- 1Department of Physical Education, School of Sports, Federal University of Santa Catarina, Florianópolis, Brazil
- 2Physical Education School, Federal University of Rio Grande do Sul, Porto Alegre, Brazil
- 3Faculty Physical Education, University of Campinas, Campinas, Brazil
- 4Department of Exercise and Sport Science, University of Wisconsin-LaCrosse, LaCrosse, WI, United States
- 5Faculty of Sport Sciences and Physical Education, University of Coimbra, Coimbra, Portugal
Background: The interpretation of young athletes' performance during pubertal years is important to support coaches' decisions, as performance may be erroneously interpreted due to the misalignment between chronological age (CA), biological age (BA) and sport age (SA).
Aim: Using a Bayesian multilevel approach, the variation in longitudinal changes in performance was examined considering the influence of CA, BA (age at menarche), SA, body size, and exposure to training among female basketball players.
Method: The study had a mixed-longitudinal design. Thirty eight female basketball players (aged 13.38 ± 1.25 years at baseline) were measured three times per season. CA, BA and SA were obtained. Anthropometric and functional measures: countermovement jump, Line drill (LD), Yo-Yo (Yo-Yo IR1). Based on the sum of the z-scores, an index of overall performance was estimated. The effects of training on longitudinal changes in performance were modeled.
Results: A decrease in the rate of improvements was apparent at about 14 years of age. When aligned for BA, the slowing of the rate of improvements is apparent about 2 years after menarche for LD. For countermovement jump longitudinal changes, when performance was aligned for BA improvements became linear. For Yo-Yo IR1 and performance index, both indicators showed a linear trend of improvement when aligned for CA and BA, separately. Older players showed higher rates of improvement for Yo-Yo IR1 and performance index from pre-season to end-season. When considering performance changes aligned for BA it was apparent an improvement of performance as players became biologically mature.
Conclusions and Implications: The alignment of CA with BA and SA provides important information for coaches. Human growth follows a genetically determined pattern, despite variation in both tempo and timing. When the effects of maturation reach their end, all the girls went through the same process. Hence, there is no need to artificially manipulate youth competitions in order to accelerate gains that sooner or later reach their peak and tend to flat their improvement curve.
Introduction
The interpretation of young athletes' performance development during pubertal years is of importance to support coaches' short- and long-term decisions. Particularly in the context of talent development where, despite ethical issues, early identification and selection is the modus operandi of high performance sport, talent selection or de-selection decisions have different levels of risks and consequences for young players (Baker et al., 2018).
Most notably, performance assessment may be confounded due to the misalignment between chronological age, biological age and sport age (accumulated training and competitive experience in sport). Therefore, the mechanisms that predict future successful players and those dropping out from organized sports are multifactorial and highly complex, especially in sports like basketball, where structured training systems start before or during puberty (Deprez et al., 2015; Soares et al., 2016; Gonçalves et al., 2018).
Basketball is a team sport that requires movement patterns that involve short, intense and repeated episodes of activity requiring frequent rapid changes in direction (Mcinnes et al., 1995; Ben Abdelkrim et al., 2007, 2010; Staunton et al., 2018; Stojanovic et al., 2018). Although basketball movement patterns mainly involve intermittent activities which are aerobic in nature, maximal intensity short-term activities (e.g., sprinting, jumping, cutting) are decisive for the performance in the game (Ben Abdelkrim et al., 2007; Stojanovic et al., 2018). Hence, when interpreting young basketball player's performance, coaches and/or researchers should consider both maximal short-term output and basketball related intermittent endurance. On the other hand, body size, particularly stature, is relevant for basketball performance (Drinkwater et al., 2008), and is highly valued by coaches when attempting to select and/or predict future outcomes (Pearson et al., 2006). However, young players vary substantially in growth and maturity status, as well as complex environmental factors, often complicates interpretation of performance in young athletes (Abbott et al., 2005; Pearson et al., 2006).
There is an emphasis in youth sports, including youth basketball, on talent identification, development and selection (Gonçalves et al., 2012). Achievement of athletic expertise at high level when adults is limited to a very narrow group of players.
In youth basketball, coaches' decisions regarding the career path of adolescent players are influenced by players size and functional performance level (Drinkwater et al., 2008; Carvalho et al., 2018). Although differences in adolescent players' physique and performance are transient, are likely exacerbated by the interactions between pubertal growth rate, chronological age and accumulated sport-specific experience (Carvalho et al., 2018; Gonçalves et al., 2018; Leonardi et al., 2018). Thus, the appropriate interpretation of the young basketball players' performance is crucial for both coach and athlete (Leonardi et al., 2018).
Available data considering functional capacities in young basketball players is mainly based on male players (Montgomery et al., 2008; Carvalho et al., 2011a,b,c; Sisic et al., 2016; Torres-Unda et al., 2016). Although there is an increasing number of female young athletes involved in intensive training programs and high level competitions, available knowledge concerning the functional capacities of young female basketball players remains scarce (Mcmanus and Armstrong, 2011). Interpretations of young female athletes' functional capacity may be complicated by sexual dimorphism (Mcmanus and Armstrong, 2011), given the large variation between-girls in the timing and tempo of biological maturation, as well as primary sex differences (Sherar et al., 2004). Hence, interpretations based on male athlete samples may be inadequate and examining functional capacity in young female athletes engaged in sport-specific training merits attention.
Understanding changes and development of performance during pubertal development is an increasingly studied topic (Nevill et al., 1998; Thomis et al., 2000; Beunen et al., 2002; De Ste Croix et al., 2002; Martin et al., 2004; Drinkwater et al., 2005; Bidaurrazaga-Letona et al., 2014; Carvalho et al., 2017). Amongst young athletes exposed to organized training and competition programs, researchers usually need a long time planning, extensive resources for data collection in the field, rather than the laboratory. Attrition from injuries or loss of interest complicates data analysis. Furthermore, the need to consider chronological age, biological age and “the age in the sport” (i.e., the amount of accumulated training and competition experience in the sport) represent a level complexity that may be difficult to appropriately fit and interpret using traditional statistical models (i.e., based on repeated measures analysis of variance) (Gueorguieva and Krystal, 2004; Kristensen and Hansen, 2004). Multilevel modeling provides a flexible and powerful approach to fit complex hierarchical structured data, such as repeated measures (Gelman and Hill, 2007; Goldstein, 2011). Furthermore, Bayesian methods are especially attractive in this context, as they perform well with small sample sizes (Van De Schoot et al., 2015), perform well with complex models such as multilevel modeling (McElreath, 2015), and allow incorporation of available prior information about the parameters in evaluating the data consequently improving out-of-sample predictions (Heino et al., 2018).
Considering a Bayesian multilevel approach, we examined the influence of chronological age, biological age (age at menarche), age in the sport, body size and composition, and exposure to training and competitive basketball season on the longitudinal changes in functional performance during the pubertal years among female basketball players.
