- 1Toulouse Football Club, Toulouse, France
- 2Division of Training and Movement Sciences, University of Potsdam, Potsdam, Germany
- 3INISA, Federal University of Mato Grosso do Sul, Campo Grande, Brazil
- 4Department of Exercise & Sport Science; Department of Nutrition, University of North Carolina, Chapel Hill, NC, United States
- 5Univ Rennes, M2S (Laboratoire Mouvement, Sport, Santé), Rennes, France
Introduction
Modern physical training in elite sport is characterized by the systematic and continuous assessment of data on competitive and training performances (Clemente et al., 2019a). In team sports, Global Positioning Systems (GPS) technology is probably the most used monitoring tool to record workloads during training and competitions (Akenhead and Nassis, 2016).
In soccer, the training load (TL) has previously been defined as the input variable that is manipulated to elicit the desired training response (Impellizzeri et al., 2019) and it can be differentiated into external and internal loads (Jaspers et al., 2017). While external TL refers to the overall activities of a player, internal TL encompasses the psycho-physiological stress imposed on the player's body (Jaspers et al., 2017). Both internal and external TLs represent the cumulative exposure of each player to training and competitions (Jaspers et al., 2018). TL can be assessed by means of internal and external measures (Impellizzeri et al., 2019). For internal measures, heart rate or rating of perceived exertion have traditionally been applied (Owen et al., 2015). For external measures, GPS data have proven to be a valid and reliable means (Nikolaidis et al., 2018). GPS measures time motion parameters represented by the distance covered and the number of efforts at different running velocities (e.g., up to 25.2 km/h), as well as bouts of acceleration and deceleration throughout an activity (e.g., up to 3 m/s2 or −3 m/s2, respectively) at different intensities (Akenhead and Nassis, 2016) over a few meters which are too short to reach high speed running (Varley et al., 2017).
However, it must be emphasized that the concept of GPS metrics based on thresholds have not yet reached consensus in the scientific literature (Rago et al., 2019b). Nevertheless, GPS data are frequently recorded to monitor external TL during game or training situations for each soccer player individually as well as for the whole team (Buchheit and Simpson, 2017). Indeed, evidence suggest that the external TL management is key to maintain physical fitness (e.g., VO2max; Clemente et al., 2019b) and match physical performances over time (Lee and Mukherjee, 2019). Thus, the total distance covered correlated negatively with the percentage of changes in mean HR during submaximal aerobic tests (Rago et al., 2019c). Moreover, the time spent in the maximal aerobic speed zone highly correlates with changes in aerobic fitness (Fitzpatrick et al., 2018). Game physical performance is random as it depends on situational factors (e.g., score, match location, opponent; Lago-Peñas, 2012; Redwood-Brown et al., 2018) and players' pitch position (Barnes et al., 2014; Ingebrigtsen et al., 2015; Akenhead et al., 2016). Thereby, monitoring of high-speed running (Sæterbakken et al., 2019) and the capacity to sustain a high number of accelerations and decelerations (Russell et al., 2016) is highly recommended during training and competition (Pettersen et al., 2018).
Beyond physical performance, external TL management is associated with sustaining musculo-tendinous injuries (Colby et al., 2017; Malone et al., 2018a). The acute to chronic workload ratio (ACWR) concept recently introduced by Gabbett (2016), has widely been used in sport science and practice. The ACWR is a mathematical calculation which consists of dividing the TL of the current week (acute TL) by the rolling average TL or exponentially weighted moving averages of the previous 4 weeks (chronic TL; Gabbett, 2016; Williams et al., 2017). Avoiding TL peaks and a progression in the increase of TL is therefore recommended to reduce the injury risk associated to TL (Gabbett, 2020; Griffin et al., 2020; Maupin et al., 2020). Along these lines, a “danger zone” for an increased injury risk has been suggested to exist when the ACWR is between 0.8 and 1.5 (Gabbett, 2016). This means that when the acute load is <0.8 times or is >1.5 times the chronic training load, the injury risk increases during the subsequent week (Gabbett, 2016). Under this situation, it seems that the injury risk can be modified by high levels of aerobic fitness, greater lower body strength, a reduced history of injuries, and a younger age of the soccer players (Malone et al., 2018a; Gabbett, 2020).
In professional soccer, low chronic external TL associated with rapid and significant increases in the acute workload, increases the risk of non-contact injury (Duhig et al., 2016; Bowen et al., 2017, 2019; Colby et al., 2017; Jaspers et al., 2018; Malone et al., 2018a). Of note, the association between the ACWR and injury risk cannot be interpreted as a manner to predict injuries (Griffin et al., 2020). There is also a critical claim against its internal validity (Impellizzeri et al., 2020). The traditional (“coupled”) ACWR calculation includes acute TL in the numerator and the chronic TL in the denominator of the equation (Gabbett, 2016). This can contribute to spurious correlations (Lolli et al., 2019; Windt and Gabbett, 2019). Meanwhile, the ACWR can help practitioners to receive a large picture of the player's TL for not exposing the player to TL errors (Drew and Finch, 2016). Even though critical reports exist on the ACWR (Wang et al., 2020), the International Olympic Committee has recommended using the ACWR to monitor injury and to provide athletes' thresholds to minimize injury occurrence throughout training programs (Soligard et al., 2016).
In modern training approaches (e.g., tactical periodization; Delgado-Bordonau and Mendez-Villanueva, 2012), training sessions combine technical, tactical, mental, and physical aspects on specific soccer drills (Dellal et al., 2012). Furthermore, the physical aspect of soccer drills, manipulation of rules, number of touches, number of players, duration of exercise, coaching encouragement, and pitch area are different parameters that impact their physical demands (Sarmento et al., 2018). On the other hand, during the season, the number and type of injuries, the status of the player (starter or not starter), having an illness or the wellbeing state are different between players (Anderson et al., 2016; Malone et al., 2018b). Thus, external TL management is a dynamic, complex and challenging task for sport scientists and technical staff to combine scientific recommendations inside the complexity of the training process (Bourdon et al., 2017).
Recently, Malone et al. (2017, 2019) provided methodological recommendations on how to collect, interpret, and report GPS data in team sports and thus to avoid some past methodological issues with its use. Nonetheless, an integration of the practical approaches combining scientific knowledge and coaches' expertise from the “field” appears to be lacking. That is, studies have made recommendations according to a specific topic such as external TL or injury prevention (Jaspers et al., 2018; Bowen et al., 2019), physical development (Clemente et al., 2019b), or game demands (Barnes et al., 2014). However, on the “field,” practitioners need to examine recommendations from a multitude of studies to manage their TL prescription and monitoring. Thus, the purpose of this opinion paper is to propose a practical approach for soccer coaches on how to use GPS data for training load monitoring on a team and individual level. We suggest that the planning of external TL should be realized on a monthly, weekly, and daily level in order to reach collective performance. Inside this collective plan, it is important to expose each player to individual external TL to enhance physical performance and lower the risk of sustaining injuries.
