- Environmental Futures Research Institute, School of Environment and Science, Griffith University, Nathan, QLD, Australia
Chickpea (Cicer arietinum L.) is an important cool season food legume, however, its production is severely constrained by the foliar disease Ascochyta blight caused by the fungus Ascochyta rabiei (syn. Phoma rabiei). Several disease management options have been developed to control the pathogen, including breeding for host plant resistance. However, the pathogen population is evolving to produce more aggressive isolates. For host resistance to be effective, the plant must quickly recognize the pathogen and instigate initial defense mechanisms, optimally at the point of contact. Given that the most resistant host genotypes display rapid pathogen recognition and response, the approach taken was to assess the type, speed and pattern of recognition via Resistance Gene Analog (RGA) transcription among resistant and susceptible cultivated chickpea varieties. RGAs are key factors in the recognition of plant pathogens and the signaling of inducible defenses. In this study, a suite of RGA loci were chosen for further investigation from both published literature and from newly mined homologous sequences within the National Center for Biotechnology Information (NCBI) database. Following their validation in the chickpea genome, 10 target RGAs were selected for differential expression analysis in response to A. rabiei infection. This was performed in a set of four chickpea varieties including two resistant cultivars (ICC3996 and PBA Seamer), one moderately resistant cultivar (PBA HatTrick) and one susceptible cultivar (Kyabra). Gene expression at each RGA locus was assessed via qPCR at 2, 6, and 24 h after A. rabiei inoculation with a previously characterized highly aggressive isolate. As a result, all loci were differentially transcribed in response to pathogen infection in at least one genotype and at least one time point after inoculation. Among these, the differential expression of four RGAs was significant and consistently increased in the most resistant genotype ICC3996 immediately following inoculation, when spore germination began and ahead of penetration into the plant’s epidermal tissues. Further in silico analyses indicated that the differentially transcribed RGAs function through ADP-binding within the pathogen recognition pathway. These represent clear targets for future functional validation and potential for selective resistance breeding for introgression into elite cultivars.
Introduction
Chickpea (Cicer arietinum L.) is a staple cool season food legume, important in the Indian sub-continent, West Asia, North Africa and grown as a high-return cash export crop in Australia and North America (Du et al., 2012). However, production is seriously constrained by fungal disease Ascochyta blight, which is the most frequent and devastating disease of chickpea crops worldwide (Sagi et al., 2017). The fungus Ascochyta rabiei (syn. Phoma rabiei), can infect all parts of the plant above ground, and at any growth stage (Sharma and Ghosh, 2016).
Australia is the second largest global producer and exporter of chickpea (ABARE report from February 2, 2016), while India is the largest chickpea producer, whose production dwarfs that of all other countries. The first recorded A. rabiei epidemic in Australia occurred in 1998 (Du et al., 2012). With growing market demand and cash return, production in northern New South Wales and southern and central Queensland has recently increased. This has led to significantly increased risk from A. rabiei due to complacency in disease management best practice from novice growers and the potential for wetter winters than in southern growing regions. During the 2012–2014 seasons, the high rainfall in these northern regions led to widespread A. rabiei epidemics; and highly aggressive clonal isolates destroyed crops of the most resistant cultivars despite repeated fungicide applications (Moore et al., 2015b). Despite the presence of the teleomorph elsewhere, the Australian population is asexual, reliant on mutational events for favorable selection and potential adaptation (Leo et al., 2015). The emergence of growing numbers of highly aggressive isolates across the growing regions indicated sufficient genetic diversity within the clonal population to select for ability to overcome the fungicides and host resistance genes employed (Mehmood et al., 2017).
Since there appears to be a growing potential for A. rabiei to evolve new pathotypes with high aggressiveness (Mehmood et al., 2017), it is important for breeders to be able to select for germplasm with the best and most stable resistance. This may in part be informed by understanding the functional pathogen recognition mechanisms, of which RGAs play a key role and are responsible for the onward signaling and activating of plant defense responses shown to be involved in many plant pathosystems (Grant et al., 1998).
Resistance Gene Analogs (RGAs) are a large gene family with conserved domains and structural features that enable classification into either nucleotide binding site leucine rich repeat (NBS-LRR) or transmembrane leucine rich repeat (TM-LRR) sub-families. They function mainly as intracellular receptors that perceive the presence of pathogen effectors by direct binding of the pathogen effector proteins, or by monitoring the modification of host proteins after associating with the pathogen, to activate multiple defense signal transductions to restrict pathogen growth (Sagi et al., 2017). Emerging evidence indicates that an intermediate vesicle-type exosomal body is involved in delivering the molecules that initiate the chickpea signaling for defense to necrotrophic fungi (Boevink, 2017). In the Chickpea – A. rabiei pathosystem, RGAs are predicted to recognize the fungus and then induce signaling of defense molecules previously identified by Coram and Pang (2006), leading to resistance in several commonly grown chickpea cultivars (i.e., PBA Seamer).
Subsequent plant defense responses are complex and diverse at the genomic level, the expression of transcription factors and protein kinases, as well as the increase in cytosolic calcium are all involved in defense signaling (Grant and Mansfield, 1999). Moreover, the speed and coordination of the host’s perception of the pathogen, signal transduction and transcriptional activation are also vital to successful defense. In the study by Coram and Pang (2006), 13.6% of chickpea complementary DNAs (cDNAs) evaluated by microarray were differentially expressed in response to A. rabiei. Further, the kinetics of differential expression after inoculation of A. rabiei highlighted the differential timing of pathogen recognition and subsequent transcriptional changes associated with the A. rabiei defense response (Coram and Pang, 2005a,b; Leo et al., 2016).
Although the earlier studies identified some key defense-related mechanisms, the underlying pathogen recognition factors were not elucidated. In addition, the defense of chickpea to ascochyta blight is multigenic and governed by resistance-quantitative trait loci (R-QTL) with many QTLs for A. rabiei resistance identified on multiple linkage groups (Santra et al., 2000; Leo et al., 2016; Sagi et al., 2017). According to Sagi et al. (2017), 121 NBS-LRR genes are associated to R-QTL for A. rabiei. Subsequent assessment of their expression levels at 12, 24, 48, and 72 hpi revealed several RGAs that are deemed functional in early pathogen recognition. However, together with those previously identified by Leo et al. (2015), they represent only a subset of the possible recognition factors and their activities at earlier and crucial time points are still unknown. Characterization and functional assessment of a wider range of RGAs at the “pre-penetration” and “during penetration” stages will provide essential information for future targeted breeding of varieties able to quickly recognize and respond to this devastating pathogen. Therefore, the aims of this study were to: (1) Identify RGA candidates present in the chickpea genome through published literature searches and sequence analyses; (2) Validate the presence of RGA candidates within key resistant chickpea genotypes; (3) Assess the putative function of the RGA candidates via transcription in response to an aggressive isolate of A. rabiei at biologically important early interaction stages; and (4) Further characterize the putative function of the most responsive RGA candidates through predictive in silico analyses.
Materials and Methods
Target RGA Loci and Development of PCR Markers
Five sequences, representative of three RGA classes which were previously characterized and considered putatively functional in resistance to fusarium wilt, rust, and ascochyta blight (Palomino et al., 2009), were initially chosen for further assessment. These included RGAs of class 01, previously detected in faba bean and RGAs of classes 02 and 03, previously detected in chickpea (Palomino et al., 2009). Additionally, four chickpea NBS-LRR RGA loci were chosen from Leo et al. (2016). Finally, three RGA sequences, reported to be up-regulated in response to A. rabiei, were chosen from Sagi et al. (2017). Simultaneously, thirteen RGA sequences were sought from chickpea sequences deposited to the NCBI database1. The 13 sequences were chosen because they represented the breadth of the RGA families and they were unanimously identifiable from the existing database. Seeking and assigning of putative RGAs was performed using known motifs for specific RGA classes (NBS-LRR family) with a 99% of within-class identity threshold, while the motif information was referenced from Sekhwal et al. (2015).
