- 1College of Plant Science and Technology, Huazhong Agricultural University, Wuhan, China
- 2National Key Laboratory of Crop Genetic Improvement, National Center of Plant Gene Research, Huazhong Agricultural University, Wuhan, China
- 3School of Chemical Science and Engineering, Royal Institute of Technology, Stockholm, Sweden
- 4Institute of Crop Science, National Wheat Improvement Center, Chinese Academy of Agricultural Sciences, Beijing, China
Genome-wide association studies (GWAS) have been widely used to dissect the complex biosynthetic processes of plant metabolome. Most studies have used single-locus GWAS approaches, such as mixed linear model (MLM), and little is known about more efficient algorithms to implement multi-locus GWAS. Here, we report a comprehensive GWAS of 20 free amino acid (FAA) levels in kernels of bread wheat (Triticum aestivum L.) based on 14,646 SNPs by six multi-locus models (FASTmrEMMA, FASTmrMLM, ISISEM-BLASSO, mrMLM, pKWmEB, and pLARmEB). Our results showed that 328 significant quantitative trait nucleotides (QTNs) were identified in total (38, 8, 92, 45, 117, and 28, respectively, for the above six models). Among them, 66 were repeatedly detected by more than two models, and 155 QTNs appeared only in one model, indicating the reliability and complementarity of these models. We also found that the number of significant QTNs for different FAAs varied from 8 to 41, which revealed the complexity of the genetic regulation of metabolism, and further demonstrated the necessity of the multi-locus GWAS. Around these significant QTNs, 15 candidate genes were found to be involved in FAA biosynthesis, and one candidate gene (TraesCS1D01G052500, annotated as tryptophan decarboxylase) was functionally identified to influence the content of tryptamine in vitro. Our study demonstrated the power and efficiency of multi-locus GWAS models in crop metabolome research and provided new insights into understanding FAA biosynthesis in wheat.
Introduction
Genome-wide association studies (GWAS) have largely been applied to the genetic dissection of complex traits in plants. With the landmark GWAS study of 107 phenotypes in Arabidopsis (Atwell et al., 2010), numerous other studies have been successfully performed, including those addressing the flowering time and grain yield in rice (Huang et al., 2012; Yang W. et al., 2014), salinity tolerance in barley (Fan et al., 2016), male inflorescence size in maize (Wu et al., 2016), floret fertility in wheat (Guo et al., 2017), and the reducing levels of cucurbitacin in cucumber domestication (Shang et al., 2014). Of these studies, the mixed linear model (MLM) has been adopted most frequently owing to its effective control of spurious associations (Yu et al., 2006). However, as a single-locus GWAS approach, MLM leads to missing some significant loci because of the conservative Bonferroni correction (0.05/me, where me is the number of effective markers) and the stringent criterion of the significance test (Wang et al., 2016). To address this issue, several multi-locus models have been developed, such as Bayesian LASSO (Hoggart et al., 2008), ISISEM-BLASSO (Tamba et al., 2017), pLARmEB (Zhang et al., 2017), and pKWmEB (Ren et al., 2018). Because of the multi-locus nature, the obvious superiority of these approaches is that no Bonferroni correction is demanded, hence, a looser significance criterion can be adopted, and more-powerful quantitative trait nucleotides (QTNs) can be detected (Wang et al., 2016).
Plants produce a vast array of metabolites that provide nutrition and medicine for humans (Saito and Matsuda, 2010; Chae et al., 2014). Unraveling the diversity of the plant metabolome and its underlying mechanism has attracted increasing research interest in the past decade (Schwab, 2003; De Luca et al., 2012). Recent research showed that GWAS coupled with metabolome analysis (mGWAS) exhibited great potential to dissect the genetic and biochemical bases of metabolome diversity (Chan et al., 2011; Chen et al., 2014; Wen et al., 2014). Similar to complex traits such as plant height and grain weight, which are usually controlled by several loci with small effects (Huang et al., 2010; Yang W. et al., 2014), the production of plant metabolites is often controlled by pathways composed of multiple genes. For instance, levels of primary metabolites, such as amino acids, fatty acids and saccharides, tend to be controlled by small effects loci (Angelovici et al., 2013; Matsuda et al., 2015). Whereas, in contrast to primary metabolites, the contents of secondary metabolites are always controlled not only by a few major loci with large effects but also by additional numerous loci with small effects (Chan et al., 2010; Riedelsheimer et al., 2012). Although the single-locus mGWAS models have succeeded in identifying a number of genetic variants associated with thousands of metabolites, this methodology ignores the joint effects of multiple genetic markers on metabolites (Chan et al., 2010; Tamba et al., 2017). Therefore, multi-locus models are a valuable alternative method for mGWAS analysis.
Bread wheat or common wheat (Triticum aestivum L.) is one of the most important crops worldwide and provides approximately 20% of the energy, protein and dietary fiber consumed for human (Ling et al., 2013). The improvement of kernel quality has been a major target in breeding for a long time (Nelson et al., 2006; Jin et al., 2016). Although the seed amino acids are mainly present as components of storage proteins, free amino acids (FAAs) can contribute significantly to be the contents of limited essential amino acids in wheat kernels (Angelovici et al., 2013). To improve the amino acid compositions, both traditional plant breeding techniques and new biotechnologies can be utilized (Fernie and Schauer, 2009). Recently, with the rapid development of the next-generation sequencing technologies, some key genes influencing FAA concentrations have been identified in rice (Chen et al., 2016), maize (Deng et al., 2017), and Arabidopsis (Angelovici et al., 2013) via mGWAS, which showed great potential to accelerate breeding for balanced AA compositions. However, to our knowledge, no studies of dissecting genetic associations with FAA levels in wheat have been reported.
Here, to understand the genetic bases underlying the natural variation and the biosynthesis of FAAs in wheat kernels, we detected the levels of 20 FAAs with an LC-MS platform (Chen et al., 2013) from a highly diverse association panel of 182 accessions. We identified 328 significant QTNs (LOD > 3.0) with six multi-locus mGWAS models and assigned 15 candidate genes involved in FAA biosynthesis. As a proof of concept, we functionally identified TraesCS1D01G052500 in vitro. Our study proved the efficiency of multi-locus GWAS models in metabolome research and provided new insights into understanding of FAA biosynthesis in wheat, which may facilitate metabolomics-based breeding for quality improvement.
Materials and Methods
Plant Material
A highly diverse association panel of 182 Triticum aestivum L. accessions, including both landraces and elite varieties (Supplementary Table S1), was described as before (Liu J. et al., 2017). All accessions were grown at Gaoyi in Hebei province and Dezhou in Shandong province during the 2016–2017 cropping season. Field trials were conducted in randomized complete blocks with three replicates at each location. Each plot contained three 2 m rows spaced 20 cm apart. Field trials followed standard agronomic wheat management practice. Ten mature seeds were randomly collected and pooled for metabolic profiling analysis.
Genotyping
Total genomic DNA was extracted from young leaves for SNP arrays. The 182 accessions were genotyped using the Illumina wheat 90 K SNP by Capital Bio Corporation, Beijing, China1. Accuracy of SNP clustering was validated visually step by step. Of the 81,587 SNPs, those with minor allele frequencies (MAFs) < 0.05 and missing data >20% were excluded from further analysis (Liu J. et al., 2017) to avoid spurious MTAs, finally, a total of 14,646 SNPs were employed in the association panel for GWAS analysis (Dong et al., 2016). The physical positions of SNPs were obtained from the International Wheat Genome Sequencing Consortium website (IWGSC)2.