Methods
Study Design and Participants
This study was based on a mixed-longitudinal design. A total of 38 adolescent female basketball players aged, on average, 13.38 (1.25) years at baseline, were measured three times per season between August 2015 and December 2017. Within the season, measurements were performed pre- (March), mid- (August) and end-season (December). The period of observation comprised two full competitive seasons (from March 2016 to December 2017), and a half season (August 2015 to December 2015). A total of 177 observations were considered for analysis, during the observation period as follows: August 2015, n = 10; December 2015, n = 9; March 2016, n = 31; August 2016, n = 37; December 2016, n = 35; March 2017, n = 24; August 2017, n = 17; December 2017, n = 14). The players considered in the present study had at least three measurements across the period of observation. The distribution of measurements per players was as follows: three measurements, n = 6; four measurements, n = 10; five measurements, n = 13; six measurements, n = 9). All The players were engaged in formal training and competition within under 13 (n = 23) and under 15 (n = 15) teams from two clubs from the Campinas metropolitan region of Brazil, and competed at regional level competition supervised by the Associação Regional de Basquetebol (ARB). During the study, all players trained regularly (~300–360 min/wk) over a 9-month season (March to November). The typical week was composed by three training sessions, with a duration of 120 min per session. In general, sessions were composed by a warm-up section (~30 min), an individual technical development section (~30 min), tactical development section (~30 min, mostly small sided games), and game session (~30 min). No player was suffering from injury at the time of testing or during 6 months before testing.
The study was approved by the Research Ethics Committee of the University of Campinas. Participants were informed about the nature of the study, that participation was voluntary and that they could withdraw from the study at any time. Players and their parents/legal guardians provided written informed consent.
Measures
Chronological age was calculated to the nearest 0.1 year by subtracting birth date from date of testing. Years of training in formal basketball were attained by interview. Age at menarche was obtained from an individual interview by the coaches of the players (female coaches in all cases). To align age at menarche with chronological age (i.e., distance to menarche) we subtracted chronological age by age at menarche. Negative values indicate time before age at menarche and positive values indicate time after age at menarche.
Anthropometric measurements were performed by a single experienced observer. Stature was measured with a portable stadiometer (Seca model 206, Hanover, MD, USA) to the nearest 0.1 cm. Body mass was measured with a calibrated portable balance (Seca model 770, Hanover, MD, USA) to the nearest 0.1 kg. The triceps, subscapular, suprailiac and medial calf skinfolds were measured and summed as a measure of relative body fat distribution. Skinfold sites were measured with a Lange skinfold caliper (Cambridge Scientific Industries, Inc., Cambridge, MD). Reliability estimates for the observer are published elsewhere (Carvalho et al., 2011a,b).
Three measures of functional capacity for basketball were considered: vertical jump with countermovement (Bosco et al., 1983), a short-term maximal running protocol, the Line drill (LD) test (Semenick, 1990; Carvalho et al., 2011a) and an intermittent endurance test, the Yo-Yo Intermittent Recovery level 1 test (Yo-Yo IR1) (Bangsbo, 1994). Based on the sum of the z-scores, we estimated an index of overall performance, i.e., functional performance index (lower-limb explosive strength, agility and anaerobic power, and intermittent endurance). Note that z-scores were reversed for the LD performance; as lower times indicate better performance.
Tests were performed in two sessions separated by at least 48 h, where the first session included the vertical jump and LD test, and the second session the Yo-Yo IR1. Before testing a standardized warm-up was taken by all athletes.
The countermovement jump test was tested on a jump mat (Multisprint System, Hidrofit, Brazil). Participants started from an upright standing position. Players were instructed to begin the jump with a downward movement, which was immediately followed by a concentric upward movement, resulting in a maximal vertical jump. During jumping, hands were held on the hips during all phases of the jumping. Three trials were allowed and the best retained for analysis. The coefficient of variation, based on replicate measures separated by 1 week in 18 players, was 6.9% (95% CI 5.1–10.5).
In the LD protocol (Semenick, 1990; Carvalho et al., 2011a), players ran 140 m as fast as possible in the form of four consecutive shuttle sprints of 5.8, 14.0, 22.2, and 28.0 m within a regulation basketball court. Players began the test one meter behind the baseline of the basketball court, where a pair of photoelectric cells (Multisprint System, Hidrofit, Brazil) was aligned with the baseline. Verbal encouragement for an all-out effort was given throughout the test. Time was recorded in seconds. Reliability estimates were reported previously (Carvalho et al., 2011a).
The Yo-Yo IR1 was performed by all players (Bangsbo, 1994). The protocol is based on repeated 2 x 20-m runs back and forth between the starting, turning, and finishing line at a progressively increased speed controlled by audio bleeps from a tape recorder (Bangsbo, 1994). The athletes have a 10-s active rest period between each bout, jogging in a distance of 2 × 5-m. Players ran until they were no longer able to maintain the required speed; the test was completed when athletes failed twice to reach the finishing line in time. Covered distance was measured in meters. Based on replicate measures on a subsample of 11 players measured twice within 1 week, the coefficient of variation was 6.0% (95% CI 4.5–9.5%), which is within the range of reproducibility reported for the Yo-Yo IR1 (Bangsbo et al., 2008).
Statistical Analysis
Modeling Functional Performance Aligned by Chronological Age or Age at Menarche
The first modeling step was to use a basic two-level polynomial growth model curve (Goldstein, 1986) to model functional performance indicators against chronological age and age at menarche, i.e., distance to menarche in years, separately. The model describes each player's successive measurements over time defining the player's change at each measurement point and its variation (level-1), differences in trajectories between players and its variation (level-2). To capture the possibility of non-linear longitudinal changes during pubertal years we considered time (chronological age or distance to menarche) coefficients up to the quadratic terms, at least. When modeling functional capacity indicators against chronological age we centered each player's value at the sample grand mean (13.94 years). This allows for the model to provide predicted values with meaningful information within the range of observations, in particular the intercept term. We allowed for between-participants variation at group-level (level-2) across the intervals of observations.
Since both time indicators were centered, we used weakly informative prior distributions for population-level, normal priors (0.50), and for group-level effects, half-cauchy priors (0.2). This conveniently allows for easier achievement of model convergence, as well as ensuring that results reflect the knowledge available from the current data.
Modeling the Influence of Body Size and Training Experience on Functional Performance Longitudinal Changes
In this step of the analysis we explored whether body size (stature, body mass and adiposity represented by the sum of four skinfolds) and years formal training experience influenced longitudinal changes in functional performance. For computational convenience and for interpretation when variables have different scales (McElreath, 2015) we used z-score transformation on both dependent variables (functional performance indicators) and independent variables (i.e., the candidate explanatory variables training experience, stature, body mass and adiposity). We added the explanatory variables to each of the basic two-level polynomial growth model modeling functional performance indicators against chronological age and distance to menarche. In the models for this step we also used weakly informative prior distributions for population-level, normal priors (0.10), and for group-level effects, half-cauchy priors (0.2).
