- Soybean Research Institution, National Center for Soybean Improvement, Key Laboratory of Biology and Genetics and Breeding for Soybean, Ministry of Agriculture, State Key Laboratory of Crop Genetics and Germplasm Enhancement, Nanjing Agricultural University, Nanjing, China
Seed-weight is one of the most important traits determining soybean yield. Hence, it is prerequisite to have detailed understanding of the genetic basis regulating seed-weight for the development of improved cultivars. In this regard, the present study used high-density interspecific linkage map of NJIR4P recombinant inbred population evaluated in four different environments to detect stable Quantitative trait loci (QTLs) as well as mine candidate genes for 100-seed weight. In total, 19 QTLs distributed on 12 chromosomes were identified in all individual environments plus combined environment, out of which seven were novel and eight are stable identified in more than one environment. However, all the novel QTLs were minor (R2 < 10%). The remaining 12 QTLs detected in this study were co-localized with the earlier reported QTLs with narrow genomic regions, and out of these only 2 QTLs were major (R2 > 10%) viz., qSW-17-1 and qSW-17-4. Beneficial alleles of all identified QTLs were derived from cultivated soybean parent (Nannong493-1). Based on Protein ANalysis THrough Evolutionary Relationships, gene annotation information, and literature search, 29 genes within 5 stable QTLs were predicted to be possible candidate genes that might regulate seed-weight/size in soybean. However, it needs further validation to confirm their role in seed development. In conclusion, the present study provides better understanding of trait genetics and candidate gene information through the use high-density inter-specific bin map, and also revealed considerable scope for genetic improvement of 100-seed weight in soybean using marker-assisted breeding.
Introduction
Soybean (Glycine max L. Merr.) is one of the most economically important crop being rich source of both edible oil and protein as well as has significant role in health, biofuel, and soil fertility improvement (Kulkarni et al., 2016). In China, soybean production has continuously declined with considerable low yield increase in the past 50 years (Liu et al., 2018). Moreover, China imports >80% of soybean for their total domestic use; hence, it is prerequisite to increase the domestic production of soybean to make country self-sufficient (Liu et al., 2018). Different yield-related traits are targeted by plant breeders to increase soybean production. In this context, seed-weight is one of the most important yield-related trait for increasing seed yield in soybean; however, it is a complex quantitative trait governed by polygenes and are highly influenced by environment, which makes it selection difficult for plant breeders (Yao et al., 2015). Furthermore, seed weight/size determines the specific soy-based food product that can be made from soybean (Cui et al., 2004; Gandhi, 2009). For instance, small-seeded cultivars are suitable for fermented soybean (natto) and sprout production, whereas large-seeded cultivars are used for boiled soybean (nimame), green soybean (edamame), soymilk, and soybean curd (tofu) (Liang et al., 2016; Teng et al., 2017; Wu et al., 2018). In addition, seed weight/size influences germination ability and seedling vigor, which in turn determines the competitive ability of the seedling for light, nutrient resources, and stress tolerance (Coomes and Grubb, 2003; Gomez, 2004; Haig, 2013).
Seed weight is one of the traits that was altered during domestication (Lee et al., 2011; Han et al., 2016). During domestication process from wild species to cultivated soybean, selecting desirable agronomic traits to keep achieving high yield allows many genes to be either directly selected or filtered out, resulting in a significant reduction of genetic diversity in soybean gene pool (Guo et al., 2010; Tang et al., 2010). Hyten et al. (2006) suggested that 50% of the genetic diversity and 81% of the rare alleles have been lost during domestication and that 60% of the genes show significant changes in allele frequency as a result of soybean domestication. It has been reported that wild soybean (Glycine soja) is an important source of genes for higher yield and related traits, quality, as well as biotic and abiotic stresses (Zhou Z. et al., 2015). Thus, it is necessary to broaden the gene pool in soybean breeding from diverse sources, especially from wild soybean (G. soja). The seed of cultivated soybean (G. max) is heavier and bigger compared to the wild accessions (Yu et al., 2017). Both wild and cultivated soybean belong to the same genus Glycine (Kim M.Y. et al., 2010), with the former having higher level of genetic diversity, as well as better adaptation to harsh environments (Stupar, 2010; Qiu et al., 2013; Zhang et al., 2017). Thus, G. soja holds great potential to improve its agriculturally important domesticated relative (G. max), beyond what is currently known (Kofsky et al., 2018). For example, comparative genomics, transcriptomics, and bioinformatics application have revealed the role of domestication in the seed weight of soybean (Lu et al., 2016; Zhao et al., 2016; Yu et al., 2017).
Quantitative trait loci (QTL) mapping using domesticated and wild progenitors have been reported to be the useful means for identifying genomic regions involved in morphological and physiological changes that distinguish crops from their wild relatives (Paterson, 2010). The wild soybean has been recently reported to be an important source of QTLs contributing to the increase in seed size in soybean. For example, Lu et al. (2017) identified a phosphatase 2C protein (PP2C-1) allele from wild soybean underlying a QTL that enhances the 100-seed weight in soybean. Although many genetic studies have been carried out in the past decades to identify QTLs for seed weight/size using different types of DNA markers through QTL mapping analyses. Currently, there are a total of 325 QTLs identified for seed-weight/size available on SoyBase1, and most of them are minor and not validated (Liu et al., 2018). In addition, knowledge for the molecular mechanism of soybean seed weight is very limited compared to other crops like rice (Wang et al., 2015; Liu et al., 2018). Till date, only two genes related to seed weight/size have been isolated from soybean viz., ln (Jeong et al., 2012) and PP2C-1 (Lu et al., 2017). Hence, it is prerequisite to identify stable QTLs for seed-weight as well as mine candidate genes underlying them to facilitate understanding of the molecular mechanisms regulating seed-weight in soybean (Kato et al., 2014). Furthermore, only few mapping populations derived from wild and domesticated soybean crosses have been used for QTLs detection of seed-weight in soybean1. Also, most of the previous studies have used low-throughput markers (such as SSR) for QTL identification of seed-weight in soybean (Panthee et al., 2005; Gai et al., 2007; Kato et al., 2014; Kulkarni et al., 2016; Wu et al., 2018). These marker systems have low resolution and larger confidence interval compared with high-density SNP markers (Hyten et al., 2010; Xu et al., 2013; Lu et al., 2017) that were revealed to be useful for high-throughput QTL mapping. Also, most of the published reports did not mine the candidate genes for seed-weight (Zhang et al., 2004; Kato et al., 2014; Kulkarni et al., 2016; Wu et al., 2018).
Therefore, by keeping the above into view the present study used high-density inter-specific genetic map of the recombinant inbred line (RIL) population (NJIR4P) derived from a cross between Nannong493-1 (G. max) and PI 342618B (G. soja) that was evaluated in multiple environments to map stable QTLs as well as mine possible candidate genes underlying 100-seed weight in soybean. Using interspecific RIL population with wide range of variation in 100-seed weight has greatly assisted in the detection of more number of major and minor QTLs regulating 100-seed weight in soybean. The use of this RIL population could enhance our understanding of molecular mechanism, evolution, and genetic regulation of seed weight in soybean. The results of the present study will be helpful in marker-assisted breeding (MAB) for developing soybean varieties with improved seed-weight.
Materials and Methods
Plant Materials and Experimental Conditions
An interspecific RIL population consisting of 161 lines were derived through single seed descent (SSD) method by crossing a soybean cultivar Nannong493-1 (G. max) with wild soybean line PI 342618B (G. soja), and this RIL population were named as NJIR4P. The Nannong493-1 parent has a higher 100-seed weight with an average value of 18.02 ± 2.60 g, whereas PI 342618B is an annual wild soybean with low 100-seed weight (1.4 g) (Xie et al., 2014). The RILs (F6:9–F6:11) along with their parents were planted in four different environments viz., Fengyang Experimental Station, Chuzhou, Anhui Province (Latitude 32°87′ N; Longitude 117°56′ E), in 2012 (FY2012), and Jiangpu Experimental Station, Nanjing, Jiangsu Province (JP) (Latitude 33°03′ N; Longitude 118°63′ E) in 2012, 2013, and 2014 (JP2012, JP2013, and JP2014). Soybean lines were planted in a single line plot of 1 m in length and 0.5 m in width in a randomized complete block design (RCBD) with three replications. Standard cultural and agronomic practices were followed in each environment (Lihua, 1982; Liu et al., 2008).
Phenotypic Analysis of 100-Seed Weight
Each row of the RILs and their parents were harvested, threshed, and dried to a suitable moisture. Four-hundred healthy dried seeds from each row were selected randomly for measurement of 100-seed weight. The 100-seed weight, i.e., weight of 100 seeds at 13% moisture content was measured by electronic balance and were repeated four times. Seed-weight was calculated for all the three replication and mean value was used for analysis. Analysis of variance (ANOVA) in each environment and combined environments (CEs) were conducted using the general linear model (GLM) and mixed procedure, respectively, in SAS (SAS Institute, 2010. SAS/STAT software version 9.2; SAS Institute Inc., Cary, NC, United States). The broad-sense heritability (H2) was calculated for both individual environments plus CE following the procedure of Hanson et al. (1956). Also genotypic coefficients of variation (GCV) was calculated by using the following formula proposed by Singh (1985): , where is the genotypic standard deviation in each environment while μ is the mean value of 100-seed weight.
QTL Mapping Analysis
In the present study, an inter-specific high-density bin map earlier developed by Wang et al. (2016) by using RAD-sequencing approach for this population was used for QTL mapping. This bin map consisted of 4,354 bin markers that were derived from 80,995 single-nucleotide polymorphisms (SNPs) distributed on all 20 soybean linkage groups/chromosomes, and has a total length of 2,136.717 cM. The average number of markers per linkage group and length of linkage group was 218 and 106.84 cM, respectively, with mean distance between bins as 0.49 cM (Supplementary Table 1). Among the NJRI4P-RIL, 46.07% were inherited their genetic background from Nannong493-1, 50.06% were from PI 342618B, and the remaining 3.87% were heterozygous genotypes. The segregation ratios of each bin marker were calculated, and only few significant segregation distortion regions were identified. In NJRI4P, out of 4,354 bin markers only 1 bin showed extreme segregation distortion at P < 0.0001 on chromosome 2, and 2 bins exhibited segregation distortion at P < 0.0005 on chromosomes 7 and 19, whereas the remaining bin markers did not show significant segregation distortion (Wang et al., 2016).
