- Department of Genetics, University of Georgia, Athens, GA, United States
The rhizosphere microbiome is known to play a crucial role in promoting plant growth, partly by countering soil-borne phytoparasites and by improving nutrient uptake. The abundance and composition of the rhizosphere and root-associated microbiota are influenced by several factors, including plant species and genotype. We hypothesize that crop domestication might influence the composition and diversity of plant-associated microbiomes. We tested the contribution of domestication to the bacterial and archaeal root and soil composition associated with six genotypes of domesticated Setaria italica and four genotypes of its wild ancestor, S. viridis. The bacterial microbiome in the rhizoplane and root endophyte compartments, and the archaea in the endophyte compartment, showed major composition differences. For instance, members of the Betaproteobacteria and Firmicutes were overrepresented in S. italica root samples compared to S. viridis. Metagenomic analysis of samples that contained both root surface-bound (rhizoplane) and inside-root (endophytic) bacteria defined two unique microbial communities only associated with S. italica roots and one only associated with S. viridis roots. Root endophytic bacteria were found in six discernible communities, of which four were primarily on S. italica and two primarily on S. viridis. Among archaea, Methanobacteria, and Methanomicrobia exhibited species-associated differences in the rhizosphere and root compartments, but most detected archaea were not classified more specifically than at the level of phylum. These results indicate a host genetic contribution to the microbial composition in Setaria, and suggest that domestication has selected for specific associations in the root and in the rhizosphere.
Introduction
Plants, like all other organisms, are surrounded by massive numbers of microbes from a huge diversity of taxa (Thompson et al., 2017). The critical associations between plants and their microbes is the result of millions of years of co-evolution. Even with more than three decades of DNA-based diagnostic tools (Pace et al., 1985; Handelsman et al., 1998; Hugenholtz et al., 1998; Muyzer and Smalla, 1998; Lundberg et al., 2012; Peiffer et al., 2013; Edwards et al., 2015), only a small percentage of microbial ecotypes have been identified and only a tiny fraction of these studied to any great depth. For example, a recent study of the earth’s microbiome diversity indicated that >1/3 of metagenome reads from plant-associated microbial communities could not be mapped to existing rRNA databases (Thompson et al., 2017). This is not surprising, because sequence-based phylogenetic analysis of all of the ∼8000 prokaryotic genomes available in 2015 resulted in the identification of 79 bacterial phyla and 21 archaeal phyla (Parks et al., 2017), yet only four bacterial phyla have been identified by whole genome sequence analysis in ∼1000 phytobacterial genomes analyzed so far (Levy et al., 2018). This could be an accurate reflection of host ranges and/or an indication of the dearth of plant-associated studies conducted to date. Perhaps because plants do not have mobile immune cells or circulating antibodies, many microbes have established durable relationships within plant tissues. In some cases, these microbes provide useful services to the plant, such as for nitrogen fixation (Werner et al., 2015; Coskun et al., 2017) or mineral uptake (Bonfante and Genre, 2010; Smith and Smith, 2011) in the root. Many microbes are pathogenic to the plant, but these constitute only a tiny percentage of the total microbiome in plant::microbe interactions, while the great majority of plant-associated microbes provide either no services or unknown services.
Because soils are the most diverse source of microbes on the planet (Roesch et al., 2007; Trevors, 2010; Delmont et al., 2011), with as many as 104 to 107 unique bacterial cells per gram of soil, every root of a field-grown plant is bathed in as many as 1011 microbial cells per gram of root and more than 30,000 prokaryotic species (Berendsen et al., 2012; Bulgarelli et al., 2015). Numerous studies have shown that the microbial compositions of soils are dramatically altered by root growth, with the diversity significantly lowered and some microbes immensely increased in abundance in the rhizosphere (Reinhold-Hurek et al., 2015;Niu et al., 2017). These root-adjacent microbes can reach abundances of >109 per gram of soil (Mendes et al., 2013) and are nurtured primarily by the exudates actively transported out of the plant root. These exudates, including organic acids, sugars, amino acids and many additional molecules, can amount to >50% of the energy/carbohydrate captured by the host plant through photosynthesis (Kuzyakov and Domanski, 2000; McNear, 2012). Hence, despite the fact that plants can be grown in sterile soils, these results suggest that soil microbes are of great importance for root and plant growth in real-world environments.
Many researchers believe that, like in the animal gut, a major role for microbial communities associated with the plant root is to physically displace pathogens and/or to generate antimicrobials against possible pathogenic microbes (Berendsen et al., 2012; Brader et al., 2017; Hacquard et al., 2017; Schlatter et al., 2017). This, of course, raises the issue of how plant::microbe interactions have co-evolved so that beneficial microbes are attracted and pathogenic microbes are discouraged. Part of the evolved plant component of this specificity is likely to involve specific signaling molecules, like the phenolics that attract and stimulate rhizobial (Cao et al., 2017) and mycorrhizal (Schmitz and Harrison, 2014) symbiosis. This signaling regimen creates a strong selection for pathogens, commensalists and mutualists (Hacquard et al., 2017) to also recognize these signals, similar to the case for the parasitic weed Striga that recognizes the same signal that attracts mycorrhizae (Lopez-Raez et al., 2017). Hence, an evolutionary arms race is expected between the host and pathogen in this process, as is also the case for specific recognition of pathogen effector molecules in plant disease resistance processes (Hacquard et al., 2017; Lopez-Raez et al., 2017).
Recent studies have shown that both host plant genetics and soil environment are major determinants of the microbial communities that abound in, on and/or near roots (Peiffer et al., 2013; Edwards et al., 2015; Goodrich et al., 2016). The plant genetic component is significant when different species are compared, but is also detected when different genotypes of the same plant species are investigated. This, then, opens the possibility that specific alleles of plant genes can be identified that determine which microbes are present in a plant::soil interaction. One dramatic variation in host plant genetics is associated with the suite of human-selected changes that are responsible for crop domestication (Wang et al., 2005; Doebley, 2006; Konishi et al., 2006; Cockram et al., 2007; Zhou et al., 2015). Most of the selected traits are for such agronomic properties as seed retention (shattering) and the day length dependence of flowering. However, there is some evidence that root traits might also be selected during domestication (Waines and Ehdaie, 2007). It makes sense that such factors as irrigation, monoculture or uniform plant spacing would put unique pressures on root development that might also affect root and rhizosphere microbial populations.
In the current study, we have chosen to use the Setaria model system, consisting of a developmentally plastic wild ancestor (S. viridis, green foxtail) and a domesticated descendant (Setaria italica, foxtail millet) (Brutnell et al., 2015). This study provides the first examination of possible effects of domestication upon the root and rhizosphere microbiomes in the genus Setaria. One previous study has investigated the rhizosphere microbiomes of several S. italica cultivars and in two locations (Jin et al., 2017). This investigation demonstrated that microhabitats and geographic location shape foxtail millet root microbial communities, but did not test for any possible role of host genetics on the root microbiome. The current study, in contrast, describes the different prokaryotic communities inside the root, on the root surface and in the surrounding rhizosphere. The results indicate that the domesticated and wild species differ in their microbes by plant genotype, but also show extensive variability in their microbiomes in replicated trials in the greenhouse and field. Six endophyte microbiome communities are identified in the greenhouse, of which four are primarily associated with S. italica.
Materials and Methods
Plant Growth Conditions and Microbial Sampling
Seeds from six accessions of S. italica (Yugu1, B100, 129-86, 84-96, 15-96, and 130-96) and four accessions of S. viridis [A10, SV9-2 (PI212625), PI230135, and 4-V (UMDEL)] were obtained from Prof. Katrien Devos. These seeds were collected from various field locations and USDA GRIN and multiplied in the University of Georgia Plant Biology Greenhouse by standard methods used for self-pollinated crops (Devos et al., 1998; Bennetzen et al., 2012; Mauro-Herrera et al., 2013; Schroder et al., 2017). The seeds were surface sterilized with 8% sodium hypochlorite (Bioworld, United States) for 10 min, followed by three rinses with sterile distilled water. Previous studies demonstrated that the disinfection of seed with 2-5% sodium hypochlorite solution eliminated any surface fungal or bacterial cells and spores (Sauer and Burroughs, 1986; Chun et al., 1997). Seed were germinated in circular-section pots (10″ in diameter and 10″ high) containing a 2:1 mixture of field soil collected from the University of Georgia farm in Bogart, GA and sand. The sand and soil mixture was steamed for 30 min. Setaria plants were grown in a greenhouse (with a 14 h photo-period and day-night temperatures of 26–20°C) and watered daily to approximately 70% soil water holding capacity. Each genotype was grown in three pots with each pot having three seedlings and the pots were randomly arranged. Neither pot location nor orientation were rotated on any specific schedule. Plants were grown for 30 days before the roots were harvested for metagenomic analyses.
Rhizosphere soil samples were taken from three individual plants (one per pot) of each genotype. Rhizosphere (Rh) was defined as the soil still attached to the roots after shaking the roots by hand, thus separating off soil not adhering tightly to the roots. Each root system with closely adhering soil was transferred to a 250 ml sterile flask containing 150 ml sterile distilled water. The bottles were shaken for 15 min. The rhizosphere soil fraction from the supernatant was precipitated after centrifugation of the extract for 30 min at 6,000 rpm and then stored at −80°C.