PCR primers flanking the selected RGA loci were designed using Primer3web (version 4.0.02) with the following criteria: melting temperature (Tm) of 59 ± 3°C, and PCR amplicon size of 150–300 base pair (bp), primer length of 18–23 nucleotides and GC content of 40–60%. Primers were synthesized by SIGMA-ALDRICH.
Plant Material and Fungal Isolates
Four chickpea genotypes with differentially known disease reactions to A. rabiei were used; ICC3996, PBA Seamer, PBA HatTrick, and Kyabra (Table 1). It is worth mentioning that even the resistance varieties are evaluating show substantial disease symptoms under many typical field epidemic situations. Seed was obtained from the National Chickpea Breeding Program, Tamworth, NSW, Australia. Seedlings were grown in 15 cm diameter pots containing commercial grade potting mix (Richgro premium mix), with 5 seed per pot/replication (six replicates per host genotype and isolate). Plants were grown in a controlled growing environment (CGE) maintained at 22 ± 1°C with a 16/8 h (light/dark) photoperiod for 14 days until inoculation. The A. rabiei isolate FT13092-1 used in this experiment was collected in 2013 from Kingsford, South Australia (by Dr. Jenny Davidson of the South Australian Research and Development Institute). Isolate FT13092-1 is highly aggressive on PBA HatTrick, Kyabra, and is moderately aggressive on ICC3996 (Grains Research and Development Corporation annual report for project #UM00052; R. Ford pers. comm.). The single-spored isolate was cultured on V8 juice agar and maintained in the incubator for 14 days at 22 ± 2°C with a 12/12 h near-UV light irradiation (350–400 nm)/dark photoperiod.
Preparation of Inoculum and Bioassay
Inoculum was prepared by adding 10 mL of sterile distilled water to the cultured plates and scraping the pycnidia with a sterile bent glass rod to release pycnidiospores. The spore suspension was then filtered through muslin cloth and the final spore concentration was adjusted to 105 spores⋅mL−1. Since three replications are sufficient to show significant consistency, three replicates (three pots) of 14-day-old seedlings were sprayed using an air-pressured hand-held sprayer with a fine mist of prepared inoculum until run-off and labeled as treated groups. Another three replicates were sprayed with sterile water and labeled as untreated groups. Tween 20 (0.02% v/v) was added to the inoculum and water as a surfactant. All plants were covered with inverted plastic cups immediately after the inoculation according to the mini-dome technique (Chen et al., 2005) to ensure maximum humidity and darkness to induce optimum spore germination (Sambasivam et al., 2017) maintained in a CGE at 22 ± 1°C. The main stems and young leaf tissues from treated and untreated groups were collected at 2, 6, and 24 hpi into 25 mL falcon tubes, snap frozen in liquid N2, and stored at −80°C until processing. Following collection of foliar tissue for transcript analyses at each of the time points from individual plants, the remaining plant was left under the bioassay conditions to develop disease symptomology to confirm a viable infection had occurred.
RNA Extraction, cDNA Preparation, and Differential Expression via RT-qPCR
RNA was extracted from the leaf and stem tissues of inoculated and uninoculated samples using a NucleoSpin® RNA Plant kit (Macherey-Nagel, Germany) according to the manufacturer’s instructions. The RNA sample purity was assessed by reading the OD260/OD280 absorption ratio using a Nano drop spectrometer (ND-1000). Total RNA (1 μg) of each sample was used for Genomic DNA (gDNA) elimination and reverse transcription using a PrimeScriptTM RT reagent Kit with gDNA Eraser (Perfect Real Time; Takara Bio, United States). The quality of cDNA and absence of gDNA were evaluated through PCR by using the primer pair used to amplify the chickpea reference gene (CAC) from Reddy et al. (2016) which produced an amplicon that spanned intron-exon boundaries. The expected amplification product size was 110 bp and this was validated by electrophoresis. The cDNA samples were then diluted (1:50) with DNase/RNase free water for RT-qPCR. Each primer pair was assessed for PCR amplification on gDNA and cDNA samples. In addition, three reference genes (ABCT, UCP, and CAC) were selected from Reddy et al. (2016) and used as Inter-Run Calibrators (IRC), since they were previously shown to be stably expressed across many chickpea varieties. All primer sequences designed are listed in Supplementary Figure 1 and Supplementary Table 1. The PCR efficiency of each primer pair was evaluated by using serially diluted cDNA samples (100, 10−1, 10−10, 10−100, 10−1000). Bio-Rad CFX Manager 3.1 software (Bio-Rad, CA, United States) and a custom R script were used to calculate the correlation coefficient (R2), slope value, and PCR amplification efficiency (E) of each primer pair combination.
A SYBR® Premix Ex TaqTM II (TIi RNaseH Plus) kit was used for assessing target gene expression using optical 96 well plates on a BIO-RAD CFX96 real-time PCR detection system (Bio-Rad laboratories) and reactions were prepared according to the manufacturer’s instructions. The PCR reactions were performed in a total volume of 25 μL containing 12.5 μL of 2x SYBR® Premix Ex TaqTM II (TIi RNaseH Plus), 0.4 μM of each primer, and 2 μL of diluted cDNA template. The reaction conditions were set as 30 s at 95°C (initial denaturation); followed by 40 cycles of 95°C for 5 s, 60°C for 30 s (fluorescence reading), and then followed by a melt curve analysis at 65–95°C every 0.5°C for 10 s. All reactions were carried out in technical duplicates. If variations between duplicates were significant, a triplicate was performed, and the two closest data points were taken. IRC were used in every single plate, because all samples in this experiment could not be analyzed in the same run. A Non Template Control (NTC) was included for each primer combination, to detect any potential contamination from gDNA and/or primer dimer (Leo et al., 2016).
RT-qPCR Data Analysis
Cq data of all RGA that were differentially expressed between chickpea genotypes and treatments were imported into LinRegPCR software version 2017.1 (Ruijter et al., 2015) for further analyses. Samples that did not amplify or produced a low, high or inconsistent Cq value (under 5 or over 40 cycles) were removed. The raw Cq values of the expression of each RGA locus were then corrected according to their respective PCR efficiencies, and the mean values of the biological triplicates were calculated. The Delta-Delta-Cq (ddCq) algorithm was used to determine relative and differential expressions among varieties and treatments (Pfaffl, 2001). An R script was then used to generate the differential expression plots of each RGA locus. Relative expression data (ddCq) above 0 meant that the RGA gene at this time point/genotype was up-regulated in the treated compared to the control group, whereas negative ddCq indicated that the RGA gene was down-regulated at that point. A heatmap was constructed and displayed using R software based on the calculated mean fold-change in expression values among genotypes and time-points after normalization with the reference genes and untreated samples. Several statistical tests were then performed to provide evidence for real differences in RGA expression levels among genotypes and following inoculation: Firstly, a Levene test was performed to verify the homogeneity of variances, followed by a Shapiro–Wilk test to assess the normality of the variances. If both conditions were met, an ANOVA test was applied to compare the significance of expression differences between treated and untreated groups, otherwise, a non-parametric Kruskal–Wallis test was used to compare the groups. If the result was significant, pairwise comparisons among all sample groups were undertaken to test which group(s) were different from others using a Tukey test. All statistical analyses were carried out in the R Language and Environment for Statistical Computing (R Core Team, 2017). All R script developed for this study can be found at https://github.com/ziwei-zhou/Thesis_R_scripts. A p-value of 0.05 was used as the significance threshold in all statistical tests.