Determination of AA Levels
A widely targeted metabolomic platform was applied to quantify the FAA contents in mature wheat kernel samples as described previously (Chen et al., 2013). The dried kernels were crushed using a mixer mill (MM 400, Retsch) for 1.2 min at 29 Hz. Then, 100 mg powder was weighted and extracted for 8 h at 4°C with 1.0 ml 70% aqueous methanol containing 0.1 mg/l lidocaine (internal standard). Extracts were centrifuged at 10,000 g for 10 min, and filtrated (SCAA-104, 0.22 μm pore size; ANPEL, Shanghai, China3 before LC–MS analysis. The HPLC conditions as follow: column, shim-pack VP-ODS C18; solvent system, water with 0.04% acetic acid and acetonitrile with 0.04% acetic acid; gradient program, 0 min, 100:0 V/V, 20.0 min, 5:95 V/V, 22.0, 5:95 V/V, 22.1, 95:5 V/V, 25.0, 95:5 V/V; flow rate, 0.25 ml min−1; temperature, 40°C; Injection volume, 5 μl. The MS parameters as follow: ion spray voltage (IS) 5,500 V; source temperature 500°C; ion source gas I (GSI), gas II (GSII), curtain gas (CUR) were set at 55, 60, and 25.0 psi, respectively, the collision gas (CAD) was high. A specific set of MRM (multiple reaction monitoring) transitions were monitored for each FAA (Supplementary Table S2), each MRM transition was obtained with a 5 ms pause time and 5 ms Dwell time, data were processed by Analyst 1.5.1 software, peak areas were integrated using a IntelliQuan algorithm. Endogenous concentrations of FAAs were quantified by calculating the peak area in comparison to standard curves obtained from authenticated standards (purchased from Sigma-Aldrich). Calibration curves were drawn by plotting at least four different concentrations of each FAA standard according to the peak area (Dong et al., 2014). Finally, to eliminate environmental effects, BLUPs (best linear unbiased predictor) across two environments were used as the phenotypic values for all subsequent analyses (Liu J. et al., 2017).
GWAS Mapping
Free amino acid levels were simultaneously studied with a single-locus GWAS model (MLM) and six multi-locus GWAS models. The single-locus model was implemented by FaST-LMM program (Lippert et al., 2011), while multi-locus models were implemented by mrMLM (Wang et al., 2016), FASTmrMLM (Tamba, 2017), FASTmrEMMA (Wen et al., 2017), ISISEM-BLASSO (Tamba et al., 2017), pLARmEB (Zhang et al., 2017), and pKWmEB (Ren et al., 2018). The critical threshold for significantly associated SNPs was set at LOD > 3.0 for the six multi-locus models, and P = 0.05/14,646 = 3.41 × 10−6 (or -log 10P -value = 5.5, Bonferroni correction) for MLM.
Statistical Analysis
We used s/ȳ × 100 to calculate the values of coefficient variation (CV, %) for each FAA, where s and ȳ are the standard deviation (SD) and the mean of each FAA in the population, respectively. Spearman’s rank correlation coefficient was used to calculate the correlation between each pair of FAAs, and statistical significance was obtained by using Student’s t-test.
In vitro Validation of Candidate Genes
Full-length cDNA of TraesCS1D01G052500 was amplified with the primer using cDNA from Huaimai20 as a template. Clones were digested with BamH I/EcoR I and directionally ligated to the pre-digested pGEX-6p-1 vector. Error-free recombinant proteins were expressed in BL-21 (DE3) competent cells after induced by adding 0.1 mM isopropyl β-D-1-thiogalactopyranoside (IPTG) and growing continually for 12 h at 16°C. Cells were harvested and suspended in the lysis buffer [contains 500 mM NaCl, 50 mM Tris-HCl (pH 8.0), 10% glycerol, 5 mM β-mercaptoethanol and 1 mM PMSF] and lysed by high pressure. The crude extract was collected and clarified by centrifugation at 14,000 g for 1 h at 4°C, and the supernatant was stored at −80°C for future experiments.
The standard in vitro enzyme assay for the role of TraesCS1D01G052500 (tryptophan as substrate) was performed in a total volume of 20 μl containing 100 ppm PLP and 50 μM substrate in 50 mM Tris-HCl buffer (pH 8.0). After incubating at 37°C for 30 min, the reaction was stopped by adding 60 μl of methanol. The reaction mixture was then filtered through a 0.2 μm filter (Millipore) before being used for LC-MS analysis.
Phylogenetic Analysis of Different Gene Families
We use the CLUSTALW (version 1.83) program to align the amino acid sequences and construct the neighbor-joining tree by MEGA5. Bootstrap values from 1,000 times are indicated at each node. Bar = 0.1 amino acid substitutions per site.
Enzyme Kinetics
To determine the kinetic difference between TraesCS1D01G052500 and its homologs in rice (OsTDC1 and OsTDC3), their activities were measured using 50 ng of purified protein expressed from E. coli, with 10–1,250 μM different tryptophan (Sigma) as substrates and a fixed concentration of 50 ppm PLP (Sigma) as co-factor. The kinetic parameters were calculated using Michaelis–Menten model (SigmaPlot software, version 14.0). All reactions were run in duplicate and repeated twice.
Results
Natural Variation of Free Amino Acids in Wheat Kernel
To assess the phenotypic variation for FAAs in dry, mature wheat kernels, the absolute levels of 20 FAAs (alanine, arginine, asparagine, aspartic acid, glutamic acid, histidine, isoleucine, leucine, lysine, methionine, phenylalanine, proline, serine, serotonin, threonine, tryptamine, tryptophan, tyramine, tyrosine, and valine in nmol/mg dry wheat kernels) were quantified using LC-MS/MS as previously described (Chen et al., 2013). Visualization of the FAA profiling was performed by hierarchical cluster analysis (HCA), and accumulation of FAAs displayed a distinct phenotypic variation according to their abundance (Figure 1). Aspartic acid, glutamic acid, alanine and serine were the most highly abundant FAAs, with average concentrations of 0.37, 0.31, 0.30, 0.30 nmol/mg, respectively, while tyramine, threonine, and tryptamine were the less abundant, with average concentrations of 0.005, 0.02, 0.03 nmol/mg, respectively (Supplementary Table S2). The content of each FAA varied widely within the association panel, with variation ranging from a 2.30-fold difference in tyrosine to a 30.36-fold difference in proline and with the genetic coefficient variation (CV, %) ranging from 15.9 to 103.2, respectively (Figure 1 and Supplementary Table S2). The relationships between 20 FAA values were evaluated by Spearman’s rank correlation, and strong positive correlations were identified between most of these FAAs, with the exceptions of tryptamine and tryptophan (Supplementary Table S3).
