- 1Department of Psychiatry and Psychotherapy, Medical University of Vienna, Vienna, Austria
- 2Division of Nuclear Medicine, Department of Biomedical Imaging and Image-Guided Therapy, Medical University of Vienna, Vienna, Austria
- 3QIMP Group, Center for Medical Physics and Biomedical Engineering, Medical University of Vienna, Vienna, Austria
- 4CBmed, Graz, Austria
- 5Ludwig Boltzmann Institute Applied Diagnostics, Vienna, Austria
- 6Division of Neuroradiology and Musculoskeletal Radiology, Department of Biomedical Imaging and Image-Guided Therapy, Medical University of Vienna, Vienna, Austria
Background: Several MR-based attenuation correction (AC) approaches were developed to conquer the challenging AC in hybrid PET/MR imaging. These AC methods are commonly evaluated on standardized uptake values or tissue concentration. However, in neurotransmitter system studies absolute quantification is more favorable due to its accuracy. Therefore, our aim was to investigate the accuracy of segmentation- and atlas-based MR AC approaches on serotonin transporter (SERT) distribution volumes and occupancy after a drug challenge.
Methods: 18 healthy subjects (7 male) underwent two [11C]DASB PET/MRI measurements in a double-blinded, placebo controlled, cross-over design. After 70 min the selective serotonin reuptake inhibitor (SSRI) citalopram or a placebo was infused. The parameters total and specific volume of distribution (VT, VS = BPP) and occupancy were quantified. All subjects underwent a low-dose CT scan as reference AC method. Besides the standard AC approaches DIXON and UTE, a T1-weighted structural image was recorded to estimate a pseudo-CT based on an MR/CT database (pseudoCT). Another evaluated AC approach superimposed a bone model on AC DIXON. Lastly, an approach optimizing the segmentation of UTE images was analyzed (RESOLUTE). PET emission data were reconstructed with all 6 AC methods. The accuracy of the AC approaches was evaluated on a region of interest-basis for the parameters VT, BPP, and occupancy with respect to the results of AC CT.
Results: Variations for VT and BPP were found with all AC methods with bias ranging from −15 to 17%. The smallest relative errors for all regions were found with AC pseudoCT (<|5%|). Although the bias between BPP SSRI and BPP placebo varied markedly with AC DIXON (<|12%|) and AC UTE (<|9%|), a high correlation to AC CT was obtained (r2∼1). The relative difference of the occupancy for all tested AC methods was small for SERT high binding regions (<|4%|).
Conclusion: The high correlation might offer a rescaling from the biased parameters VT and BPP to the true values. Overall, the pseudoCT approach yielded smallest errors and the best agreement with AC CT. For SERT occupancy, all AC methods showed little bias in high binding regions, indicating that errors may cancel out in longitudinal assessments.
Introduction
The introduction of combined imaging systems, such as positron emission tomography/computed tomography (PET/CT) proposed a number of benefits, especially for clinical routine, due to aligned structural and molecular information (Townsend et al., 2004). Furthermore, the development of combined positron emission tomography/magnetic resonance imaging (PET/MRI) systems enabled the simultaneous acquisition of functional and molecular information. This option decreases the intrasubject variability between separate measurements (e.g., caused by habituation effects, differences in motivation and attention or fluctuating intrinsic activity) (Hahn et al., 2017). This is especially of importance when functional changes should be compared to molecular changes within the same setting, e.g., after drug challenge (Sander et al., 2013).
However, PET/MRI brought along a major challenge; photons are attenuated to varying extent by different tissue types traversed on the way to the detectors. Ignoring this photon attenuation causes an erroneous reconstruction of the activity distribution (Huang et al., 1979). Hence, it is crucial that PET data is corrected for attenuation. On stand-alone PET systems, attenuation correction (AC) is performed for example with retractable radioactive rod sources of 68Ga/68Ge, rotating around the patient and generating an AC map (Bailey, 1998). In PET/CT systems, a CT is acquired and scaled from Hounsfield units (HU) to linear attenuation coefficients at 511 keV (Carney et al., 2006). The difficulty with AC in PET/MRI systems is that neither rod sources nor a CT are currently installed due to technical challenges, such as the magnetic field of the MRI (Catana et al., 2013). Another issue is that bone is insufficiently depicted with MRI compared to CT. As gold-standard a separate CT scan would be acquired, further processed and applied for AC on PET/MRI data (Andersen et al., 2014). However, this procedure exposes subjects to additional ionizing radiation and is not practicable for clinical routine. Therefore, several MR-based AC methods have been proposed of which the following are currently implemented as commercial solutions in clinical systems: Siemens Healthineers AG provides solutions such as segmentation of fat and water images (DIXON) (Martinez-Möller et al., 2009) or ultra-short echo time images (UTE) (Catana et al., 2010). General Electric provides a model based approach where a bone model is superimposed on the AC map, derived from segmentation of fat and water images (Sekine et al., 2016). In addition to these commercial approaches, several MR-AC methods have been proposed by the scientific community, such as deep learning algorithms and zero-echo-time sequences (Delso et al., 2015; Gong et al., 2018) or emission-based attenuation correction (Berker and Li, 2016). However, new MR-based AC approaches are commonly based on segmentation or a template/atlas (Ladefoged et al., 2017).
To evaluate the performance of different AC methods, the activity in tissue or the semi-quantitative measure standardized uptake value (SUV) are often used (Schulz et al., 2011; Berker et al., 2012; Burgos et al., 2014; Izquierdo-Garcia et al., 2014; Rausch et al., 2016; Ladefoged et al., 2017). However, in studies with radioligands targeting neurotransmitter proteins, quantification of distribution volumes and binding potentials (BP) are of major interest. This is usually achieved with kinetic modeling and arterial blood and/or a reference region. Ideally, only non-specifically bound radiotracer is found in a reference region (Nørgaard et al., 2019). In this study the radioligand [11C]DASB was administered to quantify the serotonin transporter (SERT) protein density in the brain. Kinetic modeling can be performed for example with the simplified reference tissue model (SRTM), a 1 or constrained 2 tissue compartment model, or a graphical analysis (Logan plot) (Ginovart et al., 2001; Nørgaard et al., 2019). An adapted radioligand administration protocol was used in this study, enabling the estimation of the BP with a ratio method (Gryglewski et al., 2017).
Furthermore, drug occupancy is frequently studied for example to elucidate the mechanisms of action of a drug or to determine the appropriate dose, potentially translating to clinical applications (Takano et al., 2016). Thus, longitudinal assessments at baseline and after application of a drug are necessary.
Our main goal was to evaluate the performance of segmentation and atlas-based AC methods in the neurotransmitter system by absolutely quantifying distribution volumes. We hypothesize that differences between the AC approaches of total volume of distribution will be comparable with the results of the literature where SUV and tissue concentration is used, for mathematical reasons (see section “Materials and Methods”). Additionally, we aimed to demonstrate the impact of a bias in the reference region on the specific volume of distribution. Finally, we evaluated the difference between the AC methods when measurements of a longitudinal study are compared.
Materials and Methods
Subjects
A total of 18 healthy subjects from a larger ongoing trial were included in this study (7 male, mean age 28.0 ± 9.6 years). To assure the healthy status, all subjects underwent medical examination including blood tests, electrocardiography, neurological and physiological tests as well as a urine drug test at the screening visit. Additionally, all subjects were screened for psychiatric disorders by an experienced psychiatrist with the Structural Clinical Interview according to DSM-IV criteria. A urine pregnancy test was acquired for all female subjects prior to the scans.
Positron Emission Tomography
All subjects underwent two measurements (mean interval: 36.0 ± 29.6 days) on a PET/MRI system (Siemens Biograph mMR) in a double-blind, placebo-controlled, cross-over design. The radioligand [11C]DASB, which specifically binds to the SERT (Wilson et al., 2013), was synthesized as described previously (Haeusler et al., 2009). Radioligand application started outside the scanner, using a syringe pump. The radioligand was infused as bolus for 1 min followed by constant infusion via a cubital vein for a total duration of 180 min. The target dose was calculated as 20 MBq/kg (effective dose for a 75 kg person: 5.55 mSv). PET data was recorded for 120–125 min in list-mode starting 30–45 min post injection. At 70 min post injection the placebo (saline) or 8 mg of the selective serotonin reuptake inhibitor (SSRI) citalopram was infused over 8 min.