The QTL analysis was performed via WinQTLCart 2.5 software (Wang et al., 2007). For the WinQTLCart 2.5 software, the model of composite interval mapping (CIM) was used with a 10 cM window at a walking speed of 1 cM. The LOD threshold was calculated using 1,000 permutations for an experimental-wise error rate of P = 0.05 to determine whether the QTL was significantly associated with (Churchill and Doerge, 1994). The CIM model was also used to identify the main QTLs in the CE with the same parameters as used in the individual environment. Mapping for CE was done using the Best Linear Unbiased Prediction (BLUP) values for each independent environment and across all environments by using the lme4 package in R (Bates et al., 2014). QTLs detected in different environments at the same, adjacent, or overlapping marker intervals were considered the same QTL (Palomeque et al., 2009, 2010; Qi et al., 2017). QTL naming was done following the nomenclature of McCouch et al. (1997), thus starting with “q,” followed by an abbreviation of the trait name (SW, seed weight) and the name of the chromosome, followed by the number of QTL detected on the same chromosome. The QTL genetic and physical positions based on the flanking markers with known positions were used to retrieve a number of earlier reported QTLs available on SoyBase2 (Williams 82.a1.v.1.1). QTLs that did not overlap with reported QTLs in both genetic and physical positions were considered as new in this study. The QTLs identified in the individual environments were presented in Venn diagram using an online tool3 (Oliveros, 2007).
Candidate Gene Prediction Analysis
In this study, QTL was considered as stable when detected in at least two environments. Model genes within the genomic physical position of the stable QTLs on the soybean genome (Williams 82.a1.v.1.1) available at SoyBase3 were downloaded. Gene ontology (GO) enrichment analysis was conducted for all the genes within each QTL region using online GO tool4. Gene classification was then carried out using Web Gene Ontology (WeGO) Annotation Plotting tool, Version 2.05 (Ye et al., 2018). The predicted candidate genes were further subjected to Protein ANalysis THrough Evolutionary Relationships (PANTHER) Classification System to classify proteins (and their genes) in order to facilitate high-throughput analysis according to family and subfamily, molecular function, biological process, and pathway6. The selected candidate genes structure analysis was carried out using http://gsds.cbi.pku.edu.cn/ (Hu et al., 2014).
Results
Phenotypic Variation of 100-Seed Weight
Mean, range, standard deviation, skewness, kurtosis, H2, and GCV among the RILs and their parents across the four different environments (FY2012, JP2012, JP2013, and JP2014), and CE are presented in Table 1. The average 100-seed weight of the Nannong493-1, PI 483460B, and RILs were 16.49–19.09, 1.23–1.40, and 1.37–11.84 g, respectively, across all the studied environments (Table 1). However, there was no clear transgressive segregation among the RIL (Figure 1). Furthermore, ANOVA were performed to evaluate the effects of genotypes/lines (G), environment (E), and their interactions (GE) on 100-seed weight. The RILs showed highly significant differences (P < 0.01) for 100-seed weight in the individual environments. ANOVA for CE showed that G, E, and GE contributed significant variation to seed weight among the RILs of NJIR4P population (Supplementary Table 2). Hence, significant influence of E and GE on 100-seed weight of soybean suggests that seed-weight is a complex quantitative trait governed by polygenes. Moreover, high H2 values in individual as well as CEs varying from 88.27 to 97.23% coupled with high GCV (>20%) suggest that considerable proportion of phenotypic variation of 100-seed weight is due to genotype.
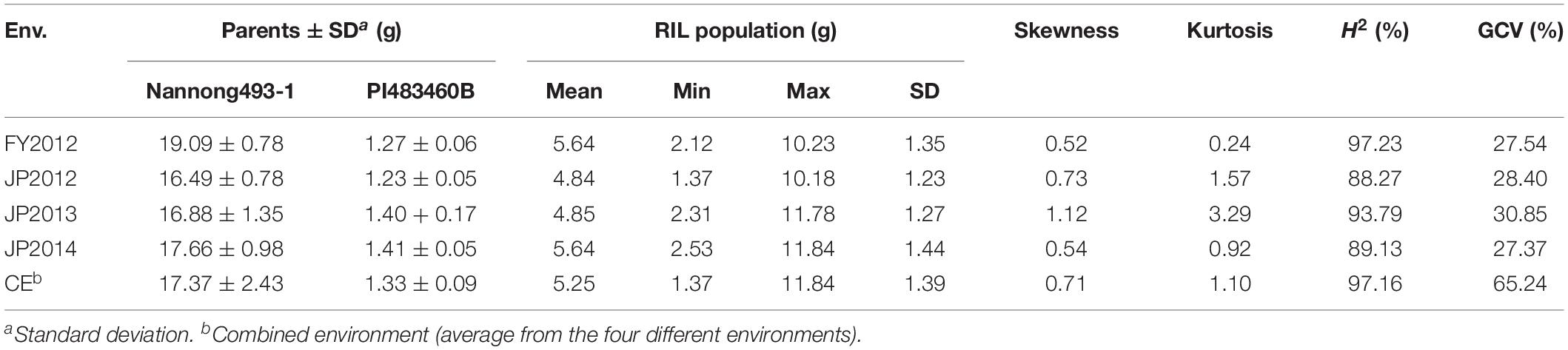
Table 1. Descriptive statistics, broad-sense heritability (H2), and genotypic coefficient of variation (GCV) of 100-seed weight in NJIR4P RIL population and two parental lines viz., Nannong493-1 and PI 483460B.
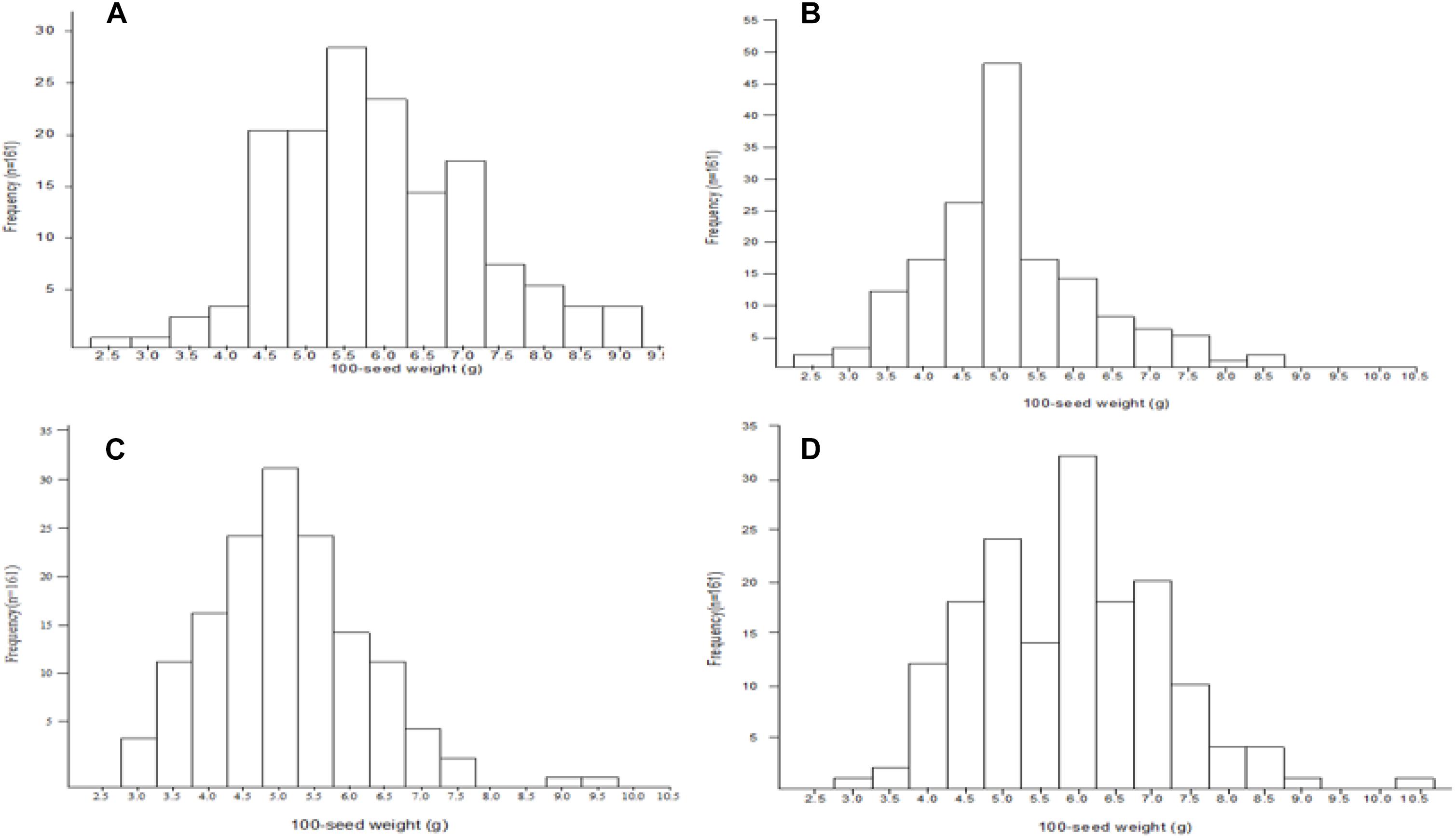
Figure 1. Frequency distribution of 100-seed weight QTLs among the NJIR4P-RIL in the various environments (A, B, C, and D for FY2012, JP2012, JP2013, and JP2014, respectively).
QTL Mapping of 100-Seed Weight Using CIM
A total of 19 QTLs associated with seed-weight were identified in all the individual environments plus CE distributed on 12 of the 20 chromosomes of soybean, and explaining 4.22–13.20% of the phenotypic variation (R2) (Figure 2 and Table 2). Out of these 19 QTLs, 7 were identified for the first time viz., qSW-2-1, qSW-2-2, qSW-2-3, qSW-6-1, qSW-19-1, qSW-19-2, and qSW-19-3, and remaining 12 QTLs have been previously reported in reference to soybean genome GmComposite2003 (SoyBase) (Table 2). The highest number of four QTLs are present on Chr17 followed by three on each Chr2 and Chr19, and the rest 10 chromosomes contain one or two QTLs each. Of the 19 QTLs identified only two are major (R2 > 10%) viz., qSW-17-1 and qSW-17-4 both are located on Chr17, and the remaining 17 QTLs identified are minor (R2 < 10%). Notably, the most prominent QTL with the highest LOD score (7.28) was identified in a 23.01 cM region on Chr17, named as qSW-17-1, explaining 13.20% of phenotypic variation. Five QTLs viz., qSW-2-1, qSW-2-2, qSW-4-2, qSW-14-1, and qSW-17-4 were identified in more than one individual environments (Figure 3), and three more QTLs viz., qSW-4-1, qSW-17-1, and qSW-17-3 were detected in one individual environment plus CE. Interestingly both major QTLs located on Chr17 (qSW-17-1 and qSW-17-4) were detected in more than one environments, suggesting the stability and consistency of these QTLs (Table 2). The remaining 11 QTLs were environment-specific QTLs identified in only one specific environment (Table 2). Out of these eight stable QTLs, two were novel QTLs identified for the first time (qSW-2-1 and qSW-2-2). All the QTLs identified for 100-seed weight in the RILs population displayed positive additive effects with positive alleles from higher seed-weight parent (Nannong493-1). Moreover, all the novel QTLs identified were minor (R2 < 10%), thus, none of the novel QTLs detected in this study was major. However, most of the previously detected QTLs were identified in a narrowed physical genomic region (Table 2). The highest number of QTLs for 100-seed weight were identified on Chr17, Chr2, and Chr19 suggest the important role of these chromosomes in governing the inheritance of seed-weight in soybean.
