaling pathways, regulation of cell-matrix adhesion, negative regulation of keratinocyte proliferation, and regulation of mitochondrial membrane permeability involved in apoptotic processes. Downregulated DEGs were significantly enriched in neutrophil-mediated immunity, degranulation, and activation involved in the immune response, positive regulation of the immune response and interleukin-8 production. Upregulated DEGs that were significantly enriched in CC, included centrosome, pericentriolar material, contractile fiber and myofibril. Downregulated DEGs that were significantly enriched in CCs included specific granule, specific granule membrane, secretory and ficolin-1-rich granule lumen. GO MF showed that the upregulated DEGs were significantly enriched in neurotrophin TRKA receptor binding, palmitoyl-CoA hydrolase activity, N6-methyladenosine-containing RNA binding, neurotrophin TRK receptor binding, and guanylate kinase activity. Downregulated DEGs were significantly enriched in calcium ion binding, metal ion binding, nucleoside-diphosphatase activity, oxidoreductase activity, acting on the aldehyde or oxo group of donors, NAD or NADP as acceptor, and tumor necrosis factor receptor superfamily binding. These results are comprehensively summarized (Table 1).
Moreover, 27 KEGG pathways were overrepresented in the DEGs. Only two upregulated DEGs, including the thyroid hormone signaling pathway and fatty acid elongation, and the 25 downregulated DEGs were significantly enriched in KEGG pathways, including measles, Toll-like receptor signaling pathway, complement and coagulation cascades, hepatitis B and influenza A, etc. The results obtained for the KEGG enrichment analyses are shown in Figure 2.
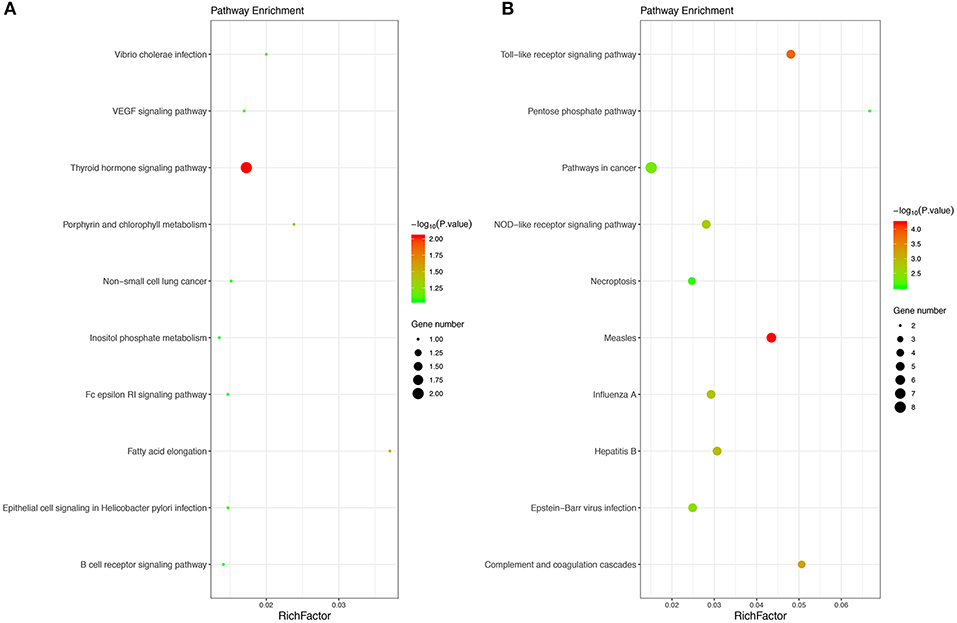
Figure 2. The top 10 KEGG pathways of upregulated (A) and downregulated (B) enriched DEGs. The size of bubble shows the enrichment score, while colors indicate enrichment significance. KEGG, Kyoto Encyclopedia of Genes and Genomes.
PPI Network Construction and Hub Gene Identification
To systematically analyze the biological functions of the obtained DEGs between the two groups, a PPI network of DEGs was constructed based on the STRING database and was visualized by Cytoscape (Figure 3A). In the PPI network, which has 54 nodes and 60 edges, it is well acknowledged that subnetwork analysis of genes plays important roles in integrated biological networks. Based on the results of the degree calculation using the cytoHubba plugin of Cytoscape, the most significant module was identified to have relatively high degrees in the regulatory network (Figure 3B).