Modeling the Effects of Exposure to 9-Months Competitive Season on Longitudinal Changes in Functional Performance
Based on the results of the previous analytical step, we explored the effects of training exposure on longitudinal changes in functional performance with indicators aligned for years of formal training in basketball, controlling for chronological age and distance to menarche. Since measurements were made and pre-, mid- and end-season across two and a half years, we first examined the pattern of change within the 9-month competitive season. A linear trend of change was observed, thus we included a dummy variable (pre-season coded as 0; mid-season coded as 1; end-season coded as 2) to identify each moment of observation in the models. We then included the dummy variable (i.e., season) in each of the initial models predicting functional performance indicators against chronological age and distance to menarche in years. We also considered an interaction term of the time variable with the dummy variable (e.g., distance to menarche interaction with season). The inclusion of the dummy variable for season and the interaction terms allow us to examine whether there are differences in players' functional performance indicators rates of change within the 9-month season, as well as changes within the 9-month season change with chronological age, distance to menarche or years of training experience (interaction term between time of measurement and season measurement).
Similar to the precedent models, we used weakly informative prior distributions for population-level, normal priors (0.10), and for group-level effects, half-cauchy priors (0.2), allowing model convergence, as well as ensuring that results reflect the knowledge available on the current data.
Model Checking and Computation
We used posterior predictive checks to confirm that we did not omit relevant interactions (Gelman et al., 2013; Vehtari et al., 2016). We used the widely applicable information criteria (WAIC) to compare models and to ensure we had not overfit our data (Gelman et al., 2013; McElreath, 2015; Vehtari et al., 2016).
For each model we run two chains for 2,000 iterations with a warm-up length of 1,000 iterations. The models were implemented with Bayesian methods via Markov Chain Monte Carlo (MCMC) simulation and using Hamiltonian Monte Carlo and its extension, the No-U-Turn Sampler using Stan (Stan Development Team, 2018), via “brms” package (Bürkner, 2017), available as a package in the R statistical language (R Core Team, 2015).
Results
The average age at menarche for the present sample of adolescent female basketball players was 11.82 (1.25) years. Five players attained menarche during the study. The posterior predictions and 90% credible intervals for indicators of functional performance aligned by chronological age and distance to age at menarche of young Brazilian female basketball players are summarized in Table 1 and Figure 1. Corresponding Bayesian multilevel models from where posterior samples were derived are summarized in Supplementary Tables 1, 2. A non-linear trend was observed for both countermovement jump and Line drill performances when aligning performance by chronological age. A decrease in the rate of improvements in both jump and Line drill changes was apparent at about 14 years of age. When aligned for distance to menarche, the slowing of the rate of improvement was apparent about 2 years after menarche for Line drill performance. As for jump performance longitudinal changes, when performance was aligned for age at menarche improvements became linear. For Yo-Yo IR1 and functional performance index, both indicators showed a linear trend of improvement in performance when aligned for chronological age and distance to menarche. For all functional performance indicators except Yo-Yo IR1, variation between players in longitudinal changes was substantial when aligned for chronological age (see Supplementary Table 1). However, between-player variation was not apparent when functional performance was aligned for age at menarche (see Supplementary Table 2).
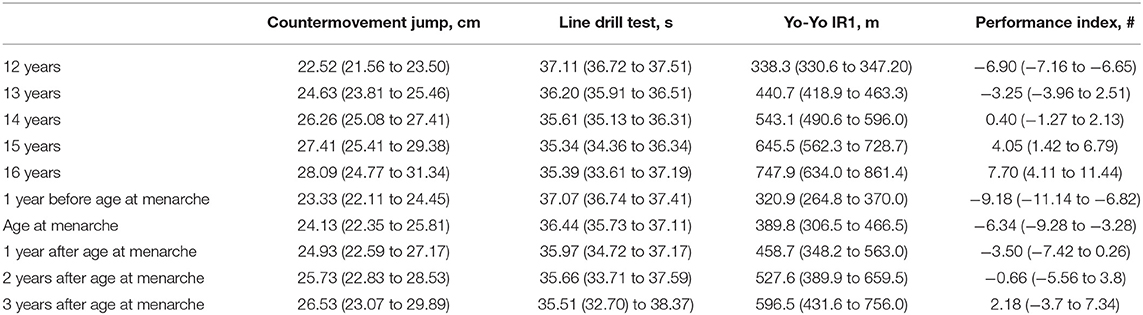
Table 1. Posterior predictions and 90% credible intervals for longitudinal changes of functional performance aligned both by chronological age and age at menarche.
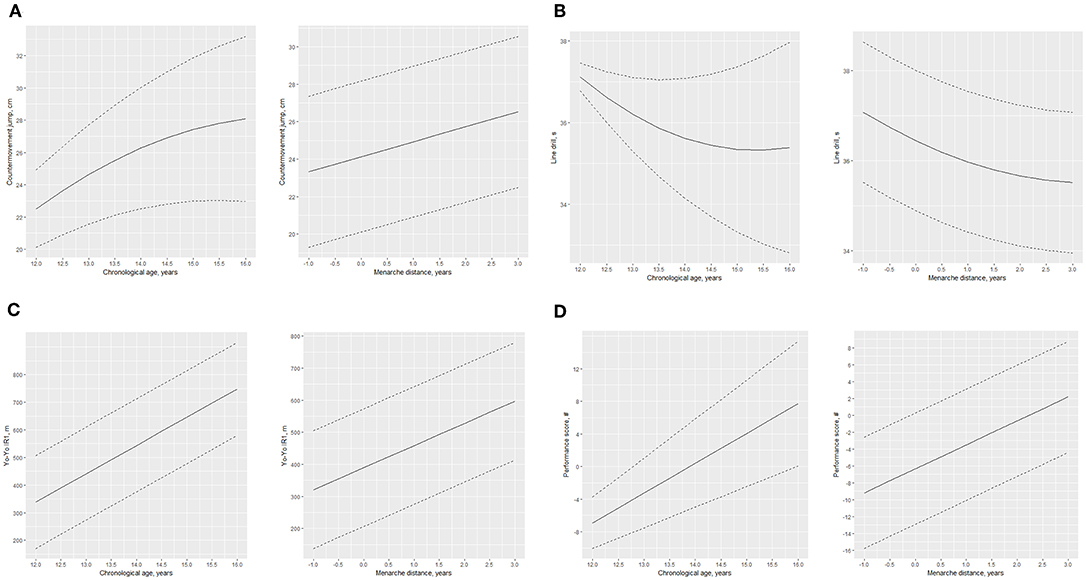
Figure 1. Countermovement jump (A), Line drill test (B), Yo-Yo IR1 (C), and performance index (D) of young female basketball players by chronological age and by menarcheal status.