The washed roots were transferred to new 50 ml tubes and rinsed once again with sterile water and a portion of these roots was then stored at −80°C. This sample (from now on called REE) constitutes root surface-bound (ectophytic, i.e., rhizoplane) and inside-root (endophytic) bacteria. The other portion of the washed roots (∼2 g) was transferred to a 15 ml falcon tube with 5 ml phosphate buffer (pH 8.0), 50 μl lysozyme (100 mg/ml), 0.5 U Chitinase, 5 μl RNAseA (100 mg/ml), 2 g sterile 710–1180 micron beads, 2 g sterile 212–300 micron glass beads and shook at 250 rpm for 30 min @ 37°C. These roots were then washed gently with sterile water and stored at −80°C. This root sample, which is enriched for the root internal (endophytic) microbiome, is called REN.
Amplification and Pyrosequencing
Each DNA sample was subjected to PCR with two sets of primers, each specific for amplification of rRNA sequences from either bacteria or archaea. For Illumina Miseq analyses, each metagenomic DNA sample used dual-indexed (Kozich et al., 2013) 16S rRNA primers to PCR amplify the V3–V4 region (Peiffer et al., 2013). The forward primer (5′–3′) had adapter sequence necessary for binding to the Illumina flow cell (underlined), i5 index sequence (x), binding sites for the Illumina sequencing primers (bold), two maximally degenerate bases (N) and conserved microbial primer 515F (caps) (aatgatacggcgaccaccgagatctacactctttccctXXXXXXXXacacgacgctcttccgatctNNGTGCCAGCMGCCGCGGTAA). Similarly, the reverse primers (5′–3′) had adapter sequences necessary for binding to the flow cell (underlined), i7 index sequence (X) to distinguish each sample, the Illumina sequencing primers (bold), and bacterial primer 806R (CAP) (caagcagaagacggcatacgagatXXXXXXgtgactggagttcagacgtgtgctcttccgatct GGACTACHVGGGTWTCTAAT). A complete list of unique index sequences is provided in Supplementary Table S1. The forward primer (5′–3′) to PCR amplify archaeal 16S rRNA gene sequence contained 454 Life Sciences primer B and conserved primer 109F sequences (ACKGCTCAGTAACACGT), while the reverse primer (GCCTTGCCAGCCCGCT XXXXXXXXXXX ACCGCGGCKGCTGGC) contained 454 Life Sciences primer A (underlined), a 11 base unique barcode (X) and the archaeal primer 529R (bold) for each sample. Further details regarding primers and barcodes used in bacterial and archaeal analysis are presented in Supplementary Table S2.
PCR reactions were performed in 50 μL with 100 ng of template DNA, 1 × Phusion High Fidelity Buffer (NEB, United States), 0.25 μM of each primer, 0.5 μM each dNTP, and 1U Phusion High-Fidelity Taq Polymerase (NEB, United States). Detailed information for primers and barcodes for each metagenomic sample is listed in Supplementary Table S2. PCR conditions were 98°C for 2 min; 26 cycles of 98°C for 10 s, 58°C for 10 s, 72°C for 30 s; with a final extension at 72°C for 10 min. Three replicate PCR reactions were performed for each sample with each primer pair. Replicate reactions were pooled and cleaned by using solid-phase reversible immobilization (SPRI) beads (Deangelis et al., 1995). DNA was quantified using a fluorometric kit (Quant-IT PicoGreen, Invitrogen). Equimolar quantities of each library (from 140 metagenomic sample libraries) were pooled. For Illumina Miseq, the 16S amplicon libraries were mixed up to 20% with Illumina-generated PhiX control libraries to artificially increase the genetic sequence diversity. The Illumina-MiSeq (PE250) run for the 16S amplicon library pool, and two runs of the Roche-454 sequencing of 16S archaeal amplicon libraries was performed at the Georgia Genomics and Bioinformatics Core (University of Georgia, Athens, GA, United States). Sequence data were submitted to Genbank under the Bioproject ID: PRJNA430270.
Sequence Analysis and Tree Construction
Illumina Miseq data were analyzed using MOTHUR MiSeq SOP1, whereas Roche-454 data were analyzed with MOTHUR 454 SOP2. Briefly, sequence datasets were trimmed, clustered and classified in MOTHUR (Schloss et al., 2009) according to the following parameters: minimum length = 200 bp, maximum length = 500 bp, average quality score = 25, nucleotide mismatches in the primer sequence = 0, the maximum number of Ns = 0. The trimmed sequences of bacterial and archaeal reads were further cleaned by removing chloroplast 16S rRNA reads and chimeric reads with remove.seq (using taxonomic classification) and UCHIME, respectively, in MOTHUR. Sequences were combined and aligned in MOTHUR using the full alignments of the rRNA small subunit sequences of the SILVA database as a template. The MOTHUR program was also used for preclustering, rarefaction analysis, distance calculations, clustering, and further analysis based on OTUs. A distance matrix was generated from the resulting sequences. Sequences were clustered into OTUs using the farthest-neighbor algorithm. Illumina 16S OTUs were further analyzed by retaining only abundant OTUs, defined as occurring >100 times in the entire dataset. For instance, a sample could have 101 reads in one sample, and it would be considered abundant, or it could have 21 reads in each of five samples, and would still be considered abundant. We rarified and normalized the reads by randomly subsampling 10,000 abundant reads from each sample. That is, differences in total read numbers between samples was not a factor in our analysis, so that all final values of OTU counts represent a percentage of an identical 10,000 reads from each sample.
Data Analysis
Bacterial and archaeal data were analyzed separately using the phylogenetic framework provided within the suite of tools in the MOTHUR and Fast UniFrac program (Schloss et al., 2009; Hamady et al., 2010). Alpha diversity values for bacterial and archaeal sequence data were estimated using the Shannon diversity index and rarefaction curves. Principal Coordinate Analysis (PCoA) was performed using the Fast UniFrac metric (Hamady et al., 2010) and visualized as the clustering of samples from different treatments (e.g., S. viridis vs. S. italica) by using the JMP Pro. Both UniFrac and AMOVA were used to compare the microbial communities. These two methods test different hypotheses and can result in different p-values. Taxonomy-based classification of bacterial and archaeal 16S rRNA gene sequences was obtained using the RDP taxonomy and Bayesian classifier (Wang et al., 2007; Cole et al., 2014).
The color-coded Clustered Image Maps (CIMs) (“heat maps”) were generated using CIMminer (Scherf et al., 2000). CIMminer utilizes a hierarchical clustering algorithm based on the average-linkage method of Sokal (1958). The OTU tables were represented graphically by coloring each cell on the basis of the abundance of a particular OTU across different samples. A dendrogram is appended to the colored table to indicate the nature of the computed relationship among OTU abundances in the table. Species- and root compartment-specific metagenomic biomarkers were identified using the linear discriminant analysis (LDA) effect size (LefSe) method (Segata et al., 2011). LefSe determines the OTUs most likely to explain differences between classes by coupling standard tests for statistical significance with additional tests that factor in biological constancy and relevance. LefSe first uses the non-parametric factorial Kruskal–Wallis (KW) sum-rank test to detect features with significant differential abundance with respect to the sample of interest. Biological consistency is subsequently investigated using a set of pairwise tests among species/compartments using the (unpaired) Wilcoxon rank-sum test (Wilcoxon, 1945; Mann and Whitney, 1947). As a last step, LEfSe employs LDA (Fisher, 1936) to estimate the effect size of each differentially abundant OTU.
Results
In our greenhouse experiments investigating Setaria growth in sterile and non-sterile soils, we have routinely observed seedling growth and root development differences between treatments and between S. italica and S. viridis responses (Supplementary Figure S1). In order to investigate the microbiomes of these Setaria, metagenome analyses were pursued.
In order to differentiate between very different below-ground plant::microbe interactions, all harvested root samples were used to generate three DNA sources. The first source was from soil washed from a root that had been pulled from the greenhouse pot (see section “Materials and Methods”). We call this sample the rhizosphere (Rh). The roots were separated into two aliquots, one treated with lysozyme and chitinase and the other untreated. The wash from the enzyme-treated roots did not produce any bacterial colonies on four different media that we tested. Thus, the root sample treatment should have removed most or all microbes on the root surface, and is thus called the root endophytic microbiome (REN). The untreated root sample is a mixture of root endophytes and ectophytes (attached to the root surface), so this sample is called REE.
We have generated Rh, REE, and REN samples from 18 plants of S. italica and 12 plants of S. viridis. Thus, a total of 90 sample types were collected from the greenhouse experiments. After DNA extraction, each sample is expected to contain both microbial genomic DNA and plant genomic DNA. Metagenomic analyses were then performed with either bacterial 16S ribosomal DNA primers or archaeal 16S ribosomal DNA primers. These primers were chosen because they do not extensively amplify host plant ribosomal DNA from either the organelles or the nucleus. These ribosomal DNA sequences were grouped into specific microbial operational taxonomic units (OTUs), which are equated with unique ecotypes when the degree of OTU similarity is >97%, which is a commonly used rule to define species by the metagenome research community (Mysara et al., 2017). Our first analyses were with sequences generated with 454 technology, but we then switched to Illumina data generation in order to get larger numbers of reads. The results shown are only for the Illumina data, but independent analyses of the two data sets yielded the same conclusions (data not shown), although these conclusions were less robust when only using the 454 data. OTU tables obtained from a total of 89 bacterial samples and 71 archaeal samples were used in the final analyses. The bacterial and archaeal OTU tables are shown in Supplementary Tables S3, S4.