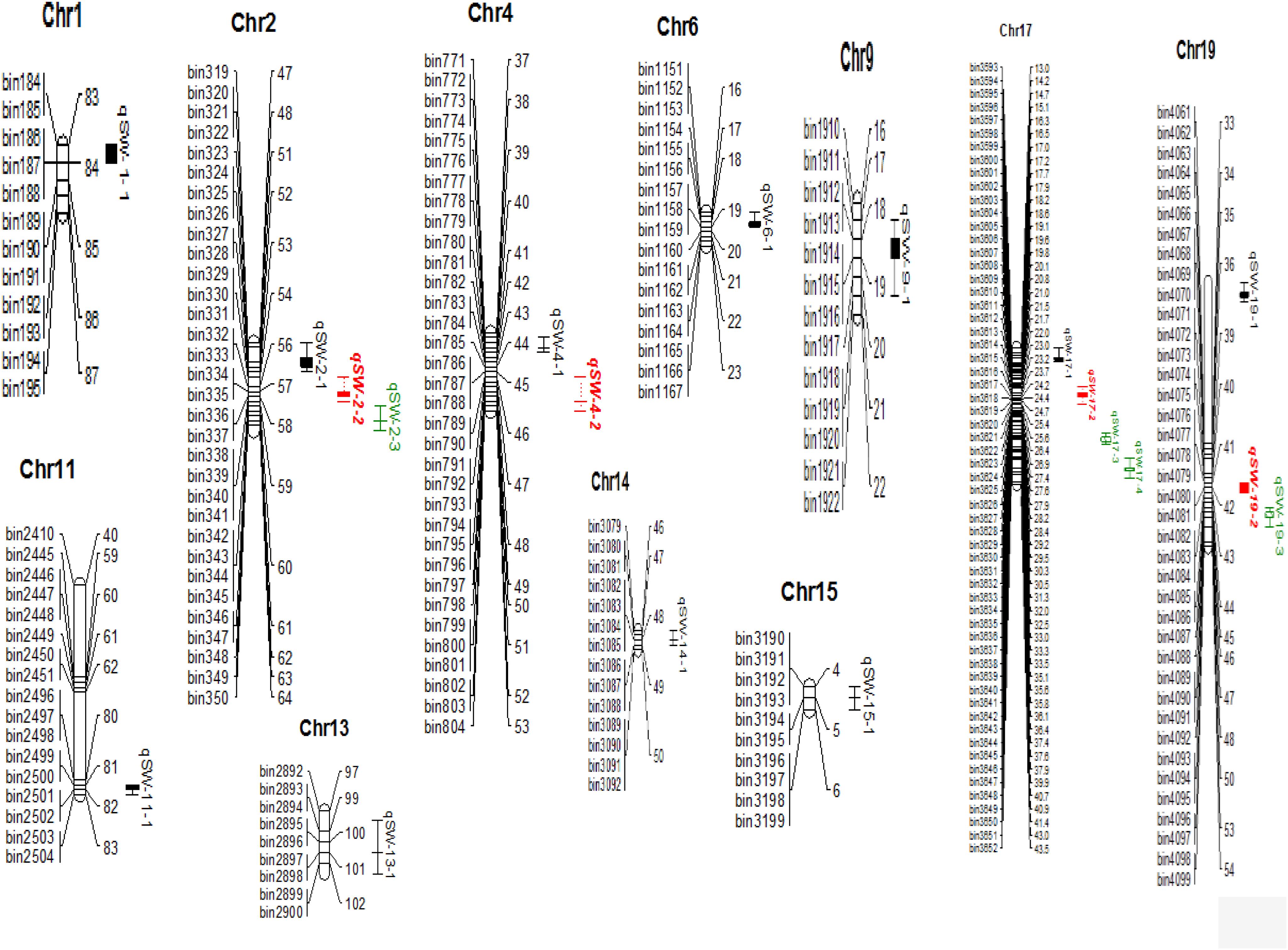
Figure 2. 100-seed weight QTLs identified in NJIR4P-RIL (complete map is not presented here, it represents only the portion where QTLs have been identified). Right side of chromosomes indicates the interval distance between markers using cM (centiMogan) as the unit; the left side of chromosomes indicates Bin-DNA markers.
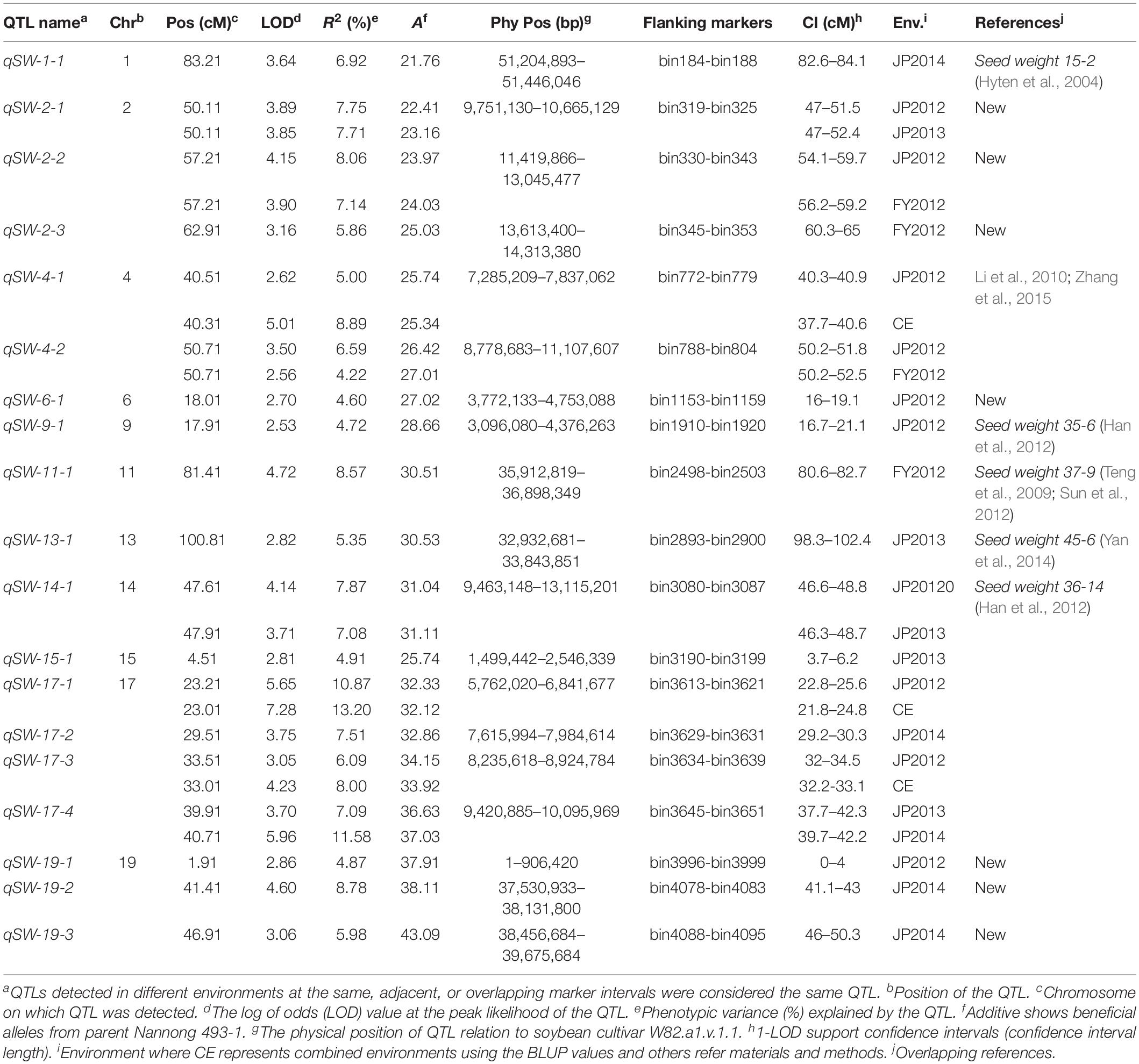
Table 2. Main QTLs identified in an interspecific RIL population (NJIR4P) in four different environments (FY2012, JP2012, JP2013, and JP2014) and combined environment (CE).
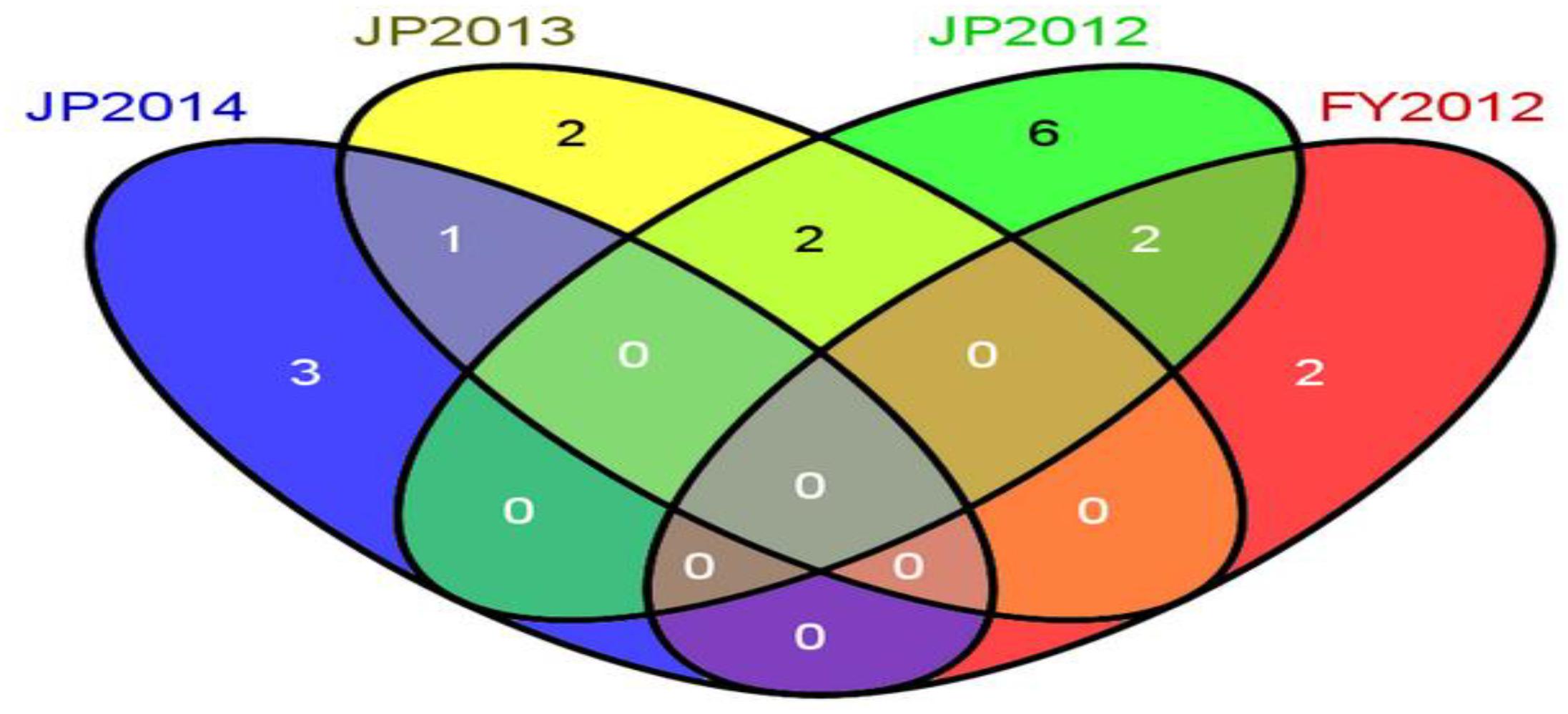
Figure 3. QTLs identified in different individual environments (FY2012, JP2012, JP2013, and JP2014).