Analysis of RGA Protein Sequences
Bioinformatics and predictive in silico tools were used to further characterize RGAs. The predicted amino acid sequence of each RGA candidate was obtained from the NCBI database and imported into InterPro 53 (Jones et al., 2014) and KOBAS 3.0 software4 (Xie et al., 2011), which were used to classify the predicted proteins into families and to predict domains and important (i.e., binding) sites. The RGA that responded with the highest transcriptional response to the pathogen was chosen for secondary structure prediction using the Position Specific Iterated – BLAST based secondary structure prediction (PSIPRED) method5 (Jones, 1999). Three-dimensional atomic models of this RGA and its potential binding sites were predicted through RaptorX software6 (Källberg et al., 2012).
Results
RGA Locus Identification and Validation
In total, 25 RGA loci were identified from previous publications and based on known RGA motifs from within the chickpea sequences within the NCBI database. These were labeled from RGA 1 to 25. PCR products of the expected sizes were successfully amplified from 23 of the targeted putative loci across all four chickpea varieties assessed (Table 2). After primer efficiency testing, 10 RGAs produced a reliable and consistent linear amplification, based on their R2 result and E value (RGAs 4, 6, 8, 9, 10, 11, 12, 15, 21, and 23).
Quantitative Real-Time Expression Profiling of the RGA Genes
Differences in the transcription levels of the selected RGAs over time, after inoculation with isolate FT-13092-1, were observed among the four chickpea genotypes assessed (Figures 1A–J). Interestingly, RGA 8 and 10 were both significantly up-regulated at the earliest timepoint assessed, at 2 hpi and in only the resistant PBA Seamer and ICC3996 genotypes (Figures 1C,E). These then remained up-regulated for the duration of the experiment, potentially indicating their ability to recognize the pathogen prior to invasion. This may indicate that they provide sustained signaling, leading to the instigation of downstream defense occurring much faster in these genotypes than in the more susceptible ones. RGA 21 and 23 showed down regulations in ICC 3996 at the beginning of the experiment, and then sharply increased to up-regulations at 6 hpi (Figures 1I,J). Meanwhile, RGA 4, 9, and 15 were initially down-regulated with a subsequent sharp increase in most chickpea genotypes, potentially indicating an overall ability of these RGA to recognize the pathogen following invasion, possibly too late for effective defense signaling (Figures 1A,D,H). While the expression profiles of RGA 6, 11, and 12 were not so significant in the plots (Figures 1B,F,G).
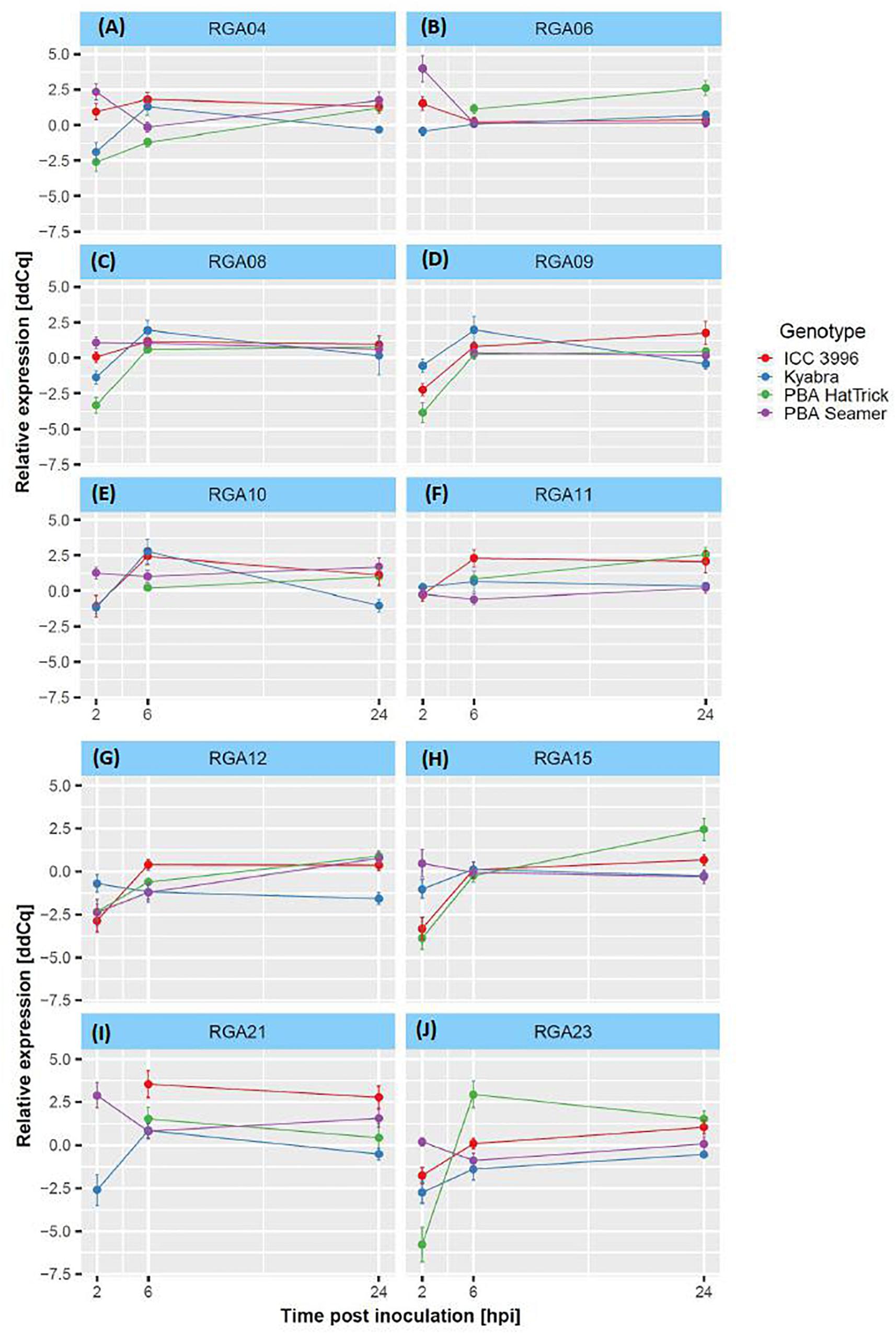
Figure 1. (A–J) Differential expression plots of 10 RGA loci among four chickpea genotypes (with reliable R2 and E-values) over the experimental time course after inoculation with the A. rabiei isolate, FT13092-1. Delta-Delta-Cq (ddCq) represents the relative expression ratio between treated and control samples, see Pfaffl (2001) and section “RT-qPCR Data Analysis” for details.
The relationships among the differential mean fold-changes of expressions of the 10 RGAs during the time-course were observed in the heatmap (Figure 2). Cluster 1 comprised of RGAs 4, 6, and 9. These were either down-regulated or unchanged for all genotypes (except in PBA HatTrick) at all time points assessed. Cluster 2 comprised of RGAs 8, 10, 21, and 23. These were up-regulated at 6 and 24 hpi and as stated above, RGA 8 and 10 were also up-regulated at 2 hpi in ICC3996, the commonly used A. rabiei resistance source in the Australian breeding program (Mehmood et al., 2017).