Bacterial diversity was higher in the Rh than in the REE or REN compartments (Supplementary Table S5). We did not find a significant difference in the microbial diversity between S. italica and S. viridis samples (Supplementary Figure S2A). Rh samples have the most OTU types that were not found in other compartments (Supplementary Figures S2B,C). The root endophytic (REN) results have extensive overlap (that is, are a subset of) the Rh and REE samples. The differences between REE and REN results are the microbes tightly affixed to the root surface, an understudied component of the microbial soil::root interaction. To determine the microbes unique to the rhizoplane (“ectophytes”), we determined the abundant OTUs in both REE and REN compartments, and then found those that were unique to the REE. We found 25 abundant OTUs that are present only on the root surface (REE) of S. italica and 126 abundant OTUs that are present only in the REE compartment of S. viridis (Supplementary Figures S2B,C). We did not find any abundant genera in REN compartments of S. italica or S. viridis genotypes that were not also found in the REE samples. This was expected, given that OTUs in the REN samples (endophytes) should be a subset of the OTUs found in the REE (endophyte plus ectophyte) samples.
The PCoA graphs in Figure 1 shows a comparison of bacterial communities between the S. italica and S. viridis hosts in Rh, REE and REN compartments. Multiple genotypes of each species were used in the analysis, and three replicates of each genotype in most cases, so these results indicate differences that separate the domesticated plants from the wild plants. PCoA showed root compartment-specific and species-dependent separation. REE samples and REN samples showed significant species-dependent separation (Figure 1A). A separate analysis of only Rh samples (Figure 1B), only REE samples (Figure 1C) and only REN samples (Figure 1D) confirmed that both REE and REN samples showed significant species-dependent separation. A non-parametric analysis of variance (AMOVA) was performed to verify whether the differences in bacterial diversity between the groups (i.e., genotype, species, and root compartment) were different than within the groups. The result showed that there were statistically significant differences in bacterial diversity between species for REE and REN compartments (p-value ∼0.001). The analysis showed that the Rh compartment exhibits less significant host species-dependent microbial associations (p-value 0.046).
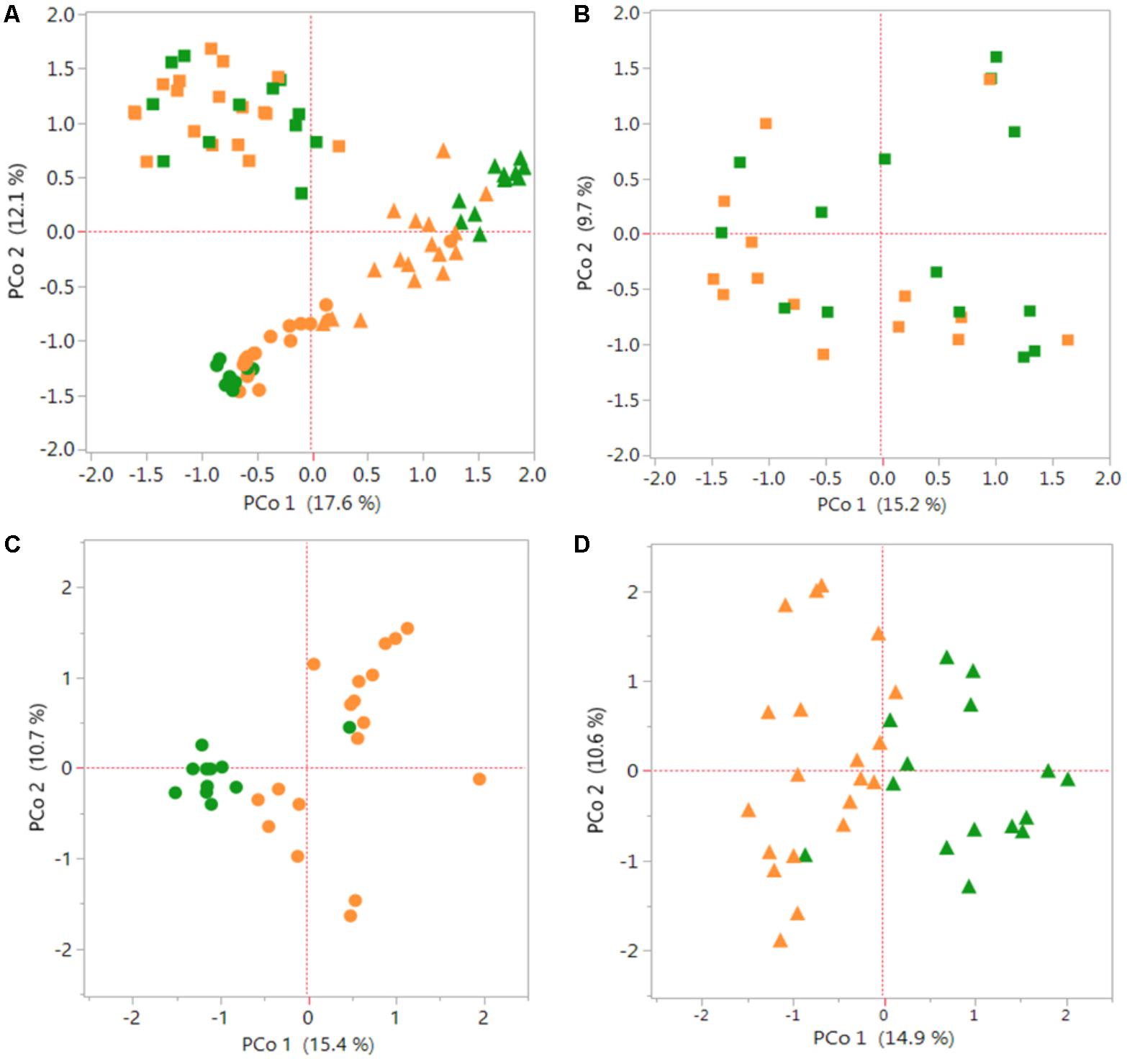
FIGURE 1. Species-dependent colonization of rhizosphere and root microbiomes in Setaria species. Bacterial OTUs of Setaria italica (orange) and S. viridis (green) were analyzed using Principal Coordinates Analysis (PCoA) plots generated from distance matrices for the Jaccard indices sets. The plot shows the first two principal axes. Rhizosphere (Rh) samples are shown as squares, root external plus root endophytic (REE) samples are shown as circles and root endophytic (REN) samples are shown as triangles. (A) Comparison of the three compartments across both species. (B–D) Figures are comparisons between species of the Rh, REE, and REN compartments individually. Non-parametric AMOVA to find significant differences between S. italica and S. viridis samples for each compartment produced p-values 0.056, 0.001, and 0.001 for Rh, REE, and REN samples, respectively.
The presence and the abundances of different phyla and genera were assessed by taxonomic assignment of all the abundant sequences using the RDP classifier, and comparative analysis was conducted using MOTHUR tools. A total of 693 bacterial genera belonging to 23 phyla were recognized by RDP taxonomy. Out of these, 366 genera from 23 phyla were represented by more than 100 reads in the entire Illumina dataset. The top three phyla of Proteobacteria (76%), Actinobacteria (11%) and Firmicutes (9%) contributed 96% of the total bacterial diversity. Among Proteobacteria, the subphylum Alphaproteobacteria were the most abundant in the rhizosphere soil. In contrast, Deltaproteobacteria were the most abundant in the REE samples and Gammaproteobacteria were most abundant in the REN samples. These general abundance characteristics are very similar to those seen with many other root::soil communities (Bulgarelli et al., 2015).
A comparative analysis of Rh samples from S. italica and S. viridis showed that Alphaproteobacteria were overrepresented in the S. italica rhizosphere, whereas Gammaproteobacteria, Deltaproteobacteria, and Firmicutes were overrepresented in the S. viridis rhizosphere soil (Figure 2). The REE fractions of S. italica roots were richer in Betaproteobacteria and Firmicutes than S. viridis roots. The REE fraction of S. viridis roots were found to be enriched with Deltaproteobacteria and Actinobacteria (Figure 2). The root endophyte compartment (REN) of S. italica was richer in Betaproteobacteria and Firmicutes compared to S. viridis. In contrast, the REN samples of S. viridis were enriched for Gammaproteobacteria (Figure 2). Sixteen genera (Actinotalea, Algiphilus, Basilea, Caldivirga, Carnimonas, Ewingella, Hungatella, Mangrovibacter, Microvirga, Mitsuaria, Oxalicibacterium, Sphingobacterium, Succinivibrio, Taonella, Telmatocola, Xylophilus), all abundantly present in rhizosphere soil, were completely absent in REE and REN compartments of both S. italica and S. viridis. Twelve genera (Actinomyces, Desulfobaculum, Thiohalobacter, Polaromonas, Hamadaea, Edwardsiella, Peredibacter, Brevibacillus, Rivibacter, Thermocladium, Ochrobactrum, Mizugakiibacter) that were abundant in REE compartments of both species were absent in the endophytic REN compartment.
