- 1Departamento de Investigación, Fundación Oceanogràfic de la Comunidad Valenciana, Valencia, Spain
- 2Department of Mathematics and Statistics, Texas A&M University–Corpus Christi, Corpus Christi, TX, United States
- 3Dolphin Quest, Honolulu, HI, United States
- 4Center for Marine Sciences and Technology, Department of Clinical Sciences, College of Veterinary Medicine, North Carolina State University, Morehead City, NC, United States
- 5Veterinary Cardiology, Alboraya, Spain
- 6Research Group on Biomedical Imaging (GIBI230), Instituto de Investigación Sanitaria La Fe, Valencia, Spain
Man-made environmental change may have significant impact on apex predators, like marine mammals. Thus, it is important to assess the physiological boundaries for survival in these species, and assess how climate change may affect foraging efficiency and the limits for survival. In the current study, we investigated whether the respiratory sinus arrhythmia (RSA) could estimate tidal volume (VT) in resting bottlenose dolphins (Tursiops truncatus). For this purpose, we measured respiratory flow and electrocardiogram (ECG) in five adult bottlenose dolphins at rest while breathing voluntarily. Initially, an exponential decay function, using three parameters (baseline heart rate, the change in heart rate following a breath, and an exponential decay constant) was used to describe the temporal change in instantaneous heart rate following a breath. The three descriptors, in addition to body mass, were used to develop a Generalized Additive Model (GAM) to predict the inspired tidal volume (VTinsp). The GAM allowed us to predict VTinsp with an average ( ± SD) overestimate of 3 ± 2%. A jackknife sensitivity analysis, where 4 of the five dolphins were used to fit the GAM and the 5th dolphin used to make predictions resulted in an average overestimate of 2 ± 10%. Future studies should be used to assess whether similar relationships exist in active animals, allowing VT to be studied in free-ranging animals provided that heart rate can be measured.
Introduction
Marine mammals forage underwater to obtain food and therefore divide their time at the surface to exchange gasses (O2 and CO2) with submersions to different depth and of varying durations. Therefore, a better understanding of the metabolic costs associated with underwater foraging, and proxies to assess energy use would help determine how environmental change may alter survival. By increasing the duration underwater, the opportunity to encounter and obtain food, and thereby the foraging efficiency, should be increased. Man-made environmental change such as over-fishing and global warming could cause changes in prey diversity, availability and location (Perry et al., 2005), which may have detrimental effects on apex marine predators like dolphins. Changes in prey type, abundance, and distribution could result in increases in both foraging duration and distance in order to obtain enough prey for survival. Overfishing will reduce the probability to encounter food, and movement of prey to deeper depths due to ocean warming will increase the transit time and reduce the available time at the prey patch. Longer foraging bouts, and/or deeper dives may reduce the foraging efficiency and thereby cause challenges to obtain sufficient food for survival (Perry et al., 2005). Thus, understanding the cardiorespiratory traits required by marine mammals to manage life in an extreme environment, the physiological constraints imposed on these animals, and how these limitations may affect physiology and survival are crucial.
When studying animals in the wild, measuring the metabolic cost directly is challenging, and a number of proxies have been proposed and tested. One method is to measure the resting metabolic rate (RMR) by measuring the O2 consumption rate (O2) during rest (Williams et al., 1993; Yazdi et al., 1999; Kastelein et al., 2000; Yeates and Houser, 2008; Noren et al., 2013; Rechsteiner et al., 2013; Worthy et al., 2013; van der Hoop et al., 2014; Fahlman et al., 2015), and a few studies have determined the diving and foraging metabolic rate of marine mammals during quasi-natural conditions (Kooyman et al., 1973; Sparling and Fedak, 2004; Fahlman et al., 2008, 2013). While RMR may not accurately reflect field metabolic rate (FMR), it provides an index about the minimal metabolic requirements of an individual or population against which FMR can be scaled (Bejarano et al., 2017). One method to scale FMR is to estimate FMR by validated metabolic proxies, such as heart rate (fH) (Young et al., 2011), activity (Enstipp et al., 2011; Fahlman et al., 2013), or respiratory frequency (fR) (Fahlman et al., 2016, 2017b; Folkow and Blix, 2017). Combining these methods, the metabolic costs for different populations and activities, such as resting, traveling, and foraging, can be defined. The Fick principle states that:
O2 = fR ×VT × (ΔO2), where VT is tidal volume and ΔO2 the O2 extracted from the air inhaled with each breath. By assuming that VT and ΔO2 are constant at steady state, it should be possible to estimate
O2 from fR (Folkow and Blix, 1992; Christiansen et al., 2014; Fahlman et al., 2016). While marine mammals are at the surface, fR can be assessed during focal observations. However, this is not practical during long periods at sea. In addition, studies have shown that both VT and ΔO2 change for different activities or during recovery from exercise (Fahlman et al., 2016, 2017b; Folkow and Blix, 2017), so the estimated
O2 could be improved by also estimating VT and ΔO2. Consequently, methods to assess pattern of breathing (fR, VT) would provide significant advances to estimate FMR in marine mammals.
Proxies to estimate FMR from breaths should accurately predict fR and VT during continuous recording from free ranging animals (Fahlman et al., 2016; Rojano-Doñate et al., 2018). Such data would allow an assessment of how changes in foraging effort (duration, activity, etc.) alter respiratory function, and estimated FMR. A number of studies have assessed lung function in marine mammals under human care (Olsen et al., 1969; Kerem et al., 1975; Matthews, 1977; Kooyman and Cornell, 1981; Fahlman et al., 2015, 2018a,b, 2019; Fahlman and Madigan, 2016), and at least in the bottlenose dolphin (Tursiops truncatus) these data are representative of their wild counterparts, in both shallow and deep diving ecotypes (Fahlman et al., 2018a,b). Such data are important to establish baseline lung function from animals with known health under controlled situations, and provide methods that will allow proxies to be validated that can predict respiratory effort in free ranging animals.
Estimating lung function of wild populations remains difficult. One alternative proxy could be to use the changes in fH associated with each breath, the Respiratory Sinus Arrhythmia (RSA) (de Burgh Daly, 1986). While RSA is universally present in a number of air-breathing vertebrates such as the toad, horse, dog, seal, and dolphin (Scholander, 1940; Hayano et al., 1996; Cooper et al., 2003; Noren et al., 2004; Harms et al., 2013; Zena et al., 2017; McDonald et al., 2018; Yaw et al., 2018; Piccione et al., 2019), and even in air-breathing fish (Grossman and Taylor, 2007), its physiological significance is debated (Hayano et al., 1996; Yasuma and Hayano, 2004). It has been suggested that RSA improves gas exchange by enhancing the ventilation-perfusion matching and reduces cardiac work (Yasuma and Hayano, 2004; Ben-Tal et al., 2012, 2014). The RSA causes fH acceleration during inspiration, and deceleration during expiration (Mortola et al., 2015). Thus, continuous recordings of fH could allow detection of fR, which when appropriately validated provide ways to estimate field metabolic rate (Fahlman et al., 2016; Rojano-Doñate et al., 2018). Considering recent progress in the development of biologging system that allow continuous recording of the electrocardiogram (ECG) in free-ranging cetaceans (Elmegaard et al., 2016; McDonald et al., 2018), we speculated that RSA may provide a novel method to estimate VT in bottlenose dolphins. Currently, there is limited availability of commercial data loggers that can measure continuous ECG, and custom built devices range from units with implantable electrodes used in pinnipeds or diving birds (Thompson and Fedak, 1993; Woakes et al., 1995; McDonald and Ponganis, 2014), to those that are attached externally using suction cups (Noren et al., 2004; Elmegaard et al., 2016).
