- Department of Sustainable Crop Production (DI.PRO.VE.S.), Università Cattolica del Sacro Cuore, Piacenza, Italy
Six commercial biocontrol agents (BCAs, containing Aureobasidium pullulans, Bacillus amyloliquefaciens, Bacillus amyloliquefaciens plantarum, Bacillus subtilis, Pythium oligandrum, or Trichoderma atroviride) were applied to ripening berries that were then incubated at one of four temperatures (T, 15, 20, 25, and 30°C) and one of four relative humidity levels (RH, 60, 80, 90, and 100%). After 1 to 13 days of incubation (BCA colonization period), the berries were inoculated with conidia of Botrytis cinerea and kept at 25°C and 100% RH for 7 days, at which time Botrytis bunch rot (BBR) was assessed. The response of BBR control to T/RH conditions and BCA colonization period differed among BCAs; the coefficients of variation among the BCAs ranged from 44.7 to 72.4%. An equation was developed that accounted for the combined effects of T, RH, and BCA colonization period on BBR control. The equation, which had an R2>0.94, could help farmers select the BCA to be used for a specific application based on weather conditions at the time of treatment and in the following days.
Introduction
The necrotrophic fungal pathogen Botrytis cinerea Pers. Fr. [teleomorph Botryotinia fuckeliana (de Bary) Whetzel] causes Botrytis bunch rot (BBR), which is a major disease of grapevines (Elad et al., 2016). Although B. cinerea can damage all vine organs, its infection of ripening berries results in especially large losses in quantity and quality (Jarvis, 1977; Williamson et al., 2007; Elad et al., 2016). Because of physiological changes in ripening berries (Kretschmer et al., 2007; Mundy and Beresford, 2007; Deytieux-Belleau et al., 2009) but also because environmental conditions during the latter part of the growing season tend to favor the infection (Nair et al., 1988; Ciliberti et al., 2015), the susceptibility of berries to B. cinerea increases with maturity (Deytieux-Belleau et al., 2009), from veraison to harvest (from growth stage (GS) 81 to GS<89 of Lorenz et al., 1995). Botrytis bunch rot on ripening berries can result from i) latent infections established during flowering, ii) direct berry infection caused by conidia, and iii) berry-to-berry infection caused by mycelium originating from previously infected berries within the cluster (Elmer and Michailides, 2007; González-Domínguez et al., 2015). The disease on ripening berries is traditionally controlled by repeated fungicide applications (González-Domínguez et al., 2019).
In recent years, use of fungicides during ripening has been subjected to increasing limitations in order to reduce or eventually eliminate chemical residues on grapes and in wines (Verger and Boobis, 2013). Negative effects on the environment and the resistance of B. cinerea populations to most chemical fungicides (Leroux, 2007; Fernández-Ortuño et al., 2016) also contribute to an increasing interest in alternatives to chemical fungicides for BBR management (Harman, 2000; Elad and Freeman, 2002; Elmer et al., 2005; Tracy, 2014).
One promising alternative for BBR management is the use of microorganisms as biocontrol agents (BCAs) (Pertot et al., 2017; Fedele et al., 2020c; Fedele et al., 2020d). BCAs may suppress B. cinerea via various modes of action, including competition for nutrients and space, antibiosis, parasitism, and induced host plant resistance (Elad and Freeman, 2002; Elmer and Reglinski, 2006; Haidar et al., 2016).
In spite of the extensive research on BCAs (O’Neill et al., 1996; Schoene, 2002; Jacometti et al., 2010; Calvo-Garrido et al., 2014; Pertot et al., 2017; Calvo-Garrido et al., 2018; Escribano-Viana et al., 2018; Rotolo et al., 2018; Calvo-Garrido et al., 2019; Fedele et al., 2020a), only a few commercial products containing BCAs are available in Europe (Nicot et al., 2016), where farmers still mainly rely on chemical fungicides for BBR control. The limited use of BCAs for BBR control may be related to their inconsistent efficacy across seasons or across local agronomic conditions (Tracy, 2014; Calvo-Garrido et al., 2019), i.e., multiple factors and complex processes generally determine BCA efficacy (Rosenheim et al., 1995). This complexity should be expected given that BCAs are living organisms that dynamically interact with the target pathogen, the host plant, and the microbial communities on the host surfaces in a changing physical environment (Fedele et al., 2020c). Fluctuating environmental conditions, in particular, strongly influence BCA survival, establishment, growth, and efficacy (Elad and Freeman, 2002; Kredics et al., 2003; Xu et al., 2010; Fedele et al., 2020c).
It follows that the successful integration of BCAs into a disease management strategy requires an understanding of their environmental requirements. Temperature, humidity, and other environmental conditions have been evaluated as key factors determining BCA efficacy (Mitchell et al., 1987; Jackson et al., 1991; Elad et al., 1993; Hannusch and Boland, 1996; Dik et al., 1999; Kredics et al., 2003; Fedele et al., 2020c), but the complex relationships between BCAs and multiple environmental factors remain poorly understood (Deacon and Berry, 1993; Whipps, 1997).
Fedele et al. (2020a) recently used a general model to study how control of BBR by a theoretical BCA is affected by the control mechanism, timing of BCA application, and environmental conditions. The authors found that temperature and moisture conditions affecting the growth and survival of the theoretical BCA were more important than its mechanism of control or the timing of its application. The latter study indicated that additional research is needed to determine how the efficacies of specific BCAs of B. cinerea are affected by fluctuating temperatures and humidity.
In the current research, we studied the effect of six commercial biocontrol products (each with different BCA) on the development of BBR on grape berries. Although conducted in growth chambers, the experiment used four temperature regimes and four relative humidity regimes that simulated a range of vineyard conditions. The basic experimental scenario involved the transfer of individual berries (at different stages of maturity) from a vineyard into growth chambers, where the berries were treated with the BCAs. After the BCAs had time to develop on the berry surfaces (1 to 13 days; this was another experimental variable) under different environmental conditions, the berries were inoculated with conidia of B. cinerea. The berries were subsequently kept under optimal conditions for disease development before they were assessed for BBR.
Materials and Methods
Plant Material
Berries were collected in 2018 and 2019 in an 11-year-old vineyard (in 2018) located at Castell’Arquato (44°51′26.1′′N 9°51′20.7′′E, 400 m a.s.l.) in the Emilia-Romagna region. The vineyard was planted with cv. Merlot, and the vines were trained using a Guyot system; the within- and between-row spacing were 1.0 and 2.3 m, respectively. Powdery and downy mildews were controlled according to an integrated pest management program (Rossi et al., 2012), and the fungicides applied were not effective against B. cinerea.
Ripening berries were collected (with their pedicels) in 2018 and 2019 on the following dates: 03, 10, and 17 September in 2018; 26 August and 2 and 9 September in 2019. The ripening stages of the berries at sampling were measured as degrees Babo (an indicator of sugar content), which were 19.3, 20.0, and 20.3 in 2018, and 16.4, 18.6, and 19.0 in 2019.
Berries were transported to the laboratory in a cooler, where they were rinsed under tap water for 3 min, disinfested with 2/3 of distilled water and 1/3 of 5% sodium hypochlorite to remove epiphytic microflora, and finally rinsed again with sterile water.
Treatment of Berries With Biocontrol Agents
Six commercial BCAs were used (Table 1). These products were dispersed in double-distilled sterile water (pH 6.5) at the label dose, and berries were treated by immersion. The viability of the BCAs was confirmed by plating the product suspensions on PDA (potato dextrose agar, Biolife Italiana S.r.l., Milano, Italy). Non-treated (NT) berries were used as the control, and these were immersed in double-distilled, sterile water.
Berries were then placed in metal boxes (20 × 15 cm) over a metal grid net so that berries did not touch each other or the box bottom; there were 15 berries per box. After treatment, boxes were incubated in growth chambers with a 12-h photoperiod and at different regimes of temperature (15, 20, 25, and 30°C) and relative humidity (60, 80, 90, and 100% RH). Different RH values were obtained by placing 200 ml of different glycerol/double-distilled water solutions (Dallyn and Fox, 1980) or double-distilled water on the bottom of the metal boxes. The true RH inside the boxes was checked with a data logger (Tinytag Plus 2, Gemini Data Loggers, Chichester, England). There were three boxes per each T/RH regime. The experiment was performed three times per year for 2 years, with berries sampled at different ripening stages.
Inoculation of Berries With Botrytis cinerea
At 1, 3, 6, 9, or 13 days after treatment with BCAs, the berries in each box were inoculated with a conidial suspension of B. cinerea, isolate 213T, which belongs to the transposa sub-species and is highly aggressive (Ciliberti et al., 2016). The conidia were obtained from 10-day-old cultures grown on PDA at 20°C and with a 12-h photoperiod using white and near-UV (UV-A at 370 nm) light (Black Light UV-A, L18 w/73, OSRAM, Munich, Germany). The conidial suspensions were prepared by flooding the dishes with sterile-distilled water and gently scraping the agar surface with a sterile rod. The suspension was passed through two layers of sterilized gauze (autoclaved at 120°C for 20 min), and the conidia were enumerated with a hemocytometer. The concentration of conidia was adjusted to 105/ml by adding double-distilled sterile water. The conidial suspension was uniformly distributed on the berries by using a hand sprayer, 1 ml of suspension per box.
The boxes were then placed in growth chambers at 25°C and with 100% RH and a 12-h photoperiod for 7 days to promote the germination of B. cinerea conidia and the development of BBR.
