- 1Center for Integrative Addiction Research (CIAR), Grüner Kreis Society, Vienna, Austria
- 2University Clinic for Psychiatry and Psychotherapeutic Medicine, Medical University Graz, Graz, Austria
- 3Institute for Religious Studies, University of Vienna, Vienna, Austria
- 4Institute of Psychology, University of Graz, Graz, Austria
Substance use disorders (SUDs) are defined by obsessive and uncontrolled consumption, which is related to neurobiological changes. Based on previous work, this study investigated potential alterations in brain structure in poly-drug use disordered (PUD) patients in comparison to controls from the normal population. This study involved a sample of 153 right-handed men aged between 18 and 41 years, comprising a clinical group of 78 PUD and a group of 75 healthy controls. Group differences in gray matter (GM) and white matter (WM), as well as cortical thickness (CT), were investigated by means of diffusion tensor imaging using automated fiber quantification (AFQ) and voxel-based morphometry. We observed significant WM impairments in PUD, especially in the bilateral corticospinal tracts and the inferior longitudinal fasciculi. Furthermore, we found reduced CT in the PUD group especially in the left insular and left lateral orbitofrontal cortex. There were no group differences in GM. In addition, PUD exhibited a higher amount of psychiatric symptoms (Brief Symptom Inventory) and impairments in cognitive functions (Wonderlic Personnel Test). In line with previous research, this study revealed substantial impairments in brain structure in the PUD group in areas linked with affective, cognitive, and motor functions. We therefore hypothesize a neurologically informed treatment approach for SUD. Future studies should consequently explore a potential positive neuroplasticity in relation to a better therapeutic outcome.
Introduction
Within the European Union, a lifetime prevalence of up to 3% for substance use disorders (SUDs) has been shown for the general population (1, 2). Correspondingly, SUD represent a significant burden on society and healthcare systems. In addition to this, the treatment of SUD has been reported to be extremely difficult due to a high proportion of therapy dropouts (3). SUD have been most prominently described as a chronic, relapsing brain disorder characterized by compulsive drug use, which produces long-term changes in the reward circuitry of the brain (4–6). Therefore, it is now widely accepted that many drugs may “hijack” the reward centers of the brain, setting in motion a downward spiral towards SUD (7). Notably, some authors have challenged this view by arguing that the complex mechanisms underlying SUD cannot be explained by neural dysfunction alone (8). In that sense, SUD have also been widely discussed in relation to dysfunctional attempts of self-medication (9) and misled attachment needs (10). Furthermore, it should be noted that premorbid brain abnormalities might also lead to severe psychiatric disturbances such as SUD (11, 12).
From a developmental perspective, childhood and adolescence represent critical periods of cortical development related to lifelong adult characteristics. This development is likely interrupted by drug misuse since most people usually start abusing drugs in puberty (13). Although acute drug intake increases dopamine neurotransmission, chronic drug consumption results in a significant decline of dopamine activity, associated with, among other things, dysregulation of the orbitofrontal cortex and the cingulate gyrus (14), which in turn is linked to maladaptive decision making (15) and increased drug craving (16) in SUD. However, because SUD patients usually show a more or less haphazard kind of poly-drug use, it is as yet largely unclear which detrimental effects are caused by the abuse of which drug (17, 18). In addition, all drugs have similar direct or indirect effects on the mesolimbic reward system. This system extends from the ventral tegmentum to the nucleus accumbens and projects to areas such as the limbic system and the orbitofrontal cortex (6).
Furthermore, different kinds of drugs have been observed as being associated with impairments of white matter (WM) (19) as well as gray matter (GM) (20, 21) in the brain. Here, a special focus has been placed on the detrimental effects of drug use (especially cannabis) on brain structure and functioning in adults and adolescents (22, 23). There is substantial evidence that heavy substance abuse might be particularly harmful to the development of WM during adolescence (19, 24–27). Correspondingly, cognitive deficits were reported in a group of methamphetamine users, which in turn were related to lower whole-brain cortical thickness (CT) (12). Therefore, chronic drug use might cause deficits in and/or a failure to develop normative cognitive abilities (12).
Numerous studies on SUD have observed positive as well as negative neuroplasticity, which generally means the alteration of the brain’s structure, as the result of various learning templates (28–30). This is consistent with the assumption that SUD represent a pathological but powerful form of learning and memory (31). Notably, numerous studies on structural neural parameters in SUD has shown impairments in various networks of the brain (Hiebler-Ragger et al., submitted, 32–34), in particular those linked with frontal volitional control and the reward-salience centers (35). In correspondence to this, recovery from SUD was observed to correlate with positive neuroplasticity, such as the return to more gyral volumes (36) and enlargement of GM after mindfulness therapy (37). Taken together, a broad knowledge of the neurobiological alterations linked with SUD, along with the brain networks associated with successful abstinence, can hence improve our understanding of SUD and its treatment in general (17).
To date, most of the research in this area has investigated the role of WM, GM, or CT and their relation to SUD independently from one another, resulting in a rather isolated picture of findings on structural brain deficits in poly-drug use disorder (PUD). Therefore, the goal of the present study was a comprehensive investigation of potential differences in WM, GM, and CT in a large sample of PUD patients compared to healthy controls. Following recent developments in the field of WM analysis, we used automated fiber quantification (AFQ) for a more detailed assessment of WM differences between PUD patients and controls. The particular strength of this study could be seen in the application of a multimodal imaging approach, assessing different characteristics of brain structure and related functions within one and the same sample of participants. Such an approach is particularly motivated by the fact that different characteristics of GM or WM morphology (such as CT, GM volume, or myelination), each of them subserving different cognitive, affective, and motor functions, may be affected in PUD in different ways. On the basis of previous work indicating that heavy substance abuse might be particularly harmful to the development of WM during adolescence (19, 24–27), and on the basis of our previous studies with PUD patients (33, 34), we expected substantial differences especially in WM integrity between PUD patients and the control group from the normal population. Available evidence (12, 20, 21) leads us to assume that different parameters of GM morphology (volume and CT) are affected as well.