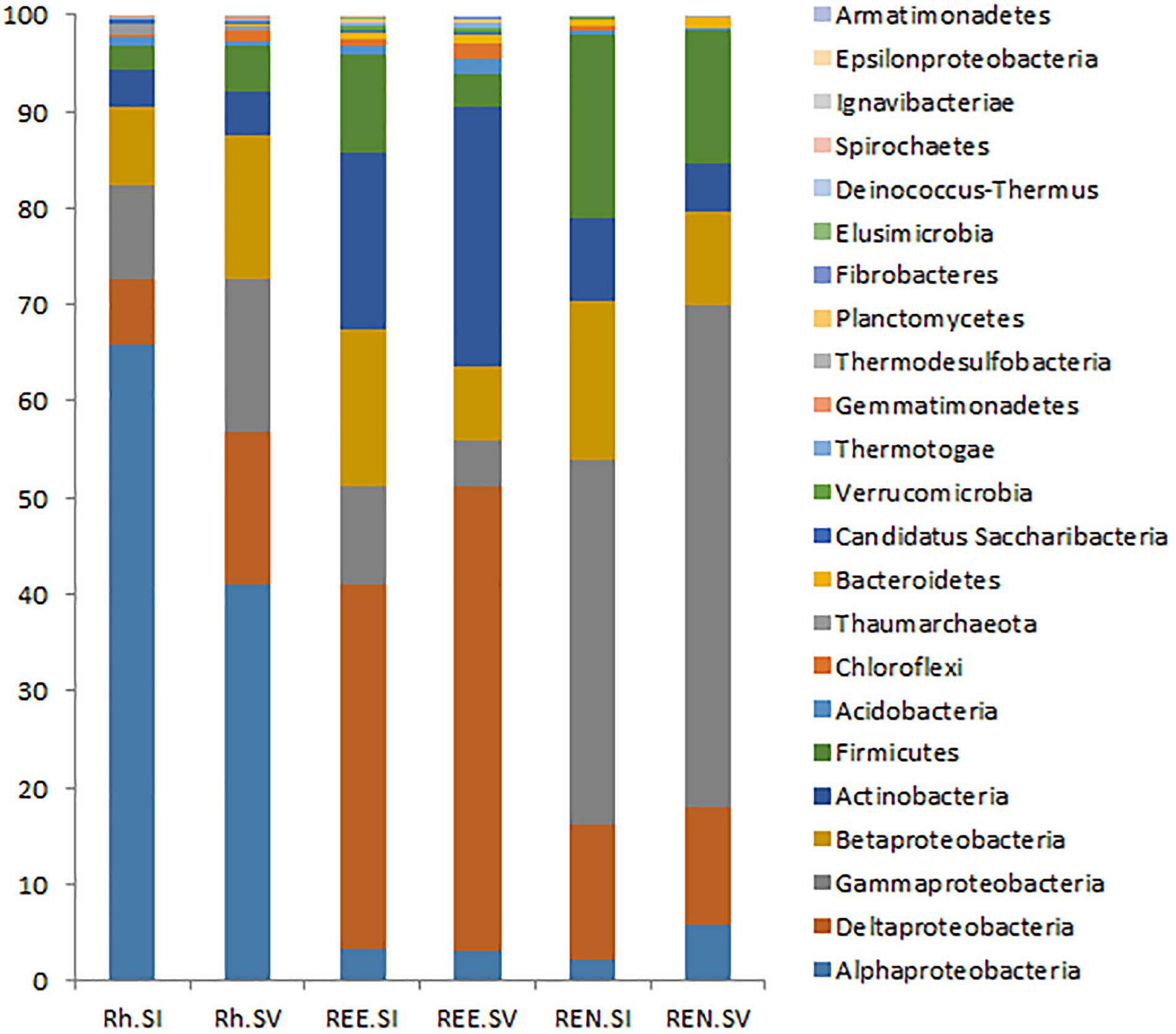
FIGURE 2. Relative abundance of bacterial phyla in the rhizosphere microbes (Rh), root external and endophytic microbes (REE) and root endophytic microbes (REN). The averages of each phylum for all S. italica samples (SI) and all S. viridis samples (SV) were shown as two bars for Rh, REE, and REN.
In order to characterize and depict the specific bacterial communities that are dominating these microbiomes, a heat map analysis is presented for the most abundant OTUs in the Rh, REE, and REN samples (Figure 3). We have also analyzed significant OTUs in each cluster by the LefSe method that predicts OTUs that are statistically different among biological samples. The significant OTUs in each heat map community are listed in the Supplementary Table S6.
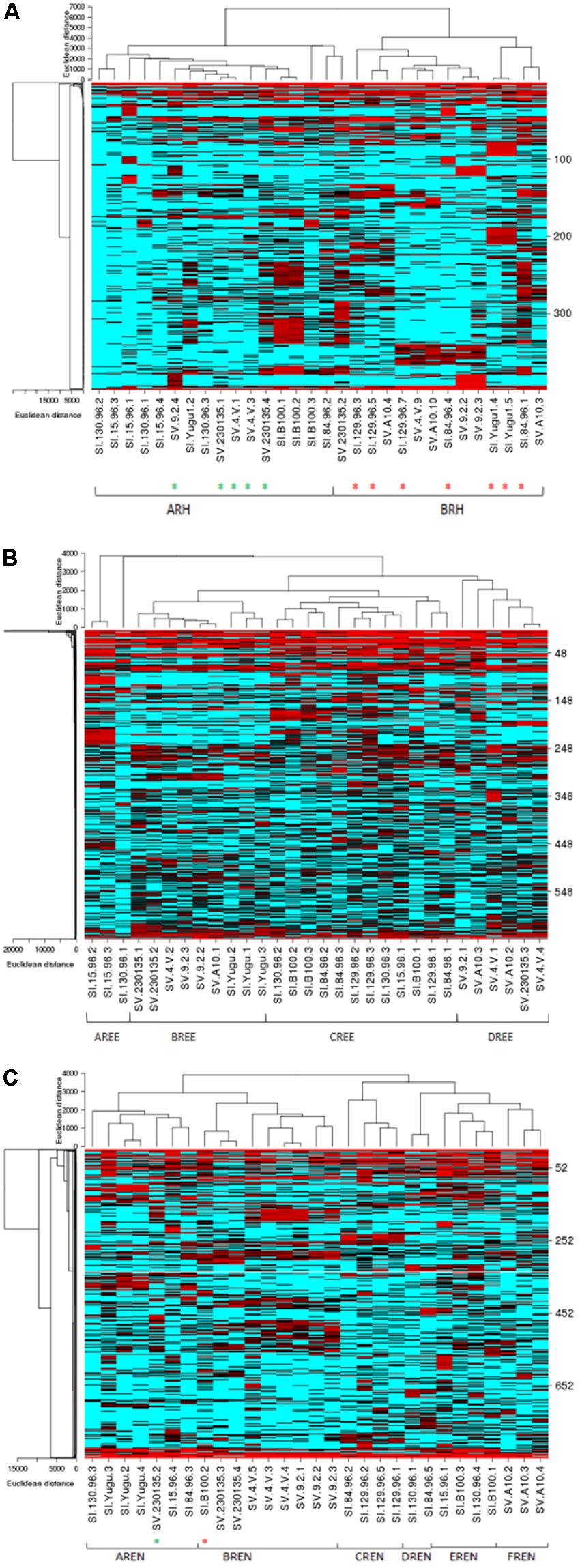
FIGURE 3. Species-dependent colonization of the rhizosphere (Rh) microbiome in Setaria species. Heat maps showing relative abundances of the most abundant bacterial OTUs in the Rh (A), REE (B), and REN (C) compartments (Y-axis) versus the Setaria genotypes (X-axis) used in the current study. Red indicates high abundance and blue indicates low abundance. The Setaria sample designations are on the bottom. The green stars at the bottom indicate S. viridis samples in an otherwise S. italica cluster and red stars indicate S. viridis samples in an otherwise S. viridis cluster. The p-values based on the Analysis of Molecular Variation (AMOVA) are 0.046∗, 0.001∗, and 0.001∗ for Rh, REN, and REE, respectively.
The heat map analyses indicated two highly separable species-dependent microbiome communities in Rh samples (Figure 3A). The ARH community predominantly has S. italica samples with three S. viridis samples. The BRH community, which is found on most of the S. viridis samples, is also found on five S. italica samples. The OTUs coded as 1 (Sphingomonas), 41 (Stakelama), 67 (Sphingomonas), 126 (Mesoaciditoga), 128 (Stakelama), and 171 (Stakelama) in our analysis are significantly enriched in the ARH community compared to the BRH community, whereas 2 (Lawsonia), 3 (Leclercia), 5 (Bhargavaea), 6 (Pseudomonas) and 7 (Massilia) are most abundant in the BRH community.
The REE samples demonstrate four highly separable microbiome community types (Figure 3B). Each REE microbial community was completely species-dependent, with AREE and CREE communities only found with S. italica germplasm and BREE and DREE communities existing only with S. viridis roots. All of the replicates of four S. italica genotypes (Yugu1, B100, 84-96, and 129-96) were associated with the CREE community. All replicates of one S. viridis (A10) genotype were associated with the DREE community (Figure 3B). Some genotypes of S. italica and S. viridis associated with more than one type of microbial community. AREE has abundances of OTUs 27 (Hathewaya), 51 (Rhizomicrobium), 63 (Clostridiaceae), 111 (Treponema), and 142 (Azospira). BREE has a high proportion of OTUs 2 (Lawsonia), 220 (Gimesia), 776 (Aquabacterium), 1430 (Aquabacterium), and 1449 (Pseudorhodoferax), while CREE has high abundance of 46 (Sporomusa), 113 (Succinivibrio), 114 (Anaerobacterium), 159 (Ideonella) and 183 (Anaerospora). DREE contains high abundances of OTUs 9 (Kitasatospora), 35 (Haliangium), 492 (Actinosynnema), 614 (Kallotenue), and 786 (Acidobacteria).