Disease Assessment
One week after the single berries were inoculated with B. cinerea, BBR severity was assessed as the percentage of the surface of each berry with BBR symptoms; the severity of the entire replicate (i.e., of the 15 berries in each box) was then determined by using the standard area diagram of Hill et al. (2010).
Data Analysis
BBR severity data were subjected to a factorial analysis of variance (ANOVA), in which the factors were BCA treatment (six BCAs plus the untreated control, NT), T/RH regime (combinations of T and RH), and the number of days that berries were incubated before they were inoculated with B. cinerea (BCA colonization period: 1, 3, 6, 9, or 13 days). The experimental design was a split-split, with BCAs as the main plots, T/RH regimes as the split plots, and BCA colonization periods as the split-split plots. The 2 years and the different ripening stages at which berries were sampled were considered blocking factors; according to statistical theory and experimental design, a blocking factor is a source of variability that is not of primary interest for the experiment (Quinn and Keough, 2002). The BBR severity data (in %) were subjected to arcsin transformation before the ANOVA to make variances homogeneous.
To assess the variability in BBR severity as affected by T/RH regime and colonization period within each BCA, coefficients of variation (CV, in %), or relative standard deviations (SDs) were calculated as the ratio of the SD to the mean; the higher the CV, the greater the variability generated by T/RH regimes and the length of the BCA colonization period.
To further investigate the variability generated by T/RH regimes and length of colonization period within each BCA, BBR severity data were expressed as disease control relative to NT as follows: the difference between BBR severity on the untreated berries (i.e., the berries that were not treated with any BCA before they were inoculated with B. cinerea) and treated berries (i.e., the berries that were treated with a BCA before they were inoculated with B. cinerea) was calculated and divided by the maximal difference found in the experiment for the specific BCA. As a hypothetical example, consider berries that were untreated or treated with BCA X and then were incubated at 15°C and 100% RH for 6 days before being inoculated with B. cinerea; in this first hypothetical example, BBR severity was 30% for untreated berries and 5% for treated berries, resulting in a 25% difference. As a second hypothetical example, consider berries that were incubated at 25°C and 100% RH for 9 days before B. cinerea inoculation and that had a BBR severity of 55% if untreated and 10% if treated with BCA X, resulting in a 45% difference, which was the greatest difference for BCA X among all combinations of T, RH, and length of colonization period. Therefore, the relative control provided by BCA X was 25/45 = 0.55 for the first example and 45/45 = 1 for the second example. As indicated by these hypothetical examples, relative control is scaled between zero (no control, i.e., no difference with respect to NT) and 1.0 (highest control, i.e., the widest difference with respect to NT). Data were rescaled as described because the aim was to evaluate the within-BCA variability in the control of BBR under different temperature and relative humidity regimes rather than to compare the efficacy of the different BCAs.
The relative control data (Y) were then fit to the following equation:
when: Y<0, Y=0; Y>1, Y=1
where α, β, ϕ, φ, λ, and ς are the equation parameters; Teq are equivalents of temperature, defined as Teq=(T-Tmin)/(Tmax-Tmin), where T is the temperature regime (in °C), and Tmin and Tmax are cardinal temperatures (in °C); dbi is the number of days between the application of BCA and inoculation with B. cinerea; and vapor pressure deficit (VPD) (in kPa) is the vapor pressure deficit calculated as follows (Buck, 1981):
where RH is relative humidity (in %).
Equation [1] was selected among a set of candidate equations by using the Akaike information criterion (AIC), which is an estimator of the relative quality of statistical models for a given set of data (Akaike, 1981). Equation parameters, Tmin, and Tmax were estimated using the non-linear regression procedure of SPSS software (IBM SPSS Statistics, version 25), which minimizes the residual sums of squares using the Marquardt algorithm.
In equation [1], the first term, [α Teqβ (1-Teq)ϕ], accounts for the effect of temperature according to the bell-shaped curve of Analytis (Analytis, 1977), with parameters α, β, and ϕ defining the top, symmetry, and size of the curve, respectively. The second term, [1-φ (1-λVPD)], accounts for the combined effect of T and RH on Y according to an asymptotic equation, where 1 is the maximum attainable value for Y, φ is the value for Y at VPD=0, and λ is proportional to the relative rate of decrease for Y when VPD increases. The third term, (1-ςdbi), accounts for the effect on Y of the time elapsed from the BCA application, where 1 is the maximum attainable value for Y and ς is proportional to the relative rate of increase for Y when time elapses.
Goodness-of-fit of equation [1] to the relative control data for the six BCAs was assessed for the whole dataset based on the adjusted R2 (which was estimated by conducting a linear regression between the observed and the predicted values), the root mean square error (RMSE), the coefficient of residual mass (CRM), and the concordance correlation coefficient (CCC) (Nash and Sutcliffe, 1970; Lin, 1989). In brief, RMSE represents the average distance of real data from the fitted line, and CRM is a measure of the tendency of the equation to overestimate or underestimate the observed values (a negative CRM indicates a tendency of the model toward overestimation) (Nash and Sutcliffe, 1970). CCC is the product of two terms: the Pearson correlation coefficient and the coefficient Cb, which indicates the difference between the best fitting line and the perfect agreement line (CCC = 1 means perfect agreement) (Madden et al., 2007).
Results
Overall BBR severity on untreated berries was 29.5±0.85%. In both years, BBR severity increased with sampling date, i.e., increased as berries ripened: BBR severity was 42.1, 56.5, and 59.7% in 2018, and was 21.2, 22.6, and 24.3% in 2019. Because this source of variability was not of primary interest in this study, it was considered a blocking factor in the ANOVA.
The ANOVA showed a significant effect of the main factors BCA, T/RH regime, and BCA colonization period (all at P<0.001), which explained 17.5, 42.4, and 4.1%, respectively, of the total variance in BBR severity. The interactions BCA × T/RH regime and BCA × BCA colonization period were also significant (P=0.009 and P=0.006, respectively), and accounted for 9.7 and 6.5% of the total variance in BBR severity, respectively. The interactions T/RH regime × BCA colonization period and BCA × T/RH regime × BCA colonization period were not significant (P>0.05 in both cases), and together accounted for 29.7% of total variance in BBR severity. These data show that the number of days that BCAs grew on the surface of berries before B. cinerea inoculation and also the T/RH regime during this period significantly affected BBR severity but that the response of each BCA to T/RH regime and colonization period differed among BCAs.
The coefficient of variation (Figure 1) of BBR severity data ranged from 44.7% [for polyversum (POL)] to 72.4% [for botector (BOT)], which indicated that some BCAs were more sensitive than other BCAs to T/RH regimes and the length of the BCA colonization period before B. cinerea inoculation.
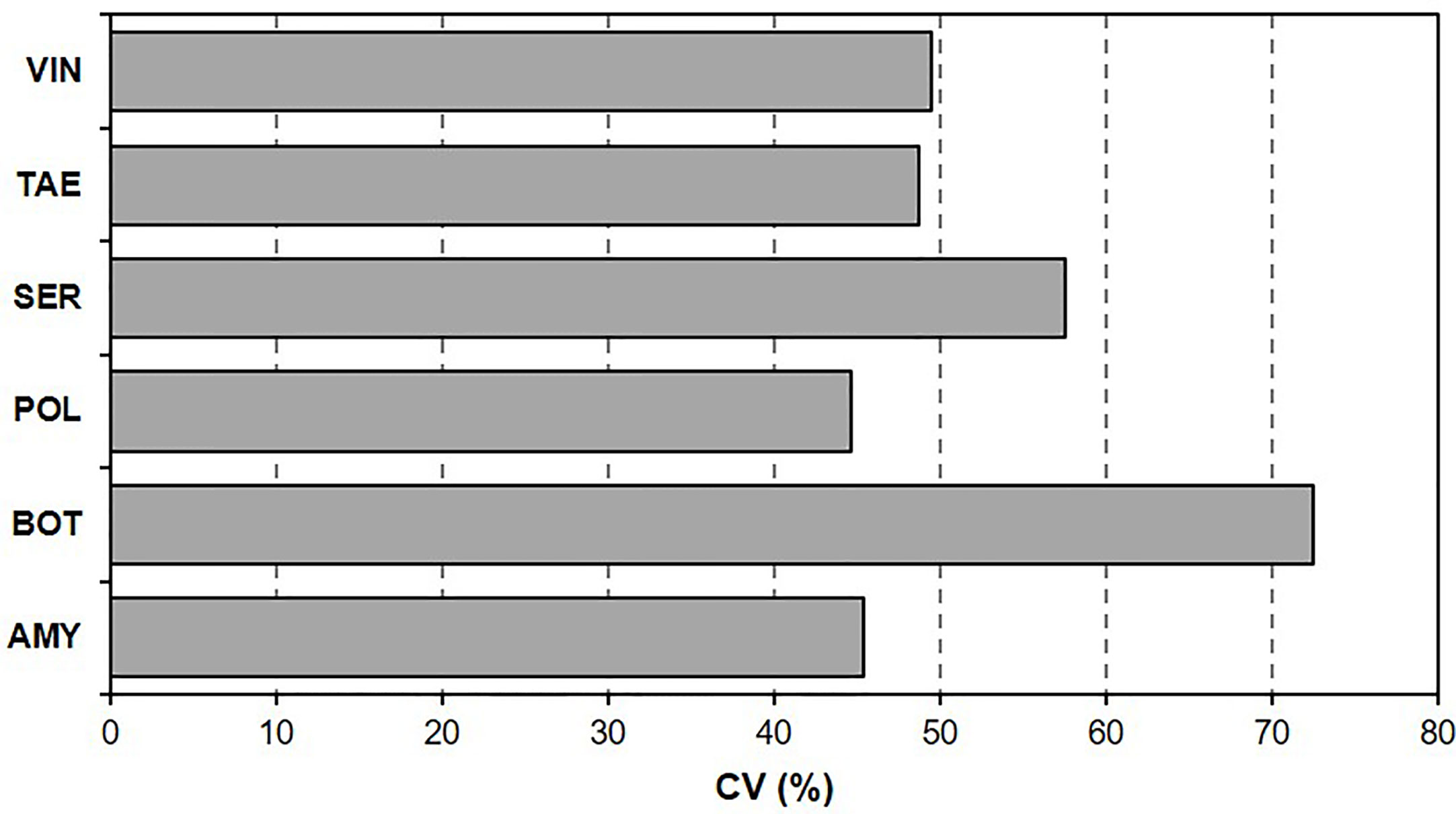
Figure 1 Coefficient of variation (CV, %) of Botrytis bunch rot (BBR) severity on grape berries. After the berries were treated with six biocontrol agents (BCAs), they were subjected to different temperature and relative humidity regimes for different BCA colonization periods before they were inoculated with Botrytis cinerea and kept under conditions that were optimal for BBR.