Magnetic Resonance Imaging
Simultaneously with the PET acquisition the standard MR-AC sequences DIXON volume-interpolated breath-hold examination (VIBE, TE1/TE2/TR = 1.23/2.46/3.60 ms, flip angle = 10°, voxel size 2.6 × 2.6 × 3.1 mm3) and ultra-short echo time (UTE, TE1/TE2/TR = 0.07/2.46/11.94 ms, flip angle = 10°, voxel size 1.6 × 1.6 × 1.6 mm3) were recorded. A magnetization prepared rapid gradient echo sequence (MP-RAGE) was acquired for a T1-weighted anatomical image (TE/TR = 4.21/3000 ms, voxel size 1 × 1 × 1.1 mm3) for an atlas-based AC approach.
Attenuation Correction Approaches
Positron emission tomography data was reconstructed with the following AC methods in order to assess their influence on SERT binding based on their availability.
CT
According to the EANM guidelines version 2 (Varrone et al., 2009) a regular- or low-dose CT can be used for attenuation correction. All subjects underwent a low-dose CT scan using a PET/CT (Siemens Biograph TruePoint PET/CT, tube potential: 120 kVp, voxel size: 0.59 × 0.59 × 1.5 mm3, effective dose: 1 mSv) to keep the radiation exposure as low as possible. For the applicability of the CT scan as AC method, it was preprocessed as described previously (Carney et al., 2006; Ladefoged et al., 2015). In short, the CT scan was co-registered to the structural MRI. Thereafter, it was segmented into bone and non-bone tissues and a bilinear scaling was applied to estimate the linear attenuation coefficients at 511 keV (Kinahan et al., 1998). AC CT was used as reference AC method.
DIXON
This approach is based on segmentation of fat and water images originating from in- and opposed-phase images (Dixon, 1984). The final AC map contains the classes soft tissue, fatty tissue, air and if applicable lung tissue (Martinez-Möller et al., 2009).
UTE
Two MR images with different ultra-short echo times are segmented into 3 distinctive tissue classes, namely bone, soft tissue and air (Catana et al., 2010).
PseudoCT
The pseudoCT was created from an individual structural T1-weighted MRI using an online tool1. The method itself is based on a database where pairs of T1-weighted MRIs and co-registered CT scans are stored. The software analyzes the best fitting MRIs, assigns weights and averages the corresponding CTs accordingly. The resulting pseudoCT was then processed equally to the CT (Burgos et al., 2014).
RESOLUTE
This AC method is based on AC UTE but additionally segments CSF as well as other tissue classes and uses continuous linear attenuation coefficients for a more accurate AC map (Ladefoged et al., 2015, 2017). For creation of the AC maps an online tool provided by the authors of Ladefoged et al. (2015) was used.
Bone Demonstrator
The bone demonstrator (BD) is a non-commercial prototype provided by Siemens Healthineers AG. An MRI, aligned with a bone model, is non-rigidly registered to the individual DIXON MR images. The registered bone model is then superimposed on the AC DIXON with continuous linear attenuation coefficients (Paulus et al., 2015; Koesters et al., 2016).
Figure 1A depicts the final AC maps of one representative subject.
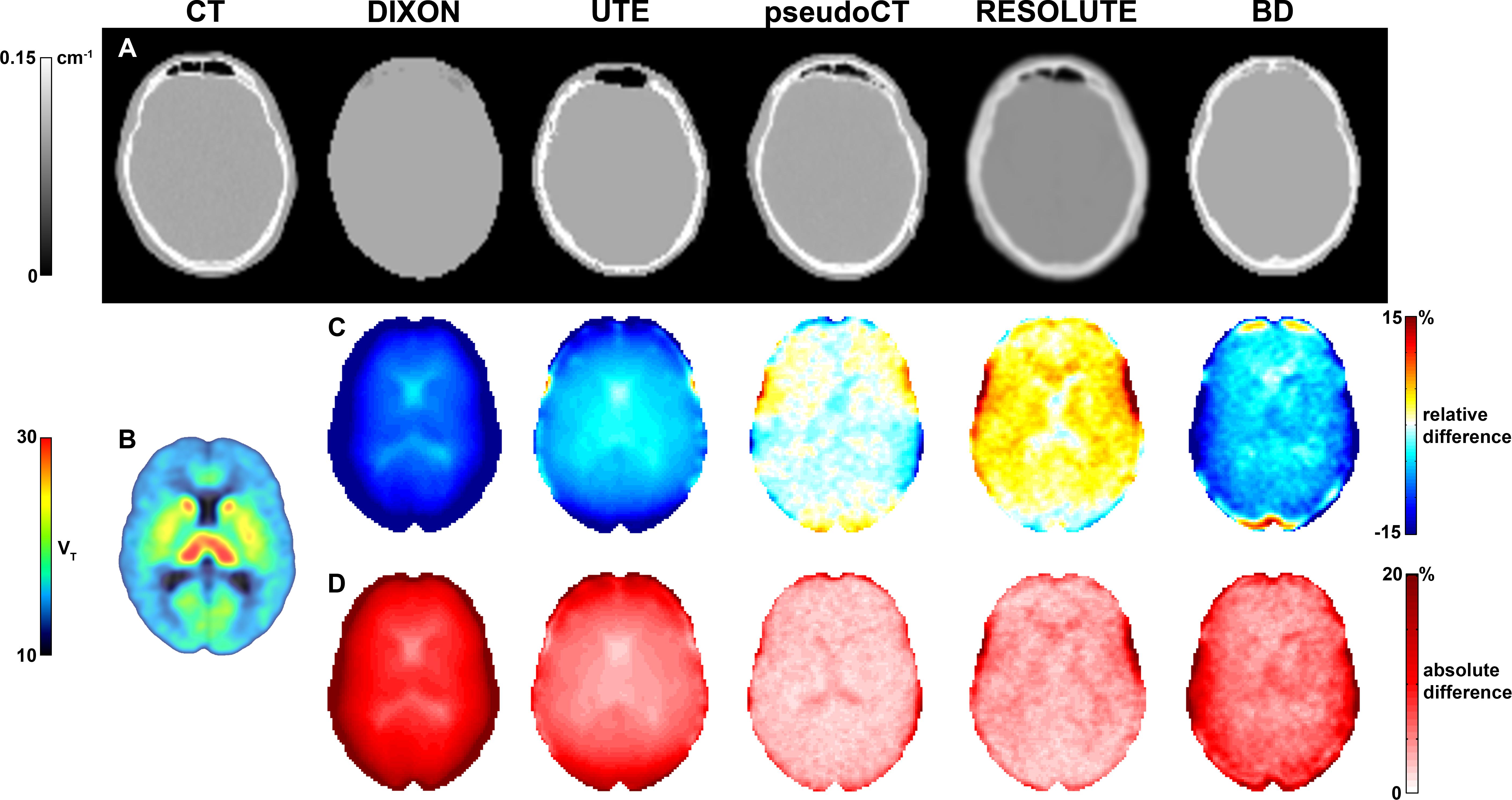
Figure 1. Panel (A) depicts the final AC maps of all described AC approaches from one representative subject, panel (B) shows the group average VT placebo reconstructed with AC CT, panels (C,D) illustrate the voxel-wise group average relative and absolute percentage difference to AC CT-based VT. Smaller differences in the center of the brain compared to areas in the proximity to bone are visible.
