- State Key Laboratory of Crop Genetics & Germplasm Enhancement, Hybrid Cotton R & D Engineering Research Center, Ministry of Education, Nanjing Agricultural University, Nanjing, China
Cotton is an important industrial crop worldwide and upland cotton (Gossypium hirsutum L.) is most widely cultivated in the world. Due to ever-increasing water deficit, drought stress brings a major threat to cotton production. Thus, it is important to reveal the genetic basis under drought stress and develop drought tolerant cotton cultivars. To address this issue, in present study, 319 upland cotton accessions were genotyped by 55,060 single nucleotide polymorphisms (SNPs) from high-density CottonSNP80K array and phenotyped nine drought tolerance related traits. The two datasets were used to identify quantitative trait nucleotides (QTNs) for the above nine traits using multi-locus random-SNP-effect mixed linear model method. As a result, a total of 20 QTNs distributed on 16 chromosomes were found to be significantly associated with six drought tolerance related traits. Of the 1,326 genes around the 20 QTNs, 205 were induced after drought stress treatment, and 46 were further mapped to Gene ontology (GO) term “response to stress.” Taken genome-wide association study (GWAS) analysis, RNA-seq data and qRT-PCR verification, four genes, RD2 encoding a response to desiccation 2 protein, HAT22 encoding a homeobox-leucine zipper protein, PIP2 encoding a plasma membrane intrinsic protein 2, and PP2C encoding a protein phosphatase 2C, were proposed to be potentially important for drought tolerance in cotton. These results will deepen our understanding of the genetic basis of drought stress tolerance in cotton and provide candidate markers to accelerate the development of drought-tolerant cotton cultivars.
Introduction
Cotton (Gossypium spp.) is the most important natural fiber crop and is also a significant oilseed crop. The upland cotton (Gossypium hirsutum L.), which accounts for 95% of the annual cotton production worldwide, is the most cultivated species. However, cotton production is limited by various abiotic and biotic stresses. Of them, drought stress becomes a major threat to substantial loss of cotton yield due to the ever-increasing scarcity of water around the world (Pettigrew, 2004). There is a urgent need to ascertain the molecular and genetic basis underlying the cotton response to drought stress and to develop cotton cultivars with improved drought tolerance.
Quantitative trait loci (QTLs) mapping was an effective tool which was generally used to reveal the genetic basis of complex quantitative traits in cotton (Li C. et al., 2013; Fang et al., 2014; Wang et al., 2014). However, using traditional molecular markers, such as restriction fragment length polymorphism (RFLP) and simple sequence repeat (SSR), only a few QTLs related to drought stress were discovered in a wide region (Levi et al., 2009; Saleem et al., 2015; Zheng et al., 2016) because of the narrow genetic diversity and low map density in modern upland cotton accessions (Fang et al., 2017; Huang et al., 2017; Sun et al., 2017). In addition, these QTLs have not been applied to cotton breeding either. Recently, with the development of functional genomics and transcriptomics, a plenty of genes were found to be involved in drought resistance, including protein kinases, transcription factors and some structural genes (Ashraf et al., 2018). And these factors are related to different signal transduction pathway in response to drought stress. For example, GbMYB5 and GhWRKY17 are positively involved in response to drought stress (Yan et al., 2014; Chen et al., 2015), while a complete MAP kinase cascade that phosphorylates and activates GhWRKY59 is involved in abscisic acid (ABA)-independent signaling pathway to regulate cotton drought responses (Li et al., 2017). And overexpression of GhNAC2 can enhance root growth and improve tolerance to drought in transgenic cotton and Arabidopsis (Gunapati et al., 2016). Nevertheless, the functional verification for only few genes related to drought tolerance was reported. How to excavate more drought stress related genes accurately and effectively and to utilize them for breeding drought-tolerant cotton cultivars remain a big challenge.
Compared with traditional molecular markers, single nucleotide polymorphisms (SNPs) are the most abundant DNA variation distributed along the genome, with high density, bi-allelic and co-dominant characteristics. Recent years, the application of SNP arrays (Hulse-Kemp et al., 2015; Cai et al., 2017), sequencing and re-sequencing (Li et al., 2015; Zhang T. et al., 2015) for upland cotton accessions made it possible to improve the resolution of genetic maps and the accuracy of QTL mapping. Genome-wide association study (GWAS) is an effective method, which can associate phenotypes with genotypes in natural populations and reveal vast natural allelic variations and candidate genes based on linkage disequilibrium (LD), and have been widely used in crop plants, such as rice (Huang et al., 2010; Yano et al., 2016), maize (Li H. et al., 2013; Yang et al., 2014), and soybean (Zhang J. et al., 2015). Based on the rapid-developed studies for genome-wide SNPs in cotton, GWAS for several important agronomic traits has been performed. Using CottonSNP63K SNP array and 719 diverse G. hirsutum accessions, GWAS was conducted by integrating different environment tests of fiber quality traits with the SNP genotyping data, and forty-six SNPs were found to be significantly associated with five fiber quality traits (Sun et al., 2017). Using the same CottonSNP63K SNP array, 503 G. hirsutum accessions were genotyped for a GWAS with sixteen agronomic traits, and a total of 324 SNPs and 160 candidate quantitative trait nucleotide (QTN) regions were found to be significantly associated with these agronomic traits (Huang et al., 2017). With genome-wide resequencing for 318 cotton landraces and modern improved accessions or lines, 119 associated loci, including 71 for yield-related traits, 45 for fiber qualities and three for resistance to Verticillium wilt were identified by GWAS (Fang et al., 2017). Similarly, with resequencing 267 cotton accessions, 19 candidate loci for fiber-quality-related traits were reported (Wang et al., 2017). Recently, through integrating genotyping variation and phenotyping data of 13 fiber-related traits across 12 environments for 419 diverse G. hirsutum accessions, 7,383 unique SNPs were found to be significantly associated with these traits. The results showed that more associated loci were identified for fiber quality than fiber yield, and fiber genes in the D subgenome were more than those in the A subgenome (Ma et al., 2018). These studies indicated that GWAS was suitable for detecting QTNs of complex traits in plants. Nevertheless, GWAS based on SNP markers and using large natural populations for drought tolerance related traits has not been reported in cotton.
To determine the key QTN regions and candidate genes significantly associated with cotton drought response, we deployed GWAS of drought stress through integrating the genotypic data of upland cotton accessions by the high-density CottonSNP80K array analysis with their various phenotypic data response to drought stress. Further, the candidate genes were screened by integrating GWAS and gene expression data with qRT-PCR confirmation. This study will provide not only elite genetic resources with candidate SNPs but also key genes to accelerate the drought stress improvement of upland cotton.
Materials and Methods
Plant Materials
A total of 319 upland cotton accessions, with 306 cultivars/lines collected from China and 13 landraces introduced from the United States, were used in this study. The accessions in China were mainly collected from four different ecological growing regions: the Yellow River region (YRR, 183), the Yangtze River region (YtRR, 82), the Northwestern inland region (NIR, 22), the Northern China region, the specifically early maturation region (NSEMR, 16), and three unknown origin cotton accessions (Supplementary Table S1).
Phenotypic Analysis
In 2015 and 2017, the 319 upland cotton accessions were planted in the green house with hydroponics and in the phytotron with soil culture in Nanjing Agricultural University, Nanjing, Jiangsu Province, China, respectively. Pilot experiments were performed to screen the suitable concentrations of Polyethylene glycol 6000 (PEG 6000) for investigating the drought tolerance capacities of different upland cotton accessions. As a result, 15% PEG 6000 was used as drought stress treatment for water culture and 10% PEG 6000 for soil culture analysis.
All the accessions were grown in 1/2 diluted Hoagland solution (Hoagland and Arnon, 1950). At the stage of seedlings with five leaves, the experimental plants were treated with 15% PEG 6000 as drought stress, and plants only grown in 1/2 diluted Hoagland solution as control. After 48 h, the indicators related to drought tolerance were measured, including plant height (PH), shoot dry matter (SDM), and root dry matter (RDM). At the same time, top second leaf of the plants were sampled to measure proline content (PC), superoxide dismutase activities (SOD), malonaldehyde content (MDA) and soluble sugar content (SS). In addition, seeds from 319 upland cotton accessions were planted in nursery soil with 10% PEG 6000 solution as drought stress treatment and only watering as control. After 7 days, hypocotyl length (HL) and germination percentage (GP) were measured. For water culture experiments, we selected six seedlings with the relatively uniform growth for each treatment. Each two as a biological replicate, and together three biological replicates were set to investigate the traits, including PH, SDM, RDM, PC, SOD, MDA, and SS. For soil culture experiments, we selected 12 well-developed seeds as a biological replicate for each treatment, with three biological replicates to measure HL and GP.
PH was measured by the length from cotyledonary node to top of the plant. SDM and RDM were measured by weight of aboveground and underground part of the plant after drying at 65°C, respectively. PC was determined by acidic ninhydrin reaction as previously described by Bates et al. (1973). SOD activities were determined by measure inhibition of photochemical reduction of nitro blue tetrazolium (NBT) according to the method of Beyer and Fridovich (1987). MDA content was determined by a modified thiobarbituric acid (TBA) reaction (Hodges et al., 1999). SS content was determined by the colorimetric method using anthrone reagent according to Odjegba and Fasidi (2006). HL was measured by the length of hypocotyl. GP was calculated by ratio of germinated seeds number and planted seeds number.