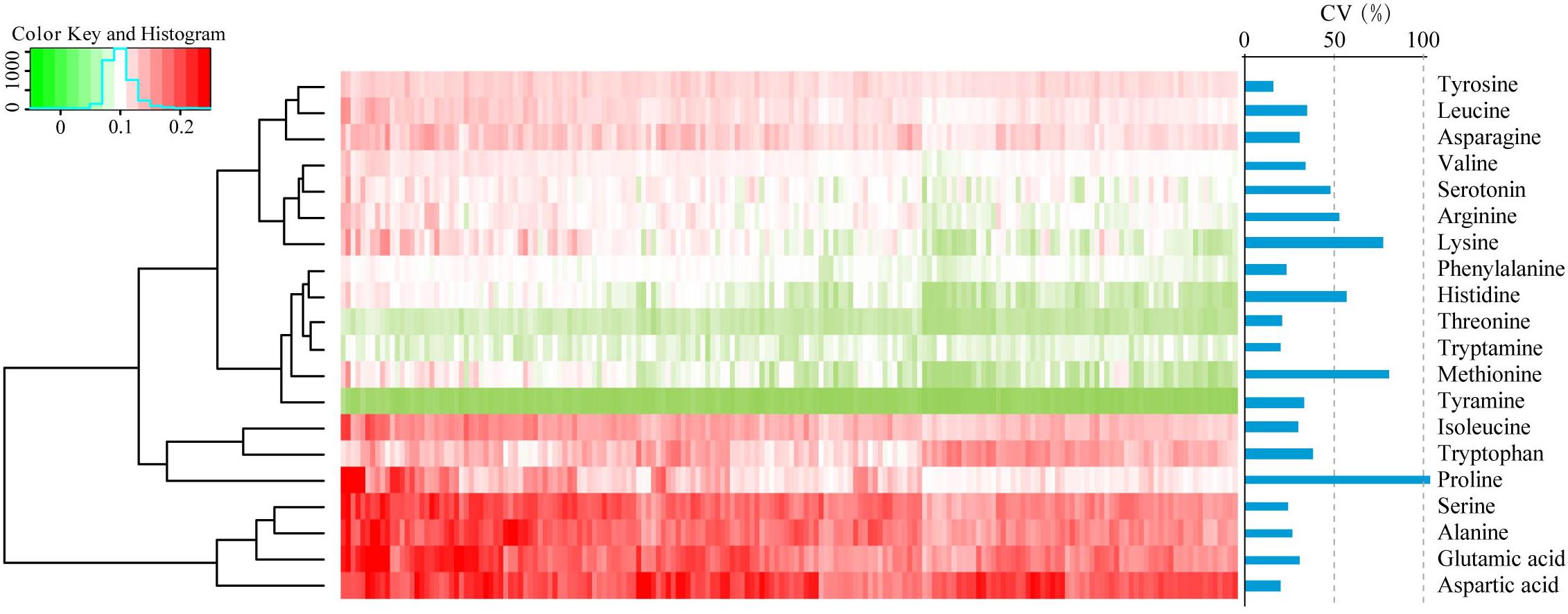
FIGURE 1. Hierarchical cluster analysis (HCA) and the coefficient variation (CV, %) of the levels of FAAs in 182 wheat accessions. Each accession is visualized in a single column, and each FAA is represented by a single row. Red indicates high level, whereas low FAA contents are shown in green.
Associated Loci Mapped by Different Models
To dissect the genetic basis of natural variation for FAA levels in mature wheat kernels, GWAS was performed using seven different models simultaneously. In total, 328 significant QTNs were identified by six multi-locus models (FASTmrEMMA, FASTmrMLM, ISISEM-BLASSO, mrMLM, pKWmEB, and pLARmEB) at a critical threshold of LOD > 3.0 (Supplementary Table S4), and the numbers of QTNs for the above six models were 38, 8, 92, 45, 117, and 28 (Table 1), respectively. Of these QTNs, 66 were detected by at least two different models; some QTNs, such as the association between lysine and SNP BS00003585_51 on chromosome 2B (747,603,047 bp), were simultaneously mapped by five different models (Supplementary Table S4). Only four significant SNP-trait associations were identified by the single-locus model (MLM) (Table 1), and could be also detected by some multi-locus models. Although 18 FAAs were found by FASTmrEMMA to be significantly associated with QTNs, the total number of QTNs is only 38, with an average of 2.1 QTNs per FAA. Comparatively, for the pKWmEB and ISISEM-BLASSO models, the average QTNs per trait reached 6.2 and 4.6, respectively (Table 1). The phenotypic variation explained by different loci varied from 0.1% (tyramine in pKWmEB) to 21.4% (aspartic acid in mrMLM), with an average of 5.6%. We also found that the same QTN shows different effects to explain the phenotypic variation in different models; for instance, the association between arginine and SNP BS00022811_51 on chromosome 7A (709,639,589 bp) with the r2 ranged from 0.1% in FASTmrEMMA to 19.7% in pKWmEB (Supplementary Table S4).
The number of significant QTNs also varied widely among different FAAs, ranging from 8 for tryptophan to 41 for tyramine (Figure 2), indicating the complex genetic regulation of FAAs. The chromosomal distribution of all identified QTNs revealed that A genome had the greatest number of significant associations, while only few QTNs were detected in the D genome (Figure 2). Since QTNs were not distributed evenly on the chromosomes (Deng et al., 2017), five QTN hotspots were observed on chromosomes 2A, 4A, 6A, 7A, and 7B, with the most obvious one being that more than 18 QTNs can be detected between 7 FAAs and SNP RAC875_c1022_3059 (located at 595,984,457 bp on chromosome 4A) (Figure 2 and Supplementary Table S4). The candidate genes underlying these QTN hotspots could include transcriptional factors, transporters or some other rate-limiting enzymes of the amino acid metabolic pathway.
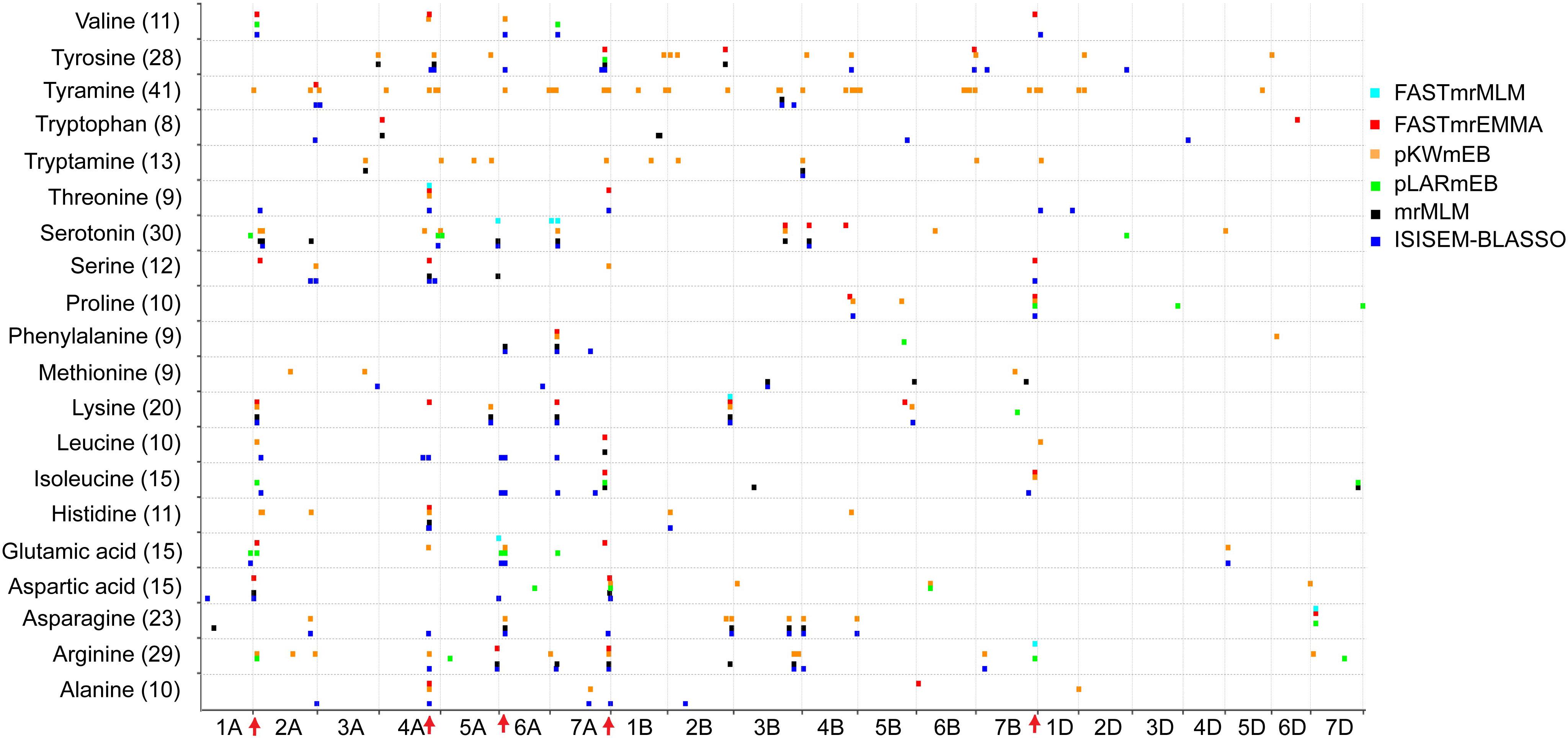
FIGURE 2. Chromosomal distribution of QTNs identified in this study. The x-axis indicates genomic locations by chromosomal order, and the significant QTNs are plotted against genome location. Each row represents one QTN identified by a different model. The red arrows show the QTN hotspots.
