- 1Texas A&M AgriLife Research and Extension Center, Texas A&M University, Weslaco, TX, United States
- 2Departamento de Fisiología, Biología Molecular y Celular, Facultad de Ciencias Exactas y Naturales, Universidad de Buenos Aires, Buenos Aires, Argentina
- 3CONICET-Universidad de Buenos Aires, Instituto de Fisiología, Biología Molecular y Neurociencias (IFIBYNE), Buenos Aires, Argentina
- 4Department of Plant Pathology and Microbiology, Texas A&M University, College Station, TX, United States
Alternative splicing (AS) is a crucial posttranscriptional mechanism of gene expression which promotes transcriptome and proteome diversity. At the molecular level, splicing and AS involves recognition and elimination of intronic regions of a precursor messenger RNA (pre-mRNA) and joining of exonic regions to generate the mature mRNA. AS generates more than one mRNA transcript (transcripts) differing in coding and/or untranslated regions (UTRs). AS can be classified into four major types including the exon skipping (ES), intron retention (IR), alternative donor (AD), and alternative acceptor (AA), of which IR is the most prevalent event in plants (Mandadi and Scholthof, 2015). In addition to these AS types, a subfamily of IR called exitrons, which has dual features of introns and protein-coding exons were first reported in Arabidopsis thaliana (Arabidopsis) and later also found in humans (Marquez et al., 2015). These spliced transcripts influence multiple biological processes such as growth, development and response to biotic and abiotic stresses in plants (Filichkin et al., 2015; Mandadi and Scholthof, 2015; Wang et al., 2018a).
Functional Relevance of AS
AS can produce aberrant or unstable transcripts with premature termination codons (PTCs). The PTC-containing transcripts are often targeted to degradation by a conserved cytoplasmic RNA degradation mechanism called NMD (non-sense-mediated mRNA decay). NMD mechanisms ensure that there is a balance or homeostasis in the functional vs. non-functional transcripts (Kalyna et al., 2012). In contrast to mammals, where NMD targets are degraded by a suppressor with morphogenetic effect on genitalia (SMG7) endonucleolytic pathway, plants NMD primarily occurs via. SMG7 exonucleolytic pathway (Shaul, 2015). AS can also produce stable transcripts, which encode proteins with altered functional domains, subcellular localization, and/or biological functions (Reddy et al., 2013; Shang et al., 2017). In humans, ~15% of genetic diseases are a result of aberrant splicing (Staiger and Brown, 2013). In plants, several studies have showed that AS has biologically significant implications in growth, development, stress-responses, and/or adaptation. For instance, AS in a MADS-box transcription factor gene, SHORT VEGETATIVE PHASE (SVP), results in multiple transcripts (SVP1 and SVP3), which encode proteins with altered interaction domains (Severing et al., 2012). Overexpression of SVP1, but not SVP3, resulted in repression of flowering (Severing et al., 2012). In rice, AS occurs in the DEHYDRATION-RESPONSIVE ELEMENT BINDING PROTE IN 2 (DREB2B) gene, but only when subjected to drought and heat stress, and results in the production of an alternative transcript which encodes the full-length functional protein that confers tolerance to the stresses (Matsukura et al., 2010). Similarly, in tobacco, the classical resistance gene (N) against Tobacco mosaic virus (TMV) is alternatively spliced, resulting in production of two forms—a short and a long transcript (Dinesh-Kumar and Baker, 2000). Functional analysis revealed that both transcripts are required in certain ratio to confer full resistance to TMV (Dinesh-Kumar and Baker, 2000). Recently, by employing high-throughput sequencing (HTS), Mandadi and Scholthof (2015), identified ~670 intron-containing genes in Brachypodium that were aberrantly spliced in response to viral infection. Several of these genes encoded resistance proteins, transcription factors, and splicing factors (Mandadi and Scholthof, 2015). Together, these studies suggest that many AS events, if not all, have biologically-significant implications in plant growth, development and response to stresses.