Methods and Materials
Participants
A total sample of 153 right-handed men between 18 and 41 years of age, composed of one clinical and one nonclinical group, was investigated. This sample integrated data from three different studies related to other research questions regarding PUD (Hiebler-Ragger et al., submitted, 33, 34). In detail, 45 participants (PUD patients: n = 29) were included from the first study (34) that focused on WM integrity in relation to attachment and personality. Sixty-five participants (PUD patients: n = 25) were included from the second study (33) that focused on WM integrity in relation to negative affective states, and 43 participants (PUD patients: n = 24) were included from the second study (Hiebler-Ragger et al., submitted) that focused on neural activation during emotion regulation efforts. Data acquisition took place over a time span of 4 years, starting in January 2014 and ending in November 2016. The clinical group (n = 78) was diagnosed for PUD (F19.2) by a licensed psychiatrist (a medical doctor specialized in psychiatry with 20 years of experience of treating SUD patients) according to the International Classification of Diseases version 10 (38). The nonclinical comparison group was comprised of students from various faculties (CG; n = 75). Students were included in the nonclinical groups if they were free from any past or present psychiatric disorder or chronic disease. With regards to the use of psychotropic substances, CG included 47 nonsmoking students who reported either no experience with illegal substances or to have tried them just a few times in their life, as well as 28 nicotine smoking students who reported using illegal substances primarily for recreation at least once a week during the last month. Psychometric assessment of the clinical participants took place in two therapeutic facilities of the “Grüner Kreis” society, where these participants were undergoing long-term SUD treatment based on the “Therapeutic Community” concept (39). The “Grüner Kreis” society (founded in 1983) is Austria’s biggest institution for long-term drug therapy. Usually, the patients stay from 6 to 18 months within the Therapeutic Community. All behavioral assessments were conducted via group testing. Participants’ consent was obtained according to the Declaration of Helsinki. Individuals were only included in the study if they did not report general MRI contraindications (e.g., head injuries, metal implants), major physical disorders, or severe cognitive impairments including acute psychotic episodes. The study was approved by the authorized ethics committee. See Table 1 for detailed demographic information.
MRI Acquisition
Imaging data were acquired on a 3T Siemens Skyra (Siemens Healtheneers, Erlangen, Germany) with a 32-channel head coil. Since the sample of this study consists of three different studies, two different sequence protocols were used, with slight variations in sequencing parameters. For all participants, T1-weighted images as well as diffusion-weighted images were acquired. Details of imaging parameters are itemized in Table 2.
MRI Data Preprocessing and Analysis
Diffusion
Data preprocessing was performed using the software package MRtrix (40) and FSL (41). First, data were visually inspected for artifacts and then denoised with the MRtrix command “dwidenoise” (42). Estimation and correction of geometric distortion was carried out with FSL’s “top up” and “eddy” using the nondiffusion-weighted images (b value = 0) collected with reverse-phase encoding direction (43). Datasets with no reverse encoding direction image available were corrected with eddy_correct. Next, individual B0 images were coregistered to the structural image using SPM12 (v7219; Wellcome Trust Centre for Neuroimaging). The coregistered T1-images were then segmented into five tissues using the “5ttgen” algorithm (44). This step is necessary to allow the estimation of the response function for each tissue-class separately. The response function was estimated for GM, WM, and cerebrospinal fluid. Fiber orientation distributions (FODs) were computed using these multitissue-constrained spherical deconvolutions (45). FODs were then used to compute whole-brain fiber tractography with 5 million tracks. As a last preprocessing step, the scale-invariant feature transform algorithm was used to reduce tractogram biases (46) reducing the number of tracks to 1 million.
Tract Quantification
Whole-brain tractography data were imported into the AFQ software package (https://github.jyeatman/AFQ) (47) running on MATLAB (2017b, The Mathworks, Natick, MA, USA), which identifies 20 major fiber tracts, including the right and left thalamic radiations, forceps major and minor of corpus callosum, right and left inferior fronto-occipital, inferior longitudinal, arcuate and uncinate fasciculi, corticospinal tract, and cingulum. To assess differences in tensor-based indices along each pathway, whole-brain tractography was normalized into the MNI space, and each fiber pathway was evenly spaced into 100 cross-sectional nodes. The mean fractional anisotropy (FA) in each node was calculated, and group differences were analyzed for each node within each pathway. Multiple comparison corrections were conducted using the AFQ software package script AFQ_MultiCompCorrection.m, which is based on Nichols and Holmes (48). Using this script, the family wise error corrected alpha value for pointwise comparison was computed for each tract to correct for multiple comparison. As a result, p values below a threshold of <0.0025 (0.05/20 tracts) were considered significant.
Voxel-Based Morphometry
Structural scans were analyzed using the Computational Anatomy Toolbox (CAT12; r 1274) implemented in SPM12, running under Matlab 2017b, to assess voxel-wise comparison of GM volume (GMV) differences. Data were visually checked and the segmented, modulated, and normalized into the MNI space (1.5mm). The sample homogeneity was checked, and the total intracranial volume (TIV) was estimated. Finally, data were smoothed with a Gaussian kernel with a full width at half maximum of 8 mm.
Cortical Thickness
The CAT12 toolbox was again used to extract CT. This fully automated method uses tissue segmentation as already done in the voxel-based morphometry (VBM) analysis and uses a projection-based algorithm to compute CT (49). Finally, surface data were smoothed with a Gaussian kernel with a full width at half maximum of 15 mm.
For statistical analysis of GMV and CT parametric-free permutation tests (TFCE toolbox, number of permutations = 10,000) were used. Age and TIV (only VBM) were included in the statistical model as regressors of no interest. Results were considered statistically significant with p < 0.05 corrected for family-wise error.
Behavioral Measures
Psychiatric Symptoms
The Brief Symptom Inventory-18 (BSI-18) (German adaptation by (50) is a short version of the highly established Symptom Checklist SCL-90-R (51). The amount of psychiatric burden for the preceding 7 days for three dimensions of psychiatric symptoms (Somatization, Depressiveness, and Anxiety) is assessed by means of 18 items (6 items for each subscale). The BSI-18 employs a 5-point rating form ranging from 1 (absolutely not) to 5 (very strong). It is also possible to sum up the 18 items into a total score: The Global Severity Index (GSI) of psychiatric symptoms. In previous research, Cronbach’s alpha was observed to be at least 0.79 for all the subdimensions (33). See Table 1 for details.
Cognitive Ability
Participants also completed the Wonderlic Personnel Test (WPT), a rough screening instrument for the assessment of intelligence (52). This test requires the processing of disordered sentences, analogies, number series, word and sentence comparisons, and geometrical figures within a given time period of 12 min. The WPT contains 50 items with increasing difficulty. The total score is generated from the number of correct responses. See Table 1 for details.