The relative contributions of formal experience of training, stature, body mass and adiposity on the longitudinal changes in functional performance indicators aligned for chronological age are summarized in Table 2, and aligned for age at menarche in Table 3. Adiposity had a negative influence on players' functional performance. Between-player differences in body mass did not influence longitudinal changes in functional performance, whether performance was modeled against chronological age or age at menarche.
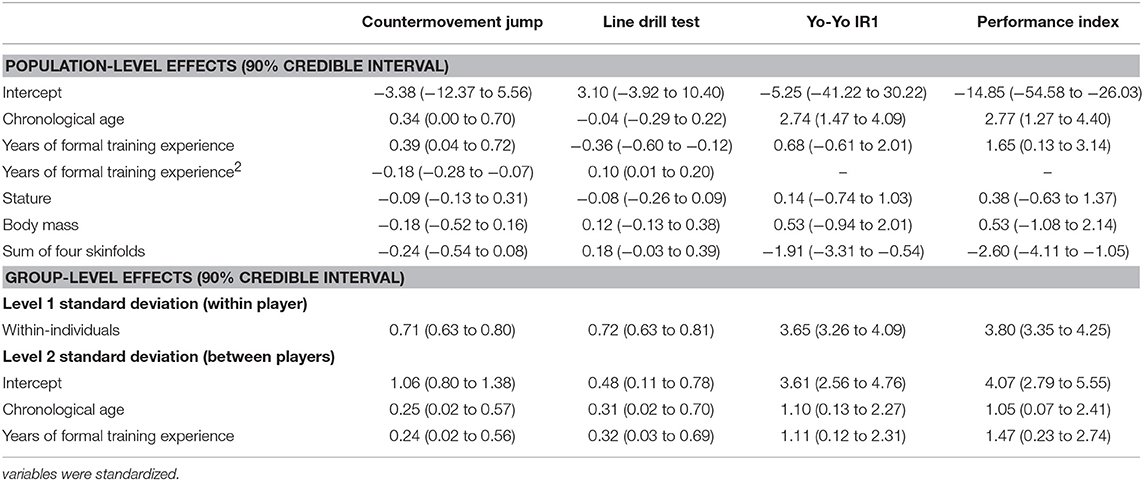
Table 2. Relative contributions of years of formal experience of basketball training, body size and adiposity on longitudinal changes in functional performance aligned by chronological age.
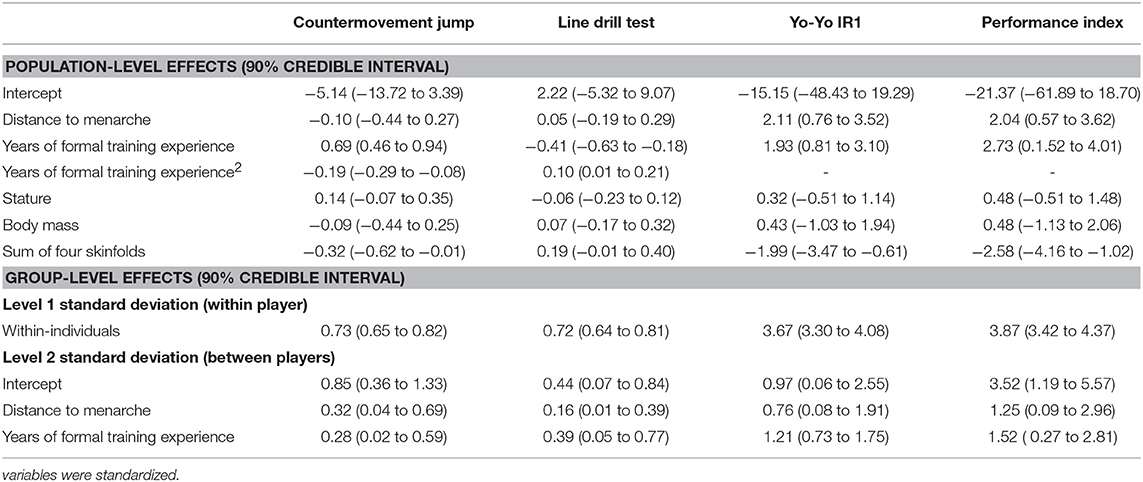
Table 3. Relative contributions of years of formal experience of basketball training, body size and adiposity on longitudinal changes in functional performance aligned by age at menarche.
The results for the model exploring the effects of exposure to 9-months competitive season on longitudinal changes in functional performance are summarized in Table 4, when aligning for chronological age, and Table 5, when aligning for age at menarche.
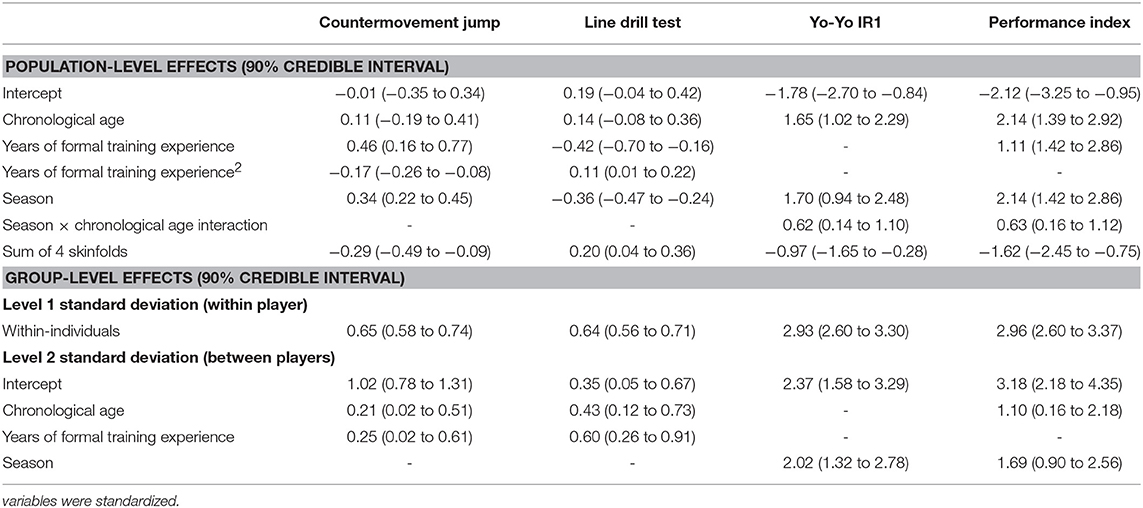
Table 4. Posterior estimates for longitudinal changes in functional performance aligned by chronological age and partitioning the influence of exposure to 10-month competitive seasons.