Gene Ontology and Candidate Gene Prediction Within Stable QTLs
Based on the number of individual environments QTL were detected, we selected five stable QTLs identified in more than one individual environments viz., qSW-2-1, qSW-2-2, qSW-4-2, qSW-14-1, and qSW-17-4 for GO and candidate gene prediction analysis. Within the physical genomic interval of qSW-2-1, qSW-2-2, qSW-4-2, qSW-14-1, and qSW-17-4, the 91, 100, 92, 137, and 70 model genes were present, respectively, and these genes as well as their gene annotation were downloaded from Soybase7. After GO enrichment analysis, we employed WeGO web-based tool to visualize the biological process, molecular function, and cellular component main categories (Figure 4). In all the five stable QTLs viz., qSW-2-1, qSW-2-2, qSW-4-2, qSW-14-1, and qSW-17-4, higher percentage of genes were associated with the terms cell part, cell, organelle, catalytic activity, binding, metabolic process, and cellular process (Figure 4). This suggests an important role of these terms in the seed development of soybean.
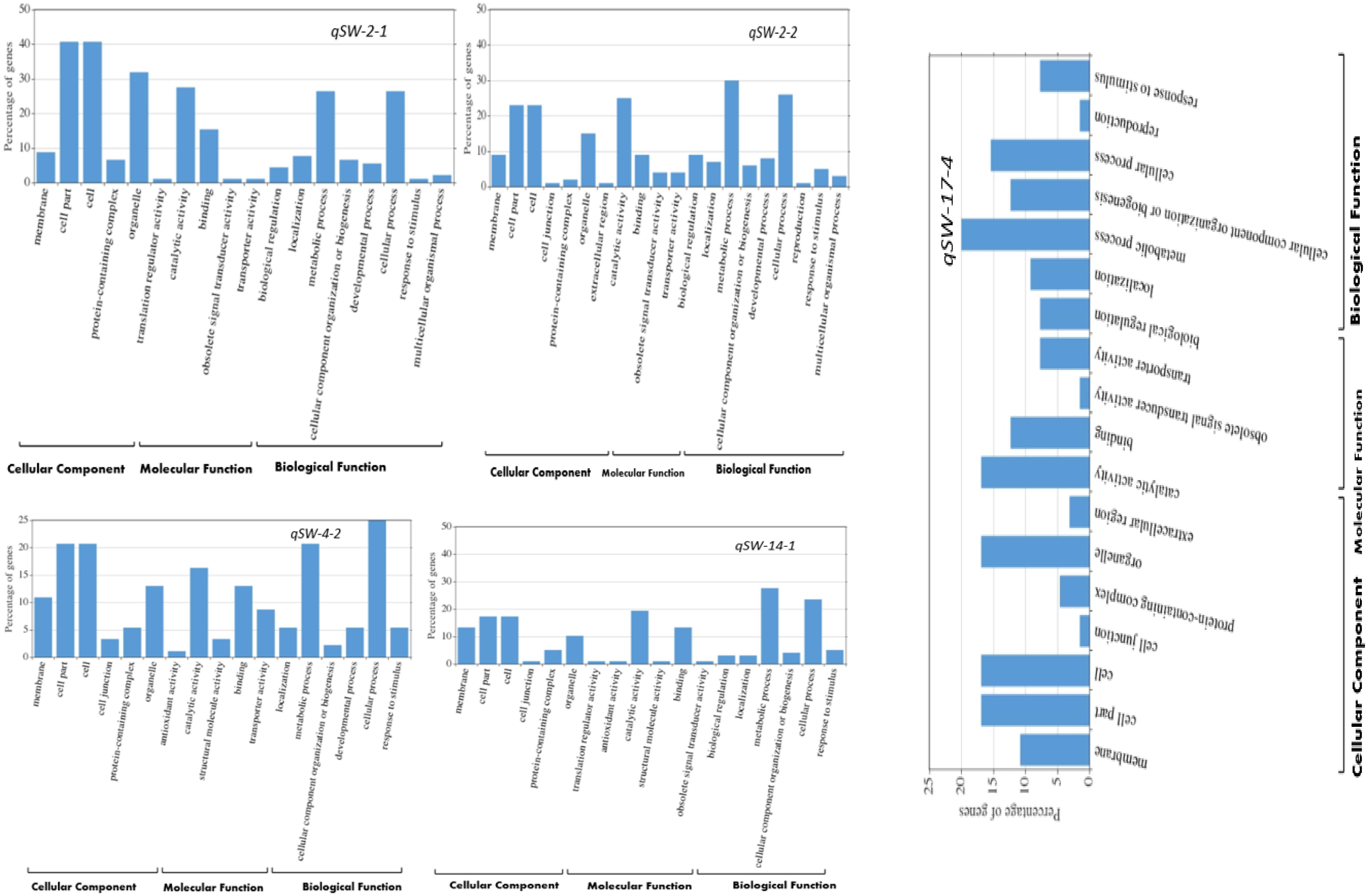
Figure 4. WeGO analysis of the genes located within the stable QTL regions viz., qSW-2-1, qSW-2-2, qSW-4-2, qSW-14-1, and qSW-17-4.
However, to identify the possible candidate genes underlying the above five stable QTLs responsible for 100-seed weight in soybean, we used PANTHER analysis, gene annotation information, and literature search. The PANTHER analysis is a comprehensive system that combines gene function, ontology, pathways, and statistical analysis tools, and enable biologists to analyze large-scale, genome-wide data from sequencing, proteomics, or gene expression experiments (Huaiyu et al., 2013). Based on the PANTHER analysis, gene annotation, as well as available literature, 29 genes out of total 490 model genes within the physical regions of the five stable QTLs were considered as possible candidate genes regulating seed-weight in soybean. Out of these 29 genes, 5 belong to ubiquitin-protein ligase (PC00234) class, 4 to carbohydrate transporter (PC00067), 3 are transporters (PC00227), 2 are involved in vesicle coat protein (PC00235), 1 in the SNARE protein (PC00034), and the remaining 18 belong to one or two other protein class (Table 3). Furthermore, Glyma02g13350, Glyma14g12220, and Glyma17g13000 genes had no protein class according to PANTHER analysis, and therefore were further analyzed using the gene expression data (RNA-seq) from phytozome database8, and their expression data revealed that these genes were highly expressed in the seed, and thus were also included as potential candidate genes. For instance, Glyma14g12220 has the domain of PP2C which is homolog to the PP2C that was demonstrated to enhance 100-seed weight by Lu et al. (2017).
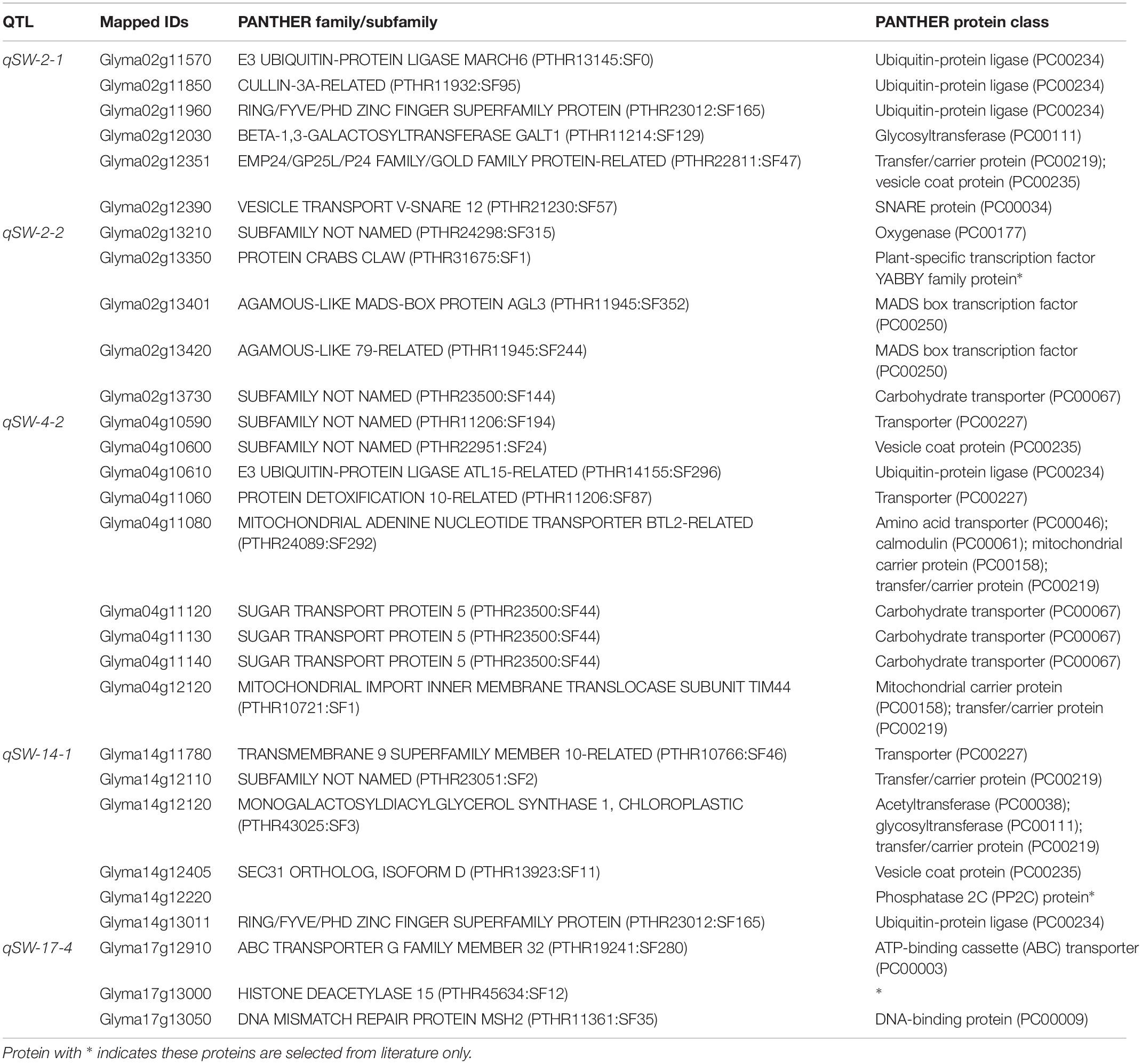
Table 3. Thirty-one possible candidate genes predicated within five stable QTL regions identified in this study based on PANTHER analysis, gene annotation, and available literature.