Relative BBR control by BCAs as affected by length of BCA colonization period and T/RH regime is shown in Figure 2. Although the dynamics of relative control over time were generally similar for the six BCAs, the BCAs differed in the time required to achieve maximal control, i.e., some BCAs were faster than others (Figure 2A). Although the responses of BBR control to T and RH (both were considered as main effects) had bell-shaped and exponential patterns, respectively, for all of the BCAs, the BCAs differed in the size of response to specific T or RH conditions (Figures 2B, C).
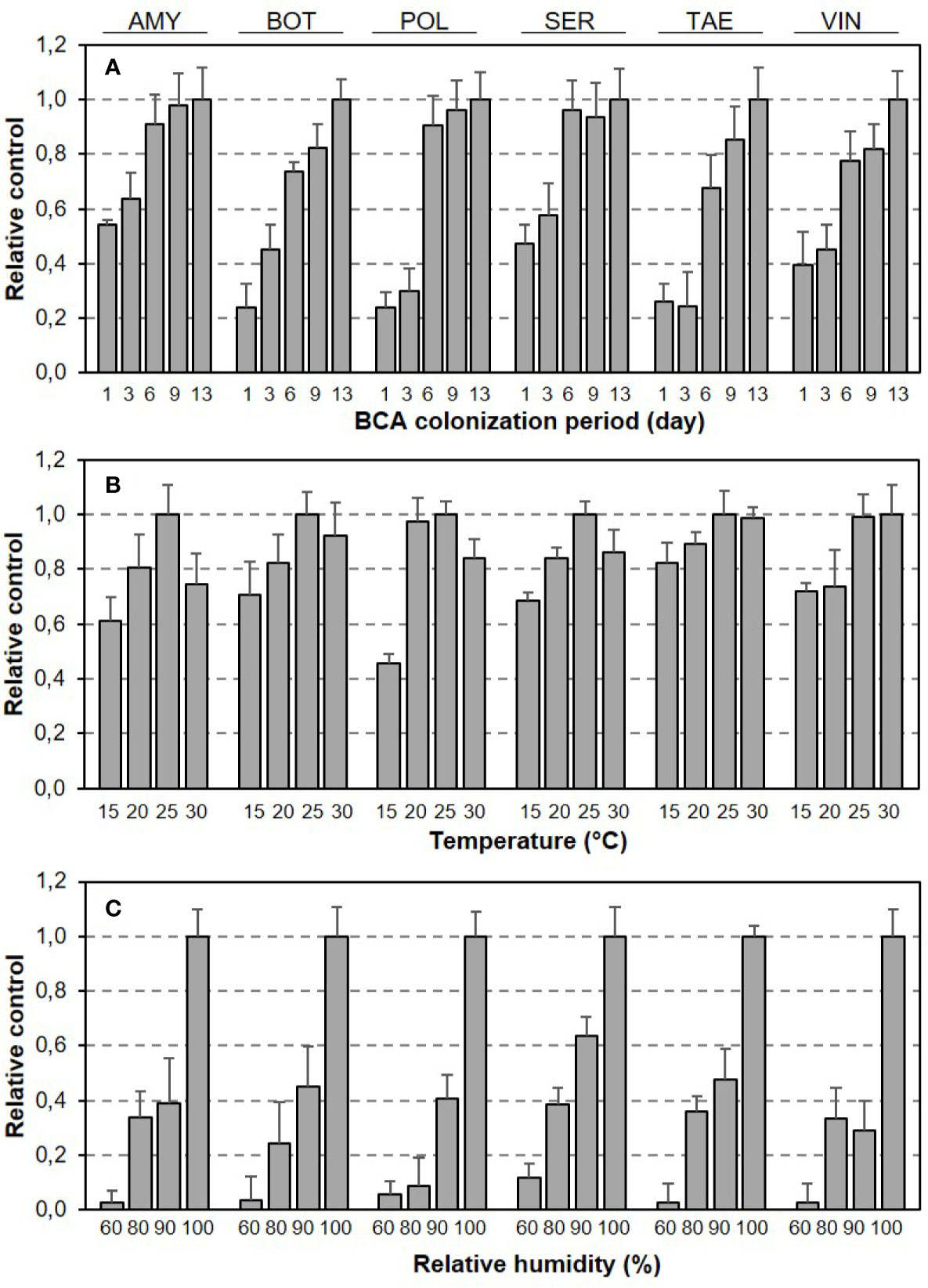
Figure 2 Relative control of Botrytis bunch rot severity on grape berries by six biocontrol agents (BCAs) as affected by (A) the length of colonization period (in days) before the BCA-treated berries were subsequently inoculated with Botrytis cinerea, and by (B) the temperature and (C) relative humidity that the berries were subjected to in the period between BCA treatment and B. cinerea inoculation. Bars are means (+ SE) of different T/RH regimes in (A) and of different numbers of days between BCA treatment B. cinerea and inoculation in (B, C).
Equation [1] provided a good fit for the relative control data for all of the BCAs, with R2>0.94, RMSE≤0.08, CRM≤0.01, and CCC>0.97 (Table 2). This indicated that solving equation [1] for any time period between the application of a BCA and the occurrence of a B. cinerea infection (in the interval 1 to 13 days), and for any combination of T (between 15 and 30°C) and RH (between 60 to 100%), provides a reliable prediction of the relative control of BBR by the treatment.
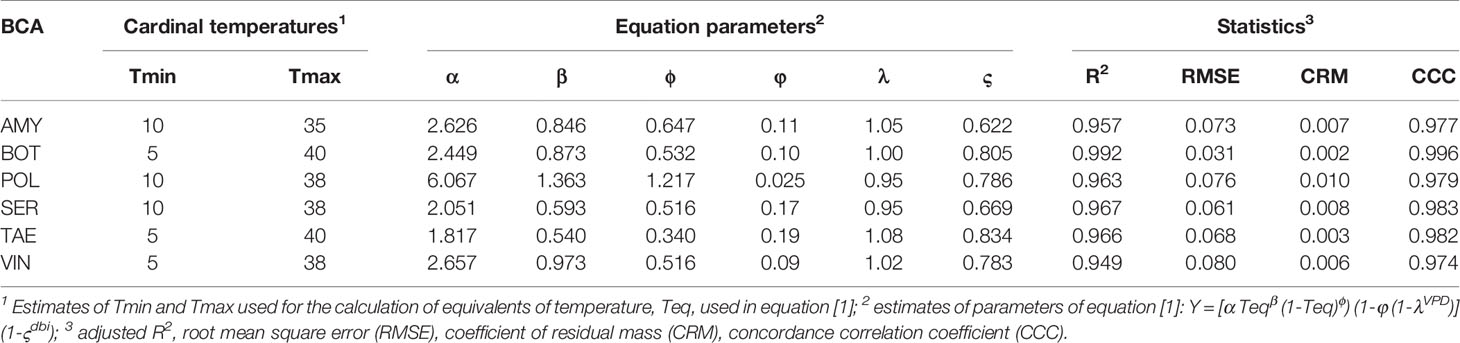
Table 2 Parameters of equation [1] for the six biocontrol agents (BCAs), and statistics for goodness-of-fit to real data.
Figure 3 provides two examples of the three-dimension plot of equation [1] with the parameters of Table 2 for BOT and POL, with dbi=13 days.