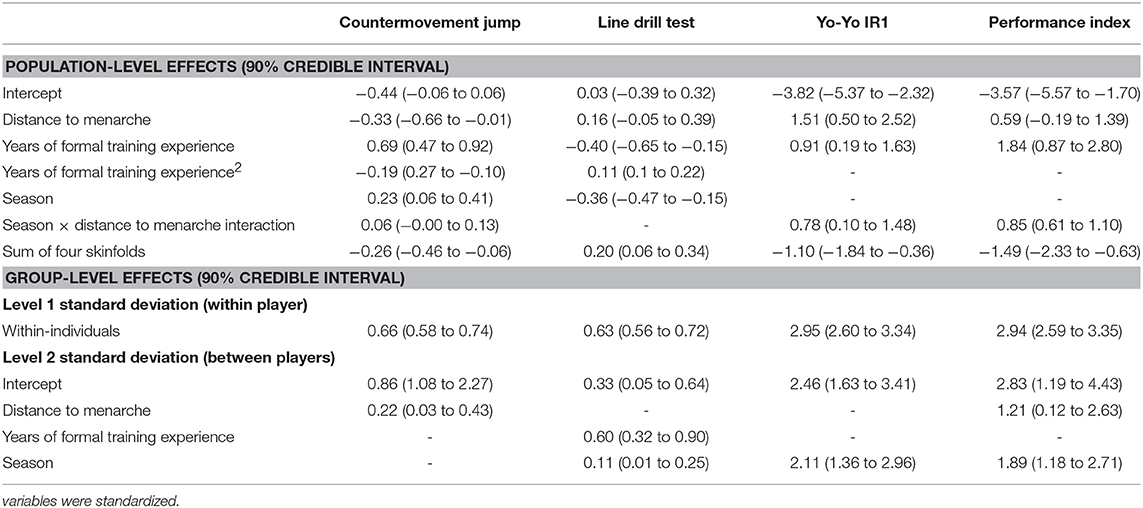
Table 5. Posterior estimates for longitudinal changes in functional performance aligned by menarche age and partitioning the influence of exposure to 10-month competitive seasons.
Considering performance changes aligned for chronological age and accounting for between players' differences in training experience and adiposity, it was observed a trend for substantial improvements of all performance indicators across the 9-months season (i.e., between pre-, mid- and end-season). Also, it was apparent that older players showed higher rates of improvement for Yo-Yo IR1 and functional performance index from pre-season to end-season. Also, when considering performance changes aligned for age at menarche (Table 5), there was an apparent substantial improvement of performance across the season. However, for countermovement jump, Yo-Yo IR1 and functional performance index from pre-season to end season improvements were substantially higher as players became biologically mature (greater distance to menarche).
Discussion
The present study modeled longitudinal changes in functional performance considering the influence of chronological age, biological age (age at menarche), age in the sport, body size and body composition, and exposure to training and competitive basketball season over the pubertal years in female basketball players. There is a body of literature that addresses the growth curves and functional performance development of pubertal girls (Nevill et al., 1998; Yagüe and De La Fuente, 1998; Armstrong et al., 2000; Thomis et al., 2000; De Ste Croix et al., 2002; Geithner et al., 2004), but mostly in non-athletic populations. A challenging question of interest with young athletes is to understand the complex interactions of growth and development with the exposure to athletic and sport-specific performance. However, to our knowledge, this is the first study that aims to align performance development with chronological age, biological age and sport experience, and to shed light on their relative effect on performance.
Chronological age and biological age are genotype variables and age in the sport is a phenotype one, meaning that the knowledge of the contribution and the interaction of each of the variables to performance in the developmental years is an important issue for researchers and practitioners. The findings in the present study showed that the genetic-determined variables tend to converge in the final stages of maturation, following a linear evolution curve, although the between-players variability does not disappear. There were substantial improvements in performance during the basketball season, and these improvements continued to occur with older players. However, performance improvements tended to slow down, leveling-off when the players approach adult maturity status. Thus, variance can be explained by sport experience, notably less evident in tests where the movement of body mass over short distances is needed, such as the LD test.
This interpretation is of relevance applied to basketball player selection. The mean age at menarche was 11.82 (1.25) years, which is earlier than worldwide observations (Eveleth and Tanner, 1991), as well as on observations based on Brazilian data (Duarte, 1993). Hence, the present sample of female basketball players was, on average, advanced in maturity status expressed by mean age at menarche. This trend is consistent with observations in adolescent male basketball players where an overrepresentation of players with advanced maturity status has been noted (Carvalho et al., 2011b, 2013, 2018; Te Wierike et al., 2015; Torres-Unda et al., 2016). Hence, youth basketball coaches likely are not be considering the transient influence of maturation when interpreting young athletes' performance.
The interpretation of the random effects allows us to determine that all the evolution paths are linear even in tests that require explosive short-term strength. The importance of body mass and adiposity on functional performance are well known (Nevill et al., 2004), particularly in young populations (Barker and Armstrong, 2011). However, there was no substantial influence of body size on longitudinal changes in performance when aligning for chronological age or age at menarche in the present sample of female adolescent basketball players. Only adiposity had a negative influence on performance, which is consistent with longitudinal observations in non-athletic girls (Welsman and Armstrong, 2000; Armstrong et al., 2001). On the other hand, it should be expected that relative gains of body fat around 25 to 30% will occur by the end of puberty in the average adolescent girl (Matthews et al., 2006; Sherar et al., 2007; Mcmanus and Armstrong, 2011). Although young athletes tend to be leaner than non-athletic girl (Mcmanus and Armstrong, 2011), it appears that coaches should still need to consider pubertal body composition changes when interpreting female players performance development.
There was substantial variability between players across the 9-month competitive season exposure, however paths of performance development remained consistent across puberty. Differences between players at the beginning of the competitive seasons remained at the end of the season, although substantial variation on rates of changes across the season highlight the need for coaches to look at the players from an athlete-centered perspective. Furthermore, differences between players' rate of change in functional performance across competitive seasons during pubertal years appeared to be positively related to chronological age and biological age.
The alignment of chronological age with biological age and accumulated years of experience in the sport provide important information for youth sport organizers and coaches. Children and adolescents' growth with age follows a pattern that is genetically determined, albeit substantial between individuals' variation in both tempo and timing of growth between individuals (Malina et al., 2004). When the effects of maturation reach their end, about 15–16 years in average girls, all the players in the present sample, despite their variability, went through the same process. Hence, there is no need to artificially manipulate youth competitions in order to accelerate gains that sooner or later reach their peak and tend to flatten their improvement curve. At the same time, coaches need to be aware of the alignment of their interventions in preparation, providing the athlete with the training stimuli that match their readiness and knowing that there is no point in trying to force or accelerate those stimuli because the gains tend to slow down with the advance in chronological and biological age. These observations are of particular relevance given the recent calls promoting biobanding as a new paradigm for youth sports and training (Cumming et al., 2017; Rogol et al., 2018). The present results suggest the need to be cautious when interpreting the young athletes' performance, the need to consider athletes development over time, and to avoid decisions (competition groups, exclusion or promotion of athletes) based on snapshots using maturity status estimations that, at best, have limited validity (Malina et al., 2012; Malina and Koziel, 2014;Koziel and Malina, 2018).