Relevant Parameters From Global Positioning System Data
The selection of reliable and relevant GPS parameters (Table 1) depends on the purported use. GPS parameters are useful to monitor the external TL (e.g., plan external TL, calculate the ACWR), both individually and collectively, to create specific training sessions and to analyze their level of specificity (Dellal et al., 2012). Based on scientific evidence, the total distance covered, the distance covered at high-speed running (HSR) measured between 19.8 and 24.8 km/h, the distance covered at sprint running (SPR) measured over 25.2 km/h, the specific maximal speed (e.g., to record in the game), the number of accelerations (≥3 m/s2), and the number of decelerations (≤ −3 m/s2) seem to be relevant GPS parameters to monitor the external TL in professional soccer (Akenhead et al., 2016; Varley et al., 2017; Malone et al., 2019). All these GPS parameters represent the volume of training sessions and games (Figueiredo et al., 2018). Total distance covered, high speed running, or sprint running related to the time (expressed in “m/min”) reflect the intensity and are used to design specific training sessions [e.g., rehabilitation sessions (Taberner et al., 2019)] and to delineate their specificity (e.g., physical activity profile similar to the game; Figueiredo et al., 2018; Whitehead et al., 2018). In addition, the distance of high-speed running efforts (i.e., sprints, accelerations, and decelerations) characterize the specifics of the game or the training session (Martín-García et al., 2018). For these GPS parameters, the average, the minimum, and the maximum values should be considered for analyses (Rago et al., 2019b).
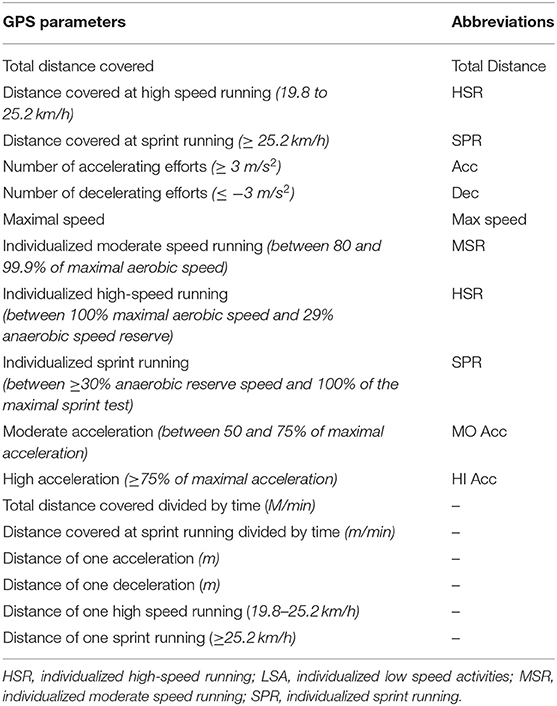
Table 1. Relevant GPS parameters to monitor training load, create specific training programs, and analyze the specificity of the training session.
The speed or acceleration threshold (e.g., high speed running between 19.8 and 24.8 km/h) has been arbitrarily defined and is equal for all players (Rago et al., 2019b). Other alternatives are to individualize the speed or acceleration thresholds. Individualized speed zones are based on a combination of maximal aerobic speed (MAS) which is derived from the Yo-yo intermittent recovery test level 1 or tested directly using another test, maximal sprint speed (MSS) which is derived from the maximal speed reached during training, and the anaerobic speed reserve (ASR) which corresponds to <80% of MAS, 80–100% of MAS, 100% of MAS, or 29% of ASR and ≥30% of ASR (Hunter et al., 2015; Rago et al., 2019a) (Table 1). For acceleration and deceleration zones, most studies used +3/−3 m·s−2 as threshold of intense/high acceleration or deceleration, respectively (Akenhead et al., 2016; Abbott et al., 2018a; Malone et al., 2019; Rago et al., 2019b). However, recent studies (Delaney et al., 2017; Rago et al., 2019b) suggest that a maximum threshold of +2/−2 m·s−2 should be preferred over +3/−3 m·s−2. Moreover, a limitation of these thresholds is that the speed is not known from which accelerations/decelerations actually begin (Rago et al., 2019a) (Table 1). Individual speed thresholds which should be adjusted according to the individual aerobic capacity show higher associations with perceptual responses to training loads compared with arbitrary speed thresholds (Rago et al., 2019a). It has to be acknowledged though that both methods showed similar sensitivity in depicting players' locomotor abilities. It seems though that the two methods should not be used interchangeably (Rago et al., 2020). The use of individualized GPS parameters (e.g., acceleration or speed threshold) transcribe the individual capacity of a player (Abbott et al., 2018b). A prerequisite of this approach is to regularly evaluate players' maximal aerobic speed and maximal sprint speed over the course of a season (Rago et al., 2019a). In the “real world” of professional soccer, it is not always possible to evaluate players' capacities to quantify the training load using individualized GPS parameters (Carling et al., 2018).
In summary, it can be recommended to determine relevant GPS parameters, based on arbitrary or individualized thresholds, as they will fit well with the training programs and their foundations (Rago et al., 2019b).
Planning the Training Load for the Whole Team While Respecting Individualization
The Importance of Analyzing Game Performance for Determining Training Load Reference Values for Each Player
The specific physical activity profile of individual players during games is used to plan monthly, weekly, and daily external TL, according to the physical demands of each player recorded during in-season games (Stevens et al., 2017; Martín-García et al., 2018). For this purpose, the game reference (Gref) values have to be quantified individually (Akenhead and Nassis, 2016). Individual Gref include every official game of the current and the previous season. For determining GPS parameters used to monitor external TL, Gref is arbitrarily calculated as the mean of the five best values recorded during official games (Table 2) as players are prepared for the most physical demanding games. For new players, as a rule, Gref is created, or according to the reference data from the literature (Ingebrigtsen et al., 2015; Suarez-Arrones et al., 2015) or with the values of the previous season on the same competition level.
The example depicted in Table 2 highlights the values of physical demands (Under 23 UEFA Champion's League Team) according to the players' positions on the pitch during games.
Scheduled Plans With Reference to Games Performance
Game reference values for each GPS parameter (Table 1) allow staff to program external TL at both collective and individual levels (Rago et al., 2019b). Collectively, external TL is calculated for each GPS parameter by a collective weighted factor (described below) of game reference values. Gref being specific to each player, calculation of external TL is individualized (Ingebrigtsen et al., 2015). For each player, external TL is calculated in meters or number of events according to the nature of the GPS parameter. For example, for high speed running, a distance to be covered in meters is calculated (Sæterbakken et al., 2019) whereas for acceleration and deceleration, the number of efforts (Varley et al., 2017) to be achieved is calculated.
It can be recommended to collectively and individually program the external TL by multiplying Gref by a weighting factor (e.g., 3.2 for weekly total distance).