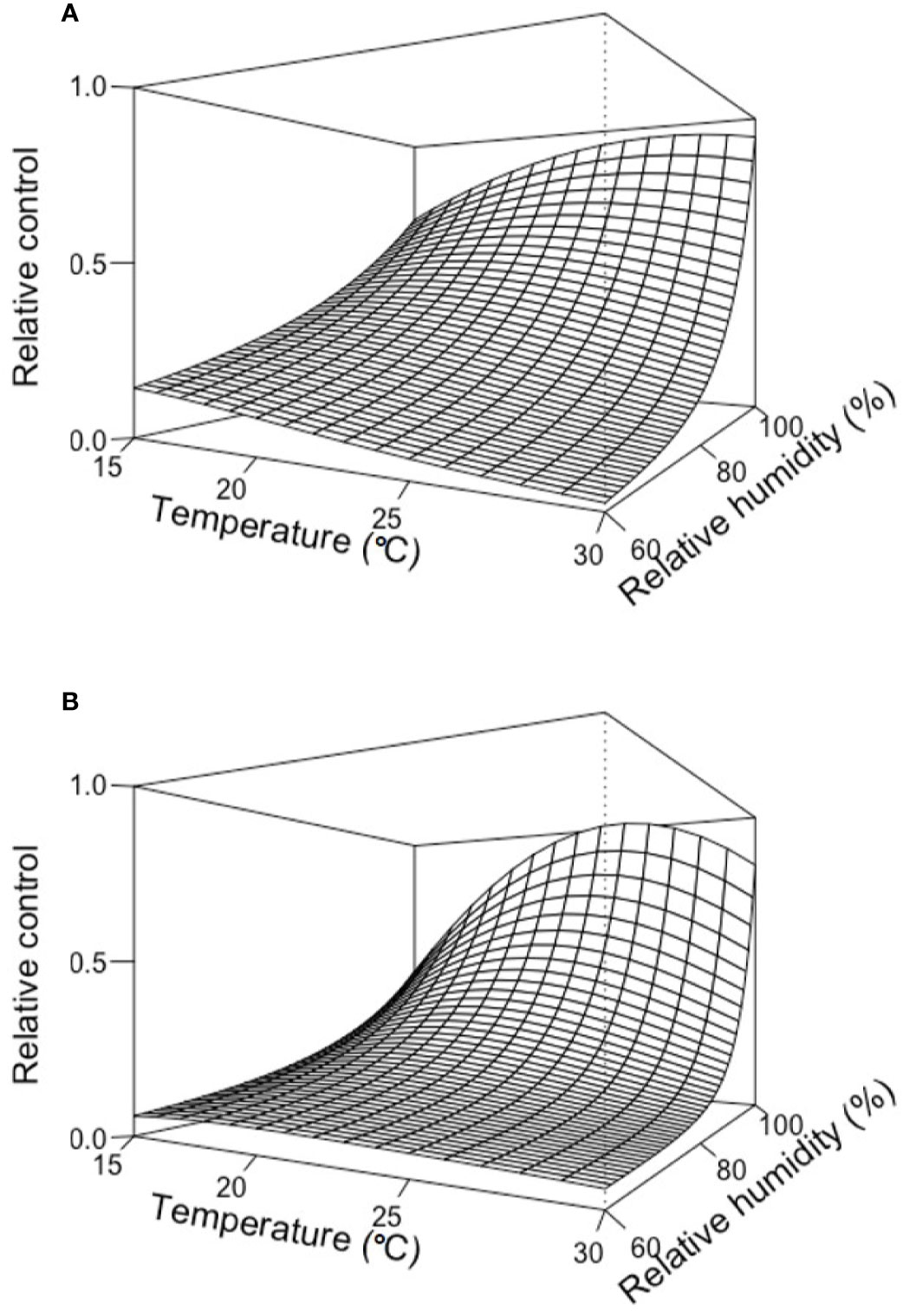
Figure 3 Relative control of Botrytis bunch rot on grape berries that were treated with two biocontrol agents (BCAs) (BOT in A and POL in B; see Table 1) and then incubated for 13 days (dbi = 13) at different temperature (T) and relative humidity (RH) values before they were inoculated with Botrytis cinerea. Following inoculation with B. cinerea, the berries were kept under T and RH conditions that favored disease development (25°C and 100% RH). The plots were generated with equation [1] and the parameters listed in Table 2.
In Figure 4, equation [1] was used to calculate the colonization period (in days) required by each BCA to provide 0.1, 0.5, and 0.9 relative control of BBR at different temperatures and with RH=100%. Some BCAs, like AMY and POL (Figures 4A, C), required shorter colonization period than others before B. cinerea inoculation to attain a specific level of control, and the length of this period was temperature-dependent. To provide 0.5 relative BBR control, for instance, both POL (Figure 4C) and VIN (Figure 4E) required fewer than 3 days at 25°C but required 6.0 and 4.3 days, respectively, at 15°C.
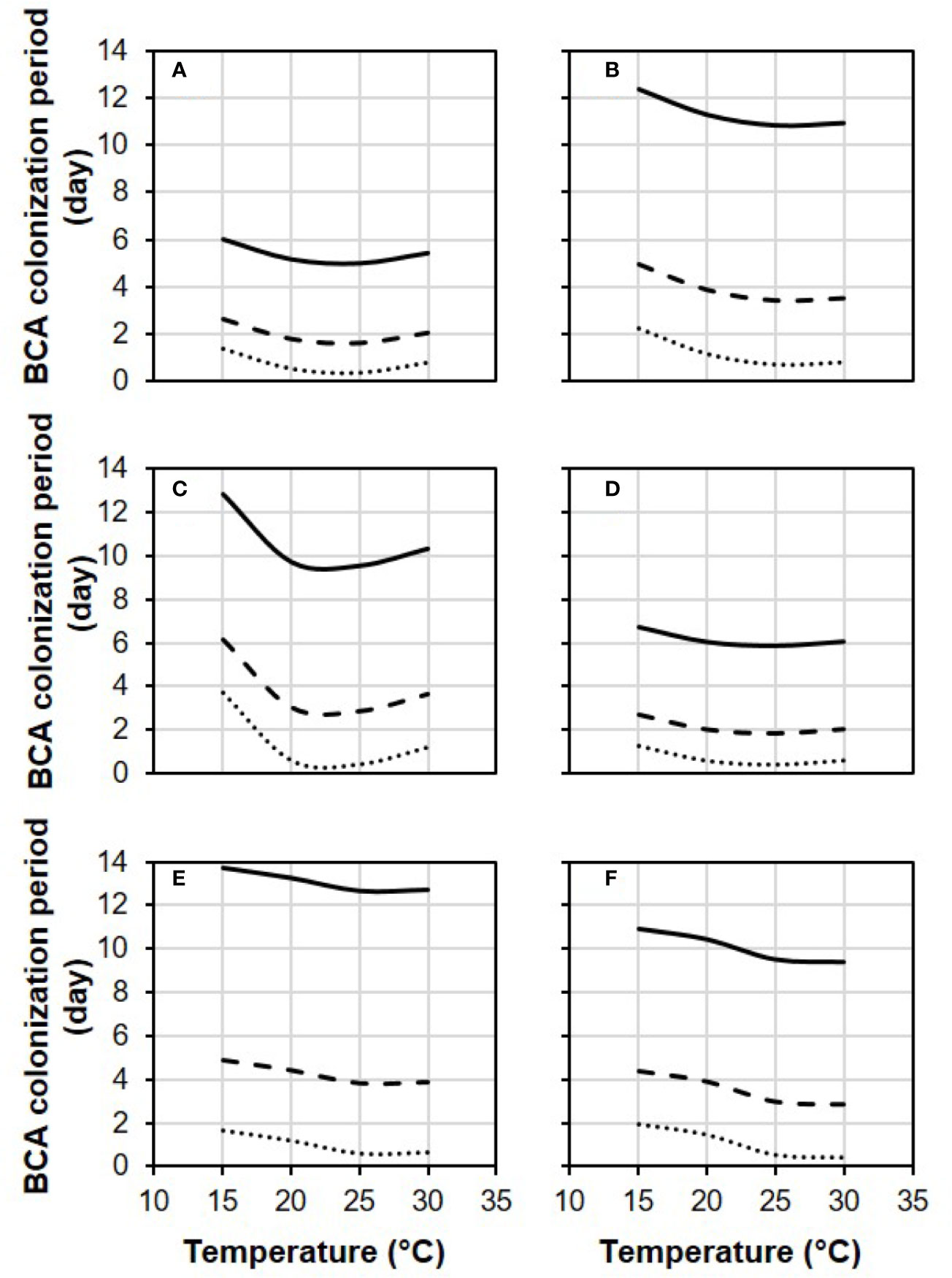
Figure 4 Effect of temperature on the length of the biocontrol agent (BCA) colonization period required by the six BCAs (A: AMY; B: BOT; C: POL; D: SER; E: TAE; F: VIN, see Table 1) to attain 0.1 (dotted line), 0.5 (dashed line), and 0.9 (solid line) relative control of Botrytis bunch rot. The colonization period is the number of days between treatment of berries with a BCA and inoculation of berries with Botrytis cinerea. Berries were treated with the BCAs listed in Table 1 and were then incubated in growth chambers at different temperatures and with different RH values (values in this figure are for RH = 100%). After 1, 3, 6, 9, and 13 days, berries were inoculated with B. cinerea and incubated at 25°C and 100% RH, which favored disease development. Lines were drawn by using the equation [1] and the parameters listed in Table 2.
In Figure 5, equation [1] was used to calculate the relative BBR control provided by the application of the six BCAs with a colonization period of 13 days between BCA treatment and B. cinerea inoculation; in Figure 5, relative control values were grouped into five categories, from low to high values. The BCAs differed in their response to T/RH, i.e., some provided higher control than others at specific combinations of temperature and relative humidity. With the combination T=20°C and RH=90% (the circles in Figure 5), for example, relative control was medium–low for BOT (Figure 5B), medium for AMY (Figure 5A) and VIN (Figure 5F), between medium and medium–high for POL (Figure 5C) and TAE (Figure 5E), and medium–high for SER (Figure 5D). In another example, i.e. with T=25°C and RH=80% (the triangles in Fig. 5), relative control was low for BOT (Figure 5B) and VIN (Figure 5F), and was medium–low for the others.