PET Data Reconstruction and Processing
Three consecutive frames (each 10 min) in tracer equilibrium, starting from 95 min post PET acquisition start, were reconstructed with an ordinary Poisson-ordered subset expectation maximization algorithm (OP-OSEM, 3 iterations, 21 subsets, voxel size 2.09 × 2.09 × 2.03 mm3). The data was corrected for dead time, randoms, scatter and attenuation, either with CT, DIXON, UTE, pseudoCT, RESOLUTE or BD. Furthermore, the data was decay-corrected to the start of the radioligand administration to be in accordance with the activity of the blood samples. The offline reconstruction tool was provided by Siemens Healthineers AG.
Subsequently, data was processed with Statistical Parametric Mapping, version 12 (SPM12)2 using default algorithms and settings unless indicated otherwise. For motion correction, data was realigned to a mean image (quality = 1) which was co-registered to the T1-weighted structural MR image. Thereafter, the MR image was normalized via tissue probability maps to MNI space. The resulting transformation matrix was then applied to the dynamic PET data. Time-activity values for the 3 mentioned frames were derived from 7 regions-of-interest (ROIs) of the Automated Anatomic Labeling atlas (Tzourio-Mazoyer et al., 2002), namely the temporal lobe, anterior cingulate cortex (ACC), amygdala, caudate, putamen, thalamus and cerebellar gray matter excluding the vermis. The left and corresponding right ROI of each hemisphere were averaged for simplification. The regions were chosen to cover areas across the whole brain with varying amount of SERT binding.
SERT Quantification
The bolus plus constant infusion protocol enabled the use of an equilibrium method to quantify the total volume of distribution (VT), the binding potential in plasma (BPP = VS) and the occupancy of the SSRI on the SERT with the 3 consecutive frames. This method has been shown to provide valid estimates of SERT binding without the need for kinetic modeling (Ginovart et al., 2001; Gryglewski et al., 2017). VT is derived from CT/CP where CT is the radioligand concentration in tissue, averaged across the 3 frames and CP the radioligand concentration in plasma. CP was calculated as the mean product of whole blood activity, the plasma and the parent fraction in arterial blood samples, drawn 120, 130 and 140 min after start of radioligand infusion. The blood time points were approximately corresponding to the start time points of the PET frames. Radioactivity was measured in whole blood and, after centrifugation, in plasma in a γ-counter (Wizard2, 3″, Perkin Elmer). Additionally, the fraction of unchanged [11C]DASB was determined using cartridges (Ginovart et al., 2001).
BPP is calculated as VT − VND where VND describes the VT of a reference region, i.e., the cerebellar gray matter for [11C]DASB (Parsey et al., 2006). Finally, the occupancy was determined as the relative change in BPP between the placebo and the SSRI scan (Innis et al., 2007). All estimated parameters are based on ROIs.
Statistical Evaluation
To assess the difference between AC methods, the relative difference (RD) of VT, BPP, and occupancy was calculated with regard to the reference AC approach CT using ROIs.
Furthermore, the absolute percentage difference (AD) was calculated as
ACX was defined as one of the investigated AC approaches (DIXON, UTE, pseudoCT, RESOLUTE, or BD). Finally, linear regression of VT, BPP, and occupancy was conducted to detect bias (slope, intercept) and to evaluate similarity (r2) of the different AC approaches with respect to AC CT.
Results
The following paragraphs outline key results for all tested AC methods. A complete list of the group mean relative and absolute percentage differences and standard deviations for VT (placebo, SSRI), BPP (placebo, SSRI) and occupancy with all proposed AC methods compared to the reference AC CT can be found in Tables 1, 2. RD and AD were mostly in a similar range indicating that the bias of a ROI of a specific AC is almost exclusively in one direction across the group. This effect is especially noticeable for the parameter VT (see Table 1). The results of the ROI-based regression between the proposed AC methods and AC CT can be found in Table 3. The values of the SSRI and placebo measurement were merged to have one r2, slope and intercept per AC approach and parameter. When analyzed separately the r2 values were rather comparable with VT placebo r2 ranging from 0.996 to 0.998 in comparison to VT SSRI r2 ranging from 0.992 to 0996. Similar results were achieved for BPP placebo (r2 ranging from 0.996 to 0.999) and BPP SSRI (r2 ranging from 0.983 to 0.992). Furthermore, slope and intercept were similar. Figure 2 depicts boxplots with relative difference of VT placebo and occupancy to the reference AC method CT. For illustration purposes Figure 1B shows the voxel-wise AC CT-based VT placebo averaged across all subjects. Figures 1C,D depict the average voxel-wise relative and absolute percentage differences of VT placebo to AC CT.
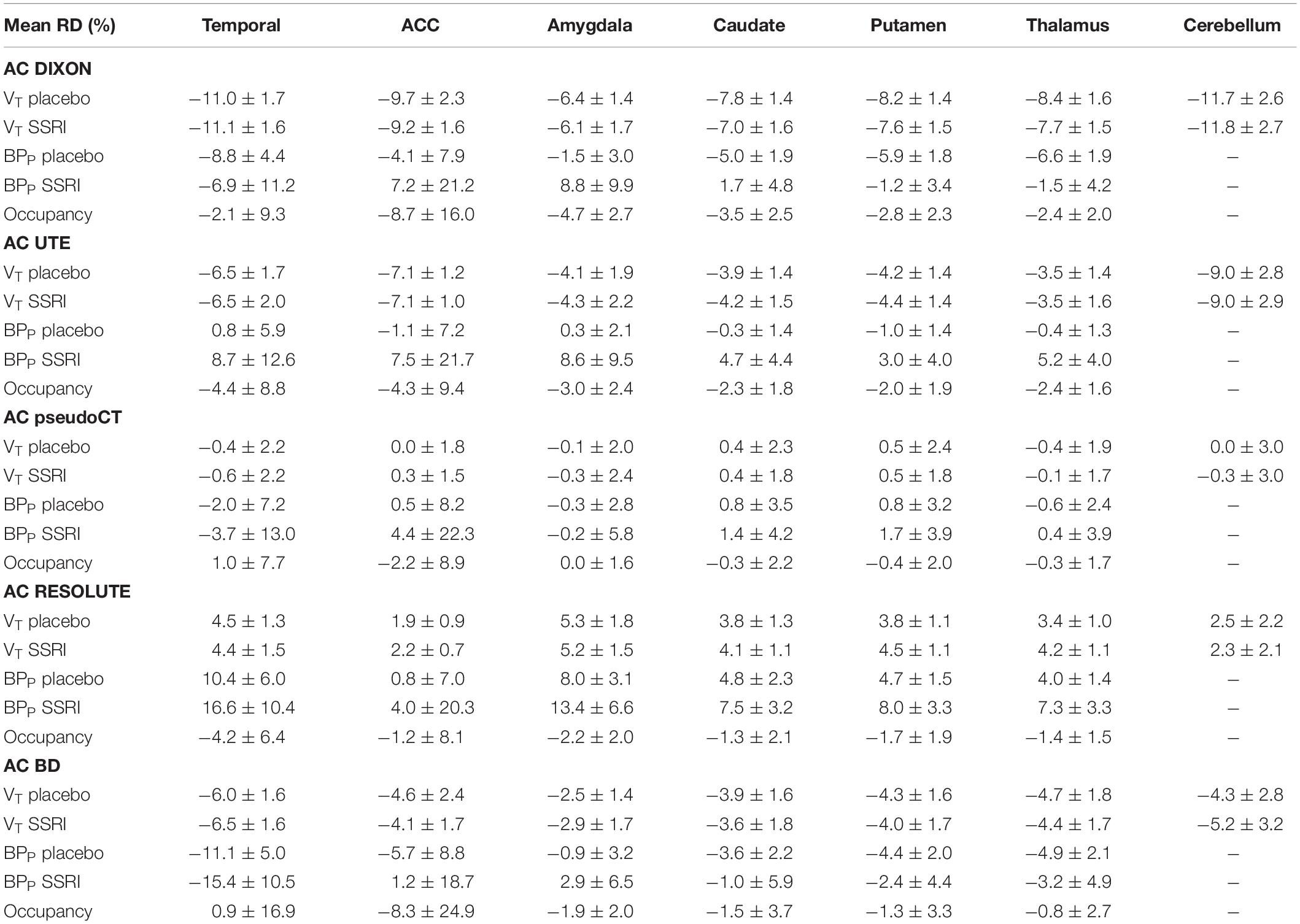
Table 1. Group mean relative difference and standard deviation of VT, BPP, and occupancy estimated with the proposed AC methods with respect to AC CT for every ROI.