Behavioral Data Analysis
For group comparisons, one-way analyses of variance were conducted. Post hoc comparisons were conducted with Tukey’s honest significant difference test. Pearson`s correlations were calculated to investigate the relationship between neural and behavioral parameters. Alpha was set to p < 0.05. Eta squared (η2) is given as estimate of effect sizes.
Data Availability
The datasets generated during and/or analyzed during the current study are available from the corresponding author on reasonable request.
Results
Demographics and Clinical Characteristics
As shown in Table 1, PUD patients were older than the controls (PUD: M = 28.71, SD = 5.15; CG: M = 25.28, SD = 3.37; p < 0.001; η² = 0.14). Analyses also revealed significant differences in education, with the CG reporting an average of 14 years (SD = 2.82) of education, whereas the PUD patients’ average was 12 years (SD = 2.58) of education (p < 0.001; η² = 0.17). At the time of data acquisition, the PUD patients were undergoing inpatient SUD treatment within a therapeutic community for a mean time of 25 weeks (SD = 18.46). They reported a history of drug abuse over an average period of 12 years (SD = 5.57; range, 2–27 years; missing values, 24). Forty-eight PUD patients were undergoing maintenance therapy, while 30 PUD participants reported living in abstinence. Fifty-three PUD patients received psychopharmacological medication (antidepressant: n = 20; antipsychotic: n = 23; anxiolytic: n = 5; other: n = 20).
PUD exhibited a significantly higher amount of Depressiveness (p < 0.01; η² = 0.10), Somatization (p < 0.05; η² = 0.04) as well as a higher score for the total Global Severity Index in the BSI-18 (p < 0.01; η² = 0.07). Accordingly, PUD patients showed no differences when compared to normative data for psychiatric inpatients (50), while CG participants exhibited less Depressiveness and a lower score in the Global Severity Index (for both p < 0.01). The intercorrelations between demographic and behavioral parameters in PUD can be retrieved from Table 3. Age was positively related to cognitive abilities (p < 0.01) as well as depression (p < 0.05) and the duration of treatment (p < 0.05).
Differences in White Matter, Gray Matter, and Cortical Thickness Between PUD and Controls
White Matter Fiber Tracts
As shown in Figure 1, PUD patients exhibited significant reductions in FA relative to controls across the entire left and the majority of nodes of the right corticospinal tract. In addition, there were significant FA reductions in posterior portions of the bilateral inferior longitudinal fasciculi and in smaller portions of the left thalamic radiation, the right inferior fronto-occipital fasciculus, and the right arcuate fasciculus.
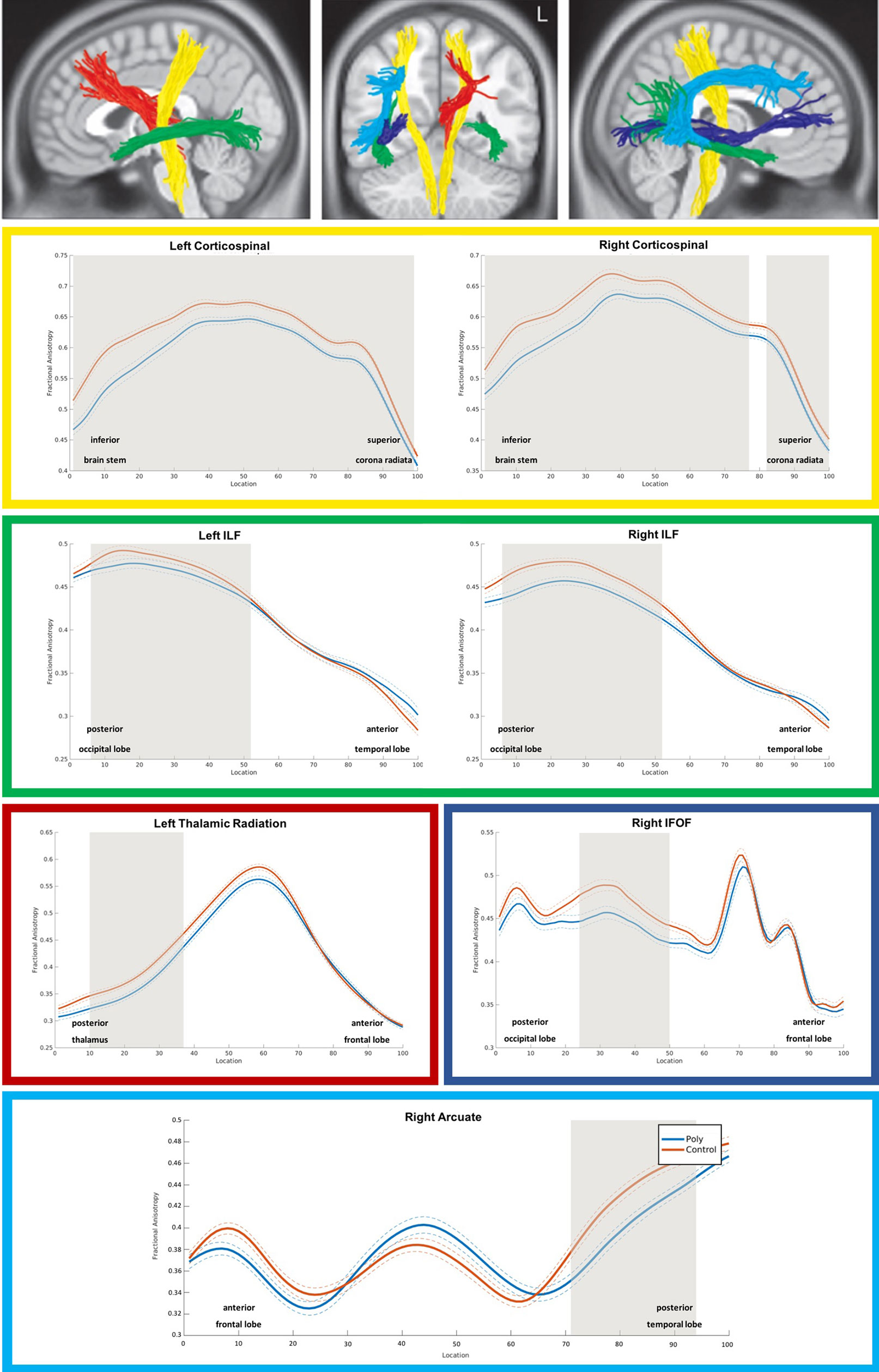
Figure 1 3D visualization and tract diffusion profiles for white matter fiber tracts showing significant differences between PUD and controls. Note. Yellow = left and right corticospinal tract; green = left and right inferiore longitudinale fasciculus (ILF); red = left thalamic radiation; blue = right inferior fronto-occipital fasciculus IFOF; cyan = right arcuate fasciculus; shades of gray in the profiles indicate nodes with significant group differences. PUD, patients with poly-drug use disorder.