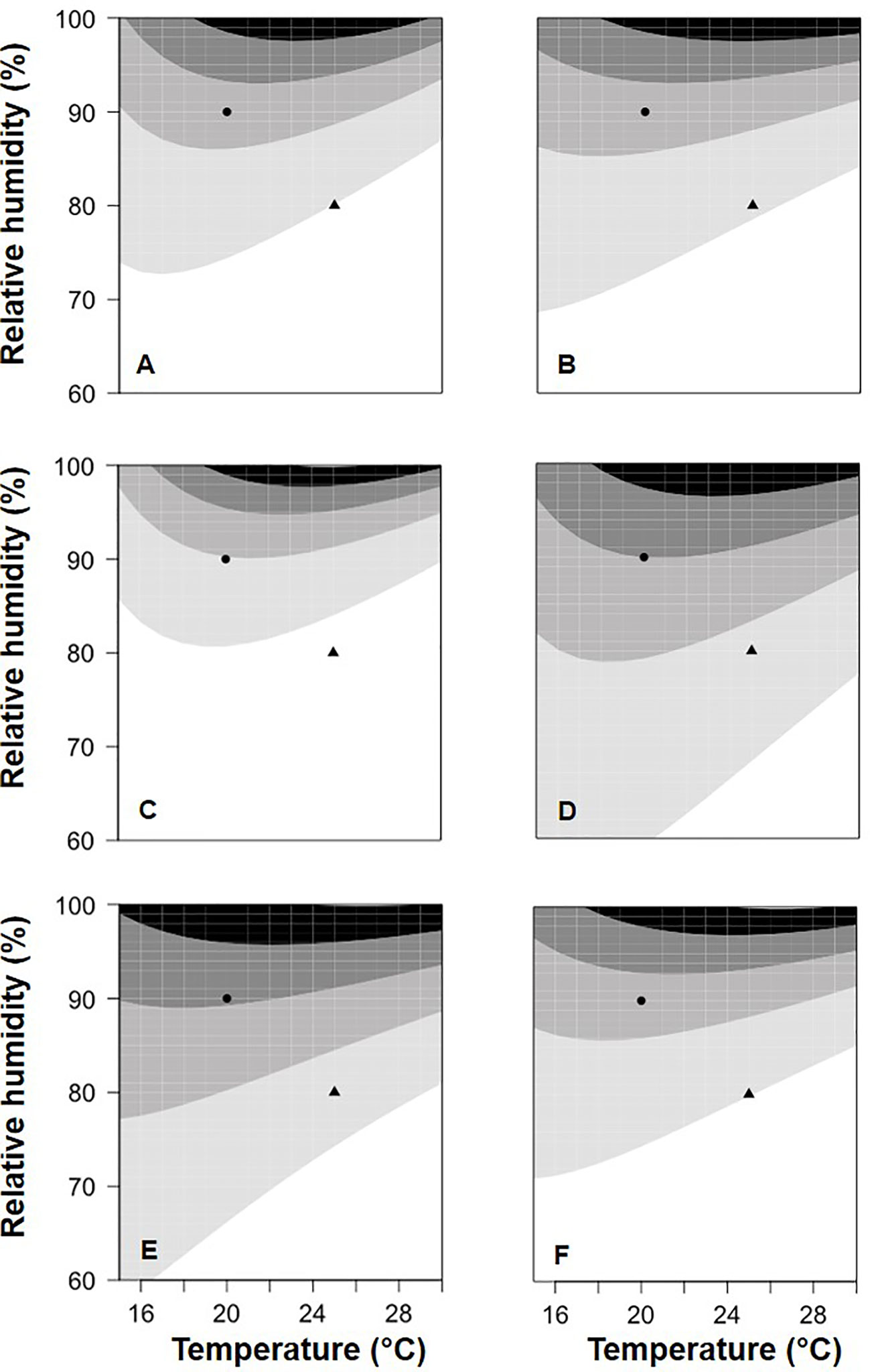
Figure 5 Relative control of Botrytis bunch rot severity on grape berries that were treated with six biocontrol agents (BCAs) (A: AMY; B: BOT; C: POL; D: SER; E: TAE; F: VIN, see Table 1) and then incubated at different temperatures (T) and with different relative humidity (RH) values. After 13 days (for the data in this figure), berries were inoculated with Botrytis cinerea and then incubated at 25°C and 100% RH, which favored disease development. The contour plots (which are based on the 3-dimensional graphs in Figure 3 with BCA colonization period of 13 days) identify five areas of relative control of berry bunch rot by the six BCAs: L (low, 0 to 0.2 relative control; the white area); ML (medium-low, 0.2 to 0.4; the light gray area); M (medium, 0.4 to 0.6; the medium gray area); MH (medium-high, 0.6 to 0.8; the dark gray area); and H (high: 0.8 to 1; the black area).
Discussion
In this research, we studied six commercial BCAs that are currently available for the control of B. cinerea in vineyards and that differ in their modes of action. Bacillus amyloliquefaciens subsp. plantarum strain D747 (AMY), Bacillus subtilis strain QST 713 (SER), and B. amyloliquefaciens strain FZB24 (TAE) are spore-forming bacteria whose major mode of action is antibiosis (Leifert et al., 1995; Borriss, 2015). Aureobasidium pullulans strains DMS 14941-14940 (BOT) are common fungal epiphytes of grapevines that can colonize wounds, produce hydrolytic enzymes, and form a biofilm (Parafati et al., 2015), and that can also produce volatile organic compounds that prevent the germination of conidia (Di Francesco et al., 2015). Pythium oligandrum strain M1 (POL) is a mycoparasitic oomycete that attacks the host fungus by lysis or penetration of hyphae (Laing and Deacon, 1991). Like other Trichoderma spp., T. atroviride strain SC1 (VIN) has multiple modes of action, including induction of plant resistance, mycoparasitism, antibiosis, and competition for space and nutrients (Pertot et al., 2013). All of these BCAs have been proved to provide control of BBR under vineyard conditions, although with variable efficacy (Pertot et al., 2017; Alessandri et al., 2018; Rotolo et al., 2018; Calvo-Garrido et al., 2019).
In this research, rather than obtain additional data on the efficacy of these BCAs in controlling BBR in vineyards, we assessed the effect of temperature and humidity on their efficacy. For this reason, we expressed data on BBR control in terms of relative control, i.e., as a proportion of the highest level of control provided by each BCA under the most favorable T/RH regime. The use of relative control enabled us to determine the best T/RH conditions for each BCA and to compare the T/RH responses among the six BCAs.
We conducted an experiment under controlled environmental conditions, in which grape berries were treated with the BCAs and inoculated with conidia of B. cinerea at different times after BCA treatment. Artificial inoculation under controlled environmental conditions has been frequently used to study relationships among microbial agents and target pathogens. Guetsky et al. (2001), for example, determined the effects of a separate or combined application of Pichia guilliermondii and Bacillus mycoides on B. cinerea spore germination, lesion formation, and lesion development on strawberry leaves under different combinations of T/RH. In another study, Hannusch and Boland (1996) examined the interactions between B. cinerea and seven BCAs under controlled environmental conditions to determine the influence of T/RH regimes on gray mold of bean. This approach makes possible to vary and study some factors (like T, RH, and length of BCA colonization period) while others that act under natural conditions are kept constant. For instance, disinfestation of berry surfaces eliminates epiphytic microbial populations that could differ among seasons, vineyards, and position on the vine and that could interact with BCAs (Zhang et al., 2008; Massart et al., 2015). Artificial inoculation also removed the effect of varying inoculum dose and time of B. cinerea infection, which may influence BBR development (Coertze et al., 1999) and therefore BCA efficacy.
BBR development in the untreated control was influenced by the ripening stage of the inoculated berries, i.e., disease severity increased as ripening advanced. This result was expected because the susceptibility of berries to infection by B. cinerea increases during ripening due to the increase in sugar and yeast assimilable nitrogen concentration, the changes in the phenolic compounds in the skin cell walls, and the decrease in water activity at the fruit surface (Kretschmer et al., 2007; Mundy and Beresford, 2007; Deytieux-Belleau et al., 2009). Because the effect of ripening stage was not a focus of this research, it was considered a blocking factor, i.e., a variable that is of no interest but that affects BBR and is controlled for, thus leading to greater accuracy in the statistical analysis (Quinn and Keough, 2002). As a consequence, the relative control provided by BCAs was not biased by the ripening stage of the collected berries.
The response to T/RH differed among BCAs and was also affected by the length of the BCA colonization period, i.e., the number of days between BCA treatment and B. cinerea inoculation. Some of the BCAs were more influenced by different environmental conditions than others, as demonstrated by the different coefficient of variation shown in Figure 1. That environmental conditions are able to influence the survival, growth, and efficacy of BCAs against B. cinerea has been abundantly demonstrated (see the recent review of Fedele et al., 2020b). A wide range of BCAs have been reported to control B. cinerea under controlled laboratory conditions and mostly with in vitro experiments, and only few have been tested with in planta studies (Nicot, 2011). Overall, few studies have provided information about the effects of both T and RH on BCA efficacy [against B. cinerea] (Fedele et al., 2020c) and, to our knowledge, no study similar to the current one has been previously reported.