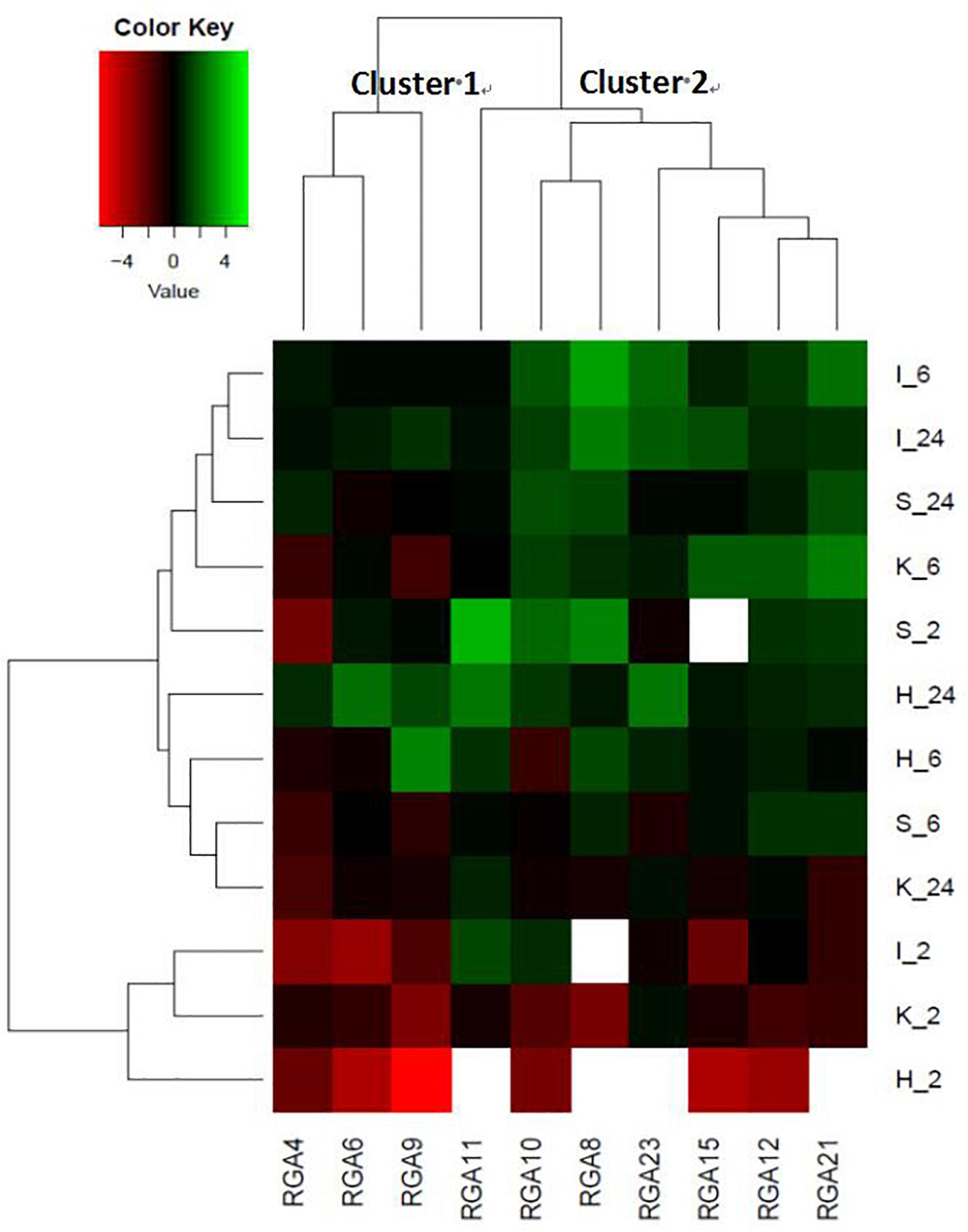
Figure 2. A heatmap representing the fold-change differences in expression among the 10 RGA target loci at 2, 6, and 24 hpi in four chickpea cultivars (PBA Seamer, PBA HatTrick, Kyabra, ICC 3996; so I_6 = ICC 3996_6hpi, same as others). Green color represents up-regulation, black color represents no change and red color represents down-regulation and color intensity indicates fold-change. No detectable expression is represented in white. The mean fold change expression values of the expression profiles for each treatment and genotype were normalized with the two mentioned reference genes and untreated samples.
Prediction of RGA Functional Groups
RGAs 8, 10, 21, and 23 were further assessed through in silico analyses to predict functional involvement in A. rabiei recognition. Their homologous super families and amino acid sequences were predicted (Table 3 and Supplementary Table 2, respectively) and NCBI reference sequences (RefSeq), gene and protein IDs were retrieved (Table 3). Domains and motifs were also predicted (Table 4). Whilst none of the four interrogated RGAs were able to be fully annotated, potentially indicating novelty, all were highly homologous (90–99% identity) with SUMM2 (KEGG orthology number K20599; Zhang et al., 2012). SUMM2 is an NB-LRR protein known to function in plant mitogen-activated protein kinase (MAPK) signaling pathways (Figure 3).
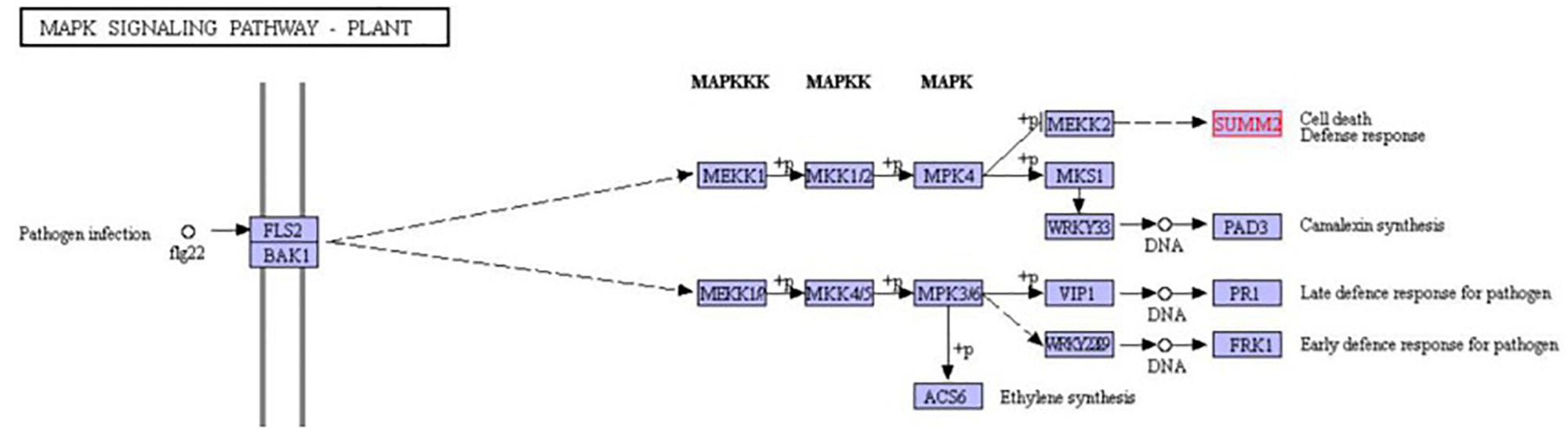
Figure 3. Part of the plant MAPK signaling pathway. The location of gene SUMM2 is labeled in red block (Kanehisa and Goto, 2000).

Table 3. Homologous super family predictions of the four chickpea target RGA sequences and their reference sequences definitions.
RGA 8 responded with the highest and earliest transcriptional response to the pathogen and so was chosen for further secondary structure prediction that revealed eight α-helices and four β-strands (Figure 4). The top predicted binding site domains for potential external sequences were identified with predicted binding residues at positions G1, G2, V3, G4, K5, T6, T7, L8, R112, M131, L139, K143, P169, and L170, and their collective predicted ligands were Magnesium ion (Mg2+), Adenosine diphosphate (ADP) and exchanging adenosine triphosphate (ATP) (Figure 5).