Gray Matter Volume
Voxel-based morphometry analyses revealed no significant differences in GMV between PUD and controls.
Cortical Thickness
Analyses revealed brain regions with significant reductions of CT in PUD relative to controls, while there were no brain regions with higher CT in the patient group (see Figure 2). The largest cluster comprised the left insular and the left lateral orbitofrontal cortex. There were also significant CT reductions in the right orbitofrontal cortex. Generally, as is the case for the lateral orbitofrontal cortex, CT reductions were bilateral. This particularly applies to regions of the inferior frontal gyri (pars opercularis) and the precentral gyri. In addition, analyses revealed CT reductions in a cluster involving the left postcentral gyrus and small portions of the supramarginal gyrus in addition to a cluster in the right inferior temporal lobe.
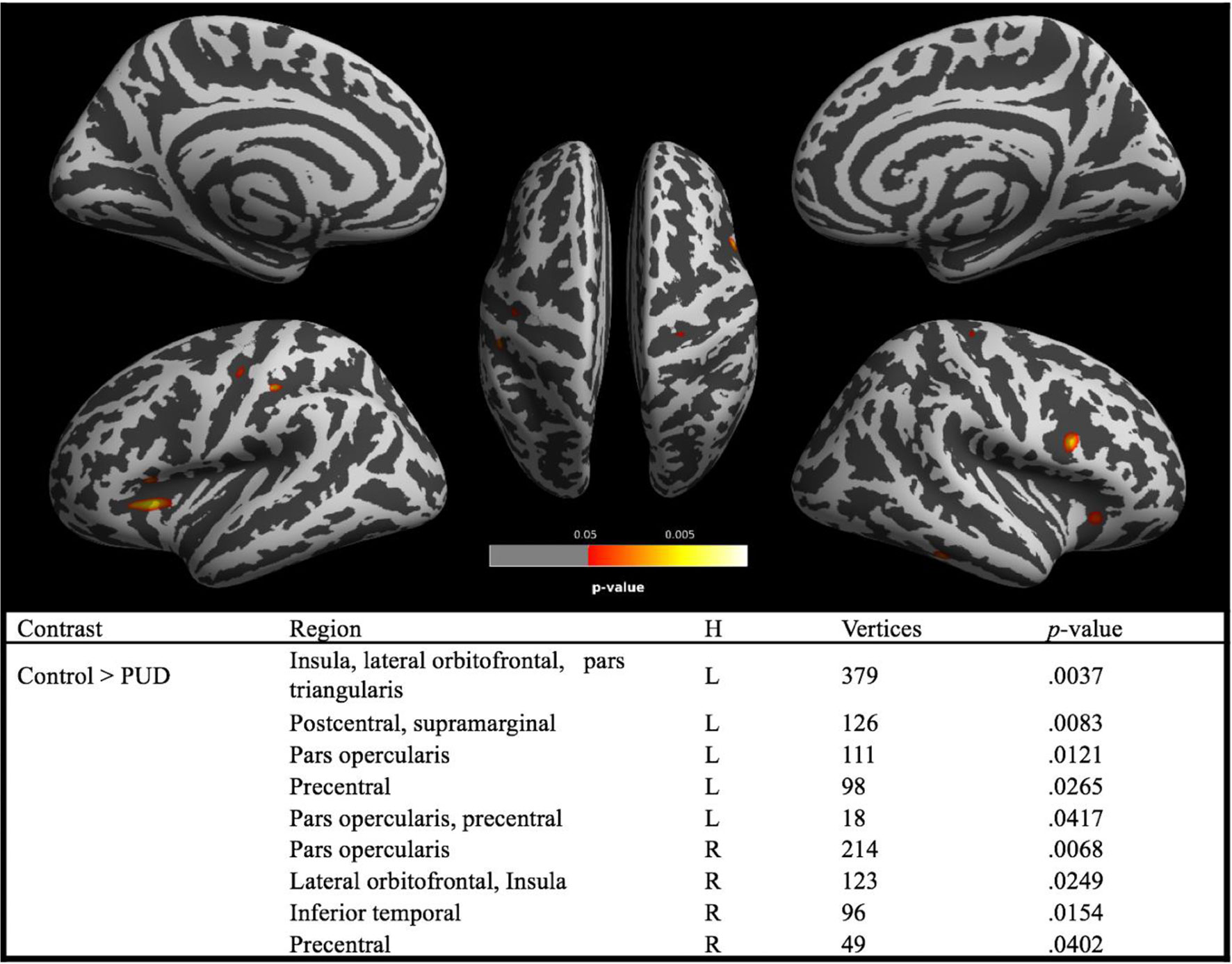
Figure 2 Brain regions with significant (red–yellow) group differences in cortical thickness between PUD and controls. Note. PUD, poly-drug users; H, hemisphere; L, left; R, right; brain regions are derived from Desikan–Killiany DK40 Atlas. Reported p values are TFCE corrected for family-wise error (p < 0.05).
Discussion
This study investigated alterations in brain structure in an unprecedented large sample of PUD patients compared to controls from the general population. Analyses revealed impaired WM integrity along with reduced CT in the PUD sample but no alterations in GM. These findings were mirrored by significant differences between PUD and healthy controls regarding behavioral measures, such as a higher amount of psychiatric symptom burden as well as lower cognitive abilities. Furthermore, our results confirm previous research indicating substantial deficits especially in WM circuitry in PUD patients (25, 26, 33, 34, 53).
Deficits in WM structure might represent a valid predictor for negative therapeutic outcome. For instance, Moeller et al. (44) reported that deficits in WM integrity are related to an increased amount of impulsivity in cocaine-dependent patients. A high amount of impulsivity has been widely shown as being a risk factor for the development of SUD (54) as well as a substantial predictor for a negative SUD therapy outcome (55). Furthermore, we observed lower CT in PUD patients, which has been linked to higher memory deficits (12) as well as reduced effortful attention performance (56). CT abnormalities have been observed to be associated with SUD such as alcohol dependence (57), marijuana misuse (58), and nicotine smoking (59), as well as nonsubstance-related disorders such as excessive internet use (60) and online gaming (61). Moreover, significant abnormalities in CT were reported in individuals with heavy prenatal alcohol exposure. These abnormalities were found to be linked with impairments in verbal recall and visuospatial dysfunction (62).