The different responses of BCAs to T/RH conditions may help explain the variability reported in other studies that applied BCAs in different vineyards, different regions, or different years (O’Neill et al., 1996; Nicot, 2011; Abbey et al., 2018). Calvo-Garrido et al. (2019), for example, recently reported that the reduction in BBR severity resulting from treatment with B. subtilis (SER) was 54 and 17% at two locations in 2015, 21% and −43% at two locations in 2016, and 46% at two locations in 2017 (pooled data). In one of these vineyards, SER provided better control than A. pullulans (BOT) in 2015 (54 vs. 46%) but the opposite was found in 2016 (21 vs. 37%). Because the materials and methods used by Calvo-Garrido et al. (2019) were consistent among years and vineyards, differences in the efficacy of SER and BOT in different seasons may be explained by different responses to weather conditions. Ojiambo and Scherm (2006) highlighted the need to identify general factors that influence the success or failure of biocontrol in plant pathology, and performed a quantitative synthesis of published research to determine the overall effectiveness of biocontrol in relation to biological factors (e.g., BCA type) and application factors (e.g., number of treatments). Our results provide further insight into understanding inconsistent results of studies conducted under different environmental conditions.
The equations developed in this work will enable researchers to predict with high accuracy the relative control of a BCA for any combination of T, RH, and time before infection by B. cinerea conidia. To our knowledge, no similar equations have been previously reported. These equations may help farmers select which BCA to use for a specific treatment based on weather conditions at the time of application and in the following days, and based on the predicted time of B. cinerea infection. An example is provided in Figure 5; when temperature and relative humidity are known for a vineyard at the time of BCA treatment and in the following days, which BCA would better express its potential to control BBR can be easily determined. Similarly, when a prediction of infection by B. cinerea is available, the BCA that would express higher potential control at that time can be determined (see Figure 4).
Temperature and humidity conditions can be recorded in the vineyard by using calibrated weather sensors that provide the data to disease prediction models (Sanna et al., 2018); the use of weather forecasts in predictive systems for disease management is also common (Bourke, 1970; Gent et al., 2013; Olatinwo and Hoogenboom, 2014), thanks to a progressive increase in their accuracy (Bauer et al., 2015) and to the availability of methods for refining forecast data using point observations (Leblanc, 2019). A mechanistic, weather-driven model for accurate prediction of B. cinerea infection risk in vineyards has been developed and validated (González-Domínguez et al., 2015; Fedele et al., 2020b). Therefore, equations developed in this work have the potential to be used in practice, but their reliability under the fluctuating temperature and humidity conditions of vineyards remains to be evaluated and their utility should be verified with field experiments (Xu, 1996; Rossi et al., 2002; Legler et al., 2012). Another limitation of this study is that it provides data only for BBR resulting from direct infection of berries by B. cinerea conidia. As noted in the Introduction, BBR can also result from latent infections established during flowering and from berry-to-berry infection caused by mycelium originating from previously infected berries within the cluster.
In this research, we did not consider the differences between the environmental requirements of B. cinerea and BCAs, because the berries were incubated at optimal conditions for B. cinerea after the B. cinerea inoculation. B. cinerea is active at a wide range of temperatures and has an optimum range of 20–25°C (Ciliberti et al., 2015). The temperature range for the optimal growth for most of the BCAs tested for B. cinerea control is 18–32°C (Fedele et al., 2020c), i.e., the ranges overlap but only partially. Consider, for example, that the optimum temperature range is 23–28°C for Aureobasidium spp. (Lima et al., 1997) and 25–30°C for Bacillus spp. (Calvo et al., 2017). Given the rapid and abundant sporulation of B. cinerea on berries at sub-optimal temperatures (Ciliberti et al., 2016), neither Aureobasidium spp. nor Bacillus spp. may be able to prevent the rapid colonization of berries by B. cinerea at temperatures below 23°C. This concern was considered by Fedele et al. (2020c) by using an environmental niche approach. Environmental niches, defined as the environmental conditions necessary for the presence of a species and for the maintenance of its population (Chesson et al., 2001), could be useful for studying the temperature and humidity conditions under which a BCA prevails over B. cinerea, and for defining the extent of environmental niche sharing between a BCA and the target pathogen. The two approaches–i.e., equations developed in the current study and the environmental niche charts from Fedele et al. (2020c)—could be usefully combined. The equations could be used to evaluate the effect of environmental conditions occurring between the time of BCA application and B. cinerea infection, and the environmental niche charts could be used to evaluate the environmental effects when both the BCA and the pathogen are present and interacting on the host surface.
Data Availability Statement
The raw data supporting the conclusions of this article will be made available by the authors, without undue reservation.
Author Contributions
VR mainly contributed to the conception and the design of the study. GF and CB carried out the experiments. VR contributed to the analysis of results. GF and VR wrote the manuscript. All authors contributed to the article and approved the submitted version.
Conflict of Interest
The authors declare that the research was conducted in the absence of any commercial or financial relationships that could be construed as a potential conflict of interest.
References
Abbey, J. A., Percival, D., Abbey, L., Asiedu, S. K., Prithiviraj, B., Schilder, A. (2018). Biofungicides as alternative to synthetic fungicide control of grey mould (Botrytis cinerea) – prospects and challenges. Biocontrol Sci. Technol. 29, 207–228. doi: 10.1080/09583157.2018.1548574
Akaike, H. (1981). Likelihood of a model and information criteria. J. Econom. 16, 3–19. doi: 10.1016/0304-4076(81)90071-3
Alessandri, S., Cavazza, F., D’Ascenzo, D., Crivelli, L., Viglione, P., Giorgino, D., et al. (2018). Experimental tests with the new biofungicide Pythium oligandrum against grey mould and sour rot on vine, grey mould on strawberry and Sclerotinia on lettuce and baby leaf. Atti, Giornate Fitopatol. Chianciano Terme (SI), Ital. 6-9 marzo 2018, Vol. Second. 347–355.
Analytis, S. (1977). Über die Relation zwischen biologischer Entwicklung und Temperatur bei phytopathogenen Pilzen. J. Phytopathol. 90, 64–76. doi: 10.1111/j.1439-0434.1977.tb02886.x
Bauer, P., Thorpe, A., Brunet, G. (2015). The quiet revolution of numerical weather prediction. Nature 525, 47–55. doi: 10.1038/nature14956
Borriss, R. (2015). “Bacillus, a plant-beneficial bacterium,” in Principles of Plant-Microbe Interactions: Microbes for Sustainable Agriculture. Ed. Lugtenberg, B. (Cham: Springer International Publishing), 379–391. doi: 10.1007/978-3-319-08575-3_40
Bourke, P. M. A. (1970). Use of weather information in the prediction of plant disease epiphytotics. Annu. Rev. Phytopathol. 8, 345–370. doi: 10.1146/annurev.py.08.090170.002021
Buck, A. L. (1981). New equations for computing vapour pressure and enhancement factor. J. Appl. Meteorol. 20, 1527–1532. doi: 10.1175/1520-0450(1981)020<1527:nefcvp>2.0.co;2
Calvo, H., Marco, P., Blanco, D., Oria, R., Venturini, M. E. (2017). Potential of a new strain of Bacillus amyloliquefaciens BUZ-14 as a biocontrol agent of postharvest fruit diseases. Food Microbiol. 63, 101–110. doi: 10.1016/j.fm.2016.11.004
Calvo-Garrido, C., Teixidó, N., Roudet, J., Viñas, I., Usall, J., Fermaud, M. (2014). Biological control of Botrytis bunch rot in Atlantic climate vineyards with Candida sake CPA-1 and its survival under limiting conditions of temperature and humidity. Biol. Control 79, 24–35. doi: 10.1016/j.biocontrol.2014.05.011
Calvo-Garrido, C., Haidar, R., Roudet, J., Gautier, T., Fermaud, M. (2018). Pre-selection in laboratory tests of survival and competition before field screening of antagonistic bacterial strains against Botrytis bunch rot of grapes. Biol. Control 124, 100–111. doi: 10.1016/j.biocontrol.2018.05.006
Calvo-Garrido, C., Roudet, J., Aveline, N., Davidou, L., Dupin, S., Fermaud, M. (2019). Microbial antagonism toward Botrytis bunch rot of grapes in multiple field tests using one Bacillus ginsengihumi strain and formulated biological control products. Front. Plant Sci. 10:105. doi: 10.3389/fpls.2019.00105
Chesson, P., Pacala, S., Neuhauser, C. (2001). “Environmental niches and ecosystem functioning,” in The Functional Consequences of Biodiversity. Eds. Kinzig, Ann, Pacala, Stephen, Tilman, David (Princeton University Press), 213–245.