Monthly
During the first 4 weeks, external TL increases progressively to reach the targeted high chronic (4 weeks) TL value. From data recorded in Dutch and English professional soccer leagues, chronic high total distance would be up to 111,500 m and chronic high speed running and sprint combined would be up to 3,727 and 6,173 m, respectively (Jaspers et al., 2018; Bowen et al., 2019). For bouts of acceleration and deceleration, data from the literature are difficult to use because of the filters that are applied by the different manufacturers (Varley et al., 2017). From these published data, we propose a monthly collective plan with one game per week (Figure 1). For each week and for each GPS parameter, Gref is weighted by a weekly factor [Fw(i)] to calculate the weekly external TL (WTL) (Equation 1). Fw(i) is arbitrarily chosen to reach a high chronic TL described in the literature (Jaspers et al., 2018; Bowen et al., 2019)
WTL is weekly external TL; Fw(i) Is the weekly weighted factor; (i) is the number of the weeks; Gref is the game reference value.
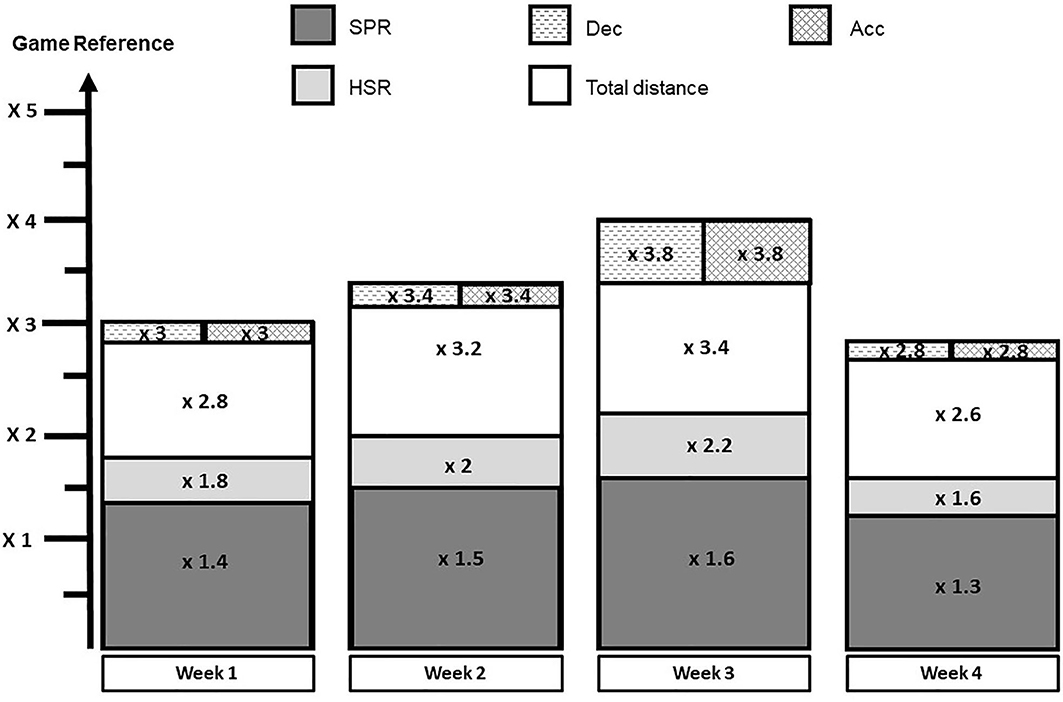
Figure 1. Example of a monthly collective plan. HSR, high-speed running; SPR, sprint running; Dec, deceleration; Acc, acceleration.
Figure 1 illustrates a collective plan of four training weeks. Total distance, high speed running, sprint, bouts of acceleration and deceleration increase gradually during the first 3 weeks by an increase in Fw(i) and a decrease during week 4. For example, for high speed running, Fw(i) is 1.8, 2.0, 2.2, and 1.6 for weeks 1, 2, 3, and 4, respectively. External TL increases correspond to an increase around ~10% between weeks (Table 3). This 10% increase between weeks guarantees an ACWR between 0.80 and 1.20 (Gabbett, 2016). Examples depicted in Table 3 show the weekly data for four different pitch positions. External TL planning is a collective framework but, in the “real world,” individual particularities force to individually adapt the monthly external load (as later explained on “adjusting individually external TL”).
To summarize, external TL should be planned to progressively reach a greater, elevated chronic external TL on a monthly basis. Game reference is weighted by a weekly factor (for example: Total distance x 2.8 on week 1, x 3.2 on week 2, x 3.4 on week 3 and x 2.6 on week 4).
Weekly
The weekly training schedule is organized around the game day (GD) (Akenhead et al., 2016). Training days are scheduled based on the number of available days for training before and after the game day (i.e., minus or plus the game day; Clemente et al., 2019a). In order to adapt the external TL depending on the game physical demand (see below), the week should start at game day and finish at game day−1. Following published data (Malone et al., 2015; Akenhead et al., 2016; Stevens et al., 2017; Martín-García et al., 2018), weekly external TL is arbitrarily distributed during the days of the week (DTL). For each day and for each GPS parameter, Gref is weighted by daily arbitrary factor [FGD(i)] to calculate daily external TL (Equation 2).
DTL is daily training load; FGD(i) is daily weighting factor; (i) is the number of the day; Gref is the game reference values.
Figure 2 illustrates week 2 of a monthly collective plan. Game day +1 is devoted to recover from matches, specifically those players who played ≥60 min, while players who played ≤ 30 min perform a compensatory training session. This figure presents published data from teams in the Netherlands and Portugal (Clemente et al., 2019a), game day +3 and game day +4 are the most important days in terms of external TL whereas an important reduction in external TL is programed for game day −1.
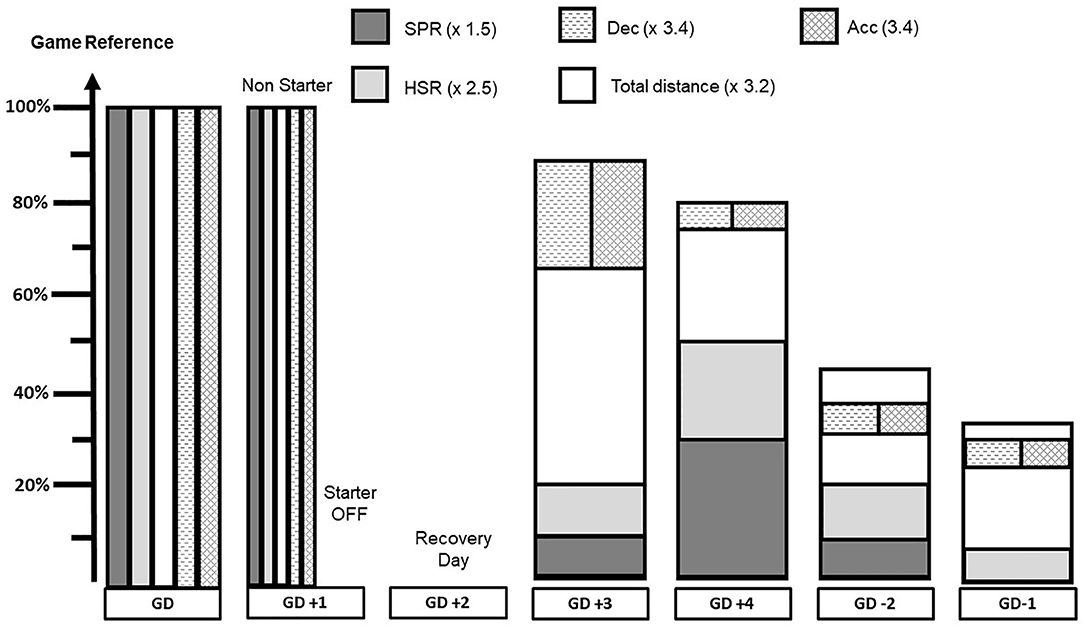
Figure 2. Example of a weekly collective plan (week 2) from individual match reference values. HSR, high-speed running; SPR, sprint running; Dec, decelerations; Acc, accelerations; GD, game day.