Factor Analysis
For the drought tolerance evaluation, factor analysis was performed using SPSS software1. Kaiser–Meyer–Olkin (KMO) measurement and Bartlett’s statistic were calculated to determine the selected variables. Factors were extracted by the cumulative-contribution-rate-more-than-85% rule and comprehensive evaluation of the drought tolerance based on factor scores (Chen, 2013).
SNP Genotyping
Genomic DNA of the 319 cotton accessions was extracted according to the method described by Paterson et al. (1993). A CottonSNP80K array containing 77,774 SNPs (Cai et al., 2017), was used to genotype the 319 accessions. Qualified DNA was hybridized to the array following the Illumina protocols. The Illumina iScan array scanner was used to scan arrays, and GenomeStudio Genotyping software (V2011.1, Illumina, Inc.) was employed to cluster SNP alleles and genotyping. All 77,774 SNPs were tested and manually adjusted as described by Cai et al. (2017). The SNP data set with a calling rate < 0.9 and MAF < 0.05 was further filtered, and the high quality data was used for subsequent analysis.
Population Characteristics and Linkage Disequilibrium Analysis
PLINK V1.90 software2 was used to conduct the similarity analysis and clustering of 319 cotton accessions. Based on the distance matrix data (1-IBS, identity-by-state), phylogenetic trees were constructed using TASSEL 5.0 software3, and visually edited by Figtree software4. The IBS matrix data was used to conduct principal component analysis (PCA). The correlation coefficient (r2) of alleles was calculated to measure linkage disequilibrium (LD) in each group level using PLINK V1.90, and LD blocks containing SNP loci associated with target traits were generated using the R software package “LD heatmap.”
GWAS and Identification of Candidate Genes
A total of 55,060 SNPs (calling rate ≥ 0.9 and MAF ≥ 0.05) were used for GWAS. To explore the SNP-trait association, multi-locus random-SNP-effect mixed linear model (mrMLM) (Wang S.B. et al., 2016) was employed using the R package “mrMLM” with the following parameters: Critical P-value in rMLM: 0.001; Search radius of candidate gene (Kb): 100; Critical LOD score in mrMLM: 3. And the Q+K model was used. Population structure (Q) matrix was calculated using admixture 1.3 with k = 3, and kinship (K) matrix was calculated by the R package “mrMLM”. Putative candidate genes were identified flanking 500 Kb of peak SNPs (the most significant SNPs). Gene ontology (GO) analysis was implemented using AgriGO5, and candidate genes in “response to stress” terms were selected for further analysis.
Transcriptome Sequencing and Quantitative Real-Time PCR Analysis
The seedlings of upland cotton genetic standard line, G. hirsutum acc. TM-1, with two simple leaves and one heart-shaped leaf, was treated with 15% PEG. The cotton leaf samples were collected in different time-points after treating 0, 6, 12, 24, 48, and 72 h, respectively. Total RNA was extracted from these samples using the Biospin plant total RNA extraction kit (Bioer Technology Co., Ltd.). After pre-processing the RNA-seq data with an NGS QC toolkit (Patel and Jain, 2012), the reads were mapped to the G. hirsutum TM-1 genome using a Tophat spliced aligner with default parameters (Trapnell et al., 2009). The genome-matched reads from each library were assembled with Cufflinks (Trapnell et al., 2012). Cuffmerge was then used to merge the individual transcript assemblies into a single transcript set. Lastly, Cuffdiff was used to detect differentially expressed genes (DEGs) with a cutoff of 0.05 q-value. Three biological replicates from each sample were used for all RNA-seq experiments.
For quantitative real-time PCR (qRT-PCR) analysis, first-strand cDNA was synthesized using the reverse transcription polymerase reaction system (Promega, United States) and adjusted to ∼100 ng/μL with a One Drop Spectrophotometer OD-1000+ (OneDrop, Nanjing, China). qRT-PCR was deployed on an ABI 7500 real-time PCR system6. The qRT-PCR amplification program previously described by Provenzano and Mocellin (2007) was used. The relative expression level was calculated using the 2-ΔCT method (Livak and Schmittgen, 2001) with three biological and technical replicates, respectively. The expression level of GhHis3 (Accession No. AF024716) was used as an internal control (Gutierrez et al., 2008). All the primers were summarized in Supplementary Table S2.
Statistical Analysis
Correlation analysis among drought tolerance traits was performed using SPSS software, ∗ and ∗∗ present the significant differences at the 5% and 1% levels, respectively. qRT-PCR data was analyzed using Excel software and shown as the mean ± SD. Multiple comparison in one-way ANOVA was conducted by LSD method at the 0.05 and 0.01 levels, which were marked by ∗ and ∗∗, respectively.
Results
Phenotypic Variation in Drought Tolerance Related Traits
To evaluate the phenotypic variation under drought stress in the natural population, the seeds or seedlings of 319 upland cotton accessions were treated in PEG stress and in well-watered controls. Nine traits related to drought stress tolerance were measured, including HL and GP at germinating stage; PH, SDM, RDM, PC, SOD activities, MDA content and SS content at seedling stage, respectively. The mean and extreme values of five drought-tolerance traits were lower under drought stress than that in control plants and four traits showed higher values under drought stress condition than that in control plants. The coefficients of variation (CV, %) of nine drought tolerance related traits ranged from 11.77 (HL) to 95.89 (PC) under drought stress, and 11.79 (HL) to 92.70 (PC) under well-watered condition, respectively. Furthermore, it showed higher CV value under drought stress treatment than that in control for most drought-tolerance related traits, indicating the wide variation under drought stress among cotton accessions used in this study (Table 1).
The relative values of the nine drought-tolerance traits were further calculated using the ratio of the phenotypic effects value under drought stress and that under well-watered control condition. Relative values of each trait were conformed to Gaussian distribution (Supplementary Figure S1). To explore the relationships among nine drought-tolerance traits, correlation analysis was conducted. As a result, relative PH (RPH), relative SDM (RSDM) and relative RDM (RRDM) showed significant and positive correlation each other. We also detected a significant and positive correlation among different biochemical index, involved in relative PC (RPC), relative MDA (RMDA), relative SS (RSS), and relative SOD (RSOD). In addition, relative HL (RHL) showed significant and positive correlation with relative GP (RGP) and RSDM. However, both RHL and RSDM showed a significant and negative correlation with RPC, RMDA, and RSS, respectively (Table 2).
Comprehensive Evaluation of Drought Tolerance
In order to identify the drought tolerance of 319 cotton accessions, factor and cluster analyses were performed with relative values of nine drought-tolerance traits. The KMO value was 0.634 (>0.5), and the Bartlett’s statistic value p < 0.05, indicating that the raw data was suitable for factor analysis. A six-factor solution that accounted for 89.45% of the total variance was obtained (Supplementary Table S3) (Chen, 2013). Factor 1 represented the biochemical index factor at seedling stage, including RPC, RMDA, and RSS. Factor 2 was regarded as the physiological index factor, including RPH and RSDM. Factors 3–6 represented RSOD, RHL, RGP, and RRDM, respectively (Supplementary Table S4). In addition, the F factor composite score for the drought-tolerance of each cotton accession was calculated by six F factors. Based on drought tolerance capacity with different F factors, cluster analysis showed that the 319 upland cotton accessions were divided into four groups. Totally, 16, 75, 207, and 21 accessions were clustered into advanced, medium, sensitive and extremely sensitive types to drought stress tolerance with F factor ranging from 0.818 to 1.938, 0.239 to 0.742, -0.637 to 0.209, -1.385 to -0.655, respectively (Supplementary Table S1).
Genetic Variation Based on SNPs
We genotyped 319 upland cotton accessions using the CottonSNP80K array. GenomeStudio software (V2011.1, Illumina, Inc.) was used to genotype with a manual corrected clustering file (Cai et al., 2017). The genotypic data revealed that these cotton accessions possessed a high average call rate of 99.39%. With low-quality (call rate < 90% and minor allele frequency < 0.05) loci filtered, a final set of 55,060 SNPs was obtained, with 30,075 and 24,985 SNPs in the At and Dt subgenomes, respectively. These SNP markers were distributed over the entire genome, expect chromosomes A02, A03, and A04 with less SNP density. In addition, the polymorphism information content (PIC) values ranged from 0.263 to 0.389 among chromosomes, and the mean PIC value of the At and Dt subgenomes was 0.338 and 0.334, respectively (Table 3).
Population Structure and Linkage Disequilibrium
Principal component analysis and neighbor-joining tree were conducted to infer population stratification. A pairwise distance matrix derived from a modified Euclidean distance for all polymorphic SNPs was calculated to construct neighbor-joining trees using TASSEL 5.0 software. As a result, the 319 accessions could be clustered into four groups, which contained 61, 33, 87, and 138 accessions, respectively. We found that the four clustered groups had no relationships with their geographic origin (Figure 1A and Supplementary Table S1). Further, clustering data in the phylogenetic tree matched to PCA results well (Figure 1B). We also performed linkage disequilibrium (LD) analysis using PLINK software and evaluated Pairwise LD using squared allele frequency correlations (r2). The LD rate declining to half its maximum value was 980 Kb, with >1000 Kb in At and 760 Kb in Dt subgenome, respectively (Figure 2).