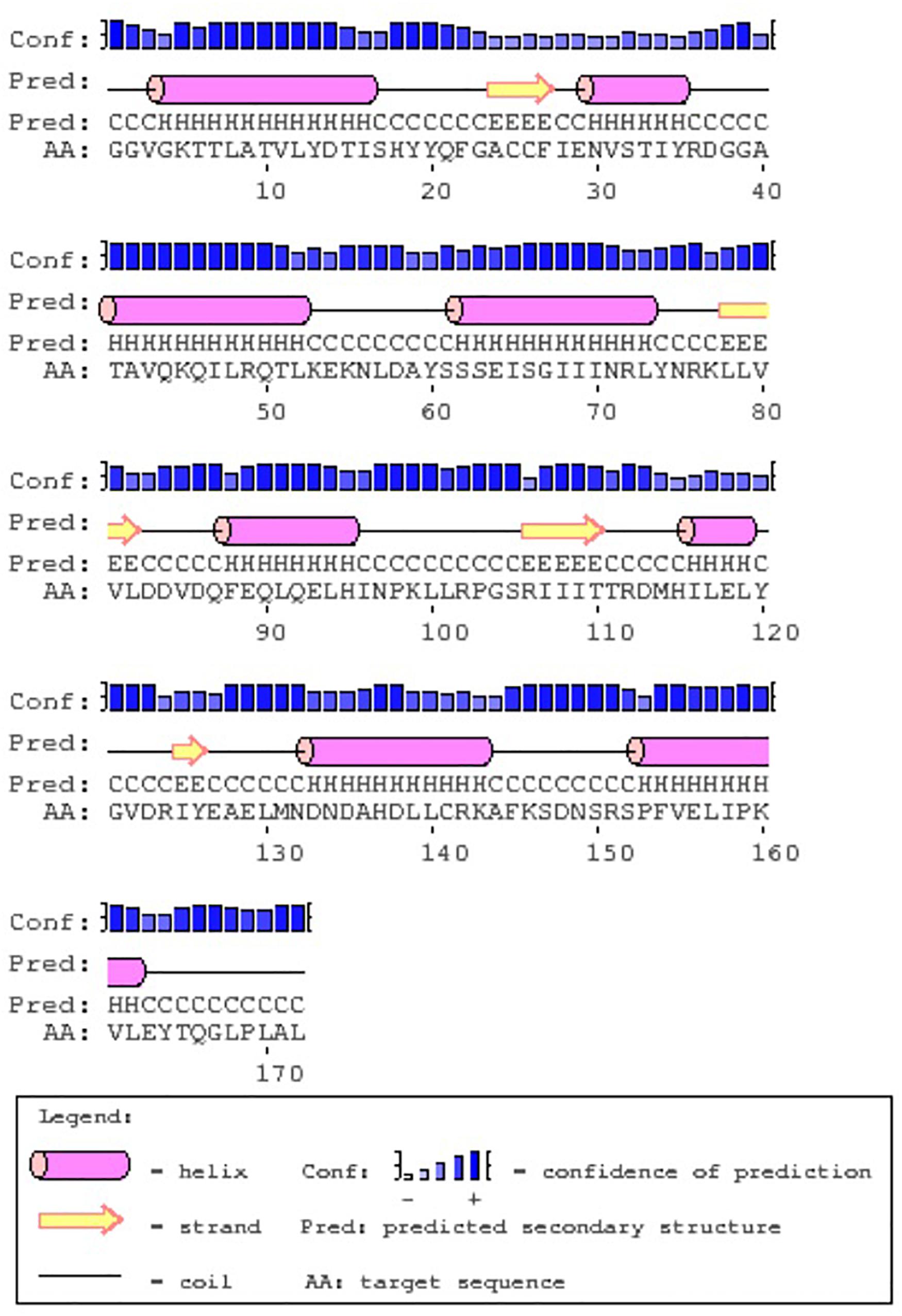
Figure 4. The predicted secondary protein structure derived from the PSIPRED server for the chickpea RGA 8.
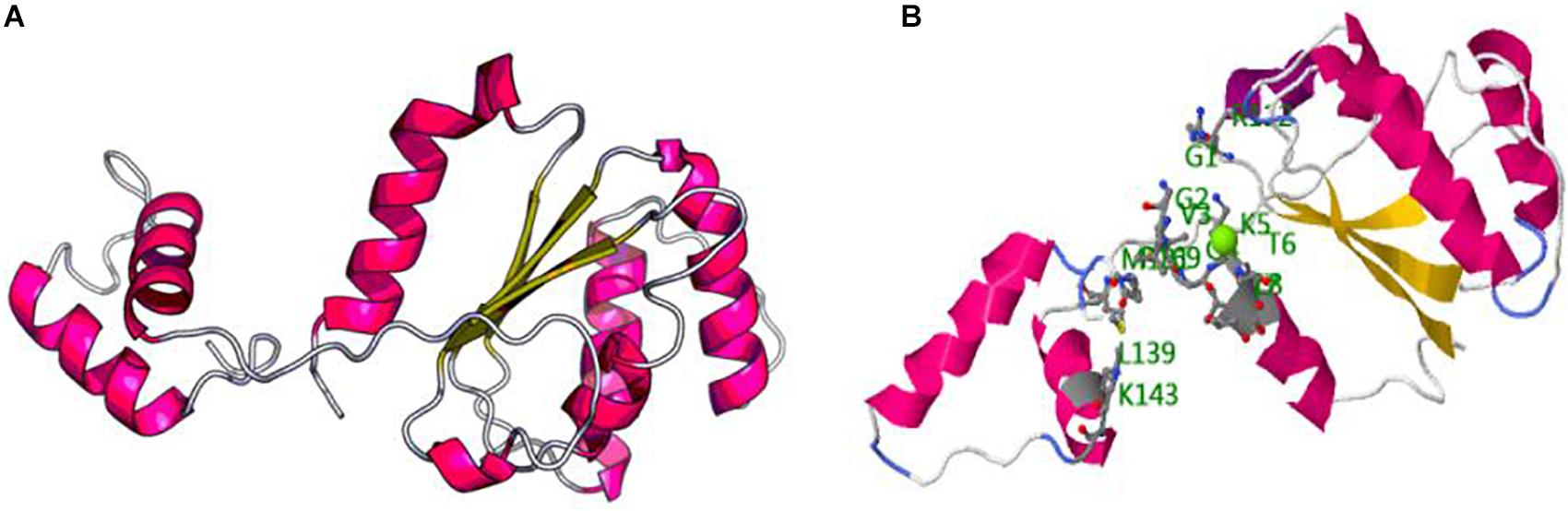
Figure 5. The predicted RGA 8 3D protein structure (A) and the proposed binding site residues (B, the red, blue, and green balls in the right picture).
Discussion
Plants have their own effective innate immune systems that they use to recognize pathogens when they come into contact or begin to invade and cause infection (Höhl et al., 1990; Ilarslan and Dolar, 2002; Jayakumar et al., 2005). Most necrotrophic pathogen-plant pathosystems utilize R-gene families otherwise known as RGAs as the receptors for initial pathogen perception (Sekhwal et al., 2015). For the chickpea-A. rabiei pathosystem, this study has assessed several existing and newly identified RGAs for their involvement in this perception process, which is proposed to lead to downstream signaling of biochemical and physical defense mechanisms (Palomino et al., 2009; Leo et al., 2016; Mehmood et al., 2017; Sagi et al., 2017).