Notably, we did not find any significant differences in GM between PUD patients and healthy controls. This is in clear contrast to previous research, where disrupted GM was observed to be related with several SUD, for example alcoholism (63), cocaine use (20), and cannabis use (23). We interpret our conflicting findings as showing that, in our rather young PUD sample, WM paths might be among the first to become affected by PUD. In fact, previous work suggested that heavy substance abuse might be particularly harmful to the development of WM during adolescence (19, 24–27). In this regard, it is conceivable that, in its earlier stages, PUD already compromises more basic “hardware” processes, e.g., motor functions as indicated by the substantial WM deficits in the bilateral corticospinal tract, while further impairments in higher order cognitive functions only result after prolonged consumption. Accordingly, at this point, we can only assume that, later in life, GM might become damaged, too. For instance, in a study of Qiu et al. (64), the mean age of the group of heroin dependents was considerably higher (M = 35 years, SD = 4.2). Here, the authors reported a progressive deterioration of WM microstructure dependent on the duration of heroin use.
From a developmental perspective, the finding of diminished CT in PUD fits nicely with the literature. For instance, Hilton Jr (29) described lower CT as a kind of premorbid cortical weakness, which leads to poor cognitive performance and could pave the way to develop a SUD later in life. In support of this notion, we observed a significantly lower level of cognitive ability, along with a lower educational status in the group of PUD patients. Even more importantly, in PUD patients, reduced CT, especially in regions of the insular and the orbitofrontal cortex, may suggest that these structural alterations mirror difficulties in affective processing, specifically emotional awareness (65) and emotional regulation (66), which are known to be compromised in SUD (67–69). This is further supported by the fact that we found pronounced WM impairments in the bilateral inferior longitudinal fasciculi and in the right inferior fronto-occipital fasciculus, which are both known as key components of a face processing network (70), with an important role in facilitating the ability to discriminate between emotional expressions in faces (71). In accordance with the general notion of impaired affective processing and emotion regulation in PUD, we previously observed a significantly reduced capacity for using cognitive reappraisal to regulate anger in PUD patients when compared to controls from the normal population (Hiebler-Ragger et al., submitted).
According to Kalivas and O’Brien (72), SUD is based on pathological changes in brain function, which are produced by a repeated pharmacological assault on the brain circuits that regulate how a person behaviorally responds to certain stimuli. Since recovery from SUD has been correlated with positive neuroplastic changes (36), neuroplasticity might therefore constitute a highly important indicator for the evaluation of therapeutic outcome. Especially for long-term treatment of SUD, neurologically informed therapeutic interventions may represent an important resource (33). Changes in cognitive and affective abilities in SUD patients during long-term treatment might be intertwined with neuroplastic effects (5, 10). Strikingly, (73) reported beneficial effects of transcranial stimulation (TMS) for the treatment of SUD, as TMS seems to facilitate long-term neurophysiological changes which have the potential to affect behaviors relating to drug craving, intake and relapse. Accordingly, a respective research focus on neuroplasticity in SUD patients may provide additional valuable information for the clinical outcome evaluation.
Limitations and Future Perspectives
The study of PUD populations has previously been discussed as being too unspecific (18). However, as a counter argument, from a clinical perspective, it is evident that a high rate of SUD patients is diagnosed with PUD because of a completely chaotic pattern of consumption (74). In this study, we did not further control for maintenance therapy since previous research revealed no differences in neural and behavioral parameters between PUD patients in maintenance therapy and abstinent patients (34). Furthermore, there was no perfect age match between the two groups, as the healthy control group was significantly younger than the SUD patients [3.43 years (see Table 1)]. However, we observed age to be weakly related with behavioral characteristics, such as Intelligence, Depression, and Duration of Treatment (Table 3). In addition, in the analysis of GM and CT, age was considered as a regressor of no interest in the statistical model. Additionally, in future research, potential gender differences might be considered another factor of study, as, for instance, Sawyer et al. (75) reported sex differences in alcoholism-related abnormalities of WM connectivity. Furthermore, in this study, we sought to focus primarily on potential differences between PUD patients and a nonsubstance use disordered control sample regarding the areas of WM and GM as well as CT, based on an enhanced sample. In further analysis, we intend to investigate potential connections between neural parameters and an extended set of behavioral parameters in PUD more in detail, which might reveal further insights concerning individual differences in PUD. These findings will be published somewhere else. The cross-sectional design in this study limits the possibilities of interpretation. Thus, we can only speculate on the causal relationship between impairments in brain structure and the development of PUD as well as on potential neuroplastic effects during PUD treatment. While the combined analyses of several neural and behavioral parameters in the rather large sample of this study reveals important insights into the clinical profile of PUD patients, a longitudinal research approach comprised of several measurement points is highly warranted in order to be able to say more about the clinical relevance of neuroplasticity for patient treatment.
Ethics Statement
This study was carried out in accordance with the recommendations of Ethic guidelines, Ethics Board, University of Graz; with written informed consent from all subjects. All subjects gave written informed consent in accordance with the Declaration of Helsinki. The protocol was approved by the Ethics board, University of Graz.
Author Contributions
HU and AF conceptualized the study. MH-R, JF, KK, KR, CP, HU, and AF collected, analyzed, and interpreted the data. HU and MH-R drafted the manuscript. CP, IP, EW, and AF critically reviewed the manuscript. All authors gave their final approval of the manuscript.
Conflict of Interest Statement
The authors declare that the research was conducted in the absence of any commercial or financial relationships that could be construed as a potential conflict of interest.