The REN samples also have six distinct microbial communities, with AREN and CREN, DREN, and EREN within the roots of S. italica samples and BREN and FREN communities inside the roots of S. viridis samples (Figure 3C). All three replicates of Yugu1 had the AREN community and all the three replicates of SV4-2 and 9-2 have BREN communities, while A10 roots hosted the FREN microbial community. AREN exhibits over-representation of 6 (Pseudomonas), 10 (Exiguobacterium), 47 (Pseudocitrobacter), 62 (Niastella), and 72 (Azorhizophilus).BREN has overrepresentation of OTUs 1 (Sphingomonas), 3 (Leclercia), 73 (Luteibacter), 83 (Achromobacter), and 286 (Siccibacter), CREN has overrepresentation of 21 (Tumebacillus), 25 (Janthinobacterium), 37 (Methylohalobius), 318 (Cedecea), and 376 (Massilia), DREN has overrepresentation of 2 (Lawsonia), 4 (Amycolatopsis), 14 (Byssovorax), 17 (Povalibacter), 23 (Mobilitalea), EREN has significantly high proportion of OTUs 31 (Serpens), 86 (Sulfuritalea), 119 (Acidobacteria), 162 (Serpens), and 212 (Leclercia), while FREN has over abundance of OTUs 278 (Enterobacter), 674 (Defluviitoga), and 682 (Puniceicoccus).
The OTU results were further analyzed to identify species-specific and compartment-specific biomarkers using the LefSe method. Six distintive (“biomarker”) OTUs were found for S. italica Rh, 14 for S. viridis Rh, 80 for S. italica REE, and 69 for S. viridis REE. In addition 51 biomarkers were found for the S. italica REN compartment, and 141 for the S. viridis REN compartment. The lists of significant OTUs are presented in Supplementary Table S7. The relative abundances of some of these exemplar biomarker OTUs are presented in Figure 4.
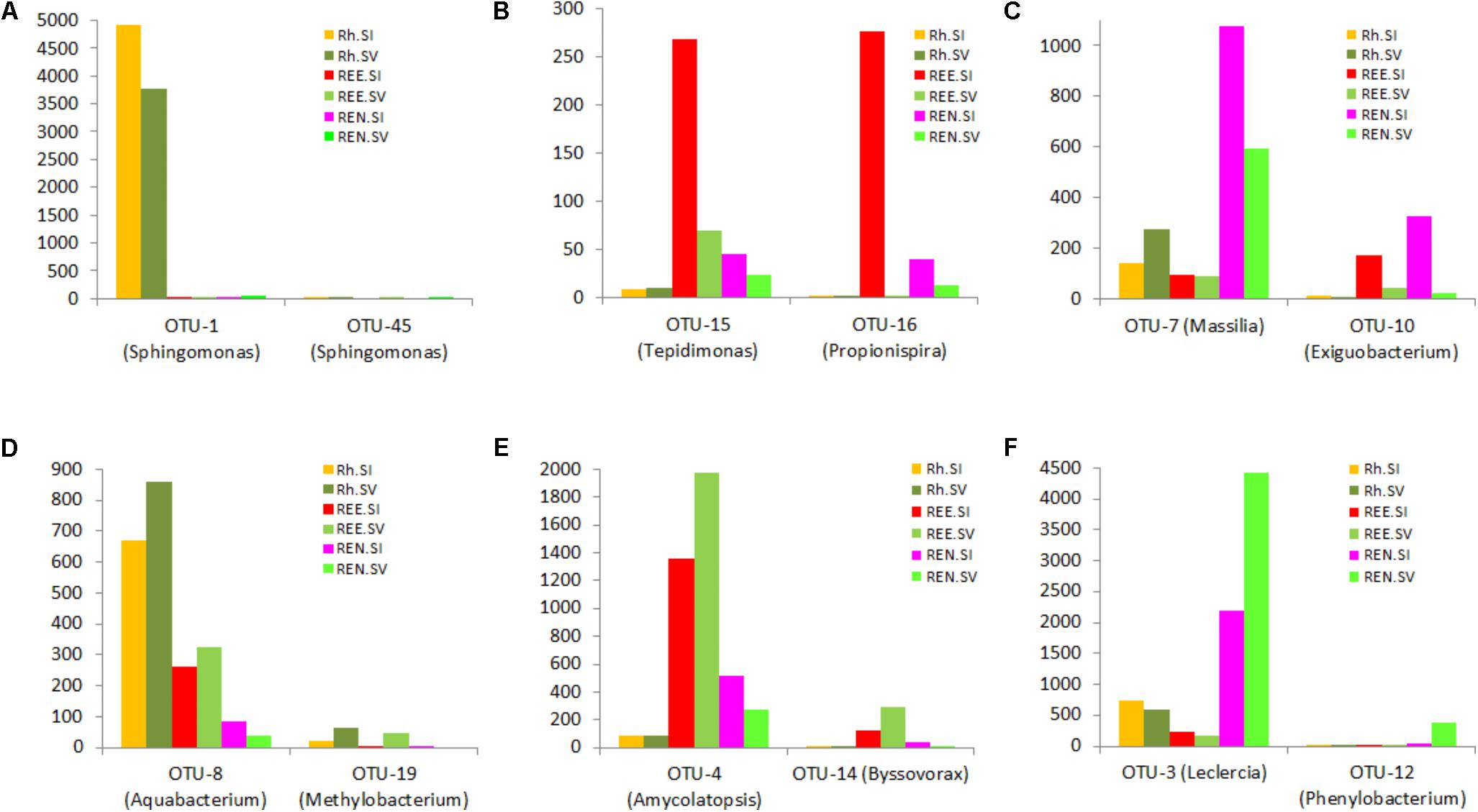
FIGURE 4. Species- and root compartment-specific significant OTUs were identified using the linear discriminant analysis (LDA) effect size (LefSe) method. A list of significant OTUs is presented in the Supplementary Data. This figure shows relative abundance of some exemplar biomarkers for S. italica Rh (A), REE (B), and REN (C) compartments and S. viridis Rh (D), REE (E), and REN (F).
The archaeal diversity was estimated in the Rh, REE and REN compartments of S. italica and S. viridis genotypes (Supplementary Table S8). S. italica roots have more archaea than S. viridis roots in all the compartments (Supplementary Figure S3). Figure 5 presents the archaeal analyses on these same Rh and REN samples. PCoA demonstrated that the separation by domesticated versus wild Setaria genotypes in these experiments is more distinct in REN samples (Figure 5B) than in Rh or REE compartments (Figure 5A and Supplementary Figure S4A). Analysis of Rh and REN samples also indicated that the archaea predominantly belong to the phylum Euryarchaeota, with lower quantities of Thaumarchaeota. Euryarchaeota classes Methanomicrobia, Methanobacteria and an unknown class of Euryarchaeota constituted 80–90% of the Rh and REN archaea of both Setaria species (Figure 5C and Supplementary Figure S4B). Thaumarchaeota classes were represented by Nitrososphaerales and Nitrosopumilales.
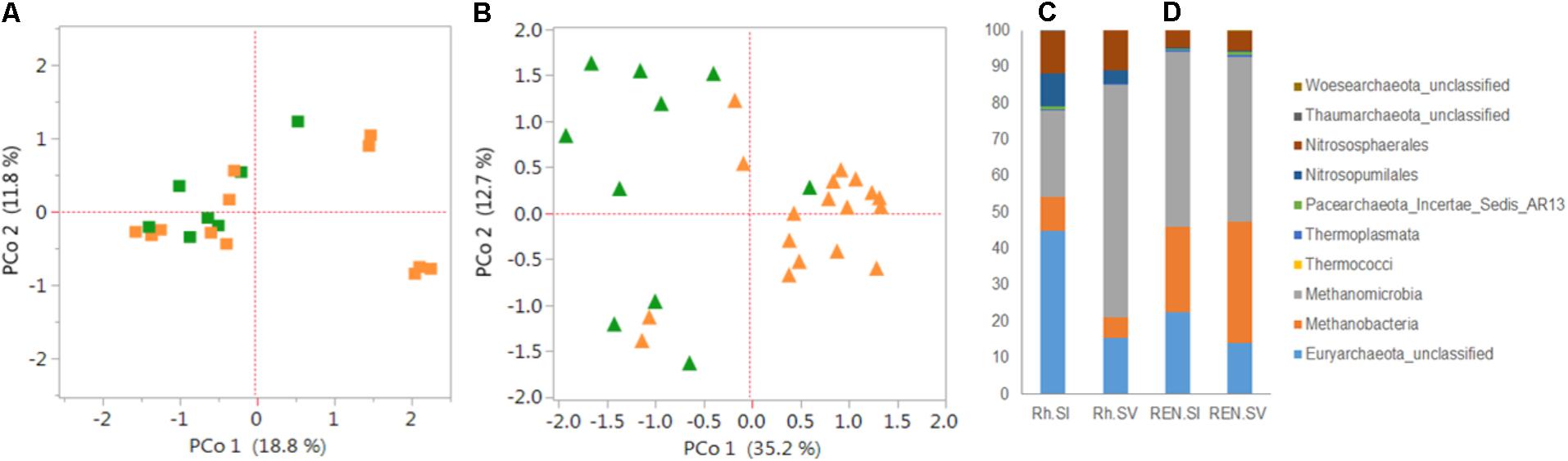
FIGURE 5. Diversity and composition of archaea in the roots and rhizospheres of S. italica and S. viridis. PCoA plots were based on 16S archaeal rRNA gene sequences for (A) rhizosphere microbiome (Rh) and (B) root endophytic microbiome (REN). The distance matrices were calculated from the Jaccard coefficients using Bray–Curtis indices. P-values calculated for the significance of the differences between S. italica and S. viridis in there results were based on unweighted UniFrac distances. The p-values were 0.12 and 0.016 for Rh and REN samples, respectively. The composition of archaeal classes for Rh samples (C) and REN samples (D) of S. italica and S. viridis.