In the current study, we tested the hypothesis that RSA can estimate VTinsp in resting bottlenose dolphins by recording fH and respiratory flow while resting at the surface. Our results provide evidence that using the RSA as a proxy allows us to estimate the average VTinsp of individual dolphins with an average (±SD) overestimation of 2 ± 10% with the data recorded.
Materials and Methods
Animals
The study protocols were approved by the Animal Care and Welfare Committee of the Oceanogràfic Foundation (OCE-17-16 and amendment OCE-29-18). Five adult male bottlenose dolphins (T. truncatus), housed at Dolphin Quest – Oahu (Honolulu, HI, United States), were used for all the experiments (Table 1). All experiments were conducted in January 2018. The dolphins were not restrained and could end the trial at any point. Prior to initiating the study, the dolphins were desensitized to the equipment and trained for novel research-associated behaviors using operant conditioning. Each trial consisted of the animal staying stationary in the water, allowing placement of the equipment. The animals were breathing while continuous measurements were made. Because of familiarity with these procedures, we assumed that the experimental data collected on lung function (respiratory flow) and fH were representative of a relaxed physiological state.
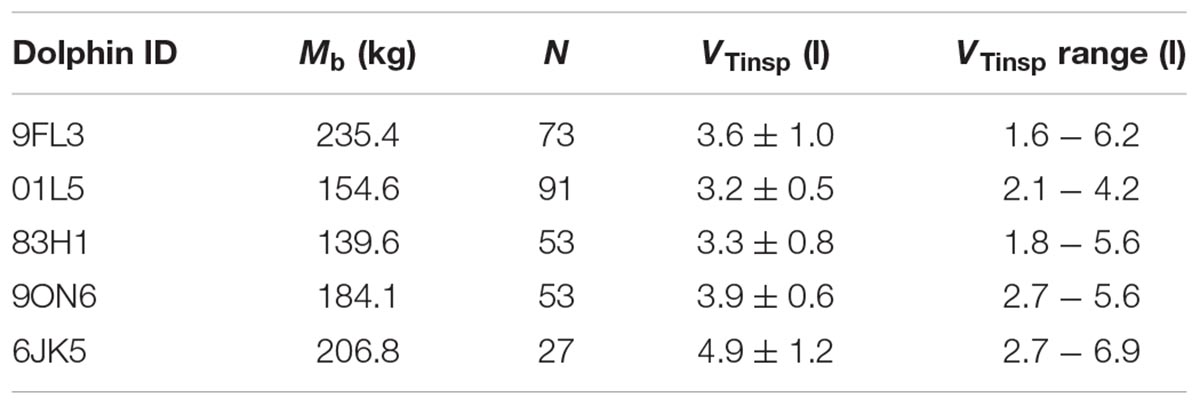
Table 1. Dolphin ID, body mass (Mb), total number of breaths analyzed (N), average ( ± SD) tidal volume (VTinsp), and VTinsp range.
Data Acquisition
A custom-made Fleisch type pneumotachometer (Mellow Design, Valencia) utilizing a low-resistance laminar flow matrix (Item # Z9A887-2, Merriam Process Technologies, Cleveland, OH, United States) was placed over the blow-hole of the dolphin (Fahlman et al., 2015). Differential pressure across the flow matrix was measured using a differential pressure transducer (ML311 Spirometer Pod, ADInstruments, Colorado Springs, CO, United States), connected to the pneumotachometer with two, 310 cm lengths of 2 mm I.D., firm walled, flexible tubing. The pneumotachometer was calibrated using a 7.0 l calibration syringe (Series 4900, Hans-Rudolph Inc., Shawnee, KS, United States). The signal was integrated and the flow determined assuming a linear response between differential pressure and flow. The linear response of the pneumotachometer was confirmed by calibrating with the 7.0 l syringe immediately before and after each trial, through a series of pump cycles at various flows. The pump cycles allowed the relationship between differential pressure and flows for the expiratory and inspiratory phases to be determined. All gas volumes were converted to standard temperature pressure dry (STPD) (Quanjer et al., 1993). Exhaled air was assumed saturated at 37°C, inhaled air volume was corrected for ambient temperature and relative humidity, and VT was calculated by integrating the flow as previously detailed (Fahlman et al., 2015).
The electrocardiogram (ECG) was recorded using three gold-plated electrodes mounted inside a silicone suction cup connected to a custom-built data recorder (UUB/1-ECGb, UFI, Morro Bay, CA, United States). The three electrodes were placed on the ventral surface: red on the right side close to the pectoral fin, yellow opposite on the left side, and green on the right side approximately 30 cm more caudally from the red. The suctions cups were filled with conducting gel (Redux Gel, Parker Laboratories) before being placed on the skin. Next, the animal rolled over to ensure the suction cups stayed in place.
The respiratory flow and ECG were recorded at 400 Hz using a data acquisition system (Powerlab 8/35, ADInstruments, Colorado Springs, CO, United States), and displayed on a computer running LabChart (v. 8.1, ADInstruments, Colorado Springs, CO, United States). Initially, the electrodes were adjusted to assure a clear ECG trace. Next, the pneumotachometer was placed over the blow-hole and the animal allowed to breathe spontaneously for up to 10 min.
We used the ECG analysis routine in LabChart to automatically detect the time between R-R peaks using the following settings; typical QRS width = 80 ms, R-waves = 300 ms, pre-P baseline = 120 ms, maximum PR = 240 ms, maximum RT = 400 ms. The detected R peaks were then manually verified and the instantaneous heart rate (ifH) determined from the time between R-R peaks.
Data Processing, Statistical Analysis and Modeling
All data were analyzed using R (version 3.4.3 – © 2017 The R Foundation for Statistical Computing) through RStudio (version 1.1.383 – © 2009–2017 RStudio, Inc.). Initially the temporal changes in ifH were described for each breath. We used a function that fit the exponential decay with time following the beginning of the inspiration for each breath:
Equation 1 was fit for each breath using the “L-BFGS-B” method of the “optim” function (Byrd et al., 1995), which optimizes parameters between imposed bounds to restrain parameters to physiologically relevant values. Breaths with fewer than seven beats after the inhalation were excluded (44 breaths).
Next the three parameters from Eq. 1 (Base Heart Rate, Decay rate, and initial change in heart rate) for each breath, and body mass (Mb) were fit against inhaled VT (VTinsp) using a loess Generalized Additive Model (GAM) (Cleveland, 1979; Hastie and Tibshirani, 1990), with the span fixed at 0.34.