We acknowledge that several limitations in the present study. The sample size is small and there was attrition between measurements. This may in part reflect the particular characteristics of context of the study (i.e., youth female basketball in Brazil), warranting caution when generalizing interpretations. Also, attrition is an important limitation in longitudinal studies during growth and training (Kemper, 2008). On the other hand, we only considered the follow-up of body dimensions and functional capacities in this study given the available time and context of assessment. Future studies may consider tracking also behavioral and in-game performance, but these pose considerable challenges when studying young players' development during pubertal growth. Nevertheless, the present data add valuable insights for the study of young female basketball players' physical and functional development. Moreover, Bayesian multilevel modeling was adopted to deal with the analytical challenges posed in a design with repeated observations within players over time, with different levels and sources of variation (within- and between-players). In contrast with traditional statistical approaches used in sports science, Bayesian multilevel modeling is a flexible and powerful approach to interpret young athletes' performance.
In summary, this study provides a description and interpretation about the development of functional performance across adolescence in female basketball players, accounting for the influence of growth, maturation and training on competitive basketball performance. It shows the need to account for chronological, biological and training experience, i.e., age in sport, and partition their influence on body size. Human growth follows a genetically determined pattern, despite substantial variation in both tempo and timing during puberty. When the effects of maturation reach their end, all the girls went through the same process. Hence, coaches, sport scientists, and others involved in the selection and development of youth basketball players should consider that there is no need to artificially manipulate youth competitions in order to accelerate gains that sooner or later reach their peak and tend to flat their improvement curve.
Ethics Statement
This study was approved and carried out in accordance with the recommendations of the local Institutional Ethics Review Board. All participants were informed about the nature of the study, that participation was voluntary and that they could withdraw from the study at any time. Players and their parents/legal guardians provided written informed consent in accordance with the Declaration of Helsinki.
Author Contributions
HC and CG were involved in the conceptualization of the study, data analysis, and the writing of the manuscript. RP and CF were involved in the conceptualization of the study and the writing of the manuscript. TL and AS were involved in the data assessment, and the writing of the manuscript. All authors contributed approved the final version of the manuscript.
Funding
TL was supported by a grant from the Coordenação de Aperfeiçoamento de Pessoal de Nível Superior. CG was supported by a grant from the Coordenação de Aperfeiçoamento de Pessoal de Nível Superior [PNPD/CAPES/2013]. The study was partially supported by São Paulo Research Foundation (FAPESP, process 2018/06402-7).
Conflict of Interest Statement
The authors declare that the research was conducted in the absence of any commercial or financial relationships that could be construed as a potential conflict of interest.
Acknowledgments
The authors thank the patience and cooperation of the young athletes, coaches and parents.
Supplementary Material
The Supplementary Material for this article can be found online at: https://www.frontiersin.org/articles/10.3389/fphys.2019.00339/full#supplementary-material
References
Abbott, A., Button, C., Pepping, G. J., and Collins, D. (2005). Unnatural selection: talent identification and development in sport. Nonlinear Dynamics Psychol. Life Sci. 9, 61–88.
Armstrong, N., Welsman, J. R., and Chia, M. Y. (2001). Short term power output in relation to growth and maturation. Br. J. Sports Med. 35, 118–124. doi: 10.1136/bjsm.35.2.118
Armstrong, N., Welsman, J. R., Williams, C. A., and Kirby, B. J. (2000). Longitudinal changes in young people's short-term power output. Med. Sci. Sports Exerc. 32, 1140–1145. doi: 10.1097/00005768-200006000-00017
Baker, J., Schorer, J., and Wattie, N. (2018). Compromising talent: issues in identifying and selecting talent in sport. Quest 70, 48–63. doi: 10.1080/00336297.2017.1333438
Bangsbo, J., Iaia, F. M., and Krustrup, P. (2008). The Yo-Yo intermittent recovery test: a useful tool for evaluation of physical performance in intermittent sports. Sports Med. 38, 37–51. doi: 10.2165/00007256-200838010-00004
Barker, A. R., and Armstrong, N. (2011). Exercise testing elite young athletes. Med. Sport Sci. 56, 106–125. doi: 10.1159/000320642
Ben Abdelkrim, N., Castagna, C., Jabri, I., Battikh, T., El Fazaa, S., and El Ati, J. (2010). Activity profile and physiological requirements of junior elite basketball players in relation to aerobic-anaerobic fitness. J. Strength Cond. Res. 24, 2330–2342. doi: 10.1519/JSC.0b013e3181e381c1
Ben Abdelkrim, N., El Fazaa, S., and El Ati, J. (2007). Time-motion analysis and physiological data of elite under-19-year-old basketball players during competition. Br. J. Sports Med. 41, 69–75; discussion 75. doi: 10.1136/bjsm.2006.032318
Beunen, G., Baxter-Jones, A. D., Mirwald, R. L., Thomis, M., Lefevre, J., Malina, R. M., et al. (2002). Intraindividual allometric development of aerobic power in 8- to 16-year-old boys. Med. Sci. Sports Exerc. 34, 503–510. doi: 10.1097/00005768-200203000-00018
Bidaurrazaga-Letona, I., Carvalho, H. M., Lekue, J. A., Santos-Concejero, J., Figueiredo, A. J., and Gil, S. M. (2014). Longitudinal field test assessment in a basque soccer youth academy: a multilevel modeling framework to partition effects of maturation. Int. J. Sports Med. 36, 234–240. doi: 10.1055/s-0034-1385881
Bosco, C., Luhtanen, P., and Komi, P. V. (1983). A simple method for measurement of mechanical power in jumping. Eur. J. Appl. Physiol. Occup. Physiol. 50, 273–282. doi: 10.1007/BF00422166
Bürkner, P.-C. (2017). brms: An R Package for Bayesian Multilevel Models using Stan. J. Stat. Softw. 80, 1–28. doi: 10.18637/jss.v080.i01
Carvalho, H. M., Goncalves, C. E., Collins, D., and Paes, R. R. (2018). Growth, functional capacities and motivation for achievement and competitiveness in youth basketball: an interdisciplinary approach. J. Sports Sci. 36, 742–748. doi: 10.1080/02640414.2017.1340654