Discussion
Domestication of monocotyledonous crops resulted in changes in several traits, including seed size, seed yield, seed dispersal, determinate growth, loss of seed dormancy, and changes in photoperiod sensitivity (Doebley et al., 2006; Cockram et al., 2007; Zhou et al., 2015). Although these were all knowingly selected, there are no reports of selecting root architecture during domestication. However, there is evidence for an unknowing selection for less root biomass in domesticated wheat (Waines and Ehdaie, 2007), and decreases in the capacity for root plasticity under selection pressures in barley and foxtail millet (Grossman and Rice, 2012; Sebastian et al., 2016). Setaria italica is believed to have been domesticated from S. viridis in Northern China around 6000 BC (Bettinger et al., 2010), probably prior to rice domestication. It has been shown that some types of roots, such as the crown roots that originate from the shoot, show reduced sensitivity to water deficit in S. italica than in S. viridis, suggesting that this response has been influenced by human selection (Sebastian et al., 2016). Hence, there is a precedent for the idea that domestication can influence root traits.
Many factors are known and/or expected to influence the microbial communities associated with plant roots. The environment plays a major factor, especially soil chemical, structural and organic composition (Girvan et al., 2003; Fierer and Jackson, 2006). Cultivation history is also known to influence subsequent microbial communities, as simply exemplified by the abundance of rhizobiales and suppression of nitrifying microbial communities that are observed in a field that has recently supported a crop of legumes (Sugiyama et al., 2014; Paungfoo-Lonhienne et al., 2017). Soil composition can vary across very small scales, due to topographic issues like slope and altitude (Robertson et al., 1993; Kravchenko et al., 2005; Garcia-Palacios et al., 2012; Tamme et al., 2016). Hence, greenhouse experiments with controlled growth, soil and microbial composition conditions should offer substantial advantages for the generation of reproducible results. However, greenhouses are not as uniform as often assumed, particularly regarding edge issues of shading, distance to ventilation, local humidity variation and distances to exterior walls (and, thus, temperature variation), although these issues can be minimized by frequent pot translocation (Brien et al., 2013; Kutta and Hubbart, 2014). The greenhouse pot experiments in this study exhibited substantial environmental variation, as shown by differences in the replicates, but still yielded consistent signals regarding the differences between the microbiomes of domesticated versus wild Setaria prokaryotic ectophytes and endophytes. The higher abundance of Betaproteobacteria and Firmicutes in the REE and REN compartments of S. italica compared to S. viridis are clear examples. In addition, many significant species-specific OTUs belonging to these phyla were identified in the LefSe analyses.
Some of the different Rh, REE, and REN communities generated in this project show little to no overlap in their predominant community members, despite initiation of the community by identical microbial community inocula. Hence, the observed communities do not represent continuums, but rather the establishment of a defined community that show varying degrees of preference for a particular host genotype.
The microbial diversity in the REE and REN compartments showed substantial OTU overlap with the microbial communities in the Rh compartment, confirming the expectation that the REE and REN communities arising from a soil inoculation will be a subsample of Rh communities. Though seeds of all the genotypes in the current study were multiplied in the same potting mix in the same greenhouse, we cannot rule out the possibility that seed-transmitted microbes may have founded differences in the endophytic communities that were represented in the seedling root. However, previous work indicated that seed endophytic communities are distinctly different from rhizosphere or root microbial communities (Philippot et al., 2013; Leff et al., 2017).
There are several studies that have shown that the plant host genotype has a small but significant impact on the composition of an associated microbiome (Peiffer et al., 2013; Edwards et al., 2015). The host plant acquires its microbiome from the surrounding environments, but selects only a tiny subset of available species for associated growth. Soil type, biological history, water quality, water abundance and several other factors have important impacts on the microbes present as well. Establishing a novel microbiome takes time, so that greenhouse experiments on seedlings, especially on tiny seedlings like those of S. viridis and S. italica, are likely to show the smallest differentiation from the inoculum and the smallest host-specific effects. For instance, the host genotype-dependence of the microbiome was less apparent in a tiny annual such as Arabidopsis (Lundberg et al., 2012) than in a larger perennial plant, Boechera stricta (Wagner et al., 2016). Hence, we believe that the differences seen in our experiments would be more dramatic with larger and older plants than in these seedlings.
There are few studies that have directly assessed the possible effects of human agricultural intervention on the microbial communities in the roots. It was shown in wheat and maize that the root and rhizosphere bacterial communities are more diverse in land races than in the modern cultivars (Germida and Siciliano, 2001; Szoboszlay et al., 2015). However, it was shown in sunflower that there was less fungal diversity in the wild germplasm compared to domesticated genotypes (Leff et al., 2017). Leff et al. (2017) argued that the domestication of sunflowers may have decreased the prevalence of pathogens associated with the plants and may have increased the prevalence of symbionts. Our results suggest that the Betaproteobacteria, which include most of the nitrogen fixers in nature (Chen et al., 2003; Santi et al., 2013), are more abundant in the REE and REN compartments of the domesticated species, S. italica. Though we did not observe dramatic difference in the over-all microbial diversity between S. italica and S. viridis, we observed more microbial phylotypes specific to S. viridis than to S. italica. This could be a reflection of the broader adaptability of S. viridis, one of the two or three most widely distributed weeds on the planet, than seen for S. italica, which was domesticated and primarily grown in only one region of China. All crop domestication and improvement are expected to be associated with the narrowing of germplasm, so this phenomenon would also predict less diversity of all kinds (including the associated microbiome) in an elite crop.
Previous studies have postulated that ancient cultivars and their wild relatives were generally exposed to more marginal soils before the invention of synthetic fertilizer–driven agricultural production, and their gene pools might have a different adaptive capacity to engage in novel microbial associations with rhizosphere microbes compared with the gene pools of present-day cultivars (Wissuwa et al., 2009; Bulgarelli et al., 2015). These observations suggest that identification of the alleles in S. viridis that promote root::soil microbe diversity might also contribute to improved adaptation of the crop foxtail millet to diverse environments. Mapping and introgression of the Setaria genes responsible for these root::soil microbe differences will be needed to test this hypothesis.
Conclusion
This study demonstrates that host genetics, including the outcomes of crop domestication, can play an important role in selecting the prokaryotes present in the plant::soil interaction network. The communities established in the rhizosphere, root surface and endophytic space are very different, but are all affected by both host genetics and the plant microenvironment. Now that these differences have been observed, future field experiments and experiments with controlled innocula can be used to determine the contributions of individual microbial species to the community and to plant performance.
Author Contributions
JB designed the experiments, analyzed the data, and wrote the manuscript. SC designed and conducted the experiments, analyzed the data, and wrote the manuscript.
Funding
This research was funded by The BioEnergy Science Center (Department of Energy, United States), endowment funds from the University of Georgia Giles Professorship and the Georgia Research Alliance.
Conflict of Interest Statement
The authors declare that the research was conducted in the absence of any commercial or financial relationships that could be construed as a potential conflict of interest.
Acknowledgments
The authors thank Prof. Katrien Devos for providing Setaria seed. They also thank Jeff Wagner and Myriam Belanger at the Georgia Genomics Facility at the University of Georgia for assistance with 454 and Illumina sequencing. Roche 454 and Illumina sequence data are deposited in the NCBI Genbank (Bioproject ID. PRJNA430270).