Discussion
Phenotypic Analysis of Seed-Weight
Seed-weight is an important economical trait controlling the yield in soybean. Therefore, developing soybean cultivars with improved seed-weight was the prime objective of soybean breeders. However, to develop the soybean cultivars with improved seed-weight, it is necessary to understand the genetic mechanisms as well as identify genetic elements associated with 100-seed weight. Seed-weight is a polygenic quantitative trait governed by multiple genes, and is highly environmentally sensitive trait. Although over the past decades many QTLs related to soybean seed-weight/size have been reported, and there are ∼325 QTLs documented for seed weight/size in the USDA Soybean Genome Database (SoyBase9). However, most of these QTLs were not stable as well as confirmed due to small sized mapping population and low-density genetic map, and hence have not been used for breeding improved seed-weight in soybean. Therefore, the aim of the present study was to utilize interspecific high-density linkage map of NJRI4P RIL population evaluated in four different environments to identify the stable QTLs as well as mine possible candidate genes for 100-seed weight in soybean. In the present study, ANOVA revealed that 100-seed weight was significantly affected by G, E, and G × E, similar as reported earlier by Fasoula et al. (2004). The RIL did not show clear transgressive segregation in any of environment, that might be due to unwanted linkages between beneficial and undesirable alleles contributed by exotic germplasm (Concibido et al., 2003; Wang et al., 2014). Furthermore, the cultivated and wild parents of RIL population showed clear and large difference in seed-weight/size confirming earlier reports that 100-seed is a domestication-related trait (Zhou L. et al., 2015; Zhou Z. et al., 2015). This wide difference between two parents of inter-specific RIL population for 100-seed weight has allowed detection of more number of QTLs including some novel QTLs. Maximum 100-seed weight of the RILs in each environment was more than three times higher than that of wild parent (PI 483460B), and also the RILs with minimum seed-weight were higher than PI 483460B indicating the usefulness of wild soybean in breeding program for specific seed size (Concibido et al., 2003; Hyten et al., 2006; Kim M.Y. et al., 2010; Lam et al., 2010; Yu et al., 2017; Kofsky et al., 2018). The higher H2-value observed for seed-weight in both the individual and CEs suggests that large proportion of trait variation is under genetic control, and these findings are similar as reported earlier by Kulkarni et al. (2016).
Genetic Control of Seed-Weight
As discussed above, many QTLs have been reported for seed-weight in soybean10. But majority of these previous studies used low-density genetic maps based on SSR or other low-throughput markers (Specht et al., 2001; Hyten et al., 2004; Panthee et al., 2005; Liu et al., 2011; Yao et al., 2015), which has low resolution with large confidence interval of QTLs not suitable for candidate gene detection (Hyten et al., 2010; Xu et al., 2013). The quality of genetic maps has great influence on the accuracy of QTL detection (Gutierrez-Gonzalez et al., 2011). In this context, high-density genetic map could identify more recombination events in a population, and will increase accuracy of QTL mapping (Xie et al., 2010). In the present study, we used high-density inter-specific bin map of NJIR4P RIL population consisting of 4,354 bin markers distributed to all 20 chromosomes of soybean with an average number of markers and distance per chromosome are 218 and 106.84 cM, respectively. The average distance between two markers was 0.49 cM (Wang et al., 2016). In addition, high-density genetic map assists in identifying tightly linked markers associated with QTLs, and provided a good foundation for analyzing quantitative traits. Moreover, the use of interspecific population would also enhance identification of genomic region(s) which was/were altered during domestication (Liu et al., 2018).
The QTLs associated with seed-weight in soybean has been mapped on all soybean linkage groups/chromosomes. In the present study, we identified a total of 19 QTLs associated with 100-seed weight using inter-specific genetic map of NJIR4P population, and these QTLs contributed significantly to the seed-weight. By comparing our QTL results with SoyBase database11, 12 QTLs have been previously reported in the same physical genomic region, and only 7 were novel identified for the first time (Table 2). The seven novel QTLs detected indicating the distinct genetic architecture of NJIR4P population, and suggest the need to use more germplasm for revealing the complex genetic basis of 100-seed weight in soybean. The physical interval of qSW-2-1, qSW-2-2, qSW-2-3, qSW-6-1, qSW-19-1, qSW-19-2, and qSW-19-3 did not overlap with any of the previously reported seed-weight QTLs, and hence were considered as novel QTLs. The qSW-1-1 was identified in the genetic interval (82.6–84.1 cM) that overlap with the seed-weight QTLs viz., Seed weight 15-2 and Seed weight 18.1-2 identified in the same genetic and physical position as reported earlier (Hyten et al., 2004; Panthee et al., 2005). Similarly, two QTLs identified on Chr4 viz., qSW-4-1 and qSW-4-2 overlapped with seed weight 47-1 corresponding to physical position of 96,434–51,252,852 bp (Li et al., 2010) and seed weight per plant 6-2 corresponding to physical position of 486,057–526,777 bp (Yao et al., 2015), respectively. The qSW-9-1 were detected in the same genomic physical interval as previously reported QTL, Seed weight 35-6 QTL (Han et al., 2012). Likewise, SW-11-1 was located in the genomic position of Seed weight 10-3 (Specht et al., 2001), Seed weight 32-1 (Li et al., 2008), and Seed weight 36-11 (Han et al., 2012). The qSW-14-1 could be the same QTL as Seed weight 36-14 (Han et al., 2012). Lu et al. (2017) identified QTL on Chr15 at the same physical interval (1,901,425–2,855,666 bp) as qSW-15-1 reported in the present study. The major and stable QTL viz., qSW-17-1 overlapped with earlier reported QTLs, Seed weight 21-1 (Gai et al., 2007), Seed weight 22-3 (Zhang et al., 2004), and Seed weight 47-2 (Li et al., 2010). Moreover, qSW-17-2 and qSW-17-3 overlapped with seed-weight QTLs previously reported by Li et al. (2010) and Wang et al. (2015), respectively. Another major and stable QTL identified on Chr17 viz., qSW-17-4 has been also reported by the number of earlier studies (Kim H.-K. et al., 2010; Kato et al., 2014; Wang et al., 2015; Zhou Z. et al., 2015; Liu et al., 2018). The seven novel QTLs identified for 100-seed weight together explained ∼46% of the phenotypic variation, which suggested potential importance of these loci for seed-weight. The QTLs identified in this study had narrow genetic and physical regions for the instance, qSW-17-4 which overlapped with Seed weight 47-2 (Li et al., 2010). In our study, qSW-17-4 was detected at genetic and physical positions of 37.7–42.3 cM and 9,420,885–10,095,969 bp, respectively, compared to Seed weight 47-2 (24.52–124.30 cM and 5,788,551–40,525,673 bp). In plant breeding, stability of QTL is essential for their use in MAB. Besides, two novel stable QTLs (qSW-2-1 and qSW-2-2) identified in the present study, the 12 QTLs for 100-seed weight have been previously co-localized in the same physical interval by earlier studies (see references in Table 2). Of the 12 QTLs previously reported, two are major QTLs with R2-value > 10% both located on Chr17 viz., qSW-17-1 and qSW-17-4 (see references in Table 2). Hence, these QTLs might also be considered as stable QTLs, and major stable QTLs can be used for further fine mapping and map-based cloning to unravel the mechanisms of seed-weight in soybean, as well as might be good for MAB. All the beneficial/positive alleles in the NJIR4P RIL population were derived from the cultivated soybean (Nannong 493-1), indicating that seed-weight was altered during domestication (Zhou L. et al., 2015; Zhou Z. et al., 2015; Lu et al., 2016). Similar to our findings, Lu et al. (2017) also reported that all the beneficial alleles for 100-seed weight were inherited from the cultivated soybean except one beneficial QTL allele viz., PP2C-1 that was derived from wild soybean parent. Although it has been revealed that wild soybean is a potential source for improving cultivated soybean in terms of yield-related traits, seed quality, and biotic and abiotic stress tolerance (Tuyen et al., 2010; Kim et al., 2011). In accordance with the earlier studies (Wang et al., 2016; Xin et al., 2016; Liu et al., 2018), our study also revealed that alleles derived from wild soybean contribute to a reduction in seed weight in all 19 seed-weight QTLs. It is not always the purpose of soybean breeders to increase seed weight/size, but also sometimes breeding program requires a suitable/optimized combination of yield-related parameters such as seed size, the number of seeds per pod, and the number of pods per plant. Hence, QTLs detected in our study would be valuable for controlling seed size via genomic breeding by design and positional cloning of the relevant genes. Furthermore, most of the QTLs detected in this study overlapped earlier reported QTLs indicating the accuracy of our mapping results. Moreover, those confirmed in this study with narrow regions could be integrated into breeding program via marker-assisted selection (MAS).