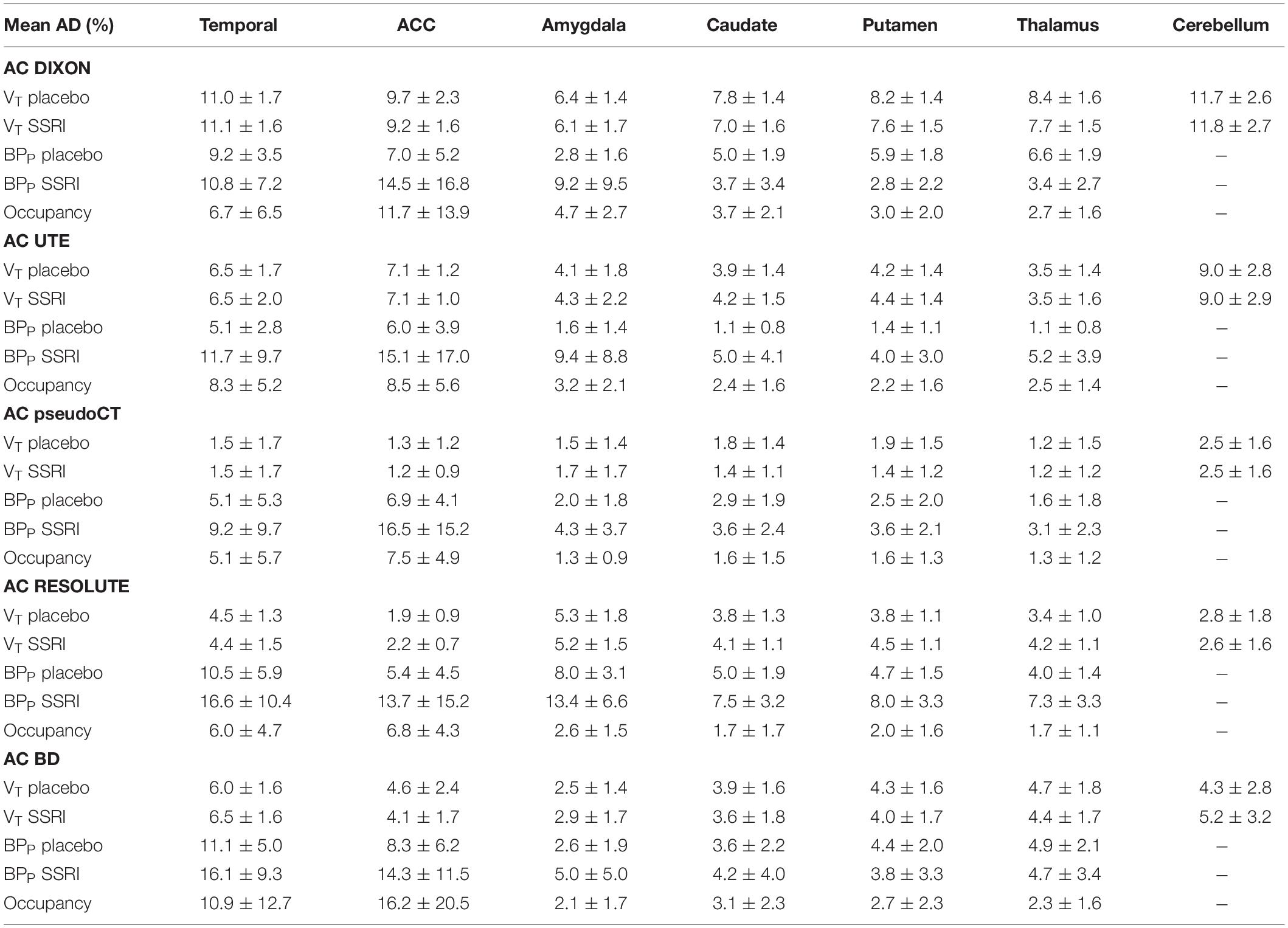
Table 2. Group mean absolute percentage difference and standard deviation of VT, BPP, and occupancy estimated with the proposed AC methods with respect to AC CT for every ROI.
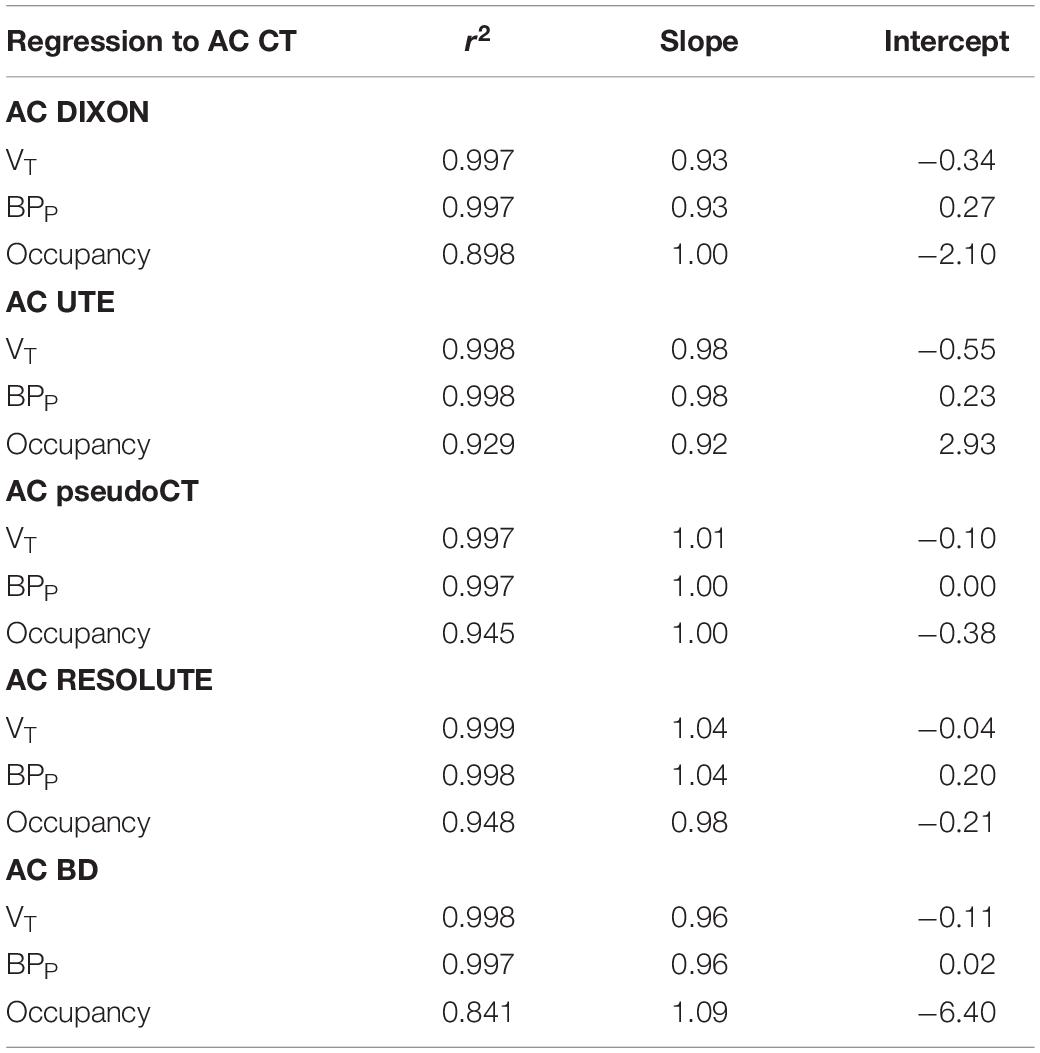
Table 3. Region-of-interest-based linear regression parameters (r2, slope, and intercept) for VT, BPP, and occupancy estimated with the proposed AC methods with respect to AC CT. The values of the placebo and SSRI scan were merged.