Supplementary Material
The Supplementary Material for this article can be found online at: https://www.frontiersin.org/articles/10.3389/fpls.2018.01183/full#supplementary-material
Footnotes
References
Bennetzen, J. L., Schmutz, J., Wang, H., Percifield, R., Hawkins, J., Pontaroli, A. C., et al. (2012). Reference genome sequence of the model plant Setaria. Nat. Biotechnol. 30, 555–561. doi: 10.1038/nbt.2196
Berendsen, R. L., Pieterse, C. M. J., and Bakker, P. A. (2012). The rhizosphere microbiome and plant health. Trends Plant Sci. 17, 478–486. doi: 10.1016/j.tplants.2012.04.001
Bettinger, R. L., Barton, L., and Morgan, C. (2010). The origins of food production in North China: a different kind of agricultural revolution. Evol. Anthropol. 19, 9–21. doi: 10.1002/evan.20236
Bonfante, P., and Genre, A. (2010). Mechanisms underlying beneficial plant-fungus interactions in mycorrhizal symbiosis. Nat. Commun. 1:48. doi: 10.1038/ncomms1046
Brader, G., Compant, S., Vescio, K., Mitter, B., Trognitz, F., Ma, L. J., et al. (2017). Ecology and genomic insights into plant-pathogenic and plant-nonpathogenic endophytes. Annu. Rev. Phytopathol. 55, 61–83. doi: 10.1146/annurev-phyto-080516-035641
Brien, C. J., Berger, B., Rabie, H., and Tester, M. (2013). Accounting for variation in designing greenhouse experiments with special reference to greenhouses containing plants on conveyor systems. Plant Methods 9:5. doi: 10.1186/1746-4811-9-5
Brutnell, T. P., Bennetzen, J. L., and Vogel, J. P. (2015). Brachypodium distachyon and Setaria viridis: model genetic systems for the grasses. Annu. Rev. Plant Biol. 66, 465–485. doi: 10.1146/annurev-arplant-042811-105528
Bulgarelli, D., Garrido-Oter, R., Munch, P. C., Weiman, A., Droge, J., Pan, Y., et al. (2015). Structure and function of the bacterial root microbiota in wild and domesticated barley. Cell Host Microbe 17, 392–403. doi: 10.1016/j.chom.2015.01.011
Cao, Y., Halane, M. K., Gassmann, W., and Stacey, G. (2017). The role of plant innate immunity in the legume-rhizobium symbiosis. Annu. Rev. Plant Biol. 68, 535–561. doi: 10.1146/annurev-arplant-042916-041030
Chen, W.-M., Moulin, L., Bontemps, C., Vandamme, P., Béna, G., and Boivin-Masson, C. (2003). Legume symbiotic nitrogen fixation by β-proteobacteria is widespread in nature. J. Bacteriol. 185, 7266–7272. doi: 10.1146/annurev-arplant-042916-041030
Chun, S. C., Schneider, R. W., and Cohn, M. A. (1997). Sodium hypochlorite: effect of solution pH on rice seed disinfestation and its direct effect on seedling growth. Plant Dis. 81, 821–824. doi: 10.1128/JB.185.24.7266-7272.2003
Cockram, J., Jones, H., Leigh, F. J., O’sullivan, D., Powell, W., Laurie, D. A., et al. (2007). Control of flowering time in temperate cereals: genes, domestication, and sustainable productivity. J. Exp. Bot. 58, 1231–1244. doi: 10.1094/PDIS.1997.81.7.821
Cole, J. R., Wang, Q., Fish, J. A., Chai, B. L., Mcgarrell, D. M., Sun, Y. N., et al. (2014). Ribosomal database project: data and tools for high throughput rRNA analysis. Nucleic Acids Res. 42, D633–D642. doi: 10.1093/nar/gkt1244
Coskun, D., Britto, D. T., Shi, W. M., and Kronzucker, H. J. (2017). How plant root exudates shape the nitrogen cycle. Trends Plant Sci. 22, 661–673. doi: 10.1016/j.tplants.2017.05.004
Deangelis, M. M., Wang, D. G., and Hawkins, T. L. (1995). Solid-phase reversible immobilization for the isolation of PCR products. Nucleic Acids Res. 23, 4742–4743. doi: 10.1016/j.tplants.2017.05.004
Delmont, T. O., Robe, P., Cecillon, S., Clark, I. M., Constancias, F., Simonet, P., et al. (2011). Accessing the soil metagenome for studies of microbial diversity. Appl. Environ. Microbiol. 77, 1315–1324. doi: 10.1128/AEM.01526-10
Devos, K. M., Wang, Z. M., Beales, J., Sasaki, Y., and Gale, M. D. (1998). Comparative genetic maps of foxtail millet (Setaria italica) and rice (Oryza sativa). Theor. Appl. Genet. 96, 63–68. doi: 10.1111/tpj.12842
Doebley, J. (2006). Plant science - Unfallen grains: how ancient farmers turned weeds into crops. Science 312, 1318–1319. doi: 10.1111/tpj.12842
Doebley, J. F., Gaut, B. S., and Smith, B. D. (2006). The molecular genetics of crop domestication. Cell 127, 1309–1321. doi: 10.1126/science.1128836
Edwards, J., Johnson, C., Santos-Medellin, C., Lurie, E., Podishetty, N. K., Bhatnagar, S., et al. (2015). Structure, variation, and assembly of the root-associated microbiomes of rice. Proc. Natl. Acad. Sci. U.S.A. 112, E911–E920. doi: 10.1073/pnas.1414592112
Fierer, N., and Jackson, R. B. (2006). The diversity and biogeography of soil bacterial communities. Proc. Natl. Acad. Sci. U.S.A. 103, 626–631. doi: 10.1128/AEM.00358-07
Fisher, R. A. (1936). The use of multiple measurements in taxonomic problems. Ann. Hum. Genet. 7, 179–188. doi: 10.1073/pnas.0507535103
Garcia-Palacios, P., Maestre, F. T., Bardgett, R. D., and De Kroon, H. (2012). Plant responses to soil heterogeneity and global environmental change. J. Ecol. 100, 1303–1314. doi: 10.1111/j.1469-1809.1936.tb02137.x
Germida, J., and Siciliano, S. (2001). Taxonomic diversity of bacteria associated with the roots of modern, recent and ancient wheat cultivars. Biol. Fert. Soils 33, 410–415. doi: 10.1111/j.1365-2745.2012.02014.x
Girvan, M. S., Bullimore, J., Pretty, J. N., Osborn, A. M., and Ball, A. S. (2003). Soil type is the primary determinant of the composition of the total and active bacterial communities in arable soils. Appl. Environ. Microbiol. 69, 1800–1809. doi: 10.1007/s003740100343
Goodrich, J. K., Davenport, E. R., Waters, J. L., Clark, A. G., and Ley, R. E. (2016). Cross-species comparisons of host genetic associations with the microbiome. Science 352, 532–535. doi: 10.1126/science.aad9379
Grossman, J. D., and Rice, K. J. (2012). Evolution of root plasticity responses to variation in soil nutrient distribution and concentration. Evol. Appl. 5, 850–857. doi: 10.1111/j.1752-4571.2012.00263.x
Hacquard, S., Spaepen, S., Garrido-Oter, R., and Schulze-Lefert, P. (2017). Interplay between innate immunity and the plant microbiota. Annu. Rev. Phytopathol. 55, 565–589. doi: 10.1146/annurev-phyto-080516-035623
Hamady, M., Lozupone, C., and Knight, R. (2010). Fast UniFrac: facilitating high-throughput phylogenetic analyses of microbial communities including analysis of pyrosequencing and PhyloChip data. ISME J. 4, 17–27. doi: 10.1038/ismej.2009.97
Handelsman, J., Rondon, M. R., Brady, S. F., Clardy, J., and Goodman, R. M. (1998). Molecular biological access to the chemistry of unknown soil microbes: a new frontier for natural products. Chem. Biol. 5, R245–R249. doi: 10.1038/ismej.2009.97
Hugenholtz, P., Goebel, B. M., and Pace, N. R. (1998). Impact of culture-independent studies on the emerging phylogenetic view of bacterial diversity. J. Bacteriol. 180, 4765–4774. doi: 10.1016/S1074-5521(98)90108-9
Jin, T., Wang, Y., Huang, Y., Xu, J., Zhang, P., Wang, N., et al. (2017). Taxonomic structure and functional association of foxtail millet root microbiome. Gigascience 6, 1–12. doi: 10.1093/gigascience/gix089
Konishi, S., Izawa, T., Lin, S. Y., Ebana, K., Fukuta, Y., Sasaki, T., et al. (2006). An SNP caused loss of seed shattering during rice domestication. Science 312, 1392–1396. doi: 10.1126/science.1126410
Kozich, J. J., Westcott, S. L., Baxter, N. T., Highlander, S. K., and Schloss, P. D. (2013). Development of a dual-index sequencing strategy and curation pipeline for analyzing amplicon sequence data on the MiSeq Illumina sequencing platform. Appl. Environ. Microbiol. 79, 5112–5120. doi: 10.1128/AEM.01043-13
Kravchenko, A. N., Robertson, G. P., Thelen, K. D., and Harwood, R. R. (2005). Management, topographical, and weather effects on spatial variability of crop grain yields. Agron. J. 97, 514–523. doi: 10.2134/agronj2005.0514
Kutta, E., and Hubbart, J. (2014). Improving understanding of microclimate heterogeneity within a contemporary plant growth facility to advance climate control and plant productivity. J. Plant Sci. 2, 167–178. doi: 10.11648/j.jps.20140205.14
Kuzyakov, Y., and Domanski, G. (2000). Carbon input by plants into the soil. J. Plant Nutr. Soil Sci. 163, 421–431. doi: 10.1002/1522-2624(200008)163:4<421::AID-JPLN421>3.0.CO;2-R
Leff, J. W., Lynch, R. C., Kane, N. C., and Fierer, N. (2017). Plant domestication and the assembly of bacterial and fungal communities associated with strains of the common sunflower. Helianthus annuus. New Phytol. 214, 412–423. doi: 10.1111/nph.14323
Levy, A., Gonzalez, I. S., Mittelviefhaus, M., Clingenpeel, S., Paredes, S. H., Miao, J. M., et al. (2018). Genomic features of bacterial adaptation to plants. Nat. Genet. 50, 138–150. doi: 10.1038/s41588-017-0012-9
Lopez-Raez, J. A., Shirasu, K., and Foo, E. (2017). Strigolactones in plant interactions with beneficial and detrimental organisms: the yin and yang. Trends Plant Sci. 22, 527–537. doi: 10.1016/j.tplants.2017.03.011
Lundberg, D. S., Lebeis, S. L., Paredes, S. H., Yourstone, S., Gehring, J., Malfatti, S., et al. (2012). Defining the core Arabidopsis thaliana root microbiome. Nature 488, 86–90. doi: 10.1038/nature11237
Mann, H. B., and Whitney, D. R. (1947). On a test of whether one of two random variables is stochastically larger than the other. Ann. Math. Stat. 18, 50–60. doi: 10.1214/aoms/1177730491
Mauro-Herrera, M., Wang, X. W., Barbier, H., Brutnell, T. P., Devos, K. M., and Doust, A. N. (2013). Genetic control and comparative genomic analysis of flowering time in Setaria (Poaceae). G3 3, 283–295. doi: 10.1534/g3.112.005207
McNear, D. H. (2012). The rhizosphere - roots, soil and everything in between. Nat. Educ. Knowl. 4:14. doi: 10.1093/aob/mcs061
Mendes, R., Garbeva, P., and Raaijmakers, J. M. (2013). The rhizosphere microbiome: significance of plant beneficial, plant pathogenic, and human pathogenic microorganisms. FEMS Microbiol. Rev. 37, 634–663. doi: 10.1111/1574-6976.12028
Muyzer, G., and Smalla, K. (1998). Application of denaturing gradient gel electrophoresis (DGGE) and temperature gradient gel electrophoresis (TGGE) in microbial ecology. Antonie Van Leeuwenhoek 73, 127–141. doi: 10.1023/A:1000669317571
Mysara, M., Vandamme, P., Props, R., Kerckhof, F. M., Leys, N., Boon, N., et al. (2017). Reconciliation between operational taxonomic units and species boundaries. FEMS Microbiol. Ecol. 93:fix029. doi: 10.1093/femsec/fix029
Niu, B., Paulson, J. N., Zheng, X. Q., and Kolter, R. (2017). Simplified and representative bacterial community of maize roots. Proc. Natl. Acad. Sci. U.S.A. 114, E2450–E2459. doi: 10.1073/pnas.1616148114
Pace, N. R., Stahl, D. A., Lane, D. J., and Olsen, G. J. (1985). Analyzing natural microbial populations by rRNA sequences. ASM News 51, 4–12.
