- 1Prestage Department of Poultry Science, North Carolina State University, Raleigh, NC, United States
- 2Department of Applied Ecology, North Carolina State University, Raleigh, NC, United States
The muscle myopathy wooden breast (WB) has recently appeared in broiler production and has a negative impact on meat quality. WB is described as hard/firm consistency found within the pectoralis major (PM). In the present study, we use machine learning from our PM and liver transcriptome dataset to capture the complex relationships that are not typically revealed by traditional statistical methods. Gene expression data was evaluated between the PM and liver of birds with WB and those that were normal. Two separate machine learning algorithms were performed to analyze the data set including the sequential minimal optimization (SMO) of support vector machines (SVMs) and Multilayer Perceptron (MLP) Artificial Neural Network (ANN). Machine learning algorithms were compared to identify genes within a gene expression data set of approximately 16,000 genes for both liver and PM, which can be correctly classified from birds with or without WB. The performance of both machine learning algorithms SMO and MLP was determined using percent correct classification during the cross-validations. By evaluating the WB transcriptome datasets by 5× cross-validation using ANNs, the expression of nine genes ranked based on Shannon Entropy (Information Gain) from PM were able to correctly classify if the individual bird was normal or exhibited WB 100% of the time. These top nine genes were all protein coding and potential biomarkers. When PM gene expression data were evaluated between normal birds and those with WB using SVMs they were correctly classified 95% of the time using 450 of the top genes sorted ranked based on Shannon Entropy (Information Gain) as a preprocessing step. When evaluating the 450 attributes that were 95% correctly classified using SVMs through Ingenuity Pathway Analysis (IPA) there was an overlap in top genes identified through MLP. This analysis allowed the identification of critical transcriptional responses for the first time in both liver and muscle during the onset of WB. The information provided has revealed many molecules and pathways making up a complex molecular mechanism involved with the progression of wooden breast and suggests that the etiology of the myopathy is not limited to activity in the muscle alone, but is an altered systemic pathology.
Introduction
The occurrence of wooden breast (WB) in commercial poultry production is rising, leaving producers with an inferior product and, ultimately, unsatisfied consumers (Mudalal et al., 2014; Petracci et al., 2015). Much of the incidence is thought to be attributed to artificial selection that has led to the development of broilers with greater muscle yield, better feed conversion rates, and faster growth. Frequent detection of muscle myopathies has been thought to be associated with increased growth rates and breast muscle yields (Sihvo et al., 2014; Trocino et al., 2015; Kuttappan et al., 2016; Abasht et al., 2019). WB is described as a hard or firm consistency deep within the pectoralis major (PM) muscle (Sihvo et al., 2014). Alterations of the meat composition have been observed including increased moisture, collagen, sodium, calcium and fat content (Zambonelli et al., 2014). When compared to PM without the myopathy, the PM meat quality with WB is lower due to greater cooking losses and greater shear force when compared to PM without the myopathy (Zambonelli et al., 2014; Trocino et al., 2015). Consequently, the meat texture of the breast is tougher and less desirable to the consumer. Extensive histological evaluation of WB has been characterized by necrosis, chronic fibrosis, infiltration of fat and connective tissue, and the presence of inflammatory cells and macrophages (Sihvo et al., 2014; Trocino et al., 2015; Kuttappan et al., 2016; Papah et al., 2017).
The severity of WB is often categorized on a scale ranging from 0 to 3 (0, normal; 1, mild; 2, moderate; 3, severe) (Trocino et al., 2015). WB lesions can be detected through manual palpation of the PM as early as 3 weeks of age (Mutryn et al., 2015). Research covering muscle myopathies in broilers reveals that myopathies have increased in recent years and have been correlated with the selection of larger breast muscle (Petracci et al., 2015). The cause of WB is currently unknown, but it is more prevalent in older, heavier male broilers than young birds (Kuttappan et al., 2017; Brothers et al., 2019). Larger breast muscle has been associated with hypertrophied muscle fibers which is thought to impact blood supply and number of satellite cells that are needed in muscle regeneration (Daughtry et al., 2017; Malila et al., 2019).
The increase in prevalence and severity of WB has the potential to result in excessive economic loses. It has been projected that these losses could exceed more than $200 million USD/yr (Kuttappan et al., 2016). Often, the PM muscle of birds with WB can appear pale, bulging, and covered in a clear viscous fluid (Sihvo et al., 2014). Considering consumers frequently purchase chicken breasts based on visual appearance of the meat, this unpleasant appearance will undoubtedly have a negative impact on sales (Petracci et al., 2015). Even if WB is undetectable by the outward appearance prior to purchase, the hardness of the inner muscle will become evident once the meat is handled or consumed. Ultimately, this is bad for the consumer, the company, and poultry production as a whole by breaking consumer trust and potentially initiating the desire for an alternative product.
Currently, researchers are investigating the nutritional, physiological, and genetic factors that surround this myopathy through the use of many common techniques that span from the inclusion of feed additives to molecular approaches using gene expression analysis and histology (Velleman and Clark, 2015; Kuttappan et al., 2016; Papah et al., 2018; Petracci et al., 2019). In the present study, we use machine learning analysis of PM and liver transcriptome datasets to capture the complex relationships that are not typically revealed by traditional statistical methods. This was achieved through the use of algorithms to identify genes within an extensive RNA sequencing dataset whose expression can be used to correctly distinguish normal tissue apart from severe/moderate WB. Previous gene expression datasets have characterized many molecular relationships present in birds with WB (Mutryn et al., 2015; Papah et al., 2018; Brothers et al., 2019). This study uses a different mechanism to evaluate gene expression data in the hopes for a more concise evaluation of the WB myopathy.
Materials and Methods
Facilities and Rearing
This experiment was conducted at the North Carolina State University Chicken Education Unit between the months of March and April, 2019. All procedures used in this study were reviewed and approved by the Institutional Animal Care and Use Committee. Eggs were collected from a resident 25-week-old broiler breeder flock of known, similar genetic background and stored for no more than 7 days at 15°C. Incubation was performed based on the methods described by Livingston (Livingston et al., 2018a). Day of hatch chicks were sex-sorted and a total of 128 male chicks were individually neck tagged and placed into eight replicate pens (1.2 m × 4.0 m; 4.8 m2) with 16 chicks per pen (blocked by location within the house). Each pen was supplied with one bell water drinker, two tube feeders, and bedded with fresh pine shavings (15 cm deep). Broilers were provided ad libitum access to a common commercial starter diet (1–14 days) and a common grower diet (14–45 days) manufactured at the NC State University Feed Mill (Table 1). Eight broilers from each pen, for a total of 64, were selected (based on experiment-wise mean BW) for processing at 45 days. Birds were evaluated for WB and samples of PM and liver tissue were collected for analysis of gene expression and stored at −80°C. PM tissue was sampled from the deep medial region of the birds left breast and liver tissue was sampled from caudal region of the left lobe.
Processing
At 45 days, selected broilers were collected and transported to the North Carolina State University Chicken Education Unit’s broiler processing facility followed by shackling and stunning in a salt saturated saline head stun cabinet. Birds were head stunned with a 110v/60hz CF2000 poultry stun knife set to 150 mA for 10 s. Broilers were exsanguinated for 120 s by opening of the jugular vein and carotid artery with a single knife cut by a trained technician followed by scalding in hot water (60°C) for 120 s. This was followed by feather picking for 30 s (Meyn Food Processing Technology B.V., Westeinde Amsterdam, Netherlands). Head and feet were removed, vent opened (VC Poultry Vent Cutter, Jarvis Product Corp., Middleton, CT, United States), and viscera and giblets removed manually. Liver and PM tissue were removed and snap frozen on liquid nitrogen for RNA sequencing analysis. Hot carcass weights (HCW) were collected prior to carcasses being air chilled at 3.0°C for approximately 24 h. At 24 h postmortem examination of the PM muscle was evaluated after breast muscle was removed from carcass and bones by a trained and experienced technician for WB using a one to four-point ordinal scale of measurement in accordance with the methods described previously by Livingston with a score of one being normal and four being most severe (Livingston et al., 2018b).
RNA Sequencing Analysis
Pectoralis major and liver tissue samples from 45 d broilers with varying severities of WB scores were obtained and preserved in RNALater. RNA extracted from the PM of 15 birds with moderate to severe WB was compared to RNA extracted from five normal PM. For liver RNA evaluation, 10 samples from birds with moderate to severe WB and five livers from birds with normal PM were used. RNA was extracted from the tissues using Qiagen RNeasy Mini protocol (Qiagen, Valencia, CA, United States) following the manufacturer’s instructions. The RNA quality was assessed by Nanodrop 2000 spectrophotometer (Thermo, United States). Two micrograms of RNA from each sample were taken to the North Carolina State University Genomics Sciences Laboratory for library preparation and sequencing on the Illumina HiSeq 2500 sequencer. RNA sequencing was analyzed using CLC Genomics Workbench (Qiagen, Valencia, CA, United States; licensed to NCSU) version 11 following the software manual1. RNA sequencing reads and annotations were mapped to the chicken genome (galgal5) from NCBI. Raw reads were processed by the default settings of reads’ quality control and adapter trimming. The false discovery rate p-value (FDRp) was calculated to correct for multiple testings’ and an FDR adjusted p ≤ 0.05 was considered statistically significant. Fold change and Log2 fold change differences in gene expression between WB scores moderate to severe and normal were also calculated.
Machine Learning Analysis
Gene expression data were analyzed from the PM and liver using the Waikato Environment for Knowledge Analysis (WEKA) version 3.8.3. Two different pattern recognition machine learning algorithms were performed to analyze the data set: sequential minimal optimization support vector machines (SVMs) and artificial neural network multilayer perceptron (MLP) (Cortes and Vapnik, 1995; Eibe et al., 2016). The machine learning algorithms were compared to identify gene expression patterns within the data set of 15,569 genes, which could be used to correctly classify birds as either exhibiting moderate to severe WB or normal (dichotomous class assignment). Briefly, the 15,569 genes from the gene expression dataset were ranked based on Shannon Entropy (Information Gain) in dichotomous classification assignment by SVMs (Keerthi et al., 2001; Eibe et al., 2016). Information gain ranking was then used to identify those gene expression patterns most relevant to assignment of each bird as having WB or normal by either MLP or SVMs. Reduction of data dimensionality for each machine learning algorithm was then performed by sequential exclusion of those gene expression patterns least relevant to the class assignment (50–2,000 per sequence). This step eliminates overfitting of the machine learning classifiers. To identify the minimum number of gene expression patterns required for classification, 1–50 genes were sequentially excluded from the dataset until only the top 2–7 remained. This step identifies underfitting of the machine learning classifiers and the point of optimal classification for the MLP and SVMs was determined to be the intersection between the underfitting and overfitting curve plots.