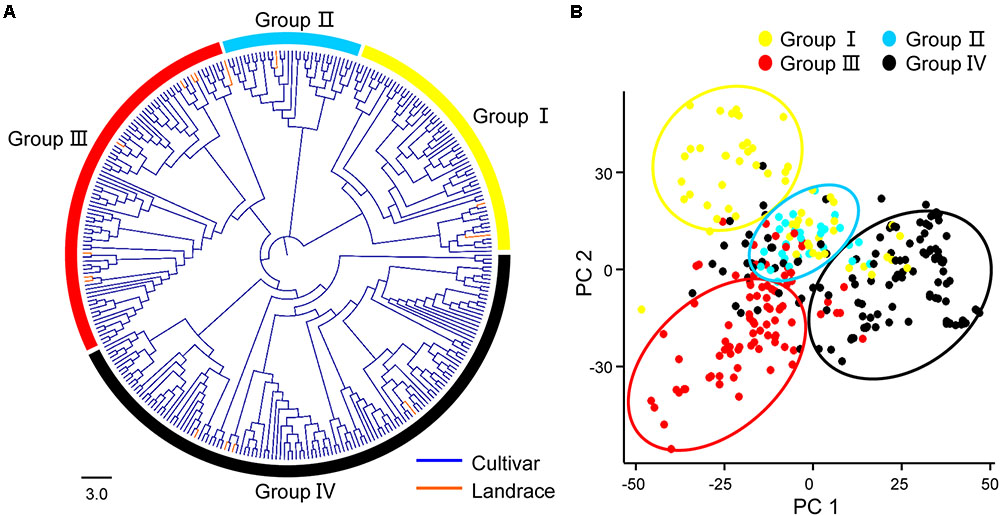
FIGURE 1. Population structure of 319 upland cotton accessions. (A) Neighbor-joining tree of 319 cotton accessions in the panel. Cultivars and Landraces are shown by blue and orange line, respectively. (B) PCA plots of the accessions.
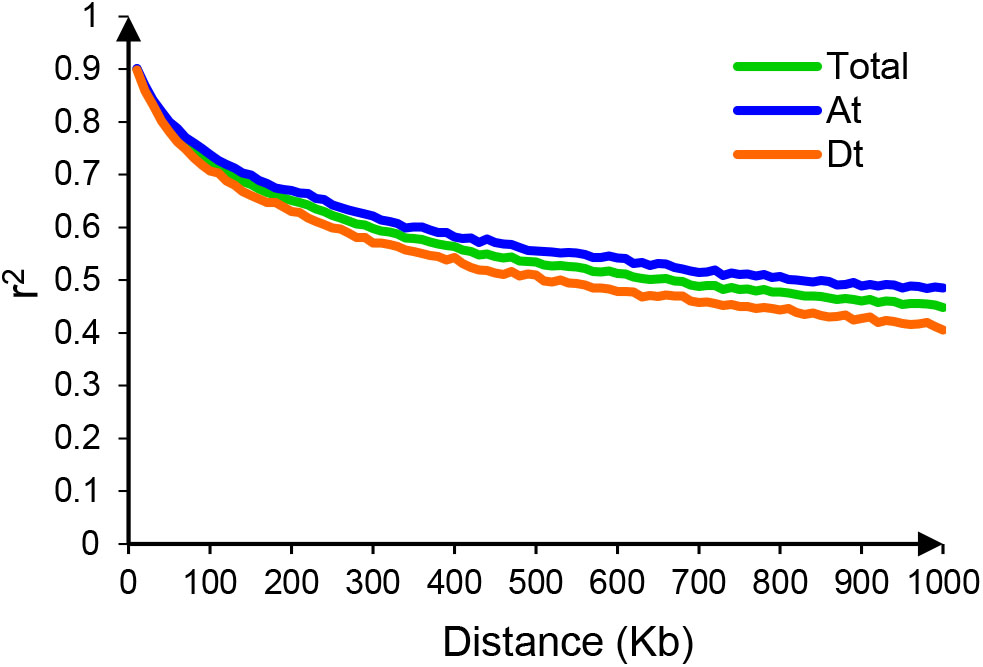
FIGURE 2. Genome-wide linkage disequilibrium (LD) decay in all cotton accessions. Different colors show the LD decay estimated in different subgenomes.
Genome-Wide Association Studies
The GWAS was conducted for the nine traits related to drought tolerance using mrMLM method with Q+K model. Twenty SNPs were found to be significantly associated with six drought tolerance related traits, with both 10 SNPs located on At and Dt subgenome (Table 4). In detail, there were three SNPs located on chromosomes A10, D01, and D13 for RRDM, explaining 22.66% of the total phenotypic variation. For RHL, six SNPs were identified on chromosomes A11, A04, A09, D04, D06, and D09, explaining 50.94% of the total phenotypic variation. Two SNPs for RPC were detected on chromosomes A11 and D07, explaining 11.51% of the total phenotypic variation. Two SNPs for RSS were identified on chromosomes D11 and D12, explaining 40.61% of the total phenotypic variation. Four SNPs for RSDM were identified on chromosomes A03, A05, A06, and D06, explaining 29.85% of the total phenotypic variation. For RPH, three SNPs were identified on chromosomes A05, A08, and D12, explaining 26.08% of the total phenotypic variation. The widespread associated loci in different chromosomes indicated that the genetic basis of drought tolerance is complex.
Candidate Genes Associated With Significant SNPs Region
Candidate genes involved in the 20 significant SNP loci were further mined by referring the LD value in the study. With flanking 500 kb of the significantly associated SNPs and G. hirsutum TM-1 genome (Zhang T. et al., 2015) as reference, 1,326 candidate genes with 630 in At and 696 in Dt subgenome were identified. The number of candidate genes associated with six traits was predicted. We found 398 candidate genes from significant SNP regions associated with RHL, while only 70 candidate genes with RPC (Table 4). These results implied that HL was involved in a complex process regulated by more regulators while the regulation of PC was relatively specific. GO analysis indicated that 1,226 candidate genes could be mapped to GO background in G. hirsutum, which were involved in several biological processes significantly associated with drought stress, such as root system development, regulation of transport, water transport and response to stress. Further, we focused on the GO term “response to stress” (SR), which contained 189 candidate genes (Figure 3A). Of them, many genes had been reported to play important roles in drought tolerance, such as RD2, PIP2, PP2C, and LEA (Aroca et al., 2006; Samota et al., 2017; Baek et al., 2018; Magwanga et al., 2018), and some key transcription factors, WRKY, NAC, and MYB (Wei et al., 2017; Kiranmai et al., 2018; Negi et al., 2018).
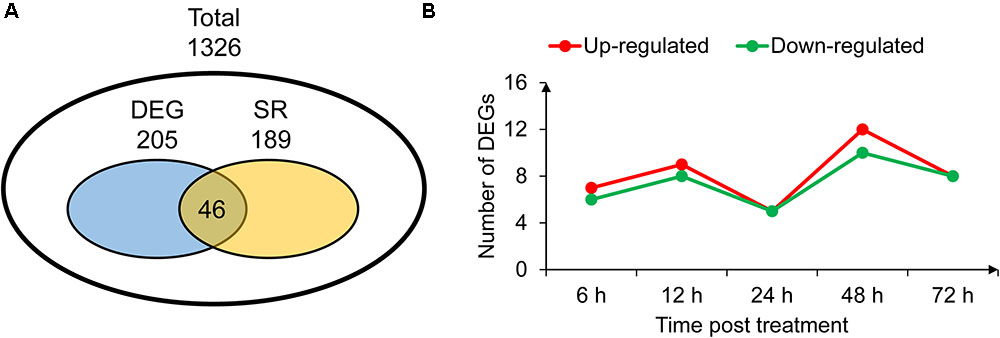
FIGURE 3. Transcriptome analysis and identification of elite alleles. (A) Distribution of the differentially expressed genes (DEGs) and response to stress genes (SR). (B) Statistics of the up-regulated and down-regulated DEGs annotated as response to stress.
Transcriptome Analysis and Identification of Elite Alleles
RNA-seq analysis was performed to further explore elite alleles which contributed to drought tolerance. RNA samples were collected from leaves of G. hirsutum acc. TM-1 at 0, 6, 12, 24, 48, and 72 h post treatment of 15% PEG. In total, 18 separate libraries were generated with three biological replicates of each sample. The reads generated by the Illumina Hiseq2000 were initially processed to remove adapter sequences and low-quality bases. Approximately 0.82 billion valid reads, each 150 nucleotides long, and roughly 40.8 Gb of nucleotides were obtained. We investigated the expression level of all 1,326 candidate genes [log2(RPKM+1) > 1] from GWAS analysis, and found that 205 were differential expression genes (DEGs) with significant induced expression under drought stress condition compared with untreated control. Among these DEGs, 46 were annotated to “response to stress” in GO dataset and other 159 were novel candidate genes (Figure 3A and Supplementary Table S5). For these 46 DEGs, up-regulated genes are more than down-regulated genes after stress-treated time points (Figure 3B). Some up-regulated genes were positively related to stress tolerance such as RD2 (Samota et al., 2017), and a few down-regulated gene were reported to be negatively related to stress tolerance such as PIP2 (Macho et al., 2018). In addition, other 143 candidate genes involved in GO term “response to stress” were not induced by drought stress (Figure 3A and Supplementary Figure S2).