High-Throughput Sequencing (HTS) for AS Analysis
Historically, our knowledge of plant alternative splicing and how it affects biological processes was primarily gleaned from studies of few plant species (e.g., Arabidopsis, rice; Modrek and Lee, 2002). However, with the rapid developments in HTS (a.k.a. next- and third-generation sequencing) technologies, particularly long-read, single-molecule real-time sequencing (SMRT) and direct RNA-sequencing platforms, the field is rapidly changing. Several existing and emerging next- generation sequencing (NGS) platforms, and bioinformatics tools are useful for genome-wide queries of AS in diverse plant species (Filichkin et al., 2010; Mandadi and Scholthof, 2015; Thatcher et al., 2016). HTS-based genome analysis studies estimated that ~33–70% of plant genes undergo AS, suggesting a broader influence of AS in shaping the functional transcriptome and proteome landscapes of plants (Pan et al., 2008; Chamala et al., 2015; Filichkin et al., 2015; Mandadi and Scholthof, 2015; Wang et al., 2018a). The seemingly lower number of genes undergoing AS in plants when compared to humans (~95%) could be due to lack of enough studies or in-depth annotations of the plant genomes. In early reports dating back to 2004, the AS rates in the model plant Arabidopsis was reported at a meager ~11.6%, when the AS rates in humans was ~42% (Iida et al., 2004). Efforts by several groups over the years, and with the advent of HTS technologies, the AS rates in Arabidopsis and humans ascended comparably to ~60 and ~95%, respectively (Wang and Brendel, 2006; Zhang et al., 2015; Laloum et al., 2018). Hence, we presume that with recent advances in HTS technologies, the AS frequencies in plant species would likely increase further. Alternatively, it is quite possible that differential gene structure/number, spliceosome composition, as well as variations in the types of tissues sampled, and detection methods could contribute to the observed lower AS rate in plants when compared to humans.
HTS-based short read (Illumina) and long read (Pacific Biosciences and Oxford Nanopore) sequencing technologies have revolutionized the field of DNA and RNA sequencing. Specifically, short read (<300 bp) RNA-sequencing (ShR RNA-seq), which integrates qualitative (gene discovery) and quantitative (gene quantification) assays, became a popular tool for genome-wide AS identification in plants as well as in other organisms. Because ShR RNA-seq provides high sequencing depth, a low error rate (<1%) and relatively-lower cost, it has been extensively used to characterize and quantify spliced transcripts in well-annotated plant genomes such as Arabidopsis (Calixto et al., 2018), Oryza sativa (Zhang and Xiao, 2018), Brachypodium distachyon (Mandadi and Scholthof, 2015), Zea mays (Thatcher et al., 2016), and Glycine max (Shen et al., 2014). Further, discovery of AS in plants was improved by the continuous development of open-source bioinformatics tools and pipelines. Identifying spliced transcripts from ShR-RNA-seq involves, mapping of high quality reads to reference genomes (HISAT2, TopHat2), transcript assembly (StringTie, Cufflinks, Trinity), AS events analysis (ASTALAVISTA), and transcript quantification (Cuffdiff, DESeq2) (Figure 1; Haas et al., 2013; Trapnell et al., 2013; Love et al., 2014; Foissac and Sammeth, 2015; Pertea et al., 2016; Irigoyen et al., 2018). Among these tools, HISAT2 and StringTie analysis pipeline (new Tuxedo package; Pertea et al., 2016) perform much faster, requires less memory and generates more accurate results over the TopHat2 and Cufflinks analysis pipeline (original Tuxedo package; Trapnell et al., 2012).