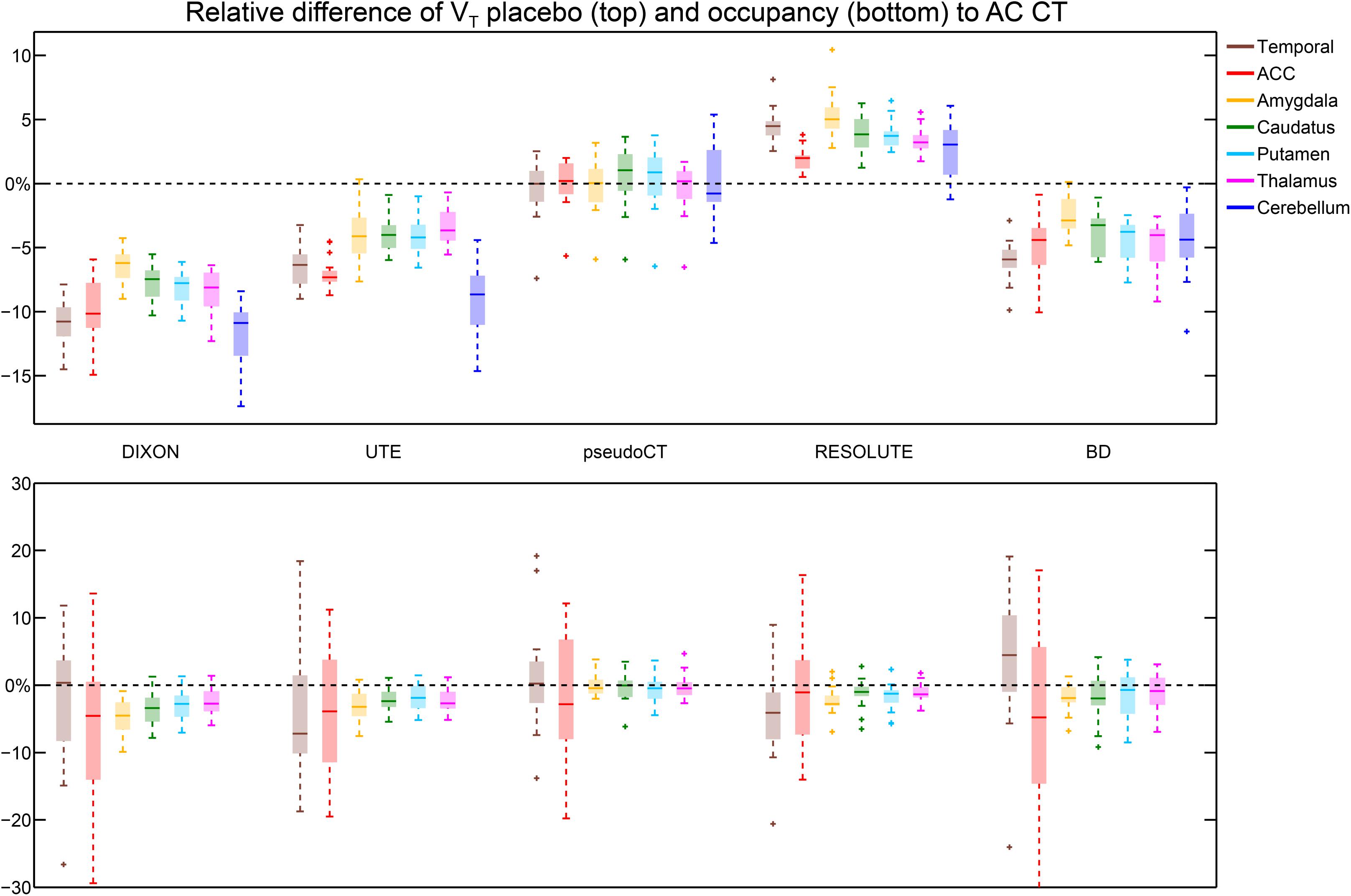
Figure 2. Top, Boxplots depicting the relative difference for each ROI of VT placebo estimated with the proposed AC methods with respect to AC CT. Outliers present for most regions of AC pseudoCT were all from the same subject. Bottom, Boxplots represent the relative difference of each ROI of occupancy compared to AC CT. The figure was limited to –30% for visual purposes. Therefore, the lower whisker of AC BD is just partly visible (actual length: –15 to –34%). Furthermore, three outliers are missing: ACC in AC DIXON (–58%), temporal lobe (–56%), and ACC in AC BD (–91%).
DIXON
VT showed the highest RD in the temporal lobe and the cerebellum with underestimations of around 11% and r2 ∼ 1. The errors of the placebo and SSRI scan were similar for VT. In contrast, for BPP the difference was markedly non-uniform between the ROIs and varied between placebo and SSRI scans. The most pronounced difference was found in the amygdala (placebo: −2%, SSRI: 9%). The bias for occupancy was less than −5%, except for the ACC with an error of approximately −9%. Regression confirmed the underestimation with a slope below 1 for VT and BPP.
UTE
Similar to VT obtained with AC DIXON, the largest RD was found in the cerebellum (−9%). The error of BPP varied between the placebo and SSRI scan. For the placebo scan the RD was as low as −1% whereas the SSRI scan showed an overestimation of 9% in the amygdala. The occupancy varied between −4 and −2% in all investigated regions.
PseudoCT
For VT, the AD across all regions was less than 3%. The RD for BPP was similar for the placebo and the SSRI scans with the exception of the ACC, where the placebo scan overestimated the BPP by 1% and the SSRI scan by 4%. The relative variation of the occupancy was between 0 and 1%. However, the AD revealed an increased bias in all estimated parameters, especially for BPP in low binding regions. Nevertheless, the results of all parameters were similar to the ones obtained with AC CT (r2 ranging from 0.95 to 1). Outliers found in Figure 2, top, were all from the same subject. However, no abnormalities were observed in the pseudoCT AC map, thus, the subject was retained in all analyses.
RESOLUTE
VT and BPP were overestimated in all regions. The largest deviation for VT was 5% in the amygdala whereas the largest RD for BPP was found in the temporal lobe (17%). The overestimations were also observed with regression (slope: 1.04, intercept 0.20). In contrast, the occupancy was underestimated in all regions by 1% (caudate) to 4% (temporal lobe). The slope and intercept of the regression was almost in alignment with the line of identity (slope between 0.98 and 1.04, intercept between −0.21 and 0.20) and had an r2 ranging from 0.95 to 1 for all parameters.
BD
The largest relative errors were found in the low binding region of the temporal lobe (VT SSRI −7%, BPP SSRI −15%). In all other areas, the bias varied between −6 and 1%. The RD of the occupancy was between −2 and 1% with an exception of −8% in the ACC. Again, compared to RD, AD was increased in low binding regions for the parameter BPP as well as occupancy. Regression showed the lowest agreement for occupancy with r2 of 0.84 slope = 1.09) and intercept = −6.40.
Overall, the agreement of VT and BPP calculations when using different AC methods was close, with r2 of approximately 1 for the placebo and the SSRI scans. In almost all regions, the smallest relative error was found for AC pseudoCT without mean relative bias in the cerebellum and ACC for VT placebo. However, AD revealed marked errors in low binding regions which were hidden in the RD alone. The regression of AC RESOLUTE and AC pseudoCT showed a slope and intercept similar to the line of identity. The standard deviation was highest for occupancy with AC BD and lowest with AC RESOLUTE in the temporal lobe and ACC, ranging from ±6 to ±16% and ±8 to ±24%, respectively.
Discussion
In this study, we analyzed the performance of different PET/MRI AC methods, namely, DIXON, UTE, pseudoCT, RESOLUTE and BD with respect to the CT-reference for the quantification of SERT binding and occupancy. In contrast to most of the previous reports we did not use tissue activity or SUV values but focused on different outcome parameters obtained from absolute quantification.