References
1. Alonso J, Angermeyer MC, Bernert S, Bruffaerts R, Brugha TS, Bryson H, et al. Prevalence of mental disorders in Europe: results from the European Study of the Epidemiology of Mental Disorders (ESEMeD) project. Acta Psychiatr Scand (2004) 109:21–7. doi: 10.1111/j.1600-0047.2004.00325.x
2. Rehm J, Room R, van den Brinkt W, Kraus L. Problematic drug use and drug use disorders in EU countries and Norway: an overview of the epidemiology. Eur Neuropsychopharmacol (2005) 15:389–97. doi: 10.1016/j.euroneuro.2005.04.004
3. Brorson HH, Arnevik EA, Rand-Hendriksen K, Duckert F. Drop-out from addiction treatment: a systematic review of risk factors. Clin Psychol Rev (2013) 33:1010–24. doi: 10.1016/j.cpr.2013.07.007
4. Ersche KD, Jones PS, Williams GB, Turton AJ, Robbins TW, Bullmore ET. Abnormal brain structure implicated in stimulant drug addiction. Science (2012) 335:601–4. doi: 10.1126/science.1214463
5. Koob GF, Volkow ND. Neurocircuitry of addiction. Neuropsychopharmacology (2010) 35:217. doi: 10.1038/npp.2009.110
6. Leshner AI. Addiction is a brain disease, and it matters. Science (1997) 278:45–7. doi: 10.1126/science.278.5335.45
7. Panksepp J, Nocjar C, Burgdorf J, Panksepp JB, Huber R. The role of emotional systems in addiction: a neuroethological perspective. In 50th Nebraska Symposium on Motivation, Mar, 2002, University of Nebraska, Lincoln, NE, US; Presented at the aforementioned symposium. University of Nebraska Press. (2004).
8. Levy N. Addiction is not a brain disease (and it matters). Front Psychiatry (2013) 4:24. doi: 10.3389/fpsyt.2013.00024
9. Khantzian EJ. The self-medication hypothesis of addictive disorders: focus on heroin and cocaine dependence. In: The cocaine crisis. New York: Springer (1987) p. 65–74. doi: 10.1007/978-1-4613-1837-8_7
10. Flores PJ. Addiction as an attachment disorder: Implications for group therapy. Int J Group Psychother (2001) 51:63–81. doi: 10.1521/ijgp.51.1.63.49730
11. Becker B, Wagner D, Koester P, Tittgemeyer M, Mercer-Chalmers-Bender K, Hurlemann R, et al. Smaller amygdala and medial prefrontal cortex predict escalating stimulant use. Brain (2015) 138:2074–86. doi: 10.1093/brain/awv113
12. Dean AC, Morales AM, Hellemann G, London ED. Cognitive deficit in methamphetamine users relative to childhood academic performance: link to cortical thickness. Neuropsychopharmacology (2018), 43:1745–52. 1. doi: 10.1038/s41386-018-0065-1
13. Crews F, He J, Hodge C. Adolescent cortical development: a critical period of vulnerability for addiction. Pharmacol Biochem Behav (2007) 86:189–99. doi: 10.1016/j.pbb.2006.12.001
14. Volkow ND, Fowler JS, Wang G-J, Swanson JM. Dopamine in drug abuse and addiction: results from imaging studies and treatment implications. Mol Psychiatry (2004) 9:557–69. doi: 10.1038/sj.mp.4001507
15. Schoenbaum G, Roesch MR, Stalnaker TA. Orbitofrontal cortex, decision-making and drug addiction. Trends Neurosci (2006) 29:116–24. doi: 10.1016/j.tins.2005.12.006
16. Volkow ND, Wang G-J, Fowler JS, Hitzemann R, Angrist B, Gatley SJ, et al. Association of methylphenidate-induced craving with changes in right striato-orbitofrontal metabolism in cocaine abusers: implications in addiction. Am J Psychiatry (1999) 156:19–26. doi: 10.1176/ajp.156.1.19
17. van Son D, Wiers RW, Catena A, Perez-Garcia M, Verdejo-García A. White matter disruptions in male cocaine polysubstance users: associations with severity of drug use and duration of abstinence. Drug Alcohol Depend (2016) 168:247–54. doi: 10.1016/j.drugalcdep.2016.09.023
18. Yücel M, Lubman DI, Solowij N, Brewer WJ. Understanding drug addiction: a neuropsychological perspective. Aust N Z J Psychiatry (2007) 41:957–68. doi: 10.1080/00048670701689444
19. Baker ST, Yücel M, Fornito A, Allen NB, Lubman DI. A systematic review of diffusion weighted MRI studies of white matter microstructure in adolescent substance users. Neurosci Biobehav Rev (2013) 37:1713–23. doi: 10.1016/j.neubiorev.2013.06.015
20. Connolly CG, Bell RP, Foxe JJ, Garavan H. Dissociated grey matter changes with prolonged addiction and extended abstinence in cocaine users. PLoS One (2013) 8:e59645. doi: 10.1371/journal.pone.0059645
21. Schiffer B, Müller BW, Scherbaum N, Forsting M, Wiltfang J, Leygraf N, et al. Impulsivity-related brain volume deficits in schizophrenia-addiction comorbidity. Brain (2010) 133:3093–103. doi: 10.1093/brain/awq153
22. Batalla A, Bhattacharyya S, Yücel M, Fusar-Poli P, Crippa JA, Nogué S, et al. Structural and functional imaging studies in chronic cannabis users: a systematic review of adolescent and adult findings. PLoS One (2013) 8:e55821. doi: 10.1371/journal.pone.0055821
23. Cousijn J, Wiers RW, Ridderinkhof KR, van den Brink W, Veltman DJ, Goudriaan AE. Grey matter alterations associated with cannabis use: results of a VBM study in heavy cannabis users and healthy controls. Neuroimage (2012) 59:3845–51. doi: 10.1016/j.neuroimage.2011.09.046
24. Bava S, Jacobus J, Thayer RE, Tapert SF. Longitudinal changes in white matter integrity among adolescent substance users. Alcohol Clin Exp Res (2013) 37:E181–9. doi: 10.1111/j.1530-0277.2012.01920.x