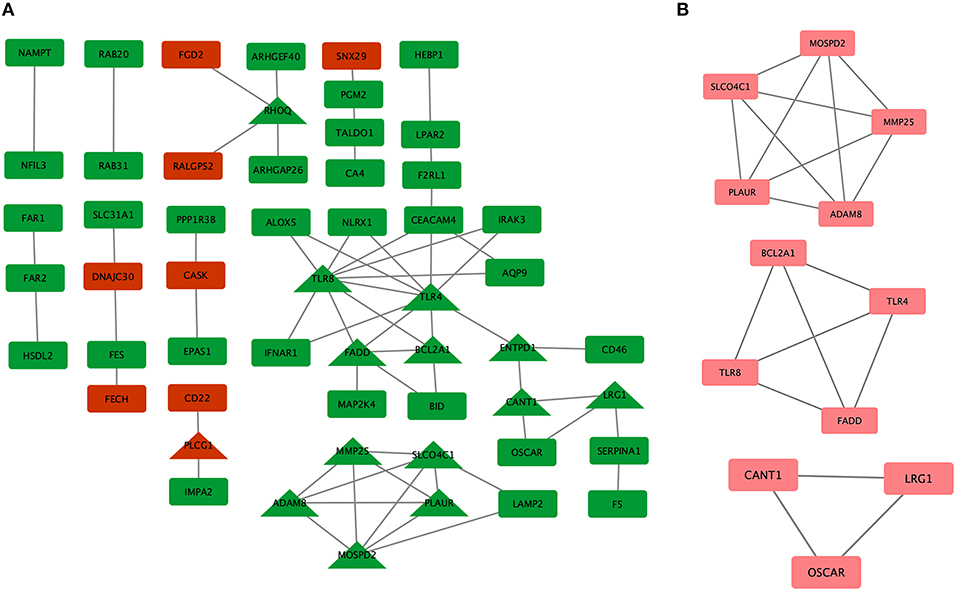
Figure 3. Protein-protein interaction (PPI) network of differentially expressed genes (DEGs) in major depressive disorder (MDD) samples. (A) Triangular nodes represent hub genes; red nodes represent upregulated genes; green nodes represent downregulated genes. (B) The most significant module was obtained from the PPI network with 12 nodes and 19 edges.
The hub genes may play significant key roles in signal transduction during the progression of MDD, which were determined from the PPI network using the cytoHubba plugin (Figure 3A). A total of 14 genes were identified as hub genes. The gene symbols, full names, and implications of these hub genes are shown in Table 2.
Integrated Network Analysis of miRNA-mRNA Interactions
According to the hub genes identified previously, miRNA-target gene interaction pairs of reverse association were predicted by the miRTarBase and TargetScan databases, respectively. Based on the identified miRNA-mRNA pairs, we compared the interaction network containing 72 miRNA-mRNA pairs and visualized them with Cytoscape software. By comparing the targets of hub genes, PLCG1 was found to be a potential target of 20 miRNAs, including hsa-miR-218, hsa-miR-1, hsa-miR-30*, hsa-miR-320a, hsa-miR-200*, hsa-miR-331, hsa-miR-369, hsa-miR-429*, and hsa-miR-34*. Moreover, MOSPD2 and ENTPD1 were the potential targets of 8 miRNAs and 13 miRNAs, respectively. The miRNA-gene regulation network is presented in Figure 4.
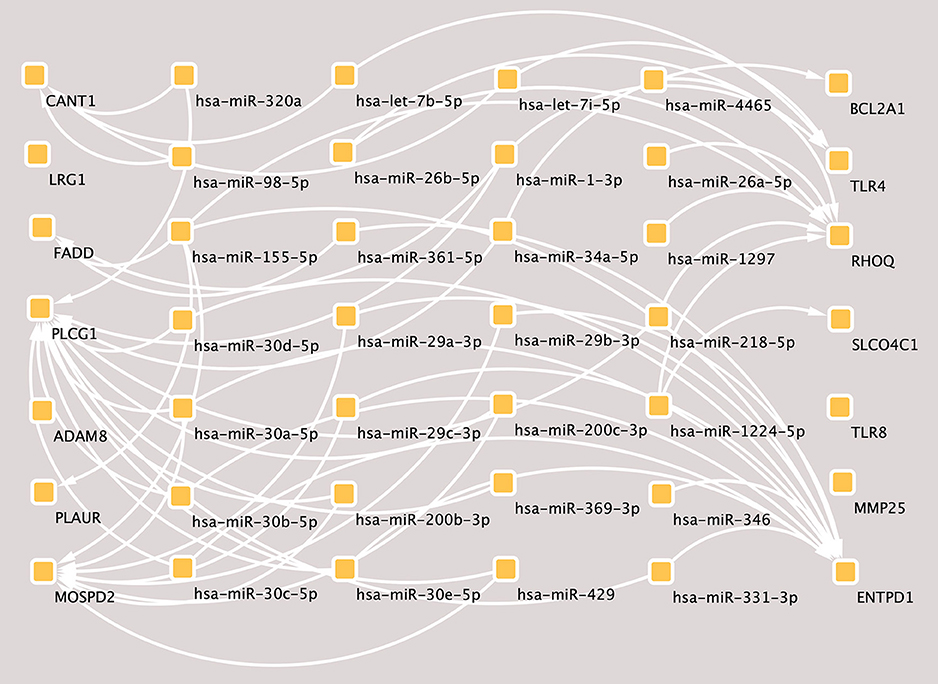
Figure 4. The miRNA-mRNAs regulatory network in major depressive disorder (MDD). Solid lines indicate interaction associations between the miRNAs and mRNAs. miRNA, microRNA; mRNA, messenger RNA.