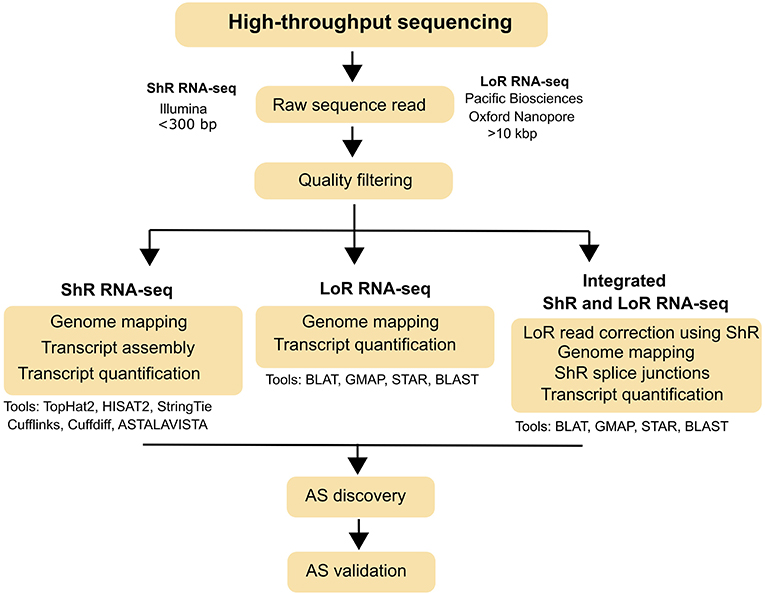
Figure 1. Typical workflow for AS identification from short read (ShR) and long read (LoR) RNA-seq. AS identification strategies using ShR and LoR RNA-seq and the various bioinformatics tools that can be used for each strategy are also indicated.
Despite the advances in ShR RNA-seq for AS discovery, this technology has limited scope in polyploids plant species (e.g., sugarcane, cotton) without reference genomes or those lacking comprehensive transcript-level annotations. To overcome this limitation, long read RNA-sequencing (LoR RNA-seq) technologies such as Pacific Biosciences (SMRT sequencing) and Oxford Nanopore (MinION) which has the ability to sequence full-length transcripts and direct RNA sequencing, have been used to study complex AS landscapes in polyploids (Liu et al., 2017; Wang et al., 2018a). LoR RNA-seq using SMRT (Iso-Seq method) and MinION can generate exceptionally long reads (>10 Kbp), which could cover most of the full-length eukaryotic transcripts and thus eliminate the need for transcript assembly (Liu et al., 2017; Ardui et al., 2018). Bioinformatics steps to identify AS from LoR RNA-seq involves mapping of high quality reads to reference genome (GMAP, BLAT) and, identifications of alternative transcripts and AS events from the alignments (Figure 1; Kent, 2002; Wu and Watanabe, 2005). For species where a reference genome is not available, the self-BLAST based pipeline on LoR RNA-seq can be used to detect AS based on the INDELs (Figure 1; Liu et al., 2017). Even though LoR RNA-seq is exceptional in resolving transcript structures, it has some limitations when compared to ShR RNA-seq including lower sequencing depth, high error-rate (up to ~15%), and being poorly suited for transcript quantifications (Ardui et al., 2018; Clark et al., 2018). These limitations in LoR RNA-seq could be mitigated by employing an integrated strategy involving both LoR and ShR RNA-seq reads (Figure 1; Koren et al., 2012). However, error correction methods are highly dependent on the availability of reference genomes and transcript annotations (Liu et al., 2017).
Validation of AS
Before embarking on functional analyses, it is recommended to validate the AS events using conventional molecular biology techniques. Reverse transcription followed by polymerase chain reaction (RT-PCR), cloning and Sanger-based sequencing are widely used approaches to confirm the presence and the sequence of the alternatively spliced transcripts (Simpson et al., 2008, 2016; Mandadi and Scholthof, 2015). Semi-quantitative RT-PCR (SqRT-PCR) and quantitative RT-PCR (qRT-PCR) can be used to quantify the alternatively spliced transcripts to understand AS regulation in different conditions (Harvey and Cheng, 2016). Both methods require cDNA synthesis as a first step, using either oligo-dT, which amplifies several mRNAs from the same sample, or specific oligos to generate cDNAs from specific transcripts. SqRT-PCR estimates relative amounts of the different templates in a sample and can be used to compare changes across different conditions/treatments. Additionally, to quantify the relative expression of the transcripts, quantitative PCR (qPCR) could be performed. In qPCR-based assays, transcript-specific primers are designed to quantify the expression levels. Subsequent to a PCR-based analysis, the various transcripts can be resolved in an agarose gel and purified for further cloning and Sanger-based sequencing to validate the sequence. Although less frequently employed, other molecular-biology methods that do not require PCR such as the Northern blotting and RNAse protection assays, can also be utilized to validate alternatively spliced transcripts, particularly when the size differences between transcripts allow a clear distinction. Furthermore, large-scale proteomics experiments (e.g., mass spectrometry) can be used to identify and study the proteins resulting from the alternatively-spliced transcripts and to support the in-silico protein sequence predictions (Tress et al., 2017).