25. Chung T, Pajtek S, Clark DB. White matter integrity as a link in the association between motivation to abstain and treatment outcome in adolescent substance users. Psychol Addict Behav (2013) 27:533. doi: 10.1037/a0026716
26. Clark DB, Chung T, Thatcher DL, Pajtek S, Long EC. Psychological dysregulation, white matter disorganization and substance use disorders in adolescence. Addiction (2012) 107:206–14. doi: 10.1111/j.1360-0443.2011.03566.x
27. Lubman DI, Yücel M, Hall WD. Substance use and the adolescent brain: a toxic combination? J Psychopharmacol (2007) 21:792–4. doi: 10.1177/0269881107078309
28. Draganski B, Gaser C, Busch V, Schuierer G, Bogdahn U, May A. Neuroplasticity: changes in grey matter induced by training. Nature (2004) 427:311. doi: 10.1038/427311a
29. Hilton DL Jr. Pornography addiction—a supranormal stimulus considered in the context of neuroplasticity. Socioaffect Neurosci Psychol (2013) 3:20767. doi: 10.3402/snp.v3i0.20767
30. Pittenger C, Duman RS. Stress, depression, and neuroplasticity: a convergence of mechanisms. Neuropsychopharmacology (2008) 33:88. doi: 10.1038/sj.npp.1301574
31. Kauer JA, Malenka RC. Synaptic plasticity and addiction. Nat Rev Neurosci (2007) 8:844. doi: 10.1038/nrn2234
32. Hiebler-Ragger M, Unterrainer H-F, Rinner A, Kapfhammer H-P. Insecure attachment styles and increased borderline personality organization in substance use disorders. Psychopathology (2016) 49:341–4. doi: 10.1159/000448177
33. Unterrainer H-F, Hiebler-Ragger M, Koschutnig K, Fuchshuber J, Tscheschner S, Url M, et al. Addiction as an attachment disorder: white matter impairment is linked to increased negative affective states in poly-drug use. Front Hum Neurosci (2017) 11. doi: 10.3389/fnhum.2017.00208
34. Unterrainer H, Hiebler M, Ragger K, Froehlich L, Koschutnig K, Schoeggl H, et al. White matter integrity in polydrug users in relation to attachment and personality: a controlled diffusion tensor imaging study. Brain Imaging Behav (2016) 10:1096–107. doi: 10.1007/s11682-015-9475-4
35. Franklin TR, Acton PD, Maldjian JA, Gray JD, Croft JR, Dackis CA, et al. Decreased gray matter concentration in the insular, orbitofrontal, cingulate, and temporal cortices of cocaine patients. Biol Psychiatry (2002) 51:134–42. doi: 10.1016/S0006-3223(01)01269-0
36. Kim SJ, Lyoo IK, Hwang J, Chung A, Sung YH, Kim J, et al. Prefrontal grey-matter changes in short-term and long-term abstinent methamphetamine abusers. Int J Neuropsychopharmacol (2006) 9:221–8. doi: 10.1017/S1461145705005699
37. Hölzel BK, Carmody J, Vangel M, Congleton C, Yerramsetti SM, Gard T, et al. Mindfulness practice leads to increases in regional brain gray matter density. Psychiatry Res Neuroimaging (2011) 191:36–43. doi: 10.1016/j.pscychresns.2010.08.006
38. Dilling H, Mombour W, Schmidt MH. Internationale Klassifikation psychischer. Sto¨rungen: ICD-10, Kapitel V (F, klinisch-diagnostische Leitlinien) (1991).
39. De Leon G. The therapeutic community: theory, model, and method. New York: Springer Publishing Co (2000). doi: 10.1891/9780826116673
40. Tournier JD, Calamante F, Connelly A. MRtrix: diffusion tractography in crossing fiber regions. Int J Imaging Syst Technol (2012) 22(1):53–66.
41. Smith SM, Jenkinson M, Woolrich MW, Beckmann CF, Behrens TE, Johansen-Berg H, Niazy RK. Advances in functional and structural MR image analysis and implementation as FSL. Neuroimage (2004) 23:208–19.
42. Veraart J, Novikov DS, Christiaens D, Ades-Aron B, Sijbers J, Fieremans E. Denoising of diffusion MRI using random matrix theory. NeuroImage (2016) 142:394–406.
43. Andersson JL, Sotiropoulos SN. An integrated approach to correction for off-resonance effects and subject movement in diffusion MR imaging. Neuroimage (2016) 125:1063–78.
44. Smith RE, Tournier JD, Calamante F, Connelly A. Anatomically-constrained tractography: improved diffusion MRI streamlines tractography through effective use of anatomical information. Neuroimage (2012) 62(3):1924–38.
45. Jeurissen B, Tournier JD, Dhollander T, Connelly A, Sijbers J. Multi-tissue constrained spherical deconvolution for improved analysis of multi-shell diffusion MRI data. NeuroImage (2014) 103:411–26.
46. Smith RE, Tournier JD, Calamante F, Connelly A. SIFT: spherical-deconvolution informed filtering of tractograms. NeuroImage (2013) 67:298–312.
47. Yeatman JD, Dougherty RF, Myall NJ, Wandell BA, Feldman HM. Tract profiles of white matter properties: automating fiber-tract quantification. PLoS One (2012) 7:e49790. doi: 10.1371/journal.pone.0049790
48. Nichols TE, Holmes AP. Nonparametric permutation tests for functional neuroimaging: a primer with examples. Hum Brain Mapp (2002) 15(1):1–25.
49. Dahnke R, Yotter RA, Gaser C. Cortical thickness and central surface estimation. NeuroImage (2013) 65:336–48.
50. Spitzer C, Hammer S, Löwe B, Grabe H, Barnow S, Rose M, et al. Die Kurzform des Brief Symptom Inventory (BSI-18): erste Befunde zu den psychometrischen Kennwerten der deutschen Version. Fortschritte der Neurologie·Psychiatrie (2011) 79:517–23. doi: 10.1055/s-0031-1281602
51. Derogatis LR. Administration, scoring, and procedures manual for the SCL-90-R. Baltimore: Clinical Psychometrics Research (1977).