Parks, D. H., Rinke, C., Chuvochina, M., Chaumeil, P. A., Woodcroft, B. J., Evans, P. N., et al. (2017). Recovery of nearly 8,000 metagenome-assembled genomes substantially expands the tree of life. Nat. Microbiol. 2, 1533–1542. doi: 10.1038/s41564-017-0012-7
Paungfoo-Lonhienne, C., Wang, W., Yeoh, Y. K., and Halpin, N. (2017). Legume crop rotation suppressed nitrifying microbial community in a sugarcane cropping soil. Sci. Rep. 7:16707. doi: 10.1038/s41598-017-17080-z
Peiffer, J. A., Spor, A., Koren, O., Jin, Z., Tringe, S. G., Dangl, J. L., et al. (2013). Diversity and heritability of the maize rhizosphere microbiome under field conditions. Proc. Natl. Acad. Sci. U.S.A. 110, 6548–6553. doi: 10.1073/pnas.1302837110
Philippot, L., Raaijmakers, J. M., Lemanceau, P., and Van Der Putten, W. H. (2013). Going back to the roots: the microbial ecology of the rhizosphere. Nat. Rev. Microbiol. 11, 789–799. doi: 10.1038/nrmicro3109
Reinhold-Hurek, B., Bunger, W., Burbano, C. S., Sabale, M., and Hurek, T. (2015). Roots shaping their microbiome: global hotspots for microbial activity. Annu. Rev. Phytopathol. 53, 403–424. doi: 10.1146/annurev-phyto-082712-102342
Robertson, G. P., Crum, J. R., and Ellis, B. G. (1993). The spatial variability of soil resources following long-term disturbance. Oecologia 96, 451–456. doi: 10.1007/BF00320501
Roesch, L. F., Fulthorpe, R. R., Riva, A., Casella, G., Hadwin, A. K. M., Kent, A. D., et al. (2007). Pyrosequencing enumerates and contrasts soil microbial diversity. ISME J. 1, 283–290. doi: 10.1038/ismej.2007.53
Santi, C., Bogusz, D., and Franche, C. (2013). Biological nitrogen fixation in non-legume plants. Ann. Bot. 111, 743–767. doi: 10.1093/aob/mct048
Sauer, D., and Burroughs, R. (1986). Disinfection of seed surfaces with sodium hypochlorite. Phytopathology 76, 745–749. doi: 10.1371/journal.ppat.1005914
Scherf, U., Ross, D. T., Waltham, M., Smith, L. H., Lee, J. K., Tanabe, L., et al. (2000). A gene expression database for the molecular pharmacology of cancer. Nat. Genet. 24, 236–244. doi: 10.1038/73439
Schlatter, D., Kinkel, L., Thomashow, L., Weller, D., and Paulitz, T. (2017). Disease suppressive soils: new insights from the soil microbiome. Phytopathology 107, 1284–1297. doi: 10.1094/PHYTO-03-17-0111-RVW
Schloss, P. D., Westcott, S. L., Ryabin, T., Hall, J. R., Hartmann, M., Hollister, E. B., et al. (2009). Introducing MOTHUR: open-source, platform-independent, community-supported software for describing and comparing microbial communities. Appl. Environ. Microbiol. 75, 7537–7541. doi: 10.1128/AEM.01541-09
Schmitz, A. M., and Harrison, M. J. (2014). Signaling events during initiation of arbuscular mycorrhizal symbiosis. J. Integr. Plant Biol. 56, 250–261. doi: 10.1111/jipb.12155
Schroder, S., Bahri, B. A., Eudy, D. M., Layton, D. J., Kellogg, E. A., and Devos, K. M. (2017). Genetic diversity and origin of North American green foxtail [Setaria viridis (L.) Beauv.] accessions. Genet. Resour. Crop Evol. 64, 367–378. doi: 10.1007/s10722-016-0363-6
Sebastian, J., Yee, M. C., Viana, W. G., Rellan-Alvarez, R., Feldman, M., Priest, H. D., et al. (2016). Grasses suppress shoot-borne roots to conserve water during drought. Proc. Natl. Acad. Sci. U.S.A. 113, 8861–8866. doi: 10.1073/pnas.1604021113
Segata, N., Izard, J., Waldron, L., Gevers, D., Miropolsky, L., Garrett, W. S., et al. (2011). Metagenomic biomarker discovery and explanation. Genome Biol. 12:R60. doi: 10.1186/gb-2011-12-6-r60
Smith, S. E., and Smith, F. A. (2011). Roles of arbuscular mycorrhizas in plant nutrition and growth: new paradigms from cellular to ecosystem scales. Annu. Rev. Plant Biol. 62, 227–250. doi: 10.1146/annurev-arplant-042110-103846
Sokal, R. R. (1958). A statistical method for evaluating systematic relationship. Univ. Kansas Sci. Bull. 28, 1409–1438.
Sugiyama, A., Ueda, Y., Zushi, T., Takase, H., and Yazaki, K. (2014). Changes in the bacterial community of soybean rhizospheres during growth in the field. PLoS One 9:e100709. doi: 10.1371/journal.pone.0100709
Szoboszlay, M., Lambers, J., Chappell, J., Kupper, J. V., Moe, L. A., and Mcnear, D. H. Jr. (2015). Comparison of root system architecture and rhizosphere microbial communities of Balsas teosinte and domesticated corn cultivars. Soil Biol. Biochem. 80, 34–44. doi: 10.1016/j.soilbio.2014.09.001
Tamme, R., Gazol, A., Price, J. N., Hiiesalu, I., and Pärtel, M. (2016). Co-occurring grassland species vary in their responses to fine-scale soil heterogeneity. J. Veg. Sci. 27, 1012–1022. doi: 10.1111/jvs.12431
Thompson, L. R., Sanders, J. G., Mcdonald, D., Amir, A., Ladau, J., Locey, K. J., et al. (2017). A communal catalogue reveals Earth’s multiscale microbial diversity. Nature 551, 457–463. doi: 10.1038/nature24621
Trevors, J. T. (2010). One gram of soil: a microbial biochemical gene library. Antonie Van Leeuwenhoek 97, 99–106. doi: 10.1007/s10482-009-9397-5
Wagner, M. R., Lundberg, D. S., Del Rio, T. G., Tringe, S. G., Dangl, J. L., and Mitchell-Olds, T. (2016). Host genotype and age shape the leaf and root microbiomes of a wild perennial plant. Nat. Commun. 7:12151. doi: 10.1038/ncomms12151
Waines, J. G., and Ehdaie, B. (2007). Domestication and crop physiology: roots of green-revolution wheat. Ann. Bot. 100, 991–998. doi: 10.1093/aob/mcm180
Wang, H., Nussbaum-Wagler, T., Li, B. L., Zhao, Q., Vigouroux, Y., Faller, M., et al. (2005). The origin of the naked grains of maize. Nature 436, 714–719. doi: 10.1038/nature03863
Wang, Q., Garrity, G. M., Tiedje, J. M., and Cole, J. R. (2007). Naive Bayesian classifier for rapid assignment of rRNA sequences into the new bacterial taxonomy. Appl. Environ. Microbiol. 73, 5261–5267. doi: 10.1128/AEM.00062-07
Werner, G. D. A., Cornwell, W. K., Cornelissen, J. H. C., and Kiers, E. T. (2015). Evolutionary signals of symbiotic persistence in the legume-rhizobia mutualism. Proc. Natl. Acad. Sci. U.S.A. 112, 10262–10269. doi: 10.1073/pnas.1424030112
Wilcoxon, F. (1945). Individual comparisons by ranking methods. Biom. Bull. 1, 80–83. doi: 10.2307/3001968
Wissuwa, M., Mazzola, M., and Picard, C. (2009). Novel approaches in plant breeding for rhizosphere-related traits. Plant Soil 321, 409–430. doi: 10.1007/s11104-008-9693-2
Keywords: endophytes, Euryarchaeota, foxtail millet, metagenome, rhizosphere, root
Citation: Chaluvadi S and Bennetzen JL (2018) Species-Associated Differences in the Below-Ground Microbiomes of Wild and Domesticated Setaria. Front. Plant Sci. 9:1183. doi: 10.3389/fpls.2018.01183
Received: 17 January 2018; Accepted: 24 July 2018;
Published: 21 August 2018.
Edited by:
Thomas P. Brutnell, Donald Danforth Plant Science Center, United StatesReviewed by:
Andrea Campisano, Edmund Mach Foundation, ItalyCollin M. Timm, Johns Hopkins University, United States
Copyright © 2018 Chaluvadi and Bennetzen. This is an open-access article distributed under the terms of the Creative Commons Attribution License (CC BY). The use, distribution or reproduction in other forums is permitted, provided the original author(s) and the copyright owner(s) are credited and that the original publication in this journal is cited, in accordance with accepted academic practice. No use, distribution or reproduction is permitted which does not comply with these terms.
*Correspondence: Srinivasa Chaluvadi, src@uga.edu Jeffrey L. Bennetzen, maize@uga.edu