Candidate Genes Underlying QTNs
Notably, the 328 significantly QTNs facilitated the assignment of candidate genes. To identify them, the flanking sequences corresponding to the SNP markers significantly associated with FAA levels were used in BLASTx search against NCBI database4. In most cases, the chemical structure combining with the existing knowledge of the biosynthetic pathway of the amino acids allowed the tentative assignment of a protein sequence that is biochemically related to the associated FAAs. Notably, 15 candidate genes involved in FAAs anabolism or catabolism were identified by mGWAS in this study (Table 2), based on the wheat reference genome information (see footnote 2).
A significant QTN between the levels of glutamic acid and the SNP Excalibur_c35310_375 was identified on chromosome 1A; this SNP is located 0.5 Mb away from TraesCS1A01G390300 (encoding a putative glutamate receptor). The high homology (58% identity at amino acid level) between TraesCS1A01G390300 and the glutamate receptor gene AtGLR3.5 (Teardo et al., 2015) suggests that TraesCS1A01G390300 is likely the candidate gene underlying this locus. The SNP RAC875_c1022_3059 was significantly associated with 7 FAAs (Supplementary Table S4), which is comprised a hotspot on chromosome 4A as mentioned above. The high sequence identity (61% at the amino acid level) between adjacently located gene TraesCS4A01G294100 (0.4 Mb to SNP RAC875_c1022_3059) and AtAPM1 (Murphy et al., 2002), an aminopeptidase in Arabidopsis, suggests that TraesCS4A01G294100 is likely the candidate gene underlying this QTN. Similarly, TraesCS4B01G020000 (also encoding a putative aminopeptidase), was assigned as the candidate gene underlying the content of asparagine. The associations were further supported by phylogenetic analysis (Figure 3A).
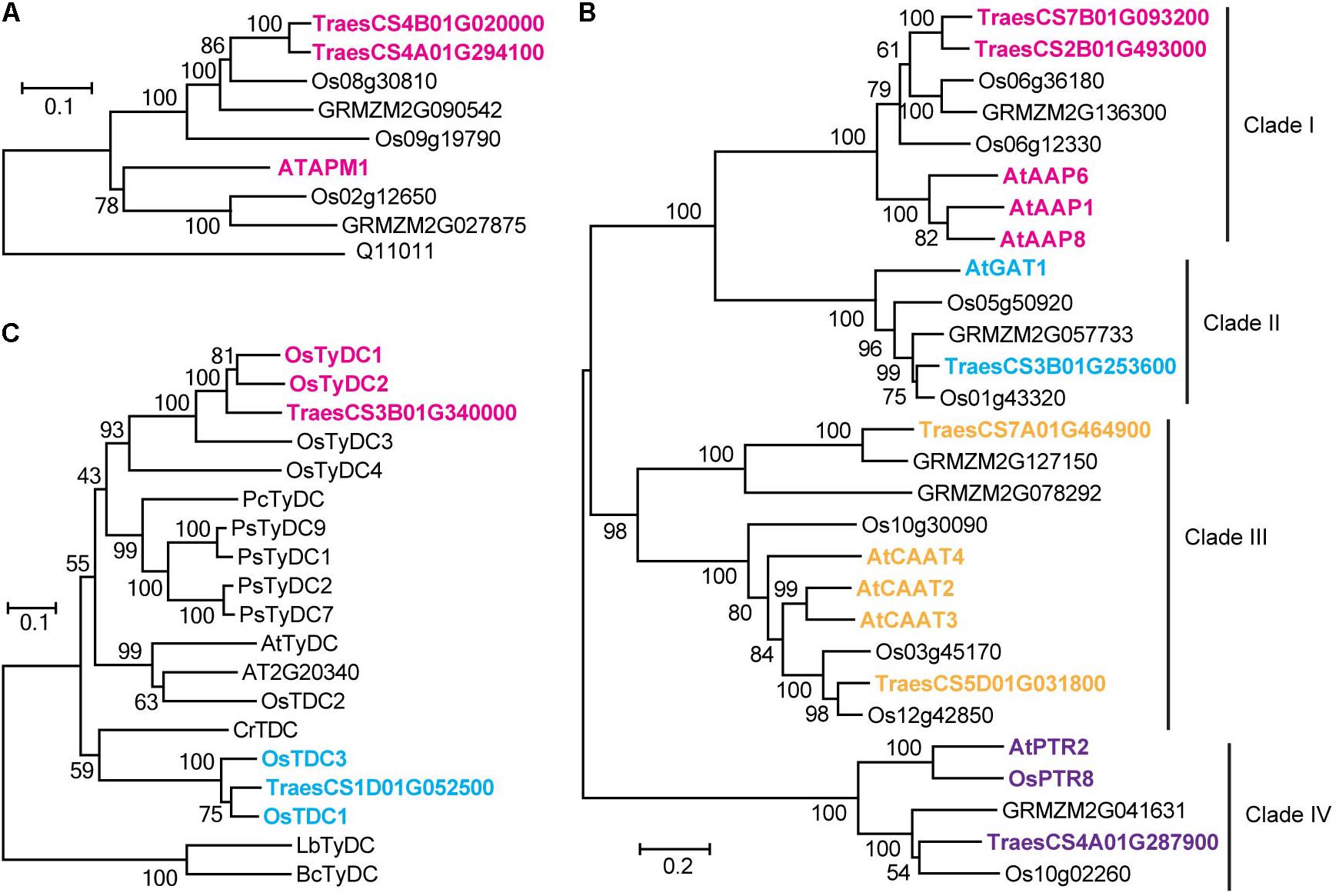
FIGURE 3. Homologous amino acid sequences of aminopeptidase gene family (A), tyrosine decarboxylase and tryptophan decarboxylase gene families (B), and amino acid permease, amino acid transporter and peptide transporter gene families (C) from multiple species were collected and aligned. The neighbor-joining trees were constructed using MEGA software and tested using bootstrap method at replication number of 1000. Phylogenetic analysis of different gene families assigned in the study. Os, Oryza sativa; At, Arabidopsis thaliana; Pc, Petroselinum crispum; Ps, Papaver somniferum; Cr, Catharanthus roseus; Lb, Lactobacillus brevis; Bc, Bacillus cereus.