Candidate Gene Analysis for Seed-Weight
It is of great interest for both theoretical study and practical breeding program to identify the actual candidate gene underlying the QTL region. Most of the earlier QTL mapping on seed-weight did not mine for candidate genes (Zhang et al., 2004; Kato et al., 2014; Wu et al., 2018), and till date only two seed weight/size-related genes have been isolated from soybean viz., ln gene has a large effect on the number of seeds per pod and seed size (Jeong et al., 2012), and recently, the PP2C-1 (protein phosphatase type-2C) allele from wild soybean accession ZYD7 was found to contribute to the increase in seed size (Lu et al., 2017). Hence, based on the available information in current literature, gene annotation as well as bioinformatics tools, the present study identified the possible candidate genes regulating the 100-seed weight in soybean that underlie the stable QTLs. A total of 490 model genes were mined from the physical regions of the five stable QTLs viz., qSW-2-1, qSW-2-2, qSW-4-2, qSW-14-1, and qSW-17-4, and out of these 29 were considered as possible candidate genes based on the PANTHER analysis, gene function, and available literature (Huaiyu et al., 2013). Based on the WeGo analysis most of the genes underlying above five stable QTLs belong to the terms cell component, catalytic activity, binding, transporting, metabolic and cellular process, and these elements were reported to be vital in seed development (Fan et al., 2006; Mao et al., 2010; Li and Li, 2014). For example, Glyma02g13210 gene underlying QTL qSW-2-2 belongs to oxygenase (PC00177) protein class, that has been demonstrated to regulate seed size in soybean (Zhao et al., 2016). Similarly, protein family E3 ubiquitin-protein ligase (PC00234) are involved in the ubiquitin-proteasome pathway, and this protein family include members from various crop species such as DA1, DAR1, DA2, and EOD1/BB (Arabidopsis), GW2 (rice), TaGW2 (Wheat), ZmGW2 (maize), and UBP15/SOD2 (Arabidopsis), and all these genes have been reported to have significant effect on seed development (Li and Li, 2014, 2016; Ge et al., 2016). Thus, Glyma02g11570, Glyma02g11850, Glyma02g11960, Glyma04g10610, and Glyma14g13011 belonging to E3 ubiquitin-protein ligase (PC00234) were considered as possible candidate genes in the present study. Furthermore, Xian-Jun et al. (2007) reported a gene underlying QTL for rice grain width and weight (GW2) that encodes a previously unknown RING-type E3 ubiquitin ligase has been demonstrated by Xian-Jun et al. (2007). They demonstrated that loss of GW2 function increased cell numbers, resulting larger spikelet hull, and accelerated the grain milk filling rate, resulting in enhanced grain width, grain weight, and yield. It has been revealed that regulation of seed development is controlled by source (leaf) and sink (seed) relationship in plants (Schnyder, 1993), which is influenced by assimilate translocation/transportation. Therefore, genes viz., Glyma02g13730, Glyma04g10590, Glyma04g11060, Glyma04g11120, Glyma04g11130, Glyma04g11140, and Glyma14g11780 belonging to carbohydrate transporter (PC00067 or PC00227) gene family were might be possible candidate genes for seed-weight. Legume seed development is closely related to metabolism and nutrient (sucrose) transport (Borisjuk et al., 2003). Candidate genes Glyma02g12351, Glyma04g10590, Glyma04g10600, and Glyma14g12405 belong to vesicle coat protein (PC00235). This protein family have been reported to be involved in protein–protein interaction and transport (Harley and Beevers, 1989; Anantharaman and Aravind, 2002). Two candidate genes Glyma02g13401 and Glyma02g13420 were members of K and MAD box protein family, and this protein family has been reported to regulate flower development in plants (Bowman et al., 1991; Ditta et al., 2004). The flower as an organ acts as either source or sink and determine the seed number, which indirectly affect the seed size and shape (Stanton, 1984; Jia et al., 2016). The Glyma04g11080 belongs to several protein classes such as amino acid transporter (PC00046); calmodulin (PC00061); mitochondrial carrier protein (PC00158); transfer/carrier protein (PC00219) which could possibly be involved in seed weight regulation. For example, in rice, Asano et al. (2002) has shown that a calmodulin-like domain protein kinase is required for storage product accumulation during seed development. Moreover, Glyma02g12030, Glyma04g12120 and Glyma14g12120 belong to one or more protein classes: such as acyltransferase (PC00038), glycosyltransferase (PC00111), and transfer/carrier protein (PC00219), and these protein classes were demonstrated to play role in seed development (Rehman et al., 2016). The Glyma17g12910 gene underlying a major stable QTL, qSW-17-4, belongs to ATP-binding cassette (ABC) transporter (PC00003) which could possibly be involved in seed development (David et al., 2010). In addition, Glyma17g13000 belongs to histone deacetylase 15 (PTHR45634:SF12) that might be involved in regulating seed weight (Shahbazian and Grunstein, 2007; Peserico and Simone, 2011). As Yang et al. (2016) demonstrated maize histone deacetylase HDA101 function and regulatory mechanism during seed development. Also, Glyma17g13050 and Glyma17g13210 which code for DNA-binding protein (PC00009) and leucine-rich repeat-containing protein, respectively, play significant role in seed development (Li and Li, 2016; Li et al., 2019). Among the predicted candidate genes, the minimum number of exons and introns was two with the maximum gene sequence of 13,670 bps for Glyma17g13050 (Supplementary Figure 1). However, few of the 29 possible candidate genes predicted in this study for 100-seed weight have been included in our on-going projects for their functional validation. Lastly, the major and stable QTLs identified in the present study will be the main focus of soybean breeders for fine mapping and MAB of soybean cultivars with improved 100-seed weight.
Conclusion
In conclusion, the present study used high-density bin map of an interspecific RIL population (NJIR4P) evaluated in multiple environments to detect QTLs as well as mine possible candidate genes controlling 100-seed weight. A total of 19 QTLs were found associated with 100-seed weight, and out of which 7 were novel (reported for the first time). In addition, out of 19 QTLs, 8 were considered as stable QTLs identified in either more one individual environments or one individual environment plus CE, and two of them were major viz., qSW-17-1 and qSW-17-4 (R2 > 10%). Moreover, most of the previously reported QTLs validated in the present study had narrow physical genomic interval. All the beneficial/positive alleles of 19 QTLs were derived from the cultivated soybean (Nannong493-1). Twenty-nine possible candidate genes were mined within the five stable QTLs and most of them are belonging to ubiquitin-protein ligase (PC00234) that have been earlier reported to play significant role in seed/organ size development and regulation. However, it needs further validation to determine their actual role in seed weight and development, although few of them have been included in our on-going projects for functional validation. Hence, after proper functional validation of these candidate genes, these candidate genes can be used for improving 100-seed weight of soybean through transgenic or MAB. Lastly, our study provides detailed information for accurate QTL localization and candidate gene discovery, and these findings will be of great use for MAS of soybean varieties with improved seed-weight.
Data Availability
All datasets generated for this study are included in the manuscript and/or the Supplementary Files.
Author Contributions
TZ conceived and designed the experiments. BK, SC, YX, FC, YZ, and JK performed the experiments. BK and JB analyzed the data. BK and JB drafted the manuscript. TZ and JB revised the manuscript.
Funding
This work was supported by the National Key R&D Program of China (2016YFD0100201), the National Natural Science Foundation of China (Grant Nos. 31571691 and 31871646), the Fundamental Research Funds for the Central Universities (KYT201801), the MOE Program for Changjiang Scholars and Innovative Research Team in University (PCSIRT_17R55), the MOE 111 Project (B08025), and the Jiangsu Collaborative Innovation Center for Modern Crop Production (JCIC-MCP) Program.
Conflict of Interest Statement
The authors declare that the research was conducted in the absence of any commercial or financial relationships that could be construed as a potential conflict of interest.
Supplementary Material
The Supplementary Material for this article can be found online at: https://www.frontiersin.org/articles/10.3389/fpls.2019.01001/full#supplementary-material
Footnotes
- ^ http://www.soybase.org/
- ^ www.soybase.org
- ^ http://bioinfogp.cnb.csic.es/tools/venny_old/venny.php
- ^ http://geneontology.org/
- ^ http://wego.genomics.org.cn/
- ^ http://pantherdb.org/
- ^ www.soybase.org
- ^ https://phytozome.jgi.doe.gov/
- ^ http://www.soybase.org
- ^ www.soybase.org
- ^ www.soybase.org
References
Anantharaman, V., and Aravind, L. (2002). The GOLD domain, a novel protein module involved in Golgi function and secretion. Genome Biol. 3, 1–7. doi: 10.1186/gb-2002-3-5-research0023
Asano, T., Noriko, K., Yuhi, O., Hirokazu, I., Tsutomu, K., Makoto, T., et al. (2002). Rice SPK, a calmodulin-like domain protein kinase, is required for storage product accumulation during seed development: phosphorylation of sucrose synthase is a possible factor. Plant Cell 14, 619–628. doi: 10.1105/tpc.010454
Bates, D., Mächler, M., Bolker, B., and Walker, S. (2014). Fitting linear mixed-effects models using lme4. Stat. Comp. 1406, 133–199. doi: 10.1007/0-387-22747-4_4
Borisjuk, L., Rolletschek, H., Wobus, U., and Weber, H. (2003). Differentiation of legume cotyledons as related to metabolic gradients and assimilate transport into seeds. J. Exp. Bot. 54, 503–512. doi: 10.1093/jxb/erg051
Bowman, J. L., Smyth, D. R., and Meyerowitz, E. M. (1991). Genetic interactions among floral homeotic genes of Arabidopsis. Development 112, 1–20. doi: 10.1016/0165-3806(91)90160-K
Churchill, G. A., and Doerge, R. W. (1994). Empirical threshold values for quantitative trait mapping. Genetics 138, 963–971.
Concibido, V., Vallee, B. L., Mclaird, P., Pineda, N., Meyer, J., Hummel, L., et al. (2003). Introgression of a quantitative trait locus for yield from Glycine soja into commercial soybean cultivars. Theor. Appl. Genet. 106, 575–582. doi: 10.1007/s00122-002-1071
Coomes, P., and Grubb, D. (2003). Colonization, tolerance, competition and seed-size variation within functional groups. Trends Ecol. Evol. 18, 283–291. doi: 10.1016/S0169-5347(03)00072-7
Cui, Z., James, A., Miyazaki, S., Wilson, R. F., and Carter, T. E. Jr. (2004). “Breeding specialty soybeans for traditional and new soyfoods,” in Soybeans as Functional Foods and Ingredients, ed. K. Liu (Champaign, IL: AOSC Press), 274–332.