As for monthly external TL planning, the weekly external TL planning is a collective framework but, in the “real world,” for each day and GPS parameter, a difference between the planned (TLplan) and the realized (TLreal) TL may occur and is referred to as TLdiff(i) (Equation 3). For each day of the week, the difference between TLplan and TLreal is distributed proportionally at FGD(i) on the subsequent days of the week (Figure 3). A weighted factor entitled FAGD(i) is also calculated (Equation 4). Finally, for each day, TLadj(i) is calculated and distributed proportionally on the subsequent days of the week (Equation 5).
TLadj(i) is the external TL difference between TLplan and TLreal on a day (i)
Whereas; FAGD(i) is the weighted factor for the difference between daily TLplan and daily TLreal; FGD(i) is the daily weighted factor; ∑(FGD≥(i)) is the sum of the daily weighted factor on the subsequent days of the week; (i) is the number of the day; ≥(i) is posterior and include (i).
Table 4 shows, for 4 different pitch positions, an example of proportional distribution calculations for total distance, high speed running, sprint, acceleration and deceleration after game day. On game day, TLplan is Gref whereas TLreal is random. On this table, in brackets, the individual distribution of match external TL during the week is expressed as percentages.
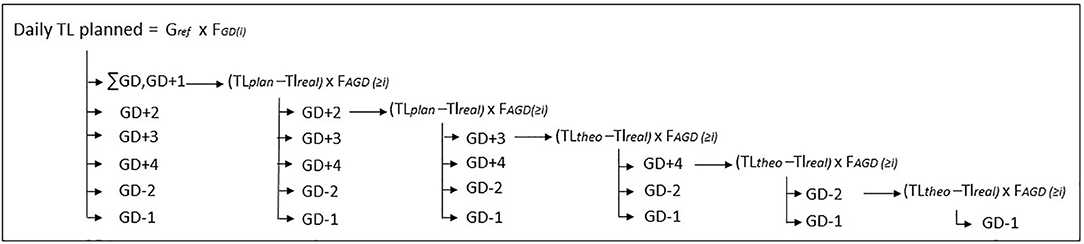
Figure 3. Proportional distribution of the difference between TLplan and TLreal for each day (see text of the paper for abbreviation explanation).
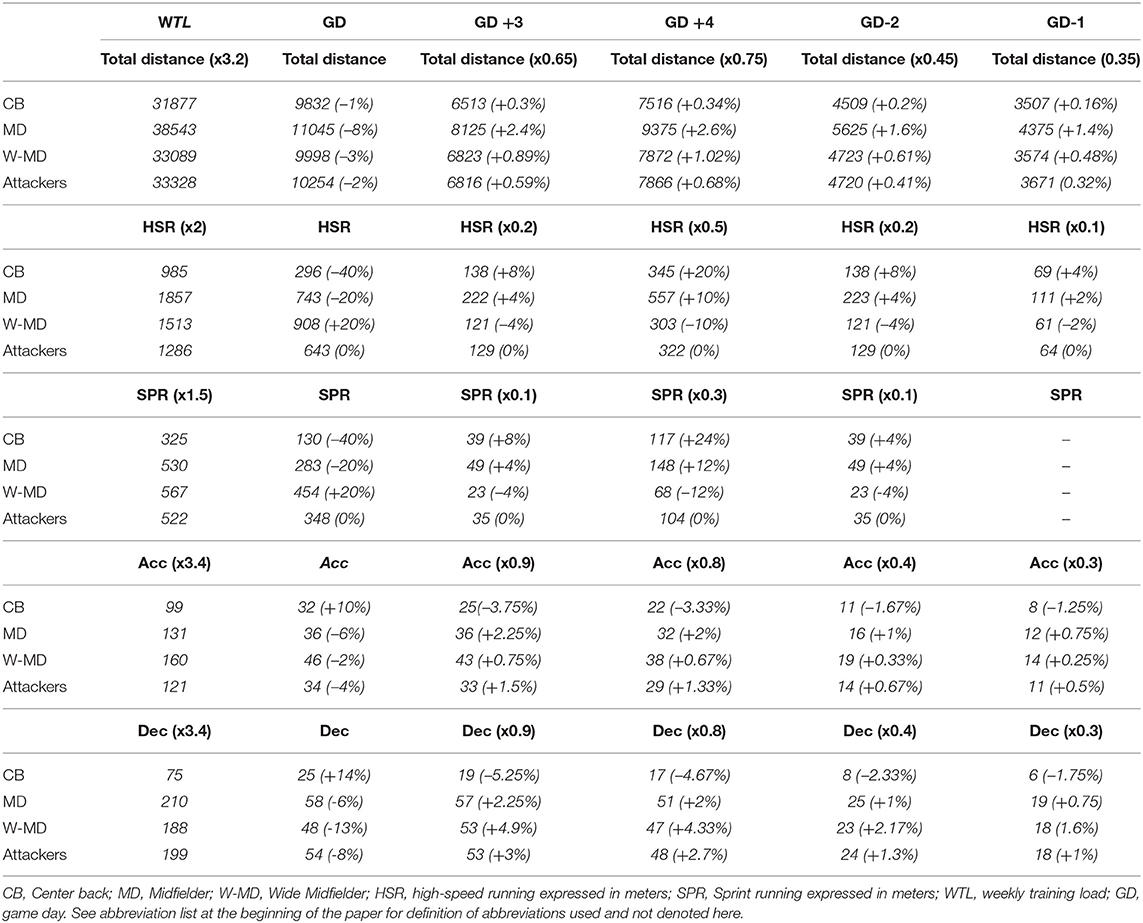
Table 4. Example of weekly external TL distribution adjusted in terms of game physical demands, for each GPS parameters.