Levels of tryptamine were significantly associated (LOD = 3.8) with the SNP BS00012936_51 on chromosome 1D that is 1.0 Mb away from TraesCS1D01G052500, which encodes a protein annotated as tryptophan decarboxylase, suggesting that TraesCS1D01G052500 catalyzes the key step of tryptamine biosynthesis. Similarly, TraesCS3B01G340000 (encoding a putative tyrosine decarboxylase) was assigned as the candidate gene underlying the levels of tyramine. The high sequence identities between TraesCS1D01G052500 and OsTDC1 (88% at the amino acid level, Kanjanaphachoat et al., 2012), TraesCS3B01G340000 and OsTyDC2 (79% at the amino acid level, Kang et al., 2007) further supported the realness of these QTNs (Figure 3B).
Six candidate genes putatively annotated as amino acid transporters (AATs) or amino acid permeases (AAPs) were identified by mGWAS (Table 2). We investigated the phylogenetic relationships among the AATs (or AAPs) by constructing the phylogenetic tree with a neighbor-joining algorithm based on the amino acid sequences of these candidate genes and a collection of nine reported genes (Dietrich et al., 2004; Hirner et al., 2006; Meyer et al., 2006; Lee et al., 2007; Yang H. et al., 2014; Santiago and Tegeder, 2016). As a result, characterized AATs (or AAPs) were sorted into four major clades (Figure 3C). Closer examination of the phylogeny in clade III reveled that TraesCS5D01G031800 lies next to AtCAAT2, AtCAAT3, and AtCAAT4, three cationic amino acid transporters from Arabidopsis (Yang H. et al., 2014), consistent with the significant QTN between the levels of glutamic acid (a typical cationic amino acid) and TraesCS5D01G031800 locus (Figure 3C and Supplementary Table S4). Our analysis also placed TraesCS2B01G493000 and TraesCS7B01G093200 close to AtAAP1, AtAAP6, and AtAAP8 (Hirner et al., 2006; Lee et al., 2007; Santiago and Tegeder, 2016) within clade I, strongly supporting the annotation of these candidates as AAPs in wheat (Figure 3C). Moreover, the high sequence identities between TraesCS3B01G253600 and AtGAT1 (63% at the amino acid level, Meyer et al., 2006), TraesCS4A01G287900 and AtPTR2 (44% at the amino acid level, Dietrich et al., 2004) provide further evidence for these assignments (Figure 3C).
Functional Identification of Candidate Genes
Although experimental validation of all candidate genes disclosed by our mGWAS analyses is beyond the scope of a single study, we nevertheless tried to show that such confirmation is possible. For this purpose, we further characterized one candidate gene and provided novel biochemical insight into the FAA biosynthesis in wheat.
As mention above, the association between TraesCS1D01G052500 and tryptamine levels suggests that TraesCS1D01G052500 is the decarboxylase that catalyzes the biosynthesis of tryptamine (Figures 3B, 4A,B). To characterize the enzymatic properties of TraesCS1D01G052500, recombinant protein was expressed with an N-terminal glutathione S-transferase (GST) tag in E. coli BL-21 and the reaction product was confirmed by commercial standard with LC-MS (Figure 4C). An obvious TDC activity showed for tryptophan, and its activity was not inhibited by tyrosine, indicating a high level of substrate specificity toward tryptophan (Supplementary Table S5). We further investigated the enzyme kinetics of TraesCS1D01G052500 and its rice homologs (OsTDC1 and OsTDC3), all of them displayed similar Kcat values for tryptophan (Supplementary Table S5), suggesting that the three proteins have similar TDC activities. Based on these results, we functionally identified TraesCS1D01G052500 as a decarboxylase that catalyzes the biosynthesis of tryptamine from tryptophan in wheat (Figure 4D), which further confirmed the correctness of our GWAS results and the candidate gene assignment.
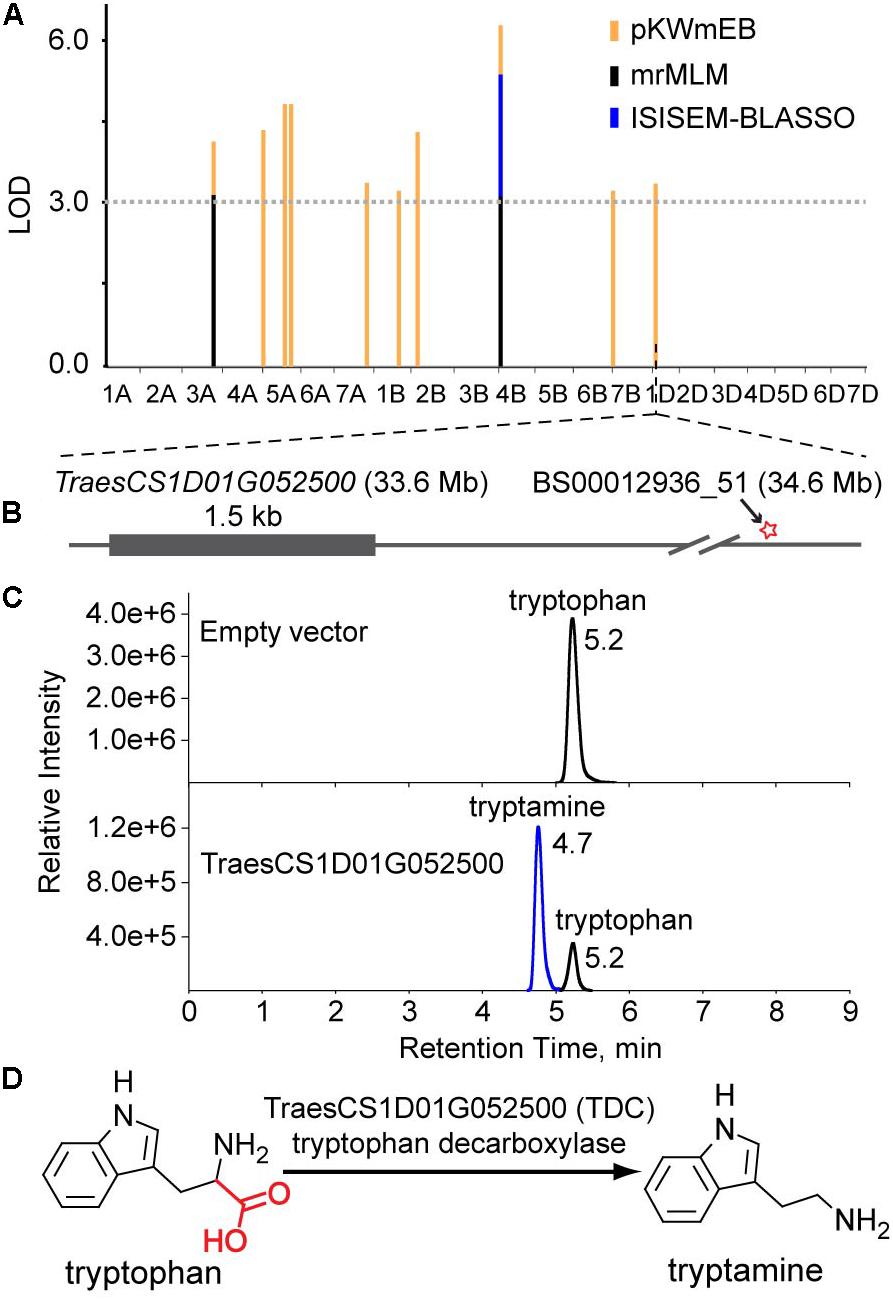
FIGURE 4. Functional identification of TraesCS1D01G052500 in vitro. (A) The multi-locus GWAS results for the tryptamine level in different models. (B) Gene model of TraesCS1D01G052500. The filled gray box represents coding sequence, and the star represents the associated site. (C) LC-MS/MS chromatograms of in vitro enzyme assays showing the enzyme activity of recombinant TraesCS1D01G052500 (Down). Protein extract from E. coli containing empty vector were used as a negative control (Up). (D) The proposed pathway of tryptamine biosynthesis in wheat.