Class assignment of all machine learning algorithms was evaluated vis-à-vis by two cross-validation strategies (classification as either WB or normal). The first being a percentage split, where 66% of the total data were randomly used for training and the remaining 34% of the data were used in testing. The second cross-validation was a stratified hold-out (n-fold) method with 5-fold, where 4-fold of the randomized gene expression data were used for the training and 1-fold was used for testing. This was repeated five times, such that all normal replicate samples were used at least once in testing and the average model performance was recorded.
The performance of the two machine learning algorithms SVMs and MLP was determined using percent correct classification during the cross-validations, which indicated the likelihood that each individual biological replicate could accurately be assigned into the classes of WB or normal based on the gene expression data provided. Kappa statistic and ROC score also were recorded. Any kappa statistic greater than 0 indicated that the machine learning classifier is performing better than random chance along with a ROC score of greater than 0.500. The random probability of chance for dichotomous assignment was assumed to be 50% based on the Law of Probability.
A negative control of machine learning was created through 10 separate randomizations of the individual birds within the dataset. The SVMs and MLP were unable to predict WB or normal, indicating the machine learning herein is true.
Pathway Analysis
Ingenuity Pathway Analysis (IPA; Qiagen, Valencia, CA, United States)2 software was used for canonical pathway analysis, upstream regulatory analysis, and gene network discovery. SVM analysis of the top 450 performing differentially expressed genes from the PM of birds with moderate/severe to normal WB were used in IPA and the top 150 were used from the liver dataset. IPA calculation of z-scores using the gene expression fold change values measures the state of activation or inhibition of the molecules involved in the molecular networks. The analysis of biological mechanisms occurring in the differentially expressed genes of the chicken in IPA are based on mammalian systems for human, rat, and mouse.
Results
Multilayer Perceptron
By using the novel approach of evaluating the WB PM transcriptome dataset by 5-fold cross-validation using MLP, the expression of nine genes (NUP43, KPNA7, DEAF1, NUD19, CCDC85A, SLC25A30, ENSGAL00000015075, PACSIN3, and RPL19) were able to correctly classify if the PM tissue from an individual bird was normal or exhibited WB in 100% of the individual genomes when using the top nine genes ranked based on Shannon Entropy (Information Gain) (Figure 1). MLP is an artificial neural network, which can distinguish data that are not linearly separable, but instead is a feed forward mechanism that maps a dataset into suitable outputs. The top nine genes in the PM were further analyzed for their individual biological roles (Table 2). When the liver transcriptome dataset was evaluated by 66% split using MLP, the expression of 75 genes were capable of correctly classifying the PM tissue of an individual bird as exhibiting WB or normal in 100% of the individual genomes (Figure 2). The kappa statistic and ROC score were optimal at 1 for both PM and liver. The individual expression of transcripts CARD19 and ITCH predicted WB or normal using MLP 5-fold cross validation 93.333% of the time whereas BUD13 and ENSGALG00000039590 individually predicted WB or normal 100% of the time using 66% split (Table 3).
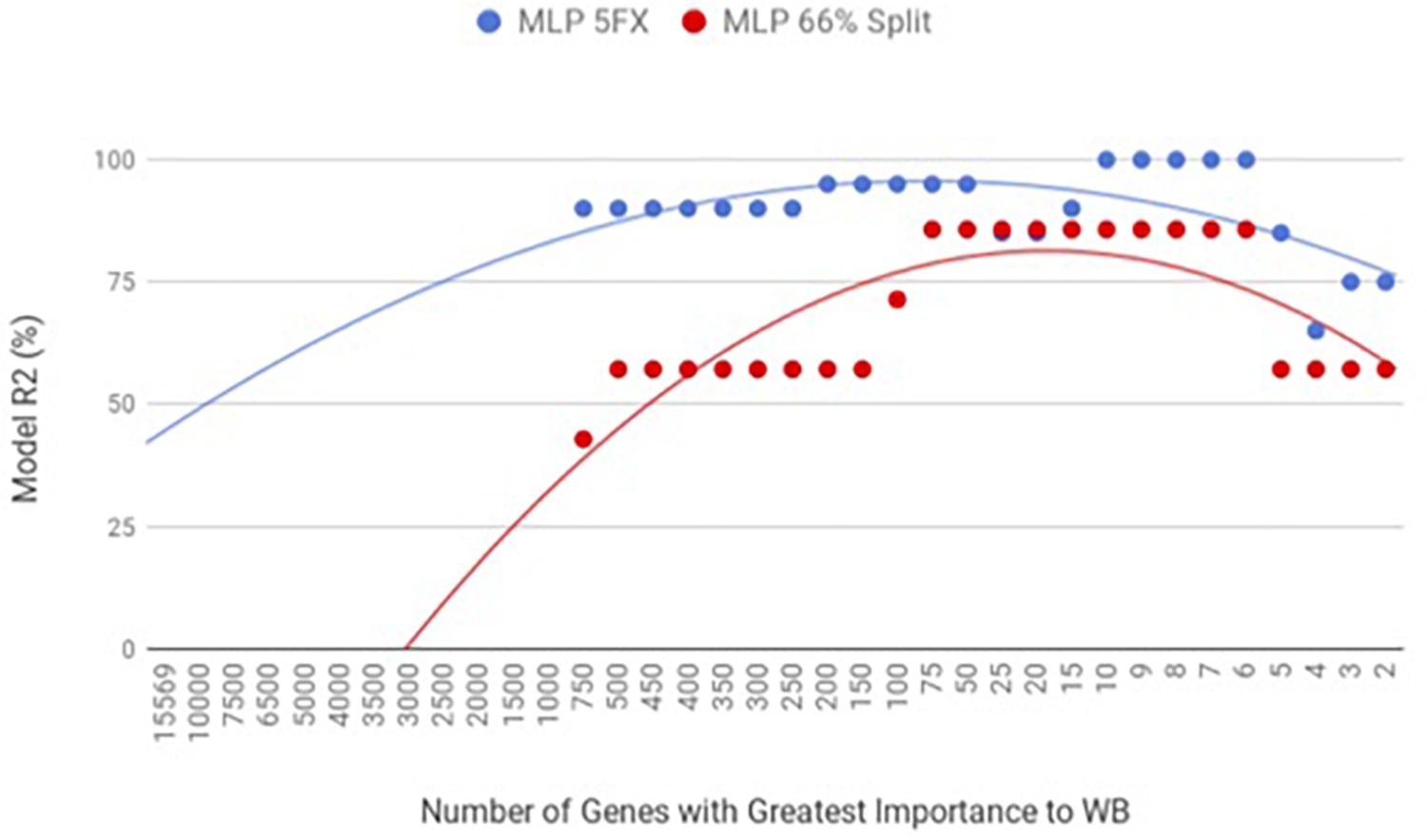
Figure 1. WB PM transcriptome dataset by 5x cross-validation and 66% percent split using Multilayer Perceptron (MLP).
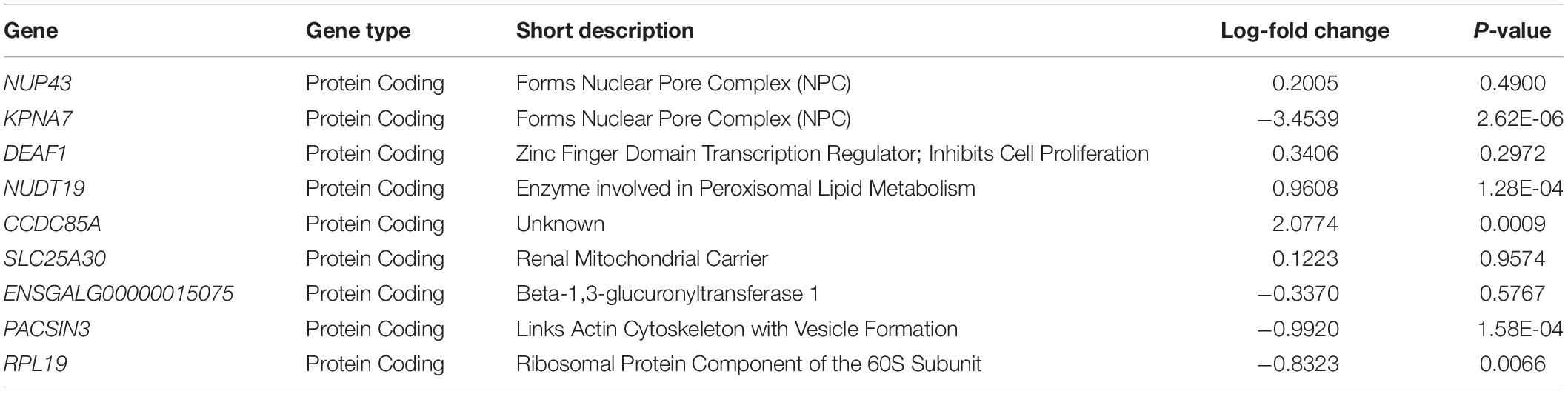
Table 2. Top nine regulators of differential expressed genes in the PM of birds with normal PM compared to severe/moderate WB by 5-fold cross-validation with 100% correct classification and percent split with 85.71% correct classification using MLP.
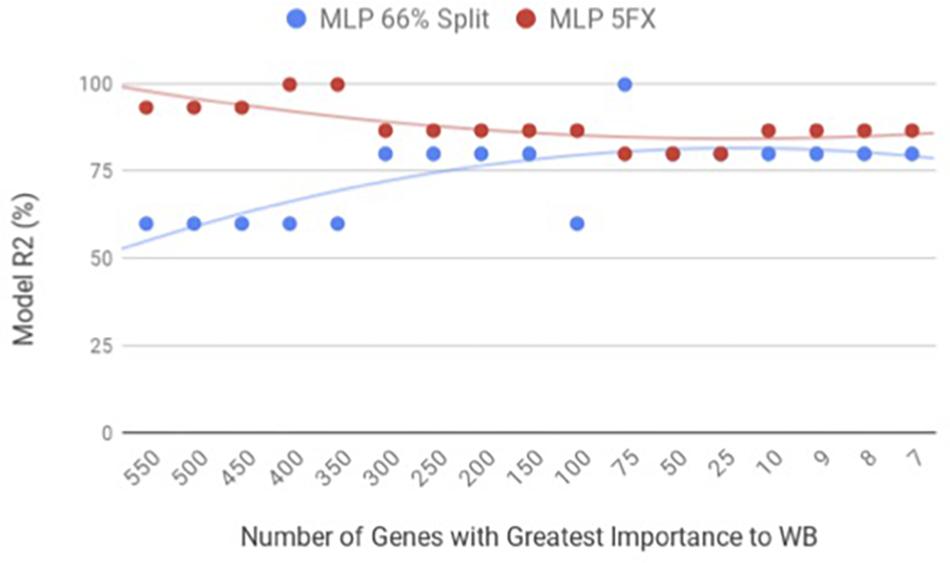
Figure 2. WB Liver transcriptome dataset by 5x cross-validation and 66% percent split using Multilayer Perceptron (MLP).