The timing of RGA expression is thus crucial for a plant to be able to recognize a pathogen fast enough to incite effective defense responses. In this study, we found that a cluster of RGAs (Cluster 2), was up-regulated by 2–6 h following inoculation with a highly aggressive A. rabiei isolate and that this was consistent with the timing of spore growth (germ tube elongation) and penetration (appressoria development) (Sambasivam et al., 2008). If a plant can recognize and initiate defense responses faster, it may be able to contain the fungus long enough for more systemic resistance responses to occur, including hormone signaling, structural rearrangement and production of pathogenesis proteins. These alert the whole plant to the presence of the pathogen and direct a concerted attack at the site of invasion. This was proposed to be the case in the lentil – Ascochyta lentis pathosystem, whereby the host genotype was able to recognize and defend itself against the pathogen faster and was able to incite production of toxic phenolic compounds in a hypersensitive response as well as strengthen the cell wall around the invading hyphae compared to the slower and susceptible genotype (Sambasivam et al., 2017; Khorramdelazad et al., 2018). The fast recognition of the pathogen by several RGAs assessed in the chickpea – A. rabiei pathosystem stands in agreement with the observation of Leo et al. (2016) and Sagi et al. (2017) who also observed up-regulation as early as 2–6 hpi.
Since ICC3996 is the most widely used resistance source in breeding new resistant chickpea cultivars in Australia (Mehmood et al., 2017), it was important to determine which of the responsive RGA are present in this genetic background. In Cluster 2 of the heatmap (Figure 2), RGA 8, 10, 21, and 23 were up-regulated at 2–24 hpi in ICC3996. The homologous super family predictions indicated a common evolutionary origin among these four RGAs as evidence by the nucleoside triphosphate hydrolase domain (P-loop NTPase) (Leipe et al., 2003). P-loop NTPase is the most prevalent nucleotide-binding protein domain, catalyzing the hydrolysis of the beta-gamma phosphate bond of a bound nucleoside triphosphate (NTP) (Arya and Acharya, 2017). It is possible that these responsive RGAs in chickpea are Signal Transduction ATPases with Numerous Domains (STAND) P-loop NTPases and may function by ATP to initiate effector-triggered immunity (ETI) signaling.
RGA 8 was up-regulated in ICC3996, PBA Seamer, and PBA HatTrick at all times assessed, indicating that this locus is robust in its response to the pathogen. Also, since PBA Seamer and PBA HatTrick are progeny of crosses containing ICC3996 as the resistance donor parent (Dr. Kristy Hobson, Australian Chickpea Breeder, pers. comm.), this highlights that RGA 8 is heritable and may be selected for as a major contributor to the resistance response. The region containing the “GGVGK” domain in RGA 8 was proposed as a magnesium ion binding site, believed to induce phospho-transfer reactions (Li et al., 2001). This region was once showed resistance in tobacco after tobacco mosaic virus (TMV) infections (Les Erickson et al., 1999), and in response to Synchytrium endobioticum in potato (Hehl et al., 1999). Further, as mentioned, the secondary structure prediction for the RGA 8 revealed eight α-helices and four β-sheets (Figure 4), which is similar to the predicted plant disease resistance gene product reported by Rigden et al. (2000), found to function in His-Asp phosphor-transfer pathways. Therefore, the function of RGA 8 within defense to A. rabiei in chickpea may logically be predicted as a receptor to trigger the phospho-transfer signaling pathway through the activation of MAPK cascades.
Interestingly, RGA 10 was up-regulated in ICC 3996 and PBA Seamer but not in PBA HatTrick. The “resistant” status of PBA HatTrick was revised from “moderately resistant” to “moderately susceptible” in February 2017 by Pulse Breeding Australia, due to a substantial increase in aggressiveness within the isolate population (Mehmood et al., 2017). Meanwhile both ICC 3996 and PBA Seamer remained “resistant” at the time. RGA 10 contains domains homologous to Arabidopsis broad-spectrum mildew resistance protein RPW8 and a putative transposon-transfer assisting protein (TTRAP) (Xiao et al., 2001; Pulavarti et al., 2013). RPW8 is involved in resistance to a broad range of powdery mildew pathogens and TTRAP is associated with a family of small bacterial proteins largely derived from Clostrium difficile (Pulavarti et al., 2013). One could postulate that the functionality of the chickpea RGA 10 may have been lost in PBA HatTrick when exposed to a new highly aggressive isolate such as the one used in this study. This highlights the evolutionary risk of relying on one or few RGA (R-genes) for sustained resistance, as has been proven over again in other crops such as cereals in the race to breed for resistance against rust pathogens and in canola against the blackleg pathogen (Burdon et al., 2014; Moore et al., 2015a; Periyannan et al., 2017; Bousset et al., 2018; Zhang and Fernando, 2018).
RGA 21 was also up-regulated at 6 and 24 hpi in ICC 3996, meanwhile, it showed up-regulation in the susceptible Kyabra at 6 hpi. As showed in Table 4, RGA 21 contains a NB-ARC domain, a AAA ATPase domain, and a Leucine Rich Repeat, all belonging to the NBS-LRR family. Meanwhile, RGA 23 contained homologs of ArgK and PhoH-like proteins. ArgK is a member of the of P-loop GTPases, involved in the transport of positively charged amino acids (lysine, arginine, and ornithine) and has arginine kinase activity (Leipe et al., 2002). Previously, this was only found to exist in eukaryotic Caenorhabditis and Leishmania species. Similarly, the PhoH-like protein is a cytoplasmic protein. which has been shown to act in phosphate regulation in Escherichia coli (Kim et al., 1993). Further analyses will determine if the chickpea genes are complete and potentially functional.
Finally, the predicted proteins of all four RGAs share high similarities with the NB-LRR protein SUMM2 (Figure 3). SUMM2 is proposed to be activated with the MEKK1-MKK1/MKK2-MPK4 cascade when the MAPK signaling pathway is disrupted by pathogen effector binding, leading to the responses that cause localized cell death (Zhang et al., 2012). This indicates the potential for these RGA candidates to activate the well characterized defense responses to A. rabiei in chickpea when the MAPK signaling pathway is potentially suppressed by A. rabiei leading to apoptosis and the observed hypersensitive response (Leo et al., 2016; Mehmood et al., 2017).
Conclusion
Although many studies have been devoted to improving chickpea resistance to A. rabiei, sustained success may in part have been limited due to a lack of accurate knowledge of the pathogen recognition mechanism and how it may lead to subsequent instigated defense mechanisms. This is despite a great deal of effort in genetic mapping and characterization of multiple contributory defense-related QTLs, and their identification in diverse genetic backgrounds (Coram and Pang, 2005a,b; Palomino et al., 2009; Sagi et al., 2017). Although the physical locations of several genes underpinning the resistance responses have been uncovered, few studies have contributed to discovering the structures and functions of the actual resistance proteins. Fortunately, a great deal of knowledge exists on resistance proteins structure and function, as well as the molecular mechanisms of defense signaling proteins in Solanaceous plants (summarized by van Ooijen et al., 2007), which provides a guiding model for exploring the classes and functions of resistance proteins in other plant species. In this research, several existing and newly identified RGAs in chickpea were classified into previously described classes and assessed for their involvement in the A. rabiei perception process, which is proposed to lead to downstream signaling of biochemical and physical defense mechanisms (Palomino et al., 2009; Leo et al., 2016; Sagi et al., 2017). In conclusion, the future directions of this study should be focused on unraveling the protein functions of the selected RGAs that were differentially expressed in the resistant chickpea varieties after A. rabiei infection. This will provide further evidence for the selection of key RGAs in resistance breeding approaches.
Author Contributions
ZZ designed and conducted the experiments, analyzed the data, and wrote the manuscript. RF directed the project, co-designed the experiments, and edited the manuscript. IB and PS assisted with the experiments and data analyses.
Funding
This work was supported by the Environmental Futures Research Institute and the School of Environment and Science, Griffith University, Australia.
Conflict of Interest Statement
The authors declare that the research was conducted in the absence of any commercial or financial relationships that could be construed as a potential conflict of interest.
Acknowledgments
Drs. Yasir Mehmood and Audrey Leo are gratefully acknowledged for providing unpublished information on transcript and histopathology studies of the chickpea – A. rabiei interactions.