Discussion
Depression is a major human blight that has become a pervasive public health problem (3, 46). Despite the rising prevalence of MDD, we lack an understanding of the distinctive pathophysiology in contrast to many other brain disorders. At present, laboratory blood tests to support MDD diagnosis are not available, so diagnosing this disorder is more challenging than measuring height (47). Recently, however, genetic insights transformed a featureless landscape into one with real scientific toeholds (48). The rapidly developing and wide use of microarray technology has revealed thousands of genetic alterations during the progression of diseases, which may provide promising targets for the early diagnosis of mental illness (49). Thus, there is a great need to identify biomarkers and provide proof of principle for a translational approach to prioritize blood biomarkers of mood state in MDD samples. In the present study, we explored the crucial genes of blood biomarkers and pathways associated with MDD by bioinformatics methods. To achieve this, two mRNA microarray datasets were analyzed to obtain DEGs and hub genes between peripheral blood from patients with MDD and that from the control group. A total of 123 DEGs (25 co-upregulated genes and 98 co-downregulated genes) and 14 hub genes were identified between the two datasets. Then, the DEGs were subjected to functional and pathway enrichment analysis, and a PPI network was constructed and integrated network analysis of miRNA-mRNA interactions performed to enhance our understanding of the molecular mechanisms of MDD.
To analyze the functional and pathway enrichment of DEGs between the subject groups, significant GO BP terms and pathways were obtained, including corticosteroid and glucocorticoid receptor signaling pathways, regulation of cell-matrix adhesion, and mitochondrial membrane permeability involved in apoptotic processes in upregulated genes. Downregulated genes were associated with neutrophil activation involved in the immune response, degranulation and mediated immunity, positive regulation of immune response and interleukin-8 production. Because the neutrophil activation involved in the immune response appeared in the downregulated genes in the pathway analysis results, it could be involved in an important part of MDD. Surprisingly, we observed in the literature that depression is mostly correlated with both peripheral inflammatory processes and alterations in cellular immunity, mainly for cell-mediated immunity. To date, a large number of studies have demonstrated that depression has been associated with positive regulation of interleukin-8 production and immune response (18, 50, 51). Euteneuer et al. (52) revealed that patients with MDD exhibited higher neutrophil and monocyte counts and an increased neutrophil to lymphocyte ratio (NLR) than controls. They also found that lower anti-inflammatory activity was related to more severe somatic depressive symptoms. Although there have been few studies on the immune response and MDD, and it is still unknown how the immune response regulates the pathology of depression. According to our analysis results, we speculate that the cellular immunity system might take part in the progression of MDD.
Based on the KEGG pathway analysis, downregulated DEGs were enriched for the Toll-like receptor signaling pathway, complement and coagulation cascades, NOD-like receptor signaling pathway, hepatitis B, measles, and influenza A. Recent data have demonstrated that NOD-like receptor pyrin containing 3 (NLRP3) activation appears to bridge the gap between immune activation and metabolic danger signals or stress exposure, which are key factors in the pathogenesis of MDD and other psychiatric disorders. TLRs also seem to be present in humans, and recent studies showed that the mRNA expression of TLR3 and TLR4 was significantly increased in the dorsolateral prefrontal cortex (DLPFC) of depressed individuals compared with controls (53, 54). Further experiments at the transcription and protein expression levels suggest that TLR3 and TLR4 appear to be unique and important in brain functions (55). There is mostly evidence for Toll-like receptors (TLRs) in the brain that are associated with depression and suicide (53). Interestingly, the Clinical Practice Research Datalink (CPRD) study from the UK-based primary care database suggests that influenza A infections are associated with a moderately increased risk of developing depression (56).