On game day −1, at the end of the week, TLplan is the result of the recorded TLreal on the previous day. It serves as an information for the technical staff to adapt, if necessary, the last collective training session. For example, if at game day−1, high speed running distance recorded [game day + (game day +2) + (game day +3) + (gamed day +4) + game day−2)] is collectively higher than what was planned, the technical staff can choose to decrease the pitch area to diminish high speed running distance while preserving the tactical objective.
To summarize, we recommend the external TL to be planned on a weekly basis. Game reference has to be weighted using a daily factor (e.g., 0.6). This should be realized on an individual level and adjusted according to an algorithm that takes TLplan and TLreal into account.
Daily
Before training, in accordance with the technical staff, we program the training session to reach the collective objective of the day with respect to the planned weekly external TL. Continuous feedback during the training session allows to adjust in “real time,” both collectively and individually, the external TL in relation to TLplan. This adjustment could be met collectively by modifying a game rule, the pitch area, and the duration of exercise; and individually by adding a specific physical exercise in order to reach TLplan of the day. Collective exercises and specific physical exercises are programmed to meet the physical demands of the match. Thereby, for each player, the distance covered for each high-speed running, sprint, acceleration and deceleration in m/min should be continuously assessed to verify the specificity of an exercise. Figure 4 shows an example of a training session in game day +3 of the second week of the monthly planned external TL. Gref of total distance, high speed running, sprint, bouts of acceleration and deceleration are weighted by 0.65, 0.2, 0.1, 0.9, and 0.9, respectively. In other words, total distance, high speed running, sprint, acceleration and deceleration represent 65, 20, 10, 90, and 90 percent of Gref, respectively. This training session combined physical (i.e., acceleration/deceleration specific endurance), technical (i.e., play with high pressure), tactical (i.e., press zone and organization formation), and mental (i.e., concentration) goals which were defined by the technical staff. At the end of the training session, two optional specific physical exercises were assigned to the players who did not reach TLplan. For this example, the values for the four different positions are presented in Table 5.
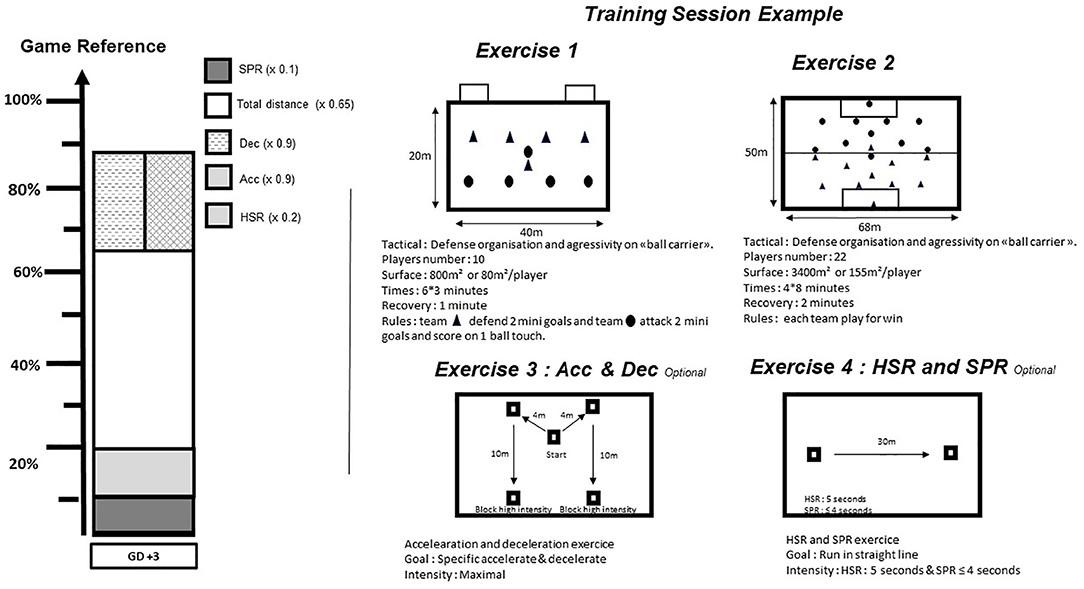
Figure 4. Example of a daily team training session. HSR, high- speed running; SPR, sprint running; Dec, decelerations; Acc, accelerations; GD, Game day.
Hence, it can be recommended to build the training sessions to reach the TLplan. Before the training session starts, the targeted TL should be calculated for each player individually. In addition, specific exercises could be prescribed which help the player to reach the calculated TL.
Adjusting Individually External TL
The great heterogeneity of the team in terms of age, physical conditioning, history of injry, etc., makes it necessary to individualize external TL for each player. First, external TL is programmed by the collective weekly weighted factor [Fw(i)] and the daily weighted factor [FGD(i)]. Subsequently, in order to meet the needs of each player, Fw(i) and FGD(i) should be individualized, either to increase or to reduce the external TL with respect to the collective external TL. Physical performance and training load adaptation assessments are used to adjust individually the external TL, on a monthly, weekly, and daily basis. Monthly, a submaximal aerobic test (e.g., 4 min at 12 km/h) and countermovement jumps provide information about the aerobic and neuromuscular performance status of the players (Halson, 2014; Buchheit et al., in press). Weekly at game day +3, heart rate variability during an orthostatic stand test (Ravé and Fortrat, 2016; Ravé et al., 2020), and blood creatine kinase (Hader et al., 2019) provide information about recovery from games. Daily, before the training a wellbeing questionnaire (e.g., muscular damage, fatigue, sleep quality) (Malone et al., 2018b) and after the training, the session rating of perceived exertion [e.g., CR-10 Borg scale; (Malone et al., 2018b)] provide information on how each player perceives strain and adaptation of each training session. All these information help practitioners to make the “right decision” about the management of external TL affecting the player(s). This prescription is dynamic, adaptable and it can be updated and adjusted daily according to the “real world” if unique situations occur.
To summarize, coaches can individually adjust the weighted factor depending on the results of physical performance tests together with the observed external TL adaptations. The continuous collection of internal and external TL data on a monthly, weekly, and daily level including data from the soccer game will help to better manage (e.g., increase or decrease) TL.
Limitations, Strengths, and Practical Applications
This practical approach tries to build a theoretical framework using knowledge from sport science to monitor training load during “real world” soccer practice. However, some limitations must be acknowledged concerning the weighted factors discussed [e.g., Fw(i) and FGD(i)]. More specifically, these factors were chosen, for the team, arbitrarily from published data and from existing monitored data. Future studies are needed to more precisely define and validate the scientific process to access these weighted factors [e.g., Fw(i) and FGD(i)] for accuracy. However, the practical approach discussed herein is, to our knowledge, the first publication attempting to combine the scientific recommendations and actual coaching experience on the field. This combined approach can open up working perspectives for practitioners for external TL prescription. In practice, it is important to be sure of the reliability of the used GPS data. Furthermore, players should keep the same device all season during both training sessions and games. After choosing the relevant GPS parameters, both arbitrarily and with individualized thresholds, the parameters used should be the same over the whole season and it is not recommended to change the parameters. The game reference for each GPS parameter used is specific for each player. The collective plan of external TL is updated on a monthly, weekly, and daily bases. Finally, the adjustment of the external TL of a player depends on the difference between TLplan and TLreal while considering the results from physical performance tests and training load adaptations.