First, we evaluated the performance of the tested AC methods with regards to the parameter VT in comparison to the reference AC CT. When using AC DIXON, VT was underestimated with a regional variability. While subcortical regions close to the center of the brain showed a lower bias, a larger RD was observed in areas close to the skull. This phenomenon and similar RDs were reported by other groups (Izquierdo-Garcia et al., 2014; Rausch et al., 2017) and demonstrated on a voxel-wise basis (Ladefoged et al., 2017). The bias is likely caused by the missing bone information (Andersen et al., 2014).
The lack of bone can be accounted for to some degree by superimposing a bone segment on the AC DIXON map (i.e., the BD method). The improvement approximately halved the errors in all regions. However, since AC BD is based on AC DIXON, errors in the latter are also transferred to BD (e.g., filled air cavities) (Rausch et al., 2017). Similarly, AC UTE offered an improvement to the RD of VT, although still with higher underestimations in the vicinity of the skull. The error arises from segmentation misclassifications in the tissue/air border areas (Aasheim et al., 2015). Discontinuities of the segmented skull and neglect of regional variation in bone density are further issues leading to inaccurate PET quantification (Ladefoged et al., 2015). However, overall, the bias was smaller than with AC DIXON and in some regions comparable to AC BD, presumably due to the additional bone segment. In AC RESOLUTE the segmentation of UTE images was optimized with additional tissue classes and masks in areas difficult to segment, such as the nasal septa, ethmoidal-, frontal sinuses and mastoid process. Another difference is that continuous linear attenuation coefficients are used for a more accurate attenuation map (Ladefoged et al., 2015). This improved the VT values, however, yielded overestimations in all regions. Our results deviate slightly from those of Ladefoged et al. (2015), who reported underestimations in some areas. However, the same group published a larger study with errors similar to the ones found in this work (Ladefoged et al., 2017). The best performance of VT in terms of bias was found with the atlas-based AC method pseudoCT, yielding high accuracy in all regions. As a limitation for this (Burgos et al., 2016) and similar approaches (Mérida et al., 2017) it is worth to mention the necessity of a representative MRI-CT database. Of note, similar RD and AD are expected between tissue concentration and VT values since VT mathematically equals the tissue concentration divided by the radioligand concentration in plasma.
The more commonly used outcome parameter for SERT and other neurotransmitter applications is BPP. By definition, the difference between BPP and VT can only arise from the reference region (here the cerebellar gray matter). It is therefore important to note that the error of the AC methods is markedly non-uniform across the brain, particularly for those methods without or with only limited modeling of bone tissue. Regions in proximity of bone (e.g., cerebellum) show a higher bias than regions in the center of the brain (e.g., striatum). Hence, outcome parameters, which are dependent on interregional differences (e.g., BPP) or ratios (distribution volume ratio) will incorporate the non-uniform error as additional source of bias. Another issue is that SSRIs decrease the binding also in the reference region. Although the cerebellar gray matter was identified as the optimal reference region for [11C]DASB, a displacement of up to 33% was described after an acute sertralin challenge (Parsey et al., 2006; Turkheimer et al., 2012). However, this will not affect the comparison between the different AC methods as differences in the reference region will be equally present for all AC approaches. Nonetheless, this may indeed have an effect when using outcome parameters such as BPP or BPND, which require an unbiased reference region. Here, lower binding or in other words small numerical uncertainties will translate into larger errors of percentage. This is a potential explanation for the differences between the SSRI and placebo scans observed for BPP when using DIXON and UTE.
Differently to this study, binding potentials are also commonly estimated with kinetic modeling. Inhomogeneous tracer uptake over time might induce uncertainties in ROIs which can influence the shape of time activity curves and therefore the outcome of the kinetic modeling (Mérida et al., 2017). However, Mansur et al. compared the AC approach pseudoCT to AC CT with the reference kinetic model SRTM as well as an arterial blood-based two tissue compartment model (2TCM) (Mansur et al., 2018). They reported a strong correlation of non-displaceable BP and VT and mostly similar differences (<5%) as (Mérida et al., 2017), who used comparable AC approaches. As similar bias to the ratio method with AC pseudoCT was found here, it might be concluded that the other AC methods of this study yield comparable differences as BPP when kinetic modeling is applied.
Given its clinical importance, SERT occupancy induced by i.v. SSRI challenge was also assessed for all AC methods. Of note, the error decreased in almost all areas compared to VT and BPP thus, indicating that the inaccuracy cancels out, except for errors in the ACC with AC DIXON and BD. For high binding regions of [11C]DASB, such as putamen, thalamus and caudate, RD did not exceed errors of −4% with reasonable SD. This is probably based on the small test–retest variability between the placebo and SSRI scan in terms of AC reproducibility since RD and AD are almost identical in both measurements. Therefore, our results might be translatable to longitudinal or occupancy studies, were two measurements are brought into context, independent of the tracer of choice.
We could demonstrate that the bias is not only dependent on the AC method but also on the investigated parameter, which is a complementary discovery to (Ladefoged et al., 2017). Interestingly, a high r2 was obtained for VT which may enable the rescaling of biased parameters to the true values achieved with AC CT on an ROI-basis. This would enable the usage of all presented AC methods, depending on the scientific question, e.g., investigation of VT in subcortical regions with [11C]DASB. However, issues might occur with other parameters such as BPP or occupancy with AC DIXON or BD. The lower r2 of the occupancy caused by fluctuating errors between the SSRI and placebo scans of BPP limit the applicability of both AC methods to ROIs in the brain center and parameters independent from other regions (e.g., VT). Furthermore, we would like to mention that the scalability does not apply to voxel level with the same accuracy (Ladefoged et al., 2017). It also has to be mentioned that a cohort of young adults was investigated (mean age 28.0 ± 9.6 years). Different results might be achieved in elderly or diseased subjects due to varying characteristics that cannot be reflected by the presented AC approaches (e.g., differences in skull density or cortical thickness). Another limitation observed in AC DIXON is the inversion of fat and water images, which leads to incorrect segmentation classes (Ladefoged et al., 2014). The tissue inversion occurs either partially or measurement specific and not subject specific, which is especially an issue in longitudinal studies. It is further an issue for AC BD as it is based on AC DIXON. Hence, the error propagates to AC BD. The impact of tissue inversion on BPP and occupancy was not evaluated due to a lack of data. Another limitation was the necessity for large ROIs in low binding regions which are mostly cortical structures for [11C]DASB. This was essential to calculate reliable BPP as these regions have almost as little binding as the reference region (i.e., cerebellum). Additionally, since the AC induced bias is non-uniform, large ROIs, spreading from near the center of the brain to the proximity of bone tissue, might average out some regional effects. Hence, dissimilar results might be achieved in cross-sectional studies for the parameters VT and BPP with tracers with a different distribution.
Conclusion
In this study, we presented the accuracy of different AC approaches for different binding parameters quantified with [11C]DASB. We could show the impact of AC on parameters relying on the accurate quantification of non-displaceable binding (such as BPP) and a decreased error if two measurements are brought into context (occupancy), for almost all investigated regions. The AC method pseudoCT performed best compared to AC CT since all examined regions and parameters showed acceptable variations within a range of 4%. Based on these results, AC pseudoCT should be considered as a reliable alternative to AC CT.
Data Availability Statement
The datasets generated for this study are available from the corresponding author upon request.
Ethics Statement
The study was approved by the Ethics Committee of the Medical University of Vienna and procedures were carried out in accordance with the Declaration of Helsinki. After a detailed explanation of the study all subjects gave written informed consent.
Author Contributions
LR, GG, MK, AH, WW, MM, and RL designed the study. GG recruited subjects and medically assisted the measurements. NB-I and WW synthesized the radioligand. LR, GJ, and MK acquired the data. HS and MM analyzed the blood samples. LR, IR, and AH performed data analysis. MHB and SK were medical supervisors. RL was the principal investigator and scientific supervisor of the study. All authors contributed in writing and reviewing the manuscript.