A PPI network was constructed to investigate the interrelationship of the DEGs, and 14 hub genes were identified, including RHOQ, TLR8, TLR4, FADD, BCL2A1, ENTPD1, CANT1, LRG1, MMP25, SLCO4C1, ADAM8, MOSPD2, PLAUR, and PLCG1. In addition to PLCG1, all other genes were downregulated in the PPI network. Inflammation is not the only cause of depression and cannot explain its entire pathophysiology, but it is an important pathogenic factor that explains one possible mechanism of depression. The subnetwork of PLCG1, BCL2A1, TLR8, FADD, and TLR4 screened out from our study has been shown to play a role in inflammation (36–39). FADD and BCL2A1 were implicated in nonapoptotic cellular processes and emerged as new actors in innate immunity and inflammation. According to a previous study, the anti-inflammatory effects and TLR profiles are predictors of the response to antidepressant treatment in patients with MDD (36, 55). As a pattern recognition receptor, TLR4 has been shown to play a vital role in neuroinflammation. The TLR4-specific inhibitor Cli-095 markedly inhibited the upregulation of TLR4 in the hippocampus and prefrontal cortex, and improved chronic unpredictable mild stress-induced depression-like behaviors in mice (57). Another study showed that stress significantly increased the expression of TLR4 and NF-κB in the hippocampus, and this phenomenon could be attenuated in TLR4 knockout mice (58). A growing body of research indicates that inflammation plays a critical role in the etiology and pathophysiology of depression.
miRNAs are a group of endogenous non-coding RNA molecules that likely regulate ~30% of human protein coding genes (59). According to the miRNA-mRNA binding data from online prediction tools, we identified genuine human miRNA-mRNA target pairs of MDD. In the present study, PLCG1 was predicted to be a potential target of 20 differentially expressed miRNAs and was upregulated in MDD. Research has shown that BDNF-mediated PLCG1 signaling is required for the formation and function of inhibitory synapses, whereby the disruption of PLCG1 signaling in the hippocampus leads to such dysfunctions. Interestingly, a clinical study showed that 5-HT1A signaling through tyrosine kinase receptors activates PLC/protein kinase C (PKC) signaling, mediating the synaptogenesis and behavioral actions of anti-depressants (60). Furthermore, previous studies have demonstrated that PLAUR plays a role in inflammation, tissue regeneration and axonal regeneration within the central nervous system (CNS) (45). In the brain, the PLAUR/Rho system seems to promote axonal recovery following a synapse function injury (61), which may be a potential target for the development of therapeutic strategies. The binding of recombinant PLAUR activation of β1 integrin via low-density lipoprotein receptor-related protein-1 (LRP1) leads to activation of the Rho family small GTPase Rac1 and Rac1-induced axonal regeneration (62). Furthermore, the miRNA-mRNA target pair network identified that an integral membrane protein ecto-ATPase enzyme, belonging to the nucleoside triphosphate diphosphohydrolase family (ENTPD1), was potentially targeted by various differentially expressed miRNAs. There is evidence that long-term depression might be modulated by ATP and/or its dephosphorylated product adenosine, such as E-NTPDases (41), which might contribute to the neural basis for learning and memory mechanisms. Molecular and cellular studies have demonstrated that the expression of neurotrophic factors, particularly brain-derived neurotrophic factors, is important for synapse function and development (63, 64).
Limitations
The results of this study should be interpreted within the context of important limitations. First, our study utilized public data, but after screening of mRNA with clinical diagnostic and prognostic predictive value, it should be further explore the function of mRNA by in vitro and in vivo experiments. Second, the samples are from the peripheral blood cells of patients, so the associated analysis of miRNA/mRNAs in the brain regions with depression-related dysfunction may validate the data and strengthen the conclusion. Third, further validation studies could lead to additional insights into the disease process as well as the validation and identification of additional functional biomarker candidates for improved clinical diagnostic of MDD patients.
Conclusion
In summary, this comprehensive bioinformatic analysis has identified numerous useful molecular targets for the future investigation of the mechanisms and selection of biomarkers for MDD. Some important biological processes and pathways, including the corticosteroid and glucocorticoid receptor signaling pathways, the Toll-like receptor signaling pathway, the NOD-like receptor signaling pathway, the neutrophil activation involved in the immune response, as well as the hub genes working in these processes, may provide novel insights into the development and progression of MDD. Furthermore, the potential molecular mechanisms that have been identified simultaneously include innate immunity, neuroinflammation, and neurotrophic factors for synapse function and development. In addition, further molecular biological experiments will be performed by our team to confirm the function of the identified genes in MDD.
Data Availability Statement
The datasets generated for this study can be found in the Gene Expression Omnibus database (GEO, www.ncbi.nlm.nih.gov/geo/): GSE76826 and GSE98793.
Author Contributions
GZ and SX conceptualized and designed the article. ZZ, YZ, YW, JL, and JA analysed and interpreted the data. GZ drafted of the article. ZY, LS, and TS were responsible for critical revision of the article for important intellectual content. TS finally approved the article.