Conclusions
GPS is a valid, reliable and relevant tool for tracking the external TL in professional soccer. Previous scientific recommendations have highlighted the importance of monitoring the external TL to reduce injury risk and optimize players' physical performance. In this opinion paper, we have proposed an approach on how to use GPS data to analyze, prescribe, and control the external TL in elite soccer, both collectively (i.e., team) and individually.
Author Contributions
GR and HZ conceived and designed the idea. All authors wrote and revised the manuscript. All authors read and approved the manuscript.
Funding
The authors acknowledge the support of the Deutsche Forschungsgemeinschaft (DFG) and Open Access Publishing Fund of the University of Potsdam, Germany.
Conflict of Interest
GR is employed by company Toulouse Football Club (Toulouse, France).
The remaining authors declare that the research was conducted in the absence of any commercial or financial relationships that could be construed as a potential conflict of interest.
Abbreviations
Acc, number of acceleration; ACWR, acute chronic workload ratio; Dec, number of deceleration; DTL, daily external training load; FAGD(i), weighting factor of the difference between the external TL planned and external TL realized; FGD(i), daily weighting factor; Fw(i), weekly weighting factor; GD, Game Day; GPS, Global Positioning Systems; Gref , game reference; HSR, high speed running; M/min, meter per minute; Max speed, maximal speed; SPR, sprint running; TL, training Load; TLadj(i), the external TL difference between the external TL planned and external TL realized; TLplan, the external TL planned; TLreal, external TL realized; VO2max, maximal oxygen consumption; WTL, weekly external training load.
References
Abbott, W., Brickley, G., and Smeeton, N. J. (2018b). An individual approach to monitoring locomotive training load in English Premier League academy soccer players. Int. J. Sports Sci. Coaching 13, 421–428. doi: 10.1177/1747954118771181
Abbott, W., Brickley, G., Smeeton, N. J., and Mills, S. (2018a). Individualizing acceleration in english premier league academy soccer players. J. Strength Cond. Res. 32, 3503–3510. doi: 10.1519/JSC.0000000000002875
Akenhead, R., Harley, J. A., and Tweddle, S. P. (2016). Examining the external training load of an English Premier League football team with special reference to acceleration. J. Strength. Cond. Res. 30, 2424–2432. doi: 10.1519/JSC.0000000000001343
Akenhead, R., and Nassis, G. P. (2016). Training load and player monitoring in high-level football: current practice and perceptions. Int. J. Sports Physiol. Perform. 11, 587–593. doi: 10.1123/ijspp.2015-0331
Anderson, L., Orme, P., Di Michele, R., Close, G. L., Milsom, J., Morgans, R., et al. (2016). Quantification of seasonal-long physical load in soccer players with different starting status from the english premier league: implications for maintaining squad physical fitness. Int. J. Sports Physiol. Perform. 11, 1038–1046. doi: 10.1123/ijspp.2015-0672
Barnes, C., Archer, D. T., Hogg, B., Bush, M., and Bradley, P. S. (2014). The evolution of physical and technical performance parameters in the English Premier League. Int. J. Sports Med. 35, 1095–1100. doi: 10.1055/s-0034-1375695
Bourdon, P. C., Cardinale, M., Murray, A., Gastin, P., Kellmann, M., Varley, M. C., et al. (2017). Monitoring athlete training loads: consensus statement. Int. J. Sports Physiol. Perform. 12, S2-161–S2-170. doi: 10.1123/IJSPP.2017-0208
Bowen, L., Gross, A. S., Gimpel, M., Bruce-Low, S., and Li, F. X. (2019). Spikes in acute:chronic workload ratio (ACWR) associated with a 5-7 times greater injury rate in English Premier League football players: a comprehensive 3-year study. Br. J. Sports. Med. 21:422. doi: 10.1136/bjsports-2018-099422
Bowen, L., Gross, A. S., Gimpel, M., and Li, F. X. (2017). Accumulated workloads and the acute:chronic workload ratio relate to injury risk in elite youth football players. Br. J. Sports Med. 51, 452–459. doi: 10.1136/bjsports-2015-095820
Buchheit, M., and Simpson, B. M. (2017). Player tracking technology: half-full or half-empty glass? Int. J. Sports Physiol. Perform. 12, S235–S241. doi: 10.1123/ijspp.2016-0499
Buchheit, M., Simpson, B. M., and Lacome, M. (in press). Monitoring cardiorespiratory fitness in professional soccer players: is it worth the prick? Int. J. Sports. Physiol. Perform.
Carling, C., Lacome, M., McCall, A., Dupont, G., Le Gall, F., Simpson, B., et al. (2018). Monitoring of post-match fatigue in professional soccer: welcome to the real world. Sports. Med. 48, 2695–2702. doi: 10.1007/s40279-018-0935-z
Clemente, F. M., Nikolaidis, P. T., Rosemann, T., and Knechtle, B. (2019b). Dose-response relationship between external load variables, body composition, and fitness variables in professional soccer players. Front. Physiol. 17:443. doi: 10.3389/fphys.2019.00443
Clemente, F. M., Owen, A., Serra-Olivares, J., Nikolaidis, P. T., Cornelis, M., van der Linden, I., et al. (2019a). Characterization of the weekly external load profile of professional soccer teams from portugal and the Netherlands. J. Hum. Kinet. 27, 155–164. doi: 10.2478/hukin-2018-0054
Colby, M. J., Dawson, B., Peeling, P., Heasman, J., Rogalski, B., Drew, M. K., et al. (2017). Multivariate modelling of subjective and objective monitoring data improve the detection of non-contact injury risk in elite Australian footballers. J. Sci Med. Sport. 20, 1068–1074. doi: 10.1016/j.jsams.2017.05.010
Delaney, J. A., Cummins, C. J., Heidi, R.T., and Grant, M.D. (2017). Importance, reliability, and usefulness of acceleration measures in team sports. J. Strength. Cond. Res. 32, 3485–3493. doi: 10.1519/JSC.0000000000001849
Delgado-Bordonau, J. L., and Mendez-Villanueva, A. (2012). Tactical periodization: MoUrinho's BesT-kePT secreT? Soccer J. (Tactics). 28–34.