To assess the sensitivity of the model, we generated five different GAMs by excluding all observations from one dolphin each time. The data from the excluded dolphin was then used to predict VTinsp. The error was computed using the formula:
where a positive value represents an overestimated prediction.
Results
Data Used for the Analysis
A total of 297 breaths were analyzed following removal of breaths with less than seven heart beats between breaths (Table 1). Only spontaneous breaths were used for the analysis, which limited the range of VT’s. In addition, as not all inspired and expired volumes are similar for each breath, we only used the VTinsp for the analysis.
The average (±SD) VTinsp was 3.8 ± 0.7 l (range: 1.6–6.9 l, see Table 1 for individual variation), and the average duration between breaths was 15.3 ± 10.7 s (range: 4–129 s). The average ifH was 74 ± 24 beats min-1 (range: 27–293 beats ⋅ min-1). The average fit parameters for Equation 1 for each dolphin are reported in Table 2.
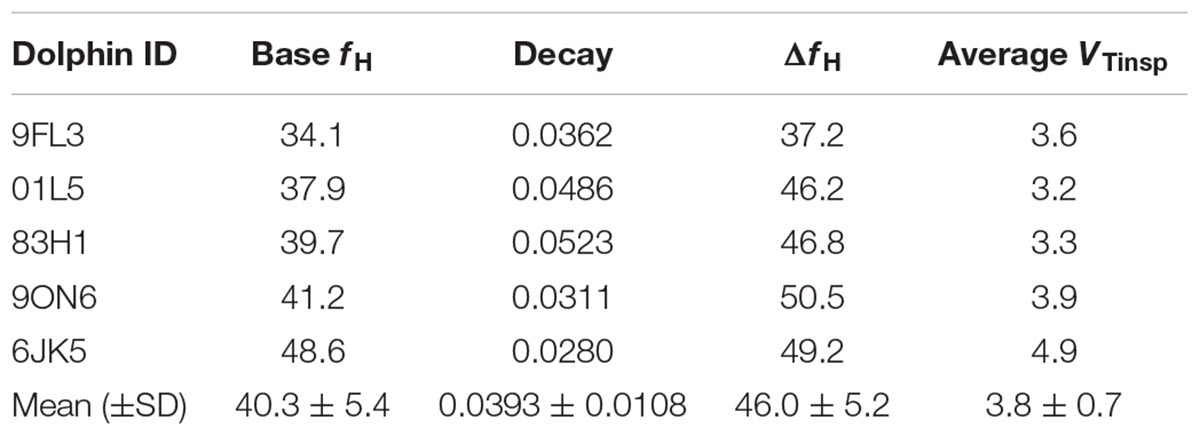
Table 2. Dolphin ID, average fit parameters for Equation 1 [base heart rate (fH), decay, initial jump (ΔfH)], and average inspired tidal volume (VTinsp).
Predicting VT From Instantaneous fH
Figure 1 shows a representative ECG trace, ifH, and respiratory flow in a dolphin over 3 breaths. The average conditions for estimating VTinsp are reported in Table 2, and the GAMs overestimated VTinsp by an average 3 ± 2% (range of individual average error: 0.3 to 7.4%, Figure 2). A sensitivity analysis was performed to assess how the prediction changed with changes in each variable (Figure 3). The decay rate and Mb had less influence on the model output as compared with base fH and the initial change in fH.
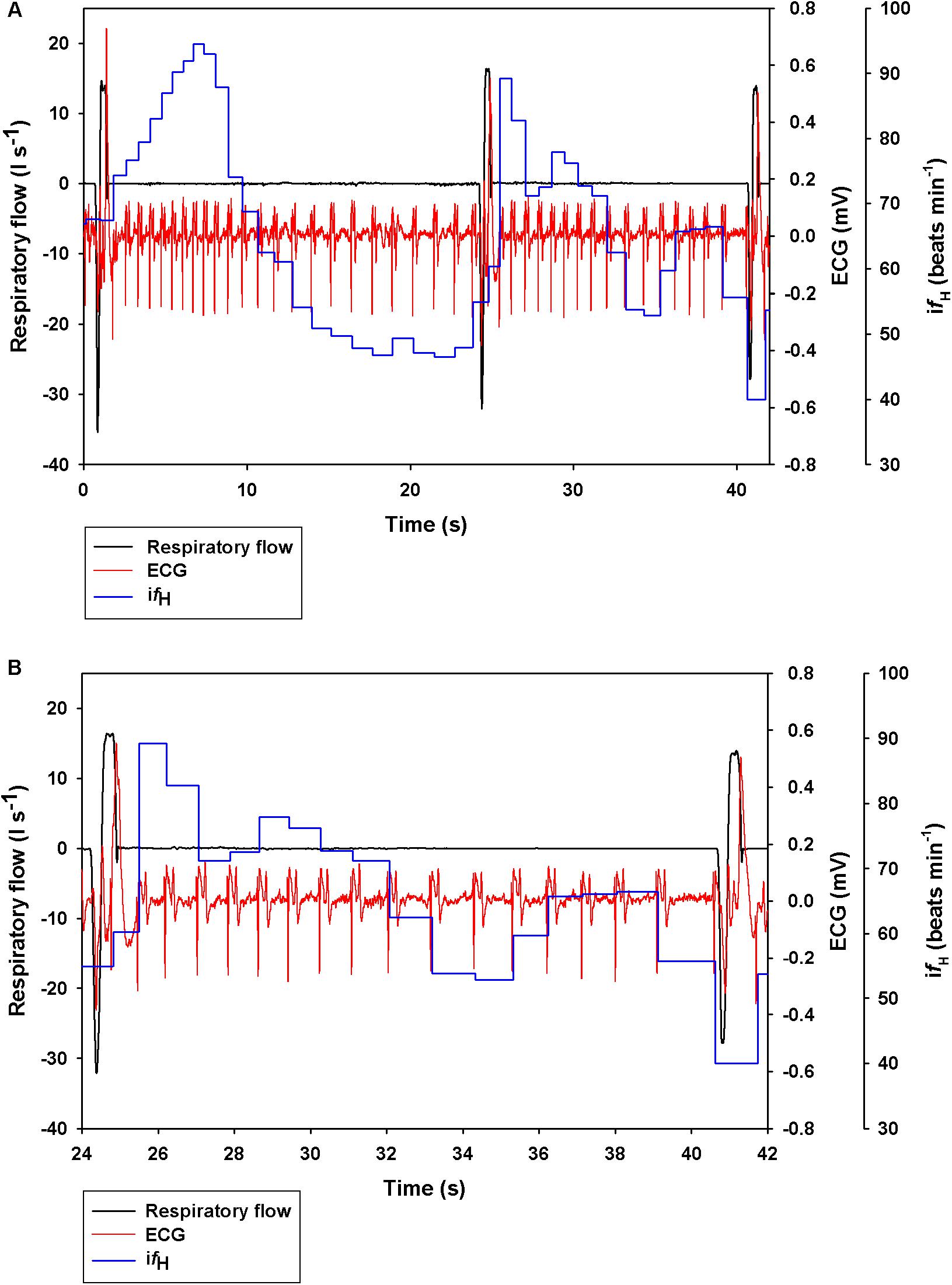
Figure 1. Representative data showing respiratory flow, ECG, and instantaneous heart rate (ifH) in a bottlenose dolphin during (A) 3 breaths, or (B) zoomed in for the 2nd breath.