Funding
This study was supported by grants from the National Natural Science Foundation of China (No. 81630031), the Beijing Municipal Science and Technology Project (No. Z171100000117016), and Tianjin Municipal Science and Technology Project (No. 17ZXMFSY00100).
Conflict of Interest
The authors declare that the research was conducted in the absence of any commercial or financial relationships that could be construed as a potential conflict of interest.
References
1. Ferrari J, Charlson FJ, Norman RE, Flaxman AD, Patten SB, Vos T, et al. The epidemiological modelling of major depressive disorder: application for the Global Burden of Disease Study 2010. PLoS ONE. (2013) 8:e69637. doi: 10.1371/journal.pone.0069637
2. Busch Y, Menke A. Blood-based biomarkers predicting response to antidepressants. J Neural Transm. (2019) 126:47–63. doi: 10.1007/s00702-018-1844-x
3. Rotenstein LS, Ramos MA, Torre M, Segal JB, Peluso MJ, Guille C, et al. Prevalence of depression: depressive symptoms, and suicidal ideation among medical students. A systematic review and meta-analysis. JAMA. (2016) 316:2214–36. doi: 10.1001/jama.2016.17324
4. Conejero, Olie E, Calati R, Ducasse D, Courtet P. Psychological pain, depression, and suicide: recent evidences and future directions. Curr Psychiatry Rep. (2018) 20:33. doi: 10.1007/s11920-018-0893-z
5. Read JR, Sharpe L, Modini M, Dear BF. Multimorbidity and depression: a systematic review and meta-analysis. J Affect Disord. (2017) 221:36–46. doi: 10.1016/j.jad.2017.06.009
6. Kupferberg, Bicks L, Hasler G. Social functioning in major depressive disorder. Neurosci Biobehav Rev. (2016) 69:313–32. doi: 10.1016/j.neubiorev.2016.07.002
7. Fried EI, Epskamp S, Nesse RM, Tuerlinckx F, Borsboom D. What are ‘good' depression symptoms? Comparing the centrality of DSM and non-DSM symptoms of depression in a network analysis. J Affect Disord. (2016) 189:314–20. doi: 10.1016/j.jad.2015.09.005
8. Schnyder N, Panczak R, Groth N, Schultze-Lutter F. Association between mental health-related stigma and active help-seeking: systematic review and meta-analysis. Br J Psychiatry. (2017) 210:261–8. doi: 10.1192/bjp.bp.116.189464
9. Lin C, Lee SH, Huang CM, Chen GY, Ho PS, Liu HL, et al. Increased brain entropy of resting-state fMRI mediates the relationship between depression severity and mental health-related quality of life in late-life depressed elderly. J Affect Disord. (2019) 250:270–7. doi: 10.1016/j.jad.2019.03.012
10. Zweerings J, Zvyagintsev M, Turetsky BI, Klasen M, Konig AA, Roecher E, et al. Fronto-parietal and temporal brain dysfunction in depression: a fMRI investigation of auditory mismatch processing. Hum Brain Mapp. (2019) 40:3657–68. doi: 10.1002/hbm.24623
11. Pettersson A, Bostrom KB, Gustavsson P, Ekselius L. Which instruments to support diagnosis of depression have sufficient accuracy? A systematic review. Nord J Psychiatry. (2015) 69:497–508. doi: 10.3109/08039488.2015.1008568
12. Ferrua P, Giorgi R, da Rosa LC, do Amaral CC, Ghisleni GC, Pinheiro RT, et al. MicroRNAs expressed in depression and their associated pathways: a systematic review and a bioinformatics analysis. J Chem Neuroanat. (2019) 100:101650. doi: 10.1016/j.jchemneu.2019.101650
13. Takahashi M, Lim PJ, Tsubosaka M, Kim HK, Miyashita M, Suzuki K, et al. Effects of increased daily physical activity on mental health and depression biomarkers in postmenopausal women. J Phys Ther Sci. (2019) 31:408–13. doi: 10.1589/jpts.31.408
14. Serati M, Redaelli M, Buoli M, Altamura AC. Perinatal major depression biomarkers: a systematic review. J Affect Disord. (2016) 193:391–404. doi: 10.1016/j.jad.2016.01.027
15. Gururajan, Clarke G, Dinan TG, Cryan JF. Molecular biomarkers of depression. Neurosci Biobehav Rev. (2016) 64:101–33. doi: 10.1016/j.neubiorev.2016.02.011