We combined the GWAS and drought-induced RNA-seq data to explore elite alleles involved in drought tolerance. As a result, four genes were further identified for potential drought tolerance. Within the association signal at D12: 3609663 which explaining approximately 19.60% of the phenotypic variation of RSS, we identified 69 candidate genes. The RNA-seq data showed that one of these genes, which encoded a response to desiccation 2 protein and named as RD2 (Gh_D12G0260), was continuously up-regulated in all five time points, especially in 72 h post PEG treatment (Figure 4). Another gene within association signal at D11: 4173831, which explaining approximately 21.01% of the phenotypic variation of RSS, was also continuously up-regulated after PEG treatment. This gene encodes a homeobox-leucine zipper protein, named HAT22 (Gh_D11G0526), which has been reported to be related to plant stress tolerance (Liu et al., 2016) (Figure 5). Within the association signal at D01: 59846909, which explaining approximately 3.27% of the phenotypic variation of RRDM, we identified a gene down-regulated after drought stress, encoding a plasma membrane intrinsic protein 2 (PIP2, Gh_D01G2086), which was involved in root water uptake and tissue hydraulic conductance (Macho et al., 2018) (Figure 6). Another gene within association signal at D04: 10799426, explaining approximately 4.30% of the phenotypic variation of RHL, was also down-regulated after PEG treatment. This gene encodes a protein phosphatase 2C, named PP2C (Gh_D04G0612), which negatively regulate ABA signaling and stress responses (Baek et al., 2018) (Figure 7). In order to validate the reliability of the RNA-seq data, we conducted qPCR assay and confirmed their expression patterns, which were kept consistent in all four candidate genes (Figures 4–7).
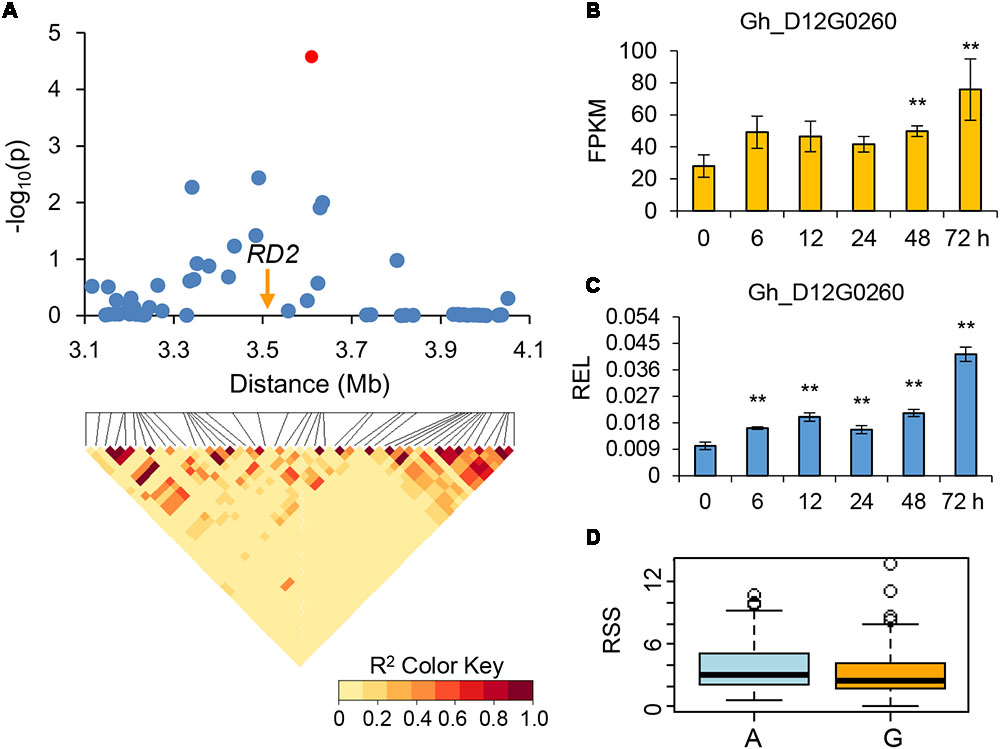
FIGURE 4. Genome-wide association study (GWAS) for drought-tolerance and identification of the candidate gene RD2 on chromosome D12. (A) Local Manhattan plot (top) and LD heat map (bottom). The red dot indicates the SNP related to the drought-tolerance trait. The arrow indicates the location of RD2. (B) The expression level of the candidate gene RD2 calculated via RNA-Seq. (C) The relative expression level (REL) of the candidate gene RD2 calculated via qRT-PCR. (D) Differences of relative soluble sugar content (RSS) among two haplotypes. ∗∗ means the 1% level of significance.
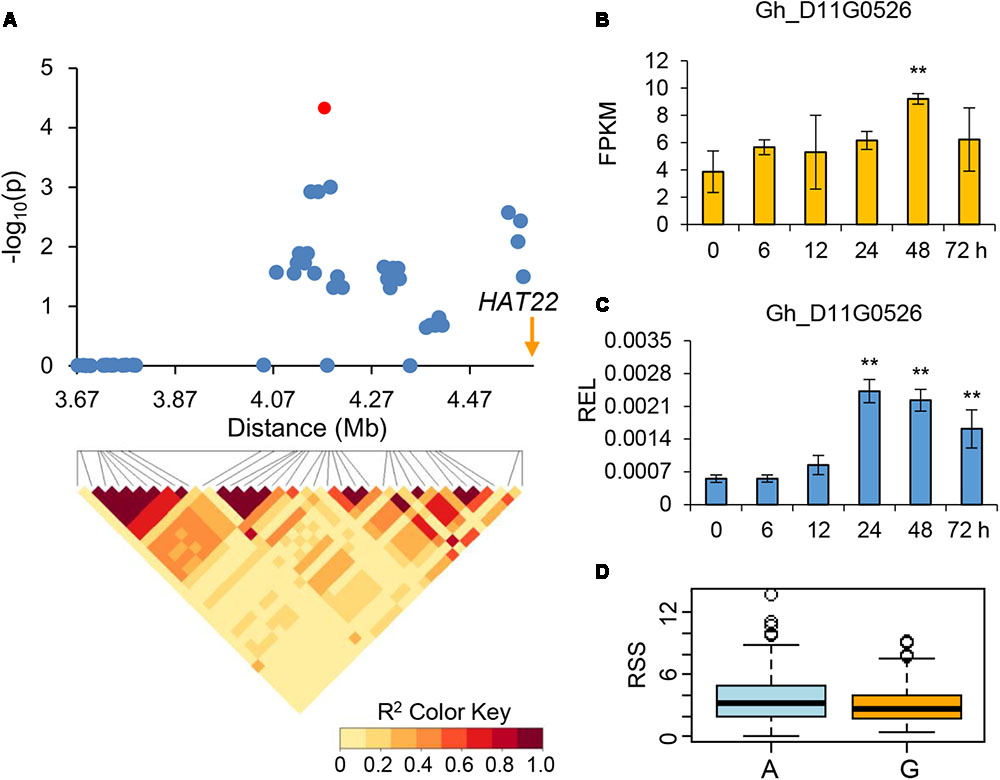
FIGURE 5. Genome-wide association study for drought-tolerance and identification of the candidate gene HAT22 on chromosome D11. (A) Local Manhattan plot (top) and LD heat map (bottom). The red dot indicates the SNP related to the drought-tolerance trait. The arrow indicates the location of HAT22. (B) The expression level of the candidate gene HAT22 calculated via RNA-Seq. (C) The relative expression level (REL) of the candidate gene HAT22 calculated via qRT-PCR. (D) Differences of relative soluble sugar content (RSS) among two haplotypes. ∗∗ means the 1% level of significance.
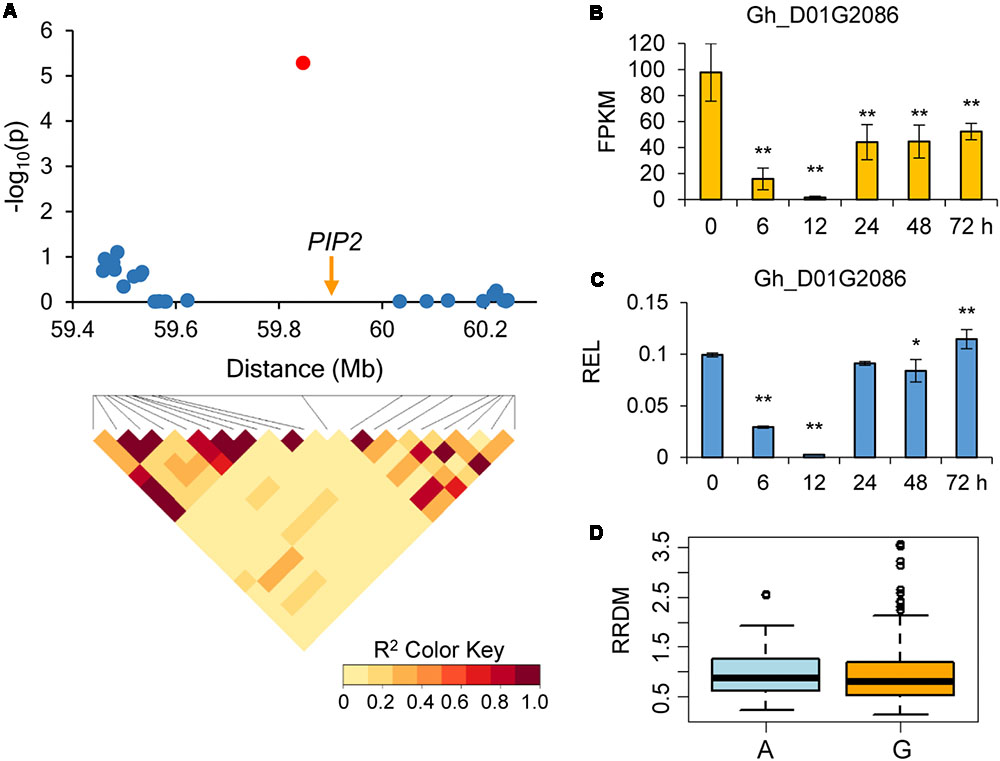
FIGURE 6. Genome-wide association study for drought-tolerance and identification of the candidate gene PIP2 on chromosome D01. (A) Local Manhattan plot (top) and LD heat map (bottom). The red dot indicates the SNP related to the drought-tolerance trait. The arrow indicates the location of PIP2. (B) The expression level of the candidate gene PIP2 calculated via RNA-Seq. (C) The relative expression level (REL) of the candidate gene PIP2 calculated via qRT-PCR. (D) Differences of relative root dry matter (RRDM) among two haplotypes. ∗ and ∗∗ mean the 5% and 1% levels of significance, respectively.