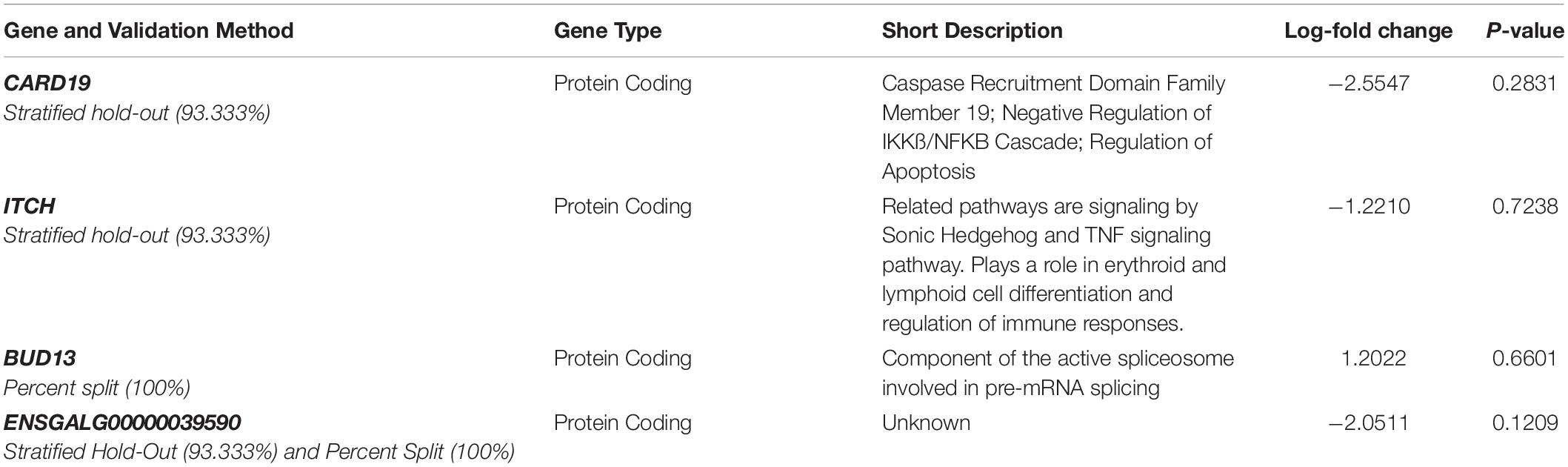
Table 3. Top regulators of differential expressed genes in the liver of birds with normal PM compared to severe/moderate WB tissues using MLP.
Sequential Minimal Optimization
When the PM gene expression data set was evaluated between birds with WB and those that were normal using WEKA SMO function of SVMs by a 5-fold cross-validation method they were correctly classified 95% of the time using 450 of the top genes ranked based on Shannon Entropy (Information Gain) as a preprocessing step (Figure 3). The kappa statistic and ROC score were optimal at 0.8571 and 0.9000, consecutively. The liver gene expression data set was evaluated using the SMO function of SVMs by both a 5-fold cross-validation method and the 66% split method was capable of predicting WB or normal 100% of the time with 100 to 200 of the top genes ranked based on Shannon Entropy (Information Gain) (Figure 4). Optimal kappa statistic and ROC scores of 1 were achieved for both methods. In both machine learning algorithms, the stratified hold-out method appeared to accurately estimate the machine learning classifier correctly more often than the percentage split method.
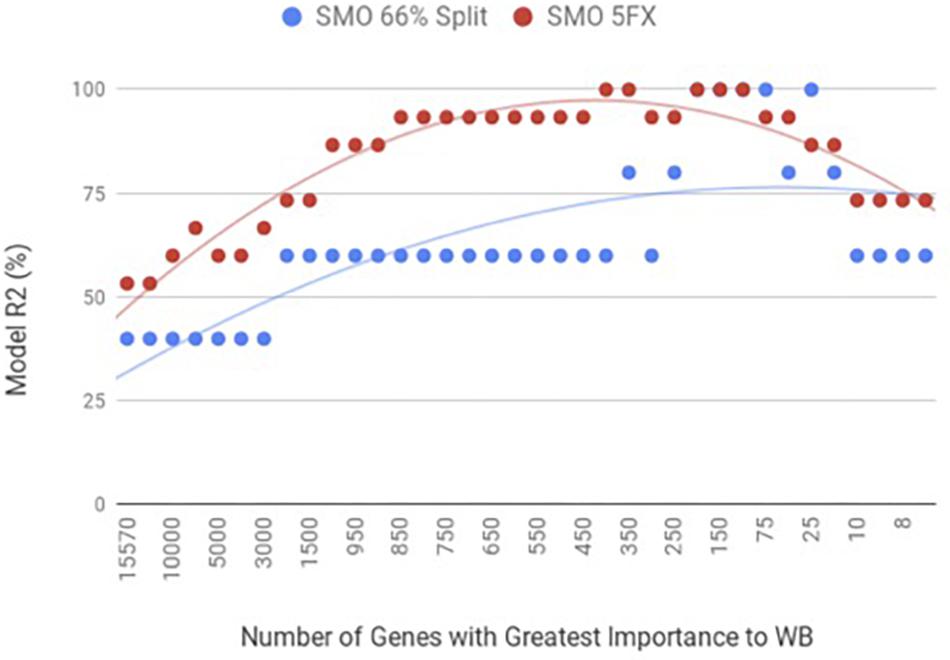
Figure 3. WB PM transcriptome dataset by 5x cross-validation and 66% percent split using Sequential Minimal Optimization (SMO).
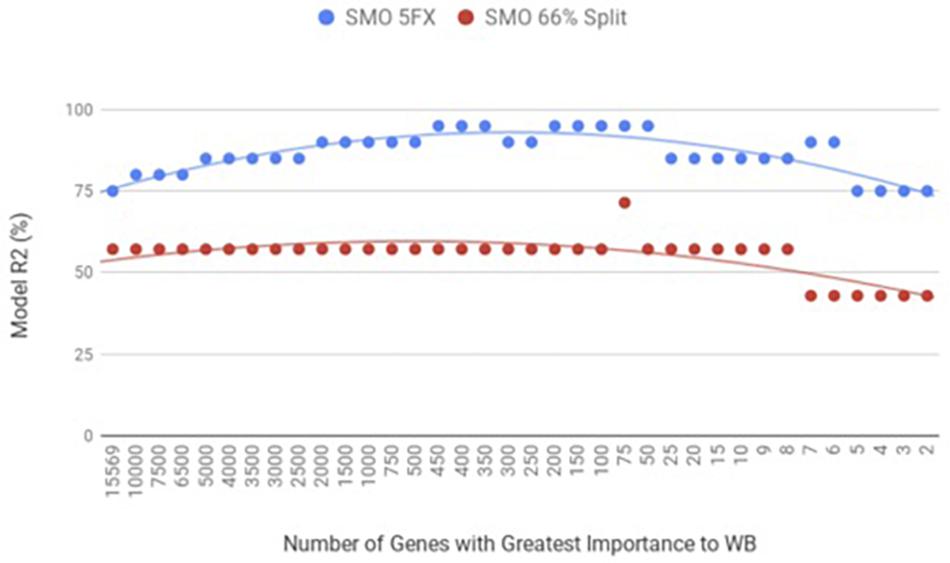
Figure 4. WB Liver transcriptome dataset by 5x cross-validation and 66% percent split using Sequential Minimal Optimization (SMO).
Ingenuity Pathway Analysis
Lastly, Ingenuity Pathway Analysis (IPA) was used to evaluate the top 450 genes ranked based on Shannon Entropy (Information Gain) from the PM transcriptome using SMO and 150 of the top genes ranked based on Shannon Entropy (Information Gain) for the liver transcriptome using SMO. Interestingly, many of the top analysis ready molecules detected in IPA were the same molecules detected using the completely separate machine learning approach of MLP. The repeatability in identification of these genes leads to greater confidence in the role they are having in the WB myopathy. These included BUD13 from the liver transcriptome dataset and CCDC85A and KPNA7 from the PM dataset (Tables 2, 3).
The top associated network function in IPA for the PM transcriptome dataset was for RNA Damage and Repair, Protein Synthesis. This pathway involves RPL19 which is a component of the 60S ribosomal subunit detected through MLP of WEKA (Figure 5). When evaluating the liver transcriptome dataset three top network associations identified were Skeletal and Muscular System Development and Function, Developmental Disorder, Hereditary Disorder (Figure 6), Connective Tissue Disorders, Hematological Disease, Hereditary Disorder (Figure 7), and Cell Cycle, Embryonic Development, Cellular Movement (Figure 8). Figure 6 depicts CARD19 which was also detected using MLP of WEKA. This network is an association of the relationship this group of molecules has in association with the skeletal and muscular system development and function. Figure 8 represents molecules in the cell cycle such as TGF-β, MAP2K1/2, and calcineurin proteins which are important in skeletal muscle myoblast regulation and differentiation.
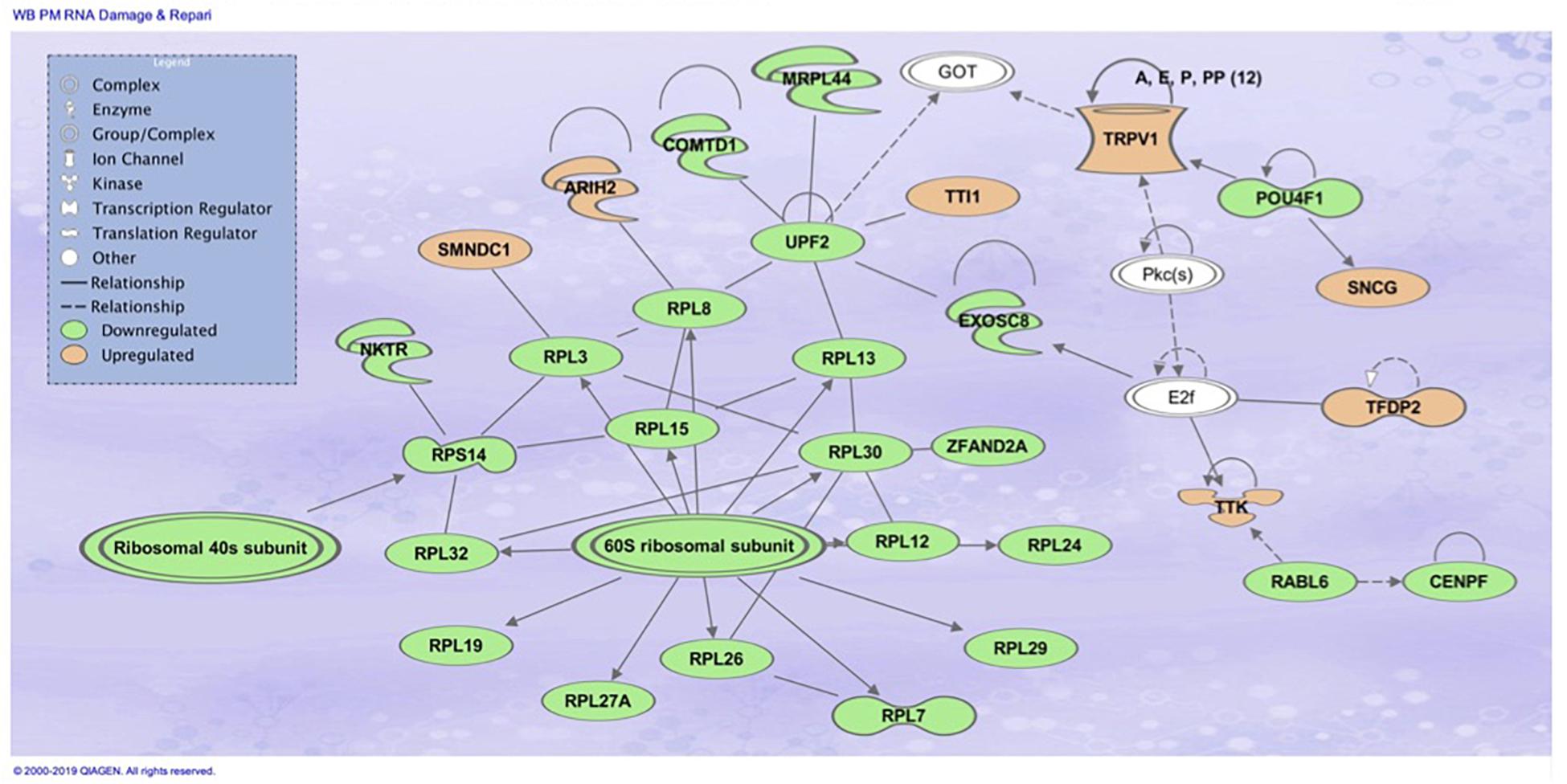
Figure 5. Top associated network function in IPA for the PM transcriptome dataset of birds with normal PM compared to severe/moderate WB: RNA Damage and Repair and Protein Synthesis.