Carvalho, H. M., Lekue, J. A., Gil, S. M., and Bidaurrazaga-Letona, I. (2017). Pubertal development of body size and soccer-specific functional capacities in adolescent players. Res. Sports Med. 25, 421–436. doi: 10.1080/15438627.2017.1365301
Carvalho, H. M., Silva, M., Eisenmann, J. C., and Malina, R. M. (2013). Aerobic fitness, maturation, and training experience in youth basketball. Int. J. Sports Physiol. Perform. 8, 428–434. doi: 10.1123/ijspp.8.4.428
Carvalho, H. M., Silva, M., Figueiredo, A. J., Gonçalves, C. E., Castagna, C., Philippaerts, R. M., et al. (2011a). Cross-validation and reliability of the line-drill test of anaerobic performance in basketball players 14-16 years. J. Strength Cond. Res. 25, 1113–1119. doi: 10.1519/JSC.0b013e3181d09e38
Carvalho, H. M., Silva, M., Figueiredo, A. J., Gonçalves, C. E., Philippaerts, R. M., Castagna, C., et al. (2011b). Predictors of maximal short-term power outputs in basketball players 14-16 years. Eur. J. Appl. Physiol. 111, 789–796. doi: 10.1007/s00421-010-1703-4
Carvalho, H. M., Silva, M., Goncalves, C. E., Philippaerts, R. M., Castagna, C., and Malina, R. M. (2011c). Age-related variation of anaerobic power after controlling for size and maturation in adolescent basketball players. Ann. Hum. Biol. 38, 721–727. doi: 10.3109/03014460.2011.613852
Cumming, S. P., Lloyd, R. S., Oliver, J. L., Eisenmann, J. C., and Malina, R. M. (2017). Bio-banding in sport: applications to competition, talent identification, and strength and conditioning of youth athletes. Strength Cond. J. 39, 34–47. doi: 10.1519/SSC.0000000000000281
De Ste Croix, M. B., Armstrong, N., Welsman, J. R., and Sharpe, P. (2002). Longitudinal changes in isokinetic leg strength in 10-14-year-olds. Ann. Hum. Biol. 29, 50–62. doi: 10.1080/03014460110057981
Deprez, D. N., Fransen, J., Lenoir, M., Philippaerts, R. M., and Vaeyens, R. (2015). A retrospective study on anthropometrical, physical fitness, and motor coordination characteristics that influence dropout, contract status, and first-team playing time in high-level soccer players aged eight to eighteen years. J. Strength Cond. Res. 29, 1692–1704. doi: 10.1519/JSC.0000000000000806
Drinkwater, E. J., Hopkins, W. G., Mckenna, M. J., Hunt, P. H., and Pyne, D. B. (2005). Characterizing changes in fitness of basketball players within and between seasons. Int. J. Perform. Anal. Sport 5, 107–125. doi: 10.1080/24748668.2005.11868342
Drinkwater, E. J., Pyne, D. B., and Mckenna, M. J. (2008). Design and interpretation of anthropometric and fitness testing of basketball players. Sports Med. 38, 565–578. doi: 10.2165/00007256-200838070-00004
Duarte, M. F. S. (1993). Physical maturation: a review with special reference to Brazilian children. Cad Saude Publica 9(Suppl. 1), 71–84. doi: 10.1590/S0102-311X1993000500008
Eveleth, P. B., and Tanner, J. M. (1991). Worldwide Variation in Human Growth. Cambridge: Cambridge University Press.
Geithner, C. A., Thomis, M. A., Vanden Eynde, B., Maes, H. H., Loos, R. J., Peeters, M., et al. (2004). Growth in peak aerobic power during adolescence. Med. Sci. Sports Exerc. 36, 1616–1624. doi: 10.1249/01.MSS.0000139807.72229.41
Gelman, A., Carlin, J. B., Stern, H. S., Dunson, D. B., Vehtari, A., and Rubin, D. B. (2013). Bayesian Data Analysis, 3rd Edn. Boca Raton, FL: Chapman & Hall/CRC Press.
Gelman, A., and Hill, J. (2007). Data Analysis Using Regression and Multilevel/Hierarchical Models. Cambridge: Cambridge University Press.
Goldstein, H. (1986). Efficient statistical modelling of longitudinal data. Ann. Hum. Biol. 13, 129–141. doi: 10.1080/03014468600008271
Gonçalves, C. E., Carvalho, H. M., and Catarino, L. (2018). “Body in movement: better measurements for better coaching,” in Perspectives on Athlete-Centred Coaching, ed S. Pill (Abingdon: Routledge).
Gonçalves, C. E., Rama, L. M., and Figueiredo, A. J. (2012). Talent identification and specialization in sport: an overview of some unanswered questions. Int. J. Sports Physiol. Perform. 7, 390–393. doi: 10.1123/ijspp.7.4.390
Gueorguieva, R., and Krystal, J. H. (2004). Move over ANOVA: progress in analyzing repeated-measures data and its reflection in papers published in the Archives of General Psychiatry. Arch. Gen. Psychiatry 61, 310–317. doi: 10.1001/archpsyc.61.3.310
Heino, M. T. J., Vuorre, M., and Hankonen, N. (2018). Bayesian evaluation of behavior change interventions: a brief introduction and a practical example. Health Psychol. Behav. Med. 6, 49–78. doi: 10.1080/21642850.2018.1428102
Kemper, H. C. G. (2008). “Longitudinal studies during growth and training: importance and principles,” in The Young Athlete, eds H. Hebestreit and O. Bar-Or (Oxford: Blackwell Publishing), 469–485.
Koziel, S. M., and Malina, R. M. (2018). Modified maturity offset prediction equations: validation in independent longitudinal samples of boys and girls. Sports Med. 48, 221–236. doi: 10.1007/s40279-017-0750-y
Kristensen, M., and Hansen, T. (2004). Statistical analyses of repeated measures in physiological research: a tutorial. Adv. Physiol. Educ. 28, 2–14. doi: 10.1152/advan.00042.2003
Leonardi, T. J., Paes, R. R., Breder, L., Foster, C., Gonçalves, C. E., and Carvalho, H. M. (2018). Biological maturation, training experience, body size and functional capacity of adolescent female basketball players: a bayesian analysis. Int. J. Sports Sci. Coach. 13, 713–722. doi: 10.1177/1747954118772489
Malina, R. M., Bouchard, C., and Bar-Or, O. (2004). Growth, Maturation, and Physical Activity. Champaign, IL: Human Kinetics.
Malina, R. M., and Koziel, S. M. (2014). Validation of maturity offset in a longitudinal sample of Polish boys. J. Sports Sci. 32, 424–437. doi: 10.1080/02640414.2013.828850
Malina, R. M., Silva, M., Figueiredo, A. J., Carling, C., and Beunen, G. P. (2012). Interrelationships among invasive and non-invasive indicators of biological maturation in adolescent male soccer players. J. Sports Sci. 30, 1705–1717. doi: 10.1080/02640414.2011.639382
Martin, R. J., Dore, E., Twisk, J., Van Praagh, E., Hautier, C. A., and Bedu, M. (2004). Longitudinal changes of maximal short-term peak power in girls and boys during growth. Med. Sci. Sports Exerc. 36, 498–503. doi: 10.1249/01.MSS.0000117162.20314.6B
Matthews, B. L., Bennell, K. L., Mckay, H. A., Khan, K. M., Baxter-Jones, A. D., Mirwald, R. L., et al. (2006). The influence of dance training on growth and maturation of young females: a mixed longitudinal study. Ann. Hum. Biol. 33, 342–356. doi: 10.1080/03014460600635951
McElreath, R. (2015). Statistical Rethinking: A Bayesian Course With Examples in R and Stan. Boca Raton, FL: Chapman & Hall/CRC Press.