Ciliberti, N., Fermaud, M., Roudet, J., Rossi, V. (2015). Environmental conditions affect Botrytis cinerea infection of mature grape berries more than the strain or transposon genotype. Phytopathology 105, 1090–1096. doi: 10.1094/PHYTO-10-14-0264-R
Ciliberti, N., Fermaud, M., Roudet, J., Languasco, L., Rossi, V. (2016). Environmental effects on the production of Botrytis cinerea conidia on different media, grape bunch trash, and mature berries. Aust. J. Grape Wine Res. 22, 262–270. doi: 10.1111/ajgw.12217
Coertze, S., Holz, G., Pathology, P., X, P. B. (1999). Surface colonization, penetration, and lesion formation on grapes inoculated fresh or after cold storage with single airborne conidia of Botrytis cinerea. Plant Dis. 83 (10), 917–924. doi: 10.1094/PDIS.1999.83.10.917
Dallyn, H., Fox, A. (1980). “Spoilage of material of reduced water activity by xerophilic fungi,” in Microbial Growth and Survival in Extreme Environments. Eds. Gould, G. H., Corry, E. L. (London and New York: Academic Press), 129–139.
Deacon, J. W., Berry, L. A. (1993). Biocontrol of soil-borne plant pathogens: concepts and their application. Pestic. Sci. 37, 417–426. doi: 10.1002/ps.2780370417
Deytieux-Belleau, C., Geny, L., Roudet, J., Mayet, V., Donèche, B., Fermaud, M. (2009). Grape berry skin features related to ontogenic resistance to Botrytis cinerea. Eur. J. Plant Pathol. 125, 551–563. doi: 10.1007/s10658-009-9503-6
Di Francesco, A., Ugolini, L., Lazzeri, L., Mari, M. (2015). Production of volatile organic compounds by Aureobasidium pullulans as a potential mechanism of action against postharvest fruit pathogens. Biol. Control 81, 8–14. doi: 10.1016/j.biocontrol.2014.10.004
Dik, A. J., Koning, G., Köhl, J. (1999). Evaluation of microbial antagonists for biological control of Botrytis cinerea stem infection in cucumber and tomato. Eur. J. Plant Pathol. 105, 115–122. doi: 10.1023/A:1008623210258
Elad, Y., Freeman, S. (2002). “Biological control of fungal plant pathogens,” in Agricultural Applications. Ed. Kempken, F. (Berlin, Heidelberg: Springer Berlin Heidelberg), 93–109. doi: 10.1007/978-3-662-03059-2_6
Elad, Y., Zimand, G., Zaqs, Y., Zuriel, S., Chet, I. (1993). Use of Trichoderma harzianum in combination or alternation with fungicides to control cucumber grey mould (Botrytis cinerea) under commercial greenhouse conditions. Plant Pathol. 42, 324–332. doi: 10.1111/j.1365-3059.1993.tb01508.x
Elad, Y., Vivier, M., Fillinger, S. (2016). “Botrytis, the Good, the Bad and the Ugly,” in Botrytis – the Fungus, the Pathogen and its Management in Agricultural Systems. Eds. Fillinger, S., Elad, Y. (Springer, Cham: Springer International Publishing), 1–15. doi: 10.1007/978-3-319-23371-0_1
Elmer, P. A. G., Michailides, T. J. (2007). “Epidemiology of Botrytis cinerea in orchard and vine crops,” in Botrytis: Biology, Pathology and Control. Eds. Elad, Y., Williamson, B., Tudzynski, P., Delen, N. (Dordrecht: Springer Netherlands), 243–272. doi: 10.1007/978-1-4020-2626-3
Elmer, P. A. G., Reglinski, T. (2006). Biosuppression of Botrytis cinerea in grapes. Plant Pathol. 55, 155–177. doi: 10.1111/j.1365-3059.2006.01348.x
Elmer, P. A. G., Hoyte, S. M., Vanneste, J. L., Reglinski, T., Wood, P. N., Parry, F. J. (2005). Biological control of fruit pathogens. New Zeal. Plant Prot. 54, 47–54.
Escribano-Viana, R., Portu, J., Garijo, P., Gutiérrez, A. R., Santamaría, P., López-Alfaro, I., et al. (2018). Evaluating a preventive biological control agent applied on grapevines against Botrytis cinerea and its influence on winemaking. J. Sci. Food Agric. 98, 4517–4526. doi: 10.1002/jsfa.8977
Fedele, G., Bove, F., González-Domínguez, E., Rossi, V. (2020a). A generic model accounting for the interactions among pathogens, host plants, biocontrol agents, and the environment, with parametrization for Botrytis cinerea on grapevines. Agronomy 10:222. doi: 10.3390/agronomy10020222
Fedele, G., González-Domínguez, E., Delière, L., Díez-navajas, A. M., Rossi, V. (2020b). Consideration of latent infections improves the prediction of Botrytis bunch rot severity in vineyards. Plant Dis. 104 (5), 1291–1297. doi: 10.1094/PDIS-11-19-2309-RE
Fedele, G., González-Domínguez, E., Rossi, V. (2020c). “Influence of environment on the biocontrol of Botrytis cinerea: a systematic literature review,” in How Research Can Stimulate the Development of Commercial Biological Control Against Plant Diseases. Eds. De Cal, A., Melgarejo, P., Magan, N. (Switzerland: Springer Nature).
Fedele, G., González-Domínguez, E., Si Ammour, M., Languasco, L., Rossi, V. (2020d). Reduction of Botrytis cinerea colonization of and sporulation on bunch trash. Plant Dis. 104, 808–816. doi: 10.1094/PDIS-08-19-1593-RE
Fernández-Ortuño, D., Torés, J. A., Chamorro, M., Pérez-García, A., de Vicente, A. (2016). Characterization of resistance to six chemical classes of site-specific fungicides registered for gray mold control on strawberry in Spain. Plant Dis. 100, 2234–2239. doi: 10.1094/pdis-03-16-0280-re
Gent, D. H., Mahaffee, W. F., McRoberts, N., Pfender, W. F. (2013). The use and role of predictive systems in disease management. Annu. Rev. Phytopathol. 51, 267–289. doi: 10.1146/annurev-phyto-082712-102356
González-Domínguez, E., Caffi, T., Ciliberti, N., Rossi, V. (2015). A mechanistic model of Botrytis cinerea on grapevines that includes weather, vine growth stage, and the main infection pathways. PloS One 10, 1–23. doi: 10.1371/journal.pone.0140444
González-Domínguez, E., Fedele, G., Caffi, T., Delière, L., Sauris, P., Gramaje, D., et al. (2019). A network meta-analysis provides new insight into fungicide scheduling for the control of Botrytis cinerea in vineyards. Pest Manage. Sci. 75, 324–332. doi: 10.1002/ps.5116
Guetsky, R., Shtienberg, D., Elad, Y., Dinoor, A. (2001). Combining biocontrol agents to reduce the variability of biological control. Phytopathology 91, 621–627. doi: 10.1094/PHYTO.2001.91.7.621
Haidar, R., Fermaud, M., Calvo-Garrido, C., Roudet, J., Deschamps, A. (2016). Modes of action for biological control of Botrytis cinerea by antagonistic bacteria. Phytopathol. Mediterr. 33, 13–34. doi: 10.14601/Phytopathol
Hannusch, D., Boland, G. J. (1996). Interactions of air temperature, relative humidity and biological control agents on grey mold of bean. Phytopathology 102, 133–142. doi: 10.1094/Phyto-86-156
Harman, G. E. (2000). Myths and dogmas of biocontrol: changes in perceptions derived from research on Trichoderma harzianum T-22. Biol. Control 84, 377–393. doi: 10.1094/PDIS.2000.84.4.377
Hill, G. N., Beresford, R. M., Evans, K. J. (2010). Tools for accurate assessment of Botrytis bunch rot (Botrytis cinerea) on wine grapes. New Zeal. Plant Prot. 63, 174–181. doi: 10.30843/nzpp.2010.63.6560
Jackson, M., Whipps, M., Lynch, M. (1991). Effects of temperature, pH and water potential on growth of four fungi with disease biocontrol potential. World J. Microbiol. Biotechnol. 7, 494–501. doi: 10.1007/BF00303376
Jacometti, M. A., Wratten, S. D., Walter, M. (2010). Review: Alternatives to synthetic fungicides for Botrytis cinerea management in vineyards. Aust. J. Grape Wine Res. 16, 154–172. doi: 10.1111/j.1755-0238.2009.0067.x
Jarvis, W. R. (1977). Botryotinia and Botrytis species: taxonomy, physiology, and pathogenicity (Ontario, Canada: Monograph, Research Branch Canada Department of Agriculture).
Kredics, L., Antal, Z., Manczinger, L., Szekeres, A., Kevei, F., Nagy, E. (2003). Influence of environmental parameters on Trichoderma strains with biocontrol potential. Food Technol. Biotechnol. 41, 37–42.
Kretschmer, M., Kassemeyer, H. H., Hahn, M. (2007). Age-dependent grey mould susceptibility and tissue-specific defence gene activation of grapevine berry skins after infection by Botrytis cinerea. J. Phytopathol. 155, 258–263. doi: 10.1111/j.1439-0434.2007.01216.x
Laing, S. A. K., Deacon, J. W. (1991). Video microscopical comparison of mycoparasitism by Pythium oligandrum, P. nunn and an unnamed Pythium species. Mycol. Res. 95, 469–479. doi: 10.1016/S0953-7562(09)80848-4
Leblanc, A. (2019). Method and system for refining weather forecasts using point observations, U.S. Patent No. 10,495,785.