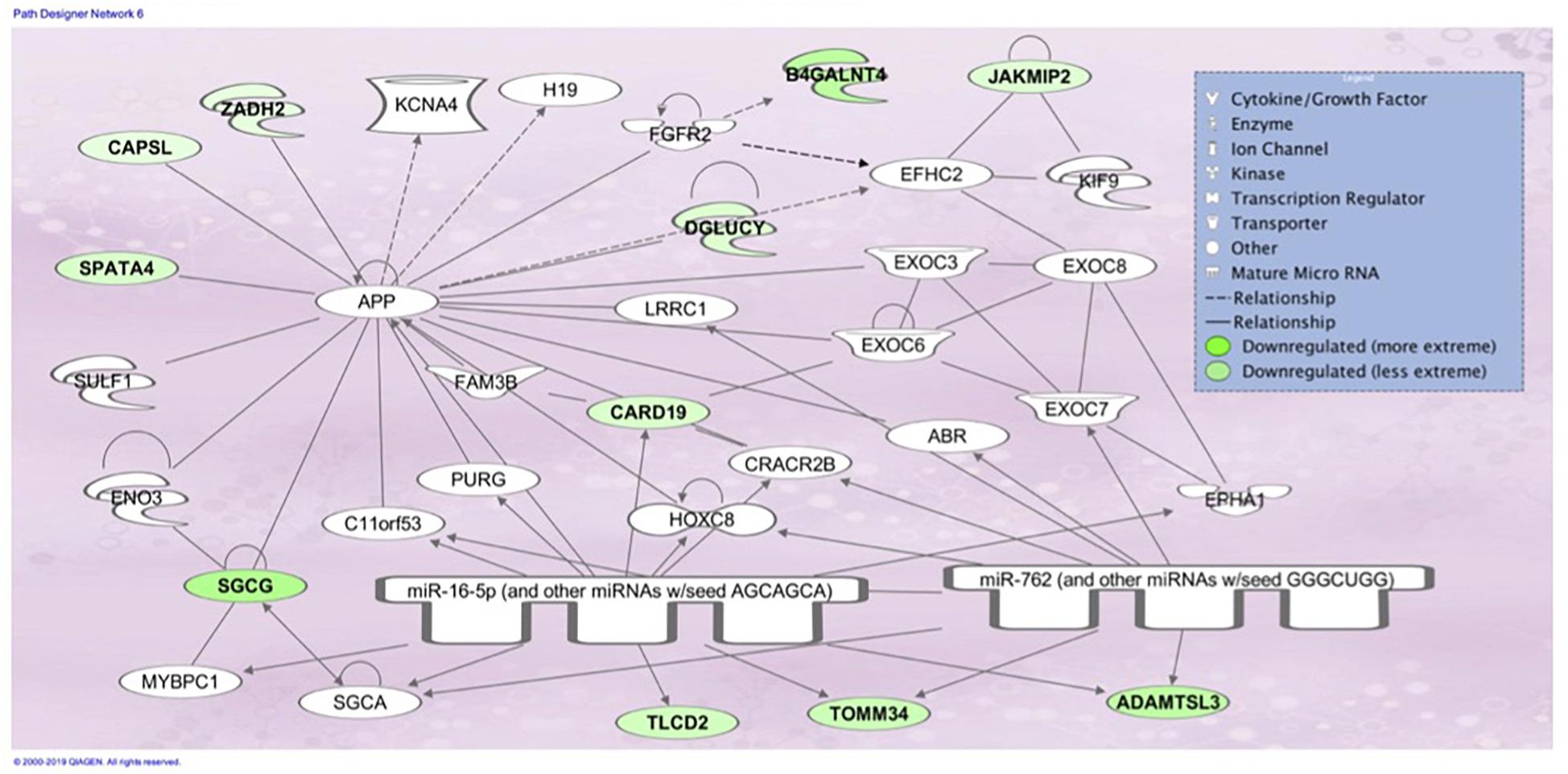
Figure 6. Associated network function in IPA for the liver transcriptome dataset of birds with normal PM compared to severe/moderate WB: Skeletal and Muscular System Development and Function, Developmental Disorder, Hereditary Disorder.
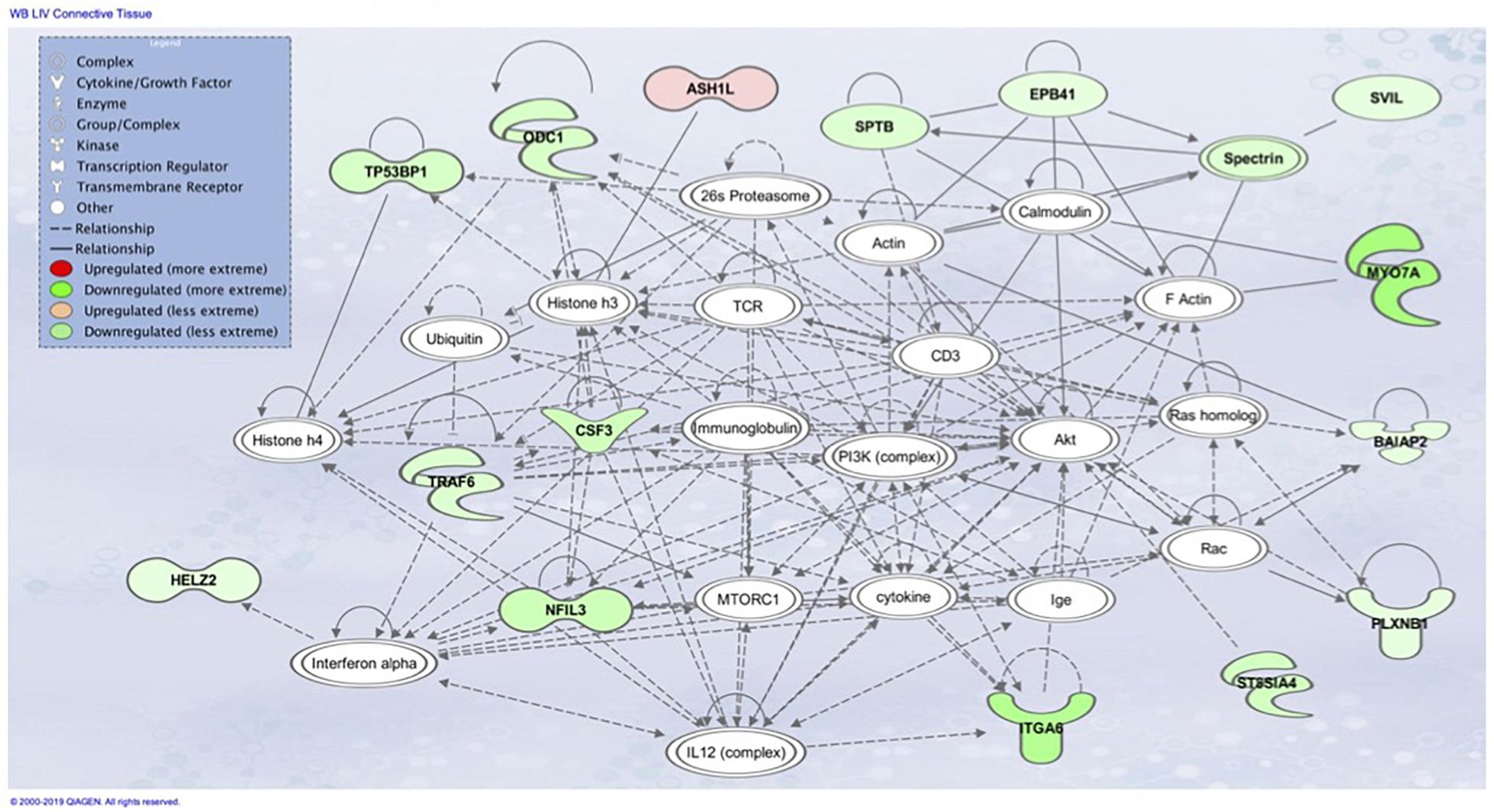
Figure 7. Associated network function in IPA for the liver transcriptome dataset of birds with normal PM compared to severe/moderate WB: Connective Tissue Disorders, Hematological Disease, Hereditary Disorder.