Funding
This scientific project was performed with the support of the Medical Imaging Cluster of the Medical University of Vienna, a grant from the Else Kröner-Fresenius-Stiftung (2014_A192), and support from Siemens (CA-ID: C00214938/06). This work was supported by the Austrian Science Fund (FWF) grant number KLI 516 to RL. LR, GG, and MK are/were recipients of DOC Fellowships of the Austrian Academy of Sciences at the Department of Psychiatry and Psychotherapy, Medical University of Vienna.
Conflict of Interest
Siemens Healthineers AG provided the Bone Demonstrator software as well as dedicated reconstruction software through a research agreement with the Medical University of Vienna.
The authors declare that the research was conducted in the absence of any commercial or financial relationships that could be construed as a potential conflict of interest.
Acknowledgments
We thank Verena Pichler and Chrysoula Vraka for radioligand synthesis, Karolin Einenkel and Benjamin Spurny for organizational support, Alexander Kautzky, Godber Mathis Godbersen, Arkadiusz Komorowski, and Thomas Vanicek for medical support, and Claes Ladefoged for providing the AC method RESOLUTE. We thank the MR-PET Team of Siemens Healthineers AG, Germany.
Footnotes
References
Aasheim, L. B., Karlberg, A., Goa, P. E., Håberg, A., Sørhaug, S., Fagerli, U. M., et al. (2015). PET/MR brain imaging: evaluation of clinical UTE-based attenuation correction. Eur. J. Nucl. Med. Mol. Imaging 42, 1439–1446. doi: 10.1007/s00259-015-3060-3
Andersen, F. L., Ladefoged, C. N., Beyer, T., Keller, S. H., Hansen, A. E., Højgaard, L., et al. (2014). Combined PET/MR imaging in neurology: MR-based attenuation correction implies a strong spatial bias when ignoring bone. Neuroimage 84, 206–216. doi: 10.1016/j.neuroimage.2013.08.042
Bailey, D. L. (1998). Transmission scanning in emission tomography. Eur. J. Nucl. Med. 25, 774–787. doi: 10.1007/s002590050282
Berker, Y., Franke, J., Salomon, A., Palmowski, M., Donker, H. C. W., Temur, Y., et al. (2012). MRI-Based attenuation correction for hybrid PET/MRI systems: a 4-class tissue segmentation technique using a combined ultrashort-echo-time/dixon MRI sequence. J. Nucl. Med. 53, 796–804. doi: 10.2967/jnumed.111.092577
Berker, Y., and Li, Y. (2016). Attenuation correction in emission tomography using the emission data - A review. Med. Phys. 43, 807–832. doi: 10.1118/1.4938264
Burgos, N., Cardoso, M. J., Thielemans, K., Modat, M., Pedemonte, S., Dickson, J., et al. (2014). Attenuation correction synthesis for hybrid PET-MR scanners: application to brain studies. IEEE Trans. Med. Imaging 33, 2332–2341. doi: 10.1109/TMI.2014.2340135
Burgos, N., Thielemans, K., Cardoso, M. J., Markiewicz, P., Jiao, J., Dickson, J., et al. (2016). “Effect of scatter correction when comparing attenuation maps: application to brain PET/MR,” in Proceedings of the 2014 IEEE Nuclear Science Symposium and Medical Imaging Conference, NSS/MIC 2014, (Seattle, WA: IEEE), doi: 10.1109/NSSMIC.2014.7430775
Carney, J. P. J., Townsend, D. W., Rappoport, V., and Bendriem, B. (2006). Method for transforming CT images for attenuation correction in PET/CT imaging. Med. Phys. 33, 976–983. doi: 10.1118/1.2174132
Catana, C., Guimaraes, A. R., and Rosen, B. R. (2013). PET and MR imaging: the odd couple or a match made in heaven? J. Nucl. Med. 54, 815–824. doi: 10.2967/jnumed.112.112771
Catana, C., van der Kouwe, A., Benner, T., Michel, C. J., Hamm, M., Fenchel, M., et al. (2010). Toward implementing an MRI-based PET attenuation-correction method for neurologic studies on the MR-PET brain prototype. J. Nucl. Med. 51, 1431–1438. doi: 10.2967/jnumed.109.069112
Delso, G., Wiesinger, F., Sacolick, L. I., Kaushik, S. S., Shanbhag, D. D., Hullner, M., et al. (2015). Clinical evaluation of zero-echo-time MR imaging for the segmentation of the skull. J. Nucl. Med. 56, 417–422. doi: 10.2967/jnumed.114.149997
Dixon, W. T. (1984). Simple proton spectroscopic imaging. Radiology 153, 189–194. doi: 10.1148/radiology.153.1.6089263
Ginovart, N., Wilson, A. A., Meyer, J. H., Hussey, D., and Houle, S. (2001). Positron emission tomography quantification of [(11)C]-DASB binding to the human serotonin transporter: modeling strategies. J. Cereb. Blood Flow Metab. 21, 1342–1353. doi: 10.1097/00004647-200111000-00010
Gong, K., Yang, J., Kim, K., El Fakhri, G., Seo, Y., and Li, Q. (2018). Attenuation correction for brain PET imaging using deep neural network based on Dixon and ZTE MR images. Phys. Med. Biol. 63:125011. doi: 10.1088/1361-6560/aac763
Gryglewski, G., Rischka, L., Philippe, C., Hahn, A., James, G. M., Klebermass, E., et al. (2017). Simple and rapid quantification of serotonin transporter binding using [11C]DASB bolus plus constant infusion. Neuroimage 149, 23–32. doi: 10.1016/j.neuroimage.2017.01.050
Haeusler, D., Mien, L. K., Nics, L., Ungersboeck, J., Philippe, C., Lanzenberger, R. R., et al. (2009). Simple and rapid preparation of [11C]DASB with high quality and reliability for routine applications. Appl. Radiat. Isot. 67, 1654–1660. doi: 10.1016/j.apradiso.2009.03.005
Hahn, A., Gryglewski, G., Nics, L., Rischka, L., Ganger, S., Sigurdardottir, H., et al. (2017). Task-relevant brain networks identified with simultaneous PET/MR imaging of metabolism and connectivity. Brain Struct. Funct. 223, 1–10. doi: 10.1007/s00429-017-1558-0
Huang, S. C., Hoffman, E. J., Phelps, M. E., and Kuhl, D. E. (1979). Quantitation in positron emission computed tomography: 2. Effects of inaccurate attenuation correction. J. Comput. Assist. Tomogr. 3, 804–814.