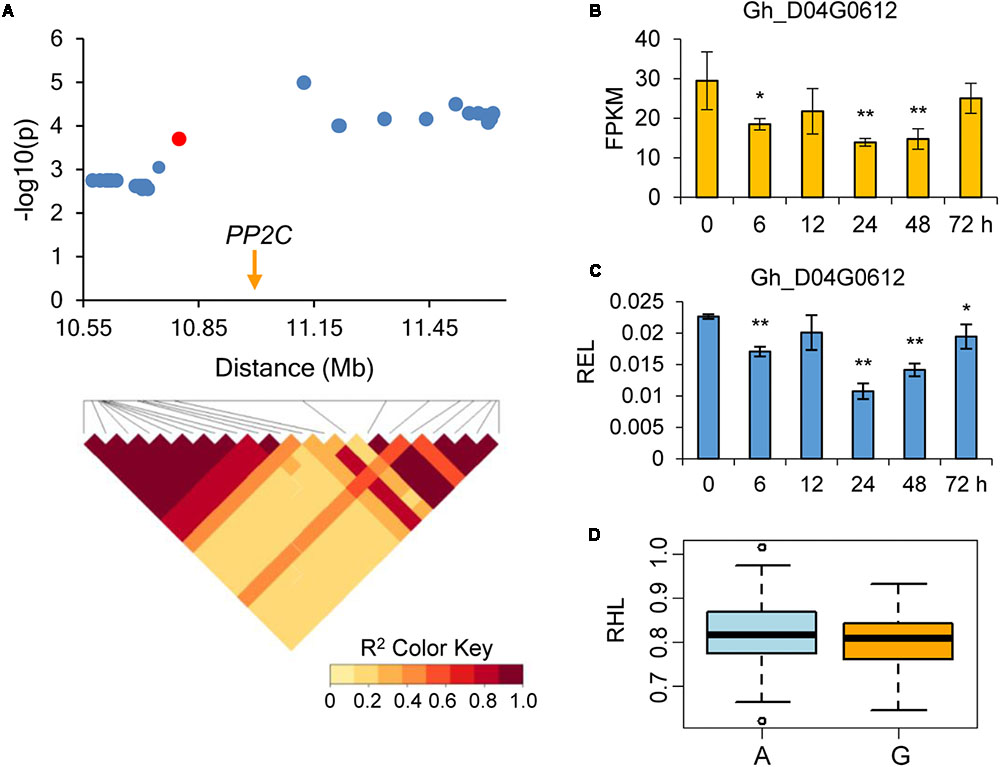
FIGURE 7. Genome-wide association study for drought-tolerance and identification of the candidate gene PP2C on chromosome D04. (A) Local Manhattan plot (top) and LD heat map (bottom). The red dot indicates the SNP related to the drought-tolerance trait. The arrow indicates the location of PP2C. (B) The expression level of the candidate gene PP2C calculated via RNA-Seq. (C) The relative expression level (REL) of the candidate gene PP2C calculated via qRT-PCR. (D) Differences of relative hypocotyl length (RHL) among two haplotypes. ∗ and ∗∗ mean the 5% and 1% levels of significance, respectively.
Discussion
Drought is a serious global problem restricting agricultural development. Previous studies on cotton drought-tolerance mainly pay attention to a single period of cotton development or a small number of indexes or accessions (Chen et al., 2013; Zheng et al., 2014; Ranjan and Sawant, 2015). In this study, 319 upland cotton accessions were collected with a high geographical diversity for genome-wide association studies. A total of nine traits related to drought-tolerance were measured containing two traits at the germinating stage (HL and GP), and seven traits at the seedling stages (PH, SDM, RDM, PC, SOD, MDA, and SS). Proline content has the largest CV, which means that proline content is sensitive to drought stress, and can be regarded as one of the most important trait related to drought tolerance. When suffered drought stress, plants can adapt in three ways: physiological responses such as reducing growth rates, molecular responses such as the increased expression in ABA biosynthetic genes, and biochemical responses such as accumulation of stress metabolites like proline, glutathione, glycinebetaine, polyamines and so on (Fang and Xiong, 2015). Accumulation of proline content, which can increase plant cell’s osmotic pressure and retard plant losing water, is an efficient mechanism to improve drought tolerance. Hypocotyl length associated to the most of SNPs and genes (Table 4), indicates that germinating stage is one of the most sensitive stages of cotton, just like sesame (Li et al., 2018). Based on drought tolerance capacity with different F factors, cluster analysis grouped the 319 upland cotton accessions as four types: 16 advanced drought-tolerant accessions, 75 medium drought-tolerant accessions, 207 drought-sensitive accessions and 21 extremly drought-sensitive accessions. In our previous report for salt-tolerance of 304 upland cotton accessions, we detected that 43 accessions were advanced salt-tolerance, 114 medium salt-tolerance, and 147 salt-sensitive (Du et al., 2016). Compared to the salt tolerance, there were relatively few drought-tolerant accessions and need to be further improved for drought tolerance in cotton breeding.
On the basis of the phylogenetic and PCA analysis, we classified the 319 accessions into four groups. However, it showed no obvious relationship with their geographic origin and this result was consistent with most previous study in Upland cotton (Cai et al., 2017; Sun et al., 2017). LD analysis of upland cotton in this study showed that the LD rate declining to half its maximum value was 980 Kb, it is longer than most other crops, such as rice (167 Kb) (Huang et al., 2012) and soybean (420 Kb) (Zhou et al., 2015). The speed of LD decay determines the capacity and resolution of marker-trait association mapping, and the causes of LD mainly including mutation, population bottlenecks, founder effects, drift, selection, migration and population admixture (Morrell et al., 2005; Mackay and Powell, 2007). We speculated that the short history and relatively high rate of self-fertilization of upland cotton breeding in China led to the slower LD decay, implying the more narrow genetic diversity of upland cotton accessions.
Drought tolerance is a complex trait and is regulated by polygenes with small effect. Common GWAS methods are all based on a fixed-SNP-effect mixed linear model (MLM) and single marker analysis, which require Bonferroni correction for multiple tests. When the number of markers is extremely large, the test is too strict. In cotton, combined the resequenced SNP data and phenotyping variation data, GWAS was performed using EMMAX method and the significance threshold was estimated as approximately P = 10-6. As a result, three loci associated with resistance to Verticillium wilt were identified (Fang et al., 2017), implying that mixed linear model with single marker analysis is too strict in GWAS for complex trait such as disease resistance. Multi-locus mixed linear model compresses the markers by the rMLM method and used the selected SNPs to further associate with traits by mrMLM method. Also due to the multi-locus nature, no multiple test correction is needed. So it shows the good effect for complex traits. Here, we used mrMLM of Wang S.B. et al. (2016) and the CottonSNP80K array, genome-wide association studies of drought-tolerance traits with natural population of upland cotton accessions were conducted, and 20 QTNs for drought-tolerance traits were identified. These associated loci were widely distributed across the entire genome and the candidate genes around the loci involved in many biological process, such as root system development, regulation of transport, water transport and response to stress. It demonstrates that drought stress response is controlled by multiple loci and numerous genes.
Compared to yield and fiber quality traits of cotton (Fang et al., 2017; Sun et al., 2017; Ma et al., 2018), the number of reported loci associated with drought tolerance is much fewer. Previous studies have identified several drought-tolerance QTLs in cotton. Based on the progenies from the cross of G. hirsutum cv. Siv’on and G. barbadense cv. F-177, a total of 33 QTLs were identified under water-limited environments, including five QTLs for different physiological traits, 11 for plant productivity and 17 for fiber quality, respectively (Saranga et al., 2001). In another study, a vast number of QTLs from 42 different studies were surveyed by comprehensive meta QTL analysis, including 132 QTLs for fiber strength, 26 for boll weight, six for gossypol, four for fruiting banch number, five for osmotic potential, three for chlorophyll and so on (Said et al., 2013). However, compared to the GWAS analysis based on high density SNPs, the low density markers and the wide QTL regions showed limitation in identification and utilization of elite genes, especially for marker-assisted selection (MAS) breeding (Zhou et al., 2015). The present study makes progresses in revealing loci related to drought-tolerance traits and identifying SNP loci and candidate genes for drought tolerance. In other studies, using 240 maize accessions and high-density markers, 61 significant SNPs related to drought tolerance were detected by GWAS analysis (Thirunavukkarasu et al., 2014). In rice, through integrating 175 upland rice accessions with 150,325 SNPs, 13 SNP markers and 50 genes associated with yield under drought conditions were identified, further, 10 genes related to drought and abiotic stress tolerance were verified (Pantalião et al., 2016). These studies could be exploited to discover drought tolerance mechanism and contribute to breeding the drought tolerance varieties in crops.