52. Wonderlic E. Wonderlic Personnel Test. Libertyville, IL: EF Wonderlic & Associates. Inc. (1999).
53. Moeller FG, Hasan KM, Steinberg JL, Kramer LA, Dougherty DM, Santos RM, et al. Reduced anterior corpus callosum white matter integrity is related to increased impulsivity and reduced discriminability in cocaine-dependent subjects: diffusion tensor imaging. Neuropsychopharmacology (2005) 30:610–7. doi: 10.1038/sj.npp.1300617
54. Crews FT, Boettiger CA. Impulsivity, frontal lobes and risk for addiction. Pharmacol Biochem Behav (2009) 93:237–47. doi: 10.1016/j.pbb.2009.04.018
55. Stevens L, Verdejo-García A, Goudriaan AE, Roeyers H, Dom G, Vanderplasschen W. Impulsivity as a vulnerability factor for poor addiction treatment outcomes: a review of neurocognitive findings among individuals with substance use disorders. J Subst Abuse Treat (2014) 47:58–72. doi: 10.1016/j.jsat.2014.01.008
56. Makris N, Gasic GP, Kennedy DN, Hodge SM, Kaiser JR, Lee MJ, et al. Cortical thickness abnormalities in cocaine addiction—a reflection of both drug use and a pre-existing disposition to drug abuse? Neuron (2008) 60:174–88. doi: 10.1016/j.neuron.2008.08.011
57. Durazzo TC, Mon A, Gazdzinski S, Meyerhoff DJ. Chronic cigarette smoking in alcohol dependence: associations with cortical thickness and N-acetylaspartate levels in the extended brain reward system. Addict Biol (2013) 18:379–91. doi: 10.1111/j.1369-1600.2011.00407.x
58. Lopez-Larson MP, Bogorodzki P, Rogowska J, McGlade E, King JB, Terry J, et al. Altered prefrontal and insular cortical thickness in adolescent marijuana users. Behav Brain Res (2011) 220:164–72. doi: 10.1016/j.bbr.2011.02.001
59. Kühn S, Schubert F, Gallinat J. Reduced thickness of medial orbitofrontal cortex in smokers. Biol Psychiatry (2010) 68:1061–5. doi: 10.1016/j.biopsych.2010.08.004
60. Hong S-B, Kim J-W, Choi E-J, Kim H-H, Suh J-E, Kim C-D, et al. Reduced orbitofrontal cortical thickness in male adolescents with internet addiction. Behav Brain Funct (2013) 9:11. doi: 10.1186/1744-9081-9-11
61. Yuan K, Cheng P, Dong T, Bi Y, Xing L, Yu D, et al. Cortical thickness abnormalities in late adolescence with online gaming addiction. PLoS One (2013) 8:e53055. doi: 10.1371/journal.pone.0053055
62. Sowell ER, Mattson SN, Kan E, Thompson PM, Riley EP, Toga AW. Abnormal cortical thickness and brain–behavior correlation patterns in individuals with heavy prenatal alcohol exposure. Cereb Cortex (2007) 18:136–44. doi: 10.1093/cercor/bhm039
63. Jernigan TL, Butters N, DiTraglia G, Schafer K, Smith T, Irwin M, et al. Reduced cerebral grey matter observed in alcoholics using magnetic resonance imaging. Alcohol Clin Exp Res (1991) 15:418–27. doi: 10.1111/j.1530-0277.1991.tb00540.x
64. Qiu Y, Jiang G, Su H, Lv X, Zhang X, Tian J, et al. Progressive white matter microstructure damage in male chronic heroin dependent individuals: a DTI and TBSS study. PLoS One (2013) 8:e63212. doi: 10.1371/journal.pone.0063212
65. Gu X, Hof PR, Friston KJ, Fan J. Anterior insular cortex and emotional awareness. J Comp Neurol (2013) 521:3371–88. doi: 10.1002/cne.23368
66. Albaugh MD, Ducharme S, Collins DL, Botteron KN, Althoff RR, Evans AC, et al. Evidence for a cerebral cortical thickness network anti-correlated with amygdalar volume in healthy youths: implications for the neural substrates of emotion regulation. Neuroimage (2013) 71:42–9. doi: 10.1016/j.neuroimage.2012.12.071
67. Carton S, Bayard S, Paget V, Jouanne C, Varescon I, Edel Y, et al. Emotional awareness in substance-dependent patients. J Clin Psychology (2010) 66:599–610. doi: 10.1002/jclp.20662
68. Kober H, Bolling D. Emotion regulation in substance use disorders. Handb Emotion Regul (2014) 2:428–46.
69. Moeller SJ, Konova AB, Parvaz MA, Tomasi D, Lane RD, Fort C, et al. Functional, structural, and emotional correlates of impaired insight in cocaine addiction. JAMA Psychiatry (2014) 71:61–70. doi: 10.1001/jamapsychiatry.2013.2833
70. Wang Y, Metoki A, Alm KH, Olson IR. White matter pathways and social cognition. Neurosci Biobehav Rev (2018) 90:350–370. doi: 10.1101/179473
71. Unger A, Alm KH, Collins JA, O’Leary JM, Olson IR. Variation in white matter connectivity predicts the ability to remember faces and discriminate their emotions. J Int Neuropsychol Soc (2016) 22:180–90. doi: 10.1017/S1355617715001009
72. Kalivas PW, O’Brien C. Drug addiction as a pathology of staged neuroplasticity. Neuropsychopharmacology (2008) 33:166. doi: 10.1038/sj.npp.1301564
73. Diana M, Raij T, Melis M, Nummenmaa A, Leggio L, Bonci A. Rehabilitating the addicted brain with transcranial magnetic stimulation. Nat Rev Neurosci (2017) 18(11):685.
74. Leccese M, Waldron HB. Assessing adolescent substance use: a critique of current measurement instruments. J Subst Abuse Treat (1994) 11:553–63. doi: 10.1016/0740-5472(94)90007-8
Keywords: DTI, gray matter, neuroplasticity, poly-drug use, VBM, white matter
Citation: Unterrainer HF, Hiebler-Ragger M, Koschutnig K, Fuchshuber J, Ragger K, Perchtold CM, Papousek I, Weiss EM and Fink A (2019) Brain Structure Alterations in Poly-Drug Use: Reduced Cortical Thickness and White Matter Impairments in Regions Associated With Affective, Cognitive, and Motor Functions. Front. Psychiatry 10:667. doi: 10.3389/fpsyt.2019.00667
Received: 30 January 2019; Accepted: 19 August 2019;
Published: 20 September 2019.
Edited by:
Scott D. Lane, University of Texas Health Science Center at Houston, United StatesReviewed by:
Domenico De Berardis, Azienda Usl Teramo, ItalyKesong Hu, Lake Superior State University, United States
Copyright © 2019 Unterrainer, Hiebler-Ragger, Koschutnig, Fuchshuber, Ragger, Perchtold, Papousek, Weiss and Fink. This is an open-access article distributed under the terms of the Creative Commons Attribution License (CC BY). The use, distribution or reproduction in other forums is permitted, provided the original author(s) and the copyright owner(s) are credited and that the original publication in this journal is cited, in accordance with accepted academic practice. No use, distribution or reproduction is permitted which does not comply with these terms.
*Correspondence: Human-Friedrich Unterrainer, human.unterrainer@univie.ac.at