Discussion
By coupling with the rapid development of LC-MS strategies, more accurate contents of metabolites can be obtained, and larger phenotypic variation can be observed (Chen et al., 2014). In this study, most of the FAAs varied widely across the association panel, such as proline with range of 30.4-fold (Supplementary Table S2), indicating the complexity of the biosynthetic processes of FAAs (Figure 1). The levels of lysine (an essential amino acid) have huge phenotypic variation, with a CV (%) of 77.2, implying the existence of a large number of alleles with high genetic diversity in the wheat germplasms (Liu Y. et al., 2017). Thus, identification of the favorable alleles and dissection of the genetic architecture underlying the levels of FAA is beneficial for improving the amino acid compositions in the future.
Dissecting the natural variation and the underlying genetic bases of metabolism is essential for the improvement of crop nutritional quality (Luo, 2015). Due to recent advances in both high-throughput metabolic profiling and sequencing technologies, mGWAS has been employed as a powerful strategy to reveal the genetic and biochemical basis of crop metabolism (Riedelsheimer et al., 2012; Wen et al., 2014; Matsuda et al., 2015). So far, most of these studies have been carried out on maize and rice. What’s more important, hundreds of significant loci were identified for various metabolites of nutritional importance, both of large effects and at high resolution, which facilitated the identification of the candidate genes (Luo, 2015). Advanced in developing the genomic toolbox (Jia et al., 2013; Ling et al., 2013; Avni et al., 2017), Matros et al. (2017) quantified 76 leaf metabolites from 135 winter wheat lines and identified several significant associations for six metabolic traits based on 17,372 SNP markers. This confirmed the potential of the mGWAS approach and provided the opportunity for a further understanding of metabolic diversity in wheat. In our study, we also mapped hundreds of QTNs for the levels of 20 FAAs in a wheat diverse association panel, however, most of them had very small effects, explaining the phenotypic variation with an average of 5.6% (Supplementary Table S4). Obviously, the limitations of mGWAS in wheat relate in part to the large size of the genome and in part to the limited availability of sets of genetic markers (Zhou et al., 2018), which leads to great difficulties to confirm the candidate genes. These constraints could be gradually complemented by applying new sequencing technologies and developing additional genomic markers (Liu Y. et al., 2017), and also, utilizing larger number of accessions and choosing more comprehensive choices of germplasms can enhance the power of mGWAS approaches, as demonstrated in rice and maize (Huang et al., 2012; Riedelsheimer et al., 2012).
As usual, variation of primary metabolites tends to be controlled by many small-effect loci. To increase the detection power of mGWAS, six multi-locus models were applied in this study. Totally, 328 significant QTNs were identified, however, only 4 SNP-trait associations were found with the single-locus model (MLM) at P ≤ 3.41 × 10−6 (Table 1 and Supplementary Table S3). These results indicated the power of these multi-locus methods. Furthermore, the common QTNs appeared in different models confirming the credibility of these multi-locus GWAS approaches.
Based on these QTNs identified by the six multi-locus methodologies, candidates that have not been identified previously can be explored by searching for a protein or protein cluster that is biochemically related to the associated FAAs encoded at these loci. As a result, our mGWAS has allowed the assignment of 15 candidate genes underlying FAA levels (Table 2). The existing knowledge of plant FAA pathways, the high sequence identities between them and known functions in rice and Arabidopsis further confirmed these candidate genes. Notably, the validation of TraesCS1D01G052500 was detected only by the pKWmEB model (Figure 4), further demonstrating the reliability and effectiveness of these multi-locus methods.
Conclusion
In this study, a comprehensive GWAS of 20 FAA levels based on 14,646 SNPs in bread wheat was performed by six multi-locus models. Among 328 significant QTNs, 66 were detected by at least two models, and 155 QTNs appeared only in one model. Fifteen candidate genes were assigned to FAA biosynthesis, and one candidate gene was functionally identified in vitro. This study proved the power and reliability of multi-locus GWAS models in plant metabolome research and provided new insights into understanding FAA biosynthesis in wheat, which may facilitate metabolomics-based breeding for quality improvement.
Author Contributions
WC, YH, and ZH conceived the project and supervised this study. YP, HL, and JC performed most of the experiments. TS, DS, and CZ participated in preparation of the materials. WC and YP analyzed the data. WC wrote the paper. All the authors discussed the results and commented on the manuscript.
Funding
This work was supported by Huazhong Agricultural University Scientific & Technological Self-innovation Foundation (Program No. 2017RC006), National Key Research and Development Program of China (2016YFE0108600 and 2016YFD0101802).
Conflict of Interest Statement
The authors declare that the research was conducted in the absence of any commercial or financial relationships that could be construed as a potential conflict of interest.