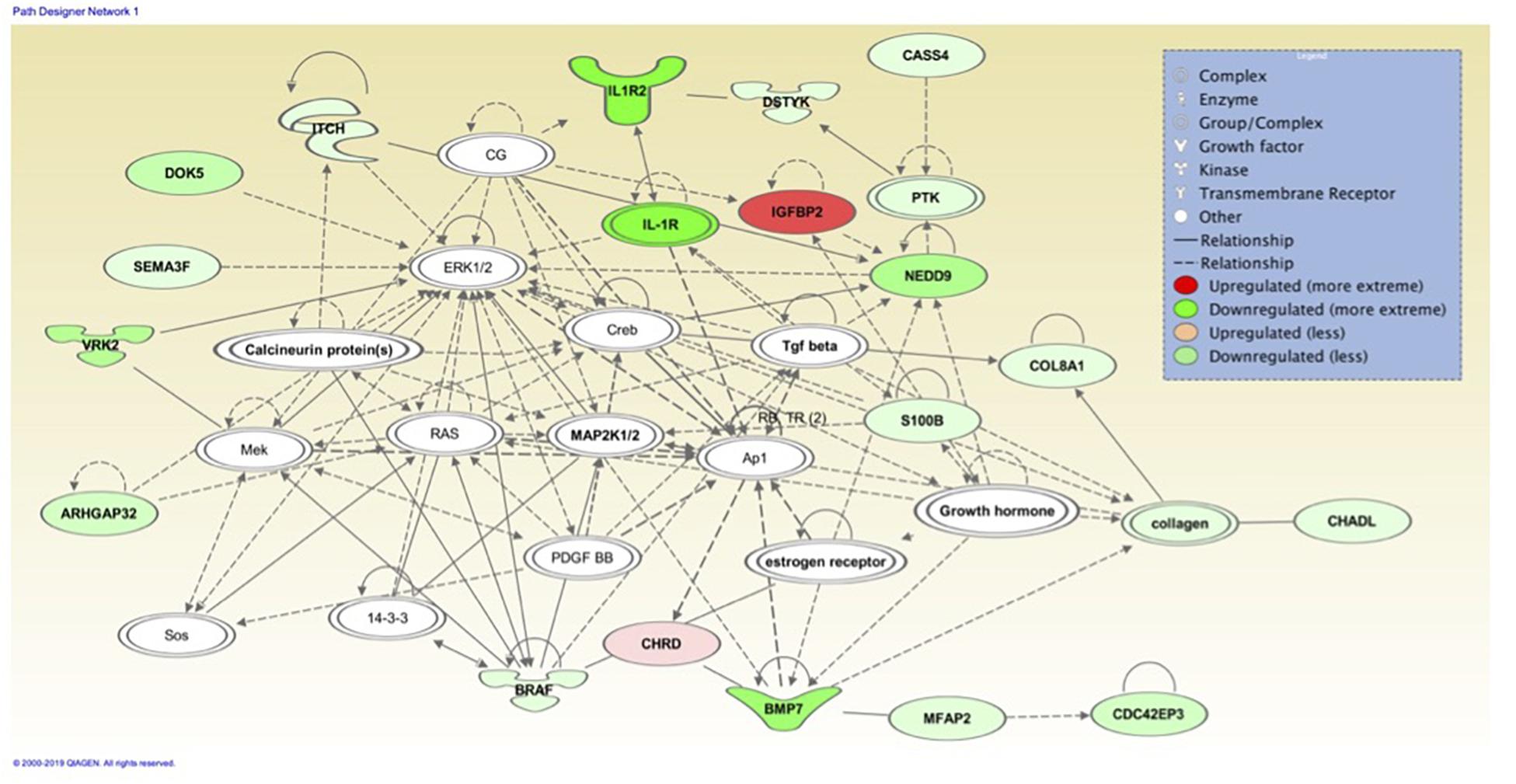
Figure 8. Associated network function in IPA for the liver transcriptome dataset of birds with normal PM compared to severe/moderate WB: Cell Cycle, Embryonic Development, Cellular Movement.
When comparing the performance of the top ranked genes between MLP and SVM, many genes were identified as top performing in both ML models. In the PM data set, evaluation of the top nine molecules with increased expression, KPNA7 was observed in both MLP (100% classification) and the top analysis ready molecules in IPA from the best performing data set using SVM (Table 2). RPL19 was observed as a top regulator in MLP with the ability to correctly classify WB or normal 100% of the time and was an affected molecule in the IPA analysis of the top associated network (Figure 5). In the same data set CCDC85A was observed as a molecule with decreased expression in both ML models and able to correctly classify WB 100% of the time using MLP (Table 2).
Assessing the liver dataset there were many overlaps using the two ML models. BUD13 was a top regulator in MLP and a top analysis ready molecule with increased expression in IPA (Table 3). The associated network related to skeletal and muscular system development and function from IPA had CARD19 as a downregulated molecule in IPA and a top regulator in MLP that was able to predict WB or normal 93.33% of the time (Figure 6). Lastly, the IPA network associated with cell cycle, embryonic development, and cellular movement included the down regulation of the ITCH molecule which was also detected in MLP as being able to correctly classify 93.33% of the time (Figure 8).
Discussion
Prior systematic studies of the abnormal muscle phenotype referred to as wooden breast using gene expression measurements has identified multiple processes that may contribute to the development of the myopathy. Processes including muscle fiber regeneration, inflammatory response, myodegeneration, hypoxia, fibrosis, lipidosis, and altered energy metabolism are likely involved in the manifestation of wooden breast (Mutryn et al., 2015; Zambonelli et al., 2016; Papah et al., 2018; Malila et al., 2019; Petracci et al., 2019). This study attempts to connect organismal level physiology and metabolism with the activity in the breast muscle by including the evaluation of liver gene expression by a novel analysis method.
Machine Learning Approach
Traditional statistics is not well designed to handle datasets which have more variables than observations therefore this is an alternative method to analyze and make interpretations of datasets. Using the traditional statistical approach on this dataset resulted in almost 1000 genes being identified as differentially expressed (FDR corrected p < 0.05) across the etiology of WB in the PM and only one gene in the liver. This large number of targets (or too few) when subjected to pathway analysis in IPA results in more pathways (or none) than can be interpreted, therefore an alternative approach was warranted. The use of machine learning on the transcriptome datasets allows for the recognition of consistent patterns or systematic relationships within the datasets and therefore can be used to make predictions. Through this process, the machine learns by building a model from example inputs and then makes predictions on new data by the learned pattern recognition. This is the first report using machine learning to identify gene expression patterns associated with WB in muscle as well as peripheral tissues that may be influencing the myopathy development.
PM Fibrosis
Evaluating the top nine genes ranked based on Shannon Entropy (Information Gain), all the genes are protein coding genes (Table 2). The top two genes NUP43 and KPNA7 are two essential Nuclear Pore Complexes (NPC). KPNA7 was also observed as one of the top nine upregulated genes with a fold-change for birds with WB (Mutryn et al., 2015). NPC’s are macromolecular proteins found within the nuclear envelope in eukaryotic cells. These complexes are surrounded by decondensed chromatin and are responsible for the exchange of large molecules such as proteins and RNA between the nucleus and cytoplasm (Kelley et al., 2010). Prior to cell division there is an increase in expression of NPC found in the nuclear envelope (NE). Once mitosis begins the NE is broken down and NPCs form subcomplexes, which are essential for later reassembly of the NPC. This process of reconstruction is unknown, however, it has been shown when the KPNA7 NPC is depleted, mitotic defects and deformation of the nucleus occur (Vuorinen et al., 2018). In the mouse, KPNA7 is mostly expressed in oocytes and zygotes and is responsible for epigenetic reprogramming which occurs during fertilization and zygotic gene activation (Hu et al., 2010). Recently, NPCs have been observed in regulation of gene expression and have been associated with both gene silencing and activation (Galy et al., 2000; Casolari et al., 2004). The expression of nucleoporins, which make up the NPCs, vary depending on cell types and changes in the structure of NPCs are used in the regulation of cellular differentiation (D’Angelo, 2018). In mammals, the tissue specific NPC, Nup210 has an effect on the regulation muscle development and maintenance. Nup210 regulates myofiber maturation, growth and even survival through the use of a dependent transcription factor Mef2C in the regulation of structural and maturation related muscle genes (D’Angelo et al., 2012; Raices et al., 2017). In the absences of Nup210 the initial formation of muscle fibers occurs during development, however in older animals abnormal muscle structure develops and muscle degeneration can even occur (Raices et al., 2017).
PM Regeneration
Another top gene, DEAF1, is a part of the SAND domain in the molecule Ski. The activity of Ski was originally identified in the chicken as a transduced retroviral oncogene, however, research has indicated homologs which are not associated with endogenous viral loci (Li et al., 1986). The c-Ski residue is primarily found in the nucleus and is highly conserved in many species. The SAND domain is involved in protein-protein interactions and is responsible for the interactions of SKI with Smad4, FHL2, and MeCP2 (Engle et al., 2008). Ski can act as an activator or repressor to gene transcription depending on the transcription factor it interacts with. C-Ski has been shown to bind with Smad4 and block activation of transforming growth factor (TGF-β). TGF-β leads to an increase in β-catenin within the cytoplasm and β-catenin is an activator of canonical Wnt signaling (Staal, 2016). TGF-β, TNF-α, and IGF-2 are growth factors that regulate myoblast differentiation rather than activation (Rosenthal et al., 1991; Li et al., 2005; Carter et al., 2009). The key role of TGF-β in muscle repair is to regulate the balance between fibrosis and regeneration. In Japanese quail, myogenic differentiation is induced through the activation of myogenic regulatory factors (MRFs), MyoD, and myogenin as well as inhibiting HDAC activity (Colmenares and Stavnezer, 1989; Kobayashi et al., 2007). Satellite cells are activated by expression of early myogenic regulatory factors, MyoD and Myf5. Later, myogenin and MRF4 are expressed. The Ski response element to myogenin is located upstream of the promoter region.
The gene RPL19 was discovered as one of the top nine regulators in the MLP model able to 100% correctly classify PM samples as WB or normal sample. This protein coding gene encodes a ribosomal protein that makes up the 60S ribosomal subunit (Davies and Fried, 1995). Like many of the genes observed, RPL19 also plays a role in genetic regulation. In mammals, this protein contains a CpG island at the 5′ transcriptional start site, which would indicate an area for modifications to the expression of this gene (Davies and Fried, 1995). RPL19 was also involved in the top molecular network associated with RNA damage and repair protein synthesis when evaluating the 450 attributes that were 95% correctly classified using SMO through Ingenuity Pathway Analysis (IPA) (Figure 5). Transcripts related to spliceosomes were also detected in IPA analysis in Figure 6, involving the previously mentioned gene BUD13 as a component of the spliceosome. Similar to this finding previous research evaluating gene expression data in the PM has indicated differential expression of small nucleolar RNAs including snoRNAs and miRNAs, which are often involved in ribosomal and protein synthesis (Zambonelli et al., 2014).