Dellal, A., Owen, A., Wong, D. P., Krustrup, P., van Exsel, M., and Mallo, J. (2012). Technical and physical demands of small vs. large sided games in relation to playing position in elite soccer. Hum. Mov. Sci. 31, 957–969. doi: 10.1016/j.humov.2011.08.013
Drew, M. K., and Finch, C. F. (2016). The relationship between training load and injury, illness and soreness: a systematic and literature review. Sports. Med. 46, 861–883. doi: 10.1007/s40279-015-0459-8
Duhig, S., Shield, A. J., Opar, D., Gabbett, T. J., Ferguson, C., and Williams, M. (2016). Effect of high-speed running on hamstring strain injury risk. Br. J. Sports. Med. 50, 1536–1540. doi: 10.1136/bjsports-2015-095679
Figueiredo, P., Nassis, G. P., and Brito, J. (2018). The within-subject correlation between salivary IgA and measures of training load in elite football players. Int. J. Sports Physiol. Perform. 14, 1–11. doi: 10.1123/ijspp.2018-0455
Fitzpatrick, J. F., Hicks, K. M., and Hayes, P. R. (2018). Dose-response relationship between training load and changes in aerobic fitness in professional youth soccer players. Int. J. Sports. Physiol. Perform. 19, 1–6. doi: 10.1123/ijspp.2017-0843
Gabbett, T. J. (2016). The training-injury prevention paradox: should athletes be training smarter and harder? Br. J. Sports Med. 50, 273–280. doi: 10.1136/bjsports-2015-095788
Gabbett, T. J. (2020). Debunking the myths about training load, injury and performance: empirical evidence, hot topics and recommendations for practitioners. Br. J. Sports. Med. 54, 58–66. doi: 10.1136/bjsports-2018-099784
Griffin, A., Kenny, I. C., Comyns, T. M., and Lyons, M. (2020). The association between the acute:chronic workload ratio and injury and its application in team sports: a systematic review. Sports. Med. 50, 561–580 doi: 10.1007/s40279-019-01218-2
Hader, K., Rumpf, M. C., Hertzog, M., Kilduff, L. P., Girard, O., and Silva, J. R. (2019). Monitoring the athlete match response: can external load variables predict post-match acute and residual fatigue in soccer? A systematic review with meta-analysis. Sports. Med. Open. 9:548. doi: 10.1186/s40798-019-0219-7
Halson, S. L. (2014). Monitoring training load to understand fatigue in athletes. Sports. Med. 44, S139–S147. doi: 10.1007/s40279-014-0253-z
Hunter, F., Bray, J.C., Towlson, C., Smith, M., Barrett, S., Madden, J., et al. (2015). Individualisation of time-motion analysis: a method comparison and case report series. Int. J. Sports. Med. 36, 41–48. doi: 10.1055/s-0034-1384547
Impellizzeri, F. M., Marcora, S. M., and Coutts, A. J. (2019). Internal and external training load: 15 years on. Int. J. Sports. Physiol Perform. 14, 270–273. doi: 10.1123/ijspp.2018-0935
Impellizzeri, F. M., Woodcock, S., Coutts, A. J., Fanchini, M., McCall, A., and Vigotsky, A. D. (2020). Acute to Random Chronic Workload Ratio is ‘as’ Associated With Injury as Acute to Actual Chronic Workload Ratio: Time to Dismiss ACWR and Its Components. Society for Transparency. Openness and Replication in Kinesiology. doi: 10.31236/osf.io/e8kt4
Ingebrigtsen, J., Dalen, T., Hjelde, G. H., Drust, B., and Wisløff, U. (2015). Acceleration and sprint profiles of a professional elite football team in match play. Eur. J. Sport Sci. 15, 101–110. doi: 10.1080/17461391.2014.933879
Jaspers, A., Brink, M. S., Probst, S. G., Frencken, W. G., and Helsen, W. F. (2017). Relationships between training load indicators and training outcomes in professional soccer. Sports Med. 47, 533–544. doi: 10.1007/s40279-016-0591-0
Jaspers, A., Kuyvenhoven, J. P., Staes, F., Frencken, W. G. P., Helsen, W. F., and Brink, M. S. (2018). Examination of the external and internal load indicators' association with overuse injuries in professional soccer players. J. Sci Med. Sport. 21, 579–585. doi: 10.1016/j.jsams.2017.10.005
Lago-Peñas, C. (2012). The role of situational variables in analysing physical performance in soccer. J. Hum. Kinet. 35, 89–95. doi: 10.2478/v10078-012-0082-9
Lee, M., and Mukherjee, S. (2019). Relationship of training load with high-intensity running in professional soccer players. Int. J. Sports Med. 40, 336–343. doi: 10.1055/a-0855-3843
Lolli, L., Batterham, A. M., Hawkins, R., Kelly, D. M., Strudwick, A. J., Thorpe, R., et al. (2019). Mathematical coupling causes spurious correlation within the conventional acute-to-chronic workload ratio calculations. Br. J. Sports. Med. 53, 921–922. doi: 10.1136/bjsports-2017-098110
Malone, J. J., Barrett, S., Barnes, C., Twist, C., and Drust, B. (2019). To infinity and beyond: the use of GPS devices within the football codes. Sci. Med in Football. 4, 82–84. doi: 10.1080/24733938.2019.1679871
Malone, J. J., Di Michele, R., Morgans, R., Burgess, D., Morton, J. P., and Drust, B. (2015). Seasonal training-load quantification in elite English premier league soccer players. Int. J. Sports. Physiol. Perform. 10, 489–497. doi: 10.1123/ijspp.2014-0352
Malone, J. J., Lovell, R., Varley, M. C., and Coutts, A. J. (2017). Unpacking the black box: applications and considerations for using GPS devices in sport. Int. J. Sports. Physiol. Perform. 12, S218–S226. doi: 10.1123/ijspp.2016-0236
Malone, S., Owen, A., Mendes, B., Hughes, B., Collins, K., and Gabbett, T. J. (2018a). High-speed running and sprinting as an injury risk factor in soccer: can well-developed physical qualities reduce the risk? J. Sci. Med Sport. 21, 257–262. doi: 10.1016/j.jsams.2017.05.016
Malone, S., Owen, A., Newton, M., Mendes, B., Tiernan, L., Hughes, B., et al. (2018b). Wellbeing perception and the impact on external training output among elite soccer players. J. Sci. Med. Sport. 21, 29–34. doi: 10.1016/j.jsams.2017.03.019
Martín-García, A., Gómez Díaz, A., Bradley, P. S., Morera, F., and Casamichana, D. (2018). Quantification of a professional football team's external load using a microcycle structure. J. Strength. Cond Res. 32, 3511–3518. doi: 10.1519/JSC.0000000000002816
Maupin, D., Schram, B., Canetti, E., and Orr, R. (2020). The relationship between acute: chronic workload ratios and injury risk in sports: a systematic review. Open. Access. J. Sports. Med. 11, 51–75. doi: 10.2147/OAJSM.S231405
Nikolaidis, P. T., Clemente, F. M., van der Linden, C. M. I., Rosemann, T., and Knechtle, B. (2018). Validity and reliability of 10-Hz global positioning system to assess in-line movement and change of direction. Front. Physiol. 15:228. doi: 10.3389/fphys.2018.00228