Legler, S. E., Caffi, T., Rossi, V. (2012). A nonlinear model for temperature-dependent development of Erysiphe necator chasmothecia on grapevine leaves. Plant Pathol. 6, 96–105. doi: 10.1111/j.1365-3059.2011.02498.x
Leifert, C., Li, H., Chidburee, S., Hampson, S., Workman, S., Sigee, D., et al. (1995). Antibiotic production and biocontrol activity by Bacillus subtilis CL27 and Bacillus pumilus CL45. J. Appl. Bacteriol. 78, 97–108. doi: 10.1111/j.1365-2672.1995.tb02829.x
Leroux, P. (2007). “Chemical Control of Botrytis and its Resistance to Chemical Fungicides,” in Botrytis: Biology, Pathology and Control. Eds. Elad, Y., Williamson, B., Tudzynski, P., Delen, N. (Dordrecht: Springer Netherlands), 195–222. doi: 10.1007/978-1-4020-2626-3_12
Lima, G., Ippolito, A., Nigro, F., Salerno, M. (1997). effectiveness of Aureobasidium pullulans and Candida oleophila against postharvest strawberry rots. Postharvest Biol. Technol. 10, 169–178. doi: 10.1016/S0925-5214(96)01302-6
Lin, L., II (1989). A Concordance Correlation Coefficient to evaluate reproducibility. Biometrics 45, 255–268. doi: 10.2307/2532051
Lorenz, D. H., Eichhorn, K. W., Bleiholder, H., Klose, R., Meier, U., Weber, E. (1995). Phenological growth stages of the grapevine (Vitis vinifera L. ssp. vinifera)- Codes and descriptions according to the extended BBCH scale. Aust. J. Grape Wine Res. 1, 100–103. doi: 10.1111/j.1755-0238.1995.tb00085.x
Madden, L. V., Hughes, G., van den Bosch, F. (2007). The Study of Plant Disease Epidemics (St Paul, MN: The American Phytopathological Society). doi: 10.1094/9780890545058
Massart, S., Margarita, M. M., Jijakli, M. H. (2015). Biological control in the microbiome era: Challenges and opportunities. Biol. Control 89, 98–108. doi: 10.1016/j.biocontrol.2015.06.003
Mitchell, J. K., Jeger, J. M., Taber, R. A. (1987). The effect of temperature on colonization of Cercosporidium personatum leafspot of peanuts by the hyperparasite Dicyma pulvinata. Agric. Ecosyst. Environ. 18, 325–332. doi: 10.1016/0167-8809(87)90098-3
Mundy, D. C., Beresford, R. M. (2007). Susceptibility of grapes to Botrytis cinerea in relation to berry nitrogen and sugar concentration. New Zeal. Plant Prot. 60, 123–127. doi: 10.30843/nzpp.2007.60.4636
Nair, N., Emmet, R., Parker, P. E. (1988). Some factors predisposing grape berries to infection by Botrytis cinerea. New Zeal. J. Exp. Agric. 16, 257–263. doi: 10.1080/03015521.1988.10425648
Nash, J. E., Sutcliffe, J. V. (1970). River flow forecasting through conceptual models part I - A discussion of principles. J. Hydrol. 10, 282–290. doi: 10.1016/0022-1694(70)90255-6
Nicot, P. C., Stewart, A., Bardin, M., Elad, Y. (2016). “Biological control and biopesticide suppression of Botrytis-incited diseases,” in Botrytis – the Fungus, the Pathogen and its Management in Agricultural Systems. Eds. Fillinger, S., Elad, Y. (Cham: Springer International Publishing), 165–187. doi: 10.1007/978-3-319-23371-0_9
Nicot, P. C. (2011). Classical and augmentative biological control against diseases and pests : critical status analysis and review of factors (IOBC/WPRS Bulletin).
Ojiambo, P. S., Scherm, H. (2006). Biological and application-oriented factors influencing plant disease suppression by biological control: A meta-analytical review. Phytopathology 96, 1168–1174. doi: 10.1094/PHYTO-96-1168
Olatinwo, R., Hoogenboom, G. (2014). “Weather-based pest forecasting for efficient crop protection,” in Integrated Pest Management: Current Concepts and Ecological Perspective (Waltham, MA: Academic Press), 59–78. doi: 10.1016/B978-0-12-398529-3.00005-1
O’Neill, T. M., Niv, A., Elad, Y., Shtienberg, D. (1996). Biological control of Botrytis cinerea on tomato stem wounds with Trichoderma harzianum. Eur. J. Plant Pathol. 102, 635–643. doi: 10.1007/BF01877244
Parafati, L., Vitale, A., Restuccia, C., Cirvilleri, G. (2015). Biocontrol ability and action mechanism of food-isolated yeast strains against Botrytis cinerea causing post-harvest bunch rot of table grape. Food Microbiol. 47, 85–92. doi: 10.1016/j.fm.2014.11.013
Pertot, I., Longa, C. M., Prodorutti, D., Michelon, L., Savazzini, F. (2013). Trichoderma atroviride SC1 for biocontrol of fungal diseases in plants, U.S. Patent No. 8,431,120.
Pertot, I., Giovannini, O., Benanchi, M., Caffi, T., Rossi, V., Mugnai, L. (2017). Combining biocontrol agents with different mechanisms of action in a strategy to control Botrytis cinerea on grapevine. Crop Prot. 97, 85–93. doi: 10.1016/j.cropro.2017.01.010
Quinn, G., Keough, M. (2002). Experimental design and data analysis for biologists (Cambridge, United Kingdom: Cambridge University Press). doi: 10.1016/S0022-0981(02)00278-2
Rosenheim, J. A., Kaya, H. K., Ehler, L. E., Marois, J. J., Jaffe, B. A. (1995). Intraguild predation among biological-control agents: theory and evidence. Biol. Control 5, 303–335. doi: 10.1006/bcon.1995.1038
Rossi, V., Pattori, E., Ravanetti, A., Giosuè, S. (2002). Effect of constant and fluctuating temperature regimes on sporulation of four fungi causing head blight of wheat. J. Plant Pathol. 84, 95–105. doi: 10.4454/jpp.v84i2.1092
Rossi, V., Caffi, T., Salinari, F. (2012). Helping farmers face the increasing complexity of decision-making for crop protection. Phytopathol. Mediterr. 51, 457–479. doi: 10.14601/Phytopathol_Mediterr-11038
Rotolo, C., De Miccolis Angelini, R. M., Dongiovanni, C., Pollastro, S., Fumarola, G., Di Carolo, M., et al. (2018). Use of biocontrol agents and botanicals in integrated management of Botrytis cinerea in table grape vineyards. Pest Manage. Sci. 74, 715–725. doi: 10.1002/ps.4767
Sanna, F., Calvo, A., Deboli, R., Merlone, A. (2018). Vineyard diseases detection: a case study on the influence of weather instruments’ calibration and positioning. Meteorol. Appl. 25.2, 228–235. doi: 10.1002/met.1685
Schoene, P. (2002). Ulocladium atrum as an antagonist of grey mould (Botrytis cinerea) in grapevine. Diss. Universitäts-und Landesbibliothek Bonn. Available at: http://hss.ulb.uni-bonn.de/2002/0149/0149.pdf
Tracy, E. F. (2014). The promise of biological control for sustainable agriculture : a stakeholder- based analysis. J. Sci. Poly. Gov. 5.
Verger, P. J. P., Boobis, A. R. (2013). Reevaluate pesticides for food security and safety. Science 341, 717–718. doi: 10.1126/science.1241572
Whipps, J. M. (1997). “Developments in the biological control of soil-borne plant pathogens,” in Advances in botanical research. Ed. Callow, J. A. (San Diego, CA, USA: Academic Press), 1–134. doi: 10.1016/S0065-2296(08)60119-6
Williamson, B., Tudzynski, B., Tudzynski, P., Van Kan, J. A. L. (2007). Botrytis cinerea: The cause of grey mould disease. Mol. Plant Pathol. 8, 561–580. doi: 10.1111/j.1364-3703.2007.00417.x
Xu, X., Robinson, J., Jeger, M., Jeffries, P. (2010). Using combinations of biocontrol agents to control Botrytis cinerea on strawberry leaves under fluctuating temperatures. Biocontrol Sci. Technol. 20, 359–373. doi: 10.1080/09583150903528114
Xu, X. M. (1996). On estimating non-linear response of fungal development under fluctuating temperatures. Plant Pathol. 45, 163–171. doi: 10.1046/j.1365-3059.1996.d01-134.x
Keywords: gray mold, biological control, biological control agents, integrated pest management, modeling, Vitis vinifera
Citation: Fedele G, Brischetto C and Rossi V (2020) Biocontrol of Botrytis cinerea on Grape Berries as Influenced by Temperature and Humidity. Front. Plant Sci. 11:1232. doi: 10.3389/fpls.2020.01232
Received: 05 May 2020; Accepted: 28 July 2020;
Published: 14 August 2020.
Edited by:
Christina Cowger, Plant Science Research Unit (USDA-ARS), United StatesReviewed by:
Antonieta De Cal, National Institute for Agricultural and Food Research and Technology (INIA), SpainDavid Ruano-Rosa, Instituto Tecnológico Agrario de Castilla y León, Spain
Copyright © 2020 Fedele, Brischetto and Rossi. This is an open-access article distributed under the terms of the Creative Commons Attribution License (CC BY). The use, distribution or reproduction in other forums is permitted, provided the original author(s) and the copyright owner(s) are credited and that the original publication in this journal is cited, in accordance with accepted academic practice. No use, distribution or reproduction is permitted which does not comply with these terms.
*Correspondence: Vittorio Rossi, dml0dG9yaW8ucm9zc2lAdW5pY2F0dC5pdA==