CCDC85A, another top regulator identified with MLP, was able to correctly classify as a single attribute WB and normal PM birds 95% of the time using stratified cross-validation. CCDC85A was also a top analysis molecule when evaluating the 450 attributes that were 95% correctly classified using SMO through Ingenuity Pathway Analysis (IPA). CCDC85A is a protein coding gene for coiled coil domain containing 85a (Iwai et al., 2008). In humans, CCDC85A is regulated by p53 and results in the degradation of β-catenin. This protein suppresses β-catenin activity through interaction with T-cell factors to result in Wnt signaling (Iwai et al., 2008). β-catenin is an activator of canonical Wnt signaling (Staal, 2016). Wnt signaling is responsible for the activation of satellite cells in adult skeletal muscle and perturbations of this pathway can result in muscle fibrosis (Cisternas et al., 2014). If Wnt signaling occurs too often, the satellite cells become exhausted and eventually lose the ability to renew (Ryall et al., 2008). This has been characterized by increased extracellular matrix molecules such as fibronectin, collagen, and macrophages leading to the inability of muscles to regenerate and ultimately the loss of activity, leading to similar traits associated with WB (Cisternas et al., 2014). The addition of Wnt3A protein in mice has been shown to increase the rate at which progenitor cells are converted from a myogenic to a fibrogenic state resulting in increased deposition of connective tissue (Brack et al., 2007). The expression of myogenic regulatory factors (MRF) responsible for normal formation of new myotubes, such as MyoD, Myf5, myogenin, and Pax3/7, are activated by Wnt (Yokoyama and Asahara, 2011). However, in the event that these progenitor cells lose the ability to mediate repair, the muscle tissue is replaced by adipose and fibrotic tissue, which also appears to be a phenotype associated with WB (Laumonier and Menetrey, 2016). Our findings were not the first to detect changes in Wnt signaling due to WB. Others have shown that WB results in statistically significant differential expression of WNT7A (Zambonelli et al., 2014). Wnt7a is responsible for stimulating skeletal muscle growth and repair through the induction of satellite cells via the mTOR pathway (Bentzinger et al., 2014). In contrast, others have found that there is an increase in gene expression of the MRF’s however, they tend to vary depending on the lineage of the bird used (Velleman and Clark, 2015). This has led to a different understanding of the disease state which may not be entirely genetic or environmental but rather both, which is commonly referred to as epigenetic. It could be that environmental conditions stimulate pathways leading to genomic modifications, potentially resulting in phenotypic alterations.
Our working hypothesis, based on the ML analysis, is that the underlying mechanism resulting in fibrosis and hence, WB, is related to genetic regulation, possibly through NPCs, CCDC85A, and β-catenin. These activate the Wnt signaling pathway via TGF-β, mTOR and IGF-II pathways, potentially resulting in WB pathology. It is possible that a pattern of Mendelian inheritance does not result in direct causation of WB, but rather modifications that result in changes in the expression of genes such as histone modifications and DNA methylation.
Following damage or rapid growth, skeletal muscle satellite cells are failing to regenerate myoblasts and results in fibrotic scar tissue; overall, because stem cells are restricted to a limited number of divisions, we hypothesize satellite cells are being exhausted and eventually resulting in WB (Sacco et al., 2010). This is similar to the hypothesis presented by Daughtry et al. (2017), who thought that a disruption in satellite cell homeostasis was involved in muscle myopathies. Throughout the life of an organism, the number of satellite cells available for regeneration of cells decreases. For satellite cells, aging has been characterized by delayed activation and the inability to proliferate and differentiate. A decrease in the efficiency of Wnt, TGF and IGF signaling pathways has been shown to limit satellite cell proliferation and myoblast differentiation (Barton-Davis et al., 1998; Carlson et al., 2009). It is known that fast twitch muscle is the leading muscle type found in the PM of broilers. Fast twitch muscle has fewer satellite cells than those of slow twitch resulting in differences in the course of muscle regeneration (Collins and Partridge, 2005). Differences depicted in fast twitch fibers include the TGF-β expression pattern, early activation of the myogenic regulatory factors, and better regeneration efficiency (Zimowska et al., 2009, 2017). After injury, satellite cells are activated by expression of early myogenic regulatory factors, MyoD and Myf5. Next, late myogenic regulatory factors are expressed, which consist of myogenin and MRF4. Pax 3/7 are paired box transcription factors that directly and indirectly regulate myogeneic regulatory factors as skeletal muscle progenitor cells. Together these altered pathways are likely contributing to the development of the PM myopathy.
Organismal Metabolic Influence – Liver Transcriptome
The liver was considered as a tissue of importance related to WB due to the vast array of metabolic functions including the responsibility to synthesize, metabolize and excrete many molecules (Zaefarian et al., 2019). In the bird, 11% of all protein synthesis occurs in the liver which are then transported via systemic circulation to other tissues (Denbow, 2000). Evaluation of the liver transcriptome dataset using IPA resulted in the identification of Skeletal and Muscular System Development and Function, Developmental Disorder, Hereditary Disorder (Figure 6), Connective Tissue Disorders, Hematological Disease, Hereditary Disorder (Figure 7) and Cell Cycle, Embryonic Development, Cellular Movement (Figure 8) network associations. Similarly when evaluating the PM through IPA analysis of differential gene expression data, connective tissue disorders, embryonic development and cell cycle pathways have previously been detected (Mutryn et al., 2015).
The association network Skeletal and Muscular System Development and Function, Developmental Disorder, Hereditary Disorder (Figure 6) depicts molecules involved in muscle function and development such as SGCG which protects and maintains the structure of muscle cells through the sarcoglycan protein. In mammals, mutations of this gene result in the loss of y-sarcoglycan protein and ultimatley muscle dystrophy and fibrosis (Heydemann et al., 2009). Disfunction in SGCG has been shown to result in enhanced TGF-β availability and therefore increased SMAD signaling leading to fibrosis (Heydemann et al., 2009). It is thought that proteins from this gene could be mediating their effect by regulating myostatin activity. Myostatin (MSTN), a family member of TGF-β, inhibits myoblast differentiation by repressing myogenic regulatory factors (Langley et al., 2002). MSTN prevents differentiation via the transcription factor SMAD3, which can be activated by both TGF-β and MSTN. Other molecules identified in IPA with changes in expression due to WB were JAKMIP2, which is invovlved in microtubule binding and CAPSL which is involved in calcium ion binding. This IPA pathway has also been detected when evaluating the PM of differential gene experssion between male and females birds with WB (Brothers et al., 2019).
CARD19 in this molecular network was also detected in MLP of WEKA as being able to predict normal or WB 93.333% using the cross-validation method (Figure 8 and Table 3). CARD proteins (caspase recruitment domain) are a domain of proteins which regulate apoptosis and inflammation (Jang et al., 2015). Studies evaluating CARD19 and its role in the IKKß and NF-kB pathway have been contradictory. Early data suggests CARD19 is a negative regulator of NF-kB, which is a transcription factors that signals IKK, however, a more recent study in mice suggests in the absence of CARD19 there was an increase in TNF-α which would subsequently increase IKKß and NF-kB (Rios et al., 2018). IKKß has been shown to decrease β-catenin activation which as previously mentioned is an activator canonical Wnt signaling (Lamberti et al., 2001; Staal, 2016).
Downstream TNF-α kinases IKKß and NF-kB play many roles in regulating physiological reactions including regulators of tuberous sclerosis complex (TSC), which repress the mechanistic Target of Rapamycin (mTOR) pathway (Lee et al., 2007). IKKß in association with TSC allows for the activation of mTOR. MTOR coordinates cell growth and is the major regulator of metabolic processes (Figure 7). Many factors are responsible for the activation of mTORC1 including Wnt signaling, growth factors, and TNF-α through interaction with TSC (Laplante and Sabatini, 2009). Activation of mTORC1 positively results in cell growth and proliferation through the activation of protein and lipid synthesis pathways. Disruptions in these pathways is associated with tumor development and fibrogenesis, and macrophage regulation. Ribosome biogenesis has been observed to be promoted through activation of mTORC1 by transcription of ribosomal RNA which can be observed as being downregulated in Figure 5 and Table 3 of the PM dataset (Mayer et al., 2004). Much of the IPA network association Connective Tissue Disorders, Hematological Disease, Hereditary Disorder invovles moleculues related to cell adhesion and cytoskeleton (Figure 7). Intersestingly, mTORC2 regulates cytoskeleton organization (Jacinto et al., 2004).
Cell Cycle, Embryonic Development, Cellular Movement (Figure 8) network association represents molecules in the cell cycle such as: ITCH, NEDD9, DOK5, and IGFBP2. ITCH in this molecular network was also detected in MLP of WEKA as being able to predict normal or WB 93.333% using the cross-validation method. The Itch protein encodes a member of the Nedd4 family ubiquitin ligases that targets specific proteins for lysosomal degradation. Itch plays a role in lymphoid cell differentiation and the regulation of immune response and pathways related to this protein include the TNF-α signaling pathway. NEDD9 plays a role in the TGF-β pathway and growth signals initiating cellular proliferation and has been identified as upregulated in hepatic fibrosis (Dooley et al., 2008). DOK5 in humans is strongly expressed in muscle and is involved in the positive activation of MAPK and possibly insulin activation (Grimm et al., 2001; Cai et al., 2003). DOK5 has been identified as a membrane associated protein triggered by insulin-like growth factor binding protein-5 (IGFBP-5) for intracellular signaling resulting in pro-fibrotic effects and is thought to promote fibrosis (Yasuoka et al., 2014). IGFBP2 is an insulin-like growth factor binding protein involved in cellular signaling. While muscle is the primarily a site for glucose disposal, the avian liver does function to control muscle growth through the allocation of resources by regulating the birds nutritional balance. The muscle has a paracrine effect, whereas the liver has an endocrine role in circulating IGFs and IGFBPs. Signaling pathways in the liver play an important role in regulating many aspects of energy metabolism and cell cycle processes (Nguyen et al., 2014). Circulating molecules have been identified that play a role of signaling between the liver and muscle (Liu et al., 2013). Some signaling pathways are only responsive within the liver while others are only responsive in the muscle (proliferation and differentiation). Most of the IPA pathways identified resulted in an effect which would be detected within skeletal muscle. Liver and muscle are important tissues in understanding regulation of metabolic homeostasis, genes involved in glycogenesis, glycolysis, and lipogenesis are responsive in both liver and muscle even though the expression patterns are very different between the two tissues. It is not unexpected to observe effects of the liver on muscle development since the liver is the major location of protein, lipid, and carbohydrate metabolism supporting the rapid growth of broiler chickens.
Histological evaluation of WB has revealed multifocal degeneration and necrosis of fibers and accumulation of immune related cells such as macrophages, heterophils and lymphocytes (Sihvo et al., 2014; Trocino et al., 2015; Kuttappan et al., 2016). Affected areas have characteristics of fibrosis separating muscle fibers and thickening of the interstitium. Fibrosis has been characterized as hardening or scaring of tissue as a result of the accumulation of the extra cellular matrix proteins, including collagen and fibronectin eventually leading to loss of activity to the tissue (Wynn, 2008). Fibrosis has been detected in various tissues lung, liver, kidney and skeletal muscle, however, the mechanism resulting in fibrosis has been similar in tissues (Cisternas et al., 2014). Pathways detected through IPA of the liver and PM have indicated many molecules which directly and indirectly lead to tissue fibrosis. The fibrosis associated with WB may be driven by signal(s) originating in the liver or other tissues.