Supplementary Material
The Supplementary Material for this article can be found online at: https://www.frontiersin.org/articles/10.3389/fpls.2018.01196/full#supplementary-material
Footnotes
- ^ http://www.capitalbiotech.com/
- ^ http://www.wheatgenome.org/
- ^ www.anpel.com.cn/
- ^ http://www.ncbi.nlm.nih.gov/
References
Angelovici, R., Lipka, A. E., Deason, N., Gonzalez-Jorge, S., Lin, H., Cepela, J., et al. (2013). Genome-wide analysis of branched-chain amino acid levels in Arabidopsis seeds. Plant Cell 25, 4827–4843. doi: 10.1105/tpc.113.119370
Atwell, S., Huang, Y. S., Vilhjalmsson, B. J., Willems, G., Horton, M., Li, Y., et al. (2010). Genome-wide association study of 107 phenotypes in Arabidopsis thaliana inbred lines. Nature 465, 627–631. doi: 10.1038/nature08800
Avni, R., Nave, M., Barad, O., Baruch, K., Twardziok, S. O., Gundlach, H., et al. (2017). Wild emmer genome architecture and diversity elucidate wheat evolution and domestication. Science 357, 93–97. doi: 10.1126/science.aan0032
Chae, L., Kim, T., Nilo-Poyanco, R., and Rhee, S. Y. (2014). Genomic signatures of specialized metabolism in plants. Science 344, 510–513. doi: 10.1126/science.1252076
Chan, E. K., Rowe, H. C., Corwin, J. A., Joseph, B., and Kliebenstein, D. J. (2011). Combining genome-wide association mapping and transcriptional networks to identify novel genes controlling glucosinolates in Arabidopsis thaliana. PLoS Biol. 9:e1001125. doi: 10.1371/journal.pbio.1001125
Chan, E. K., Rowe, H. C., Hansen, B. G., and Kliebenstein, D. J. (2010). The complex genetic architecture of the metabolome. PLoS Genet. 6:e1001198. doi: 10.1371/journal.pgen.1001198
Chen, W., Gao, Y., Xie, W., Gong, L., Lu, K., Wang, W., et al. (2014). Genome-wide association analyses provide genetic and biochemical insights into natural variation in rice metabolism. Nat. Genet. 46, 714–721. doi: 10.1038/ng.3007
Chen, W., Gong, L., Guo, Z., Wang, W., Zhang, H., Liu, X., et al. (2013). A novel integrated method for large-scale detection, identification, and quantification of widely targeted metabolites: application in the study of rice metabolomics. Mol. Plant 6, 1769–1780. doi: 10.1093/mp/sst080
Chen, W., Wang, W., Peng, M., Gong, L., Gao, Y., Wan, J., et al. (2016). Comparative and parallel genome-wide association studies for metabolic and agronomic traits in cereals. Nat. Commun. 7:12767. doi: 10.1038/ncomms12767
De Luca, V., Salim, V., Atsumi, S. M., and Yu, F. (2012). Mining the biodiversity of plants: a revolution in the making. Science 336, 1658–1661. doi: 10.1126/science.1217410
Deng, M., Li, D., Luo, J., Xiao, Y., Liu, H., Pan, Q., et al. (2017). The genetic architecture of amino acids dissection by association and linkage analysis in maize. Plant Biotechnol. J. 15, 1250–1263. doi: 10.1111/pbi.12712
Dietrich, D., Hammes, U., Thor, K., Suter-Grotemeyer, M., Fluckiger, R., Slusarenko, A. J., et al. (2004). AtPTR1, a plasma membrane peptide transporter expressed during seed germination and in vascular tissue of Arabidopsis. Plant J. 40, 488–499. doi: 10.1111/j.1365-313X.2004.02224.x
Dong, X., Chen, W., Wang, W., Zhang, H., Liu, X., and Luo, J. (2014). Comprehensive profiling and natural variation of flavonoids in rice. J. Integr. Plant Biol. 56, 876–886. doi: 10.1111/jipb.12204
Dong, Y., Liu, J., Zhang, Y., Geng, H., Rasheed, A., Xiao, Y., et al. (2016). Genome-wide association of stem water soluble carbohydrates in bread wheat. PLoS One 11:e0164293. doi: 10.1371/journal.pone.0164293
Fan, Y., Zhou, G., Shabala, S., Chen, Z., Cai, S., Li, C., et al. (2016). Genome-wide association study reveals a new QTL for salinity tolerance in barley (Hordeum vulgare L.). Front. Plant Sci. 7:946. doi: 10.3389/fpls.2016.00946
Fernie, A. R., and Schauer, N. (2009). Metabolomics-assisted breeding: a viable option for crop improvement? Trends Genet. 25, 39–48. doi: 10.1016/j.tig.2008.10.010
Guo, Z., Chen, D., Alqudah, A. M., Roder, M. S., Ganal, M. W., and Schnurbusch, T. (2017). Genome-wide association analyses of 54 traits identified multiple loci for the determination of floret fertility in wheat. New Phytol. 214, 257–270. doi: 10.1111/nph.14342
Hirner, A., Ladwig, F., Stransky, H., Okumoto, S., Keinath, M., Harms, A., et al. (2006). Arabidopsis LHT1 is a high-affinity transporter for cellular amino acid uptake in both root epidermis and leaf mesophyll. Plant Cell 18, 1931–1946. doi: 10.1105/tpc.106.041012
Hoggart, C. J., Whittaker, J. C., De Iorio, M., and Balding, D. J. (2008). Simultaneous analysis of all SNPs in genome-wide and re-sequencing association studies. PLoS Genet. 4:e1000130. doi: 10.1371/journal.pgen.1000130
Huang, X., Wei, X., Sang, T., Zhao, Q., Feng, Q., Zhao, Y., et al. (2010). Genome-wide association studies of 14 agronomic traits in rice landraces. Nat. Genet. 42, 961–967. doi: 10.1038/ng.695
Huang, X., Zhao, Y., Wei, X., Li, C., Wang, A., Zhao, Q., et al. (2012). Genome-wide association study of flowering time and grain yield traits in a worldwide collection of rice germplasm. Nat. Genet. 44, 32–39. doi: 10.1038/ng.1018
Jia, J. Z., Zhao, S. C., Kong, X. Y., Li, Y. R., Zhao, G. Y., He, W. M., et al. (2013). Aegilops tauschii draft genome sequence reveals a gene repertoire for wheat adaptation. Nature 496, 91–95. doi: 10.1038/nature12028
Jin, H., Wen, W., Liu, J., Zhai, S., Zhang, Y., Yan, J., et al. (2016). Genome-wide QTL mapping for wheat processing quality parameters in a gaocheng 8901/zhoumai 16 recombinant inbred line population. Front. Plant Sci. 7:1032. doi: 10.3389/fpls.2016.01032
Kang, S., Kang, K., Lee, K., and Back, K. (2007). Characterization of rice tryptophan decarboxylases and their direct involvement in serotonin biosynthesis in transgenic rice. Planta 227, 263–272. doi: 10.1007/s00425-007-0614-z
Kanjanaphachoat, P., Wei, B., Lo, S., Wang, I., Wang, C., Yu, S., et al. (2012). Serotonin accumulation in transgenic rice by over-expressing tryptophan decarboxylase results in a dark brown phenotype and stunted growth. Plant Mol. Biol. 78, 525–543. doi: 10.1007/s11103-012-9882-5
Lee, Y. H., Foster, J., Chen, J., Voll, L. M., Weber, A. P., and Tegeder, M. (2007). AAP1 transports uncharged amino acids into roots of Arabidopsis. Plant J. 50, 305–319. doi: 10.1111/j.1365-313X.2007.03045.x
Ling, H., Zhao, S., Liu, D., Wang, J., Sun, H., Zhang, C., et al. (2013). Draft genome of the wheat a-genome progenitor Triticum urartu. Nature 496, 87–90. doi: 10.1038/nature11997
Lippert, C., Listgarten, J., Liu, Y., Kadie, C. M., Davidson, R. I., and Heckerman, D. (2011). FaST linear mixed models for genome-wide association studies. Nat. Methods 8, 833–835. doi: 10.1038/Nmeth.1681