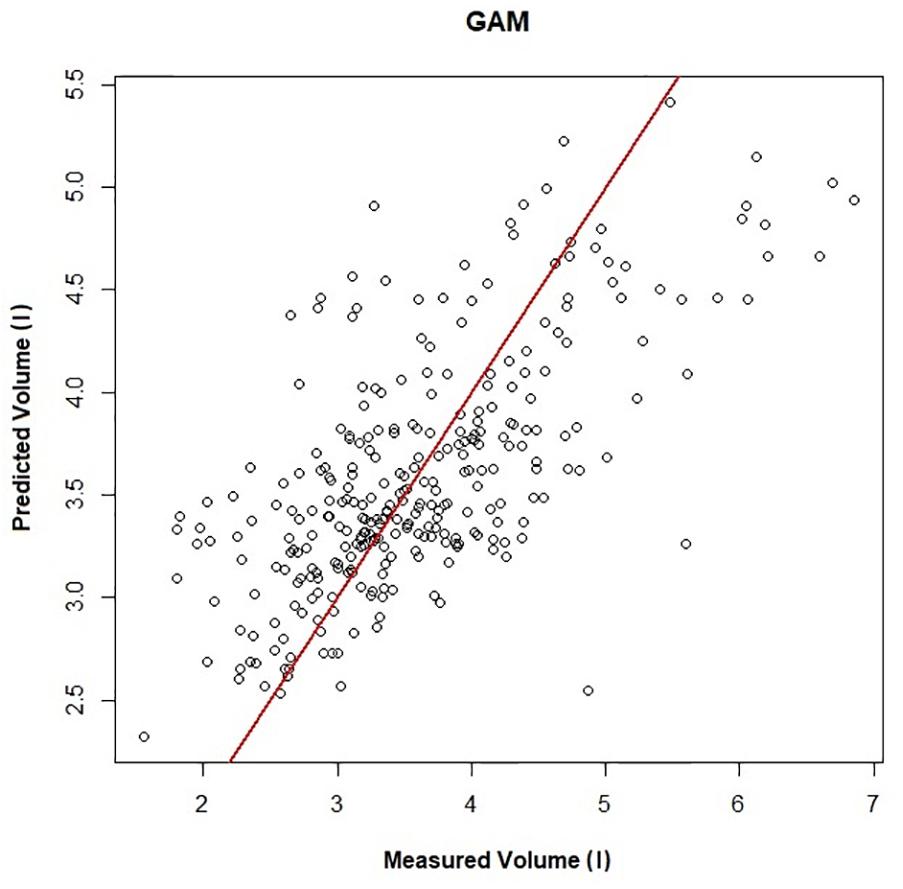
Figure 2. Predicted vs. measured inspired tidal volume (r2 = 0.45). The GAMs model is used to generate the predicted volume, and measured volume is the inspired tidal volume measured using the pneumotachometer, red line is the line of unity.
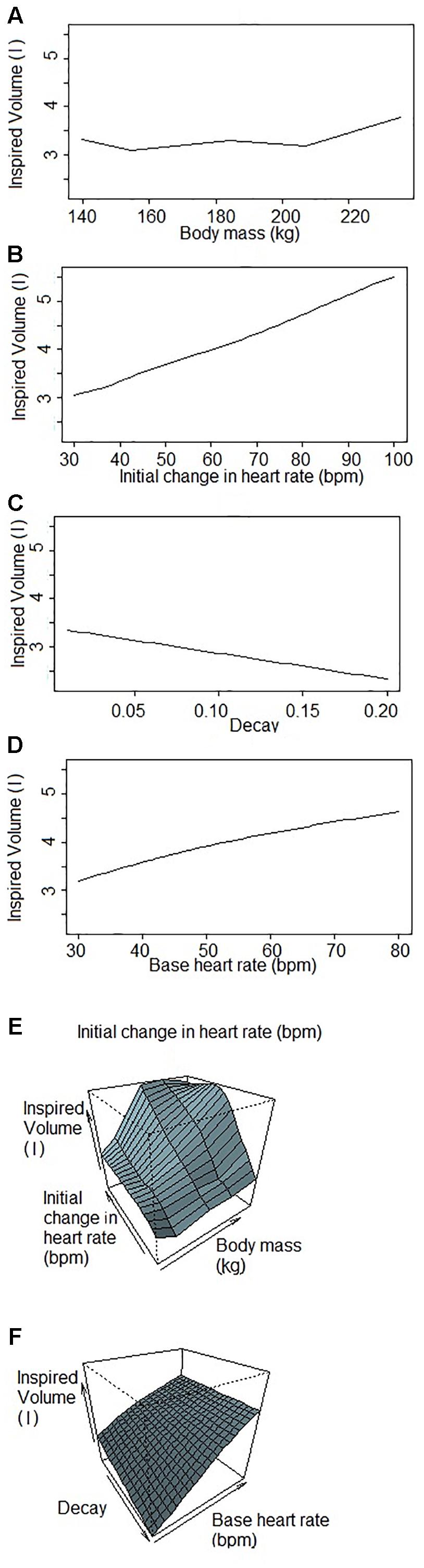
Figure 3. Sensitivity analysis of each variable used to predict inspired tidal volume by the Generalized Additive Model when one (or two) factor(s) changes while others are fixed. Inspired volumes are in liters. (A) Inspired volume as a function of body mass. (B) Inspired volume as a function of the initial change in heart rate. The initial jump is the parameter of the GAM that explains the most variation in inspired volume of the four parameters. (C) Inspired volume as a function of the decay. The decay is the parameter that explains the lowest variation in the GAM. (D) Inspired volume as a function of the base heart rate. The base heart rate is the variable that has the second most influence on the inspired volume predicted by the GAM. (E,F) Inspired volume as a function of two parameters (E) body mass and initial change in heart rate; (F) decay and base heart rate. These figures illustrate the covariance of the parameters that have consequences for the predicted inspired volume.
By removing one dolphin, fitting the GAMs with the four dolphins, and then predicting VTinsp for the 5th dolphin resulted in an average (±SD) overestimation of 2 ± 10%, (range of individual average error: -10 to 18%, Figures 4A,B). The error for individual breaths ranged from 107 to -45%, with 95% confidence limits ranging between 12 to -7% (median: 12 to -10%, Figure 4B).