Lastly, the AS analysis combined with functional genetic experiments will ultimately allow understanding of the biological significance of the various alternatively spliced transcripts. These methods typically involve selective overexpression or knockdown of the transcripts (and the encoded proteins) using stable or transient plant transformations, followed by evaluation of the trait of interest. Biochemical experiments to decipher the encoded protein localization, protein-protein, and protein-RNA interactions can also provide mechanistic insights into the functions of the alternatively spliced transcripts (Dinesh-Kumar and Baker, 2000; Severing et al., 2012; Staiger and Brown, 2013; Szakonyi and Duque, 2018; Wang et al., 2018b).
Upcoming Research and Conclusions
In the past few years, HTS technologies has unraveled the breadth of AS that is occurring in plants (Shen et al., 2014; Mandadi and Scholthof, 2015; Thatcher et al., 2016; Calixto et al., 2018; Zhang and Xiao, 2018). The availability of vast amounts of omics data (currently >1 Petabases), largely based on ShR RNA-seq and gene-level analysis, within the publicly available repositories such as the NCBI SRA database (Leinonen et al., 2011) offer new opportunities to data mine and uncover AS landscapes among diverse plants and conditions. Such studies will allow global determination of conserved AS landscapes, patterns, and phenomenon occurring among the diverse evolutionary lineages of plants. Furthermore, combining the ShR RNA-seq data with LoR RNA-seq will allow discovery of the low-abundant transcripts, and/or resolve inadequacies that exist in reconstructing transcripts and complex transcript structures. The HTS will also advance our knowledge of AS landscapes and processes in complex polyploid plant genomes, which has largely remained understudied when compared to diploid plant genomes. Lastly, despite being well-positioned to study AS in plants at an unprecedented scale using HTS technologies, the challenge ahead lies in deciphering the biological relevance and molecular function of the various alternatively spliced transcripts and the encoded proteins. Thus, we suggest that the AS research community place equal emphasis in the AS validation using reverse-genetic approaches.
Author Contributions
RB, SI, EP, and KM designed the study and prepared the manuscript for submission. All authors have read and approved the manuscript.
Funding
This study was supported in part by funds from USDA-NIFA-AFRI (2016-67013-24738), and Texas A&M AgriLife Research Insect Vectored Diseases Seed Grant (114190-96210) to KM.
Conflict of Interest Statement
The authors declare that the research was conducted in the absence of any commercial or financial relationships that could be construed as a potential conflict of interest.