16. Manchia M, Fanos V. Targeting aggression in severe mental illness: the predictive role of genetic, epigenetic, and metabolomic markers. Prog Neuropsychopharmacol Biol Psychiatry. (2017) 77:32–41. doi: 10.1016/j.pnpbp.2017.03.024
17. Lindqvist D, Dhabhar FS, James SJ, Hough CM, Jain FA, Bersani FS, et al. Oxidative stress, inflammation and treatment response in major depression. Psychoneuroendocrinology. (2017) 76:197–205. doi: 10.1016/j.psyneuen.2016.11.031
18. Herron JW, Nerurkar L, Cavanagh J. Neuroimmune biomarkers in mental illness. Curr Top Behav Neurosci. (2018) 40:45–78. doi: 10.1007/7854_2018_45
19. Liu CS, Adibfar A, Herrmann N, Gallagher D, Lanctot KL. Evidence for inflammation-associated depression. Curr Top Behav Neurosci. (2017) 31:3–30. doi: 10.1007/7854_2016_2
20. Miyata S, Kurachi M, Okano Y, Sakurai N, Kobayashi A, Harada K, et al. Blood transcriptomic markers in patients with late-onset major depressive disorder. PLoS ONE. (2016) 11:e0150262. doi: 10.1371/journal.pone.0150262
21. Leday GGR, Vertes PE, Richardson S, Greene JR, Regan T, Khan S, et al. Replicable and coupled changes in innate and adaptive immune gene expression in two case-control studies of blood microarrays in major depressive disorder. Biol Psychiatry. (2018) 83:70–80. doi: 10.1016/j.biopsych.2017.01.021
22. Tusher VG, Tibshirani R, Chu G. Significance analysis of microarrays applied to the ionizing radiation response. Proc Natl Acad Sci USA. (2001) 98:5116–21. doi: 10.1073/pnas.091062498
23. Ritchie ME, Phipson B, Wu D, Hu Y, Law CW, Shi W, et al. limma powers differential expression analyses for RNA-sequencing and microarray studies. Nucleic Acids Res. (2015) 43:e47. doi: 10.1093/nar/gkv007
24. Chen H, Boutros PC. VennDiagram: a package for the generation of highly-customizable Venn and Euler diagrams in R. BMC Bioinformatics. (2011) 12:35. doi: 10.1186/1471-2105-12-35
25. Chen EY, Tan CM, Kou Y, Duan Q, Wang Z, Meirelles GV, et al. Enrichr: interactive and collaborative HTML5 gene list enrichment analysis tool. BMC Bioinformatics. (2013) 14:128. doi: 10.1186/1471-2105-14-128
26. Kuleshov MV, Jones MR, Rouillard AD, Fernandez NF, Duan Q, Wang Z, et al. Enrichr: a comprehensive gene set enrichment analysis web server 2016 update. Nucleic Acids Res. (2016) 44:W90–7. doi: 10.1093/nar/gkw377
27. Gonzalez-Castro TB, Tovilla-Zarate CA, Genis-Mendoza AD, Juarez-Rojop IE, Nicolini H, Lopez-Narvaez ML, et al. Identification of gene ontology and pathways implicated in suicide behavior: systematic review and enrichment analysis of GWAS studies. Am J Med Genet B Neuropsychiatr Genet. (2019) 180:320–29. doi: 10.1002/ajmg.b.32731
28. Szklarczyk D, Morris JH, Cook H, Kuhn M, Wyder S, Simonovic M, et al. The STRING database in 2017: quality-controlled protein-protein association networks, made broadly accessible. Nucleic Acids Res. (2017) 45:D362–8. doi: 10.1093/nar/gkw937
29. Shannon P, Markiel A, Ozier O, Baliga NS, Wang JT, Ramage D, et al. Cytoscape: a software environment for integrated models of biomolecular interaction networks. Genome Res. (2003) 13:2498–504. doi: 10.1101/gr.1239303
30. Scardoni G, Tosadori G, Faizan M, Spoto F, Fabbri F, Laudanna C. Biological network analysis with CentiScaPe: centralities and experimental dataset integration. F1000Res. (2014) 3:139. doi: 10.12688/f1000research.4477.1
31. Agarwal V, Bell GW, Nam JW, Bartel DP. Predicting effective microRNA target sites in mammalian mRNAs. Elife. (2015) 4:1–38. doi: 10.7554/eLife.05005
32. Chou CH, Shrestha S, Yang CD, Chang NW, Lin YL, Liao KW, et al. miRTarBase update 2018: a resource for experimentally validated microRNA-target interactions. Nucleic Acids Res. (2018) 46:D296–302. doi: 10.1093/nar/gkx1067
33. Yang YR, Kang DS, Lee C, Seok H, Follo MY, Cocco L, et al. Primary phospholipase C and brain disorders. Adv Biol Regul. (2016) 61:80–5. doi: 10.1016/j.jbior.2015.11.003