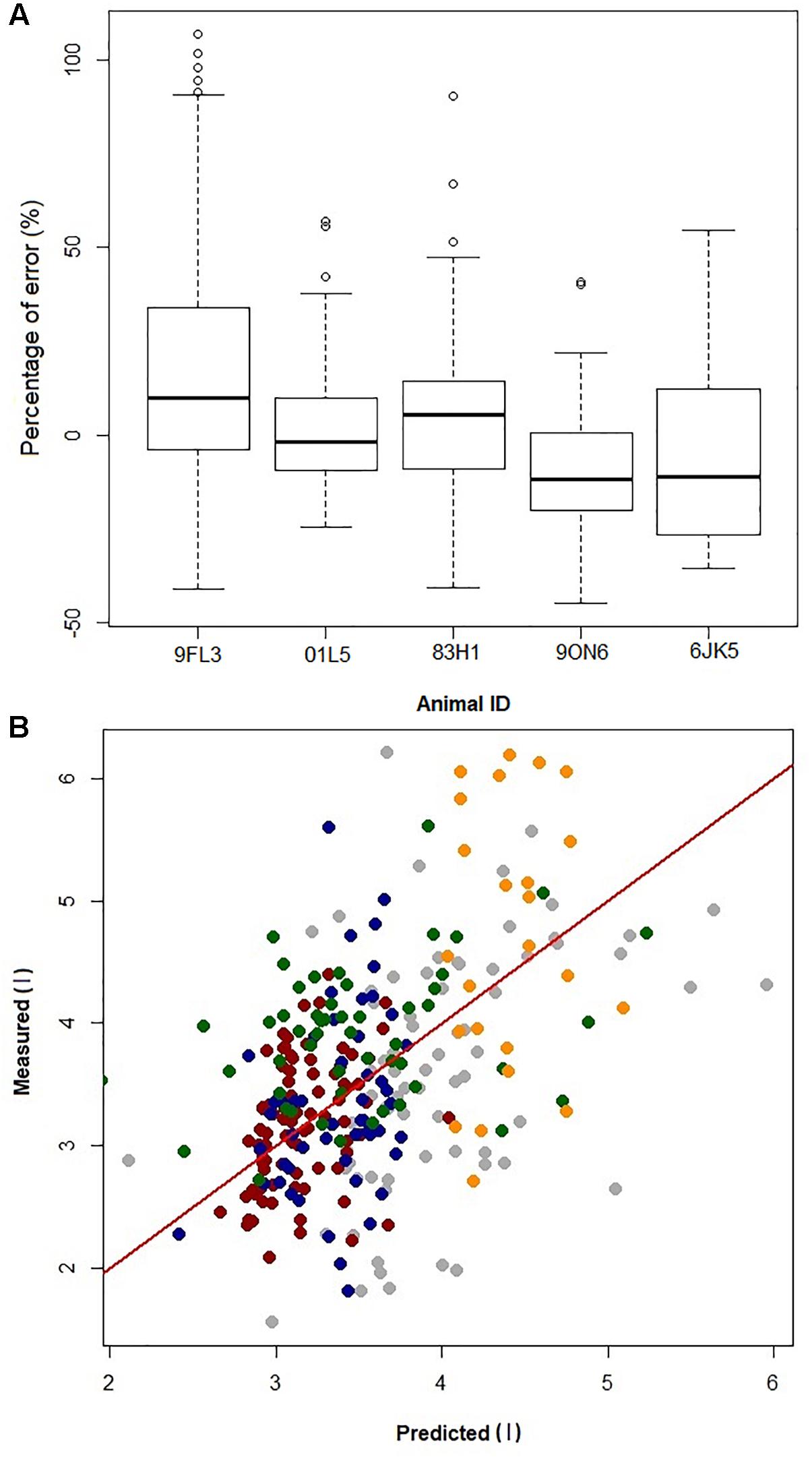
Figure 4. (A) Boxplot of prediction error [error = (predicted–measured)/ measured × 100] from jackknife sensitivity analysis, where the data from one dolphin (Animal ID) is removed to generate the GAMs and the resulting GAMs model is used to predict VTinsp for that dolphin. (B) Plot of error in prediction of a single dolphin VT when building the GAM using data from the other four dolphins. Gray = 9FL3; Red = 01L5; Blue = 83H1; Green = 9ON6; Orange = 6JK5; Red line is identity line.
Discussion
The main objective with the current study was to determine if the changes in fH associated with RSA can be used to predict the VTinsp in the bottlenose dolphin. For this purpose, we collected continuous ECG and respiratory flow in bottlenose dolphins. A jackknife method to resample the data showed that RSA, in addition to Mb, can be used to predict the average VTinsp of an individual dolphin to within 2 ± 10% of the measured value, and all individual average prediction errors were less than 20%. This shows that the GAMs should be able to predict the average VTinsp of individual dolphins using data from another population of bottlenose dolphins. If future studies can verify a similar relationship in active animals, RSA could be a useful proxy to estimate VTinsp from free-ranging marine mammals as methods to continuously measure fH are developed.
The average ifH reported in the current study was similar to those reported in previous studies in the bottlenose dolphin (ranging from 60 to 105 beats min-1) (Noren et al., 2004, 2012; Houser et al., 2010), when the fH is calculated without accounting for the RSA. However, in our past study, using trans-thoracic echocardiography to measure fH and stroke volume, it was pointed out that estimating fH without accounting for the RSA will result in average surface fH values that are confounded by the fR (Miedler et al., 2015). This is particularly problematic in marine mammals, with an fR ranging from 1 to 5 breaths ⋅ min-1 (Piscitelli et al., 2013; Fahlman et al., 2017a). Consequently, estimating resting fH without accounting for the RSA will overestimate the resting fH. As these resting fH’s have been used to assess the magnitude of the cardiovascular changes associated with diving they would erroneously overestimate the magnitude of the dive response (Fahlman et al., unpublished). The average base fH in the current study (Table 2, 40 ± 5 beats min-1) was similar to those reported in our past study in the bottlenose dolphin (fH = 41 ± 9 beats min-1) (Miedler et al., 2015), where the RSA was accounted for. Consequently, the base fH reported in the current study is a more appropriate value for the resting fH in the bottlenose dolphin. If this value is used, it provides an interesting perspective as that value is similar to the diving bradycardia reported in previous studies (Noren et al., 2012). Thus, we propose that future studies should evaluate the resting fH in voluntary diving animals after correcting for the RSA.
While the current method clearly shows that RSA is useful to estimate VTinsp, there are a number of limitations with the current method. First of all, due to the limited data set, we aimed to reduce the number of parameters used in the model. To simplify the analysis the current method did not include the duration between breaths. We analyzed each breath separately, which was both time consuming and does not account for the dependence between breaths. However, breathing and fH are continuous data. Future studies could assess time-series methods to predict VTinsp, which allows the dependence between breaths to be considered. For Equation 1, there was considerable variation in the fitted values for the base fH. When accounting for the changes in fH associated with a breath there is usually minimal variation in the base fH within one dolphin (Miedler et al., 2015). The large variation could be related to varying duration between breaths, which may alter the base fH. Thus, for breaths close together the fH may not have reached the base fH before the next breath, and may have influenced the fH variation for the next breath. Based on the current analysis, this method cannot accurately predict the VTinsp of individual breaths, but was able to provide reliable average estimated VTinsp for each animal based on the GAMs fitted to the other animals. This is similar to the method using fH to estimate field metabolic rate, where the there are limitations to estimate the metabolic cost for each dive, but where large data sets are able to estimate the energy requirements for different activities (Fahlman et al., 2004; Halsey et al., 2007; Young et al., 2011). Given the limitations with the current data, we propose that further development of this method may provide an interesting approach to study cardiorespiratory physiology in free-ranging marine mammals.