Genetic basis of drought tolerance is complex. Previous studies have reported many genes that are responsive to drought stress in many plants (Song X. et al., 2016; Wang N. et al., 2016; Ma et al., 2017). However, it is difficult to identify candidate genes from the enormous gene pool. In present study, we performed GWAS to identify elite QTNs in natural population and further screen candidate genes by combining with RNA-seq data. As a result, 46 candidate genes with both annotated as response to stress and differential expression under drought stress were selected. Of these genes, RD2, encoding a response to desiccation protein, was a key gene for cotton drought tolerance. In rice, expression analyses showed that both RD1 and RD2 genes up-regulated under drought stress due to seed-priming, and RD2 was increased more significantly than RD1 in tolerance to drought stress, especially on priming with paclobutrazol in drought-tolerant plant and with salicylic acid in drought-sensitive plant (Samota et al., 2017). Another candidate gene HAT22, which also named ABIG1 and encoded a homeobox protein, is a member of the HD-Zip II family. Expression of HAT22 mRNA increased under drought and ABA treatment. There was less leaf yellowing in HAT22 mutants than wild type plants with drought conditions. Moreover, some stress related genes such as ABA and ethylene response loci were regulated by HAT22 (Liu et al., 2016). In the plasma membrane, PIPs are the most plentiful aquaporins with two types, PIP1 and PIP2. There are five and eight isoforms of PIP1 and PIP2 in Arabidopsis thaliana, respectively. Generally, PIP1 proteins behave a low efficiency for water transport while PIP2 proteins in plant have a high capacity for water transport (Maurel et al., 2008). Previous studies showed that PP2Cs played a crucial role in regulation of signal transduction pathways. PP2Cs are negative regulators of stress-induced MAPK pathways, ABA signaling and receptor kinase signaling. In turn, expression of PP2C was transcriptionally controlled by developmental signals, ABA and stress response (Schweighofer et al., 2004). Abscisic acid (ABA) is an important plant hormone, and regulates plant development and resistance to biotic and abiotic stresses. It controls transpirational water loss by regulating the stomatal opening and closure to resist drought stress. Moreover, ABA can increase plant cell’s osmotic pressure, and expedite chlorophyll breakdown and leaf senescence (Wilkinson and Davies, 2002). By detecting the 46 candidate genes, we found most of them involved in ABA signal pathway and were reported to be related to drought response, such as PIP2, HK1, GOLS1, and ADC2 (Zhai et al., 2010; Héricourt et al., 2016; Song C. et al., 2016; Macho et al., 2018), indicating ABA signal pathway play crucial roles in response to drought tolerance in cotton. In summary, identification of more drought-tolerance related genes/QTLs enlarges new insight into mechanisms of drought response, and high-throughput genotyping platforms are powerful tools for complex traits dissection and development of drought tolerance varieties in future cotton breeding.
Conclusion
We genotyped 319 upland cotton accessions using the CottonSNP80K array and phenotyped nine drought-tolerance related traits. By SNP-trait GWAS, we identified 20 SNPs significantly associated with drought-tolerance traits. Integrating the GWAS and RNA-seq data with qRT-PCR verification, we identified four candidate genes RD2, HAT22, PIP2, and PP2C for improving drought stress. Our study provides valuable information to explore molecular mechanisms underlying cotton drought tolerance, and supplies new resource for the improvement of drought-tolerance in future cotton breeding efforts.
Author Contributions
WG conceived the study. SH and YL contributed to phenotyping investigation. GZ and WL performed the GWAS and RNA-Seq analysis. SH, JF, EN, LL, and DZ contributed to the experimental data analysis and discussion. SH, GZ, DZ, and WG wrote the manuscript. All authors read and approved the manuscript.
Funding
This program was financially supported in part by the Fundamental Research Funds for the Central Universities (KYYJ201601 and KYYJ201701), six talent peaks project in Jiangsu province (2015-NY-002), Qing Lan Project for Science and Technology Innovation Team in Jiangsu Province (No. 6), and Jiangsu Collaborative Innovation Center for Modern Crop Production project (No. 10).
Conflict of Interest Statement
The authors declare that the research was conducted in the absence of any commercial or financial relationships that could be construed as a potential conflict of interest.
The reviewer ZL and handling Editor declared their shared affiliation at time of review.
Supplementary Material
The Supplementary Material for this article can be found online at: https://www.frontiersin.org/articles/10.3389/fpls.2018.01276/full#supplementary-material
FIGURE S1 | Phenotypic distributions for drought-tolerance traits. RPH, relative plant height; RSDM, relative shoot dry matter; RRDM, relative root dry matter; RPC, relative proline content; RSOD, relative superoxide dismutase activity; RMDA, relative malonaldehyde content; RSS, relative soluble sugar content; RHL, relative hypocotyl length; RGP, relative germination percentage. Gaussian curve is shown in orange line for each trait.
FIGURE S2 | Expression pattern of the candidate genes involved in drought response. (A) Genes were annotated as stress response by Go analysis and also differential expression under drought stress. (B) Genes were not annotated as stress response by Go analysis but differential expression under drought stress. (C) Genes were annotated as stress response but not induced expression under drought stress. The scales represent the fold change value of gene expression level.
TABLE S1 | Information on 319 cotton accessions used in this study.
TABLE S2 | Primers used in this study.
TABLE S3 | Eigenvalues of six principal components and their contribution and accumulative contribution.
TABLE S4 | Loading matrix of each in six principal components.
TABLE S5 | Information on 46 candidate genes related to stress response with significantly differential expression under drought stress.
Footnotes
- ^http://www.spss.com.cn/
- ^http://www.cog-genomics.org/plink2/
- ^https://tassel.bitbucket.io/
- ^http://tree.bio.ed.ac.uk/
- ^http://bioinfo.cau.edu.cn/agriGO/analysis.php
- ^http://www.lifetechnologies.com
References
Aroca, R., Ferrante, A., Vernieri, P., and Chrispeels, M. J. (2006). Drought, abscisic acid and transpiration rate effects on the regulation of PIP aquaporin gene expression and abundance in Phaseolus vulgaris plants. Ann. Bot. 98, 1301–1310. doi: 10.1093/aob/mcl219
Ashraf, J., Zuo, D., Wang, Q., Malik, W., Zhang, Y., Abid, M. A., et al. (2018). Recent insights into cotton functional genomics: progress and future perspectives. Plant Biotechnol. J. 16, 699–713. doi: 10.1111/pbi.12856
Baek, W., Lim, C. W., and Lee, S. C. (2018). A DEAD-box RNA helicase, RH8, is critical for regulation of ABA signaling and the drought stress response via inhibition of PP2CA activity. Plant Cell Environ. 41, 1593-1604. doi: 10.1111/pce.13200
Bates, L., Waldren, R., and Teare, I. (1973). Rapid determination of free proline for water-stress studies. Plant Soil 39, 205–207. doi: 10.1007/BF00018060
Beyer, W., and Fridovich, I. (1987). Assaying for superoxide dismutase activity: some large consequences of minor changes in conditions. Anal. Biochem. 161, 559–566. doi: 10.1016/0003-2697(87)90489-1
Cai, C., Zhu, G., Zhang, T., and Guo, W. (2017). High-density 80 K SNP array is a powerful tool for genotyping G. hirsutum accessions and genome analysis. BMC Genomics 18:654. doi: 10.1186/s12864-017-4062-2
Chen, T., Li, W., Hu, X., Guo, J., Liu, A., and Zhang, B. (2015). A cotton MYB transcription factor, GbMYB5, is positively involved in plant adaptive response to drought stress. Plant Cell Physiol. 56, 917–929. doi: 10.1093/pcp/pcv019
Chen, Y. (2013). Analyzing blends of herbivore-induced volatile organic compounds with factor analysis: revisiting “cotton plant, Gossypium hirsutum L., defense in response to nitrogen fertilization”. J. Econ. Entomol. 106, 1053–1057. doi: 10.1603/EC12178
Chen, Y., Liu, Z., Feng, L., Zheng, Y., Li, D., and Li, X. (2013). Genome-wide functional analysis of cotton (Gossypium hirsutum) in response to drought. PLoS One 8:e80879. doi: 10.1371/journal.pone.0080879
Du, L., Cai, C., Wu, S., Zhang, F., Hou, S., and Guo, W. (2016). Evaluation and exploration of favorable qtl alleles for salt stress related traits in cotton. PLoS One 11:e0151076. doi: 10.1371/journal.pone.0151076
Fang, D., Jenkins, J., Deng, D., McCarty, J., Li, P., and Wu, J. (2014). Quantitative trait loci analysis of fiber quality traits using a random-mated recombinant inbred population in Upland cotton (Gossypium hirsutum L.). BMC Genomics 15:397. doi: 10.1186/1471-2164-15-397
Fang, L., Wang, Q., Hu, Y., Jia, Y., Chen, J., Liu, B., et al. (2017). Genomic analyses in cotton identify signatures of selection and loci associated with fiber quality and yield traits. Nat. Genet. 49, 1089–1098. doi: 10.1038/ng.3887
Fang, Y., and Xiong, L. (2015). General mechanisms of drought response and their application in drought resistance improvement in plants. Cell Mol. Life Sci. 72, 673–689. doi: 10.1007/s00018-014-1767-0
Gunapati, S., Naresh, R., Ranjan, S., Nigam, D., Hans, A., Verma, P. C., et al. (2016). Expression of GhNAC2 from G. herbaceum, improves root growth and imparts tolerance to drought in transgenic cotton and Arabidopsis. Sci. Rep. 6:24978. doi: 10.1038/srep24978
Gutierrez, L., Mauriat, M., Guénin, S., Pelloux, J., Lefebvre, J. F., Louvet, R., et al. (2008). The lack of a systematic validation of reference genes: a serious pitfall undervalued in reverse transcription-polymerase chain reaction (RT-PCR) analysis in plants. Plant Biotechnol. J. 6, 609–618. doi: 10.1111/j.1467-7652.2008.00346.x
Héricourt, F., Chefdor, F., Djeghdir, I., Larcher, M., Lafontaine, F., Courdavault, V., et al. (2016). Functional divergence of poplar histidine-aspartate kinase HK1 paralogs in response to osmotic stress. Int. J. Mol. Sci. 17:2061. doi: 10.3390/ijms17122061
Hoagland, D., and Arnon, D. (1950). The water culture method for growing plants without soil. Calif. Agric. Exp. Stn. Circ. 347, 357–359.