Conclusion
In conclusion, using a machine learning approach, we were able to identify predictors that were able to accurately differentiate normal tissue from WB tissue using liver and PM transcriptomes from individual birds. Through the use of IPA, predictors from both PM and liver tissue identified gene networks associated with skeletal muscle disorders and other networks that could be associated with the development of WB. Given that gene expression data from both PM and liver transcriptomes were able to predict WB or normal tissue using select genes, with some redundancy between tissues, suggests that WB is the result of systematic disruptions in one or more regulatory pathways involving abnormal muscle development, deposition, or maintenance. The data herein suggests that WB phenotype could potentially be mediated through genes which ultimately result in the up- or down- regulation of pathways that are largely involved with metabolic regulation and basic cellular maintenance, such as Wnt and mTOR, respectively. In mammals, dysregulation in either of these canonical pathways has been shown to result in similar characteristics identified in WB and further investigation of these pathways in chickens exhibiting WB is warranted.
Data Availability Statement
The datasets generated and analyzed for this study can be found in the NCBI-Gene Expression Omnibus database under Accession Series GSE144000: https://www.ncbi.nlm.nih.gov/geo/query/acc.cgi?acc=GSE144000.
Ethics Statement
The animal study was reviewed and approved by the NC State Institutional Animal Care and Use Committee, 15-061-A, approved on 07/06/2015.
Author Contributions
ML, KL, and CA conceived and designed the experiment. ML and KL performed the animal experiment. CA managed the RNA sequencing data collection and analysis. CP and BR analyzed the data. CP drafted the manuscript and it was revised and reviewed by BR, ML, KL, and CA.
Funding
This project was supported by a grant from United States Poultry and Egg Association (2016-0126).
Conflict of Interest
The authors declare that the research was conducted in the absence of any commercial or financial relationships that could be construed as a potential conflict of interest.
Acknowledgments
The authors would like to thank those who contributed to this project including Shelly Nolin, Allysa Stern, and Coltin Caraway for their support and guidance.
Footnotes
- ^ http://resources.qiagenbioinformatics.com/manuals/clcgenomicsworkbench/current/User_Manual.pdf
- ^ http://www.ingenuity.com
References
Abasht, B., Zhou, N., Lee, W. R., Zhuo, Z., and Peripolli, E. (2019). The metabolic characteristics of susceptibility to wooden breast disease in chickens with high feed efficiency. Poult. Sci. 98, 3246–3256. doi: 10.3382/ps/pez183
Barton-Davis, E. R., Shoturma, D. I., Musaro, A., Rosenthal, N., and Sweeney, H. L. (1998). Viral mediated expression of insulin-like growth factor I blocks the aging-related loss of skeletal muscle function. Proc. Natl. Acad. Sci. U.S.A. 95, 15603–15607. doi: 10.1073/pnas.95.26.15603
Bentzinger, C. F., Maltzahn, J., von Dumont, N. A., Stark, D. A., Wang, Y. X., Nhan, K., et al. (2014). Wnt7a stimulates myogenic stem cell motility and engraftment resulting in improved muscle strength. J. Cell Biol. 205, 97–111. doi: 10.1083/JCB.201310035
Brack, A. S., Conboy, M. J., Roy, S., Lee, M., Kuo, C. J., Keller, C., et al. (2007). Increased wnt signaling during aging alters muscle stem cell fate. Science 129:2865. doi: 10.1126/science.1144090
Brothers, B., Zhuo, Z., Papah, M. B., and Abasht, B. (2019). RNA-Seq analysis reveals spatial and sex differences in pectoralis major muscle of broiler chickens contributing to difference in susceptibility to wooden breast disease. Front. Physiol. 10:764. doi: 10.3389/fphys.2019.00764
Cai, D., Dhe-Paganon, S., Melendez, P. A., Lee, J., and Shoelson, S. E. (2003). Two new substrates in insulin signaling, IRS5/DOK4 and IRS6/DOK5. J. Biol. Chem. 278, 25323–25330. doi: 10.1074/jbc.M212430200
Carlson, M. E., Conboy, M. J., Hsu, M., Barchas, L., Jeong, J., Agrawal, A., et al. (2009). Relative roles of TGF-β1 and Wnt in the systemic regulation and aging of satellite cell responses. Aging Cell 8, 676–689. doi: 10.1111/j.1474-9726.2009.00517.x
Carter, E. J., Cosgrove, R. A., Gonzalez, I., Eisemann, J. H., Lovett, F. A., Cobb, L. J., et al. (2009). MEK5 and ERK5 are mediators of the pro-myogenic actions of IGF-2. J. Cell Sci. 122, 3104–3112. doi: 10.1242/jcs.045757
Casolari, J. M., Brown, C. R., Komili, S., West, J., Hieronymus, H., and Silver, P. A. (2004). Genome-wide localization of the nuclear transport machinery couples transcriptional status and nuclear organization. Cell 117, 427–439. doi: 10.1016/S0092-8674(04)00448-9
Cisternas, P., Henriquez, J. P., Brandan, E., and Inestrosa, N. C. (2014). Wnt signaling in skeletal muscle dynamics: myogenesis, neuromuscular synapse and fibrosis. Mol. Neurobiol. 49, 574–589. doi: 10.1007/s12035-013-8540-5
Collins, C. A., and Partridge, T. A. (2005). Self-renewal of the adult skeletal muscle satellite cell. Cell Cycle 4, 1338–1341. doi: 10.4161/cc.4.10.2114
Colmenares, C., and Stavnezer, E. (1989). The ski oncogene induces muscle differentiation in quail embryo cells. Cell 59, 293–303. doi: 10.1016/0092-8674(89)90291-2
D’Angelo, M. A. (2018). Nuclear pore complexes as hubs for gene regulation. Nucleus 9, 142–148. doi: 10.1080/19491034.2017.1395542
D’Angelo, M. A., Gomez-Cavazos, J. S., Mei, A., Lackner, D. H., and Hetzer, M. W. (2012). A change in nuclear pore complex composition regulates cell differentiation. Dev. Cell 22, 446–458. doi: 10.1016/J.DEVCEL.2011.11.021
Daughtry, M. R., Berio, E., Shen, Z., Suess, E. J. R., Shah, N., Geiger, A. E., et al. (2017). Satellite cell-mediated breast muscle regeneration decreases with broiler size. Poult. Sci. 96, 3457–3464. doi: 10.3382/ps/pex068
Davies, B., and Fried, M. (1995). The L19 ribosomal protein gene (RPL19): gene organization, chromosomal mapping, and novel promoter region. Genomics 25, 372–380. doi: 10.1016/0888-7543(95)80036-L
Denbow, D. M. (2000). “Gastrointestinal anatomy and physiology,” in Sturkie’s Avian Physiology, 5th Edn, ed. E. Whittow, (New York, NY: Academic Press).
Dooley, S., Hamzavi, J., Ciuclan, L., Godoy, P., Ilkavets, I., Ehnert, S., et al. (2008). Hepatocyte-specific Smad7 expression attenuates TGF-β–mediated fibrogenesis and protects against liver damage. Gastroenterology 135, 642–659. doi: 10.1053/j.gastro.2008.04.038
Eibe, F., Hall, M. A., and Witten, I. H. (2016). The WEKA Workbench. Online Appendix for Data Mining: Practical Machine Learning Tools and Techniques. San Francisco, CA: Morgan Kaufmann Publishers Inc.
Engle, K. M., Mei, T.-S., Wasa, M., and Yu, J.-Q. (2008). NIH public access. Acc. Chem. Res. 45, 788–802. doi: 10.1038/jid.2014.371
Galy, V., Olivo-Marin, J.-C., Scherthan, H., Doye, V., Rascalou, N., and Nehrbass, U. (2000). Nuclear pore complexes in the organization of silent telomeric chromatin. Nature 403, 108–112. doi: 10.1038/47528
Grimm, J., Sachs, M., Britsch, S., Di Cesare, S., Schwarz-Romond, T., Alitalo, K., et al. (2001). Novel p62dok family members, dok-4 and dok-5, are substrates of the c-Ret receptor tyrosine kinase and mediate neuronal differentiation. J. Cell Biol. 154, 345–354. doi: 10.1083/jcb.200102032
Heydemann, A., Ceco, E., Lim, J. E., Hadhazy, M., Ryder, P., Moran, J. L., et al. (2009). Latent TGF-beta-binding protein 4 modifies muscular dystrophy in mice. J. Clin. Invest. 119, 3703–3712. doi: 10.1172/JCI39845
Hu, J., Wang, F., Yuan, Y., Zhu, X., Wang, Y., Zhang, Y., et al. (2010). Novel importin-α family member Kpna7 is required for normal fertility and fecundity in the mouse. J. Biol. Chem. 285, 33113–33122. doi: 10.1074/jbc.M110.117044
Iwai, A., Hijikata, M., Hishiki, T., Isono, O., Chiba, T., and Shimotohno, K. (2008). Coiled-coil domain containing 85B suppresses the β-catenin activity in a p53-dependent manner. Oncogene 27, 1520–1526. doi: 10.1038/sj.onc.1210801
Jacinto, E., Loewith, R., Schmidt, A., Lin, S., Rüegg, M. A., Hall, A., et al. (2004). Mammalian TOR complex 2 controls the actin cytoskeleton and is rapamycin insensitive. Nat. Cell Biol. 6, 1122–1128. doi: 10.1038/ncb1183
Jang, T. H., Kim, S. H., Jeong, J. H., Kim, S., Kim, Y. G., and Park, H. H. (2015). Crystal structure of caspase recruiting domain (CARD) of apoptosis repressor with CARD (ARC) and its implication in inhibition of apoptosis. Sci. Rep. 5:9847. doi: 10.1038/srep09847
Keerthi, S. S., Shevade, S. K., Bhattacharyya, C., and Murthy, K. R. K. (2001). “Improvements to platt’s SMO algorithm for SVM classifier design,” in Proceedings of the Neural Computation (Germany: MITP), 637–649.