The VTinsp in the current study were of limited range (average ± SD = 3.8 ± 0.7 l, range: 1.6–6.9 l). While variation in the VTinsp during voluntary breaths is difficult to control, animals under managed care can be trained to perform maximal respiratory effort, which allows VT to vary over a much greater range (Kooyman and Cornell, 1981; Fahlman et al., 2015). For example, maximal respiratory efforts in dolphins in the weight range of the current study would increase the VT range to around 20 l (Kooyman and Cornell, 1981; Fahlman et al., 2015). It would also be useful to include females and individuals from other age classes to increase the range of variation and allow the model to be used for wild dolphin populations.
In addition, future studies should also assess whether this method is robust enough to study active or free-ranging dolphins. During natural dives fR and VT may both be highly variable and irregular, and alterations in the relationship between RSA, vagal tone, fR, and VT (de Burgh Daly, 1986; Ben-Tal et al., 2014; Guillén-Mandujano and Carrasco-Sosa, 2014; Mortola et al., 2016) may limit the accuracy of a model developed for dolphins at rest. Thus, changes in activity state, e.g., exercise, rest, travel, diving, may significantly influence autonomic tone and alter the relationship.
Finally, the GAM model does not provide an estimate of uncertainty around the predicted values with new data (data that were not in the dataset used to fit the model), nor does it provide a prediction equation for the non-parametric part of the model. To avoid these drawbacks, additional measurements with a larger range of VTinsp, fR, and activity states would help define a prediction equation that could be used in free-ranging dolphins. In addition, we propose that this method could be used for other cetaceans and marine mammal species that exhibit significant RSA. If future studies are able to verify that this method is able to estimate fR and VTinsp in actively swimming or diving dolphins this method may provide a predictive procedure for free-ranging mammals that may significantly enhance our knowledge of how marine mammals partition energy use during diving, and how the environment may limit foraging efficiency.
In summary, we show that that RSA can be used to accurately predict the average VTinsp of individual resting bottlenose dolphins with an average overestimated error of 2 ± 10%. While a number of factors appear to alter RSA (de Burgh Daly, 1986), the universal existence of RSA in vertebrates, and the suggestions that it is independent of body size (Piccione et al., 2019), could provide a method to study cardiorespiratory physiology in free ranging marine vertebrates, from marine mammals, to birds and reptiles, unraveling important mechanisms to understand the ecophysiology of these species.
Data Availability Statement
The data used in this study are freely available at the following link: osf.io/buwdp.
Author Contributions
FC performed the data and statistical analysis and drafted the first draft of the manuscript. BS-B provided statistical advice and edited the manuscript. JR-L supervised all animal training and helped with all research trials. CH helped conceive the study and helped consult with the methods. SM helped with the trials and the analysis. AF conceived the study, designed the experiments, collected the data, provided funding, helped with the data analysis, and helped to draft the manuscript. All authors helped to revise the various drafts and gave final permission to publish the study.
Funding
Funding for this project was provided by the Office of Naval Research (ONR YIP Award # N000141410563 and ONR Award # N000141613088). Dolphin Quest provided in kind support of animals, crew, and access to resources.
Conflict of Interest Statement
The authors declare that the research was conducted in the absence of any commercial or financial relationships that could be construed as a potential conflict of interest.
Acknowledgments
A special thanks to all the trainers and staff at Dolphin Quest Oahu, who made this study possible, and to Peter Madsen and Julie Van der Hoop who provided helpful advice and comments on early versions of this manuscript. We thank the reviewers who provides constructive criticism, which we believe significantly improved this article.
References
Bejarano, A. C., Wells, R. S., and Costa, D. P. (2017). Development of a bioenergetic model for estimating energy requirements and prey biomass consumption of the bottlenose dolphin Tursiops truncatus. Ecol. Model. 356, 162–172. doi: 10.1016/j.ecolmodel.2017.05.001
Ben-Tal, A., Shamailov, S. S. J., and Paton, F. R. (2012). Evaluating the physiological significance of respiratory sinus arrhythmia: looking beyond ventilation–perfusion efficiency. J. Physiol. 590, 1989–2008. doi: 10.1113/jphysiol.2011.222422
Ben-Tal, A., Shamailov, S. S. J., and Paton, F. R. (2014). Central regulation of heart rate and the appearance of respiratory sinus arrhythmia: new insights from mathematical modeling. Math. Biosci. 255, 71–82. doi: 10.1016/j.mbs.2014.06.015
Christiansen, F., Rasmussen, M. H., and Lusseau, D. (2014). Inferring energy expenditure from respiration rates in minke whales to measure the effects of whale watching boat interactions. J. Exp. Mar. Biol. Ecol. 459, 96–104. doi: 10.1016/j.jembe.2014.05.014
Cleveland, W. S. (1979). Robust locally weighted regression and smoothing scatterplots. J. Am. Stat. Assoc. 74, 829–836. doi: 10.1080/01621459.1979.10481038
Cooper, H. E., Parkes, M. J., and Clutton-Brock, T. H. (2003). CO2-dependent components of sinus arrhythmia from the start of breath holding in humans. Am. J. Physiol. Heart Circ. Physiol. 285, H841–H848. doi: 10.1152/ajpheart.01101.2002
de Burgh Daly, M. (1986). “Interactions Between Respiration and Circulation,” in Handbook of Physiology-the Respiratory System, Control of Breathing, Eds N. S. Cherniak and J. G. Widdicombe (Bethesda, MD: American Physiological Society).
Elmegaard, S. L., Johnson, M., Madsen, P. T., and McDonald, B. I. (2016). Cognitive control of heart rate in diving harbor porpoises. Curr. Biol. 26, R1167–R1176. doi: 10.1016/j.cub.2016.10.020
Enstipp, M. R., Ciccione, S., Gineste, B., Milbergue, M., Ballorain, K. Y., Ropert-Coudert, Y., et al. (2011). Energy expenditure of freely swimming adult green turtles (Chelonia mydas) and its link with body acceleration. J. Exp. Biol. 214, 4010–4020. doi: 10.1242/jeb.062943
Fahlman, A., Brodsky, M., Miedler, S., Dennison, S., Ivančić, M., Levine, G. et al. (2019). Ventilation and gas exchange before and after voluntary static surface breath-holds in clinically healthy bottlenose dolphins, Tursiops truncatus. J. Exp. Biol. (in press). doi: 10.1242/jeb.192211
Fahlman, A., Brodsky, M., Wells, R., McHugh, K., Allen, J., Barleycorn, A., et al. (2018a). Field energetics and lung function in wild bottlenose dolphins, Tursiops truncatus, in sarasota bay florida. Roy. Soc. Open. Sci. 5:171280. doi: 10.1098/rsos.171280
Fahlman, A., McHugh, K., Allen, J., Barleycorn, A., Allen, A., Sweeney, J., et al. (2018b). Resting metabolic rate and lung function in wild offshore common bottlenose dolphins, Tursiops truncatus, near bermuda. Front. Physiol. 9:886. doi: 10.3389/fphys.2018.00886
Fahlman, A., Handrich, Y., Woakes, A. J., Bost, C. A., Holder, R., Duchamp, C., et al. (2004). Effect of fasting on the VO2-fh relationship in king penguins, aptenodytes patagonicus. Am. J. Physiol. 287, R870–R877.