34. Jang HJ, Yang YR, Kim JK, Choi JH, Seo YK, Lee YH, et al. Phospholipase C-gamma1 involved in brain disorders. Adv Biol Regul. (2013) 53:51–62. doi: 10.1016/j.jbior.2012.09.008
35. Mayer S, Kumar R, Jaiswal M, Soykan T, Ahmadian MR, Brose N, et al. Collybistin activation by GTP-TC10 enhances postsynaptic gephyrin clustering and hippocampal GABAergic neurotransmission. Proc Natl Acad Sci USA. (2013) 110:20795–800. doi: 10.1073/pnas.1309078110
36. Hung YY, Huang KW, Kang HY, Huang GY, Huang TL. Antidepressants normalize elevated Toll-like receptor profile in major depressive disorder. Psychopharmacology. (2016) 233:1707–14. doi: 10.1007/s00213-015-4087-7
37. Wang L, Chen J. [Progress in studies on TLR4 signaling pathway and major depressive disorder]. Zhong Nan Da Xue Xue Bao Yi Xue Ban. (2017) 42:725–9. doi: 10.11817/j.issn.1672-7347.2017.06.020
38. Garcia-Fuster MJ, Diez-Alarcia R, Ferrer-Alcon M, La Harpe R, Meana JJ, Garcia-Sevilla JA. FADD adaptor and PEA-15/ERK1/2 partners in major depression and schizophrenia postmortem brains: basal contents and effects of psychotropic treatments. Neuroscience. (2014) 277:541–51. doi: 10.1016/j.neuroscience.2014.07.027
39. Zhang C, Wu Z, Hong W, Wang Z, Peng D, Chen J, et al. Influence of BCL2 gene in major depression susceptibility and antidepressant treatment outcome. J Affect Disord. (2014) 155:288–94. doi: 10.1016/j.jad.2013.11.010
40. Yegutkin GG. Enzymes involved in metabolism of extracellular nucleotides and nucleosides: functional implications and measurement of activities. Crit Rev Biochem Mol Biol. (2014) 49:473–97. doi: 10.3109/10409238.2014.953627
41. Cognato GP, Vuaden FC, Savio LE, Bellaver B, Casali E, Bogo MR, et al. Nucleoside triphosphate diphosphohydrolases role in the pathophysiology of cognitive impairment induced by seizure in early age. Neuroscience. (2011) 180:191–200. doi: 10.1016/j.neuroscience.2011.01.065
42. Zhan Y, Yang YT, You HM, Cao D, Liu CY, Zhou CJ, et al. Plasma-based proteomics reveals lipid metabolic and immunoregulatory dysregulation in post-stroke depression. Eur Psychiatry. (2014) 29:307–15. doi: 10.1016/j.eurpsy.2014.03.004
43. Naus S, Richter M, Wildeboer D, Moss M, Schachner M, Bartsch JW. Ectodomain shedding of the neural recognition molecule CHL1 by the metalloprotease-disintegrin ADAM8 promotes neurite outgrowth and suppresses neuronal cell death. J Biol Chem. (2004) 279:16083–90. doi: 10.1074/jbc.M400560200
44. Di Mattia T, Tomasetto C, Alpy F. [MOSPD2, an inter-organelle connector]. Med Sci. (2019) 35:23–5. doi: 10.1051/medsci/2018313
45. Ozpercin PU, Kendirlioglu BK, Sozen S, Yuksel O, Cihnioglu R, Kalelioglu T, et al. Decreased circulating urokinase plasminogen activator receptor (uPAR) concentration in acute episodes of bipolar disorder; could it be a reflection of axonal injury? Psychoneuroendocrinology. (2018) 90:122–6. doi: 10.1016/j.psyneuen.2018.02.009
46. Gonzalez-Forteza C, Torre AEH, Vacio-Muro MLA, Peralta R, Wagner FA. [Depression among adolescents: a hidden problem for public health and clinical practice]. Bol Med Hosp Infant Mex. (2015) 72:149–55. doi: 10.1016/j.bmhimx.2015.05.006
48. Hyman S. Mental health: depression needs large human-genetics studies. Nature. (2014) 515:189–91. doi: 10.1038/515189a
49. Bian Y, Yang L, Zhao M, Li Z, Xu Y, Zhou G, et al. Identification of key genes and pathways in post-traumatic stress disorder using microarray analysis. Front Psychol. (2019) 10:302. doi: 10.3389/fpsyg.2019.00302
50. Memon A, Sundquist K, Ahmad A, Wang X, Hedelius A, Sundquist J. Role of IL-8, CRP and epidermal growth factor in depression and anxiety patients treated with mindfulness-based therapy or cognitive behavioral therapy in primary health care. Psychiatry Res. (2017) 254:311–16. doi: 10.1016/j.psychres.2017.05.012