References
Ardui, S., Ameur, A., Vermeesch, J. R., and Hestand, M. S. (2018). Single molecule real-time (SMRT) sequencing comes of age: applications and utilities for medical diagnostics. Nucleic Acids Res. 46, 2159–2168. doi: 10.1093/nar/gky066
Calixto, C. P. G., Guo, W., James, A. B., Tzioutziou, N. A., Entizne, J. C., Panter, P. E., et al. (2018). Rapid and dynamic alternative splicing impacts the arabidopsis cold response transcriptome. Plant Cell 30, 1424–1444. doi: 10.1105/tpc.18.00177
Chamala, S., Feng, G., Chavarro, C., and Barbazuk, W. B. (2015). Genome-wide identification of evolutionarily conserved alternative splicing events in flowering plants. Front. Bioeng. Biotechnol. 3:33. doi: 10.3389/fbioe.2015.00033
Clark, M., Wrzesinski, T., Garcia-Bea, A., Kleinman, J., Hyde, T., Weinberger, D., et al. (2018). Long-read sequencing reveals the splicing profile of the calcium channel gene CACNA1C in human brain. bioRxiv [preprint]. doi: 10.1101/260562
Dinesh-Kumar, S. P., and Baker, B. J. (2000). Alternatively spliced N resistance gene transcripts: Their possible role in Tobacco mosaic virus resistance. Proc. Natl. Acad. Sci. U.S.A. 97, 1908–1913. doi: 10.1073/pnas.020367497
Filichkin, S., Priest, H. D., Megraw, M., and Mockler, T. C. (2015). Alternative splicing in plants: directing traffic at the crossroads of adaptation and environmental stress. Curr. Opin. Plant Biol. 24, 125–135. doi: 10.1016/j.pbi.2015.02.008
Filichkin, S. A., Priest, H. D., Givan, S. A., Shen, R., Bryant, D. W., Fox, S. E., et al. (2010). Genome-wide mapping of alternative splicing in Arabidopsis thaliana. Genome Res. 20, 45–58. doi: 10.1101/gr.093302.109
Foissac, S., and Sammeth, M. (2015). Analysis of alternative splicing events in custom gene datasets by AStalavista. Methods Mol. Biol. 1269, 379–392. doi: 10.1007/978-1-4939-2291-8_24
Haas, B. J., Papanicolaou, A., Yassour, M., Grabherr, M., Blood, P. D., Bowden, J., et al. (2013). De novo transcript sequence reconstruction from RNA-seq using the Trinity platform for reference generation and analysis. Nat. Protoc. 8, 1494–1512. doi: 10.1038/nprot.2013.084
Harvey, S. E., and Cheng, C. (2016). Methods for characterization of alternative RNA splicing. Methods Mol. Biol. 1402, 229–241. doi: 10.1007/978-1-4939-3378-5_18
Iida, K., Seki, M., Sakurai, T., Satou, M., Akiyama, K., Toyoda, T., et al. (2004). Genome-wide analysis of alternative pre-mRNA splicing in Arabidopsis thaliana based on full-length cDNA sequences. Nucleic Acids Res. 32, 5096–5103. doi: 10.1093/nar/gkh845
Irigoyen, S., Bedre, R. H., Scholthof, K. B., and Mandadi, K. K. (2018). Genomic approaches to analyze alternative splicing, a key regulator of transcriptome and proteome diversity in Brachypodium distachyon. Methods Mol. Biol. 1667, 73–85. doi: 10.1007/978-1-4939-7278-4_7
Kalyna, M., Simpson, C. G., Syed, N. H., Lewandowska, D., Marquez, Y., Kusenda, B., et al. (2012). Alternative splicing and nonsense-mediated decay modulate expression of important regulatory genes in Arabidopsis. Nucleic Acids Res. 40, 2454–2469. doi: 10.1093/nar/gkr932
Kent, W. J. (2002). BLAT–the BLAST-like alignment tool. Genome Res. 12, 656–664. doi: 10.1101/gr.229202
Koren, S., Schatz, M. C., Walenz, B. P., Martin, J., Howard, J. T., Ganapathy, G., et al. (2012). Hybrid error correction and de novo assembly of single-molecule sequencing reads. Nat. Biotechnol. 30, 693–700. doi: 10.1038/nbt.2280
Laloum, T., Martin, G., and Duque, P. (2018). Alternative splicing control of abiotic stress responses. Trends Plant Sci. 23, 140–150. doi: 10.1016/j.tplants.2017.09.019