Hodges, D., Forney, C., Prange, R., and DeLong, J. (1999). Improving the thiobarbituric acid-reactive-substances assay for estimating lipid peroxidation in plant tissues containing anthocyanin and other interfering compounds. Planta 207, 604–611. doi: 10.1017/S0031182002002585
Huang, C., Nie, X., Shen, C., You, C., Li, W., Zhao, W., et al. (2017). Population structure and genetic basis of the agronomic traits of upland cotton in China revealed by a genomewide association study using high-density SNPs. Plant Biotechnol. J. 15, 1374–1386. doi: 10.1111/pbi.12722
Huang, X., Kurata, N., Wei, X., Wang, Z. X., Wang, A., Zhao, Q., et al. (2012). A map of rice genome variation reveals the origin of cultivated rice. Nature 490, 497–501. doi: 10.1038/nature11532
Huang, X., Wei, X., Sang, T., Zhao, Q., Feng, Q., Zhao, Y. et al. (2010). Genome-wide association studies of 14 agronomic traits in rice landraces. Nat. Genet. 42, 961–967. doi: 10.1038/ng.695
Hulse-Kemp, A. M., Lemm, J., Plieske, J., Ashrafi, H., Buyyarapu, R., Fang, D. D., et al. (2015). Development of a 63K SNP array for cotton and high-density mapping of intraspecific and interspecific populations of Gossypium spp. G3 5, 1187–1209. doi: 10.1534/g3.115.018416
Kiranmai, K., Lokanadha Rao, G., Pandurangaiah, M., Nareshkumar, A., Amaranatha, Reddy, V., Lokesh, U., et al. (2018). A novel WRKY transcription factor, MuWRKY3 (Macrotyloma uniflorum Lam. Verdc.) enhances drought stress tolerance in transgenic Groundnut (Arachis hypogaea L.) Plants. Front. Plant Sci. 9:346. doi: 10.3389/fpls.2018.00346
Levi, A., Paterson, A., Barak, V., Yakir, D., Wang, B., Chee, P., et al. (2009). Field evaluation of cotton near-isogenic lines introgressed with QTLs for productivity and drought related traits. Mol. Breed. 23, 179–195. doi: 10.1007/s11032-008-9224-0
Li, C., Wang, X., Dong, N., Zhao, H., Xia, Z., Wang, R., et al. (2013). QTL analysis for early-maturing traits in cotton using two upland cotton (Gossypium hirsutum L.) crosses. Breed. Sci. 63, 154–163. doi: 10.1270/jsbbs.63.154
Li, H., Peng, Z. Y., Yang, X. H., Wang, W. D., Fu, J. J., Wang, J. H., et al. (2013). Genome-wide association study dissects the genetic architecture of oil biosynthesis in maize kernels. Nat. Genet. 45, 43–50. doi: 10.1038/ng.2484
Li, D., Dossa, K., Zhang, Y., Wei, X., Wang, L., Zhang, Y., et al. (2018). GWAS uncovers differential genetic bases for drought and salt tolerances in sesame at the germination stage. Genes 9:87 doi: 10.3390/genes9020087
Li, F., Fan, G., Lu, C., Xiao, G., Zou, C., Kohel, R. J., et al. (2015). Genome sequence of cultivated Upland cotton (Gossypium hirsutum TM-1) provides insights into genome evolution. Nat. Biotechnol. 33, 524–530. doi: 10.1038/nbt.3208
Li, F., Li, M., Wang, P., Cox, K. L. Jr., Duan, L., Dever, J. K., et al. (2017). Regulation of cotton (Gossypium hirsutum) drought responses by mitogen-activated protein (MAP) kinase cascade-mediated phosphorylation of GhWRKY59. New Phytol. 215, 1462–1475. doi: 10.1111/nph.14680
Liu, T., Longhurst, A. D., Talavera-Rauh, F., Hokin, S. A., and Barton, M. K. (2016). The Arabidopsis transcription factor ABIG1 relays ABA signaled growth inhibition and drought induced senescence. eLife 5:e13768. doi: 10.7554/eLife.13768.
Livak, K. J., and Schmittgen, T. D. (2001). Analysis of relative gene expression data using real-time quantitative PCR and the 2(-Delta Delta C(T)) Method. Methods 25, 402–408. doi: 10.1006/meth.2001.1262
Ma, H., Chen, J., Zhang, Z., Ma, L., Yang, Z., Zhang, Q., et al. (2017). MAPK kinase 10.2 promotes disease resistance and drought tolerance by activating different MAPKs in rice. Plant J. 92, 557–570. doi: 10.1111/tpj.13674
Ma, Z. Y., He, S. P., Wang, X. F., Sun, J. L., Zhang, Y., Zhang, G. Y., et al. (2018). Resequencing a core collection of upland cotton identifies genomic variation and loci influencing fiber quality and yield. Nat. Genet. 50, 803–813. doi: 10.1038/s41588-018-0119-7
Macho, R. M., Herrera, R. M., Brejcha, R., Schäffner, A. R., Tanaka, N., Fujiwara, T., et al. (2018). Boron toxicity reduces water transport from root to shoot in Arabidopsis plants. Evidence for a reduced transpiration rate and expression of major pip aquaporin genes. Plant Cell Physiol. 59, 836–844. doi: 10.1093/pcp/pcy026
Mackay, I., and Powell, W. (2007). Methods for linkage disequilibrium mapping in crops. Trends Plant Sci. 12, 57–63. doi: 10.1016/j.tplants.2006.12.001
Magwanga, R. O., Lu, P., Kirungu, J. N., Lu, H., Wang, X., Cai, X., et al. (2018). Characterization of the late embryogenesis abundant (LEA) proteins family and their role in drought stress tolerance in upland cotton. BMC Genet. 19:6. doi: 10.1186/s12863-017-0596-1
Maurel, C., Verdoucq, L., Luu, D., and Santoni, V. (2008). Plant aquaporins: membrane channels with multiple integrated functions. Annu. Rev. Plant Biol. 59, 595–624. doi: 10.1146/annurev.arplant.59.032607.092734
Morrell, P. L., Toleno, D. M., Lundy, K. E., and Clegg, M. T. (2005). Low levels of linkage disequilibrium in wild barley (Hordeum vulgare ssp. spontaneum) despite high rates of self-fertilization. Proc. Natl. Acad. Sci. U.S.A. 102, 2442–2447. doi: 10.1073/pnas.0409804102
Negi, S., Tak, H., and Ganapathi, T. R. (2018). A banana NAC transcription factor (MusaSNAC1) impart drought tolerance by modulating stomatal closure and H2O2 content. Plant Mol. Biol. 96, 457–471. doi: 10.1007/s11103-018-0710-4
Odjegba, V., and Fasidi, I. (2006). Effects of heavy metals on some proximate composition of Eichhornia crassipes. J. Appl. Sci. Environ. Mgt. 10, 83–87. doi: 10.4314/jasem.v10i1.17309
Pantalião, G., Narciso, M., Guimarães, C., Castro, A., Colombari, J., Breseghello, F., et al. (2016). Genome wide association study (GWAS) for grain yield in rice cultivated under water deficit. Genetica 144, 651–664. doi: 10.1007/s10709-016-9932-z
Patel, R. K., and Jain, M. (2012). NGS QC Toolkit: a toolkit for quality control of next generation sequencing data. PLoS One 7:e30619. doi: 10.1371/journal.pone.0030619
Paterson, A. H., Brubaker, C. L., and Wendel, J. F. (1993). A rapid method for extraction of cotton (Gossypium spp.) genomic DNA suitable for RFLP or PCR analysis. Plant Mol. Biol. Rep. 11, 122–127. doi: 10.1007/BF02670470
Pettigrew, W. T. (2004). Physiological consequences of moisture deficit stress in cotton. Crop Sci. 44, 1265–1272. doi: 10.2135/cropsci2004.1265
Provenzano, M., and Mocellin, S. (2007). Complementary techniques: validation of gene expression data by quantitative real time PCR. Adv. Exp. Med. Biol. 593, 66–73. doi: 10.1007/978-0-387-39978-2_7
Ranjan, A., and Sawant, S. (2015). Genome-wide transcriptomic comparison of cotton (Gossypium herbaceum) leaf and root under drought stress. 3Biotech 5, 585–596. doi: 10.1007/s13205-014-0257-2
Said, J. I., Lin, Z., Zhang, X., Song, M., and Zhang, J. (2013). A comprehensive meta QTL analysis for fiber quality, yield, yield related and morphological traits, drought tolerance, and disease resistance in tetraploid cotton. BMC Genomics 14:776. doi: 10.1186/1471-2164-14-776
Saleem, M., Malik, T., Shakeel, A., and Ashraf, M. (2015). QTL mapping for some important drought tolerant traits in upland cotton. J. Anim. Plant Sci. 25, 502–509.