Mcinnes, S. E., Carlson, J. S., Jones, C. J., and Mckenna, M. J. (1995). The physiological load imposed on basketball players during competition. J. Sports Sci. 13, 387–397. doi: 10.1080/02640419508732254
Mcmanus, A. M., and Armstrong, N. (2011). Physiology of elite young female athletes. Med. Sport Sci. 56, 23–46. doi: 10.1159/000320626
Montgomery, P. G., Pyne, D. B., Hopkins, W. G., and Minahan, C. L. (2008). Seasonal progression and variability of repeat-effort line-drill performance in elite junior basketball players. J. Sports Sci. 26, 543–550. doi: 10.1080/02640410701654298
Nevill, A. M., Holder, R. L., Baxter-Jones, A., Round, J. M., and Jones, D. A. (1998). Modeling developmental changes in strength and aerobic power in children. J. Appl. Physiol. 84, 963–970. doi: 10.1152/jappl.1998.84.3.963
Nevill, A. M., Stewart, A. D., Olds, T., and Holder, R. (2004). Are adult physiques geometrically similar? The dangers of allometric scaling using body mass power laws. Am. J. Phys. Anthropol. 124, 177–182. doi: 10.1002/ajpa.10351
Pearson, D. T., Naughton, G. A., and Torode, M. (2006). Predictability of physiological testing and the role of maturation in talent identification for adolescent team sports. J. Sci. Med. Sport 9, 277–287. doi: 10.1016/j.jsams.2006.05.020
R Core Team (2015). R: A Language and Environment for Statistical Computing. Vienna: R Foundation for Statistical Computing.
Rogol, A. D., Cumming, S. P., and Malina, R. M. (2018). Biobanding: a new paradigm for youth sports and training. Pediatrics 142:e20180423. doi: 10.1542/peds.2018-0423
Semenick, D. (1990). Tests and measurements: the line drill test. Strength Cond. J. 12, 47–49. doi: 10.1519/0744-0049(1990)012<0047:TLDT>2.3.CO;2
Sherar, L. B., Baxter-Jones, A. D., and Mirwald, R. L. (2004). Limitations to the use of secondary sex characteristics for gender comparisons. Ann. Hum. Biol. 31, 586–593. doi: 10.1080/03014460400001222
Sherar, L. B., Baxter-Jones, A. D., and Mirwald, R. L. (2007). The relationship between body composition and onset of menarche. Ann. Hum. Biol. 34, 673–677. doi: 10.1080/03014460701660502
Sisic, N., Jelicic, M., Pehar, M., Spasic, M., and Sekulic, D. (2016). Agility performance in high-level junior basketball players: the predictive value of anthropometrics and power qualities. J. Sports Med. Phys. Fitness 56, 884–893.
Soares, A. L. A. L., Leonardi, T. J., Reverdito, R. S., Gonçalves, C. E., Paes, R. R., and Carvalho, H. M. (2016). Variabilidade do desempenho no Line-Drill test em adolescentes jogadores de basquetebol. Rev. Bras Med. Esporte 22, 5. doi: 10.1590/1517-869220162206157686
Stan Development Team (2018). Stan Modeling Language Users Guide and Reference Manual, Version 2.18.0. Available online at: http://mc-stan.org
Staunton, C., Wundersitz, D., Gordon, B., Custovic, E., Stanger, J., and Kingsley, M. (2018). The effect of match schedule on accelerometry-derived exercise dose during training sessions throughout a competitive basketball season. Sports (Basel) 6:E69. doi: 10.3390/sports6030069
Stojanovic, E., Stojiljkovic, N., Scanlan, A. T., Dalbo, V. J., Berkelmans, D. M., and Milanovic, Z. (2018). The activity demands and physiological responses encountered during basketball match-play: a systematic review. Sports Med. 48, 111–135. doi: 10.1007/s40279-017-0794-z
Te Wierike, S. C., Elferink-Gemser, M. T., Tromp, E. J., Vaeyens, R., and Visscher, C. (2015). Role of maturity timing in selection procedures and in the specialisation of playing positions in youth basketball. J. Sports Sci. 33, 337–345. doi: 10.1080/02640414.2014.942684
Thomis, M., Rogers, D. M., Beunen, G. P., Woynarowska, B., and Malina, R. M. (2000). Allometric relationship between body size and peak VO2 relative to age at menarche. Ann. Hum. Biol. 27, 623–633. doi: 10.1080/03014460050178704
Torres-Unda, J., Zarrazquin, I., Gravina, L., Zubero, J., Seco, J., Gil, S. M., et al. (2016). Basketball performance is related to maturity and relative age in elite adolescent players. J. Strength Cond. Res. 30, 1325–1332. doi: 10.1519/JSC.0000000000001224
Van De Schoot, R., Broere, J. J., Perryck, K. H., Zondervan-Zwijnenburg, M., and Van Loey, N. E. (2015). Analyzing small data sets using Bayesian estimation: the case of posttraumatic stress symptoms following mechanical ventilation in burn survivors. Eur. J. Psychotraumatol. 6, 25216. doi: 10.3402/ejpt.v6.25216
Vehtari, A., Gelman, A., and Gabry, J. (2016). Practical Bayesian model evaluation using leave-one-out cross-validation and WAIC. J. Stat Comput 27:1413. doi: 10.1007/s11222-016-9696-4
Welsman, J. R., and Armstrong, N. (2000). Longitudinal changes in submaximal oxygen uptake in 11- to 13-year-olds. J. Sports Sci. 18, 183–189. doi: 10.1080/026404100365081
Keywords: youth sports, menarche, athletes, Bayesian multilevel modeling, adolescence
Citation: Carvalho HM, Leonardi TJ, Soares ALA, Paes RR, Foster C and Gonçalves CE (2019) Longitudinal Changes of Functional Capacities Among Adolescent Female Basketball Players. Front. Physiol. 10:339. doi: 10.3389/fphys.2019.00339
Received: 09 December 2018; Accepted: 13 March 2019;
Published: 04 April 2019.
Edited by:
Filipe Manuel Clemente, Polytechnic Institute of Viana do Castelo, PortugalReviewed by:
Daniele Conte, Lithuanian Sports University, LithuaniaAna Ruivo Alves, Polytechnic Institute of Beja, Portugal
Copyright © 2019 Carvalho, Leonardi, Soares, Paes, Foster and Gonçalves. This is an open-access article distributed under the terms of the Creative Commons Attribution License (CC BY). The use, distribution or reproduction in other forums is permitted, provided the original author(s) and the copyright owner(s) are credited and that the original publication in this journal is cited, in accordance with accepted academic practice. No use, distribution or reproduction is permitted which does not comply with these terms.
*Correspondence: Humberto M. Carvalho, hmoreiracarvalho@gmail.com