Leinonen, R., Sugawara, H., Shumway, M., and International Nucleotide Sequence Database, C. (2011). The sequence read archive. Nucleic Acids Res. 39, D19–21. doi: 10.1093/nar/gkq1019
Liu, X., Mei, W., Soltis, P. S., Soltis, D. E., and Barbazuk, W. B. (2017). Detecting alternatively spliced transcript isoforms from single-molecule long-read sequences without a reference genome. Mol. Ecol. Resour. 17, 1243–1256. doi: 10.1111/1755-0998.12670
Love, M. I., Huber, W., and Anders, S. (2014). Moderated estimation of fold change and dispersion for RNA-seq data with DESeq2. Genome Biol. 15:550. doi: 10.1186/s13059-014-0550-8
Mandadi, K. K., and Scholthof, K.-B. G. (2015). Genome-wide analysis of alternative splicing landscapes modulated during plant-virus interactions in Brachypodium distachyon. Plant Cell 27, 71–85. doi: 10.1105/tpc.114.133991
Marquez, Y., Hopfler, M., Ayatollahi, Z., Barta, A., and Kalyna, M. (2015). Unmasking alternative splicing inside protein-coding exons defines exitrons and their role in proteome plasticity. Genome Res. 25, 995–1007. doi: 10.1101/gr.186585.114
Matsukura, S., Mizoi, J., Yoshida, T., Todaka, D., Ito, Y., Maruyama, K., et al. (2010). Comprehensive analysis of rice DREB2-type genes that encode transcription factors involved in the expression of abiotic stress-responsive genes. Mol. Genet. Genomics 283, 185–196. doi: 10.1007/s00438-009-0506-y
Modrek, B., and Lee, C. (2002). A genomic view of alternative splicing. Nat. Genet. 30, 13–19. doi: 10.1038/ng0102-13
Pan, Q., Shai, O., Lee, L. J., Frey, B. J., and Blencowe, B. J. (2008). Deep surveying of alternative splicing complexity in the human transcriptome by high-throughput sequencing. Nat. Genet. 40, 1413–1415. doi: 10.1038/ng.259
Pertea, M., Kim, D., Pertea, G. M., Leek, J. T., and Salzberg, S. L. (2016). Transcript-level expression analysis of RNA-seq experiments with HISAT, StringTie and Ballgown. Nat. Protoc. 11, 1650–1667. doi: 10.1038/nprot.2016.095
Reddy, A. S. N., Marquez, Y., Kalyna, M., and Barta, A. (2013). Complexity of the alternative splicing landscape in plants. Plant Cell 25, 3657–3683. doi: 10.1105/tpc.113.117523
Severing, E. I., Van Dijk, A. D., Morabito, G., Busscher-Lange, J., Immink, R. G., and Van Ham, R. C. (2012). Predicting the impact of alternative splicing on plant MADS domain protein function. PLoS ONE 7:e30524. doi: 10.1371/journal.pone.0030524
Shang, X., Cao, Y., and Ma, L. (2017). Alternative splicing in plant genes: a means of regulating the environmental fitness of plants. Int. J. Mol. Sci. 18:18020432. doi: 10.3390/ijms18020432
Shaul, O. (2015). Unique aspects of plant nonsense-mediated mRNA decay. Trends Plant Sci. 20, 767–779. doi: 10.1016/j.tplants.2015.08.011
Shen, Y., Zhou, Z., Wang, Z., Li, W., Fang, C., Wu, M., et al. (2014). Global dissection of alternative splicing in paleopolyploid soybean. Plant Cell 26, 996–1008. doi: 10.1105/tpc.114.122739
Simpson, C. G., Fuller, J., Calixto, C. P., Mcnicol, J., Booth, C., Brown, J. W., et al. (2016). Monitoring alternative splicing changes in Arabidopsis Circadian clock genes. Methods Mol. Biol. 1398, 119–132. doi: 10.1007/978-1-4939-3356-3_11
Simpson, C. G., Fuller, J., Maronova, M., Kalyna, M., Davidson, D., Mcnicol, J., et al. (2008). Monitoring changes in alternative precursor messenger RNA splicing in multiple gene transcripts. Plant J. 53, 1035–1048. doi: 10.1111/j.1365-313X.2007.03392.x