Samota, M. K., Sasi, M., Awana, M., Yadav, O. P., Amitha Mithra, S. V., Tyagi, A., et al. (2017). Elicitor-induced biochemical and molecular manifestations to improve drought tolerance in rice (Oryza Sativa L.) through seed-priming. Front. Plant Sci. 8:934. doi: 10.3389/fpls.2017.00934
Saranga, Y., Menz, M., Jiang, C., Wright, R., Yakir, D., and Paterson, A. (2001). Genomic dissection of genotype x environment interactions conferring adaptation of cotton to arid conditions. Genom. Res. 11, 1988–1995. doi: 10.1101/gr.157201
Schweighofer, A., Hirt, H., and Meskiene, I. (2004). Plant PP2C phosphatases: emerging functions in stress signaling. Trends Plant Sci. 9, 236–243. doi: 10.1016/j.tplants.2004.03.007
Song, C., Chung, W., and Lim, C. (2016). Overexpression of heat shock factor gene HsfA3 increases galactinol levels and oxidative stress tolerance in Arabidopsis. Mol. Cells 39, 477–483. doi: 10.14348/molcells.2016.0027
Song, X., Zhang, Y., Wu, F., and Zhang, L. (2016). Genome-wide analysis of the maize (Zea may L.) CPP-like gene family and expression profiling under abiotic stress. Genet. Mol. Res. 15:gmr.15038023. doi: 10.4238/gmr.15038023
Sun, Z., Wang, X., Liu, Z., Gu, Q., Zhang, Y., Li, Z., et al. (2017). Genome-wide association study discovered genetic variation and candidate genes of fibre quality traits in Gossypium hirsutum L. Plant Biotechnol. J. 15, 982–996. doi: 10.1111/pbi.12693
Thirunavukkarasu, N., Hossain, F., Arora, K., Sharma, R., Shiriga, K., Mittal, S., et al. (2014). Functional mechanisms of drought tolerance in subtropical maize (Zea mays L.) identified using genome-wide association mapping. BMC Genomics 15:1182. doi: 10.1186/1471-2164-15-1182
Trapnell, C., Pachter, L., and Salzberg, S. L. (2009). TopHat: discovering splice junctions with RNA-Seq. Bioinformatics 25, 1105–1111. doi: 10.1093/bioinformatics/btp120
Trapnell, C., Roberts, A., Goff, L., Pertea, G., Kim, D., Kelley, D. R., et al. (2012). Differential gene and transcript expression analysis of RNA-seq experiments with TopHat and Cufflinks. Nat. Protoc. 7, 562–578. doi: 10.1038/nprot.2012.016
Wang, M., Li, C., and Wang, Q. (2014). Quantitative trait loci mapping and genetic dissection for lint percentage in upland cotton (Gossypium hirsutum). J. Genet. 93, 371–378. doi: 10.1007/s12041-014-0385-9
Wang, M., Tu, L., Lin, M., Lin, Z., Wang, P., Yang, Q., et al. (2017). Asymmetric subgenome selection and cis-regulatory divergence during cotton domestication. Nat. Genet. 49, 579–587. doi: 10.1038/ng.3807
Wang, N., Liu, Y., Cong, Y., Wang, T., Zhong, X., Yang, S., et al. (2016). Genome-wide identification of soybean U-box E3 ubiquitin ligases and roles of GmPUB8 in negative regulation of drought stress response in Arabidopsis. Plant Cell Physiol. 28, 72–88. doi: 10.1093/pcp/pcw068
Wang, S. B., Feng, J. Y., Ren, W. L., Huang, B., Zhou, L., Wen, Y. J., et al. (2016). Improving power and accuracy of genome-wide association studies via a multi-locus mixed linear model methodology. Sci. Rep. 6:19444. doi: 10.1038/srep19444
Wei, Q., Zhang, F., Sun, F., Luo, Q., Wang, R., Hu, R., et al. (2017). A wheat MYB transcriptional repressor TaMyb1D regulates phenylpropanoid metabolism and enhances tolerance to drought and oxidative stresses in transgenic tobacco plants. Plant Sci. 265, 112–123. doi: 10.1016/j.plantsci.2017.09.020
Wilkinson, S., and Davies, W. (2002). ABA-based chemical signaling: the co-ordination of responses to stress in plants. Plant Cell Environ. 25, 195-210. doi: 10.1046/j.0016-8025.2001.00824.x
Yan, H., Jia, H., Chen, X., Hao, L., An, H., and Guo, X. (2014). The cotton WRKY transcription factor GhWRKY17 functions in drought and salt stress in transgenic Nicotiana benthamiana through ABA signaling and the modulation of reactive oxygen species production. Plant Cell Physiol. 55, 2060–2076. doi: 10.1093/pcp/pcu133
Yang, N., Lu, Y. L., Yang, X. H., Huang, J., Zhou, Y., Ali, F., et al. (2014). Genome wide association studies using a new nonparametric model reveal the genetic architecture of 17 agronomic traits in an enlarged maize association panel. PLoS Genet. 10:e1004573. doi: 10.1371/journal.pgen.1004573
Yano, K., Yamamoto, E., Aya, K., Takeuchi, H., Lo, P. C., Hu, L., et al. (2016). Genome-wide association study using whole-genome sequencing rapidly identifies new genes influencing agronomic traits in rice. Nat. Genet. 48, 927–934. doi: 10.1038/ng.3596
Zhai, H., Bai, X., Zhu, Y., Li, Y., Cai, H., Ji, W., et al. (2010). A single-repeat R3-MYB transcription factor MYBC1 negatively regulates freezing tolerance in Arabidopsis. Biochem. Biophys. Res. Commun. 394, 1018–1023. doi: 10.1016/j.bbrc.2010.03.114
Zhang, T., Hu, Y., Jiang, W., Fang, L., Guan, X., Chen, J., et al. (2015). Sequencing of allotetraploid cotton (Gossypium hirsutum L. acc. TM-1) provides a resource for fiber improvement. Nat. Biotechnol. 33, 531–537. doi: 10.1038/nbt.3207
Zhang, J., Singh, A., Mueller, D. S., and Singh, A. K. (2015). Genome-wide association and epistasis studies unravel the genetic architecture of sudden death syndrome resistance in soybean. Plant J. 84, 1124–1136. doi: 10.1111/tpj.13069
Zheng, J., Oluoch, G., Riaz Khan, M. K., Wang, X., Cai, X., Zhou, Z., et al. (2016). Mapping QTLs for drought tolerance in an F2:3 population from an inter-specific cross between Gossypium tomentosum and Gossypium hirsutum. Genet. Mol. Res. 15 gmr.15038477. doi: 10.4238/gmr.15038477.
Zheng, M., Meng, Y., Yang, C., Zhou, Z., Wang, Y., and Chen, B. (2014). Protein expression changes during cotton fiber elongation in response to drought stress and recovery. Proteomics 14, 1776–1795. doi: 10.1002/pmic.201300123
Keywords: upland cotton, drought stress, genome-wide association study, single nucleotide polymorphism (SNP), RNA-sequencing
Citation: Hou S, Zhu G, Li Y, Li W, Fu J, Niu E, Li L, Zhang D and Guo W (2018) Genome-Wide Association Studies Reveal Genetic Variation and Candidate Genes of Drought Stress Related Traits in Cotton (Gossypium hirsutum L.). Front. Plant Sci. 9:1276. doi: 10.3389/fpls.2018.01276
Received: 10 May 2018; Accepted: 14 August 2018;
Published: 03 September 2018.
Edited by:
Yuan-Ming Zhang, Huazhong Agricultural University, ChinaReviewed by:
Zhongxu Lin, Huazhong Agricultural University, ChinaBaohong Zhang, East Carolina University, United States
Copyright © 2018 Hou, Zhu, Li, Li, Fu, Niu, Li, Zhang and Guo. This is an open-access article distributed under the terms of the Creative Commons Attribution License (CC BY). The use, distribution or reproduction in other forums is permitted, provided the original author(s) and the copyright owner(s) are credited and that the original publication in this journal is cited, in accordance with accepted academic practice. No use, distribution or reproduction is permitted which does not comply with these terms.
*Correspondence: Wangzhen Guo, moelab@njau.edu.cn