Fahlman, A., Loring, S. H., Levine, G., Rocho-Levine, J., Austin, T., and Brodsky, M. (2015). Lung mechanics and pulmonary function testing in cetaceans J. Exp. Biol. 218, 2030–2038. doi: 10.1242/jeb.119149
Fahlman, A., and Madigan, J. (2016). Respiratory function in voluntary participating patagonia sea lions in sternal recumbency. Front. Physiol. 7:528. doi: 10.3389/fphys.2016.00528
Fahlman, A., Moore, M. J., and Garcia-Parraga, D. (2017a). Respiratory function and mechanics in pinnipeds and cetaceans. J. Exp. Biol. 220, 1761–1763. doi: 10.1242/jeb.126870
Fahlman, A., van der Hoop, J., Moore, M. J., Levine, G., Rocho-Levine, J., and Brodsky, M. (2017b). Response to ‘On the importance of understanding physiology when estimating energetics in cetaceans’. Biol. Open 6, 307–308. doi: 10.1242/bio.023143
Fahlman, A., Svärd, C., Rosen, D. A. S., Jones, D. R., and Trites, A. W. (2008). Metabolic costs of foraging and the management of O2 and CO2 stores in steller sea lions. J. Exp. Biol. 211, 3573–3580. doi: 10.1242/jeb.023655
Fahlman, A., Svärd, C., Rosen, D. A. S., Wilson, R. S., and Trites, A. W. (2013). Activity as a proxy to estimate metabolic rate and to partition the metabolic cost of diving vs. breathing in pre- and post-fasted Steller sea lions. Aquat. Biol. 18, 175–184. doi: 10.3354/ab00500
Fahlman, A., van der Hoop, J., Moore, M., Levine, G., Rocho-Levine, J., and Brodsky, M. (2016). Estimating energetics in cetaceans from respiratory frequency: why we need to understand physiology. Biol. Open 15, 436–442. doi: 10.1242/bio.017251
Folkow, L. P., and Blix, A. S. (1992). Metabolic rates of minke whales (Balaenoptera acutorostrata) in cold water. Acta. Physiol. Scand. 146, 141–150. doi: 10.1111/j.1748-1716.1992.tb09402.x
Folkow, L. P., and Blix, A. S. (2017). On the importance of understanding physiology when estimating energetics in cetaceans. Biol. Open 6, 306–308. doi: 10.1242/bio.023929
Grossman, P., and Taylor, E. W. (2007). Toward understanding respiratory sinus arrhythmia: relations to cardiac vagal tone, evolution and biobehavioral functions. Biol. Psychol. 74, 263–285. doi: 10.1016/j.biopsycho.2005.11.014
Guillén-Mandujano, A., and Carrasco-Sosa, S. (2014). Additive effect of simultaneously varying respiratory frequency and tidal volume on respiratory sinus arrhythmia. Auton. Neurosci. 186, 69–76. doi: 10.1016/j.autneu.2014.08.003
Halsey, L. G., Fahlman, A., Handrich, Y., Schmidt, A., Woakes, A. J., and Butler, P. J. (2007). How accurately can we estimate energetic costs in a marine top predator, the king penguin? Zoology 110, 81–92.
Harms, C. A., Jensen, E. D., Townsend, F. I., Hansen, L. J., Schwacke, L. H., and Rowles, T. K. (2013). Electrocardiograms of bottlenose dolphins (Tursiops truncatus) out of water: habituated colelction versus wild postcapture animals. J. Zoo Wildlife Med. 44, 972–981. doi: 10.1638/2013-0093.1
Hayano, J., Yasuma, F., Okada, A., Mukai, S., and Fujinami, T. (1996). Respiratory sinus arrhythmia. a phenomenon improving pulmonary gas exchange and circulatory efficiency. Circulation 94, 842–847. doi: 10.1161/01.CIR.94.4.842
Houser, D. S., Dankiewicz-Talmadge, L. A., Stockard, T. K., and Ponganis, P. J. (2010). Investigation of the potential for vascular bubble formation in a repetitively diving dolphin. J. Exp. Biol. 213, 52–62. doi: 10.1242/jeb.028365
Kastelein, R. A., Mosterd, J., Schooneman, N. M., and Wiepkema, P. R. (2000). Food consumption, growth, body dimensions, and respiration rates of captive false killer whales (Pseudorca crassidens). Aquat. Mam. 26, 33–44.
Kerem, D. H., Kylstra, J. A., and Saltzman, H. A. (1975). Respiratory flow rates in the sea lion. Undersea Biomed. Res. 2, 20–27.
Kooyman, G. L., and Cornell, L. H. (1981). Flow properties of expiration and inspiration in a trained bottle-nosed porpoise. Physiol. Zool. 54, 55–61. doi: 10.1086/physzool.54.1.30155804
Kooyman, G. L., Kerem, D. H., Campbell, W. B., and Wright, J. J. (1973). Pulmonary gas exchange in freely diving weddell seals (Leptonychotes weddelli). Resp. Physiol. 17, 283–290. doi: 10.1016/0034-5687(73)90003-0
Matthews, R. C. (1977). Pulmonary Mechanics of California Sea Lions, Zalophus Californianus. San Diego: San Diego State University.