David, B., Fred, B., Alexandra, B., John, S., Stephen, G., Reinhard, J., et al. (2010). Characterization of Arabidopsis ABCG11/WBC11, an ATP binding cassette (ABC) transporter that is required for cuticular lipid secretion. Plant J. 52, 485–498. doi: 10.1111/j.1365-313X.2007.03252.x
Ditta, G., Pinyopich, A., Robles, P., Pelaz, S., and Yanofsky, M. F. (2004). The gene of arabidopsis thaliana functions in floral organ and meristem identity. Curr. Biol. 14, 1935–1940. doi: 10.1016/j.cub.2004.10.028
Fan, C., Xing, Y., Mao, H., Lu, T., Han, B., Xu, C., et al. (2006). GS3, a major QTL for grain length and weight and minor QTL for grain width and thickness in rice, encodes a putative transmembrane protein. Theor. Appl. Genet. 112, 1164–1171. doi: 10.1016/j.cub.2004.10.028
Fasoula, V. A., Harris, D. K., and Boerma, H. R. (2004). Validation and designation of quantitative trait loci for seed protein, Seed Oil, and seed weight from two soybean populations. Crop Sci. 44, 1218–1225. doi: 10.2135/cropsci2004.1218
Gai, J., Wang, Y., Wu, X., and Chen, S. (2007). A comparative study on segregation analysis and QTL mapping of quantitative traits in plants—with a case in soybean. Front. Agric. Chin. 1, 1–7. doi: 10.1007/s11703-007-0001-3
Ge, L., Yu, J., Wang, H., Luth, D., Bai, G., Wang, K., et al. (2016). Increasing seed size and quality by manipulating BIG SEEDS1 in legume species. Proc. Natl. Acad. Sci. U.S.A. 113, 12414–12419. doi: 10.1073/pnas.1611763113
Gomez, J. M. (2004). Bigger is not always better: conflicting selective pressures on seed size in Quercus ilex. Evolution 58, 71–80. doi: 10.1111/j.0014-3820.2004.tb01574.x
Guo, J., Wang, Y., Song, C., Zhou, J., Qiu, L., Huang, H., et al. (2010). A single origin and moderate bottleneck during domestication of soybean (Glycine max): implications from microsatellites and nucleotide sequences. Ann. Bot. 106, 505–514. doi: 10.1093/aob/mcq125
Gutierrez-Gonzalez, J. J., Vuong, T. D., Zhong, R., Yu, O., Lee, J.-D., Shannon, G., et al. (2011). Major locus and other novel additive and epistatic loci involved in modulation of isoflavone concentration in soybean seeds. Theor. Appl. Genet. 123, 1375–1385. doi: 10.1007/s00122-011-1673-x
Haig, D. (2013). Kin conflict in seed development: an interdependent but fractious collective. Annu. Rev. Cell Dev. Biol. 29, 189–211. doi: 10.1146/annurev-cellbio-101512-122324
Han, Y., Li, D., Zhu, D., Li, H., Li, X., Teng, W., et al. (2012). QTL analysis of soybean seed weight across multi-genetic backgrounds and environments. Theor. Appl. Genet. 125, 671–683. doi: 10.1007/s00122-012-1859-x
Han, Y., Zhao, X., Liu, D., Li, Y., Lightfoot, D. A., Yang, Z., et al. (2016). Domestication footprints anchor genomic regions of agronomic importance in soybeans. New Phytol. 209, 871–884. doi: 10.1111/nph.13626
Hanson, C. H., Robinson, H. F., and Comstock, R. E. (1956). Biometrical studies of yield in segregating populations of korean lespedeza. Agron. J. 48:10. doi: 10.2134/agronj1956.00021962004800060008x
Harley, S. M., and Beevers, L. (1989). Coated vesicles are involved in the transport of storage proteins during seed development in Pisum sativum L. Plant Phys. 91, 674–678. doi: 10.1104/pp.91.2.674
Hu, B., Jin, J., Guo, A. Y., Zhang, H., Luo, J., and Gao, G. (2014). GSDS 2.0: an upgraded gene feature visualization server. Bioinformatics 31, 1296–1297. doi: 10.1093/bioinformatics/btu817
Huaiyu, M., Anushya, M., Casagrande, J. T., and Thomas, P. D. (2013). Large-scale gene function analysis with the PANTHER classification system. Nat. Prot. 8, 1551–1566. doi: 10.1038/nprot.2013.092
Hyten, D. L., Choi, I. Y., Song, Q. J., Specht, J. E., Carter, T. E. Jr., Shoemaker, R. C., et al. (2010). A high density integrated genetic linkage map of soybean and the development of a 1536 universal soy linkage panel for quantitative trait locus mapping. Crop Sci. 50, 960–968. doi: 10.2135/cropsci2009.06.0360
Hyten, D. L., Pantalone, V. R., Sams, C. E., Saxton, A. M., Landau-Ellis, D., Stefaniak, T. R., et al. (2004). Seed quality QTL in a prominent soybean population. Theor. Appl. Genet. 109, 552–561. doi: 10.1007/s00122-004-1661-5
Hyten, D. L., Qijian, S., Youlin, Z., Ik-Young, C., Nelson, R. L., Costa, J. M., et al. (2006). Impacts of genetic bottlenecks on soybean genome diversity. Proc. Natl. Acad. Sci. U.S.A. 103, 16666–16671. doi: 10.1073/pnas.0604379103
Jeong, N., Suh, S. J., Kim, M.-H., Lee, S., Moon, J.-K., Kim, H. S., et al. (2012). Ln is a key regulator of leaflet shape and number of seeds per pod in soybean. Plant Cell 24, 4807–4818. doi: 10.1105/tpc.112.104968
Jia, N., Liu, X., and Gao, H. (2016). A DNA2 homolog is required for DNA damage repair, cell cycle regulation, and meristem maintenance in plants. Plant Phys. 171, 318–333. doi: 10.1104/pp.16.00312
Kato, S., Sayama, T., Fujii, K., Yumoto, S., Kono, Y., Hwang, T.-Y., et al. (2014). A major and stable QTL associated with seed weight in soybean across multiple environments and genetic backgrounds. Theor. Appl. Genet. 127, 1365–1374. doi: 10.1007/s00122-014-2304-0
Kim, H.-K., Kim, Y.-C., Kim, S.-T., Son, B.-G., Choi, Y.-W., Kang, J.-S., et al. (2010). Analysis of quantitative trait loci (QTLs) for seed size and fatty acid composition using recombinant inbred lines in soybean. J. Life Sci. 20, 1186–1192.
Kim, M. Y., Sunghoon, L., Kyujung, V., Tae-Hyung, K., Soon-Chun, J., Ik-Young, C., et al. (2010). Whole-genome sequencing and intensive analysis of the undomesticated soybean (Glycine soja Sieb. and Zucc.) genome. Proc. Natl. Acad. Sci. U.S.A. 107, 22032–22037. doi: 10.1073/pnas.1009526107
Kim, M., Hyten, D. L., Niblack, T. L., and Diers, B. W. (2011). Stacking resistance alleles from wild and domestic soybean sources improves soybean cyst nematode resistance. Crop Sci. 51, 934–943. doi: 10.1073/pnas.1009526107
Kofsky, J., Zhang, H., and Song, B. H. (2018). The untapped genetic reservoir: the past, current, and future applications of the wild soybean (Glycine soja). Front. Plant Sci. 9:949. doi: 10.3389/fpls.2018.00949
Kulkarni, K. P., Kim, M., Shannon, J. G., and Lee, J. D. (2016). Identification of quantitative trait loci controlling soybean seed weight in recombinant inbred lines derived from PI 483463 (Glycine soja)בHutcheson’(G. max). Plant Breed. 135, 614–620. doi: 10.1111/pbr.12407
Lam, H.-M., Xun, X., Xin, L., Wenbin, C., Guohua, Y., Fuk-Ling, W., et al. (2010). Resequencing of 31 wild and cultivated soybean genomes identifies patterns of genetic diversity and selection. Nat. Genet. 42, 1053–1059. doi: 10.1038/ng.715
Lee, G.-A., Crawford, G. W., Li, L., Yuka, S., and Xuexiang, C. (2011). Archaeological soybean (Glycine max) in east asia: does size matter? PLoS One 6:e26720. doi: 10.1371/journal.pone.0026720
Li, D., Sun, M., Han, Y., Teng, W., and Li, W. (2010). Identification of QTL underlying soluble pigment content in soybean stems related to resistance to soybean white mold (Sclerotinia sclerotiorum). Euphytica 172, 49–57. doi: 10.1007/s10681-009-0036-z
Li, N., and Li, Y. (2014). Ubiquitin-mediated control of seed size in plants. Front. Plant Sci. 5:332. doi: 10.3389/fpls.2014.00332
Li, N., and Li, Y. (2016). Signaling pathways of seed size control in plants. Curr. Opin. Plant Biol. 33, 23–32. doi: 10.1016/j.pbi.2016.05.008
Li, N., Xu, R., and Li, Y. (2019). Molecular networks of seed size control in plants. Ann. Rev. Plant Biol. 70, 1–30. doi: 10.1146/annure-arplant-050718-095851
Li, W., Zheng, D.-H., Van, K., and Lee, S.-H. (2008). QTL mapping for major agronomic traits across two years in soybean (Glycine max L. Merr.). J. Crop Sci. Biotechnol. 11, 171–190. doi: 10.1270/jsbbs.66.191
Liang, H., Lanjie, X. U., Yongliang, Y. U., Yang, H., Wei, D., and Zhang, H. (2016). Identification of QTLs with main, epistatic and QTL by environment interaction effects for seed shape and hundred-seed weight in soybean across multiple years. J. Genet. 95, 475–477. doi: 10.1007/s12041-016-0648-8
Lihua, C. Y. D. (1982). The principle of high-yielding soybean and its culture technique. Acta Agron. Sin. 1:006.
Liu, D., Yan, Y., Fujita, Y., and Xu, D. (2018). Identification and validation of QTLs for 100-seed weight using chromosome segment substitution lines in soybean. Breed. Sci. 68, 442–448. doi: 10.1270/jsbbs.17127
Liu, W., Kim, M. Y., Van, K., Lee, Y.-H., Li, H., Liu, X., et al. (2011). QTL identification of yield-related traits and their association with flowering and maturity in soybean. J. Crop Sci. Biotechnol. 14, 65–70. doi: 10.1007/s12892-010-0115-7
Liu, X., Jin, J., Wang, G., and Herbert, S. (2008). Soybean yield physiology and development of high-yielding practices in Northeast China. Field Crops Res. 105, 157–171. doi: 10.1016/j.fcr.2007.09.003
Lu, X., Li, Q. T., Xiong, Q., Li, W., Bi, Y. D., Lai, Y. C., et al. (2016). The transcriptomic signature of developing soybean seeds reveals the genetic basis of seed trait adaptation during domestication. Plant J. 86, 530–544. doi: 10.1111/tpj.1318
Lu, X., Xiong, Q., Cheng, T., Li, Q.-T., Liu, X.-L., Bi, Y.-D., et al. (2017). A PP2C-1 allele underlying a quantitative trait locus enhances soybean l O0-Seed weight. Mol. Plant 10, 670–684. doi: 10.1016/j.molp.2017.03.006
Mao, H., Sun, S., Yao, J., Wang, C., Yu, S., Xu, C., et al. (2010). Linking differential domain functions of the GS3 protein to natural variation of grain size in rice. Proc. Natl. Acad. Sci. U.S.A. 107, 19579–19584. doi: 10.1073/pnas.1014419107
McCouch, S. R., Chen, X., Panaud, O., Temnykh, S., Xu, Y., Yong, G. C., et al. (1997). Microsatellite marker development, mapping and applications in rice genetics and breeding. Plant Mol. Biol. 35, 89–99. doi: 10.1023/a:1005711431474
Oliveros, J. (2007). An Interactive Tool for Comparing Lists with Venn’s Diagrams. Available at: http://bioinfogp.cnb.csic.es/tools/venny_old/venny.php (accessed December 20, 2018).
Palomeque, L., Li-Jun, L., Li, W., Hedges, B., Cober, E. R., and Rajcan, I. (2009). QTL in mega-environments: II. Agronomic trait QTL co-localized with seed yield QTL detected in a population derived from a cross of high-yielding adapted×high-yielding exotic soybean lines. Theor. Appl. Genet. 119, 429–436. doi: 10.1007/s00122-009-1048-8
Palomeque, L., Liu, L.-J., Li, W., Hedges, B. R., Cober, E. R., Smid, M. P., et al. (2010). Validation of mega-environment universal and specific QTL associated with seed yield and agronomic traits in soybeans. Theor. Appl. Genet. 120, 997–1003. doi: 10.1007/s00122-009-1227-7
Panthee, D. R., Pantalone, V. R., West, D. R., Saxton, A. M., and Sams, C. E. (2005). Quantitative trait loci for seed protein and oil concentration, and seed size in soybean. Crop Sci. 45, 2015–2022. doi: 10.2135/cropsci2004.0720
Paterson, A. H. (2010). Tansley review No. 137. what has QTL mapping taught us about plant domestication? New Phytol. 154, 591–608. doi: 10.1046/j.1469-8137.2002.00420.x
Peserico, A., and Simone, C. (2011). Physical and functional HAT/HDAC interplay regulates protein acetylation balance. J. Biomed. Biotechnol. 2011:371832. doi: 10.1155/2011/371832
Qi, Z., Xiaoying, Z., Huidong, Q., Dawei, X., Xue, H., Hongwei, J., et al. (2017). Identification and validation of major QTLs and epistatic interactions for seed oil content in soybeans under multiple environments based on a high-density map. Euphytica 213:162.