Innis, R. B., Cunningham, V. J., Delforge, J., Fujita, M., Gjedde, A., Gunn, R. N., et al. (2007). Consensus nomenclature for in vivo imaging of reversibly binding radioligands. J. Cereb. Blood Flow Metab. 27, 1533–1539. doi: 10.1038/sj.jcbfm.9600493
Izquierdo-Garcia, D., Hansen, A. E., Forster, S., Benoit, D., Schachoff, S., Furst, S., et al. (2014). An SPM8-based approach for attenuation correction combining segmentation and nonrigid template formation: application to simultaneous PET/MR brain imaging. J. Nucl. Med. 55, 1825–1830. doi: 10.2967/jnumed.113.136341
Kinahan, P. E., Townsend, D. W., Beyer, T., and Sashin, D. (1998). Attenuation correction for a combined 3D PET/CT scanner. Med. Phys. 25, 2046–2053. doi: 10.1118/1.598392
Koesters, T., Friedman, K. P., Fenchel, M., Zhan, Y., Hermosillo, G., Babb, J., et al. (2016). Dixon sequence with superimposed model-based bone compartment provides highly accurate PET/MR attenuation correction of the brain. J. Nucl. Med. 57, 918–924. doi: 10.2967/jnumed.115.166967
Ladefoged, C. N., Benoit, D., Law, I., Holm, S., Kjaer, A., Hojgaard, L., et al. (2015). Region specific optimization of continuous linear attenuation coefficients based on UTE (RESOLUTE): application to PET/MR brain imaging. Phys. Med. Biol. 60, 8047–8065. doi: 10.1088/0031-9155/60/20/8047
Ladefoged, C. N., Hansen, A. E., Keller, S. H., Holm, S., Law, I., Beyer, T., et al. (2014). Impact of incorrect tissue classification in Dixon-based MR-AC: fat-water tissue inversion. EJNMMI Phys. 1:101. doi: 10.1186/s40658-014-0101-0
Ladefoged, C. N., Law, I., Anazodo, U., St. Lawrence, K., Izquierdo-Garcia, D., Catana, C., et al. (2017). A multi-centre evaluation of eleven clinically feasible brain PET/MRI attenuation correction techniques using a large cohort of patients. Neuroimage 147, 346–359. doi: 10.1016/j.neuroimage.2016.12.010
Mansur, A., Newbould, R., Searle, G. E., Redstone, C., Gunn, R. N., and Hallett, W. A. (2018). PET-MR attenuation correction in dynamic brain PET using [11 C]cimbi-36: a direct comparison with PET-CT. IEEE Trans. Radiat. Plasma Med. Sci. 2, 483–489. doi: 10.1109/TRPMS.2018.2852558
Martinez-Möller, A., Souvatzoglou, M., Delso, G., Bundschuh, R. A., Chefd’hotel, C., Ziegler, S. I., et al. (2009). Tissue classification as a potential approach for attenuation correction in whole-body PET/MRI: evaluation with PET/CT data. J. Nucl. Med. 50, 520–526. doi: 10.2967/jnumed.108.054726
Mérida, I., Reilhac, A., Redouté, J., Heckemann, R. A., Costes, N., and Hammers, A. (2017). Multi-atlas attenuation correction supports full quantification of static and dynamic brain PET data in PET-MR. Phys. Med. Biol. 62, 2834–2858. doi: 10.1088/1361-6560/aa5f6c
Nørgaard, M., Ganz, M., Svarer, C., Feng, L., Ichise, M., Lanzenberger, R., et al. (2019). Cerebral serotonin transporter measurements with [11C]DASB: a review on acquisition and preprocessing across 21 PET centres. J. Cereb. Blood Flow Metab. 39, 210–222. doi: 10.1177/0271678X18770107
Parsey, R. V., Kent, J. M., Oquendo, M. A., Richards, M. C., Pratap, M., Cooper, T. B., et al. (2006). Acute occupancy of brain serotonin transporter by sertraline as measured by [11C]DASB and positron emission tomography. Biol. Psychiatry 59, 821–828. doi: 10.1016/j.biopsych.2005.08.010
Paulus, D. H., Quick, H. H., Geppert, C., Fenchel, M., Zhan, Y., Hermosillo, G., et al. (2015). Whole-Body PET/MR imaging: quantitative evaluation of a novel model-based MR attenuation correction method including bone. J. Nucl. Med. 56, 1061–1066. doi: 10.2967/jnumed.115.156000
Rausch, I., Rischka, L., Ladefoged, C. N., Furtner, J., Fenchel, M., Hahn, A., et al. (2017). PET/MRI for oncological brain imaging: a comparison of standard MR-based attenuation corrections with a novel, model-based approach for the Siemens mMR PET/MR system. J. Nucl. Med. 58, 1519–1525. doi: 10.2967/jnumed.116.186148
Rausch, I., Rust, P., Difranco, M. D., Lassen, M., Stadlbauer, A., Mayerhoefer, M. E., et al. (2016). Reproducibility of MRI Dixon-based attenuation correction in combined PET/MR with applications for lean body mass estimation. J. Nucl. Med. 57, 1096–1102. doi: 10.2967/jnumed.115.168294
Sander, C. Y., Hooker, J. M., Catana, C., Normandin, M. D., Alpert, N. M., Knudsen, G. M., et al. (2013). Neurovascular coupling to D2/D3 dopamine receptor occupancy using simultaneous PET/functional MRI. Proc. Natl. Acad. Sci. U.S.A. 110, 11169–11174. doi: 10.1073/pnas.1220512110
Schulz, V., Torres-Espallardo, I., Renisch, S., Hu, Z., Ojha, N., Börnert, P., et al. (2011). Automatic, three-segment, MR-based attenuation correction for whole-body PET/MR data. Eur. J. Nucl. Med. Mol. Imaging 38, 138–152. doi: 10.1007/s00259-010-1603-1
Sekine, T., Burgos, N., Warnock, G., Huellner, M., Buck, A., ter Voert, E. E. G. W., et al. (2016). Multi-atlas-based attenuation correction for brain 18F-FDG PET imaging using a time-of-flight PET/MR scanner: comparison with clinical single-atlas- and CT-based attenuation correction. J. Nucl. Med. 57, 1258–1264. doi: 10.2967/jnumed.115.169045
Takano, A., Varrone, A., Gulyás, B., Salvadori, P., Gee, A., Windhorst, A., et al. (2016). Guidelines to PET measurements of the target occupancy in the brain for drug development. Eur. J. Nucl. Med. Mol. Imaging. 43, 2255–2262. doi: 10.1007/s00259-016-3476-4
Townsend, D. W., Carney, J. P. J., Yap, J. T., and Hall, N. C. (2004). PET/CT today and tomorrow. J. Nucl. Med. 45(Suppl. 1), 4S–14S. doi: 10.1111/j.1476-5829.2010.00218.x
Turkheimer, F. E., Selvaraj, S., Hinz, R., Murthy, V., Bhagwagar, Z., Grasby, P., et al. (2012). Quantification of ligand PET studies using a reference region with a displaceable fraction: application to occupancy studies with 11C-DASB as an example. J. Cereb. Blood Flow Metab. 32, 70–80. doi: 10.1038/jcbfm.2011.108
Tzourio-Mazoyer, N., Landeau, B., Papathanassiou, D., Crivello, F., Etard, O., Delcroix, N., et al. (2002). Automated anatomical labeling of activations in SPM using a macroscopic anatomical parcellation of the MNI MRI single-subject brain. Neuroimage 15, 273–289. doi: 10.1006/nimg.2001.0978
Varrone, A., Asenbaum, S., Vander Borght, T., Booij, J., Nobili, F., Någren, K., et al. (2009). EANM procedure guidelines for PET brain imaging using [18F]FDG, version 2. Eur. J. Nucl. Med. Mol. Imaging 36, 2103–2110. doi: 10.1007/s00259-009-1264-0
Keywords: attenuation correction, PET/MRI, serotonin transporter, [11C]DASB, occupancy, absolute quantification
Citation: Rischka L, Gryglewski G, Berroterán-Infante N, Rausch I, James GM, Klöbl M, Sigurdardottir H, Hartenbach M, Hahn A, Wadsak W, Mitterhauser M, Beyer T, Kasper S, Prayer D, Hacker M and Lanzenberger R (2019) Attenuation Correction Approaches for Serotonin Transporter Quantification With PET/MRI. Front. Physiol. 10:1422. doi: 10.3389/fphys.2019.01422
Received: 03 May 2019; Accepted: 04 November 2019;
Published: 22 November 2019.
Edited by:
Claudia Kuntner, Austrian Institute of Technology (AIT), AustriaReviewed by:
Paul Cumming, University of Bern, SwitzerlandCristina Lois, Massachusetts General Hospital, Harvard Medical School, United States
Copyright © 2019 Rischka, Gryglewski, Berroterán-Infante, Rausch, James, Klöbl, Sigurdardottir, Hartenbach, Hahn, Wadsak, Mitterhauser, Beyer, Kasper, Prayer, Hacker and Lanzenberger. This is an open-access article distributed under the terms of the Creative Commons Attribution License (CC BY). The use, distribution or reproduction in other forums is permitted, provided the original author(s) and the copyright owner(s) are credited and that the original publication in this journal is cited, in accordance with accepted academic practice. No use, distribution or reproduction is permitted which does not comply with these terms.
*Correspondence: Rupert Lanzenberger, rupert.lanzenberger@meduniwien.ac.at