Supplementary Material
The Supplementary Material for this article can be found online at: https://www.frontiersin.org/articles/10.3389/fpls.2019.00644/full#supplementary-material
Footnotes
- ^ https://www.ncbi.nlm.nih.gov
- ^ http://bioinfo.ut.ee/primer3-0.4.0/
- ^ https://www.ebi.ac.uk/interpro/
- ^ http://kobas.cbi.pku.edu.cn/annotate.php
- ^ http://bioinf.cs.ucl.ac.uk/psipred/
- ^ http://raptorx.uchicago.edu/
References
Arya, P., and Acharya, V. (2017). Plant STAND P-loop NTPases: a current perspective of genome distribution, evolution, and function. Mol. Genet. Genomics 293, 17–31. doi: 10.1007/s00438-017-1368-1363
Boevink, P. C. (2017). Exchanging missives and missiles: the roles of extracellular vesicles in plant–pathogen interactions. J. Exp. Bot. 68, 5411–5414. doi: 10.1093/jxb/erx369
Bousset, L., Sprague, S. J., Thrall, P. H., and Barrett, L. G. (2018). Spatio-temporal connectivity and host resistance influence evolutionary and epidemiological dynamics of the canola pathogen Leptosphaeria maculans. Evol. Appl. 11, 1354–1370. doi: 10.1111/eva.12630
Burdon, J. J., Barrett, L. G., Rebetzke, G., and Thrall, P. H. (2014). Guiding deployment of resistance in cereals using evolutionary principles. Evol. Appl. 7, 609–624. doi: 10.1111/eva.12175
Chen, W., McPhee, K. E., and Muehlbauer, F. J. (2005). Use of a Mini-dome bioassay and grafting to study resistance of chickpea to ascochyta blight. J. Phytopathol. 153, 579–587. doi: 10.1111/j.1439-0434.2005.01022.x
Coram, T. E., and Pang, E. C. (2005a). Isolation and analysis of candidate ascochyta blight defense genes in chickpea. part I. generation and analysis of an expressed sequence tag (EST) library. Physiol. Mol. Plant Pathol. 66, 192–200. doi: 10.1016/j.pmpp.2005.08.003
Coram, T. E., and Pang, E. C. (2005b). Isolation and analysis of candidate ascochyta blight defense genes in chickpea. part II. microarray expression analysis of putative defense-related ESTs. Physiol. Mol. Plant Pathol. 66, 201–210. doi: 10.1016/j.pmpp.2005.08.002
Coram, T. E., and Pang, E. C. (2006). Expression profiling of chickpea genes differentially regulated during a resistance response to Ascochyta rabiei. Plant Biotechnol. J. 4, 647–666. doi: 10.1111/j.1467-7652.2006.00208.x
Du, W., Zhao, X., Raju, T., Davies, P., and Trethowan, R. (2012). Identification of Ascochyta rabiei disease resistance in chickpea genotypes. Euphytica 186, 697–704. doi: 10.1007/s10681-011-0554-3
Grant, M., and Mansfield, J. (1999). Early events in host-pathogen interactions. Curr. Opin. Plant Biol. 2, 312–319. doi: 10.1016/s1369-5266(99)80055-7
Grant, M. R., McDowell, J. M., Sharpe, A. G., de Torres Zabala, M., Lydiate, D. J., and Dangl, J. L. (1998). Independent deletions of a pathogen-resistance gene in Brassica and Arabidopsis. Proc. Natl. Acad. Sci. U.S.A. 95, 15843–15848. doi: 10.1073/pnas.95.26.15843
Hehl, R., Faurie, E., Hesselbach, J., Salamini, F., Whitham, S., Baker, B., et al. (1999). TMV resistance gene N homologues are linked to Synchytrium endobioticum resistance in potato. Theor. Appl. Genet. 98, 379–386. doi: 10.1007/s001220051083
Höhl, B., Pfautsch, M., and Barz, W. (1990). Histology of disease development in resistant and susceptible cultivars of chickpea (Cicer arietinum L.) inoculated with spores of Ascochyta rabiei. J. Phytopathol. 129, 31–45. doi: 10.1111/j.1439-0434.1990.tb04287.x
Ilarslan, H., and Dolar, F. S. (2002). Histological and ultrastructural changes in leaves and stems of resistant and susceptible chickpea cultivars to Ascochyta rabiei. J. Phytopathol. 150, 340–348. doi: 10.1046/j.1439-0434.2002.00763.x
Jayakumar, P., Gan, Y. T., Gossen, B. D., Warkentin, T. D., and Banniza, S. (2005). Ascochyta blight of chickpea: infection and host resistance mechanisms. Can. J. Plant Pathol. 27, 499–509. doi: 10.1080/07060660509507251
Jones, D. T. (1999). Protein secondary structure prediction based on position-specific scoring matrices1. J. Mol. Biol. 292, 195–202. doi: 10.1006/jmbi.1999.3091
Jones, P., Binns, D., Chang, H. Y., Fraser, M., Li, W., McAnulla, C., et al. (2014). InterProScan 5: genome-scale protein function classification. Bioinformatics 30, 1236–1240. doi: 10.1093/bioinformatics/btu031
Källberg, M., Wang, H., Wang, S., Peng, J., Wang, Z., Lu, H., et al. (2012). Template-based protein structure modeling using the RaptorX web server. Nat. Protoc. 7:1511. doi: 10.1038/nprot.2012.085
Kanehisa, M., and Goto, S. (2000). KEGG: kyoto encyclopedia of genes and genomes. Nucleic Acids Res. 28, 27–30. doi: 10.1093/nar/28.1.27
Khorramdelazad, M., Bar, I., Whatmore, P., Smetham, G., Bhaaskarla, V., Yang, Y., et al. (2018). Transcriptome profiling of lentil through the first 24 hours of Ascochyta lentis infection reveals key defence response genes. BMC Genomics 19:108. doi: 10.1186/s12864-018-4488-1
Kim, S. K., Makino, K., Amemura, M., Shinagawa, H., and Nakata, A. (1993). Molecular analysis of the phoH gene, belonging to the phosphate regulon in Escherichia coli. J. Bacteriol. 175, 1316–1324. doi: 10.1128/jb.175.5.1316-1324.1993
Leipe, D. D., Koonin, E. V., and Aravind, L. (2003). Evolution and classification of P-loop kinases and related proteins. J. Mol. Biol. 333, 781–815. doi: 10.1016/j.jmb.2003.08.040
Leipe, D. D., Wolf, Y. I., Koonin, E. V., and Aravind, L. (2002). Classification and evolution of P-loop GTPases and related ATPases1. J. Mol. Biol. 317, 41–72. doi: 10.1006/jmbi.2001.5378
Leo, A. E., Ford, R., and Linde, C. C. (2015). Genetic homogeneity of a recently introduced pathogen of chickpea. Ascochyta rabiei, to Australia. Biol. Invas. 17, 609–623. doi: 10.1007/s10530-014-0752-8
Leo, A. E., Linde, C. C., and Ford, R. (2016). Defence gene expression profiling to Ascochyta rabiei aggressiveness in chickpea. Theor. Appl. Genet. 129, 1333–1345. doi: 10.1007/s00122-016-2706-2
Les Erickson, F., Holzberg, S., Calderon-Urrea, A., Handley, V., Axtell, M., Corr, C., et al. (1999). The helicase domain of the TMV replicase proteins induces the N-mediated defense response in tobacco. Plant J. 18, 67–75. doi: 10.1046/j.1365-313X.1999.00426.x
Li, L., Tutone, A. F., Drummond, R. S., Gardner, R. C., and Luan, S. (2001). A novel family of magnesium transport genes in Arabidopsis. Plant Cell 13, 2761–2775. doi: 10.1105/tpc.13.12.2761
Mehmood, Y., Sambasivam, P., Kaur, S., Davidson, J., Leo, A. E., Hobson, K., et al. (2017). Evidence and consequence of a highly adapted clonal haplotype within the Australian Ascochyta rabiei population. Front. Plant Sci. 8:1029. doi: 10.3389/fpls.2017.01029
Moore, J. W., Herrera-Foessel, S., Lan, C., Schnippenkoetter, W., Ayliffe, M., Huerta-Espino, J., et al. (2015a). A recently evolved hexose transporter variant confers resistance to multiple pathogens in wheat. Nat. Genet. 47:1494. doi: 10.1038/ng.3439
Moore, K., Hobson, K., Sambasivam, P., Ford, R., Harden, S., Nash, P., et al. (2015b). Chickpea Ascochyta - is the Pathogen Changing and What are the Implications for Management. Available at: https://grdc.com.au/resources-and-publications/grdc-update-papers/tab-content/grdc-update-papers/2015/02/chickpea-ascochyta-is-the-pathogen-changing-and-what-are-the-implications-for-management (accessed February 24, 2015).