Staiger, D., and Brown, J. W. S. (2013). Alternative splicing at the intersection of biological timing, development, and stress responses. Plant Cell 25, 3640–3656. doi: 10.1105/tpc.113.113803
Szakonyi, D., and Duque, P. (2018). Alternative splicing as a regulator of early plant development. Front. Plant Sci. 9, 1174–1174. doi: 10.3389/fpls.2018.01174
Thatcher, S. R., Danilevskaya, O. N., Meng, X., Beatty, M., Zastrow-Hayes, G., Harris, C., et al. (2016). Genome-wide analysis of alternative splicing during development and drought stress in Maize. Plant Physiol. 170, 586–599. doi: 10.1104/pp.15.01267
Trapnell, C., Hendrickson, D. G., Sauvageau, M., Goff, L., Rinn, J. L., and Pachter, L. (2013). Differential analysis of gene regulation at transcript resolution with RNA-seq. Nat. Biotechnol. 31:46. doi: 10.1038/nbt.2450
Trapnell, C., Roberts, A., Goff, L., Pertea, G., Kim, D., Kelley, D. R., et al. (2012). Differential gene and transcript expression analysis of RNA-seq experiments with TopHat and Cufflinks. Nat. Protocols 7, 562–578. doi: 10.1038/nprot.2012.016
Tress, M. L., Abascal, F., and Valencia, A. (2017). Alternative splicing may not be the key to proteome complexity. Trends Biochem. Sci. 42, 98–110. doi: 10.1016/j.tibs.2016.08.008
Wang, B. B., and Brendel, V. (2006). Genomewide comparative analysis of alternative splicing in plants. Proc. Natl. Acad. Sci. U.S.A. 103, 7175–7180. doi: 10.1073/pnas.0602039103
Wang, M., Wang, P., Liang, F., Ye, Z., Li, J., Shen, C., et al. (2018a). A global survey of alternative splicing in allopolyploid cotton: landscape, complexity and regulation. New Phytol. 217, 163–178. doi: 10.1111/nph.14762
Wang, Y., Zhang, T., Song, X., Zhang, J., Dang, Z., Pei, X., et al. (2018b). Identification and functional analysis of two alternatively spliced transcripts of ABSCISIC ACID INSENSITIVE3 (ABI3) in linseed flax (Linum usitatissimum L.). PLoS ONE 13:e0191910. doi: 10.1371/journal.pone.0191910
Wu, T. D., and Watanabe, C. K. (2005). GMAP: a genomic mapping and alignment program for mRNA and EST sequences. Bioinformatics 21, 1859–1875. doi: 10.1093/bioinformatics/bti310
Zhang, C., Yang, H., and Yang, H. (2015). Evolutionary character of alternative splicing in plants. Bioinform Biol. Insights 9, 47–52. doi: 10.4137/BBI.S33716
Keywords: alternative splicing, high-throughput sequencing, bioinformatics, RNA-seq, PCR, non-sense-mediated decay
Citation: Bedre R, Irigoyen S, Petrillo E and Mandadi KK (2019) New Era in Plant Alternative Splicing Analysis Enabled by Advances in High-Throughput Sequencing (HTS) Technologies. Front. Plant Sci. 10:740. doi: 10.3389/fpls.2019.00740
Received: 21 March 2019; Accepted: 17 May 2019;
Published: 04 June 2019.
Edited by:
Laigeng Li, Institute of Plant Physiology and Ecology, Shanghai Institutes for Biological Sciences (CAS), ChinaReviewed by:
Peng Xu, University of Alabama at Birmingham, United StatesXiangjia Min, Youngstown State University, United States
Dominika Lewandowska, James Hutton Institute, United Kingdom
Copyright © 2019 Bedre, Irigoyen, Petrillo and Mandadi. This is an open-access article distributed under the terms of the Creative Commons Attribution License (CC BY). The use, distribution or reproduction in other forums is permitted, provided the original author(s) and the copyright owner(s) are credited and that the original publication in this journal is cited, in accordance with accepted academic practice. No use, distribution or reproduction is permitted which does not comply with these terms.
*Correspondence: Kranthi K. Mandadi, kkmandadi@tamu.edu