51. Peirce JM, Alvina K. The role of inflammation and the gut microbiome in depression and anxiety. J Neurosci Res. (2019) 97:1223–41. doi: 10.1002/jnr.24476
52. Euteneuer F, Dannehl K, Del Rey A, Engler H, Schedlowski M, Rief W. Peripheral immune alterations in major depression: the role of subtypes and pathogenetic characteristics. Front Psychiatry. (2017) 8:250. doi: 10.3389/fpsyt.2017.00250
53. Pandey GN, Rizavi HS, Ren X, Bhaumik R, Dwivedi Y. Toll-like receptors in the depressed and suicide brain. J Psychiatr Res. (2014) 53:62–8. doi: 10.1016/j.jpsychires.2014.01.021
54. Pandey GN, Rizavi HS, Bhaumik R, Ren X. Innate immunity in the postmortem brain of depressed and suicide subjects: role of toll-like receptors. Brain Behav Immun. (2019) 75:101–11. doi: 10.1016/j.bbi.2018.09.024
55. Hung YY, Kang HY, Huang KW, Huang TL. Association between toll-like receptors expression and major depressive disorder. Psychiatry Res. (2014) 220:283–6. doi: 10.1016/j.psychres.2014.07.074
56. Bornand D, Toovey S, Jick SS, Meier CR. The risk of new onset depression in association with influenza–A population-based observational study. Brain Behav Immun. (2016) 53:131–37. doi: 10.1016/j.bbi.2015.12.005
57. Fu S, Wang J, Hao C, Dang H, Jiang S. Tetramethylpyrazine ameliorates depression by inhibiting TLR4-NLRP3 inflammasome signal pathway in mice. Psychopharmacology. (2019) 236:2173–85. doi: 10.1007/s00213-019-05210-6
58. Cheng Y, Pardo M, Armini RS, Martinez A, Mouhsine H, Zagury JF, et al. Stress-induced neuroinflammation is mediated by GSK3-dependent TLR4 signaling that promotes susceptibility to depression-like behavior. Brain Behav Immun. (2016) 53:207–22. doi: 10.1016/j.bbi.2015.12.012
59. Ha M, Kim VN. Regulation of microRNA biogenesis. Nat Rev Mol Cell Biol. (2014) 15:509–24. doi: 10.1038/nrm3838
60. Albert PR, Vahid-Ansari F. The 5-HT1A receptor: signaling to behavior. Biochimie. (2019) 161:34–45. doi: 10.1016/j.biochi.2018.10.015
61. Merino P, Diaz A, Yepes M. Urokinase-type plasminogen activator (uPA) and its receptor (uPAR) promote neurorepair in the ischemic brain. Receptors Clin Investig. (2017) 4:e1552.
62. Gonias SL, Gaultier A, Jo M. Regulation of the urokinase receptor (uPAR) by LDL receptor-related protein-1 (LRP1). Curr Pharm Des. (2011) 17:1962–9. doi: 10.2174/138161211796718224
63. Duman RS, Li N. A neurotrophic hypothesis of depression: role of synaptogenesis in the actions of NMDA receptor antagonists. Philos Trans R Soc Lond B Biol Sci. (2012) 367:2475–84. doi: 10.1098/rstb.2011.0357
Keywords: major depressive disorder (MDD), Gene Expression Omnibus (GEO), hub genes, enrichment analysis, protein-protein interaction network (PPI)
Citation: Zhang G, Xu S, Zhang Z, Zhang Y, Wu Y, An J, Lin J, Yuan Z, Shen L and Si T (2020) Identification of Key Genes and the Pathophysiology Associated With Major Depressive Disorder Patients Based on Integrated Bioinformatics Analysis. Front. Psychiatry 11:192. doi: 10.3389/fpsyt.2020.00192
Received: 18 November 2019; Accepted: 27 February 2020;
Published: 03 April 2020.
Edited by:
Shaohua Hu, Zhejiang University, ChinaReviewed by:
George P. Chrousos, National and Kapodistrian University of Athens, GreeceTaolin Chen, Sichuan University, China
Xiang Yang Zhang, University of Texas Health Science Center at Houston, United States
Copyright © 2020 Zhang, Xu, Zhang, Zhang, Wu, An, Lin, Yuan, Shen and Si. This is an open-access article distributed under the terms of the Creative Commons Attribution License (CC BY). The use, distribution or reproduction in other forums is permitted, provided the original author(s) and the copyright owner(s) are credited and that the original publication in this journal is cited, in accordance with accepted academic practice. No use, distribution or reproduction is permitted which does not comply with these terms.
*Correspondence: Tianmei Si, c2kudGlhbi1tZWlAMTYzLmNvbQ==