Palomino, C., Fernández-Romero, M. D., Rubio, J., Torres, A., Moreno, M. T., and Millán, T. (2009). Integration of new CAPS and dCAPS-RGA markers into a composite chickpea genetic map and their association with disease resistance. Theor. Appl. Genet. 118, 671–682. doi: 10.1007/s00122-008-0928-7
Periyannan, S., Milne, R. J., Figueroa, M., Lagudah, E. S., and Dodds, P. N. (2017). An overview of genetic rust resistance: from broad to specific mechanisms. PLoS Pathog. 13:e1006380. doi: 10.1371/journal.ppat.1006380
Pfaffl, M. W. (2001). A new mathematical model for relative quantification in real-time RT–PCR. Nucleic Acids Res. 29:e45.
Plett, J. M., Plett, K. L., Bithell, S. L., Mitchell, C., Moore, K., Powell, J. R., et al. (2016). Improved Phytophthora resistance in commercial chickpea (Cicer arietinum) varieties negatively impacts symbiotic gene signalling and symbiotic potential in some varieties. Plant Cell Environ. 39, 1858–1869. doi: 10.1111/pce.12757
Pulavarti, S. V., Eletsky, A., Lee, H. W., Acton, T. B., Xiao, R., Everett, J. K., et al. (2013). Solution NMR structure of CD1104B from pathogenic Clostridium difficile reveals a distinct α-helical architecture and provides first structural representative of protein domain family PF14203. J. Struct. Funct. Genomics 14, 155–160. doi: 10.1007/s10969-013-9164-8
Pulse Breeding Australia (2018). Chickpea. Available at: http://www.pulseaus.com.au/growing-pulses/bmp/chickpea (accessed May 12, 2018).
R Core Team (2017). R: A language and environment for statistical computing. Vienna: R Foundation for Statistical Computing.
Reddy, D. S., Bhatnagar-Mathur, P., Reddy, P. S., Cindhuri, K. S., Ganesh, A. S., and Sharma, K. K. (2016). Identification and validation of reference genes and their impact on normalized gene expression studies across cultivated and wild cicer species. PLoS One 11:e0148451. doi: 10.1371/journal.pone.0148451
Rigden, D. J., Mello, L. V., and Bertioli, D. J. (2000). Structural modeling of a plant disease resistance gene product domain. Proteins Struct. Funct. Bioinform. 41, 133–143. doi: 10.1002/1097-0134(20001001)41
Ruijter, J. M., Villalba, A. R., Hellemans, J., Untergasser, A., and van den Hoff, M. J. (2015). Removal of between-run variation in a multi-plate qPCR experiment. Biomol. Detect. Q. 5, 10–14. doi: 10.1016/j.bdq.2015.07.001
Sagi, M. S., Deokar, A. A., and Tar’an, B. (2017). Genetic analysis of NBS-LRR gene family in chickpea and their expression profiles in response to Ascochyta blight infection. Front. Plant Sci. 8:838. doi: 10.3389/fpls.2017.00838
Sambasivam, P., Taylor, P. W. J., and Ford, R. (2017). Pathogenic variation and virulence related responses of Ascochyta lentis on lentil. Eur. J. Plant Pathol. 147, 265–277. doi: 10.1007/s10658-016-0999-2
Sambasivam, P., Taylor, P. W. J., Pang, E. C. K., and Ford, R. (2008). Screening of lentil accessions for resistance to Australian isolates of Ascochyta lentis. J. Plant Pathol. 90(2, Suppl.):239. doi: 10.3389/fpls.2017.01038
Santra, D., Tekeoglu, M., Ratnaparkhe, M., Kaiser, W. J., and Muehlbauer, F. J. (2000). Identification and mapping of QTLs conferring resistance to ascochyta blight in chickpea. Crop Sci. 40, 1606–1612.
Sekhwal, M. K., Li, P., Lam, I., Wang, X., Cloutier, S., and You, F. M. (2015). Disease resistance gene analogs (RGAs) in plants. Int. J. Mol. Sci. 16, 19248–19290. doi: 10.3390/ijms160819248
Sharma, M., and Ghosh, R. (2016). An update on genetic resistance of chickpea to ascochyta blight. Agronomy 6:18. doi: 10.3390/agronomy6010018
van Ooijen, G., van den Burg, H. A., Cornelissen, B. J., and Takken, F. L. (2007). Structure and function of resistance proteins in solanaceous plants. Annu. Rev. Phytopathol. 45, 43–72. doi: 10.1146/annurev.phyto.45.062806.094430
Xiao, S., Ellwood, S., Calis, O., Patrick, E., Li, T., Coleman, M., et al. (2001). Broad-spectrum mildew resistance in Arabidopsis thaliana mediated by RPW8. Science 291, 118–120. doi: 10.1126/science.291.5501.118
Xie, C., Mao, X., Huang, J., Ding, Y., Wu, J., Dong, S., et al. (2011). KOBAS 2.0: a web server for annotation and identification of enriched pathways and diseases. Nucleic Acids Res. 39(Suppl._2), W316–W322. doi: 10.1093/nar/gkr483
Zhang, X., and Fernando, W. D. (2018). Insights into fighting against blackleg disease of Brassica napus in Canada. Crop Pasture Sci. 69, 40–47.
Keywords: Resistance Gene Analogs, ascochyta blight, chickpea, host resistance, expression profiling
Citation: Zhou Z, Bar I, Sambasivam PT and Ford R (2019) Determination of the Key Resistance Gene Analogs Involved in Ascochyta rabiei Recognition in Chickpea. Front. Plant Sci. 10:644. doi: 10.3389/fpls.2019.00644
Received: 14 December 2018; Accepted: 29 April 2019;
Published: 17 May 2019.
Edited by:
Jose C. Jimenez-Lopez, Consejo Superior de Investigaciones Científicas (CSIC) Granada, SpainReviewed by:
Maryam Nasr Esfahani, Lorestan University, IranTom Warkentin, University of Saskatchewan, Canada
Eva Madrid, Max Planck Institute for Plant Breeding Research, Germany
Copyright © 2019 Zhou, Bar, Sambasivam and Ford. This is an open-access article distributed under the terms of the Creative Commons Attribution License (CC BY). The use, distribution or reproduction in other forums is permitted, provided the original author(s) and the copyright owner(s) are credited and that the original publication in this journal is cited, in accordance with accepted academic practice. No use, distribution or reproduction is permitted which does not comply with these terms.
*Correspondence: Rebecca Ford, UmViZWNjYS5mb3JkQGdyaWZmaXRoLmVkdS5hdQ==