Owen, A. L., Forsyth, J. J., Wong del, P., Dellal, A., Connelly, S. P., and Chamari, K. (2015). Heart rate-based training intensity and its impact on injury incidence among elite-level professional soccer players. J. Strength Cond. Res. 29, 1705–1712. doi: 10.1519/JSC.0000000000000810
Pettersen, S. A., Johansen, H. D., Baptista, I. A. M., Halvorsen, P., and Johansen, D. (2018). Quantified soccer using positional data: a case study. Front. Physiol. 6:866. doi: 10.3389/fphys.2018.00866
Rago, V., Brito, J., Figueiredo, P., Costa, J., Barreira, D., Krustrup, P., et al. (2019b). Methods to collect and interpret external training load using microtechnology incorporating GPS in professional football: a systematic review. Res. Sports. Med. 22, 1–22. doi: 10.1080/15438627.2019.1686703
Rago, V., Brito, J., Figueiredo, P., Krustrup, P., and Rebelo, A. (2019a). Relationship between external load and perceptual responses to training in professional football: effects of quantification method. Sports (Basel). 7:68. doi: 10.3390/sports7030068
Rago, V., Brito, J., Figueiredo, P., Krustrup, P., and Rebelo, A. (2020). Application of individualized speed zones to quantify external training load in professional soccer. J. Hum. Kinetics. 72, 279–289. doi: 10.2478/hukin-2019-0113
Rago, V., Krustrup, P., Martín-Acero, R., Rebelo, A., and Mohr, M. (2019c). Training load and submaximal heart rate testing throughout a competitive period in a top-level male football team. J. Sports. Sci. 26, 1–8. doi: 10.1080/02640414.2019.1618534
Ravé, G., and Fortrat, J. O. (2016). Heart rate variability in the standing position reflects training adaptation in professional soccer players. Eur. J. Appl. Physiol. 116, 1575–1582. doi: 10.1007/s00421-016-3416-9
Ravé, G., Zouhal, H., Boullosa, D., Doyle-Baker, P. K., Saeidi, A., Abderrahman, A. B., et al. (2020). Heart rate variability is correlated with perceived physical fitness in elite soccer players. J. Hum. Kinet. 31, 141–150. doi: 10.2478/hukin-2019-0103
Redwood-Brown, A. J., O'Donoghue, P. G., Nevill, A. M., Saward, C., Dyer, N., and Sunderland, C. (2018). Effects of situational variables on the physical activity profiles of elite soccer players in different score line states. Scand. J. Med. Sci Sports. 28, 2515–2526. doi: 10.1111/sms.13271
Russell, M., Sparkes, W., Northeast, J., Cook, C. J., Love, T. D., Bracken, R. M., et al. (2016). Changes in acceleration and deceleration capacity throughout professional soccer match-play. J. Strength. Cond Res. 30, 2839–2844. doi: 10.1519/JSC.0000000000000805
Sæterbakken, A., Haug, V, Fransson, D., Grendstad, H. N., Gundersen, H. S., Moe, V. F., et al. (2019). Match running performance on three different competitive standards in norwegian soccer. Sports. Med. Int. Open. 16, E82–E88. doi: 10.1055/a-0943-3682
Sarmento, H., Clemente, F. M., Harper, D., da Costa, I. T., Owen, A., and Figueiredo, A. J. (2018). Small sided games in soccer-a systematic review. Int. J. Perform. Anal. Sport. 18, 693–749. doi: 10.1080/24748668.2018.1517288
Soligard, T., Schwellnus, M., Alonso, J. M., Bahr, R., Clarsen, B., Dijkstra, H. P., et al. (2016). How much is too much? (Part 1) International Olympic Committee consensus statement on load in sport and risk of injury. Br. J. Sports. Med. 50, 1030–1041. doi: 10.1136/bjsports-2016-096581
Stevens, T. G. A., de Ruiter, C. J., Twisk, J. W. R., Savelsbergh, G. J. P., and Beek, P. J. (2017). Quantification of in-season training load relative to match load in professional Dutch Eredivisie football players. Sci. Med. Football. 2, 117–125. doi: 10.1080/24733938.2017.1282163
Suarez-Arrones, L., Torreño, N., Requena, B., Sáez De Villarreal, E., Casamichana, D., Barbero-Alvarez, J. C., et al. (2015). Match-play activity profile in professional soccer players during official games and the relationship between external and internal load. J. Sports. Med. Phys. Fitness 55, 1417–1422.
Taberner, M., Allen, T., and Cohen, D. D. (2019). Progressing rehabilitation after injury: consider the ‘control-chaos continuum’. Br. J. Sports. Med. 53, 1132–1136. doi: 10.1136/bjsports-2018-100157
Varley, M. C., Jaspers, A., Helsen, W. F., and Malone, J. J. (2017). Methodological considerations when quantifying high-intensity efforts in team sport using global positioning system technology. Int. J. Sports Physiol. Perform. 12, 1059–1068. doi: 10.1123/ijspp.2016-0534
Wang, C., Vargas, J. T., Stokes, T., Steele, R., and Shrier, I. (2020). Analyzing activity and injury: lessons learned from the acute:chronic workload ratio. Sports. Med. 50, 1243–1254. doi: 10.1007/s40279-020-01280-1
Whitehead, S., Till, K., Weaving, D., and Jones, B. (2018). The use of microtechnology to quantify the peak match demands of the football codes: a systematic review. Sports. Med. 48, 2549–2575. doi: 10.1007/s40279-018-0965-6
Williams, S., West, S., Cross, M. J., and Stokes, K. A. (2017). Better way to determine the acute:chronic workload ratio? Br. J. Sports. Med. 51, 209–210. doi: 10.1136/bjsports-2016-096589
Keywords: acute chronic workload ratio, injury risk, physical performance, monitoring, external training load
Citation: Ravé G, Granacher U, Boullosa D, Hackney AC and Zouhal H (2020) How to Use Global Positioning Systems (GPS) Data to Monitor Training Load in the “Real World” of Elite Soccer. Front. Physiol. 11:944. doi: 10.3389/fphys.2020.00944
Received: 09 May 2020; Accepted: 14 July 2020;
Published: 20 August 2020.
Edited by:
Luca Paolo Ardigò, University of Verona, ItalyReviewed by:
Pantelis Theodoros Nikolaidis, University of West Attica, GreeceBeat Knechtle, University Hospital Zurich, Switzerland
Copyright © 2020 Ravé, Granacher, Boullosa, Hackney and Zouhal. This is an open-access article distributed under the terms of the Creative Commons Attribution License (CC BY). The use, distribution or reproduction in other forums is permitted, provided the original author(s) and the copyright owner(s) are credited and that the original publication in this journal is cited, in accordance with accepted academic practice. No use, distribution or reproduction is permitted which does not comply with these terms.
*Correspondence: Urs Granacher, urs.granacher@uni-potsdam.de; Hassane Zouhal, hassane.zouhal@univ-rennes2.fr
†ORCID: Urs Granacher orcid.org/0000-0002-7095-813X
‡Present address: Daniel Boullosa Sport and Exercise Science, James Cook University, Townsville, QLD, Australia