Liu, J., He, Z., Rasheed, A., Wen, W., Yan, J., Zhang, P., et al. (2017). Genome-wide association mapping of black point reaction in common wheat (Triticum aestivum L.). BMC Plant Biol. 17:220. doi: 10.1186/s12870-017-1167-3
Liu, Y., Lin, Y., Gao, S., Li, Z., Ma, J., Deng, M., et al. (2017). A genome-wide association study of 23 agronomic traits in Chinese wheat landraces. Plant J. 91, 861–873. doi: 10.1111/tpj.13614
Luo, J. (2015). Metabolite-based genome-wide association studies in plants. Curr. Opin. Plant Biol. 24, 31–38. doi: 10.1016/j.pbi.2015.01.006
Matros, A., Liu, G., Hartmann, A., Jiang, Y., Zhao, Y., Wang, H., et al. (2017). Genome-metabolite associations revealed low heritability, high genetic complexity, and causal relations for leaf metabolites in winter wheat (Triticum aestivum). J. Exp. Bot. 68, 415–428. doi: 10.1093/jxb/erw441
Matsuda, F., Nakabayashi, R., Yang, Z., Okazaki, Y., Yonemaru, J., Ebana, K., et al. (2015). Metabolome-genome-wide association study dissects genetic architecture for generating natural variation in rice secondary metabolism. Plant J. 81, 13–23. doi: 10.1111/tpj.12681
Meyer, A., Eskandari, S., Grallath, S., and Rentsch, D. (2006). AtGAT1, a high affinity transporter for gamma-aminobutyric acid in Arabidopsis thaliana. J. Biol. Chem. 281, 7197–7204. doi: 10.1074/jbc.M510766200
Murphy, A. S., Hoogner, K. R., Peer, W. A., and Taiz, L. (2002). Identification, purification, and molecular cloning of N-1-naphthylphthalmic acid-binding plasma membrane-associated aminopeptidases from Arabidopsis. Plant Physiol. 128, 935–950. doi: 10.1104/pp.010519
Nelson, J. C., Andreescu, C., Breseghello, F., Finney, P. L., Gualberto, D. G., Bergman, C. J., et al. (2006). Quantitative trait locus analysis of wheat quality traits. Euphytica 149, 145–159. doi: 10.1007/s10681-005-9062-7
Ren, W. L., Wen, Y. J., Dunwell, J. M., and Zhang, Y. M. (2018). pKWmEB: integration of kruskal-wallis test with empirical bayes under polygenic background control for multi-locus genome-wide association study. Heredity 120, 208–218. doi: 10.1038/s41437-017-0007-4
Riedelsheimer, C., Lisec, J., Czedik-Eysenberg, A., Sulpice, R., Flis, A., Grieder, C., et al. (2012). Genome-wide association mapping of leaf metabolic profiles for dissecting complex traits in maize. Proc. Natl. Acad. Sci. U.S.A. 109, 8872–8877. doi: 10.1073/pnas.1120813109
Saito, K., and Matsuda, F. (2010). Metabolomics for functional genomics, systems biology, and biotechnology. Annu. Rev. Plant Biol. 61, 463–489. doi: 10.1146/annurev.arplant.043008.092035
Santiago, J. P., and Tegeder, M. (2016). Connecting source with sink: the role of Arabidopsis AAP8 in phloem loading of amino acids. Plant Physiol. 171, 508–521. doi: 10.1104/pp.16.00244
Schwab, W. (2003). Metabolome diversity: too few genes, too many metabolites? Phytochemistry 62, 837–849.
Shang, Y., Ma, Y., Zhou, Y., Zhang, H., Duan, L., Chen, H., et al. (2014). Biosynthesis, regulation, and domestication of bitterness in cucumber. Science 346, 1084–1088. doi: 10.1126/science.1259215
Tamba, C. L. (2017). A Fast mrMLM Algorithm Improves Statistical Power, Accuracy and Computational Efficiency of Multi-locus Genome-Wide Association Studies. Doctoral dissertation, Nanjing Agricultural University, Nanjing.
Tamba, C. L., Ni, Y. L., and Zhang, Y. M. (2017). Iterative sure independence screening EM-Bayesian LASSO algorithm for multi-locus genome-wide association studies. PLoS Comput. Biol. 13:e1005357. doi: 10.1371/journal.pcbi.1005357
Teardo, E., Carraretto, L., De Bortoli, S., Costa, A., Behera, S., Wagner, R., et al. (2015). Alternative splicing-mediated targeting of the Arabidopsis GLUTAMATE RECEPTOR3.5 to mitochondria affects organelle morphology. Plant Physiol. 167, 216–227. doi: 10.1104/pp.114.242602
Wang, S. B., Feng, J. Y., Ren, W. L., Huang, B., Zhou, L., Wen, Y. J., et al. (2016). Improving power and accuracy of genome-wide association studies via a multi-locus mixed linear model methodology. Sci. Rep. 6:19444. doi: 10.1038/srep19444
Wen, W., Li, D., Li, X., Gao, Y., Li, W., Li, H., et al. (2014). Metabolome-based genome-wide association study of maize kernel leads to novel biochemical insights. Nat. Commun. 5, 3438. doi: 10.1038/ncomms4438
Wen, Y. J., Zhang, H., Ni, Y. L., Huang, B., Zhang, J., Feng, J. Y., et al. (2017). Methodological implementation of mixed linear models in multi-locus genome-wide association studies. Brief. Bioinform. doi: 10.1093/bib/bbw145 [Epub ahead of print].
Wu, X., Li, Y., Shi, Y., Song, Y., Zhang, D., Li, C., et al. (2016). Joint-linkage mapping and GWAS reveal extensive genetic loci that regulate male inflorescence size in maize. Plant Biotechnol. J. 14, 1551–1562. doi: 10.1111/pbi.12519
Yang, H., Krebs, M., Stierhof, Y. D., and Ludewig, U. (2014). Characterization of the putative amino acid transporter genes AtCAT2, 3 & 4: the tonoplast localized AtCAT2 regulates soluble leaf amino acids. J. Plant Physiol. 171, 594–601. doi: 10.1016/j.jplph.2013.11.012
Yang, W., Guo, Z., Huang, C., Duan, L., Chen, G., Jiang, N., et al. (2014). Combining high-throughput phenotyping and genome-wide association studies to reveal natural genetic variation in rice. Nat. Commun. 5:5087. doi: 10.1038/ncomms6087
Yu, J., Pressoir, G., Briggs, W. H., Bi, I. V., Yamasaki, M., Doebley, J. F., et al. (2006). A unified mixed-model method for association mapping that accounts for multiple levels of relatedness. Nat. Genet. 38, 203–208. doi: 10.1038/ng1702
Zhang, J., Feng, J. Y., Ni, Y. L., Wen, Y. J., Niu, Y., Tamba, C. L., et al. (2017). pLARmEB: integration of least angle regression with empirical Bayes for multilocus genome-wide association studies. Heredity 118, 517–524. doi: 10.1038/hdy.2017.8
Keywords: wheat, free amino acid (FAA), genome-wide association studies, multi-locus models, QTNs
Citation: Peng Y, Liu H, Chen J, Shi T, Zhang C, Sun D, He Z, Hao Y and Chen W (2018) Genome-Wide Association Studies of Free Amino Acid Levels by Six Multi-Locus Models in Bread Wheat. Front. Plant Sci. 9:1196. doi: 10.3389/fpls.2018.01196
Received: 27 May 2018; Accepted: 26 July 2018;
Published: 14 August 2018.
Edited by:
Zhenyu Jia, University of California, Riverside, United StatesCopyright © 2018 Peng, Liu, Chen, Shi, Zhang, Sun, He, Hao and Chen. This is an open-access article distributed under the terms of the Creative Commons Attribution License (CC BY). The use, distribution or reproduction in other forums is permitted, provided the original author(s) and the copyright owner(s) are credited and that the original publication in this journal is cited, in accordance with accepted academic practice. No use, distribution or reproduction is permitted which does not comply with these terms.
*Correspondence: Yuanfeng Hao, haoyuanfeng@caas.cn; Wei Chen, chenwei0609@mail.hzau.edu.cn
†These authors have contributed equally to this work