Kelley, J. B., Talley, A. M., Spencer, A., Gioeli, D., and Paschal, B. M. (2010). Karyopherin alpha7 (KPNA7), a divergent member of the importin alpha family of nuclear import receptors. BMC Cell Biol. 11:63. doi: 10.1186/1471-2121-11-63
Kobayashi, N., Goto, K., Horiguchi, K., Nagata, M., Kawata, M., Miyazawa, K., et al. (2007). c-Ski activates MyoD in the nucleus of myoblastic cells through suppression of histone deacetylases. Genes Cell 12, 375–385. doi: 10.1111/j.1365-2443.2007.01052.x
Kuttappan, V. A., Hargis, B. M., and Owens, C. M. (2016). White striping and woody breast myopathies in the modern poultry industry: a review. Poult. Sci. 95, 2724–2733. doi: 10.3382/ps/pew216
Kuttappan, V. A., Owens, C. M., Coon, C., Hargis, B. M., and Vazquez-A Non, M. (2017). Research Note Incidence of broiler breast myopathies at 2 different ages and its impact on selected raw meat quality parameters. Poult. Sci. 96, 3005–3009. doi: 10.3382/ps/pex072
Lamberti, C., Lin, K.-M., Yamamoto, Y., Verma, U., Verma, I. M., Byers, S., et al. (2001). Regulation of-catenin function by the IB Kinases∗. J. Biol. Chem. 276, 42276–42286. doi: 10.1074/jbc.M104227200
Langley, B., Thomas, M., Bishop, A., Sharma, M., Gilmour, S., and Kambadur, R. (2002). Myostatin inhibits myoblast differentiation by down-regulating MyoD expression. J. Biol. Chem. 277, 49831–49840. doi: 10.1074/JBC.M204291200
Laplante, M., and Sabatini, D. M. (2009). mTOR signaling at a glance. J. Cell Sci. 122, 3589–3594. doi: 10.1242/jcs.051011
Laumonier, T., and Menetrey, J. (2016). Muscle injuries and strategies for improving their repair. J. Exp. Orthop. 3:15. doi: 10.1186/s40634-016-0051-7
Lee, D.-F., Kuo, H.-P., Chen, C.-T., Hsu, J.-M., Chou, C.-K., Wei, Y., et al. (2007). IKKβ suppression of TSC1 links inflammation and tumor angiogenesis via the mTOR pathway. Cell 130, 440–455. doi: 10.1016/J.CELL.2007.05.058
Li, Y., Turck, C. M., Teumer, J. K., and Stavnezert, E. (1986). Unique sequence, ski, in sloan-kettering avian retroviruses with properties of a new cell-derived oncogene. J. Virol. 57, 1065–1072. doi: 10.1128/jvi.57.3.1065-1072.1986
Li, Y.-P., Chen, Y., John, J., Moylan, J., Jin, B., Mann, D. L., et al. (2005). TNF-α acts via p38 MAPK to stimulate expression of the ubiquitin ligase atrogin1/MAFbx in skeletal muscle. FASEB J. 19, 362–370. doi: 10.1096/fj.04-2364com
Liu, S., Brown, J. D., Stanya, K. J., Homan, E., Leidl, M., Inouye, K., et al. (2013). A diurnal serum lipid integrates hepatic lipogenesis and peripheral fatty acid use. Nature 502, 550–554. doi: 10.1038/nature12710
Livingston, M. L., Ferket, P. R., Brake, J., and Livingston, K. A. (2018a). Dietary amino acids under hypoxic conditions exacerbates muscle myopathies including wooden breast and white stripping. Poult. Sci. 98, 1517–1527. doi: 10.3382/ps/pey463
Livingston, M. L., Landon, C., Barnes, H. J., and Brake, J. (2018b). White striping and wooden breast myopathies of broiler breast muscle is affected by time-limited feeding, genetic background, and egg storage. Poult. Sci. 98, 217–226. doi: 10.3382/ps/pey333
Malila, Y., Thanatsang, K., Arayamethakorn, S., Uengwetwanit, T., Srimarut, Y., Petracci, M., et al. (2019). Absolute expressions of hypoxia-inducible factor-1 alpha (HIF1A) transcript and the associated genes in chicken skeletal muscle with white striping and wooden breast myopathies. PLoS One 14:e0220904. doi: 10.1371/journal.pone.0220904
Mayer, C., Zhao, J., Yuan, X., and Grummt, I. (2004). mTOR-dependent activation of the transcription factor TIF-IA links rRNA synthesis to nutrient availability. Genes Dev. 18, 423–434. doi: 10.1101/gad.285504
Mudalal, S., Babini, E., Cavani, C., and Petracci, M. (2014). Quantity and functionality of protein fractions in chicken breast fillets affected by white striping. Poult. Sci. 93, 2108–2116. doi: 10.3382/ps.2014-03911
Mutryn, M. F., Brannick, E. M., Fu, W., Lee, W. R., and Abasht, B. (2015). Characterization of a novel chicken muscle disorder through differential gene expression and pathway analysis using RNA-sequencing. BMC Genom. 16:399. doi: 10.1186/s12864-015-1623-0
Nguyen, T. T., Almon, R. R., Dubois, D. C., Sukumaran, S., Jusko, W. J., and Androulakis, I. P. (2014). Tissue-specific gene expression and regulation in liver and muscle following chronic corticosteroid administration. Gene Regul. Syst. Biol. 8, 75–87. doi: 10.4137/GRSB.S13134
Papah, M. B., Brannick, E. M., Schmidt, C. J., and Abasht, B. (2017). Evidence and role of phlebitis and lipid infiltration in the onset and pathogenesis of wooden breast disease in modern broiler chickens. Avian Pathol. 46, 623–643. doi: 10.1080/03079457.2017.1339346
Papah, M. B., Brannick, E. M., Schmidt, C. J., and Abasht, B. (2018). Gene expression profiling of the early pathogenesis of wooden breast disease in commercial broiler chickens using RNA-sequencing. PLoS One 13:e0207346. doi: 10.1371/journal.pone.0207346
Petracci, M., Mudalal, S., Soglia, F., and Cavani, C. (2015). Meat quality in fast-growing broiler chickens. Worlds Poult. Sci. J. 71, 363–374. doi: 10.1017/S0043933915000367
Petracci, M., Soglia, F., Madruga, M., Carvalho, L., Ida, E., and Estévez, M. (2019). Wooden-breast, white striping, and spaghetti meat: causes, consequences and consumer perception of emerging broiler meat abnormalities. Compr. Rev. Food Sci. Food Saf. 18, 565–583. doi: 10.1111/1541-4337.12431
Raices, M., Bukata, L., Sakuma, S., Borlido, J., Hernandez, L. S., Hart, D. O., et al. (2017). Nuclear pores regulate muscle development and maintenance by assembling a localized Mef2C complex. Dev. Cell 41, 540–554. doi: 10.1016/j.devcel.2017.05.007
Rios, K. E., Liem, A., Kashyap, A., Maynard, S., Paul, S., and Schaefer, B. C. (2018). CARD19: a mitochondrial caspase recruitment domain protein with a role in regulating pro-inflammatory innate immune responses. J. Immunol. 200:169. doi: 10.1007/3-540-30683-8_249
Rosenthal, S. M., Brunetti, A., Brown, E. J., Mamula, P. W., and Goldfine, I. D. (1991). Regulation of insulin-like growth factor (IGF) I receptor expression during muscle cell differentiation. Potential autocrine role of IGF-II. J. Clin. Invest. 87, 1212–1219. doi: 10.1172/JCI115121
Ryall, J. G., Schertzer, J. D., and Lynch, G. S. (2008). Cellular and molecular mechanisms underlying age-related skeletal muscle wasting and weakness. Biogerontology 9, 213–228. doi: 10.1007/s10522-008-9131-0
Sacco, A., Mourkioti, F., Tran, R., Choi, J., Llewellyn, M., Kraft, P., et al. (2010). Short telomeres and stem cell exhaustion model duchenne muscular dystrophy in mdx/mTR mice. Cell 143, 1059–1071. doi: 10.1016/j.cell.2010.11.039
Sihvo, H. K., Immonen, K., and Puolanne, E. (2014). Myodegeneration with fibrosis and regeneration in the pectoralis major muscle of broilers. Vet. Pathol. 51, 619–623. doi: 10.1177/0300985813497488
Staal, F. J. T. (2016). Wnt signalling meets epigenetics. Stem Cell Invest. 3, 38–38. doi: 10.21037/sci.2016.08.01
Trocino, A., Piccirillo, A., Birolo, M., Radaelli, G., Bertotto, D., Filiou, E., et al. (2015). Effect of genotype, gender and feed restriction on growth, meat quality and the occurrence of white striping and wooden breast in broiler chickens. Poult. Sci. 94, 2996–3004. doi: 10.3382/ps/pev296
Velleman, S. G., and Clark, D. L. (2015). Histopathologic and myogenic gene expression changes associated with wooden breast in broiler breast muscles. Avian Dis. 59, 410–418. doi: 10.1637/11097-042015-Reg.1
Vuorinen, E. M., Rajala, N. K., Ihalainen, T. O., and Kallioniemi, A. (2018). Depletion of nuclear import protein karyopherin alpha 7 (KPNA7) induces mitotic defects and deformation of nuclei in cancer cells. BMC Cancer 18:325. doi: 10.1186/s12885-018-4261-5
Wynn, T. (2008). Cellular and molecular mechanisms of fibrosis. J. Pathol. 214, 199–210. doi: 10.1002/path.2277
Yasuoka, H., Yamaguchi, Y., and Feghali-Bostwick, C. A. (2014). The membrane-associated adaptor protein DOK5 is upregulated in systemic sclerosis and associated with IGFBP-5-induced fibrosis. PLoS One 9:e87754. doi: 10.1371/journal.pone.0087754
Yokoyama, S., and Asahara, H. (2011). The myogenic transcriptional network. Cell. Mol. Life Sci. 68, 1843–1849. doi: 10.1007/s00018-011-0629-2
Zaefarian, F., Abdollahi, M. R., Cowieson, A., and Ravindran, V. (2019). Avian liver: the forgotten organ. Animal 9:E63. doi: 10.3390/ANI9020063
Zambonelli, P., Zappaterra, M., Soglia, F., Petracci, M., Sirri, F., Cavani, C., et al. (2014). Genetics and genomics detection of differentially expressed genes in broiler pectoralis major muscle affected by white striping-wooden breast myopathies. Poult. Sci. 95, 2771–2785. doi: 10.3382/ps/pew268
Zambonelli, P., Zappaterra, M., Soglia, F., Petracci, M., Sirri, F., Cavani, C., et al. (2016). Detection of differentially expressed genes in broiler pectoralis major muscle affected by white striping – wooden breast myopathies. Poult. Sci. 95, 2771–2785. doi: 10.3382/ps/pew268
Zimowska, M., Duchesnay, A., Dragun, P., Oberbek, A., Moraczewski, J., and Martelly, I. (2009). Immunoneutralization of TGF β1 improves skeletal muscle regeneration: effects on myoblast differentiation and glycosaminoglycan content. Int. J. Cell Biol. 2009, 1–16. doi: 10.1155/2009/659372
Keywords: wooden breast, machine learning, poultry transcriptomics, support vector machines, artificial neural networks, transforming growth factor
Citation: Phillips CA, Reading BJ, Livingston M, Livingston K and Ashwell CM (2020) Evaluation via Supervised Machine Learning of the Broiler Pectoralis Major and Liver Transcriptome in Association With the Muscle Myopathy Wooden Breast. Front. Physiol. 11:101. doi: 10.3389/fphys.2020.00101
Received: 04 September 2019; Accepted: 27 January 2020;
Published: 25 February 2020.
Edited by:
Sandra G. Velleman, The Ohio State University, United StatesReviewed by:
Casey M. Owens, University of Arkansas, United StatesMassimiliano Petracci, University of Bologna, Italy
Sami Dridi, University of Arkansas, United States
Copyright © 2020 Phillips, Reading, Livingston, Livingston and Ashwell. This is an open-access article distributed under the terms of the Creative Commons Attribution License (CC BY). The use, distribution or reproduction in other forums is permitted, provided the original author(s) and the copyright owner(s) are credited and that the original publication in this journal is cited, in accordance with accepted academic practice. No use, distribution or reproduction is permitted which does not comply with these terms.
*Correspondence: Chris M. Ashwell, Y21hc2h3ZWxAbmNzdS5lZHU=