Qiu, L.-J., Xing, L.-L., Guo, Y., Wang, J., and Chang, R.-Z. (2013). A platform for soybean molecular breeding: the utilization of core;collections for food security. Plant Mol. Biol. 83, 41–50. doi: 10.1007/s11103-013-0076-6
Rehman, H. M., Nawaz, M. A., Le, B., Shah, Z. H., Krishnamurthy, P., Ahmad, M. Q., et al. (2016). Genome-wide analysis of Family-1 UDP-glycosyltransferases in soybean confirms their abundance and varied expression during seed development. J. Plant Phys. 206, 87–97. doi: 10.1016/j.jplph.2016.08.017
Schnyder, H. (1993). The role of carbohydrate storage and redistribution in the source-sink relations of wheat and barley during grain filling-a review. New Phytol. 123, 233–245. doi: 10.1111/j.1469-8137.1993.tb03731.x
Shahbazian, M. D., and Grunstein, M. (2007). Functions of site-specific histone acetylation and deacetylation. Annu. Rev. Biochem. 76, 75–100. doi: 10.1146/annurev.biochem.76.052705.162114
Singh, R. (1985). Biometrical Methods in Quantitative Genetic Analysis, Revised Edn. Ludhiana: Kalyani Publishers, 318.
Specht, J. E., Chase, K., Macrander, M., Graef, G. L., Chung, J., Markwell, J. P., et al. (2001). Soybean response to water. Crop Sci. 41, 493–509. doi: 10.2135/cropsci2001.412493x
Stanton, M. L. (1984). Developmental and genetic sources of seed weight variation in Raphanus raphanistrum L. (Brassicaceae). Am. J. Bot. 71, 1090–1098. doi: 10.2307/2443384
Stupar, R. M. (2010). Into the wild: the soybean genome meets its undomesticated relative. Proc. Natl. Acad. Sci. U.S.A. 107, 21947–21948. doi: 10.1073/pnas.1016809108
Sun, Y. N., Pan, J. B., Shi, X. L., Du, X. Y., Wu, Q., Qi, Z. M., et al. (2012). Multi-environment mapping and meta-analysis of 100-seed weight in soybean. Mol. Biol. Rep. 39, 9435–9443. doi: 10.1007/s11033-012-1808-4
Tang, H. B., Sezen, U., and Paterson, A. H. (2010). Domestication and plant genomes. Curr. Opin. Plant Biol. 13, 160–166. doi: 10.1016/j.pbi.2009.10.008
Teng, W., Feng, L., Li, W., Wu, D., Zhao, X., Han, Y., et al. (2017). Dissection of the genetic architecture for soybean seed weight across multiple environments. Crop Pasture Sci. 68, 358–365. doi: 10.1071/CP16462
Teng, W., Han, Y., Du, Y., Sun, D., Zhang, Z., Qiu, L., et al. (2009). QTL analyses of seed weight during the development of soybean (Glycine max L. Merr.). Heredity 102, 372–380. doi: 10.1038/hdy.2008.108
Tuyen, D. D., Lal, S. K., and Xu, D. H. (2010). Identification of a major QTL allele from wild soybean (Glycine soja Sieb. & Zucc.) for increasing alkaline salt tolerance in soybean. Theor. Appl. Genet. 121, 229–236. doi: 10.1007/s00122-010-1304-y
Wang, J., Chen, P. Y., Wang, D. C., Shannon, G., Shi, A. N., Zeng, A. L., et al. (2015). Identification of quantitative trait loci for oil content in soybean seed. Crop Sci. 55, 23–34. doi: 10.2135/cropsci2014.04.0280
Wang, S., Basten, C., and Zeng, Z. (2007). Windows QTL Cartographer 2.5. Raleigh, NC: Department of Statistics, North Carolina State University.
Wang, W., He, Q., Yang, H., Xiang, S., Xing, G., Zhao, T., et al. (2014). Identification of QTL/segments related to seed-quality traits in G. Soja using chromosome segment substitution lines. Plant Genet. Res. 12, S65–S69. doi: 10.1017/S147926211400029X
Wang, W., Liu, M., Wang, Y., Li, X., Cheng, S., Shu, L., et al. (2016). Characterizing two inter-specific bin maps for the exploration of the QTLs/Genes that confer three soybean evolutionary traits. Front. Plant Sci. 7:1248. doi: 10.3389/fpls.2016.01248
Wu, D., Zhan, Y., Sun, Q., Xu, L., Lian, M., Zhao, X., et al. (2018). Identification of quantitative trait loci underlying soybean (Glycine max [L.] Merr.) seed weight including main, epistatic and QTL × environment effects in different regions of Northeast China. Plant Breed. 137, 194–202. doi: 10.1111/pbr.12574
Xian-Jun, S., Wei, H., Min, S., Mei-Zhen, Z., and Hong-Xuan, L. (2007). A QTL for rice grain width and weight encodes a previously unknown RING-type E3 ubiquitin ligase. Nat. Genet. 39, 623–630. doi: 10.1038/ng2014
Xie, F. T., Niu, Y., Zhang, J., Bu, S. H., Zhang, H. Z., Geng, Q. C., et al. (2014). Fine mapping of quantitative trait loci for seed size traits in soybean. Mol. Breed. 34, 2165–2178. doi: 10.1007/s11032-014-0171-7
Xie, W., Qi, F., Huihui, Y., Xuehui, H., Qiang, Z., Yongzhong, X., et al. (2010). Parent-independent genotyping for constructing an ultrahigh-density linkage map based on population sequencing. Proc. Natl. Acad. Sci. U.S.A. 107, 10578–10583. doi: 10.1073/pnas.1005931107
Xin, D., Qi, Z., Jiang, H., Hu, Z., Zhu, R., Hu, J., et al. (2016). QTL location and epistatic effect analysis of 100-seed weight using wild soybean (Glycine soja Sieb. & Zucc.) chromosome segment substitution lines. PLoS One 11:e0149380. doi: 10.1371/journal.pone.0149380
Xu, X., Zeng, L., Tao, Y., Vuong, T., Wan, J., Boerma, R., et al. (2013). Pinpointing genes underlying the quantitative trait loci for root-knot nematode resistance in palaeopolyploid soybean by whole genome resequencing. Proc. Natl. Acad. Sci. U.S.A. 110, 13469–13474. doi: 10.1073/pnas.1222368110
Yan, L., Xing, L. L., Yang, C. Y., Chang, R. Z., Zhang, M. C., and Qiu, L. J. (2014). Identification of quantitative trait loci associated with soybean seed protein content using two populations derived from crosses between Glycine max and Glycine soja. Plant Genet. Res. 12, S104–S108.
Yang, H., Liu, X., Xin, M., Du, J., Hu, Z., Peng, H., et al. (2016). Genome-wide mapping of targets of maize histone deacetylase HDA101 reveals its function and regulatory mechanism during seed development. Plant Cell 28, 629–645. doi: 10.1105/tpc.15.00691
Yao, D., Liu, Z. Z., Zhang, J., Liu, S. Y., Qu, J., Guan, S. Y., et al. (2015). Analysis of quantitative trait loci for main plant traits in soybean. Genet. Mol. Res. 14, 6101–6109. doi: 10.4238/2015.June.8.8
Ye, J., Zhang, Y., Cui, H., Liu, J., Wu, Y., Cheng, Y., et al. (2018). WEGO 2.0: a web tool for analyzing and plotting GO annotations, 2018 update. Nucleic Acids Res. 46, W71–W75. doi: 10.1093/nar/gky400
Yu, C., Qu, Z., Zhang, Y., Zhang, X., Lan, T., Adelson, D. L., et al. (2017). Seed weight differences between wild and domesticated soybeans are associated with specific changes in gene expression. Plant Cell Rep. 36, 1417–1426. doi: 10.1007/s00299-017-2165-5
Zhang, H., Song, Q., Griffin, J. D., and Song, B. H. (2017). Genetic architecture of wild soybean (Glycine soja) response to soybean cyst nematode (Heterodera glycines). Mol. Genet.Genomics 292, 1–9. doi: 10.1007/s00438-017-1345-x
Zhang, W. K., Wang, Y. J., Luo, G. Z., Zhang, J. S., He, C. Y., Wu, X. L., et al. (2004). QTL mapping of ten agronomic traits on the soybean (Glycine max L. Merr.) genetic map and their association with EST markers. Theor. Appl. Genet. 108, 1131–1139.
Zhang, Y., He, J., Wang, Y., Xing, G., Zhao, J., Li, Y., et al. (2015). >. Establishment of a 100-seed weight quantitative trait locus-allele matrix of the germplasm population for optimal recombination design in soybean breeding programmes. J. Exp. Bot. 66, 6311–6325
Zhao, B., Dai, A., Wei, H., Yang, S., Wang, B., Jiang, N., et al. (2016). Arabidopsis KLU homologue GmCYP78A72 regulates seed size in soybean. Plant Mol. Biol. 90, 33–47. doi: 10.1007/s11103-015-0392-0
Zhou, L., Wang, S. B., Jian, J., Geng, Q. C., Wen, J., Song, Q., et al. (2015). Identification of domestication-related loci associated with flowering time and seed size in soybean with the RAD-seq genotyping method. Sci. Rep. 5:9350. doi: 10.1038/srep09350
Keywords: soybean, seed-weight, QTL, candidate gene, marker-assisted breeding
Citation: Karikari B, Chen S, Xiao Y, Chang F, Zhou Y, Kong J, Bhat JA and Zhao T (2019) Utilization of Interspecific High-Density Genetic Map of RIL Population for the QTL Detection and Candidate Gene Mining for 100-Seed Weight in Soybean. Front. Plant Sci. 10:1001. doi: 10.3389/fpls.2019.01001
Received: 08 May 2019; Accepted: 17 July 2019;
Published: 04 September 2019.
Edited by:
Sergio J. Ochatt, INRA, UMR1347 Agroécologie, FranceReviewed by:
Juan Jose Ferreira, Servicio Regional de Investigación y Desarrollo Agroalimentario (SERIDA), SpainZhaoming Qi, Northeast Agricultural University, China
Copyright © 2019 Karikari, Chen, Xiao, Chang, Zhou, Kong, Bhat and Zhao. This is an open-access article distributed under the terms of the Creative Commons Attribution License (CC BY). The use, distribution or reproduction in other forums is permitted, provided the original author(s) and the copyright owner(s) are credited and that the original publication in this journal is cited, in accordance with accepted academic practice. No use, distribution or reproduction is permitted which does not comply with these terms.
*Correspondence: Javaid Akhter Bhat, javid.akhter69@gmail.com; Tuanjie Zhao, tjzhao@njau.edu.cn