McDonald, B. I., Johnson, M., and Madsen, P. T. (2018). Dive heart rate in harbour porpoises is influenced by exercise and expectations. J. Exp. Biol. 221:jeb168740. doi: 10.1242/jeb.168740
McDonald, B. I., and Ponganis, P. J. (2014). Deep-diving sea lions exhibit extreme bradycardia in long duration dives. J. Exp. Biol. 217, 1525–1534. doi: 10.1242/jeb.098558
Miedler, S., Fahlman, A., Valls Torres, M., Álvaro Álvarez, T., and Garcia-Parraga, D. (2015). Evaluating cardiac physiology through echocardiography in bottlenose dolphins: using stroke volume and cardiac output to estimate systolic left ventricular function during rest and following exercise. J. Exp. Biol. 218, 3604–3610. doi: 10.1242/jeb.131532
Mortola, J. P., Marghescu, D., and Siegrist-Johnstone, R. (2015). Respiratory sinus arrhythmia in young men and women at different chest wall configurations. Clin. Sci. 128, 507–516. doi: 10.1042/CS20140543
Mortola, J. P., Marghescu, D., and Siegrist-Johnstone, R. (2016). Thinking about breathing: effects on respiratory sinus arrhythmia. Resp. Physiol. Neurobiol. 223, 28–36. doi: 10.1016/j.resp.2015.12.004
Noren, D. P., Holt, M. M., Dunkin, R. C., and Williams, T. M. (2013). The metabolic cost of communicative sound production in bottlenose dolphins (Tursiops truncatus). J. Exp. Biol. 216, 1624–1629. doi: 10.1242/jeb.083212
Noren, S. R., Cuccurullo, V., and Williams, T. M. (2004). The development of diving bradycardia in bottlenose dolphins (Tursiops truncatus). J. Comp. Physiol. B. 174, 139–147. doi: 10.1007/s00360-003-0398-9
Noren, S. R., Kendall, T., Cuccurullo, V., and Williams, T. M. (2012). The dive response redefined: underwater behavior influences cardiac variability in freely diving dolphins. J. Exp. Biol. 215, 2735–2741. doi: 10.1242/jeb.069583
Olsen, C. R., Hale, F. C., and Elsner, R. (1969). Mechanics of ventilation in the pilot whale. Resp. Physiol. 7, 137–149. doi: 10.1016/0034-5687(69)90001-2
Perry, A. L., Low, P. J., Ellis, J. R., and Reynolds, J. D. (2005). Climate change and distribution shifts in marine fishes. Science 308, 1912–1915. doi: 10.1126/science.1111322
Piccione, G., Giudice, E., Giannetto, C., and Mortola, J. P. (2019). The magnitude of respiratory sinus arrhythmia of a large mammal (the horse) is like that of humans. Resp. Physiol. Neurobiol. 259, 170–172. doi: 10.1016/j.resp.2018.09.006
Piscitelli, M. A., Raverty, S. A., Lillie, M. A., and Shadwick, R. E. (2013). A review of cetacean lung morphology and mechanics. J. Morphol. 274, 1425–1440. doi: 10.1002/jmor.20192
Quanjer, P. H., Tammeling, G. J., Cotes, J. E., Pedersen, O. F., Peslin, R., and Yernault, J.-C. (1993). Lung volumes and forced ventilatory flows. Euro. Resp. J. 6, 5–40. doi: 10.1183/09041950.005s1693
Rechsteiner, E. U., Rosen, D. A. S., and Trites, A. W. (2013). Energy requirements of pacific white-sided dolphins (Lagenorhynchus obliquidens) as predicted by a bioenergetic model. J. Mam. 94, 820–832. doi: 10.1371/journal.pone.0105958
Rojano-Doñate, L., McDonald, B. I., Wisniewska, D. M., Johnson, M., Teilmann, J., Wahlberg, M., et al. (2018). High field metabolic rates of wild harbour porpoises. J. Exp. Biol. 221:jeb185827. doi: 10.1242/jeb.185827
Scholander, P. F. (1940). Experimental Investigations on the Respiratory Function in Diving Mammals and Birds. Oslo: I kommisjon hos Jacob Dybwad.
Sparling, C. E., and Fedak, M. A. (2004). Metabolic rates of captive grey seals during voluntary diving. J. Exp. Biol. 207, 1615–1624. doi: 10.1242/jeb.00952
Thompson, D., and Fedak, M. A. (1993). Cardiac responses of grey seals during diving at sea. J. Exp. Biol. 174, 139–154.
van der Hoop, J. M., Fahlman, A., Hurst, T., Rocho-Levine, J., Shorter, A. K., Petrov, V., et al. (2014). Bottlenose dolphins modify behavior to reduce metabolic effect of tag attachment. J. Exp. Biol. 217, 4229–4236. doi: 10.1242/jeb.108225
Williams, T. M., Friedl, W. A., and Haun, J. E. (1993). The physiology of bottlenose dolphins (Tursiops truncatus): heart rate, metabolic rate and plasma lactate concentration during exercise. J. Exp. Biol. 179, 31–46.
Woakes, A. J., Butler, P. J., and Bevan, R. M. (1995). Implantable data logging system for heart rate and body temperature: its application to the estimation of field metabolic rates in antarctic predators. Med. Biol. Eng. Comput. 33, 145–151. doi: 10.1007/BF02523032
Worthy, G. A. J., Worthy, T. A. M., Yochem, P. K., and Dold, C. (2013). Basal metabolism of an adult male killer whale (Orcinus orca). Mar. Mam. Sci. 30, 1229–1237. doi: 10.1242/jeb.137513
Yasuma, F., and Hayano, J.-I. (2004). Respiratory sinus arrhythmia. Chest 125, 683–690. doi: 10.1378/chest.125.2.683
Yaw, T. J., Kraus, M. S., Ginsburg, A., Clayton, L. A., Hadfield, C. A., and Gelzer, A. R. (2018). Comparison of a smartphone-based electrocardiogram device with standard six-lead electrocardiogram in the Atlantic bottlenose dolphin (Tursiops truncatus). J. Zoo Wildlife Med. 49, 689–695. doi: 10.1638/2017-0140.1
Yazdi, P., Kilian, A., and Culik, B. M. (1999). Energy expenditure of swimming bottlenose dolphins (Tursiops truncatus). Mar. Biol. 134, 601–607. doi: 10.1007/s002270050575
Yeates, L. C., and Houser, D. S. (2008). Thermal tolerance in bottlenose dolphins (Tursiops truncatus). J. Exp. Biol. 211, 3249–3257. doi: 10.1242/jeb.020610
Young, B. L., Rosen, D. A., Hindle, A. G., Haulena, M., and Trites, A. W. (2011). Dive behaviour impacts the ability of heart rate to predict oxygen consumption in steller sea lions (Eumetopias jubatus) foraging at depth. J. Exp. Biol. 214, 2267–2275. doi: 10.1242/jeb.047340
Keywords: electrocardiogram, spirometry, marine mammals, diving physiology, cardiorespiratory
Citation: Cauture F, Sterba-Boatwright B, Rocho-Levine J, Harms C, Miedler S and Fahlman A (2019) Using Respiratory Sinus Arrhythmia to Estimate Inspired Tidal Volume in the Bottlenose Dolphin (Tursiops truncatus). Front. Physiol. 10:128. doi: 10.3389/fphys.2019.00128
Received: 16 October 2018; Accepted: 01 February 2019;
Published: 19 February 2019.
Edited by:
John T. Fisher, Queen’s University, CanadaReviewed by:
Nicolle Jasmin Domnik, Queen’s University, CanadaFilippo Garofalo, Università della Calabria, Italy
James Duffin, Thornhill Research Inc., Canada
Copyright © 2019 Cauture, Sterba-Boatwright, Rocho-Levine, Harms, Miedler and Fahlman. This is an open-access article distributed under the terms of the Creative Commons Attribution License (CC BY). The use, distribution or reproduction in other forums is permitted, provided the original author(s) and the copyright owner(s) are credited and that the original publication in this journal is cited, in accordance with accepted academic practice. No use, distribution or reproduction is permitted which does not comply with these terms.
*Correspondence: Fabien Cauture, Zi5jYXV0dXJlQGdtYWlsLmNvbQ== Andreas Fahlman, YWZhaGxtYW5Ad2hvaS5lZHU=